the Creative Commons Attribution 4.0 License.
the Creative Commons Attribution 4.0 License.
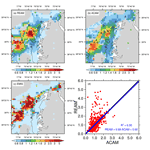
Comprehensive evaluations of diurnal NO2 measurements during DISCOVER-AQ 2011: effects of resolution-dependent representation of NOx emissions
Jianfeng Li
Ruixiong Zhang
Charles Smeltzer
Andrew Weinheimer
Jay Herman
K. Folkert Boersma
Edward A. Celarier
Russell W. Long
James J. Szykman
Ruben Delgado
Anne M. Thompson
Travis N. Knepp
Lok N. Lamsal
Scott J. Janz
Matthew G. Kowalewski
Xiong Liu
Caroline R. Nowlan
Nitrogen oxides (NOx = NO + NO2) play a crucial role in the formation of ozone and secondary inorganic and organic aerosols, thus affecting human health, global radiation budget, and climate. The diurnal and spatial variations in NO2 are functions of emissions, advection, deposition, vertical mixing, and chemistry. Their observations, therefore, provide useful constraints in our understanding of these factors. We employ a Regional chEmical and trAnsport model (REAM) to analyze the observed temporal (diurnal cycles) and spatial distributions of NO2 concentrations and tropospheric vertical column densities (TVCDs) using aircraft in situ measurements and surface EPA Air Quality System (AQS) observations as well as the measurements of TVCDs by satellite instruments (OMI: the Ozone Monitoring Instrument; GOME-2A: Global Ozone Monitoring Experiment – 2A), ground-based Pandora, and the Airborne Compact Atmospheric Mapper (ACAM) instrument in July 2011 during the DISCOVER-AQ campaign over the Baltimore–Washington region. The model simulations at 36 and 4 km resolutions are in reasonably good agreement with the regional mean temporospatial NO2 observations in the daytime. However, we find significant overestimations (underestimations) of model-simulated NO2 (O3) surface concentrations during nighttime, which can be mitigated by enhancing nocturnal vertical mixing in the model. Another discrepancy is that Pandora-measured NO2 TVCDs show much less variation in the late afternoon than simulated in the model. The higher-resolution 4 km simulations tend to show larger biases compared to the observations due largely to the larger spatial variations in NOx emissions in the model when the model spatial resolution is increased from 36 to 4 km. OMI, GOME-2A, and the high-resolution aircraft ACAM observations show a more dispersed distribution of NO2 vertical column densities (VCDs) and lower VCDs in urban regions than corresponding 36 and 4 km model simulations, likely reflecting the spatial distribution bias of NOx emissions in the National Emissions Inventory (NEI) 2011.
- Article
(15691 KB) - Full-text XML
-
Supplement
(5836 KB) - BibTeX
- EndNote
Nitrogen oxides (NOx = NO + NO2) are among the most important trace gases in the atmosphere due to their crucial role in the formation of ozone (O3) and secondary aerosols and their role in the chemical transformation of other atmospheric species, such as carbon monoxide (CO) and volatile organic compounds (VOCs) (Cheng et al., 2017, 2018; Fisher et al., 2016; Li et al., 2019; Liu et al., 2012; Ng et al., 2017; Peng et al., 2016; Zhang and Wang, 2016). NOx is emitted by both anthropogenic activities and natural sources. Anthropogenic sources account for about 77 % of the global NOx emissions, and fossil fuel combustion and industrial processes are the primary anthropogenic sources, which contribute to about 75 % of the anthropogenic emissions (Seinfeld and Pandis, 2016). Other important anthropogenic sources include agriculture and biomass and biofuel burning. Soils and lightning are two major natural sources. Most NOx is emitted as NO, which is then oxidized to NO2 by oxidants, such as O3, the hydroperoxyl radical (HO2), and organic peroxy radicals (RO2).
The diurnal variations in NO2 controlled by physical and chemical processes reflect the temporal patterns of these underlying controlling factors, such as NOx emissions, chemistry, deposition, advection, diffusion, and convection. Therefore, the observations of NO2 diurnal cycles can be used to evaluate our understanding of NOx-related emission, chemistry, and physical processes (Frey et al., 2013; Jones et al., 2000; Judd et al., 2018). For example, Brown et al. (2004) analyzed the diurnal patterns of surface NO, NO2, NO3, N2O5, HNO3, OH, and O3 concentrations along the east coast of the United States (US) during the New England Air Quality Study (NEAQS) campaign in the summer of 2002 and found that the predominant nighttime sink of NOx through the hydrolysis of N2O5 had an efficiency on par with daytime photochemical loss over the ocean surface off the New England coast. Van Stratum et al. (2012) investigated the contribution of boundary layer dynamics to chemistry evolution during the DOMINO (Diel Oxidant Mechanisms in relation to Nitrogen Oxides) campaign in 2008 in Spain and found that entrainment and boundary layer growth in daytime influenced mixed-layer NO and NO2 diurnal cycles on the same order of chemical transformations. David and Nair (2011) found that the diurnal patterns of surface NO, NO2, and O3 concentrations at a tropical coastal station in India from November 2007 to May 2009 were closely associated with sea breeze and land breeze, which affected the availability of NOx through transport. They also thought that monsoon-associated synoptic wind patterns could strongly influence the magnitudes of NO, NO2, and O3 diurnal cycles. The monsoon effect on surface NO, NO2, and O3 diurnal cycles was also observed in China by Tu et al. (2007) on the basis of continuous measurements of NO, NO2, and O3 at an urban site in Nanjing from January 2000–February 2003.
In addition to surface NO2 diurnal cycles, the daily variations in NO2 vertical column densities (VCDs) were also investigated in previous studies. For example, Boersma et al. (2008) compared NO2 tropospheric VCDs (TVCDs) retrieved from OMI (the Ozone Monitoring Instrument) and SCIAMACHY (SCanning Imaging Absorption SpectroMeter for Atmospheric CHartography) in August 2006 around the world. They found that the diurnal patterns of different types of NOx emissions could strongly affect the NO2 TVCD variations between OMI and SCIAMACHY and that intense afternoon fire activity resulted in an increase in NO2 TVCDs from 10:00 to 13:30 LT (local time) over tropical biomass burning regions. Boersma et al. (2009) further investigated the NO2 TVCD change from SCIAMACHY to OMI in different seasons of 2006 in Israeli cities and found that there was a slight increase in NO2 TVCDs from SCIAMACHY to OMI in winter due to increased NOx emissions from 10:00 to 13:30 LT and a sufficiently weak photochemical sink and that the TVCDs from OMI were lower than SCIAMACHY in summer due to a strong photochemical sink of NOx.
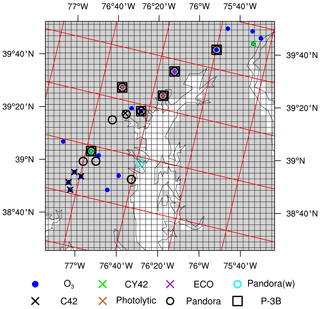
Figure 1The locations of surface and P-3B aircraft observations during the DISCOVER-AQ 2011 campaign. We mark the 36 km REAM grid cells with red lines and the 4 km REAM grid cells with black lines. Gray shading denotes land surface in the nested 4 km WRF domain, while the white area denotes ocean or water surface. Blue dots denote surface O3 observation sites. Cross marks denote surface NO2 observation sites, and their colors denote different measurement instruments: green for the Thermo Electron 42C-Y NOy analyzer, dark orchid for the Ecotech Model 9841/9843 T-NOy analyzers, black for the Thermo Model 42C NOx analyzer, and chocolate for the Teledyne API model 200eup photolytic NOx analyzer. Circles denote Pandora sites, and the cyan circle denotes a Pandora site (USNA) on a ship. Black squares denote the inland P-3B aircraft spiral locations.
All of the above research, however, exploited only NO2 surface or satellite VCD measurements. Due to the availability of ground-based NO2 VCD observations, some recent studies tried to investigate the diurnal relationships between NO2 surface concentrations and NO2 VCDs (Kollonige et al., 2018; Thompson et al., 2019). For example, Zhao et al. (2019) converted Pandora direct-sun and zenith-sky NO2 VCDs to NO2 surface concentrations using concentration-to-partial-column ratios and found that the derived concentrations captured the observed NO2 surface diurnal and seasonal variations well. Knepp et al. (2015) related the daytime variations in NO2 TVCD measurements by ground-based Pandora instruments to the variations in coincident NO2 surface concentrations using a planetary boundary layer height (PBLH) factor over the periods July 2011–October 2011 at the NASA Langley Research Center in Hampton, Virginia, and July 2011 at the Padonia and Edgewood sites in Maryland for the DISCOVER-AQ experiment, showing the importance of boundary layer vertical mixing on NO2 vertical distributions and the ability of NO2 VCD measurements to infer hourly boundary layer NO2 variations. DISCOVER-AQ, the Deriving Information on Surface conditions from Column and Vertically Resolved Observations Relevant to Air Quality experiment (https://discover-aq.larc.nasa.gov/, last access: 6 April 2019), was designed to better understand the relationship between boundary layer pollutants and satellite observations (Flynn et al., 2014; Reed et al., 2015). Figure 1 shows the sampling locations of the summer DISCOVER-AQ 2011 campaign in the Baltimore–Washington metropolitan region. In this campaign, the NASA P-3B aircraft flew spirals over six air quality monitoring sites (Aldino – rural and suburban, Edgewood – coastal and urban, Beltsville – suburban, Essex – coastal and urban, Fairhill – rural, and Padonia – suburban) (Table S1 in the Supplement) and the Chesapeake Bay (Cheng et al., 2017; Lamsal et al., 2014) and measured 245 NO2 profiles in 14 flight days in July (Zhang et al., 2016). During the same period, the NASA UC-12 aircraft flew across the Baltimore–Washington region at an altitude of about 8 km above sea level (a.s.l.), using the Airborne Compact Atmospheric Mapper (ACAM) to map the distributions of NO2 VCDs below the aircraft (Lamsal et al., 2017). Furthermore, ground-based instruments were deployed to measure NO2 surface concentrations, NO2 VCDs, and other physical properties of the atmosphere (Anderson et al., 2014; Reed et al., 2015; Sawamura et al., 2014). Satellite OMI and GOME-2A (Global Ozone Monitoring Experiment – 2A) instruments provided NO2 TVCD measurements over the campaign region at 13:30 and 09:30 LT, respectively. These concurrent measurements of NO2 VCDs, surface NO2, and vertically resolved distributions of NO2 during the DISCOVER-AQ 2011 campaign, therefore, provide a comprehensive dataset to evaluate NO2 diurnal and spatial variabilities and processes affecting NO2 concentrations.
Section 2 describes the measurement datasets in detail. The Regional chEmistry and trAnsport Model (REAM), also described in Sect. 2, is applied to simulate the NO2 observations during the DISCOVER-AQ campaign in July 2011. The evaluations of the simulated diurnal cycles of surface NO2 concentrations, NO2 vertical profiles, and NO2 TVCDs are discussed in Sect. 3 through comparisons with observations. In Sect. 3, we also investigate the differences between NO2 diurnal cycles on weekdays and weekends and their implications for NOx emission characteristics. To corroborate our evaluation of NOx emissions based on NO2 diurnal cycles, we further compare observed NOy (reactive nitrogen compounds) concentrations with REAM simulation results in Sect. 3. Moreover, we assess the resolution dependence of REAM simulation results in light of the observations and discuss the potential distribution biases of NOx emissions by comparing the 36 and 4 km REAM simulation results with OMI, GOME-2A, and high-resolution ACAM NO2 VCDs. Finally, we summarize the study in Sect. 4.
2.1 REAM
REAM has been widely applied in many studies (Cheng et al., 2017; Choi et al., 2008; Li et al., 2019; R. Zhang et al., 2018; Y. Zhang et al., 2016; Zhao et al., 2009). The model has a horizontal resolution of 36 km and 30 vertical layers in the troposphere. Meteorology fields are from a Weather Research and Forecasting (WRF; version 3.6) model simulation with a horizontal resolution of 36 km. We summarize the physics parameterization schemes of the WRF simulation in Table S2. The WRF simulation is initialized and constrained by the NCEP coupled forecast system model version 2 (CFSv2) products (http://rda.ucar.edu/datasets/ds094.0/, last access: 10 March 2015) (Saha et al., 2011). The chemistry mechanism in REAM is based on GEOS-Chem v11.01 with updated aerosol uptake of isoprene nitrates (Fisher et al., 2016) and revised treatment of wet scavenging processes (Luo et al., 2019). A 2∘ × 2.5∘ GEOS-Chem simulation provides the chemical boundary and initial conditions.
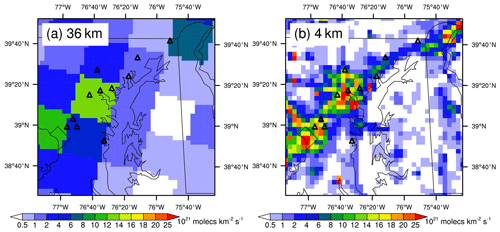
Figure 2Distributions of NOx emissions for the (a) 36 km and (b) 4 km REAM simulations around the DISCOVER-AQ 2011 region. Here NOx emissions refer to the mean values () in 1 week (Monday–Sunday).
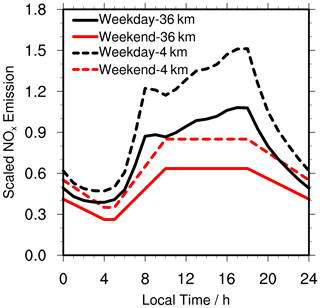
Figure 3Relative diurnal profiles of weekday and weekend NOx emissions () in the DISCOVER-AQ 2011 region (the 36 and 4 km grid cells over the 11 inland Pandora sites shown in Fig. 1) for the 36 and 4 km REAM. All the profiles are scaled by the 4 km weekday emission average value ().
Biogenic VOC emissions in REAM are from MEGAN v2.10 (Guenther et al., 2012). Anthropogenic emissions on weekdays are from the National Emission Inventory 2011 (NEI2011) (EPA, 2014) from the Pacific Northwest National Laboratory (PNNL), which has an initial resolution of 4 km and is regridded to REAM 36 km grid cells (Fig. 2). Weekday emission diurnal profiles are from NEI2011. The weekday-to-weekend emission ratios and weekend emission diurnal profiles are based on previous studies (Beirle et al., 2003; Boersma et al., 2009; Choi et al., 2012; de Foy, 2018; DenBleyker et al., 2012; Herman et al., 2009; Judd et al., 2018; Kaynak et al., 2009; Kim et al., 2016). These studies suggested that weekend NOx emissions were 20 %–50 % lower than weekday emissions, and the weekend NOx emission diurnal cycles were different from weekdays; therefore, we specify a weekend-to-weekday NOx emission ratio of in this study. The resulting diurnal variations in weekday and weekend NOx emissions over the DISCOVER-AQ 2011 region are shown in Fig. 3. The diurnal emission variation is lower on weekends than on weekdays.
To understand the effects of model resolutions on the temporospatial distributions of NO2, we also conduct a REAM simulation with a horizontal resolution of 4 km during the DISCOVER-AQ campaign. A 36 km REAM simulation (discussed in Sect. 3.2) provides the chemical initial and hourly boundary conditions. Meteorology fields are from a nested WRF simulation (36, 12, 4 km) with cumulus parameterization turned off in the 4 km domain (Table S2). Figure 1 shows a comparison of the 4 and 36 km REAM grid cells with DISCOVER-AQ observations, and Fig. 2 shows a comparison of NOx emission distributions between the 4 and 36 km REAM simulations. The comparison of NOx emission diurnal variations over the DISCOVER-AQ 2011 region between the 4 and 36 km REAM is shown in Fig. 3.
2.2 NO2 TVCD measurements by OMI and GOME-2A
The OMI instrument onboard the sun-synchronous NASA EOS Aura satellite with an Equator-crossing time of around 13:30 LT was developed by the Finnish Meteorological Institute and the Netherlands Agency for Aerospace Programs to measure solar backscattering radiation in the visible and ultraviolet bands (Levelt et al., 2006; Russell et al., 2012). The radiance measurements are used to derive trace gas concentrations in the atmosphere, such as O3, NO2, HCHO, and SO2 (Levelt et al., 2006). OMI has a nadir resolution of 13 km × 24 km and provides daily global coverage (Levelt et al., 2006).
Two widely used archives of OMI NO2 VCD products are available, NASA OMNO2 (v4.0) (https://disc.gsfc.nasa.gov/datasets/OMNO2_003/summary, last access: 26 September 2020) and KNMI DOMINO (v2.0) (https://www.temis.nl/airpollution/no2.php, last access: 14 January 2015). Although both use Differential Optical Absorption Spectroscopy (DOAS) algorithms to derive NO2 slant column densities, they have differences in spectral fitting, stratospheric and tropospheric NO2 slant column density (SCD) separation, a priori NO2 vertical profiles, air mass factor (AMF) calculation, etc. (Boersma et al., 2011; Bucsela et al., 2013; Chance, 2002; Krotkov et al., 2017; Lamsal et al., 2021; Marchenko et al., 2015; Oetjen et al., 2013; van der A et al., 2010; Van Geffen et al., 2015). Both OMNO2 and DOMINO have been extensively evaluated with field measurements and models (Boersma et al., 2009, 2011; Choi et al., 2020; Hains et al., 2010; Huijnen et al., 2010; Ionov et al., 2008; Irie et al., 2008; Lamsal et al., 2014, 2021; Oetjen et al., 2013). The estimated uncertainty in the DOMINO TVCD product includes an absolute component of 1.0 × 1015 molecules cm−2 and a relative AMF component of 25 % (Boersma et al., 2011), while the uncertainty in the OMNO2 TVCD product ranges from ∼ 30 % under clear-sky conditions to ∼ 60 % under cloudy conditions (Lamsal et al., 2014; Oetjen et al., 2013; Tong et al., 2015). In order to reduce uncertainties in this study, we only use TVCD data with effective cloud fractions < 0.2, solar zenith angle (SZA) < 80∘, and albedo ≤ 0.3. Both positive and negative TVCDs are considered in the calculation. The data affected by row anomaly are excluded (Boersma et al., 2018; R. Zhang et al., 2018).
