the Creative Commons Attribution 4.0 License.
the Creative Commons Attribution 4.0 License.
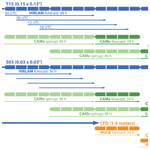
Downscaling system for modeling of atmospheric composition on regional, urban and street scales
Alexander Mahura
Alexander Baklanov
Bjarne Amstrup
Ashraf Zakey
In this study, the downscaling modeling chain for prediction of weather and atmospheric composition is described and evaluated against observations. The chain consists of interfacing models for forecasting at different spatiotemporal scales that run in a semi-operational mode. The forecasts were performed for European (EU) regional and Danish (DK) subregional-urban scales by the offline coupled numerical weather prediction HIRLAM and atmospheric chemical transport CAMx models, and for Copenhagen city-street scale by the online coupled computational fluid dynamics M2UE model.
The results showed elevated NOx and lowered O3 concentrations over major urban, industrial, and transport land and water routes in both the EU and DK domain forecasts. The O3 diurnal cycle predictions in both these domains were equally good, although O3 values were closer to observations for Denmark. At the same time, the DK forecast of NOx and NO2 levels was more biased (with a better prediction score of the diurnal cycle) than the EU forecast, indicating a necessity to adjust emission rates. Further downscaling to the street level (Copenhagen) indicated that the NOx pollution was 2-fold higher on weekends and more than 5 times higher during the working day with high pollution episodes. Despite high uncertainty in road traffic emissions, the street-scale model effectively captured the NOx and NO2 diurnal cycles and the onset of elevated pollution episodes.
The demonstrated downscaling system could be used in future online integrated meteorology and air quality research and operational forecasting, as well as for impact assessments on environment, population, and decision making for emergency preparedness and safety measures planning.
- Article
(11280 KB) - Full-text XML
- BibTeX
- EndNote
Progress in numerical weather and atmospheric composition modeling and continuously increasing supercomputing power make it possible to perform downscaling and nesting from the global to the local scales, reaching necessary horizontal and vertical resolutions for very detailed local meteorology and pollution forecasts. Global- and regional-scale atmospheric chemistry transport (ACT) modeling systems were actively developed and applied during the last decade. These were developed and applied within the frameworks of multiple EU FP6, FP7 and H2020 Monitoring of Atmospheric Composition and Climate (MACC) projects (Hollingsworth et al., 2008; Simmons, 2010; Peuch et al., 2014, 2016). Such systems mostly simulate the regional background of air pollution, for instance, in the Copernicus Atmosphere Monitoring Service (CAMS; Grasso, 2017). These large-scale models with increased resolution have limited performance improvement for rural areas (Schaap et al., 2015) but have better modeling scores when reproducing spatiotemporal pollution gradients in and near large urban areas. As a matter of fact, urban and street air pollution is usually several times higher than the pollution at regional scale over suburbs and rural areas and is mostly associated with both local emission sources (Falasca and Curci, 2018) and dominating urban weather conditions (González et al., 2018). Therefore, improvements in the performance of high-resolution models for urban areas are closely linked to the availability and quality of emission inventories at high spatiotemporal resolution as well as accurate simulation of urban meteorology (Mahura et al., 2008a, b; Pepe et al., 2016).
Generally, within the deterministic modeling approach, there are two options applicable to downscaling modeling. It can be performed with a single model capable of local grid refinement (Gettelman et al., 2018) or nesting of numerical grids (Petersen et al., 2005; Lauwaet et al., 2013). It can be also realized as a chain of different resolution models interfacing with each other (Temel et al., 2018; Veratti et al., 2020). In the latter case, the need for different models is justified by the fact that mesoscale models cannot capture local-scale processes accurately, since the fine-scale flow patterns should be explicitly resolved rather than parameterized (Schlünzen et al., 2011; Ching, 2013). As for the air-quality prediction systems, offline (Kukkonen et al., 2012) and online (Baklanov et al., 2014) approaches of coupling meteorological and chemical transport models have been commonly used. Each of these has its own advantages and limitations. Currently, there are several studies describing and evaluating systems capable of downscaling modeling of weather and atmospheric composition from regional to microscales. Amongst these, the study by San José et al. (2021) performed operational microscale simulations, but this model has a very basic chemistry scheme predominately applicable to cold seasons. The others did not consider an operational aspect and are unlikely to be able to run their microscale models in operational mode. The operational runtime constraints will not allow it because of the complexity of gas-phase chemistry mechanisms (Kwak et al., 2015) and because of the requirements for numerical grid resolution in large eddy simulation models (Khan et al., 2021).
The goal of this paper is to demonstrate and evaluate the applicability of the downscaling system coupled with computational fluid dynamics model on the operational modeling of an acute air pollution episode. This system consists of several models with nested numerical grids where meteorological and atmospheric composition prediction of regional and subregional-urban scales is performed. Three interfacing and coupled models – (i) numerical weather prediction (HIRLAM; Undén et al., 2002); (ii) atmospheric chemical transport (CAMx; Ramboll Environ, 2016; Amstrup et al., 2010a, b); and (iii) computational fluid dynamics (M2UE; Nuterman et al., 2010; Sabatino et al., 2011) – were used for airflow and pollution prediction at city-street scale (on example of Copenhagen, Denmark). In the Methodology section, we first describe the applied models and their configurations and, second, their interplay within a semi-operational setup of the downscaling system. The “Results and discussion” section presents the models' evaluation of two urban observation sites (at roof and street levels) as well as recommendations about possible improvements in the downscaling system to achieve better prediction quality of forecasting at the finest scales.