For AMF calculation, DOMINO used daily TM4 model results with a resolution of 3∘ × 2∘ as a priori NO2 vertical profiles (Boersma et al., 2007, 2011), while OMNO2 v4.0 used monthly mean values from the Global Modeling Initiative (GMI) model with a resolution of 1∘ × 1.25∘. The relatively coarse horizontal resolution of the a priori NO2 profiles in the retrievals can introduce uncertainties in the spatial and temporal characteristics of NO2 TVCDs at satellite pixel scales. For comparison purposes, we also use 36 km REAM simulation results as the a priori NO2 profiles to compute the AMFs and NO2 TVCDs with the DOMINO algorithm. The 36 km REAM NO2 data are first regridded to OMI pixels to calculate the corresponding tropospheric AMFs, which are then applied to compute OMI NO2 TVCDs by dividing the tropospheric SCDs from the DOMINO product by our updated AMFs.
The GOME-2 instrument onboard the polar-orbiting MetOp-A satellite (now referred to as GOME-2A) is an improved version of GOME-1 launched in 1995 and has an overpass time of 09:30 LT and a spatial resolution of 80 km × 40 km (Munro et al., 2006; Peters et al., 2012). GOME-2A measures backscattered solar radiation in the range from 240 to 790 nm, which is used for VCD retrievals of trace gases, such as O3, NO2, BrO, and SO2 (Munro et al., 2006). We use the KNMI TM4NO2A v2.3 GOME-2A NO2 VCD product archived on http://www.temis.nl/airpollution/no2col/no2colgome2_v2.php (last access: 22 January 2015) (Boersma et al., 2007, 2011). GOME-2A-derived NO2 VCDs have been validated with SCIAMACHY and MAX-DOAS measurements (Irie et al., 2012; Peters et al., 2012; Richter et al., 2011). As in the case of OMI, we use the same criteria to filter the NO2 TVCD data and recalculate the tropospheric AMF values and GOME-2A TVCDs using the daily 36 km REAM NO2 profiles (09:00–10:00 LT).
2.3 Pandora ground-based NO2 VCD measurements
Pandora is a small direct sun spectrometer which measures sun and sky radiance from 270 to 530 nm with a 0.5 nm resolution and a 1.6∘ field of view (FOV) for the retrieval of the total VCDs of NO2 with a precision of about 5.4 × 1014 molecules cm−2 (2.7 × 1014 molecules cm−2 for NO2 SCD) and a nominal accuracy of 2.7 × 1015 molecules cm−2 under clear-sky conditions (Herman et al., 2009; Lamsal et al., 2014; Zhao et al., 2020). There were 12 Pandora sites operating in the DISCOVER-AQ campaign (Fig. 1). Six of them are the same as the P-3B aircraft spiral locations (Aldino, Edgewood, Beltsville, Essex, Fairhill, and Padonia) (Table S1 and Fig. 1). The other six sites are Naval Academy (Annapolis, Maryland) (USNA – ocean), University of Maryland College Park (UMCP – urban), University of Maryland Baltimore County (UMBC – urban), Smithsonian Environmental Research Center (SERC – rural and coastal), Oldtown in Baltimore (Oldtown – urban), and Goddard Space Flight Center (GSFC – urban and suburban) (Table S1 and Fig. 1). In this study, we exclude the USNA site as its measurements were conducted on a ship (“Pandora(w)” in Fig. 1), and there were no other surface observations in the corresponding REAM grid cell. Including the data from the USNA site has a negligible effect on the comparisons of observed and simulated NO2 TVCDs. In our analysis, we ignore Pandora measurements with SZA > 80∘ (Fig. S1 in the Supplement) and exclude the data when fewer than three valid measurements are available within an hour to reduce the uncertainties in the hourly averages due to the significant variations in Pandora observations (Fig. S2).
Since Pandora measures total NO2 VCDs, we need to subtract stratosphere NO2 VCDs from the total VCDs to compute TVCDs. As shown in Fig. S3, stratosphere NO2 VCDs show a clear diurnal cycle with an increase during daytime due in part to the photolysis of reactive nitrogen reservoirs such as N2O5 and HNO3 (Brohede et al., 2007; Dirksen et al., 2011; Peters et al., 2012; Sen et al., 1998; Spinei et al., 2014), which is consistent with the significant increase in stratospheric NO2 VCDs from GOME-2A to OMI. In this study, we use the GMI model-simulated stratospheric NO2 VCDs in Fig. S3 to calculate the Pandora NO2 TVCDs. The small discrepancies between the GMI stratospheric NO2 VCDs and satellite products do not change the pattern of Pandora NO2 TVCD diurnal variations or affect the conclusions in this study.
2.4 ACAM NO2 VCD measurements
The ACAM instrument onboard the UC-12 aircraft consists of two thermally stabilized spectrometers in the ultraviolet, visible, and near-infrared range. The spectrometer in the ultraviolet and visible band (304–520 nm) with a resolution of 0.8 nm and a sampling of 0.105 nm can be used to detect NO2 in the atmosphere. The native ground resolution of UC-12 ACAM NO2 measurements is 0.5 km × 0.75 km at a flight altitude of about 8 km a.s.l. and a nominal ground speed of 100 m s−1 during the DISCOVER-AQ 2011 campaign (Lamsal et al., 2017), thus providing high-resolution NO2 VCDs below the aircraft.
In this study, we mainly use the ACAM NO2 VCD product described by Lamsal et al. (2017), which applied a pair-average co-adding scheme to produce NO2 VCDs at a ground resolution of about 1.5 km (cross-track) × 1.1 km (along-track) to reduce noise impacts. In their retrieval of ACAM NO2 VCDs, they first used the DOAS fitting method to generate differential NO2 SCDs relative to the SCDs at an unpolluted reference location. Then they computed above- and below-aircraft AMFs at both sampling and reference locations based on the vector linearized discrete ordinate radiative transfer code (VLIDORT) (Spurr, 2008). In the computation of AMFs, the a priori NO2 vertical profiles were from a combination of high-resolution (4 km) CMAQ (the Community Multiscale Air Quality Modeling System) model outputs in the boundary layer and GMI simulation (2∘ × 2.5∘) results elsewhere in the atmosphere. Finally, the below-aircraft NO2 VCDs at the sampling locations were generated by dividing below-aircraft NO2 SCDs at the sampling locations by the corresponding below-aircraft AMFs. The below-aircraft NO2 SCDs were the differences between the total and above-aircraft NO2 SCDs. The total NO2 SCDs were the sum of DOAS-fitting-generated differential NO2 SCDs and NO2 SCDs at the reference location, and the above-aircraft NO2 SCDs were derived based on above-aircraft AMFs, GMI NO2 profiles, and OMNO2 stratospheric NO2 VCDs (Lamsal et al., 2017). The ACAM NO2 VCD product had been evaluated via comparisons with other independent observations during the DISCOVER-AQ 2011 campaign, such as P-3B aircraft, Pandora, and OMNO2, and the uncertainty in individual below-aircraft NO2 VCD is about 30 % (Lamsal et al., 2017). To keep the consistency of ACAM NO2 VCDs, we exclude NO2 VCDs measured at altitudes <8 km a.s.l., which accounts for about 6.8 % of the total available ACAM NO2 VCD data. We regrid the 1.5 km×1.1 km ACAM NO2 VCDs to the 4 km REAM grid cells (Fig. 1), which are then used to evaluate the distribution of NO2 VCDs in the 4 km REAM simulation. As a supplement in Sect. 3.7, we also assess the 4 km REAM simulation by using the UC-12 ACAM NO2 VCDs produced by the Smithsonian Astrophysical Observatory (SAO) algorithms, archived on https://www-air.larc.nasa.gov/cgi-bin/ArcView/discover-aq.dc-2011?UC12=1#LIU.XIONG/ (last access: December 31, 2019) (Liu et al., 2015a, b). This product is an early version of the SAO algorithm used to produce the Geostationary Trace gas and Aerosol Sensor Optimization (GeoTASO) and the GEOstationary Coastal and Air Pollution Events (GEO-CAPE) Airborne Simulator (GCAS) airborne observations in later airborne campaigns (Nowlan et al., 2016, 2018).
2.5 Surface NO2 and O3 measurements
The measurement of NOx is based on the chemiluminescence of electronically excited , produced from the reaction of NO with O3, and the strength of the chemiluminescence from the decay of to NO2 is proportional to the number of NO molecules present (Reed et al., 2016). NO2 concentrations can be measured with this method by converting NO2 to NO first through catalytic reactions (typically on the surface of heated molybdenum oxide (MoOx) substrate) or photolytic processes (Lamsal et al., 2015; Reed et al., 2016). However, for the catalytic method, reactive nitrogen compounds other than NOx (NOz), such as HNO3, peroxyacetyl nitrate (PAN), and other organic nitrates, can also be reduced to NO on the heated surface, thus causing an overestimation of NO2. The magnitude of the overestimation depends on the concentrations and the reduction efficiencies of interference species, both of which are uncertain. The photolytic approach, which employs broadband photolysis of ambient NO2, offers more accurate NO2 measurements (Lamsal et al., 2015).
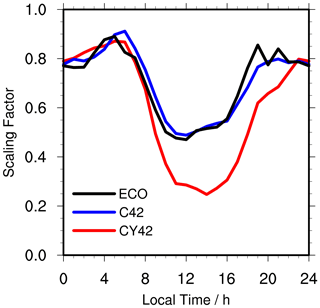
Figure 4Hourly ratios of NO2 measurements from the Teledyne API model 200 eup photolytic NOx analyzer to NO2 from coincident catalytic instruments for 2011 July. “CY42” denotes the ratios of photolytic NO2 to NO2 from the Thermo Electron 42C-Y NOy analyzer in Edgewood, “C42” denotes the ratios of photolytic NO2 to NO2 from the Thermo Model 42C NOx analyzer in Padonia, and “ECO” denotes the ratios of photolytic NO2 to NO2 from the Ecotech Model 9841 T-NOy analyzer in Padonia. “ECO” ratios are also used to scale NO2 measurements from the Ecotech Model 9843 T-NOy analyzer.
There were 11 NOx monitoring sites operating in the DISCOVER-AQ region during the campaign (Fig. 1), including those from the EPA Air Quality System (AQS) monitoring network and those deployed for the DISCOVER-AQ campaign. Nine of them measured NO2 concentrations by a catalytic converter. The other two sites (Edgewood and Padonia) had NO2 measurements from both catalytic and photolytic methods. Different stationary catalytic instruments were used during the campaign: Thermo Electron 42C-Y NOy analyzer, Thermo Model 42C NOx analyzer, Thermo Model 42I-Y NOy analyzer, and Ecotech Model 9843 and 9841 T-NOy analyzers. In addition, a mobile platform – NATIVE (Nittany Atmospheric Trailer and Integrated Validation Experiment) with a Thermo Electron 42C-Y NOy analyzer installed – was also deployed at the Edgewood site. The photolytic measurements of NO2 in Edgewood and Padonia were from Teledyne API model 200eup photolytic NOx analyzers. We scale catalytic NO2 measurements using the diurnal ratios of NO2 photolytic measurements to NO2 from the corresponding catalytic analyzers (Fig. 4). Figure 4 shows the lowest photolytic–catalytic ratio in the afternoon, which reflects the production of nitrates and other reactive nitrogen compounds from NOx in the daytime. When photolytic measurements are available, we only use the photolytic observations in this study; otherwise, we use the scaled catalytic measurements.
Nineteen surface O3 monitoring sites were operating in the DISCOVER-AQ region during the campaign (Fig. 1). They measured O3 concentrations by using a federal equivalent method (FEM) based on the UV absorption of O3 (https://cfpub.epa.gov/si/si_public_file_download.cfm?p_download_id=520887&Lab=NERL, last access: 12 July 2021) with an uncertainty of 5 ppb.
2.6 Aircraft measurements of NO2 vertical profiles
In this study, we mainly use the NO2 concentrations measured by the National Center for Atmospheric Research (NCAR) four-channel chemiluminescence instrument (P-CL) onboard the P-3B aircraft for the evaluation of REAM-simulated NO2 vertical profiles. The instrument has an NO2 measurement uncertainty of 10 %–15 % and a 1 s, 1σ detection limit of 30 pptv.
NO2 measurements from aircraft spirals provide us with NO2 vertical profiles. Figure 1 shows the locations of the aircraft spirals during the DISCOVER-AQ campaign, except for the Chesapeake Bay spirals over the ocean. There were only six spirals available over the Chesapeake Bay, which have ignorable impacts on the following analyses. Therefore, we do not use them in this study. The remaining 239 spirals in the daytime for July 2011 are used to compute the average profiles of NO2 for the six inland sites (Fig. 1).
The aircraft measurements were generally sampled from a height of about 300 (above ground level) in the boundary layer to 3.63 in the free troposphere. We bin these measurements to REAM vertical levels. In order to make up the missing observations between the surface and 300 m, we apply quadratic polynomial regressions by using aircraft data below 1 km and coincident NO2 surface measurements.
Table 1Comparison of the concentrations of NOy and its components between REAM and P-3B aircraft measurements during the DISCOVER-AQ campaign.
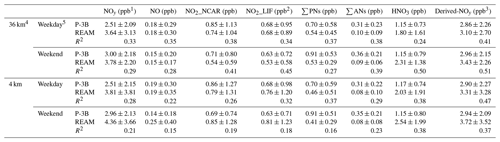
1 For P-3B, the concentrations of NOy, NO, and NO2_NCAR were measured by using the NCAR four-channel chemiluminescence instrument. The measurement uncertainties are 10 %, 10 %–15 %, and 10 % for NO, NO2, and NOy, respectively. The 1 s, 1σ detection limits are 20, 30, and 20 pptv for NO, NO2, and NOy, respectively (https://discover-aq.larc.nasa.gov/wp-content/uploads/sites/140/2020/05/Weinheimer20101005_DISCOVERAQ_AJW.pdf, last access: 28 June 2019). For REAM, NOy is the sum of NO, NO2, total peroxyacyl nitrates (∑PNs), total alkyl nitrates (∑ANs) (including alkyl nitrates and hydroxyalkyl nitrates), HNO3, HONO, 2 × N2O5, HNO4, first-generation C5 carbonyl nitrate (nighttime isoprene nitrate ISN1: C5H8NO4), 2 × C5 dihydroxy dinitrate (DHDN: C5H10O8N2), methyl peroxy nitrate (MPN: CH3O2NO2), propanone nitrate (PROPNN: CH3C(=O)CH2ONO2), nitrate from methyl vinyl ketone (MVKN: HOCH2CH(ONO2)C(=O)CH3), nitrate from methacrolein (MARCN: HOCH2C(ONO2)(CH3)CHO), and ethanol nitrate (ETHLN: CHOCH2ONO2).
2 For P-3B, the concentrations of NO2_LIF, ∑PNs, ∑ANs, and HNO3 were measured by applying the thermal-dissociation laser-induced fluorescence (TD-LIF) technique. The accuracy of TD-LIF measurements of NO2, ∑PNs, ∑ANs, and HNO3 is better than 15 %, and the detection limit for the sum of NO2, ∑PNs, ∑ANs, and HNO3 is ∼ 10 ppt 10 s−1 (Day et al., 2002).
3 To compare NOy concentrations from TD-LIF measurements with those from REAM, we calculate derived-NOy as the sum of NO, NO2_LIF, ∑PNs, ∑ANs, and HNO3. Only when the concentrations of all the five species are available at the same hour in the same grid cell can we calculate derived-NOy at the given hour in the given grid cell. Therefore, in Table 1, the averaged derived-NOy values are not exactly equal to the sum of averaged NO, NO2_LIF, ∑PNs, ∑ANs, and HNO3 concentrations that only depend on the availability of a single species. In addition, the measurement times and frequencies between NOy and derived-NOy are not the same. A comparison between these two types of data needs coincident sampling, as described in the main text.
4 Mean NOx emissions over the six P-3B spiral sites are close (relative difference < 4 %) between the 36 and 4 km REAM (Table S1).
5 Due to different sampling times and locations between weekdays and weekends, we do not recommend a direct comparison between weekday and weekend values here.
In addition to using NO2 concentrations from the NCAR four-channel instrument to evaluate REAM-simulated NO2 vertical profiles, we also use P-3B NO, NO2, and NOy concentrations measured by the NCAR four-channel instrument and NO2, total peroxyacyl nitrates (∑PNs), total alkyl nitrates (∑ANs) (including alkyl nitrates and hydroxyalkyl nitrates), and HNO3 concentrations measured by the thermal-dissociation laser-induced fluorescence (TD-LIF) technique (Day et al., 2002; Thornton et al., 2000; Wooldridge et al., 2010) to evaluate the concentrations of NOy from REAM (Table 1). All these P-3B measurements are vertically binned to REAM grid cells for comparisons with REAM results. In addition, below the P-3B spirals, four NOy observation sites at Padonia, Edgewood, Beltsville, and Aldino were operating to provide continuous hourly NOy surface concentrations during the campaign, which we also use to evaluate REAM-simulated NOy surface concentrations in this study. We summarize the information of available observations at the 11 inland Pandora sites in Table S1.
3.1 Evaluation of WRF-simulated meteorological fields
We evaluate the performances of the 36 km and nested 4 km WRF simulations using temperature, potential temperature, relative humidity (RH), and wind measurements from the P-3B spirals (Fig. 1) and precipitation data from the NCEP (National Centers for Environmental Prediction) Stage IV precipitation dataset. Generally, P-3B spirals range from ∼ 300 m to ∼ 3.63 km in height above the ground level (a.g.l.). As shown in Fig. S4, both the 36 km and nested 4 km WRF simulations simulate temperature well with R2 = 0.98. Both WRF simulations show good agreement with P-3B measurements in U wind (36 km: R2 = 0.77; 4 km: R2 = 0.76), V wind (36 km: R2 = 0.79; 4 km: R2 = 0.78), wind speed (36 km: R2 = 0.67; 4 km: R2 = 0.67), and wind direction (Figs. S4 and S5). We further compare the temporal evolutions of vertical profiles for temperature, potential temperature, RH, U wind, and V wind below 3 km from the P-3B observations with those from the 36 km and nested 4 km WRF simulations in Fig. S6. Both WRF simulations capture the temporospatial variations in P-3B-observed vertical profiles well except that RH below 1.5 km is significantly underestimated between 09:00 and 17:00 LT in both WRF simulations. The evaluations above suggest that WRF-simulated wind fields are good and comparable at 4 and 36 km resolutions, but potential dry biases exist in both WRF simulations.
The NCEP Stage IV precipitation dataset provides hourly precipitation across the contiguous United States (CONUS) with a resolution of ∼ 4 km based on the merging of rain gauge data and radar observations (Lin and Mitchell, 2005; Nelson et al., 2016). The Stage IV dataset is useful for evaluating model simulations, satellite precipitation estimates, and radar precipitation estimates (Davis et al., 2006; Gourley et al., 2011; Kalinga and Gan, 2010; Lopez, 2011; Yuan et al., 2008). We obtain the Stage IV precipitation data for July 2011 from the NCAR/UCAR Research Data Archive (https://rda.ucar.edu/datasets/ds507.5/, last access: 28 December 2019). As shown in Figs. S7 and S8, both the 36 km and nested 4 km WRF simulations generally predict much less precipitation (in precipitation amount and duration) compared to the Stage IV data in July 2011 around the DISCOVER-AQ campaign region, especially for the nested 4 km WRF simulation, consistent with the aforementioned underestimated RH and dry bias in WRF simulations. The precipitation biases in the WRF model will affect REAM simulations of trace gases, leading to high biases of soluble species due to underestimated wet scavenging. Clouds interfere with satellite observations. Therefore, the precipitation bias does not affect model evaluations with satellite measurements of NO2. Aircraft measurements were also taken on non-precipitating days.
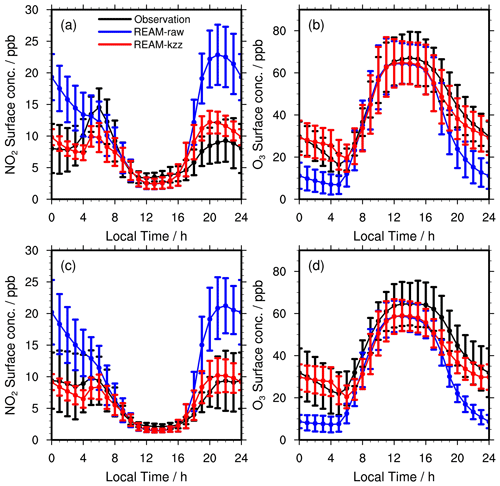
Figure 5Diurnal cycles of surface (a, c) NO2 and (b, d) O3 concentrations on (a, b) weekdays and (c, d) weekends during the DISCOVER-AQ campaign in the DISCOVER-AQ region (the 36 km grid cells over the 11 inland Pandora sites shown in Fig. 1). Black lines denote the mean observations from all the 11 NO2 surface monitoring sites and 19 O3 surface sites during the campaign (Fig. 1), as mentioned in Sect. 2.5. “REAM-raw” (blue lines) denotes the coincident 36 km REAM simulation results with WRF-YSU-simulated kzz data, and “REAM-kzz” (red lines) is the coincident 36 km REAM simulation results with updated kzz data. See the main text for details. Vertical bars denote corresponding standard deviations.
3.2 Effect of boundary layer vertical mixing on the diurnal variations in surface NO2 concentrations
3.2.1 36 km model simulation in comparison to the surface observations
Figure 5a and b show the observed and 36 km REAM-simulated diurnal cycles of surface NO2 and O3 concentrations on weekdays in July 2011 in the DISCOVER-AQ region. REAM with WRF-YSU-simulated vertical diffusion coefficient (kzz) values significantly overestimates NO2 concentrations and underestimates O3 concentrations at night, although it captures the patterns of the diurnal cycles of surface NO2 and O3: an O3 peak and an NO2 minimum around noontime. Here, YSU denotes the Yonsei University planetary boundary layer (PBL) scheme (Shin and Hong, 2011) used by our WRF simulations (Table S2). At night, the reaction of O3 + NO → O2 + NO2 produces NO2 but removes O3. Since most NOx emissions are in the form of NO, the model biases of low O3 and high NO2 occur at the same time. Since there are no significant chemical sources of O3 at night, mixing of O3-rich air above the surface is the main source of O3 supply near the surface. Therefore, the nighttime model biases with WRF-YSU-simulated kzz data in Fig. 5 indicate that vertical mixing may be underestimated at night.
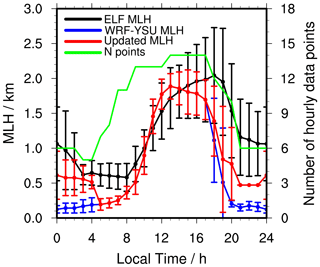
Figure 6ELF-observed and model-simulated diurnal variations in MLH at the UMBC site during the Discover-AQ campaign. “ELF MLH” denotes ELF-derived MLHs by using the covariance wavelet transform method. “WRF-YSU MLH” denotes the 36 km WRF-YSU kzz-determined MLHs, and “Updated MLH” denotes updated kzz-determined MLHs. See the main text for details. Vertical bars denote standard deviations. For the ELF MLHs, there are 13 506 1 min measurements in total during the campaign, and we bin them into hourly data. The green line corresponding to the right y axis shows the diurnal variations in the number of hourly ELF data points.
During the DISCOVER-AQ campaign, WRF-simulated vertical wind velocities are very low at night and have little impact on vertical mixing (Fig. S9a). The nighttime vertical mixing is mainly attributed to turbulent mixing. However, Hu et al. (2012) found that the YSU scheme underestimated nighttime PBL vertical turbulent mixing in WRF, which is consistent with Fig. 6, showing that WRF-YSU kzz-determined mixed-layer heights (MLHs) are significantly lower than lidar observations in the late afternoon and at night at the UMBC site during the DISCOVER-AQ campaign (Knepp et al., 2017). Here, the kzz-determined MLH refers to the mixing height derived by comparing kzz to its background values (Hong et al., 2006) but not the PBLH outputs from WRF. UMBC is an urban site (Table S1), surrounded by a mixture of constructed materials and vegetation. The UMBC lidar MLH data were derived from the Elastic Lidar Facility (ELF) attenuated backscatter signals by using the covariance wavelet transform (CWT) method and had been validated against radiosonde measurements (N (number of data points) = 24; R2 = 0.89; bias (ELF – radiosonde) = 0.03 ± 0.23 km), radar wind profiler observations (N = 659; R2 = 0.78; bias = −0.21 ± 0.36 km), and Sigma Space mini-micropulse lidar data (N = 8122; R2 = 0.85; bias = 0.02 ± 0.22 km) from the Howard University Beltsville Research Campus (HUBRC) in Beltsville, Maryland (38.058∘ N, 76.888∘ W) in the daytime during the DISCOVER-AQ campaign (Compton et al., 2013). It is noteworthy that although CWT is not designed to detect the nocturnal boundary layer (NBL), it does consider the residue layer (RL) and distinguish it from MLH in the early morning after sunrise, which is similar to nighttime conditions. Therefore, CWT can detect nighttime MLHs, although with large uncertainties due to the hard-coded assumption of RL = 1 km in the algorithm and insufficient vertical resolution of the technique. In addition, the sunrise and sunset time in July 2011 is about 05:00 and 19:30 LT (https://gml.noaa.gov/grad/solcalc/sunrise.html, last access: 27 May 2021), respectively. Figure 6 shows that WRF-YSU kzz-determined MLHs are significantly lower than ELF observations after sunrise between 05:00 and 08:00 LT and before sunset between 18:00 and 20:00 LT. Even if we do not consider MLHs at night (19:30–05:00 LT), we can still conclude that WRF-YSU underestimates vertical mixing in the early morning after sunrise and the late afternoon before sunset, enabling a reasonable assumption that WRF-YSU also underestimates nighttime vertical mixing. Moreover, the nighttime MLHs in Fig. 6 are comparable to those measured by the Vaisala CL51 ceilometer at the Chemistry And Physics of the Atmospheric Boundary Layer Experiment (CAPABLE) site in Hampton, Virginia (Knepp et al., 2017). Finally, we want to emphasize that different definitions of NBL can result in significantly different NBL heights (Breuer et al., 2014). In this study, we follow Knepp et al. (2017) to use MLHs derived from aerosol backscatter signals as the measure of vertical pollutant mixing within the boundary layer, which is simulated by kzz in REAM.
To improve nighttime PBL vertical turbulent mixing in REAM, we increase kzz below 500 m between 18:00 and 05:00 LT to 5 m s−2 if the WRF-YSU-computed kzz < 5 m s−2, which significantly increases the kzz-determined MLHs at night (Fig. 6), leading to the decreases in simulated surface NO2 and the increases in surface O3 concentrations at night (Fig. 5). The assigned value of 5 m s−2 is arbitrary. Changing this value to 2 or 10 m s−2 can also alleviate the biases of model-simulated nighttime surface NO2 and O3 concentrations (Fig. S10). Considering the potential uncertainties in nighttime NOx emissions, an alternative solution to correct the model nighttime simulation biases is to reduce NOx emissions, which can decrease the consumption of O3 through the process of NOx titration mentioned above (O3 + NO → O2 + NO2). Our sensitivity tests (not shown) indicate that it is necessary to reduce NOx emissions by 50 %–67 % to eliminate the model nighttime simulation biases, but we cannot find good reasons to justify this level of NOx emission reduction only at night.
The updated REAM simulation of surface NO2 diurnal pattern in Fig. 5a is in good agreement with previous studies (Anderson et al., 2014; David and Nair, 2011; Gaur et al., 2014; Reddy et al., 2012; Zhao et al., 2019). Daytime surface NO2 concentrations are much lower compared to nighttime, and NO2 concentrations reach a minimum around noontime. As shown in Fig. S11, under the influence of vertical turbulent mixing, the surface layer NOx emission diurnal pattern is similar to the surface NO2 diurnal cycle in Fig. 5a, emphasizing the importance of turbulent mixing on modulating surface NO2 diurnal variations. The highest boundary layer (Fig. 6) due to solar radiation leads to the lowest surface layer NOx emissions (Fig. S11), and, therefore, the smallest surface NO2 concentrations occur around noontime (Fig. 5a). Transport, which is mainly attributed to advection and turbulent mixing, is another critical factor affecting surface NO2 diurnal variations (Fig. S11). The magnitudes of transport fluxes (Fig. S11) are proportional to horizontal and vertical gradients of NOx concentrations and are therefore generally positively correlated to surface NO2 concentrations. However, some exceptions exist, reflecting different strengths of advection (U, V, and W) and turbulent mixing (kzz) at different times. For example, in the early morning, NO2 surface concentrations peak between 05:00 and 06:00 LT (Fig. 5a), while transport fluxes peak between 07:00 and 08:00 LT (Fig. S11). The delay of the peak is mainly due to lower turbulent mixing between 05:00 and 06:00 LT than other daytime hours in the model (Fig. 6). Chemistry also contributes to surface NO2 diurnal variations mainly through photochemical sinks in the daytime and N2O5 hydrolysis at nighttime. Chemistry fluxes in Fig. S11 are not only correlated to the strength of photochemical reactions and N2O5 hydrolysis (chemistry fluxes per unit NOx) but are also proportional to NOx surface concentrations. Therefore, chemistry fluxes in Fig. S11 cannot directly reflect the impact of solar radiation on photochemical reactions. It can, however, still be identified by comparing afternoon chemistry contributions: from 13:00 to 15:00 LT, surface layer NOx emissions and NO2 concentrations are increasing (Figs. S11 and 5a); however, chemistry losses are decreasing as a result of the reduction in photochemical sinks with weakening solar radiation. The contributions of vertical mixing and photochemical sinks to NO2 concentrations can be further corroborated by daytime variations in NO2 vertical profiles and TVCDs discussed in Sects. 3.3 and 3.4.
Figure 5c shows the diurnal variation on weekends is also simulated well in the improved 36 km model. The diurnal variation in surface NO2 concentrations (REAM: 1.5–10.2 ppb; observations: 2.1–9.8 ppb) is lower than on weekdays (REAM: 2.4–12.2 ppb; observations: 3.3–14.5 ppb), reflecting lower magnitude and variation in NOx emissions on weekends (Fig. 3). Figure 5d also shows an improved simulation of surface O3 concentrations at nighttime due to the improved MLH simulation (Fig. 6).
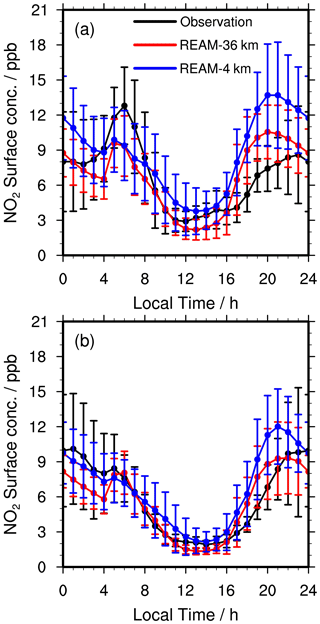
Figure 7Diurnal cycles of observed and simulated average surface NO2 concentrations over Padonia, Oldtown, Essex, Edgewood, Beltsville, and Aldino (Table S1) on (a) weekdays and (b) weekends. Black lines denote mean observations from the six sites. Red lines denote coincident 36 km REAM simulation results, and blue lines are for coincident 4 km REAM simulation results. Error bars denote standard deviations.
3.2.2 4 km model simulation in comparison to the surface observations
The results of 4 km REAM simulations with original WRF-YSU kzz (not shown) are very similar to Fig. 5 since WRF-simulated nocturnal vertical mixing is insensitive to the model horizontal resolution. Applying the modified nocturnal mixing in the previous section also greatly reduced the nighttime NO2 overestimate and O3 underestimate in the 4 km REAM simulations. All the following analyses are based on REAM simulations with improved nocturnal mixing. Figure 7 shows that mean surface NO2 concentrations simulated in the 4 km model are higher than the 36 km results over Padonia, Oldtown, Essex, Edgewood, Beltsville, and Aldino (Table S1), leading to generally higher biases compared to the observations in the daytime. A major cause is that the observation sites are located in regions of high NOx emissions (Fig. 2). At a higher resolution of 4 km, the high emissions around the surface sites are apparent compared to rural regions. At the coarser 36 km resolution, spatial averaging greatly reduces the emissions around the surface sites. On average, NOx emissions () around the six surface NO2 observation sites are 67 % higher in the 4 km than the 36 km REAM simulations (Table S1). The resolution dependence of model results will be further discussed in the model evaluations using the other in situ and remote sensing measurements.
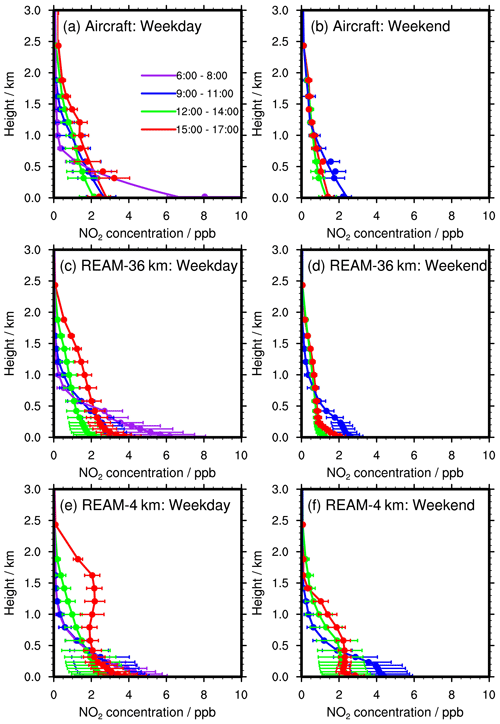
Figure 8Temporal evolutions of NO2 vertical profiles below 3 km on (a, c, e) weekdays and (b, d, f) weekends from the (a, b) P-3B aircraft and (c, d) 36 km and (e, f) 4 km REAM during the DISCOVER-AQ campaign. Horizontal bars denote the corresponding standard deviations. In (a, b), dots denote aircraft measurements, while lines below 1 km are based on quadratic polynomial fitting, as described in Sect. 2.6. The fitting values are mostly in reasonable agreement with the aircraft and surface measurements in the boundary layer. On weekends, no aircraft observations were made between 06:00 and 08:00 LT, and therefore no corresponding model profiles are shown.
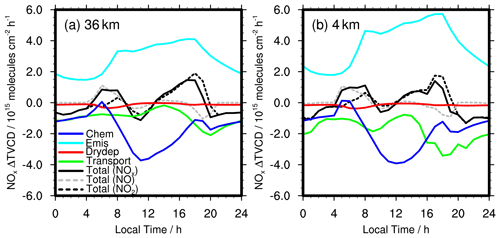
Figure 9Contributions of emission, chemistry, transport, and dry deposition to NOx TVCD diurnal variations over the 11 inland Pandora sites (Table S1 and Fig. 1) on weekdays in July 2011 for the (a) 36 km and (b) 4 km REAM simulations. “Chem” refers to net NOx chemistry production; “Emis” refers to NOx emissions; “Drydep” denotes NOx dry depositions; “Transport” includes advection, turbulent mixing, lightning NOx production, and wet deposition. “Total (NOx)” is the hourly change in NOx TVCDs ((TVCD) = TVCDt+1 − TVCDt). “Total (NO2)” is the hourly change in NO2 TVCDs, and “Total (NO)” is the hourly change in NO TVCDs.
3.3 Diurnal variations in NO2 vertical profiles
Figure 8a and c show the temporal variations in P-3B-observed and 36 km REAM-simulated NO2 vertical profiles in the daytime on weekdays during the DISCOVER-AQ campaign. The 36 km REAM reproduces the observed characteristics of NO2 vertical profiles well in the daytime (R2 = 0.89), which are strongly affected by vertical mixing and photochemistry (Y. Zhang et al., 2016). When vertical mixing is weak in the early morning (06:00–08:00 LT), NO2, released mainly from surface NOx sources, is concentrated in the surface layer, and the vertical gradient is large. As vertical mixing becomes stronger after 08:00 LT, NO2 concentrations below 500 m decrease significantly, while those over 500 m increase from 06:00–08:00 to 12:00–14:00 LT. It is noteworthy that MLHs and NOx emissions are comparable between 12:00–14:00 and 15:00–17:00 LT (Figs. 3 and 6); however, NO2 concentrations between 15:00 and 17:00 LT are significantly higher than between 12:00 and 14:00 LT in the whole boundary layer, reflecting the impact of the decreased photochemical loss of NOx in the late afternoon. In fact, photochemical losses affect all the daytime NO2 vertical profiles, which can be easily identified by NO2 TVCD process diagnostics discussed in Sect. 3.4 (Fig. 9).