2.1 Description of models
2.1.1 HIRLAM
The numerical weather prediction (NWP) model used in the current downscaling system is the DMI's version of the High-Resolution Limited Area Model (HIRLAM; Undén et al., 2002; Petersen et al., 2005). It has basic components such as the hydrostatic semi-Lagrangian dynamical core, digital filtering initialization (Lynch and Huang, 1992), physical parameterization of radiation processes (Savijärvi, 1990), cloud-microphysics soft transition condensation scheme (Sass, 2002), turbulence parametrization (Cuxart et al., 2000), and the interactions between soil–biosphere–atmosphere surface scheme (ISBA; Noilhan and Planton, 1989). The ISBA scheme also includes the building effect parametrization (BEP; Martilli et al., 2002) module to account for effects associated with urban anthropogenic heat flux and roughness. The model is forced by meteorological lateral boundary conditions and 3D-VAR assimilated ground-based and satellite observation data (Gustafsson et al., 2001; Lindskog et al., 2001). The lateral boundaries are received every 6 h interval from the European Centre for Medium-Range Weather Forecasts (ECMWF) and had a horizontal grid resolution of 0.15∘ × 0.15∘ .
In this study, the chosen modeling domains were used for operational numerical weather prediction at DMI. These two domains with crude (T15: 0.15∘ × 0.15∘), and fine (S03: 0.03∘ × 0.03∘) horizontal grids were applied for European regional-subregional-urban Denmark scale modeling (Fig. 1a), respectively. Vertically, 40 hybrid pressure levels (starting from 32 m above ground) were used in the T15 domain and 65 such levels (starting from 12 m above ground) – in the S03 domain. The upper boundary reached 10 hPa at the top of the atmosphere in both computational domains.
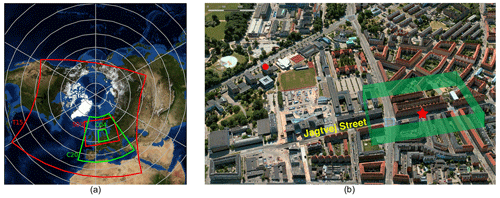
Figure 1(a) Operational and research modeling domains for European regional scale (HIRLAM-T15+CAMx-C20) and subregional-urban Denmark scale (HIRLAM-S03+CAMx-C04) forecasts; (b) Nørrebro district of Copenhagen urban area with two air-quality monitoring stations, where the red circle indicates a roof level air quality observation at H. C. Ørsted Institute and the red star indicates Jagtvej Street level observation; the green trapezoid shows the M2UE modeling domain (extracted from © Apple Maps 2020).
There are approximately 8 × 8 horizontal grids cells (each cell is about 3 × 3 km size) covering the Copenhagen metropolitan area in the S03 domain. According to Mahura et al. (2008b), the HIRLAM model with the activated BEP module must have at least 4 times more grid cells to make a significant prediction of the effect of city area on meteorology. Thus, the BEP module was disabled during operational forecasting with the S03 domain, considering the employed numerical grid as well as city size and urban characteristics of Copenhagen. The urban effects were taken into account through roughness parameter and meteorological data assimilated from urban and suburban stations.
2.1.2 CAMx
The Comprehensive Air Quality Model with Extensions (CAMx; Ramboll Environ, 2016) is the finite volume ACT model for regional and urban scales employed for air quality forecasting in our downscaling system. An updated version of carbon bond IV (CB-IV) mechanism (Gery et al., 1989) with improved isoprene chemistry (Yarwood et al., 2005) and two-mode aerosol microphysics scheme were used in the current setup. The tropospheric ultraviolet and visible radiation model (Madronich and Focke, 1999) was applied to calculate photolysis rate coefficients. Further details about gas-phase chemistry and aerosol microphysics together with wet (Seinfeld and Pandis, 2016) and dry (Wesely, 1989; Slinn and Slinn, 1980) deposition schemes are described in the model's user guide (Ramboll Environ, 2016).
For this study, two computational domains (Fig. 1a) were selected within the ACT model for modeling on regional (C20: 0.2∘ × 0.2∘) and subregional-urban scales (C04: 0.04∘ × 0.04∘) forced by meteorological output (with a coupling interval of 1 h) from HIRLAM-T15 and HIRLAM-S03 modeling domains, respectively. The vertical resolution of CAMx numerical grid is defined by the meteorological driver and, therefore, corresponds to 25 HIRLAM hybrid pressure levels covering the lowest 3 km of the troposphere. The chemical initial and boundary conditions for regional-scale modeling are represented by climatological mean values, and those for the subregional-urban scale modeling are obtained from the regional-scale CAMx model output.
The emission rates were calculated from emission inventory provided by TNO (Kuenen et al., 2010). The EMEP emission inventory was also available, but at a substantially lower resolution of 0.5∘ × 0.5∘, and therefore, the TNO inventory was used. The inventory has longitude–latitude resolution of 0.125∘ × 0.0625∘ and covers all of Europe, western Russia and northern Africa. It includes gases (NOx, SO2, CO, NMVOC, NH3, CH4) and lumped primary aerosols with diameters less than 2.5 and 10 µm. The emission rates are associated with different anthropogenic sources attributed to various anthropogenic activities according to the Selected Nomenclature for sources of Air Pollution (SNAP).