Figure 8b and d also show the observed and 36 km REAM-simulated vertical profiles on weekends. Similar to Figs. 5 and 7, observed and simulated concentrations of NO2 are lower on weekends than on weekdays. Some of the variations from weekend profiles are due to a lower number of observations (47 spirals) on weekends. The overall agreement between the observed vertical profiles and 36 km model results is good on weekends (R2 = 0.87). Between 15:00 and 17:00 LT, the model simulates a larger gradient than what the combination of aircraft and surface measurements indicates. It may be related to the somewhat underestimated MLHs in the late afternoon in the model (Fig. 6).
On weekdays, most simulated vertical profiles at the 4 km resolution (Fig. 8e) are similar to 36 km results in part because the average NOx emissions over the six P-3B spiral sites are about the same, 4 % lower in the 4 km than the 36 km REAM simulations (Table S1). A clear exception is the 4 km REAM-simulated vertical profile Between 15:00 and 17:00 LT when the model greatly overestimates boundary layer NOx mixing and concentrations. The main reason is that WRF-simulated vertical velocities (W) in the late afternoon are much larger in the 4 km simulation than the 36 km simulation (Fig. S9), which can explain the simulated fully mixed boundary layer between 15:00 and 17:00 LT. Since it is not designed to run at the 4 km resolution, and it is commonly assumed that convection can be resolved explicitly at high resolutions, the Kain–Fritsch (new Eta) convection scheme is not used in the nested 4 km WRF simulation (Table S2); it may be related to the large vertical velocities in the late afternoon, when thermal instability is the strongest. Appropriate convection parameterization is likely still necessary for 4 km simulations (Zheng et al., 2016), which may also help alleviate the underestimation of precipitation in the nested 4 km WRF simulation as discussed in Sect. 3.1.
The same rapid boundary layer mixing due to vertical transport is present in the 4 km REAM-simulated weekend vertical profile (Fig. 8f), although the mixing height is lower. Fewer spirals (47) and distinct transport effect due to different NO2 horizontal gradients between the 4 and 36 km REAM simulations (discussed in detail in Sect. 3.6) may cause the overestimation of weekend profiles in the 4 km REAM simulation.
3.4 Daytime variation in NO2 TVCDs
We compare satellite, P-3B aircraft, and model-simulated TVCDs with Pandora measurements, which provide continuous daytime observations. The locations of Pandora sites are shown in Table S1 and Fig. 1. Among the Pandora sites, four sites are located significantly above the ground level: UMCP (∼ 20 m), UMBC (∼ 30 m), SERC (∼ 40 m), and GSFC (∼ 30 m). The other sites are 1.5 To properly compare Pandora to other measurements and model simulations, we calculate the missing TVCDs between the Pandora site heights and ground surface by multiplying the Pandora TVCDs with model-simulated TVCD fractions of the corresponding columns. The resulting correction is 2 %–21 % () for the four sites significantly above the ground surface, but the effect on the averaged daytime TVCD variation at all Pandora sites is small (Fig. S12). In the following analysis, we use the updated Pandora TVCD data.
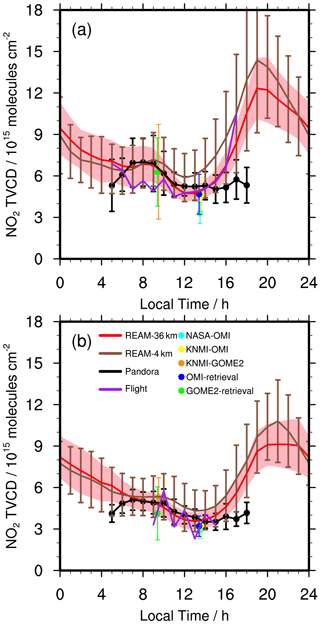
Figure 10Daily variations in NO2 TVCDs on (a) weekdays and (b) weekends during the DISCOVER-AQ campaign. “REAM-36km” refers to the 36 km REAM simulation results over the 11 inland Pandora sites. “REAM-4 km” refers to the 4 km REAM simulation results over the 11 inland Pandora sites. “Pandora” refers to updated Pandora TVCD data. “Flight” denotes P-3B-aircraft-derived NO2 VCDs below 3.63 km. “NASA-OMI” denotes the OMI NO2 TVCDs retrieved by NASA over the Pandora sites; “KNMI-OMI” denotes the OMI NO2 TVCDs from KNMI; “KNMI-GOME2” is the GOME-2A NO2 TVCDs from KNMI. “OMI-retrieval” and “GOME2-retrieval” denote OMI and GOME-2A TVCDs retrieved by using the KNMI DOMINO algorithm with corresponding 36 km REAM vertical profiles, respectively. The vertical bars denote corresponding standard deviations for all data except the 36 km REAM simulation results, the standard deviations of which are shown with pink shading. We list NO2 TVCD values at 09:30 and 13:30 LT in Table S3.
The weekday diurnal variations in NO2 TVCDs from satellites, Pandora, 4 and 36 km REAM, and the P-3B aircraft are shown in Fig. 10a. We calculate aircraft-derived TVCDs by using Eq. (1):
where t is time, caircraft () denotes aircraft NO2 concentrations (mixing ratios) at each level at time t, ρREAM (molecules cm−3) is the density of air from 36 km REAM at the corresponding level, VREAM (cm3) is the volume of the corresponding 36 km REAM grid cell, and AREAM (cm2) is the surface area (36 km × 36 km). In the calculation, we only use NO2 concentrations below 3.63 because few aircraft measurements were available above this height in the campaign. Missing tropospheric NO2 above 3.63 in the aircraft TVCD calculation has little impact on our analyses as the 36 km REAM model simulation shows that 85 % ± 7 % of tropospheric NO2 is located below 3.63 between 06:00 and 17:00 LT in the DISCOVER-AQ region, which is roughly consistent with the GMI model results with 85 %–90 % tropospheric NO2 concentrated below 5 km (Lamsal et al., 2014). It should be noted that only six P-3B spirals are available during the campaign, less than the samplings of 11 inland Pandora sites.
The 4 km REAM-simulated NO2 TVCDs are mostly higher than the 36 km results and the observations in daytime on weekdays (Fig. 10a). However, since the standard deviations of the data are much larger than the model difference, the 4 and 36 km model results generally show similar characteristics relative to the observations. REAM simulation results are in reasonable agreement with Pandora, P-3B aircraft, and satellite daytime NO2 TVCDs, except that NASA-derived OMI (OMNO2) TVCDs are somewhat lower than other datasets, which may be partly due to biased a priori vertical profiles from the GMI model in the NASA retrieval in the campaign (Lamsal et al., 2014, 2021). TVCDs derived by using the DOMINO algorithm and 36 km REAM NO2 vertical profiles are in agreement with those from KNMI, which indicates that the TM4 model from KNMI provides reasonable estimates of a priori NO2 vertical profiles on weekdays in the DISCOVER-AQ region in summer.
We find evident decreases in NO2 TVCDs from GOME-2A to OMI in Fig. 10a, which is consistent with Pandora, REAM results, and previous studies that showed decreasing NO2 TVCDs from SCIAMACHY to OMI due to photochemical losses in summer (Boersma et al., 2008, 2009). P-3B aircraft TVCDs also show this decrease feature but have large variations due in part to the limited aircraft sampling data.
Pandora NO2 TVCD data have different characteristics from REAM-simulated and P-3B-aircraft-measured TVCDs between 05:00 and 07:00 and between 14:00 and 18:00 LT (Fig. 10a). Between 05:00 and 07:00 LT, Pandora data show a significant increase in NO2 TVCDs, but REAM and aircraft TVCDs generally decrease, except for 4 km REAM TVCDs, with a slight increase from 06:00 to 07:00 LT on weekdays. Between 14:00 and 18:00 LT, Pandora TVCDs have little variation, but REAM and aircraft TVCDs increase significantly. The relatively flat Pandora TVCDs in the late afternoon compared to REAM and P-3B aircraft measurements are consistent with Lamsal et al. (2017), which found that Pandora VCDs were 26 %–30 % lower than UC-12 ACAM measurements from 16:00 to 18:00 LT during the DISCOVER-AQ campaign. We show the simulated effects of emission, chemistry, transport, and dry deposition on NOx TVCDs in Fig. 9. The simulated early-morning slight decrease in NO2 TVCDs is mainly due to the chemical transformation between NO2 and NO favoring the accumulation of NO under low-O3 and low-HO2 and low-RO2 conditions; thus NO TVCDs increase significantly, but NO2 TVCDs continue decreasing slowly during the period. The increase in the late afternoon is primarily due to the decrease in photochemistry-related sinks. The reasons for the discrepancies of NO2 TVCDs between Pandora and REAM results during the above two periods are unclear. Large SZAs in the early morning and the late afternoon (Fig. S1) lead to the higher uncertainties in Pandora measurements (Herman et al., 2009), although we have excluded Pandora measurements with SZA > 80∘. In addition, Pandora is a sun-tracking instrument with a small effective FOV and is sensitive to local conditions within a narrow spatial range, which may differ significantly from the average properties of 36 and 4 km grid cells depending upon the time of the day (Fig. S13) (Herman et al., 2009, 2018, 2019; Judd et al., 2018, 2019, 2020; Lamsal et al., 2017; Reed et al., 2015). As we mentioned above, ∼ 85 % of tropospheric NO2 is located below 3.63 km in the DISCOVER-AQ 2011 region based on the 36 km REAM simulation results. The Pandora FOV of 1.6∘ is approximately equivalent to a nadir horizontal extension of only 0.1 km ( km) at 3.63 and 30 m at 1.0 Therefore, Pandora measures different air columns of NO2 at different times of the day, especially in the morning and afternoon, when SZA is large, as shown in Fig. S13. Considering the potential spatial heterogeneity of boundary layer NO2, it is possible that the morning (east), noontime (nadir), and afternoon (west) NO2 VCDs are significantly different from each other. Unlike Pandora, satellites and aircraft are far from the ground surface and cover large areas; therefore, the impact of SZA on their NO2 VCD measurements is insignificant compared to Pandora measurements. Another possible reason is that Pandora instruments had few observations in the early morning, and the resulting average may not be representative (Fig. S2).
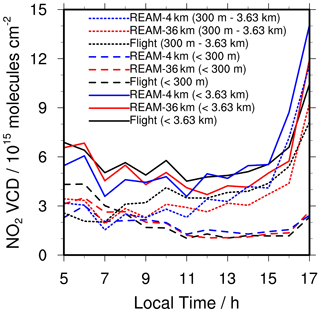
Figure 11Weekday hourly variations in NO2 VCDs at different height (a.g.l.) bins (< 3.63 , < 300 , and 300 m ∼ 3.63 ) based on P-3B-aircraft-derived datasets and the 36 and 4 km REAM results. “Flight” denotes P-3B-aircraft-derived NO2 VCDs, “REAM-36km” denotes coincident 36 km REAM-simulated VCDs, and “REAM-4 km” denotes coincident 4 km REAM-simulated VCDs.
To further understand the daytime variation in NO2 TVCDs, we examine P-3B-aircraft-data-derived and REAM-simulated NO2 VCD variations for different height bins (Fig. 11). NO2 VCDs below 3.63 display a U-shaped pattern from 05:00 to 17:00 LT. In the morning, as vertical mixing becomes stronger after sunrise, high-NOx air in the lower layer is mixed with low-NOx air in the upper layer. The increase in NOx vertical mixing above 300 m is sufficient to counter the increase in photochemical loss in the morning. Conversely, the NO2 VCDs below 300 m decrease remarkably from sunrise (about 06:00 LT) to around noontime due to both vertical mixing and the increase in photochemical strength. From 13:00 to 16:00 LT, NO2 VCDs increase slowly, reflecting a relative balance among emissions, transport, chemistry, and dry depositions. The sharp jump of the VCDs from 16:00 to 17:00 LT is mainly due to dramatically reduced chemical loss. And 4 km REAM-simulated NO2 VCDs at 0.30–3.63 km between 16:00 and 17:00 LT are much higher than 36 km results partly because of the rapid vertical mixing in the 4 km REAM simulation (Figs. 8 and S9).
Similar to NO2 surface concentrations and vertical profiles in Figs. 7 and 8, the NO2 TVCD variation is also smaller on weekends than on weekdays, but the day–night pattern is similar (Fig. 10). Although the 4 km REAM NO2 TVCDs are generally higher than the 36 km results and observations in the daytime, considering their large standard deviations, NO2 TVCDs from both simulations are comparable to satellite products, Pandora, and P-3B aircraft observations most of the time on weekends. The exception is that Pandora TVCDs have different variation patterns in the early morning and late afternoon from REAM simulations, similar to those found on weekdays.
3.5 Model comparisons with NOy measurements
NOy is longer-lived than NOx, and NOy concentrations are not affected by chemistry as much as NOx. We obtain two types of NOy concentrations from the P-3B aircraft in the DISCOVER-AQ campaign: one is NOy concentrations directly measured by the NCAR four-channel instrument, corresponding to the sum of NO, NO2, ∑PNs, ∑ANs, HNO3, N2O5, HNO4, HONO, and the other reactive nitrogenic species in REAM (all the other species are described in Table 1), and the other one, which we name “derived-NOy”, is the sum of NO from the NCAR four-channel instrument and NO2 (NO2_LIF), ∑PNs, ∑ANs, and HNO3 measured by the TD-LIF technique, corresponding to NO, NO2, ∑PNs, ∑ANs, and HNO3 in REAM (Table 1). On average, P-3B derived-NOy concentrations (2.88 ± 2.24 ppb) are 17 % higher than coincident P-3B NOy concentrations (2.46 ± 2.06 ppb), with R2 = 0.75, generally reflecting consistency between these two types of measurements. As shown in Table 1, on weekdays, the 36 km REAM NOy concentrations are 45 % larger than P-3B, with R2 = 0.33, and the 36 km REAM derived-NOy concentrations are 8 % larger than P-3B, with R2 = 0.41. The 4 km REAM shows similar results, suggesting that REAM simulations generally reproduce the observed NOy and derived-NOy concentrations within the uncertainties, although the average values from REAM are somewhat larger than the observations due in part to the underestimate of precipitation in the WRF model simulations resulting in underestimated wet scavenging of HNO3 in REAM. The concentrations of weekday NO, NO2, and ∑PNs from REAM simulations are also comparable to the observations. However, weekday ∑ANs concentrations are 68 % lower in the 36 km REAM than observations, suggesting that the chemistry mechanism in REAM may need further improvement to better represent isoprene nitrates. It is noteworthy that, since ∑ANs only account for a small fraction (∼ 11 %) of observed derived-NOy, the absolute difference between REAM-simulated and P-3B-observed ∑ANs concentrations is still small compared to HNO3. Weekday HNO3 concentrations are significantly higher in REAM simulations (36 km: 57 %, 0.65 ppb; 4 km: 74 %, 0.86 ppb) than P-3B observations, which is the main reason for the somewhat larger NOy and derived-NOy concentrations in REAM compared to P-3B observations. The higher HNO3 concentrations in REAM may be related to the underestimation of precipitation in the corresponding WRF simulations, as discussed in Sect. 3.1 (Figs. S7 and S8), leading to the underestimated wet scavenging of HNO3, especially for the 4 km REAM simulation.
We also examine the weekday diurnal variations in derived-NOy vertical profiles from P-3B and REAM simulations in Fig. S14. Generally, both 36 and 4 km REAM simulations capture the variation characteristics of observed vertical profiles, which are similar to those for NO2 in Fig. 8. REAM derived-NOy concentrations are comparable to P-3B observations at most vertical levels on weekdays. Some larger derived-NOy concentrations in the model results can be partially explained by larger HNO3 concentrations in REAM, such as those below 1 km between 09:00 and 11:00 LT for the 36 km REAM and those below 2.0 km between 12:00 and 17:00 LT for the 4 km REAM (Fig. S15).
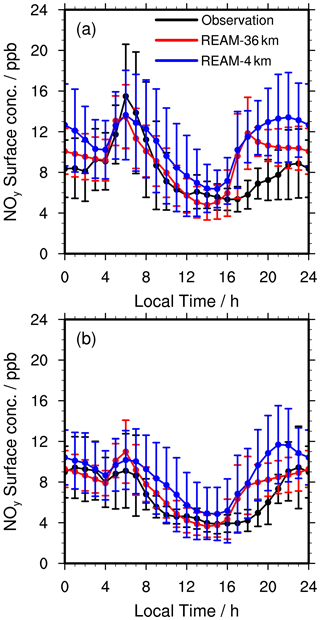
Figure 12Diurnal cycles of observed and simulated average surface NOy concentrations at Padonia, Edgewood, Beltsville, and Aldino on (a) weekdays and (b) weekends. Vertical bars denote the corresponding standard deviations. It is noteworthy that the mean NOx emissions over Padonia, Edgewood, Beltsville, and Aldino are 99 % higher in the 4 than the 36 km REAM simulations (Table S1).
Figure 12 shows the comparison of the diurnal cycles of surface NOy concentrations observed at Padonia, Edgewood, Beltsville, and Aldino during the DISCOVER-AQ campaign with those from the REAM simulations. Generally, the REAM simulations reproduce the observed surface NOy diurnal cycles except for the spikes around 17:00–20:00 LT due to still-underestimated MLHs (Fig. 6). The 4 km simulation results have a higher bias than 36 km results relative to the observations in the daytime, similar to the comparisons of NO2 surface concentrations and TVCDs in Figs. 7 and 10 due to higher emissions around the observation sites in 4 than 36 km simulations (Table S1 and Fig. 2).
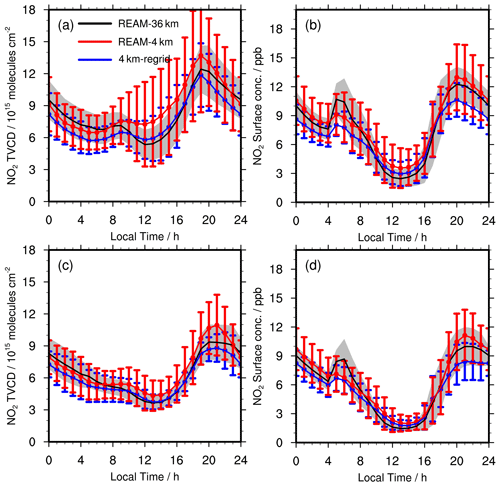
Figure 13Comparisons of NO2 (a, c) TVCDs and (b, d) surface concentrations over the 11 inland Pandora sites between the 4 and 36 km REAM simulations on (a, b) weekdays and (c, d) weekends for July 2011. “REAM-36km” (black lines) denotes the 36 km REAM simulation results; “REAM-4 km” (red lines) denotes the 4 km REAM simulation results; “4 km-regrid” (blue lines) refers to the 36 km values by regridding the 4 km REAM simulation results into 36 km REAM grid cells. The vertical bars denote corresponding standard deviations for all data except the 36 km REAM simulation results, the standard deviations of which are shown with gray shading.
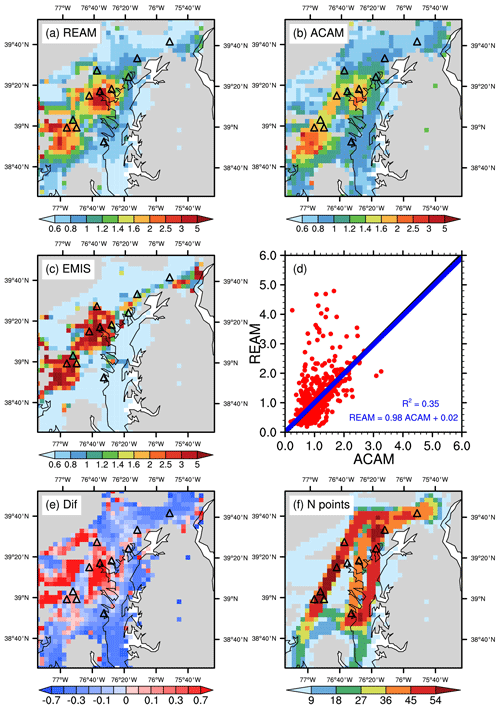
Figure 14Distributions of the scaled mean (a) 4 km REAM-simulated NO2 VCDs below the UC-12 aircraft and (b) coincident ACAM measurements on weekdays in July 2011. (c) The distribution of the scaled NEI2011 NOx emissions on weekdays. (d) The scatterplot of the scaled 4 km REAM and ACAM NO2 VCDs from (a, b). Panel (e) shows the relative differences between (a) and (b) (). (f) The distribution of the number of data points used to calculate grid cell mean NO2 VCDs in (a, b). Here, we scale all values (VCDs and NOx emissions) based on their corresponding domain averages. The domain averages of ACAM and coincident 4 km REAM NO2 VCDs are 4.7 ± 2.0 and 4.6 ± 3.2 × 1015 molecules cm−2, respectively.
3.6 Resolution dependence of NOx emission distribution
We showed previously that the 4 km REAM-simulated NO2 and NOy surface concentrations and NO2 TVCDs are higher than observations in the daytime in comparison to the corresponding 36 km REAM results (Figs. 7, 10, and 12). An examination of monthly mean NO2 surface concentrations and TVCDs for July 2011 also shows that 4 km simulation results are significantly higher than the 36 km results over the 11 inland Pandora sites in the daytime (Fig. 13). The process-level diagnostics in Fig. 9 indicate that the mean contribution of NOx emissions to NOxΔTVCDs in the 4 km simulation is 1.32 × 1015 larger than that in the 36 km simulation between 09:00 and 16:00 LT, while the absolute mean contributions of chemistry and transport (they are negative in Fig. 9, so we use absolute values here) in the 4 km simulation are 0.26 × 1015 and 0.87 × 1015 larger than the 36 km simulation, respectively. The contributions of dry deposition to NOxΔTVCDs are negligible compared to other factors in both simulations (Fig. 9). Therefore, the 34 % higher NOx emissions over the 11 inland Pandora sites (Table S1 and Fig. 3) are the main reason for the larger daytime NO2 surface concentrations and TVCDs in the 4 km than the 36 km REAM simulations (Fig. 13). The significantly different contribution changes between NOx emissions (1.32 × 1015 , or about one-third) and chemistry (0.26 × 1015 , or about 8 %) reflect potential chemical nonlinearity (Li et al., 2019; Silvern et al., 2019; Valin et al., 2011) and transport effect. Different transport contributions between the 4 km and the 36 km REAM are mainly caused by their different NOx horizontal gradients (Figs. 2, 14, and 15), while the impact of wind fields is small since we do not find significant differences in horizontal wind components between the two simulations except for some lower wind speeds below 1000 m for the 36 km WRF simulation compared to the nested 4 km WRF simulation (Fig. S16). Our sensitivity tests with the WRF single-moment three-class (WSM3) simple ice scheme (not shown) can improve the wind speed comparison below 1000 m between the 36 km and nested 4 km WRF simulations but still produce similar NOx simulation results as WSM6 shown here. Therefore, the somewhat lower wind speeds below 1000 m in the 36 km WRF simulation are not the reason for the difference between the 4 and 36 km REAM simulations. The impact of transport on the two REAM simulations can be further verified by the comparison of NO2 TVCDs over the six P-3B spiral sites between the two simulations (Fig. S17). Mean NOx emissions over the six P-3B spiral sites are close (relative difference < 4 %) between the two simulations (Table S1 and Fig. S17). From 09:00 to 12:00 LT, the contributions of NOx emissions to NOxΔTVCDs are 2.50 × 1015 and 2.49 × 1015 for the 36 and 4 km REAM simulations, respectively, and the contributions of chemistry are also close between the two simulations (36 km: −2.62 × 1015 ; 4 km: −2.69 × 1015 ). However, the contributions of transport are −0.39 × 1015 and 0.03 × 1015 for the 36 and 4 km REAM simulations, respectively, leading to larger NO2 TVCDs in the 4 km REAM simulation than the 36 km REAM from 09:00–12:00 LT (Fig. S17c). Since horizontal wind fields over the six P-3B spiral sites are comparable between two simulations (Figs. S4, S5, S6, and S16), and larger NOx horizontal gradients are found near the P-3B spiral sites for the 4 km REAM (Fig. 2), we attribute the different transport contributions between the two simulations to a much larger NOx emission gradient around the measurement locations in 4 than 36 km emission distributions.
We regrid the 4 km REAM results into the grid cells of the 36 km REAM, which can significantly reduce the impact of different NOx emission distributions and associated transport on the two simulations. Compared to the original 4 km REAM results, the regridded surface NO2 concentrations and TVCDs over the 11 inland Pandora sites are much closer to the 36 km REAM results (Fig. 13). After regridding the 4 km REAM results into 36 km REAM grid cells, we also find more comparable NOy surface concentrations between the regridded 4 km results and the 36 km REAM results (Fig. S18). The remaining discrepancies between the regridded results and the 36 km REAM results may be due to chemical nonlinearity and other meteorological effects, such as larger vertical wind in the 4 km REAM (Fig. S9) and their different kzz values in the PBL. Although other factors, such as chemical nonlinearity and vertical diffusion, may affect the 36 and 4 km REAM simulations differently, the difference between 4 and 36 km simulations of reactive nitrogen is largely due to that of NOx emissions.
The 4 and 36 km simulation difference depends on the location of the observations. In some regions, the NOx emission difference between 4 and 36 km simulations is small. The comparison of NOy measurements from P-3B spirals with coincident REAM results in Table 1 suggests that the 4 and 36 km REAM simulations produce similar NOy (relative difference ∼ 4 %) and derived-NOy (relative difference ∼ 6 %) concentrations on weekdays, and both simulation results are comparable to the observations. The NOy similarity over the P-3B spiral sites between the 36 and 4 km REAM simulations is consistent with the comparable NOx emissions over (relative difference < 4 %) the six P-3B spiral sites between the two simulations (Table S1). The differences between the 4 km model simulation results and P3-B observations are larger on weekends than on weekdays (Table 1) due to the limited weekend sampling since model-simulated monthly mean values show similar differences between the 4 and 36 km REAM simulations on weekends as on weekdays (not shown).
3.7 Evaluation of 36 and 4 km NOx distribution with OMI, GOME-2A, and ACAM measurements
The evaluation of model simulations of surface, aircraft, and satellite observations tends to point out a higher bias in 4 than 36 km model simulations. We note that this comparison is based on the averages of multiple sites. NOx emissions at individual sites are not always higher in the 4 than 36 km REAM, such as SERC, Fairhill, and Essex, with much higher 36 km NOx emissions than 4 km NOx emissions (Table S1). We conduct individual-site comparisons of surface NO2 concentrations, surface NOy concentrations, NO2 vertical profiles, derived-NOy vertical profiles, and NO2 TVCDs of the 36 km REAM and the 4 km REAM results relative to the corresponding observations in Figs. S19–S23. The 36 km simulation results can be larger, smaller, or comparable to the 4 km simulation results, and both simulations can produce higher, lower, or similar results as the observations for different variables at different sites. The varying model biases depending on the observation site reflect the different spatial distributions of NOx emissions between the 36 and 4 km REAM simulations (Fig. 2) and suggest potential distribution biases of NOx emissions in both simulations.
Here we examine the 4 km model-simulated NO2 VCDs with high-resolution ACAM measurements onboard the UC-12 aircraft in Figs. 14 and S24, respectively. The spatial distributions of ACAM and 4 km REAM NO2 VCDs are generally consistent with R2 = 0.35 on weekdays and R2 = 0.50 on weekends. The domain averages of ACAM and 4 km REAM NO2 VCDs are 4.7 ± 2.0 and 4.6 ± 3.2 × 1015 molecules cm−2 on weekdays and 3.0 ± 1.7 and 3.3 ± 2.7 × 1015 molecules cm−2 on weekends, respectively. The spatial distributions of ACAM and 4 km REAM NO2 VCDs are highly correlated with the spatial distribution of 4 km NEI2011 NOx emissions. All three distributions capture two strong peaks around Baltimore and Washington, DC, urban regions and another weak peak in the northeast corner of the domain (Wilmington, Delaware) (Figs. 14 and S24). However, Figs. 14 and S24 clearly show that NO2 VCDs from the 4 km REAM simulation are more concentrated in Baltimore and Washington, DC, urban regions than ACAM, which are also reflected by the higher NO2 VCD standard deviations of the 4 km REAM results than ACAM. Several Pandora sites are in the highest NO2 VCD regions, where the 4 km REAM generally produces larger NO2 VCDs than ACAM, which explains why the NO2 TVCDs over the 11 Pandora sites from the 4 km REAM simulation are higher than the observations (Fig. 10) and the 36 km REAM results (Fig. 13) around noontime. Horizontal transport cannot explain the NO2 VCD distribution biases in the 4 km REAM simulation due to the following reasons. Firstly, horizontal wind fields are simulated as well by the nested 4 km WRF simulation as the 36 km WRF compared to P-3B measurements, as discussed in Sect. 3.1. Secondly, the prevailing northwest wind in the daytime (Fig. S5) should move NOx eastward, but we find no significant eastward shift in NO2 VCDs compared to NOx emissions in both ACAM and 4 km REAM distributions (Fig. 14). Therefore, we attribute the distribution inconsistency between ACAM and the 4 km REAM to the distribution biases of NEI2011 NOx emissions at the 4 km resolution since the average below-aircraft NO2 VCDs between ACAM and the 4 km REAM are about the same.
It is noteworthy that the number of data points used to calculate grid cell mean NO2 VCDs varies significantly across the domain, as shown in Figs. 14f and S24f. To mitigate potential sampling errors, we only consider the grid cells with ≥ 10 data points on weekdays in Fig. S25. Whether we scale NO2 VCDs using the corresponding domain averages (Fig. S25) or not (not shown), the 4 km REAM generally shows more concentrated NO2 VCDs in Baltimore and Washington, DC, urban regions but more dispersed NO2 VCDs in rural areas than ACAM, consistent with our discussion above. In addition, about 91 % of ACAM NO2 VCD data are measured from 08:00–16:00 LT, and only using ACAM NO2 VCDs between 08:00 and 16:00 LT for the above comparison does not affect our results shown here. Moreover, to minimize the effect of overestimated afternoon vertical mixing (Fig. 8) on the 4 km REAM simulation results, we also examine the comparison between ACAM NO2 VCDs from 09:00–14:00 LT with coincident 4 km REAM results, which produces similar results as shown here. Finally, considering the NOx lifetime difference between morning and noontime, we also analyze the NO2 VCD data between 11:00 and 14:00 LT, and similar results are found.
We also evaluate the NO2 VCD distributions from the 4 km REAM simulation on weekdays and weekends with ACAM NO2 VCDs below the U-12 aircraft obtained from https://www-air.larc.nasa.gov/cgi-bin/ArcView/discover-aq.dc-2011?UC12=1#LIU.XIONG/ (last access: 31 December 2019) in Figs. S26 and S27. Although the domain mean ACAM NO2 VCDs in Figs. S26 and S27 are higher than coincident 4 km REAM results due to the different retrieval method from Lamsal et al. (2017), such as different above-aircraft NO2 VCDs and different a priori NO2 vertical profiles, we can still find clear distribution inconsistencies between the 4 km REAM and ACAM NO2 VCDs. The 4 km REAM NO2 VCDs are more concentrated in the Baltimore and Washington, DC, urban regions than this set of ACAM data, which is consistent with the conclusions derived from the ACAM dataset retrieved by Lamsal et al. (2017).
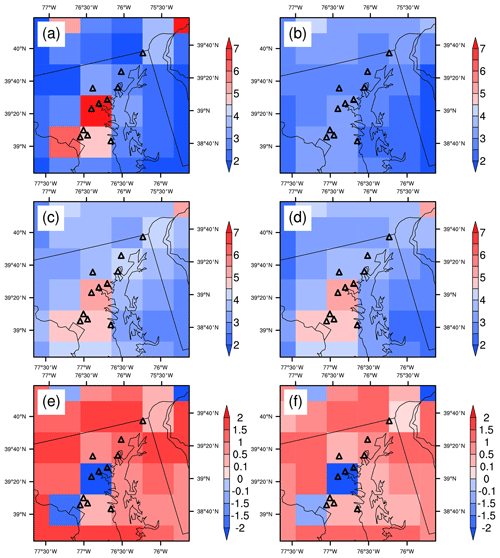
Figure 15Distributions of weekday NO2 TVCDs around the DISCOVER-AQ 2011 region for 13:30 LT in July 2011: (a) the 36 km REAM simulation results, (b) the NASA OMI product (OMNO2), (c) the KNMI OMI product, (d) the retrieved OMI NO2 TVCDs by using the KNMI DOMINO algorithm with corresponding 36 km REAM vertical profiles, (e) the distribution of the NO2 TVCD differences (c minus a) between KNMI OMI and 36 km REAM, and (f) the difference (d minus a) between retrieved OMI NO2 TVCDs and the 36 km REAM results. The NO2 TVCD unit is 1015 molecules cm−2.
The potential distribution bias of the NEI2011 NOx emissions at 36 km resolution is analyzed by comparing the 36 km REAM-simulated NO2 TVCDs with those retrieved by OMI and GOME-2A, as shown in Figs. 15 (OMI, 13:00 LT) and S28 (GOME-2A, 09:30 LT). Both KNMI and our retrievals based on the 36 km REAM NO2 vertical profiles show that OMI and GOME-2A NO2 TVCDs have lower spatial variations than the corresponding 36 km REAM simulation results. OMI and GOME-2A retrievals have lower NO2 TVCDs around the Baltimore and Washington, DC, urban regions and higher values in relatively rural regions than the 36 km REAM. The distribution bias of the 36 km REAM NO2 TVCDs is also identified on weekends through their comparison with OMI and GOME-2A retrievals (not shown). The good agreement between simulated and observed wind suggests that the model horizontal transport error cannot explain such an urban–rural contrast between satellite observations and 36 km REAM simulation results. However, two caveats deserve attention. Firstly, the 36 km REAM cannot resolve urban areas as detailed as the 4 km REAM (Fig. 14), and urban and rural regions may coexist in one 36 km grid cell. Secondly, the OMI and GOME-2A pixels can be much larger than 36 km REAM grid cells, possibly leading to more spatially homogenous distributions of satellite NO2 TVCD data.
3.8 Implications for NOx emissions
The analysis of Sect. 3.7 indicates that the NEI2011 NOx emission distributions at 36 and 4 km resolutions are likely biased for the Baltimore–Washington region. The distribution bias of NOx emission inventories is corroborated by the comparison of the NOx emission inventory derived from the CONsolidated Community Emissions Processor Tool, Motor Vehicle (CONCEPT MV) v2.1 with that estimated by the Sparse Matrix Operator Kernel Emissions (SMOKE) v3.0 model with the Motor Vehicle Emissions Simulator (MOVES) v2010a (DenBleyker et al., 2012). CONCEPT, with finer vehicle activity information as input, produced wider-spread but less-concentrated running exhaust NOx emissions compared to MOVES in the Denver urban area for July 2008 (DenBleyker et al., 2012). In addition, Canty et al. (2015) found that CMAQ 4.7.1, with on-road emissions from MOVES and off-road emissions from the National Mobile Inventory Model (NMIM), overestimated NO2 TVCD over urban regions and underestimated NO2 TVCDs over rural areas in the northeastern US for July and August 2011 compared to the OMNO2 product. The urban–rural contrast was also found in Texas during the 2013 DISCOVER-AQ campaign in the studies of Souri et al. (2016, 2018), implying distribution uncertainties in NOx emissions, although these studies and Canty et al. (2015) focused more on polluted regions with overestimated NOx emissions in their conclusions. The emission distribution bias may also explain why Anderson et al. (2014) have different results from our simulated concentrations in Table 1. In their study, they compared in situ observations with a nested CMAQ simulation with a resolution of 1.33 km. It is difficult to build up a reliable emission inventory for the whole US at very high resolutions with currently available datasets due to the significant inhomogeneity of NOx emissions (Marr et al., 2013), but we can still expect significant improvements in the temporal-spatial distributions of NOx emissions in the near future as GPS-based information starts to be used in the National Emissions Inventory (NEI) estimates (DenBleyker et al., 2017).