2.1.3 M2UE
For the city-street scale modeling, the Microscale Model for Urban Environment (M2UE) was developed and applied. It is a computational fluid dynamics (CFD) code for airflow and pollution prediction in an urban environment (Nuterman et al., 2010), which is able to simulate a complex aerodynamics in heterogeneous urban canopy with penetrable (vegetation) and impenetrable (buildings) obstacles and traffic-induced turbulence. The model includes a three-dimensional system of Reynolds equations, k−ε (Launder and Spalding, 1974) and cubic eddy-viscosity (Craft et al., 1996) turbulence closure schemes, and “advection–diffusion” equations to simulate atmospheric chemistry transport. A numerical solution of governing equations is based on the fully implicit time advancing scheme and the finite volume method (Patankar, 1980; Ilin, 1995; van Leer, 1974). An ordinary uniform or nonuniform regular 3D grid with a fictitious domain method (Aloyan et al., 1982; Vabishchevich, 1991) is used in the model. The M2UE model is coupled with a simplified atmospheric gas-phase chemistry mechanism. This mechanism is based on Stockwell and Goliff (2002) with an extra chemical reaction included: SO2 + OH ⟶ H2SO4 + HO2. The full list of chemical reactions and the corresponding rate parameter for this reaction are given in Table A1, Appendix A. Such simple chemistry is dictated by the fact that the model was run in a semi-operational mode, which implies relatively short forecasting time at an available computer facility. In the future, when more powerful supercomputers become available, the mechanism could be extended or improved with a more comprehensive one.
The computational domain (a section of Jagtvej Street and surroundings) used in the current study is explicitly defined by buildings, roads, and vegetation of the Copenhagen metropolitan (Fig. 1b) area obtained from the high-resolution Danish Elevation Model data set (Flatman et al., 2016). The horizontal and vertical extent of the computational domain is 500 × 500 × 100 m (detailed grid is shown in Fig. A2). The distance between buildings in the center of the computational domain, inlet and outlet boundaries is 5Hmax, where Hmax=20 m is the tallest building height. The distance between the tallest building and top boundary was 4Hmax. Therefore, the current microscale domain extent and size is an acceptable compromise between the best practices and guidelines (Franke et al., 2007) as well as total operational runtime (should not exceed 12 h in order to satisfy modeling system requirements for the entire modeling chain). The horizontal and vertical numerical grids with local refinement of grid cells ranging from 1 m at the Jagtvej Street to 5 m near the domain's boundaries was used. The Jagtvej Street emission inventory was derived from hourly traffic counts (Berkowicz et al., 2004) and emissions for different types of road vehicles (Chao et al., 2018). The traffic volume at the Jagtvej Street is similar to measured diurnal variations (Berkowicz et al., 2004, 2006). The emissions are given in Appendix A (see Fig. A1), where only one peak of concentration was observed during morning hours and also proven by street-level observations. Note that the emissions utilized in large- and microscale models are different. The CAMx model uses annual emission inventory from TNO. It is preprocessed to account for monthly, weekly, and diurnal emission variations. M2UE uses traffic counts measured at the Jagtvej Street of Copenhagen to derive more accurate emissions (Fig. A1) and, hence, accounts for very local spatiotemporal features specific to this street.
Previously, the M2UE model contributed to the COST Action 732 evaluation exercise. This exercise was devoted to microscale model quality evaluation and assurance. Moreover, the dispersion patterns of chemical species in typical urban canyons were investigated in previous studies (Franke et al., 2007; Schatzmann et al., 2010; Nuterman et al., 2010, 2011; Sabatino et al., 2011) and would not add value to this research. Since the model's dispersion capabilities were thoroughly tested, it seemed interesting and valuable to focus on operational aspects of the problem and, hence, to apply the CFD M2UE model using real-time weather and atmospheric composition fields. Therefore, the purpose of the current study is the concept proof (of the possibility of running the CFD type model operationally coupled with gas-phase chemistry), rather than a rigorous full-scale evaluation of the proposed downscaling chain.
2.2 Description of the downscaling model system
In the developed downscaling model system (Fig. 2), both the European regional (hereafter referred to as the EU) and the subregional-urban Denmark scale (hereafter referred to as the DK) forecasts are performed by the offline coupled HIRLAM and CAMx models. After up-to-date meteorological data assimilation and boundary conditions from ECMWF become available, the HIRLAM-T15 produces a 48 h meteorological forecast for the EU domain. This NWP model is always fully spun up, because this forecast is performed in a continuous manner four times per day (at 00:00, 06:00, 12:00 and 18:00 UTC). Afterwards, the meteorological output is postprocessed, interpolated, and adapted to drive the CAMx-C20 model with a spin-up time of 48 h and the forecast length of 24 h. The spin-up integration is the model's cold-start run prior to the current forecasted day. The entire cycle of offline coupled HIRLAM-T15+CAMx-C20 modeling chain takes approximately 4.5 h of CPU time.
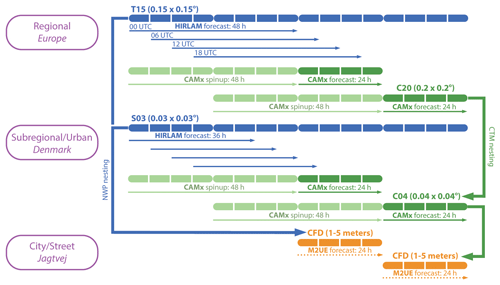
Figure 2Downscaling modeling system: from the European regional-scale forecast (HIRLAM-T15+CAMx-C20) to the subregional-urban Denmark (HIRLAM-T03+CAMx-C04) and city-street scale for Copenhagen (CFD M2UE); the blue, green, and orange horizontal bars indicate NWP HIRLAM, CTM CAMx, and CFD M2UE forecasts, respectively; horizontal and vertical arrows indicate the forecasts' cycles and downscaling (data flow), correspondingly.