Here, we emphasize that our study is not necessarily contradictory to recent studies concerning the overestimation of NEI NOx emissions (Anderson et al., 2014; Canty et al., 2015; McDonald et al., 2018; Souri et al., 2016, 2018; Travis et al., 2016). Different types of observations in different periods and locations are analyzed for various purposes. This study focuses more on the spatial distribution of NOx emissions in NEI2011, while previous studies are concerned more about the NOx emission magnitudes in highly polluted sites, although the spatial distribution issue was also mentioned in some of the studies. If we limit our analyses to those observations in Figs. 7, 10, and 12 and the 4 km REAM, we would also conclude an overestimation of NEI NOx emissions. Considering the significant heterogeneity of NOx emissions, the spatial distribution of NOx emissions is a critical factor in evaluating NOx emissions and improving emission estimation and air quality models, which deserves more attention in future studies, especially when chemical and transport models are moving to higher and higher resolutions.
We investigate the diurnal cycles of surface NO2 concentrations, NO2 vertical profiles, and NO2 TVCDs using REAM model simulations on the basis of the observations from air quality monitoring sites, aircraft, Pandora, OMI, and GOME-2A during the DISCOVER-AQ 2011 campaign. We find that WRF-simulated nighttime kzz-determined MLHs are significantly lower than ELF lidar measurements. Increasing nighttime mixing from 18:00–05:00 LT in the REAM simulations, we significantly improve REAM simulations of nighttime surface NO2 and O3 concentrations.
The REAM simulation reproduces the observed regional mean diurnal cycles of surface NO2 and NOy concentrations, NO2 vertical profiles, and NO2 TVCDs well on weekdays. Observed NO2 concentrations in the boundary layer and TVCDs on weekends are significantly lower than on weekdays. By specifying a weekend-to-weekday NOx emission ratio of and applying a less variable NOx emission diurnal profile on weekends than weekdays, REAM can simulate the weekend observations well. Two issues are also noted. First, Pandora TVCDs show different variations from aircraft-derived and REAM-simulated TVCDs in the early morning and late afternoon, which may be due to the uncertainties in Pandora measurements at large SZAs and the small effective FOV of Pandora. Second, the weekday OMI NO2 TVCDs derived by NASA are somewhat lower than the KNMI OMI product, P-3B-aircraft-derived TVCDs, Pandora, and REAM results; the difference may be caused by the a priori vertical profiles used in the NASA retrieval.
While a higher-resolution simulation is assumed to be superior at a priori, the large observation dataset during DISCOVER-AQ 2011 offers the opportunity of a detailed comparison of 4 and 36 km model simulations. Through the comparison, we find two areas that have not been widely recognized. The first is not using convection parameterization in high-resolution WRF simulations since convection can be resolved explicitly, and most convection parameterizations are not designed for high-resolution simulations. We find that 4 km WRF tends to overestimate boundary layer mixing and vertical transport in the late afternoon, leading to a high model bias in simulated NO2 vertical profiles compared to P-3B aircraft observations. The reasons for this late-afternoon bias in 4 km WRF simulations and model modifications to mitigate this bias need further studies.
A second issue is related to the spatial distribution of NOx emissions in NEI2011. In general, the 4 km simulation results tend to have a high bias relative to the 36 km results on the regional mean observations. However, for individual sites, relative to the 36 km model simulations, the 4 km model results can show larger, smaller, or similar biases compared to the observations depending upon observation location. Based on process diagnostics and analyses, we find that the bias discrepancies between the 36 and 4 km REAM simulations are mainly attributed to their different NOx emissions and their spatial gradients at different sites. The comparison of 4 km ACAM NO2 VCD measurements from the UC-12 aircraft with coincident 4 km REAM results shows that 4 km REAM NO2 VCDs are more concentrated in urban regions than the ACAM observations. OMI and GOME-2A data also show less spatially varying NO2 TVCD distributions with lower NO2 TVCDs around the Baltimore–Washington urban regions and higher TVCDs in surrounding rural areas than corresponding 36 km REAM simulation results. Further model analysis indicates that the 36 and 4 km VCD discrepancies are due primarily to the distribution bias of NEI2011 NOx emissions at 36 and 4 km resolutions. Our results highlight the research need to improve the methodologies and datasets to improve the spatial distributions in emission estimates.
The DISCOVER-AQ 2011 campaign datasets are archived on https://doi.org/10.5067/AIRCRAFT/DISCOVER-AQ/AEROSOL-TRACEGAS (NASA/LARC/SD/ASDC, 2014). EPA air quality monitoring datasets are from https://aqs.epa.gov/aqsweb/airdata/download_files.html (last access: 23 June 2015, EPA, 2021). The NASA OMI NO2 product is from https://doi.org/10.5067/Aura/OMI/DATA2017 (Krotkov et al., 2019). The KNMI OMI NO2 product is from https://www.temis.nl/airpollution/no2.php (last access: 14 January 2015, KNMI/ESA, 2004). We obtain the KNMI GOME-2A NO2 VCD archives from http://www.temis.nl/airpollution/no2col/no2colgome2_v2.php (last access: 22 January 2015, KNMI/BIRA-IASB, 2011). The GMI MERRA-2 simulation results are from https://portal.nccs.nasa.gov/datashare/dirac/gmidata2/users/mrdamon/Hindcast-Family/HindcastMR2/2011/stations/ (last access: 14 May 2019, NASA, 2017). We obtain the UC-12 ACAM NO2 VCD product by Xiong Liu from https://www-air.larc.nasa.gov/cgi-bin/ArcView/discover-aq.dc-2011?UC12=1#LIU.XIONG/ (last access: 31 December 2019, Liu, 2019). The Stage IV precipitation data are downloaded from https://doi.org/10.5065/D69Z93M3 (CODIAC et al., 2000). The NCEP CFSv2 6-hourly product is available at https://doi.org/10.5065/D61C1TXF (Saha et al., 2011). REAM simulation results for this study and the UC-12 ACAM NO2 VCD product by Lamsal et al. (2017, https://doi.org/10.1002/2016JD025483) are available upon request.
The supplement related to this article is available online at: https://doi.org/10.5194/acp-21-11133-2021-supplement.
JL and YW designed the study. JL, RZ, and CS updated the REAM model. JL conducted model simulations. KFB developed the DOMINO algorithm, CS applied the algorithm to REAM vertical profiles, and JL updated the retrieval algorithm and did the retrieval by using REAM NO2 vertical profiles. AW, JH, EAC, RWL, JJS, RD, AMT, TNK, LNL, SJJ, MGK, XL, and CRN made various measurements in the DISCOVER-AQ 2011 campaign. JL conducted the analyses with discussions with YW, RZ, CS, AW, JH, KFB, EAC, RWL, JJS, RD, AMT, TNK, LNL, SJJ, MGK, XL, and CRN. JL and YW led the writing of the manuscript with input from all other coauthors. All coauthors reviewed the manuscript.
The authors declare that they have no conflict of interest.
Publisher's note: Copernicus Publications remains neutral with regard to jurisdictional claims in published maps and institutional affiliations.
This work was supported by the NASA ACMAP program. We thank Chun Zhao for providing us the PNNL NEI2011 emission inventory. We thank Yuzhong Zhang and Jenny Fisher for providing the updated GEOS-Chem chemistry mechanism files and thank Yuzhong Zhang, Yongjia Song, Hang Qu, Ye Cheng, Aoxing Zhang, Yufei Zou, and Ziming Ke for discussion with Jianfeng Li. We thank Susan Strahan for providing the GMI outputs download link.
This research has been supported by the National Aeronautics and Space Administration (ACMAP program).
This paper was edited by Yugo Kanaya and reviewed by two anonymous referees.
Anderson, D. C., Loughner, C. P., Diskin, G., Weinheimer, A., Canty, T. P., Salawitch, R. J., Worden, H. M., Fried, A., Mikoviny, T., and Wisthaler, A.: Measured and modeled CO and NOy in DISCOVER-AQ: An evaluation of emissions and chemistry over the eastern US, Atmos. Environ., 96, 78–87, https://doi.org/10.1016/j.atmosenv.2014.07.004, 2014.
Beirle, S., Platt, U., Wenig, M., and Wagner, T.: Weekly cycle of NO2 by GOME measurements: a signature of anthropogenic sources, Atmos. Chem. Phys., 3, 2225–2232, https://doi.org/10.5194/acp-3-2225-2003, 2003.
Boersma, K. F., Eskes, H. J., Veefkind, J. P., Brinksma, E. J., van der A, R. J., Sneep, M., van den Oord, G. H. J., Levelt, P. F., Stammes, P., Gleason, J. F., and Bucsela, E. J.: Near-real time retrieval of tropospheric NO2 from OMI, Atmos. Chem. Phys., 7, 2103–2118, https://doi.org/10.5194/acp-7-2103-2007, 2007.
Boersma, K. F., Jacob, D. J., Eskes, H. J., Pinder, R. W., Wang, J., and Van Der A, R. J.: Intercomparison of SCIAMACHY and OMI tropospheric NO2 columns: Observing the diurnal evolution of chemistry and emissions from space, J. Geophys. Res.-Atmos., 113, D16S26, https://doi.org/10.1029/2007JD008816, 2008.
Boersma, K. F., Jacob, D. J., Trainic, M., Rudich, Y., DeSmedt, I., Dirksen, R., and Eskes, H. J.: Validation of urban NO2 concentrations and their diurnal and seasonal variations observed from the SCIAMACHY and OMI sensors using in situ surface measurements in Israeli cities, Atmos. Chem. Phys., 9, 3867–3879, https://doi.org/10.5194/acp-9-3867-2009, 2009.
Boersma, K. F., Eskes, H. J., Dirksen, R. J., van der A, R. J., Veefkind, J. P., Stammes, P., Huijnen, V., Kleipool, Q. L., Sneep, M., Claas, J., Leitão, J., Richter, A., Zhou, Y., and Brunner, D.: An improved tropospheric NO2 column retrieval algorithm for the Ozone Monitoring Instrument, Atmos. Meas. Tech., 4, 1905–1928, https://doi.org/10.5194/amt-4-1905-2011, 2011.
Boersma, K. F., Eskes, H. J., Richter, A., De Smedt, I., Lorente, A., Beirle, S., van Geffen, J. H. G. M., Zara, M., Peters, E., Van Roozendael, M., Wagner, T., Maasakkers, J. D., van der A, R. J., Nightingale, J., De Rudder, A., Irie, H., Pinardi, G., Lambert, J.-C., and Compernolle, S. C.: Improving algorithms and uncertainty estimates for satellite NO2 retrievals: results from the quality assurance for the essential climate variables (QA4ECV) project, Atmos. Meas. Tech., 11, 6651–6678, https://doi.org/10.5194/amt-11-6651-2018, 2018.
Breuer, H., Ács, F., Horváth, Á., Németh, P., and Rajkai, K.: Diurnal course analysis of the WRF-simulated and observation-based planetary boundary layer height, Adv. Sci. Res., 11, 83–88, https://doi.org/10.5194/asr-11-83-2014, 2014.
Brohede, S., McLinden, C. A., Berthet, G., Haley, C. S., Murtagh, D., and Sioris, C. E.: A stratospheric NO2 climatology from Odin/OSIRIS limb-scatter measurements, Can. J. Phys., 85, 1253–1274, https://doi.org/10.1139/p07-141, 2007.
Brown, S. S., Dibb, J. E., Stark, H., Aldener, M., Vozella, M., Whitlow, S., Williams, E. J., Lerner, B. M., Jakoubek, R., and Middlebrook, A. M.: Nighttime removal of NOx in the summer marine boundary layer, Geophys. Res. Lett., 31, L07108, https://doi.org/10.1029/2004GL019412, 2004.
Bucsela, E. J., Krotkov, N. A., Celarier, E. A., Lamsal, L. N., Swartz, W. H., Bhartia, P. K., Boersma, K. F., Veefkind, J. P., Gleason, J. F., and Pickering, K. E.: A new stratospheric and tropospheric NO2 retrieval algorithm for nadir-viewing satellite instruments: applications to OMI, Atmos. Meas. Tech., 6, 2607–2626, https://doi.org/10.5194/amt-6-2607-2013, 2013.
Canty, T. P., Hembeck, L., Vinciguerra, T. P., Anderson, D. C., Goldberg, D. L., Carpenter, S. F., Allen, D. J., Loughner, C. P., Salawitch, R. J., and Dickerson, R. R.: Ozone and NOx chemistry in the eastern US: evaluation of CMAQ/CB05 with satellite (OMI) data, Atmos. Chem. Phys., 15, 10965–10982, https://doi.org/10.5194/acp-15-10965-2015, 2015.
Chance, K.: OMI Algorithm Theoretical Basis Document: OMI Trace Gas Algorithms, Smithsonian Astrophysical Observatory, Cambridge, MA, USA2.0, 78 pp., available at: https://ozoneaq.gsfc.nasa.gov/media/docs/ATBD-OMI-04.pdf (last access: 31 May 2017), 2002.
Cheng, Y., Wang, Y., Zhang, Y., Chen, G., Crawford, J. H., Kleb, M. M., Diskin, G. S., and Weinheimer, A. J.: Large biogenic contribution to boundary layer O3-CO regression slope in summer, Geophys. Res. Lett., 44, 7061–7068, https://doi.org/10.1002/2017GL074405, 2017.
Cheng, Y., Wang, Y., Zhang, Y., Crawford, J. H., Diskin, G. S., Weinheimer, A. J., and Fried, A.: Estimator of surface ozone using formaldehyde and carbon monoxide concentrations over the eastern United States in summer, J. Geophys. Res.-Atmos., 123, 7642–7655, https://doi.org/10.1029/2018JD028452, 2018.
Choi, S., Lamsal, L. N., Follette-Cook, M., Joiner, J., Krotkov, N. A., Swartz, W. H., Pickering, K. E., Loughner, C. P., Appel, W., Pfister, G., Saide, P. E., Cohen, R. C., Weinheimer, A. J., and Herman, J. R.: Assessment of NO2 observations during DISCOVER-AQ and KORUS-AQ field campaigns, Atmos. Meas. Tech., 13, 2523–2546, https://doi.org/10.5194/amt-13-2523-2020, 2020.
Choi, Y., Wang, Y., Zeng, T., Cunnold, D., Yang, E. S., Martin, R., Chance, K., Thouret, V., and Edgerton, E.: Springtime transitions of NO2, CO, and O3 over North America: Model evaluation and analysis, J. Geophys. Res., 113, D20311, https://doi.org/10.1029/2007JD009632, 2008.
Choi, Y., Kim, H., Tong, D., and Lee, P.: Summertime weekly cycles of observed and modeled NOx and O3 concentrations as a function of satellite-derived ozone production sensitivity and land use types over the Continental United States, Atmos. Chem. Phys., 12, 6291–6307, https://doi.org/10.5194/acp-12-6291-2012, 2012.
CODIAC, EOL, NCAR, UCAR, CPC, NCEP, NWS, NOAA, and DOC: NCEP/CPC Four Kilometer Precipitation Set, Gauge and Radar, Research Data Archive at the National Center for Atmospheric Research, Computational and Information Systems Laboratory [data set], Boulder, CO, USA, https://doi.org/10.5065/D69Z93M3, 2000.
Compton, J. C., Delgado, R., Berkoff, T. A., and Hoff, R. M.: Determination of planetary boundary layer height on short spatial and temporal scales: A demonstration of the covariance wavelet transform in ground-based wind profiler and lidar measurements, J. Atmos. Ocean. Tech., 30, 1566–1575, https://doi.org/10.1175/JTECH-D-12-00116.1, 2013.
David, L. M. and Nair, P. R.: Diurnal and seasonal variability of surface ozone and NOx at a tropical coastal site: Association with mesoscale and synoptic meteorological conditions, J. Geophys. Res., 116, D10303, https://doi.org/10.1029/2010JD015076, 2011.
Davis, C., Brown, B., and Bullock, R.: Object-based verification of precipitation forecasts. Part I: Methodology and application to mesoscale rain areas, Mon. Weather Rev., 134, 1772–1784, https://doi.org/10.1175/MWR3145.1, 2006.
Day, D. A., Wooldridge, P. J., Dillon, M. B., Thornton, J. A., and Cohen, R. C.: A thermal dissociation laser-induced fluorescence instrument for in situ detection of NO2, peroxy nitrates, alkyl nitrates, and HNO3, J. Geophys. Res.-Atmos., 107, ACH 4-1–ACH 4-14, https://doi.org/10.1029/2001JD000779, 2002.
de Foy, B.: City-level variations in NOx emissions derived from hourly monitoring data in Chicago, Atmos. Environ., 176, 128–139, https://doi.org/10.1016/j.atmosenv.2017.12.028, 2018.
DenBleyker, A., Morris, R. E., Lindhjem, C. E., Parker, L. K., Shah, T., Koo, B., Loomis, C., and Dilly, J.: Temporal and Spatial Detail in Mobile Source Emission Inventories for Regional Air Quality Modeling, 2012 International Emission Inventory Conference, Florida, US, 13–16 August 2012, available at: https://gaftp.epa.gov/Air/nei/ei_conference/EI20/session1/adenbleyker.pdf (last access: 27 January 2019), 2012.
DenBleyker, A., Koupal, J., DeFries, T., and Palacios, C.: Improvement of Default Inputs for MOVES and SMOKE-MOVES, Final Report, CRC Project A-100, Eastern Research Group, Inc., Austin, TX, 86, available at: http://crcsite.wpengine.com/wp-content/uploads/2019/05/ERG_FinalReport_CRCA100_28Feb2017.pdf (last access: 19 July 2018), 2017.
Dirksen, R. J., Boersma, K. F., Eskes, H. J., Ionov, D. V., Bucsela, E. J., Levelt, P. F., and Kelder, H. M.: Evaluation of stratospheric NO2 retrieved from the Ozone Monitoring Instrument: Intercomparison, diurnal cycle, and trending, J. Geophys. Res., 116, D08305, https://doi.org/10.1029/2010JD014943, 2011.
EPA: Profile of the 2011 National Air Emissions Inventory, U.S. Environmental Protection Agency, available at https://www.epa.gov/sites/production/files/2015-08/documents/lite_finalversion_ver10.pdf (last access: 13 May 2019), 2014.