As soon as the EU scale forecast is completed, the DK scale forecast of meteorology and atmospheric composition is initiated. In general, the entire procedure of model runs and interplay is very similar to the EU modeling chain. However, the differences are in boundary conditions and the forcing and resolution of numerical grids. The HIRLAM-S03 meteorological forecast is forced by outer model lateral boundaries, while the CAMx-C04 atmospheric composition forecast is forced by meteorology from HIRLAM-S03, plus chemical boundary and initial conditions from CAMx-C20. For each integration cycle, new boundary fields are read at 1 h intervals. So, the models retrieve updated meteorological and chemical fields from the previous low-resolution outer domains. The forecast length of HIRLAM-S03 and CAMx-C04 runs is 36 and 24 h, respectively. The forecast cycle used 4 h of CPU time since the DK forecast initiation.
After the urban-scale forecast completed, the street-scale (hereafter referred to as the street) forecast is launched for a section of Jagtvej Street of Copenhagen and surroundings. For the Jagtvej Street domain inlet boundaries, the Dirichlet conditions with constant profiles of airflow characteristics (from HIRLAM-S03), averaged urban roughness, and atmospheric composition fields (from CAMx-C04) are chosen to drive the M2UE model. In particular, the output from S03 and C04 is linearly interpolated from four surrounding grid points into a grid point corresponding to the M2UE domain. The interpolated fields are used to derive the mean inlet conditions under the assumption of an equilibrium boundary layer with a power-law velocity profile and the assumption of local equilibria for turbulent parameters (Richards and Hoxey, 1993). As for the chemical fields, the interpolated values are considered to be urban background concentrations. The outlet boundary condition is of the Neumann type with zero normal derivatives of all meteorological and chemical concentration variables corresponding to a fully developed and well-mixed flow. These above-mentioned boundary conditions follow the COST Action 732 best practices and guidelines (Franke et al., 2007) for microscale meteorological and atmospheric composition models and ensure consistency with each other. The forecast for 24 h is performed after HIRLAM-S03+CAMx-C04 completed runs, and it takes ∼1.5 h of CPU time. Since the M2UE model is very computationally expensive, the 24 h forecast is performed with 24 instant integration cycles forced by the hourly output of the HIRLAM-CAMx models. In this case, the spin-up time is just several minutes, as it is for the individual M2UE forecast. It is reasonable to approach performing the forecast in this way, because the boundary conditions and emission rates for each integration cycle are constant and only the rapid-photochemical reactions of O3, NOx, and NO2 are of principal interest in the study. Due to the fact that the nested M2UE domain and roof-level observation site are spatially located very close to each other and within the same grid cell of the DK domain, the time series of CFD boundary conditions are identical for O3, NOx, and NO2. Therefore, the air-flow regime was assumed to be steady during an instant integration cycle. The ratio of horizontal resolution of HIRLAM-S03+CAMx-C04 and M2UE-Jagtvej domains exceeds a permissible limit of 5 (Sokhi et al., 2018). However, the runtime of the entire modeling chain equals 10 CPU hours, and adding an intermediate domain (or chain of domains) between these three model domains will obviously lead to increased runtime over 12 h. Such large span of forecasting time is considered to be too large, taking into account the time constraints for operational tasks and cases when the models' rerun is required (not to mention preprocessing and postprocessing the large amount of models' input and output). Thus, such downscaling is a trade-off between acceptable runtime and permissible resolution.
The example of the downscaling modeling chain realized in this study in a semi-operational mode with coupled HIRLAM+CAMx+M2UE models is described and evaluated with a focus on Denmark and Copenhagen for a specific meteorological situation.
During 4–5 September 2011 the meteorological situation was the following: the cyclonic system originated over Iceland and moved southward towards the British Islands and then eastward towards the Jutland Peninsula of Denmark with gradually changing atmospheric pressure from 990 to 1010 hPa. The cold front associated with the cyclone passed over UK and Denmark on these days. On 4 September morning, the cold front approached the northern part of the Jutland Peninsula, then propagated towards the island of Zealand, and brought up to 10 mm of accumulated (6 h) precipitation. Over Denmark, during night–morning hours on 5 September, the precipitation increased on average up to 15–20 mm (with several local maxima up to 40 mm). During these days, on a diurnal cycle the air temperature's range was 18–24 and 12–18 ∘C (on 4 and 5 September, respectively) with further gradual cooling. The vertical sounding diagrams (Schleswig, Germany, 54.53∘ N, 9.55∘ E – the closest station to Denmark) showed the presence of the temperature inversion layer extended up to 1.5 km (at noon on 4 September) and reduced to 500 m altitude (at night on 5 September), and then a layer of smaller depth remained at the top of the boundary layer at noon.
A near-surface spatial distribution of O3 and NOx concentrations (Fig. 3a, b) within the EU domain is shown for 5 September 2011, at 10:00 UTC. As seen, the elevated NOx concentration of up to 50 µg m−3 was observed over urban, industrial, and port areas of Denmark and neighboring countries. In addition, the North Sea oil extraction and international shipping were other sources of elevated NOx levels over the Danish territorial waters. The near-surface O3 concentration varied within the range of 40–150 µg m−3, with local minima over and downwind the areas of anthropogenic NOx sources (Fig. 3a) where ozone was depressed due to the NOx titration.
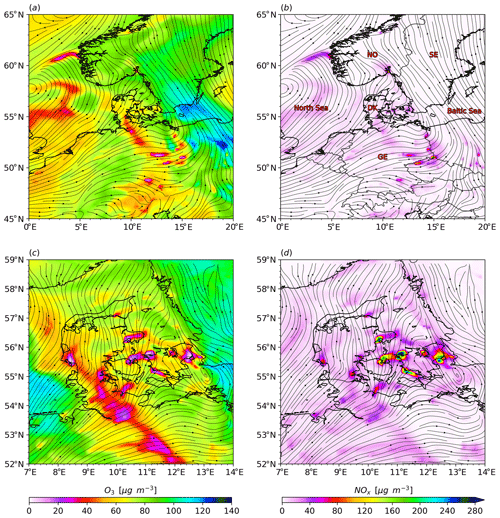
Figure 3Simulation output on 5 September 2011, 10:00 UTC: HIRLAM-T15+CAMx-C20 results for concentrations in [µg m−3] of (a) O3 and (b) NOx within the European regional-scale domain and HIRLAM-S03+CAMx-C04 results for concentrations of (c) O3 and (d) NOx within the Denmark subregional-urban scale domain.
As for the nested DK domain (Fig. 3c, d), it is seen from the near-surface wind and concentrations patterns that the mesoscale circulation as well as the local anthropogenic emission sources were resolved in greater detail due to the finer S03-C04 horizontal resolution. Therefore, one can observe additional local concentration maxima of NOx with higher levels up to 250 µg m−3 over Denmark. These were mostly localized near the same type of anthropogenic sources as those mentioned above in the EU domain. As a result, there were more O3 depression spots including those over the northern Jutland Peninsula's highways over the territories of Denmark and Germany. However, the background values of the near-surface ozone did not change significantly at large distances from the anthropogenic NOx sources.
In Fig. 4, the time series of O3, NOx, and NO2 observations provided by the Danish air-quality monitoring program (Ellerman et al., 2012) and modeled concentrations by the downscaling system are presented. Those observations were performed by automatic stations (Fig. 1b) at the roof level of H. C. Ørsted Institute and at Jagtvej Street (only NOx, NO2, particulate matter, and benzene were measured on the street; O3 was not available for that time at this location). As seen in Fig. 4a, the T15-C20 and S03-C04 results showed an ability to effectively reproduce the O3 diurnal cycle (EU_Rp=0.81 and DK_Rp=0.8, with p≪5 %) for both domains at regional and subregional-urban scales. However, the forecasted O3 at the EU scale was generally overestimated in comparison to the modeling results at the DK scale (EU_RMSE = 31 vs. DK_RMSE = 19). Although the NOx and NO2 levels were generally overestimated in the DK scale forecast (Fig. 4b, c) when compared to the EU scale forecast and observations (EU_RMSE = 23 vs. DK_RMSE = 47 for NOx and EU_RMSE = 13 vs. DK_RMSE = 33 for NO2), the diurnal cycle at the DK scale is better captured according to obtained Pearson correlation values (EU_Rp=0.56 vs. DK_Rp=0.69 for NOx and EU_Rp=0.64 vs. DK_Rp=0.72 for NO2). And it is particularly notable when the NOx and NO2 peaks were observed during several morning hours (on 5 September 2011, 07:00 UTC). This was caused by the complex unfavorable meteorological conditions over the Copenhagen metropolitan area and high emission rates mainly from intense morning traffic. The timing and levels of NOx and NO2 peaks were well captured by the DK scale forecast in contrast to the EU one, which poorly predicted it with slightly elevated NOx and NO2 values. Note the O3 depression at the same time, which was not captured by the EU air-quality forecast. Since the primary sources of atmospheric nitrogen are anthropogenic emissions, its overestimated concentrations are attributed to the preprocessing and treatment of emission rates. It can be related to the interpolation of the original gridded emissions into the meshes of ACT model, which is apparently insufficient, and therefore, some additional rescaling adjustments in terms of emission rates and their time dynamics are required.
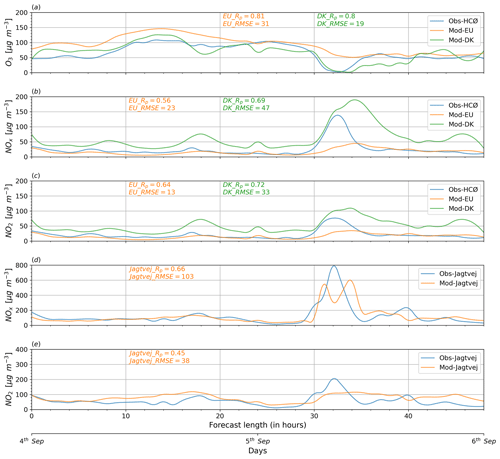
Figure 4Time series of observations vs. HIRLAM+CAMx+M2UE forecasts for (a) O3, (b, d) NOx and (c, e) NO2 for the European, Denmark, and Copenhagen modeling domains during Sunday–Monday 4–5 September 2011; (a, b, c) HCØ roof level observations of O3, NOx, and NO2 vs. modeling results for EU and DK domains; (d, e) Jagtvej Street level observations of NOx and NO2 vs. M2UE. Pearson correlation Rp and root mean square error RMSE of modeled vs. observed concentrations for three domains.
The diurnal cycles of NOx and NO2 concentrations observed and modeled for the Jagtvej Street are shown in Fig. 4d and e. Following analysis and calculated correlation values (Rp=0.66 and Rp=0.45 for NOx and NO2, respectively), the street-scale M2UE model effectively forecasted the observed NOx and NO2 diurnal cycles, including the onset of an elevated air pollution episode on 5 September (see forecast length 30–35 h). It corresponded to the local time of 8–10 h of a typical working day (Monday) when the most intense road traffic is observed. However, the model's quantitative estimate of NOx concentration is substantially biased (with RMSE = 103) particularly during 5 September 2011. In spite of the captured elevated NOx levels, the model predicted two peaks at 31 and 34 h of forecast length and depression at 32 h, while the time series of observations showed only one peak at 32 h, which was approximately 200 µg m−3 higher than the modeled peaks. Note that the model also exhibited elevated NO2 levels (with RMSE = 38) during the high air pollution episode with the concentrations underestimated.