EPA: Air Data: Pre-Generated Data Files, U.S. Environmental Protection Agency [data set], available at: https://aqs.epa.gov/aqsweb/airdata/download_files.html (last access: 23 June 2015), 2021.
Fisher, J. A., Jacob, D. J., Travis, K. R., Kim, P. S., Marais, E. A., Chan Miller, C., Yu, K., Zhu, L., Yantosca, R. M., Sulprizio, M. P., Mao, J., Wennberg, P. O., Crounse, J. D., Teng, A. P., Nguyen, T. B., St. Clair, J. M., Cohen, R. C., Romer, P., Nault, B. A., Wooldridge, P. J., Jimenez, J. L., Campuzano-Jost, P., Day, D. A., Hu, W., Shepson, P. B., Xiong, F., Blake, D. R., Goldstein, A. H., Misztal, P. K., Hanisco, T. F., Wolfe, G. M., Ryerson, T. B., Wisthaler, A., and Mikoviny, T.: Organic nitrate chemistry and its implications for nitrogen budgets in an isoprene- and monoterpene-rich atmosphere: constraints from aircraft (SEAC4RS) and ground-based (SOAS) observations in the Southeast US, Atmos. Chem. Phys., 16, 5969–5991, https://doi.org/10.5194/acp-16-5969-2016, 2016.
Flynn, C. M., Pickering, K. E., Crawford, J. H., Lamsal, L., Krotkov, N., Herman, J., Weinheimer, A., Chen, G., Liu, X., and Szykman, J.: Relationship between column-density and surface mixing ratio: Statistical analysis of O3 and NO2 data from the July 2011 Maryland DISCOVER-AQ mission, Atmos. Environ., 92, 429–441, https://doi.org/10.1016/j.atmosenv.2014.04.041, 2014.
Frey, M. M., Brough, N., France, J. L., Anderson, P. S., Traulle, O., King, M. D., Jones, A. E., Wolff, E. W., and Savarino, J.: The diurnal variability of atmospheric nitrogen oxides (NO and NO2) above the Antarctic Plateau driven by atmospheric stability and snow emissions, Atmos. Chem. Phys., 13, 3045–3062, https://doi.org/10.5194/acp-13-3045-2013, 2013.
Gaur, A., Tripathi, S. N., Kanawade, V. P., Tare, V., and Shukla, S. P.: Four-year measurements of trace gases (SO2, NOx, CO, and O3) at an urban location, Kanpur, in Northern India, J. Atmos. Chem., 71, 283–301, https://doi.org/10.1007/s10874-014-9295-8, 2014.
Gourley, J. J., Hong, Y., Flamig, Z. L., Wang, J., Vergara, H., and Anagnostou, E. N.: Hydrologic evaluation of rainfall estimates from radar, satellite, gauge, and combinations on Ft. Cobb basin, Oklahoma, J. Hydrometeorol., 12, 973–988, https://doi.org/10.1175/2011JHM1287.1, 2011.
Guenther, A. B., Jiang, X., Heald, C. L., Sakulyanontvittaya, T., Duhl, T., Emmons, L. K., and Wang, X.: The Model of Emissions of Gases and Aerosols from Nature version 2.1 (MEGAN2.1): an extended and updated framework for modeling biogenic emissions, Geosci. Model Dev., 5, 1471–1492, https://doi.org/10.5194/gmd-5-1471-2012, 2012.
Hains, J. C., Boersma, K. F., Kroon, M., Dirksen, R. J., Cohen, R. C., Perring, A. E., Bucsela, E., Volten, H., Swart, D. P. J., and Richter, A.: Testing and improving OMI DOMINO tropospheric NO2 using observations from the DANDELIONS and INTEX-B validation campaigns, J. Geophys. Res., 115, D05301, https://doi.org/10.1029/2009JD012399, 2010.
Herman, J., Cede, A., Spinei, E., Mount, G., Tzortziou, M., and Abuhassan, N.: NO2 column amounts from ground-based Pandora and MFDOAS spectrometers using the direct-Sun DOAS technique: Intercomparisons and application to OMI validation, J. Geophys. Res., 114, D13307, https://doi.org/10.1029/2009JD011848, 2009.
Herman, J., Spinei, E., Fried, A., Kim, J., Kim, J., Kim, W., Cede, A., Abuhassan, N., and Segal-Rozenhaimer, M.: NO2 and HCHO measurements in Korea from 2012 to 2016 from Pandora spectrometer instruments compared with OMI retrievals and with aircraft measurements during the KORUS-AQ campaign, Atmos. Meas. Tech., 11, 4583–4603, https://doi.org/10.5194/amt-11-4583-2018, 2018.
Herman, J., Abuhassan, N., Kim, J., Kim, J., Dubey, M., Raponi, M., and Tzortziou, M.: Underestimation of column NO2 amounts from the OMI satellite compared to diurnally varying ground-based retrievals from multiple PANDORA spectrometer instruments, Atmos. Meas. Tech., 12, 5593–5612, https://doi.org/10.5194/amt-12-5593-2019, 2019.
Hong, S.-Y., Noh, Y., and Dudhia, J.: A new vertical diffusion package with an explicit treatment of entrainment processes, Mon. Weather Rev., 134, 2318–2341, https://doi.org/10.1175/MWR3199.1, 2006.
Hu, X., Doughty, D. C., Sanchez, K. J., Joseph, E., and Fuentes, J. D.: Ozone variability in the atmospheric boundary layer in Maryland and its implications for vertical transport model, Atmos. Environ., 46, 354–364, https://doi.org/10.1016/j.atmosenv.2011.09.054, 2012.
Huijnen, V., Eskes, H. J., Poupkou, A., Elbern, H., Boersma, K. F., Foret, G., Sofiev, M., Valdebenito, A., Flemming, J., Stein, O., Gross, A., Robertson, L., D'Isidoro, M., Kioutsioukis, I., Friese, E., Amstrup, B., Bergstrom, R., Strunk, A., Vira, J., Zyryanov, D., Maurizi, A., Melas, D., Peuch, V.-H., and Zerefos, C.: Comparison of OMI NO2 tropospheric columns with an ensemble of global and European regional air quality models, Atmos. Chem. Phys., 10, 3273–3296, https://doi.org/10.5194/acp-10-3273-2010, 2010.
Ionov, D. V., Timofeyev, Y. M., Sinyakov, V. P., Semenov, V. K., Goutail, F., Pommereau, J. P., Bucsela, E. J., Celarier, E. A., and Kroon, M.: Ground-based validation of EOS-Aura OMI NO2 vertical column data in the midlatitude mountain ranges of Tien Shan (Kyrgyzstan) and Alps (France), J. Geophys. Res., 113, D15S08, https://doi.org/10.1029/2007JD008659, 2008.
Irie, H., Kanaya, Y., Akimoto, H., Tanimoto, H., Wang, Z., Gleason, J. F., and Bucsela, E. J.: Validation of OMI tropospheric NO2 column data using MAX-DOAS measurements deep inside the North China Plain in June 2006: Mount Tai Experiment 2006, Atmos. Chem. Phys., 8, 6577–6586, https://doi.org/10.5194/acp-8-6577-2008, 2008.
Irie, H., Boersma, K. F., Kanaya, Y., Takashima, H., Pan, X., and Wang, Z. F.: Quantitative bias estimates for tropospheric NO2 columns retrieved from SCIAMACHY, OMI, and GOME-2 using a common standard for East Asia, Atmos. Meas. Tech., 5, 2403–2411, https://doi.org/10.5194/amt-5-2403-2012, 2012.
Jones, A. E., Weller, R., Wolff, E. W., and Jacobi, H. W.: Speciation and rate of photochemical NO and NO2 production in Antarctic snow, Geophys. Res. Lett., 27, 345–348, https://doi.org/10.1029/1999GL010885, 2000.
Judd, L. M., Al-Saadi, J. A., Valin, L. C., Pierce, R. B., Yang, K., Janz, S. J., Kowalewski, M. G., Szykman, J. J., Tiefengraber, M., and Mueller, M.: The Dawn of Geostationary Air Quality Monitoring: Case Studies from Seoul and Los Angeles, Front. Environ. Sci., 6, 85, https://doi.org/10.3389/fenvs.2018.00085, 2018.
Judd, L. M., Al-Saadi, J. A., Janz, S. J., Kowalewski, M. G., Pierce, R. B., Szykman, J. J., Valin, L. C., Swap, R., Cede, A., Mueller, M., Tiefengraber, M., Abuhassan, N., and Williams, D.: Evaluating the impact of spatial resolution on tropospheric NO2 column comparisons within urban areas using high-resolution airborne data, Atmos. Meas. Tech., 12, 6091–6111, https://doi.org/10.5194/amt-12-6091-2019, 2019.
Judd, L. M., Al-Saadi, J. A., Szykman, J. J., Valin, L. C., Janz, S. J., Kowalewski, M. G., Eskes, H. J., Veefkind, J. P., Cede, A., Mueller, M., Gebetsberger, M., Swap, R., Pierce, R. B., Nowlan, C. R., Abad, G. G., Nehrir, A., and Williams, D.: Evaluating Sentinel-5P TROPOMI tropospheric NO2 column densities with airborne and Pandora spectrometers near New York City and Long Island Sound, Atmos. Meas. Tech., 13, 6113–6140, https://doi.org/10.5194/amt-13-6113-2020, 2020.
Kalinga, O. A. and Gan, T. Y.: Estimation of rainfall from infrared-microwave satellite data for basin-scale hydrologic modelling, Hydrol. Process., 24, 2068–2086, https://doi.org/10.1002/hyp.7626, 2010.
Kaynak, B., Hu, Y., Martin, R. V., Sioris, C. E., and Russell, A. G.: Comparison of weekly cycle of NO2 satellite retrievals and NOx emission inventories for the continental United States, J. Geophys. Res., 114, D05302, https://doi.org/10.1029/2008JD010714, 2009.
Kim, S. W., McDonald, B., Baidar, S., Brown, S., Dube, B., Ferrare, R., Frost, G., Harley, R., Holloway, J., and Lee, H. J.: Modeling the weekly cycle of NOx and CO emissions and their impacts on O3 in the Los Angeles-South Coast Air Basin during the CalNex 2010 field campaign, J. Geophys. Res.-Atmos., 121, 1340–1360, https://doi.org/10.1002/2015JD024292, 2016.
Knepp, T., Pippin, M., Crawford, J., Chen, G., Szykman, J., Long, R., Cowen, L., Cede, A., Abuhassan, N., and Herman, J.: Estimating surface NO2 and SO2 mixing ratios from fast-response total column observations and potential application to geostationary missions, J. Atmos. Chem., 72, 261–286, https://doi.org/10.1007/s10874-013-9257-6, 2015.
Knepp, T. N., Szykman, J. J., Long, R., Duvall, R. M., Krug, J., Beaver, M., Cavender, K., Kronmiller, K., Wheeler, M., Delgado, R., Hoff, R., Berkoff, T., Olson, E., Clark, R., Wolfe, D., Van Gilst, D., and Neil, D.: Assessment of mixed-layer height estimation from single-wavelength ceilometer profiles, Atmos. Meas. Tech., 10, 3963–3983, https://doi.org/10.5194/amt-10-3963-2017, 2017.
KNMI/BIRA-IASB: Total and Tropospheric NO2 columns from GOME2 (METOP-A), TEMIS [data set], available at: http://www.temis.nl/airpollution/no2col/no2colgome2_v2.php (last access: 22 January 2015), 2011.
KNMI/ESA: Tropospheric NO2 from satellites, TEMIS [data set], available at http://www.temis.nl/airpollution/no2.php (last access: 14 January 2015), 2004.
Kollonige, D. E., Thompson, A. M., Josipovic, M., Tzortziou, M., Beukes, J. P., Burger, R., Martins, D. K., van Zyl, P. G., Vakkari, V., and Laakso, L.: OMI Satellite and Ground-Based Pandora Observations and Their Application to Surface NO2 Estimations at Terrestrial and Marine Sites, J. Geophys. Res.-Atmos., 123, 1441–1459, https://doi.org/10.1002/2017JD026518, 2018.
Krotkov, N. A., Lamsal, L. N., Celarier, E. A., Swartz, W. H., Marchenko, S. V., Bucsela, E. J., Chan, K. L., Wenig, M., and Zara, M.: The version 3 OMI NO2 standard product, Atmos. Meas. Tech., 10, 3133–3149, https://doi.org/10.5194/amt-10-3133-2017, 2017.
Krotkov, N. A., Lamsal, L. N., Marchenko, S. V., Bucsela, E. J., Swartz, W. H., Joiner, J., and the OMI core team: OMI/Aura Nitrogen Dioxide (NO2) Total and Tropospheric Column 1-orbit L2 Swath 13x24 km V003, Goddard Earth Sciences Data and Information Services Center (GES DISC) [data set], Greenbelt, MD, USA, https://doi.org/10.5067/Aura/OMI/DATA2017, 2019.
Lamsal, L. N., Krotkov, N. A., Celarier, E. A., Swartz, W. H., Pickering, K. E., Bucsela, E. J., Gleason, J. F., Martin, R. V., Philip, S., Irie, H., Cede, A., Herman, J., Weinheimer, A., Szykman, J. J., and Knepp, T. N.: Evaluation of OMI operational standard NO2 column retrievals using in situ and surface-based NO2 observations, Atmos. Chem. Phys., 14, 11587–11609, https://doi.org/10.5194/acp-14-11587-2014, 2014.
Lamsal, L. N., Duncan, B. N., Yoshida, Y., Krotkov, N. A., Pickering, K. E., Streets, D. G., and Lu, Z.: US NO2 trends (2005–2013): EPA Air Quality System (AQS) data versus improved observations from the Ozone Monitoring Instrument (OMI), Atmos. Environ., 110, 130–143, https://doi.org/10.1016/j.atmosenv.2015.03.055, 2015.
Lamsal, L. N., Janz, S. J., Krotkov, N. A., Pickering, K. E., Spurr, R. J. D., Kowalewski, M. G., Loughner, C. P., Crawford, J. H., Swartz, W. H., and Herman, J.: High-resolution NO2 observations from the Airborne Compact Atmospheric Mapper: Retrieval and validation, J. Geophys. Res.-Atmos., 122, 1953–1970, https://doi.org/10.1002/2016JD025483, 2017.
Lamsal, L. N., Krotkov, N. A., Vasilkov, A., Marchenko, S., Qin, W., Yang, E.-S., Fasnacht, Z., Joiner, J., Choi, S., Haffner, D., Swartz, W. H., Fisher, B., and Bucsela, E.: Ozone Monitoring Instrument (OMI) Aura nitrogen dioxide standard product version 4.0 with improved surface and cloud treatments, Atmos. Meas. Tech., 14, 455–479, https://doi.org/10.5194/amt-14-455-2021, 2021.
Levelt, P. F., Hilsenrath, E., Leppelmeier, G. W., van den Oord, G. H. J., Bhartia, P. K., Tamminen, J., de Haan, J. F., and Veefkind, J. P.: Science objectives of the ozone monitoring instrument, IEEE T. Geosci. Remote, 44, 1199–1208, https://doi.org/10.1109/TGRS.2006.872336, 2006.
Li, J., Wang, Y., and Qu, H.: Dependence of summertime surface ozone on NOx and VOC emissions over the United States: Peak time and value, Geophys. Res. Lett., 46, 3540–3550, https://doi.org/10.1029/2018GL081823, 2019.
Lin, Y. and Mitchell, K. E.: the NCEP stage II/IV hourly precipitation analyses: Development and applications, 19th Conf. Hydrology, American Meteorological Society, San Diego, CA, USA, 10–13 January 2005, available at: https://ams.confex.com/ams/pdfpapers/83847.pdf (last access: 30 March 2020), 2005.
Liu, C., Liu, X., Kowalewski, M., Janz, S., González Abad, G., Pickering, K., Chance, K., and Lamsal, L.: Analysis of ACAM data for trace gas retrievals during the 2011 DISCOVER-AQ campaign, J. Spectrosco., 2015, 827160, https://doi.org/10.1155/2015/827160, 2015a.
Liu, C., Liu, X., Kowalewski, M. G., Janz, S. J., González Abad, G., Pickering, K. E., Chance, K., and Lamsal, L. N.: Characterization and verification of ACAM slit functions for trace-gas retrievals during the 2011 DISCOVER-AQ flight campaign, Atmos. Meas. Tech., 8, 751–759, https://doi.org/10.5194/amt-8-751-2015, 2015b.
Liu, X.: ACAM O3, NO2 and HCHO columns from SAO retrieval algorithm, NASA Langley Atmospheric Science Data Center DAAC [data set], available at: https://www-air.larc.nasa.gov/cgi-bin/ArcView/discover-aq.dc-2011?UC12=1#LIU.XIONG/, last access: 31 December 2019.
Liu, Z., Wang, Y., Gu, D., Zhao, C., Huey, L. G., Stickel, R., Liao, J., Shao, M., Zhu, T., Zeng, L., Amoroso, A., Costabile, F., Chang, C.-C., and Liu, S.-C.: Summertime photochemistry during CAREBeijing-2007: ROx budgets and O3 formation, Atmos. Chem. Phys., 12, 7737–7752, https://doi.org/10.5194/acp-12-7737-2012, 2012.
Lopez, P.: Direct 4D-Var assimilation of NCEP stage IV radar and gauge precipitation data at ECMWF, Mon. Weather Rev., 139, 2098–2116, https://doi.org/10.1175/2010MWR3565.1, 2011.
Luo, G., Yu, F., and Schwab, J.: Revised treatment of wet scavenging processes dramatically improves GEOS-Chem 12.0.0 simulations of surface nitric acid, nitrate, and ammonium over the United States, Geosci. Model Dev., 12, 3439–3447, https://doi.org/10.5194/gmd-12-3439-2019, 2019.
Marchenko, S., Krotkov, N., Lamsal, L., Celarier, E., Swartz, W., and Bucsela, E.: Revising the slant column density retrieval of nitrogen dioxide observed by the Ozone Monitoring Instrument, J. Geophys. Res.-Atmos., 120, 5670–5692, https://doi.org/10.1002/2014JD022913, 2015.
Marr, L. C., Moore, T. O., Klapmeyer, M. E., and Killar, M. B.: Comparison of NOx Fluxes Measured by Eddy Covariance to Emission Inventories and Land Use, Environ. Sci. Technol., 47, 1800–1808, https://doi.org/10.1021/es303150y, 2013.
McDonald, B., McKeen, S., Cui, Y. Y., Ahmadov, R., Kim, S.-W., Frost, G. J., Pollack, I., Peischl, J., Ryerson, T. B., and Holloway, J.: Modeling Ozone in the Eastern US using a Fuel-Based Mobile Source Emissions Inventory, Environ. Sci. Technol., 52, 7360–7370, https://doi.org/10.1021/acs.est.8b00778, 2018.
Munro, R., Eisinger, M., Anderson, C., Callies, J., Corpaccioli, E., Lang, R., Lefebvre, A., Livschitz, Y., and Albinana, A. P.: GOME-2 on MetOp, Proc. of The 2006 EUMETSAT Meteorological Satellite Conference, Helsinki, Finland, 12–16 June 2006, 1216, p. 48, 2006.
NASA: NCCS Dataportal – Datashare, NASA Center for Climate Simulation [data set], available at: https://portal.nccs.nasa.gov/datashare/dirac/gmidata2/users/mrdamon/Hindcast-Family/HindcastMR2/2011/stations/ (last access: 14 May 2019), 2017.
NASA/LARC/SD/ASDC: DISCOVER-AQ P-3B Aircraft in-situ Trace Gas Measurements, NASA Langley Atmospheric Science Data Center DAAC [data set], https://doi.org/10.5067/AIRCRAFT/DISCOVER-AQ/AEROSOL-TRACEGAS, 2014.
Nelson, B. R., Prat, O. P., Seo, D.-J., and Habib, E.: Assessment and implications of NCEP Stage IV quantitative precipitation estimates for product intercomparisons, Weather Forecast., 31, 371–394, https://doi.org/10.1175/WAF-D-14-00112.1, 2016.
Ng, N. L., Brown, S. S., Archibald, A. T., Atlas, E., Cohen, R. C., Crowley, J. N., Day, D. A., Donahue, N. M., Fry, J. L., Fuchs, H., Griffin, R. J., Guzman, M. I., Herrmann, H., Hodzic, A., Iinuma, Y., Jimenez, J. L., Kiendler-Scharr, A., Lee, B. H., Luecken, D. J., Mao, J., McLaren, R., Mutzel, A., Osthoff, H. D., Ouyang, B., Picquet-Varrault, B., Platt, U., Pye, H. O. T., Rudich, Y., Schwantes, R. H., Shiraiwa, M., Stutz, J., Thornton, J. A., Tilgner, A., Williams, B. J., and Zaveri, R. A.: Nitrate radicals and biogenic volatile organic compounds: oxidation, mechanisms, and organic aerosol, Atmos. Chem. Phys., 17, 2103–2162, https://doi.org/10.5194/acp-17-2103-2017, 2017.
Nowlan, C. R., Liu, X., Leitch, J. W., Chance, K., González Abad, G., Liu, C., Zoogman, P., Cole, J., Delker, T., Good, W., Murcray, F., Ruppert, L., Soo, D., Follette-Cook, M. B., Janz, S. J., Kowalewski, M. G., Loughner, C. P., Pickering, K. E., Herman, J. R., Beaver, M. R., Long, R. W., Szykman, J. J., Judd, L. M., Kelley, P., Luke, W. T., Ren, X., and Al-Saadi, J. A.: Nitrogen dioxide observations from the Geostationary Trace gas and Aerosol Sensor Optimization (GeoTASO) airborne instrument: Retrieval algorithm and measurements during DISCOVER-AQ Texas 2013, Atmos. Meas. Tech., 9, 2647–2668, https://doi.org/10.5194/amt-9-2647-2016, 2016.
Nowlan, C. R., Liu, X., Janz, S. J., Kowalewski, M. G., Chance, K., Follette-Cook, M. B., Fried, A., González Abad, G., Herman, J. R., Judd, L. M., Kwon, H.-A., Loughner, C. P., Pickering, K. E., Richter, D., Spinei, E., Walega, J., Weibring, P., and Weinheimer, A. J.: Nitrogen dioxide and formaldehyde measurements from the GEOstationary Coastal and Air Pollution Events (GEO-CAPE) Airborne Simulator over Houston, Texas, Atmos. Meas. Tech., 11, 5941–5964, https://doi.org/10.5194/amt-11-5941-2018, 2018.
Oetjen, H., Baidar, S., Krotkov, N. A., Lamsal, L. N., Lechner, M., and Volkamer, R.: Airborne MAX-DOAS measurements over California: Testing the NASA OMI tropospheric NO2 product, J. Geophys. Res.-Atmos., 118, 7400–7413, https://doi.org/10.1002/jgrd.50550, 2013.
Peng, J., Hu, M., Guo, S., Du, Z., Zheng, J., Shang, D., Zamora, M. L., Zeng, L., Shao, M., and Wu, Y.-S.: Markedly enhanced absorption and direct radiative forcing of black carbon under polluted urban environments, P. Natl. Acad. Sci. USA, 113, 4266–4271, https://doi.org/10.1073/pnas.1602310113, 2016.
Peters, E., Wittrock, F., Großmann, K., Frieß, U., Richter, A., and Burrows, J. P.: Formaldehyde and nitrogen dioxide over the remote western Pacific Ocean: SCIAMACHY and GOME-2 validation using ship-based MAX-DOAS observations, Atmos. Chem. Phys., 12, 11179–11197, https://doi.org/10.5194/acp-12-11179-2012, 2012.
Reddy, B. S. K., Kumar, K. R., Balakrishnaiah, G., Gopal, K. R., Reddy, R. R., Sivakumar, V., Lingaswamy, A. P., Arafath, S. M., Umadevi, K., and Kumari, S. P.: Analysis of diurnal and seasonal behavior of surface ozone and its precursors (NOx) at a semi-arid rural site in Southern India, Aerosol Air Qual. Res., 12, 1081–1094, https://doi.org/10.4209/aaqr.2012.03.0055 2012.
Reed, A. J., Thompson, A. M., Kollonige, D. E., Martins, D. K., Tzortziou, M. A., Herman, J. R., Berkoff, T. A., Abuhassan, N. K., and Cede, A.: Effects of local meteorology and aerosols on ozone and nitrogen dioxide retrievals from OMI and pandora spectrometers in Maryland, USA during DISCOVER-AQ 2011, J. Atmos. Chem., 72, 455–482, https://doi.org/10.1007/s10874-013-9254-9, 2015.
Reed, C., Evans, M. J., Di Carlo, P., Lee, J. D., and Carpenter, L. J.: Interferences in photolytic NO2 measurements: explanation for an apparent missing oxidant?, Atmos. Chem. Phys., 16, 4707–4724, https://doi.org/10.5194/acp-16-4707-2016, 2016.
Richter, A., Begoin, M., Hilboll, A., and Burrows, J. P.: An improved NO2 retrieval for the GOME-2 satellite instrument, Atmos. Meas. Tech., 4, 1147–1159, https://doi.org/10.5194/amt-4-1147-2011, 2011.
Russell, A. R., Valin, L. C., and Cohen, R. C.: Trends in OMI NO2 observations over the United States: effects of emission control technology and the economic recession, Atmos. Chem. Phys., 12, 12197–12209, https://doi.org/10.5194/acp-12-12197-2012, 2012.
Saha, S., Moorthi, S., Wu, X., Wang, J., Nadiga, S., Tripp, P., Behringer, D., Hou, Y. T., Chuang, H.-y., and Iredell, M.: NCEP climate forecast system version 2 (CFSv2) 6-hourly products, Research Data Archive at the National Center for Atmospheric Research, Computational and Information Systems Laboratory [data set], https://doi.org/10.5065/D61C1TXF, 2011.
Sawamura, P., Müller, D., Hoff, R. M., Hostetler, C. A., Ferrare, R. A., Hair, J. W., Rogers, R. R., Anderson, B. E., Ziemba, L. D., Beyersdorf, A. J., Thornhill, K. L., Winstead, E. L., and Holben, B. N.: Aerosol optical and microphysical retrievals from a hybrid multiwavelength lidar data set – DISCOVER-AQ 2011, Atmos. Meas. Tech., 7, 3095–3112, https://doi.org/10.5194/amt-7-3095-2014, 2014.
Seinfeld, J. H. and Pandis, S. N.: Atmospheric chemistry and physics: from air pollution to climate change, John Wiley & Sons, Inc, Hoboken, New Jersey, 2016.
Sen, B., Toon, G. C., Osterman, G. B., Blavier, J.-F., Margitan, J. J., Salawitch, R. J., and Yue, G. K.: Measurements of reactive nitrogen in the stratosphere, J. Geophys. Res.-Atmos., 103, 3571–3585, https://doi.org/10.1029/97JD02468, 1998.
Shin, H. H. and Hong, S.-Y.: Intercomparison of planetary boundary-layer parametrizations in the WRF model for a single day from CASES-99, Bound.-Lay. Meteorol., 139, 261–281, https://doi.org/10.1007/s10546-010-9583-z, 2011.
Silvern, R. F., Jacob, D. J., Mickley, L. J., Sulprizio, M. P., Travis, K. R., Marais, E. A., Cohen, R. C., Laughner, J. L., Choi, S., Joiner, J., and Lamsal, L. N.: Using satellite observations of tropospheric NO2 columns to infer long-term trends in US NOx emissions: the importance of accounting for the free tropospheric NO2 background, Atmos. Chem. Phys., 19, 8863–8878, https://doi.org/10.5194/acp-19-8863-2019, 2019.
Souri, A. H., Choi, Y., Jeon, W., Li, X., Pan, S., Diao, L., and Westenbarger, D. A.: Constraining NOx emissions using satellite NO2 measurements during 2013 DISCOVER-AQ Texas campaign, Atmos. Environ., 131, 371–381, https://doi.org/10.1016/j.atmosenv.2016.02.020, 2016.
Souri, A. H., Choi, Y., Pan, S., Curci, G., Nowlan, C. R., Janz, S. J., Kowalewski, M. G., Liu, J., Herman, J. R., and Weinheimer, A. J.: First top-down estimates of anthropogenic NOx emissions using high-resolution airborne remote sensing observations, J. Geophys. Res.-Atmos., 123, 3269–3284, https://doi.org/10.1002/2017JD028009, 2018.
Spinei, E., Cede, A., Swartz, W. H., Herman, J., and Mount, G. H.: The use of NO2 absorption cross section temperature sensitivity to derive NO2 profile temperature and stratospheric–tropospheric column partitioning from visible direct-sun DOAS measurements, Atmos. Meas. Tech., 7, 4299–4316, https://doi.org/10.5194/amt-7-4299-2014, 2014.
Spurr, R.: LIDORT and VLIDORT: Linearized pseudo-spherical scalar and vector discrete ordinate radiative transfer models for use in remote sensing retrieval problems, in: Light Scattering Reviews 3, edited by: Kokhanovsky, A. A., Springer, Berlin, Heidelberg, 229–275, https://doi.org/10.1007/978-3-540-48546-9_7, 2008.
Thompson, A. M., Stauffer, R. M., Boyle, T. P., Kollonige, D. E., Miyazaki, K., Tzortziou, M., Herman, J. R., Abuhassan, N., Jordan, C. E., and Lamb, B. T.: Comparison of Near-Surface NO2 Pollution With Pandora Total Column NO2 During the Korea–United States Ocean Color (KORUS OC) Campaign, J. Geophys. Res.-Atmos., 124, 13560–13575, https://doi.org/10.1029/2019JD030765, 2019.
Thornton, J. A., Wooldridge, P. J., and Cohen, R. C.: Atmospheric NO2: In situ laser-induced fluorescence detection at parts per trillion mixing ratios, Anal. Chem., 72, 528–539, https://doi.org/10.1021/ac9908905, 2000.
Tong, D., Lamsal, L., Pan, L., Ding, C., Kim, H., Lee, P., Chai, T., Pickering, K. E., and Stajner, I.: Long-term NOx trends over large cities in the United States during the great recession: Comparison of satellite retrievals, ground observations, and emission inventories, Atmos. Environ., 107, 70–84, https://doi.org/10.1016/j.atmosenv.2015.01.035, 2015.
Travis, K. R., Jacob, D. J., Fisher, J. A., Kim, P. S., Marais, E. A., Zhu, L., Yu, K., Miller, C. C., Yantosca, R. M., Sulprizio, M. P., Thompson, A. M., Wennberg, P. O., Crounse, J. D., St. Clair, J. M., Cohen, R. C., Laughner, J. L., Dibb, J. E., Hall, S. R., Ullmann, K., Wolfe, G. M., Pollack, I. B., Peischl, J., Neuman, J. A., and Zhou, X.: Why do models overestimate surface ozone in the Southeast United States?, Atmos. Chem. Phys., 16, 13561–13577, https://doi.org/10.5194/acp-16-13561-2016, 2016.
Tu, J., Xia, Z.-G., Wang, H., and Li, W.: Temporal variations in surface ozone and its precursors and meteorological effects at an urban site in China, Atmos. Res., 85, 310–337, https://doi.org/10.1016/j.atmosres.2007.02.003, 2007.
Valin, L. C., Russell, A. R., Hudman, R. C., and Cohen, R. C.: Effects of model resolution on the interpretation of satellite NO2 observations, Atmos. Chem. Phys., 11, 11647–11655, https://doi.org/10.5194/acp-11-11647-2011, 2011.
van der A, R. J., Eskes, H. J., Roozendael, M. V., De Smedt, I., Blond, N., Boersma, F., Weiss, A., and van Peet, J. C. A.: Algorithm Document Tropospheric NO2, KNMI, the Netherlands, 23 pp., available at: https://d37onar3vnbj2y.cloudfront.net/static/docs/AD_NO2.pd (last access: 22 February 2021), 2010.
van Geffen, J. H. G. M., Boersma, K. F., Van Roozendael, M., Hendrick, F., Mahieu, E., De Smedt, I., Sneep, M., and Veefkind, J. P.: Improved spectral fitting of nitrogen dioxide from OMI in the 405–465 nm window, Atmos. Meas. Tech., 8, 1685–1699, https://doi.org/10.5194/amt-8-1685-2015, 2015.
van Stratum, B. J. H., Vilà-Guerau de Arellano, J., Ouwersloot, H. G., van den Dries, K., van Laar, T. W., Martinez, M., Lelieveld, J., Diesch, J.-M., Drewnick, F., Fischer, H., Hosaynali Beygi, Z., Harder, H., Regelin, E., Sinha, V., Adame, J. A., Sörgel, M., Sander, R., Bozem, H., Song, W., Williams, J., and Yassaa, N.: Case study of the diurnal variability of chemically active species with respect to boundary layer dynamics during DOMINO, Atmos. Chem. Phys., 12, 5329–5341, https://doi.org/10.5194/acp-12-5329-2012, 2012.
Wooldridge, P. J., Perring, A. E., Bertram, T. H., Flocke, F. M., Roberts, J. M., Singh, H. B., Huey, L. G., Thornton, J. A., Wolfe, G. M., Murphy, J. G., Fry, J. L., Rollins, A. W., LaFranchi, B. W., and Cohen, R. C.: Total Peroxy Nitrates (∑PNs) in the atmosphere: the Thermal Dissociation-Laser Induced Fluorescence (TD-LIF) technique and comparisons to speciated PAN measurements, Atmos. Meas. Tech., 3, 593–607, , 2010.
Yuan, H., McGinley, J. A., Schultz, P. J., Anderson, C. J., and Lu, C.: Short-range precipitation forecasts from time-lagged multimodel ensembles during the HMT-West-2006 campaign, J. Hydrometeorol., 9, 477–491, https://doi.org/10.1175/2007JHM879.1, 2008.
Zhang, R., Wang, Y., Smeltzer, C., Qu, H., Koshak, W., and Boersma, K. F.: Comparing OMI-based and EPA AQS in situ NO2 trends: towards understanding surface NOx emission changes, Atmos. Meas. Tech., 11, 3955–3967, https://doi.org/10.5194/amt-11-3955-2018, 2018.
Zhang, Y. and Wang, Y.: Climate-driven ground-level ozone extreme in the fall over the Southeast United States, P. Natl. Acad. Sci. USA, 113, 10025–10030, https://doi.org/10.1073/pnas.1602563113, 2016.
Zhang, Y., Wang, Y., Chen, G., Smeltzer, C., Crawford, J., Olson, J., Szykman, J., Weinheimer, A. J., Knapp, D. J., and Montzka, D. D.: Large vertical gradient of reactive nitrogen oxides in the boundary layer: Modeling analysis of DISCOVER-AQ 2011 observations, J. Geophys. Res.-Atmos., 121, 1922–1934, https://doi.org/10.1002/2015JD024203, 2016.
Zhao, C., Wang, Y., Choi, Y., and Zeng, T.: Summertime impact of convective transport and lightning NOx production over North America: modeling dependence on meteorological simulations, Atmos. Chem. Phys., 9, 4315–4327, https://doi.org/10.5194/acp-9-4315-2009, 2009.
Zhao, X., Griffin, D., Fioletov, V., McLinden, C., Davies, J., Ogyu, A., Lee, S. C., Lupu, A., Moran, M. D., Cede, A., Tiefengraber, M., and Müller, M.: Retrieval of total column and surface NO2 from Pandora zenith-sky measurements, Atmos. Chem. Phys., 19, 10619–10642, https://doi.org/10.5194/acp-19-10619-2019, 2019.
Zhao, X., Griffin, D., Fioletov, V., McLinden, C., Cede, A., Tiefengraber, M., Müller, M., Bognar, K., Strong, K., Boersma, F., Eskes, H., Davies, J., Ogyu, A., and Lee, S. C.: Assessment of the quality of TROPOMI high-spatial-resolution NO2 data products in the Greater Toronto Area, Atmos. Meas. Tech., 13, 2131–2159, https://doi.org/10.5194/amt-13-2131-2020, 2020.
Zheng, Y., Alapaty, K., Herwehe, J. A., Del Genio, A. D., and Niyogi, D.: Improving high-resolution weather forecasts using the Weather Research and Forecasting (WRF) Model with an updated Kain–Fritsch scheme, Mon. Weather Rev., 144, 833–860, https://doi.org/10.1175/MWR-D-15-0005.1, 2016.