There are several reasons why the M2UE model was able to make a qualitatively good prediction in general but had difficulties at capturing the elevated NOx and NO2 concentrations. First of all, the coupling interval span of the offline coupled models (HIRLAM+CAMx) plays an important role in constraining the influence of mesoscale disturbances on the evolution of atmospheric composition (Korsholm et al., 2011). It might give an offset in the timing and position of the predicted concentrations. As seen in Fig. 4a, b, and c, the effect of that at the onset of the high air pollution episode (where its slight offset of approximately half an hour) was observed and then inherited by the nested microscale model. The second reason is that the M2UE forcing was provided on an hourly basis by the HIRLAM-S03+CAMx-C04 modeling chain. Such forcing is rather crude in terms of time and horizontal resolution for the CFD model, which is capable of predicting very fine spatiotemporal turbulent flow scales occurring within meters and seconds. The third reason is an uncertainty in local road traffic emissions used by the M2UE model, although some traffic count data and information about emissions from different types of vehicles were available (Chao et al., 2018). Despite these above-mentioned shortcomings, the presented downscaling modeling system was able to produce good-quality atmospheric composition forecasts across different spatial and temporal scales.
In this study, the downscaling modeling system for real-time prediction of weather and atmospheric composition up to the street scale has been described and tested. It consists of several interfacing models. The forecasts were performed at the regional and subregional-urban scales by the offline coupled operational numerical weather prediction HIRLAM and atmospheric chemical transport CAMx models and at the city-street scale by the online coupled computational fluid dynamics M2UE model. The downscaling system was tested for the Europe (EU), Denmark (DK), and Copenhagen street domains in a semi-operational forecasting mode and evaluated against existing observations for a high pollution episode of 4–5 September 2011.
The results of modeling showed elevated NOx and lowered O3 concentrations over major urban, industrial, and transport land and water routes in both the EU and DK scale forecasts. Comparison of these forecasts showed higher levels of NOx and slightly lower O3 in the DK domain because of higher NOx emission rates and titration. The O3 diurnal cycle predictions in both the EU and DK domains are equally good, although O3 values were closer to observations for DK. At the same time, the DK scale forecast of NOx and NO2 levels was more biased (with better prediction score of the diurnal cycle) than the EU scale forecast. Further downscaling to the street level indicated that the NOx pollution (mainly from road traffic) was 2-fold higher on weekends and more than 5 times higher during the working day with high pollution episodes. Despite high uncertainty in road traffic emissions, the M2UE model effectively captured the NOx and NO2 diurnal cycles and the onset of elevated pollution episodes. However, in order to arrive at the final conclusion about the performance of individual systems' components and the entire downscaling chain, evaluation for a more extended time period and of additional measurement sites around Denmark is needed.
A potential improvement of this downscaling system can come from employing online coupled (instead of offline coupled) mesoscale models. Such an approach could help to minimize problems associated with offline coupling interval and therefore produce a better fit of modeling results with observations, as well as better identify the timing and location of elevated pollution episodes. As for the local-scale predictions, an intermediate additional nested domain between subregional-urban and microscale runs for the Copenhagen metropolitan area could provide improvement for local-scale airflow and pollution forecasting scores. However, these will be possible once a better-quality high-resolution emission inventory becomes available. The demonstrated downscaling system with the suggested above improvements could be used in future online integrated meteorology and air quality research and operational forecasting. Such a system can be also applied for advanced planning safety measures, postaccidental analysis, emergency preparedness, health, and environment impact assessments.
Keeping in mind the main uncertainties (e.g., street-level emissions, increasing number of urban observations), there are several possibilities for improving demonstrated urban-street level forecasts using different types of observations (e.g., from remote sensing, crowd sourcing and citizen science) in urban areas. These observations and recently developing methods such as the bias correction techniques, machine learning, artificial intelligence, and neural networks can also improve air-quality forecasting (see overview, e.g., in Bai et al., 2018).
Chemical reactions and rate parameters are the same as in Stockwell and Goliff (2002), except chemical reaction (SO2 +OH ⟶ H2SO4 + HO2) with the rate parameter from (Sander et al., 2006):
where T is temperature [K] and Cair is air concentration [molecules/cm3].
The HIRLAM model (Undén et al., 2002) source code is available under the signed HIRLAM code transfer and use agreement (http://hirlam.org, last access: 20 June 2021). The CAMx model (Ramboll Environ, 2016) source code is an open-source software (can be downloaded from https://camx-wp.azurewebsites.net/getmedia/CAMx_v6.40.src.161223.tgz, last access: 20 June 2021). The M2UE model source code is available from the authors upon request.
The modeling chain (T15, S03, C20, C04, and M2UE) output can be reproduced through subsequent runs of these models at supercomputer facility (HPC). Simulation output for a one day run of the models – T15, S03, C20, C04, and M2UE – produced 176, 132, 4.4, 6.8, and 11.28 GB, respectively. In this study, covering a four-day period including 2–3 September as a spin-up, the total generated output is equal to 1.322 TB.
AB, AM designed the research; BA and RN created and set up integrated downscaling of the models; AZ developed a local-scale gas-phase chemistry mechanism; RN performed models' evaluation; RN, AM, and AB wrote the paper.
The authors declare that they have no conflict of interest.
Publisher's note: Copernicus Publications remains neutral with regard to jurisdictional claims in published maps and institutional affiliations.
This article is part of the special issue “Air Quality Research at Street-Level (ACP/GMD inter-journal SI)”. It is not associated with any conference.
The research leading to these results has received funding from the European Union's Seventh Framework Programme (FP7/2007–2013) under grant agreement no. 218793 (MACC – Monitoring of Atmospheric Composition and Climate; 2009–2011). The authors are thankful to the PEEX (Pan-Eurasian Experiment; https://www.atm.helsinki.fi/peex, last access: 20 June 2021) program for long-term collaboration within the PEEX's Modelling Platform and Impact on Society tasks. The authors are thankful to Bent H. Sass and Jens H. Sorensen (Danish Meteorological Institute, DMI; Copenhagen, Denmark) for administrative support. Special thanks to DMI's IT and eScience departments for computing advice and support on HPC CRAY-XT5, and in particular to Jacob Weismann (DMI) for the M2UE model optimization.
This research has been supported by the FP7 Environment (grant no. 218793).
This paper was edited by Karine Sartelet and reviewed by two anonymous referees.
Aloyan, A., Baklanov, A., and Penenko, V.: Fictitious regions in numerical simulation of quarry ventilation, Soviet Meteorology and Hydrology, 7, 32–37, 1982.
Amstrup B., Baklanov, A., Feddersen, H., Lorenzen, T., Mahura, A., Nuterman, R., Weismann, J., Caian, M., Dumitrache, R., Rada, C., and Craciunescu, V.: Core-downstream processing chain test cases. Evaluation of current R-ENS individual and ensemble forecasts in the Copenhagen and Bucharest areas, in EU FP7 MACC Report D_OINT_2.4.1-2, edited by: Baklanov, A. and Mahura, A., Danish Meteorological Institute, Copenhagen, 36 pp., 2010a.
Amstrup B., Baklanov, A., Lorenzen, T., Mahura, A., Nuterman, R., Weismann, J., Banciu, D., Tascu, S., Pietrisi, M., Dumitrache, R., and Caian, M.: Core-downstream processing chain test cases. Setup of the two downscaling model configurations, in EU FP7 MACC Report D_OINT_2.5.1-2, edited by: Baklanov, A. and Nuterman, R., Danish Meteorological Institute, Copenhagen, 18 pp., 2010b.
Bai, L., Wang, J., Ma, X., and Lu, X.: Air Pollution Forecasts: An Overview, Int. J. Environ. Res. Pu., 15, 780, https://doi.org/10.3390/ijerph15040780, 2018.
Baklanov, A., Schlünzen, K., Suppan, P., Baldasano, J., Brunner, D., Aksoyoglu, S., Carmichael, G., Douros, J., Flemming, J., Forkel, R., Galmarini, S., Gauss, M., Grell, G., Hirtl, M., Joffre, S., Jorba, O., Kaas, E., Kaasik, M., Kallos, G., Kong, X., Korsholm, U., Kurganskiy, A., Kushta, J., Lohmann, U., Mahura, A., Manders-Groot, A., Maurizi, A., Moussiopoulos, N., Rao, S. T., Savage, N., Seigneur, C., Sokhi, R. S., Solazzo, E., Solomos, S., Sørensen, B., Tsegas, G., Vignati, E., Vogel, B., and Zhang, Y.: Online coupled regional meteorology chemistry models in Europe: current status and prospects, Atmos. Chem. Phys., 14, 317–398, https://doi.org/10.5194/acp-14-317-2014, 2014.
Berkowicz, R., Britter, R., and Di Sabatino, S.: Optimisation of Modelling Methods for Traffic Pollution in Streets (TRAPOS), National Environment Research Institute, Roskilde, Denmark, 114 pp., 2004.
Berkowicz, R., Winther, M., and Ketzel, M.: Traffic pollution modelling and emission data, Environ. Modell. Softw., 21, 454–460, https://doi.org/10.1016/j.envsoft.2004.06.013, 2006.
Chao, E. L., Rosen, J. A., Hu, P. S., Schmitt, R., Sprung, M. J., Nguyen, L. X., Riley, D., Parker, K., Young, L., Zhang, J., Beningo, S., Chambers, M., Ford, C., Notis, K., Liu, M., and Smith-Pickel, S.: National Transportation Statistics, Bureau of Transportation Statistics, U.S. Department of Transportation, Washington, DC, USA, 469 pp., 2018.
Ching, J.: A perspective on urban canopy layer modeling for weather, climate and air quality applications, Urban Climate, 3, 13–39, https://doi.org/10.1016/j.uclim.2013.02.001, 2013.
Craft, T. J., Launder, B. E., and Suga, K.: Development and application of a cubic eddy-viscosity model of turbulence, Int. J. Heat Fluid Fl., 17, 108–115, https://doi.org/10.1016/0142-727X(95)00079-6, 1996.
Cuxart, J., Bougeault, P., and Redelsperger, J.-L.: A turbulence scheme allowing for mesoscale and large-eddy simulations, Quart. J. Roy. Meteor. Soc., 126, 1–30, https://doi.org/10.1002/qj.49712656202, 2000.
Ellermann, T., Nøjgaard, J., Nordstrøm, C., Brandt, J., Christensen, J., Ketzel, M., and Jensen, S.: The Danish Air Quality Monitoring Program. Annual Summary for 2011, DCE – Danish Centre for Environment and Energy, Aarhus: Aarhus University, 63 pp., available at: https://www2.dmu.dk/Pub/SR37.pdf (last access: 20 June 2021), 2012.
Falasca, S. and Curci, G.: High-resolution air quality modeling: Sensitivity tests to horizontal resolution and urban canopy with WRF-CHIMERE, Atmos. Environ., 187, 241–254, https://doi.org/10.1016/j.atmosenv.2018.05.048, 2018.
Flatman, A., Rosenkranz, B., Evers, K., Bartels, P., Kokkendorff, S., Knudsen, T., and Nielsen, T.: Quality assessment report to the Danish Elevation Model (DK-DEM), Agency for Data Supply and Efficiency, Copenhagen, 26 pp., 2016.
Franke J., Hellsten, A., Schlünzen, H., Carissimo, B. (Eds.): Best Practice Guideline for the CFD Simulation of Flows in the Urban Environment: COST Action 732 Quality Assurance and Improvement of Microscale Meteorological Models, COST Office, Hamburg, Germany, ISBN: 3-00-018312-4, 2007.
Gery, M., Whitten, G., Killus, J., and Dodge, M.: A photochemical kinetics mechanism for urban and regional scale computer modelling, J. Geophys. Res., 94, 12925–12956, https://doi.org/10.1029/JD094iD10p12925, 1989.
Gettelman, A., Callaghan, P., Larson, V. E., Zarzycki, C. M., Bacmeister, J. T., Lauritzen, P. H., Bogenschutz, P. A., and Neale, R. B.: Regional climate simulations with the Community Earth System Model, J. Adv. Model. Earth Sy., 10, 1245–1265, https://doi.org/10.1002/2017MS001227, 2018.
González, C. M., Ynoue, R. Y., Vara-Vela, A., Rojas, N. Y., and Aristizábal, B. H.: High-resolution air quality modeling in a medium-sized city in the tropical Andes: Assessment of local and global emissions in understanding ozone and PM10 dynamics, Atmos. Pollut. Res., 9, 934–948, https://doi.org/10.1016/j.apr.2018.03.003, 2018.
Grasso, O.: Interim evaluation of Copernicus, Final report, Imelpgriuimerie centrale, Luxembourg, 224 pp., https://doi.org/10.2873/373088, 2017.
Gustafsson, N., Berre, L., Hörnquist, S., Huang, X.-Y., Lindskog, M., Navascués, B., Mogensen, K. S., and Thorsteinsson, S.: Three-dimensional variational data assimilation for a limited area model. Part I: General formulation and the background error constraint, Tellus A, 53, 425–446, https://doi.org/10.3402/tellusa.v53i4.12198, 2001.
Hollingsworth, A., Engelen, R. J., Textor, C., Benedetti, A., Boucher, O., Chevallier, F., Dethof, A., Elbern, H., Eskes, H., Flemming, J., Granier, C., Kaiser, J. W., Morcrette, J.-J., Rayner, P., Peuch, V.-H., Rouil, L., Schultz, M. G., Simmons, A. J., and The GEMS Consortium: Towards a monitoring and forecasting system for atmospheric composition: The GEMS Project, B. Am. Meteorol. Soc., 89, 1147–1164, https://doi.org/10.1175/2008BAMS2355.1, 2008.
Ilin, V.: Methods of Incomplete Factorization for Solving the Alge- braic Systems [in Russian], Fizmatlit, Moscow, 286 pp., 1995.
Khan, B., Banzhaf, S., Chan, E. C., Forkel, R., Kanani-Sühring, F., Ketelsen, K., Kurppa, M., Maronga, B., Mauder, M., Raasch, S., Russo, E., Schaap, M., and Sühring, M.: Development of an atmospheric chemistry model coupled to the PALM model system 6.0: implementation and first applications, Geosci. Model Dev., 14, 1171–1193, https://doi.org/10.5194/gmd-14-1171-2021, 2021.
Korsholm, U., Baklanov, A., and Sørensen, J.: Status and Evaluation of Enviro-HIRLAM: Differences Between Online and Offline Models, in Integrated Systems of Meso-Meteorological and Chemical Transport Models, edited by: Baklanov, A., Mahura, A., and Sokhi, R., Springer, Berlin, 61–74, 2011.
Kuenen, J., van der Gon, H., Visschedijk, A., van der Brugh, H., Finardi, S., Radice, P., d'Allura, A., Beevers, S., Theloke, J., Uzbasich, M., Honoreì, C., and Perrussel, O.: A Base Year (2005) MEGAPOLI European Gridded Emission Inventory (Final Version), MEGAPOLI Scientific Report 10–17, DMI, Copenhagen, 39 pp., ISBN 978-87-993898-8-9, 2010.
Kukkonen, J., Olsson, T., Schultz, D. M., Baklanov, A., Klein, T., Miranda, A. I., Monteiro, A., Hirtl, M., Tarvainen, V., Boy, M., Peuch, V.-H., Poupkou, A., Kioutsioukis, I., Finardi, S., Sofiev, M., Sokhi, R., Lehtinen, K. E. J., Karatzas, K., San José, R., Astitha, M., Kallos, G., Schaap, M., Reimer, E., Jakobs, H., and Eben, K.: A review of operational, regional-scale, chemical weather forecasting models in Europe, Atmos. Chem. Phys., 12, 1–87, https://doi.org/10.5194/acp-12-1-2012, 2012.
Kwak, K.-H., Baik, J.-J., Ryu, Y.-H., and Lee, S.-H.: Urban air quality simulation in a high-rise building area using a CFD model coupled with mesoscale meteorological and chemistry-transport models, Atmos. Environ., 100, 167–177, https://doi.org/10.1016/j.atmosenv.2014.10.059, 2015.
Launder, B. E. and Spalding, D. B.: The numerical computation of turbulent flows, Comput. Method. Appl. M., 3, 269–289, https://doi.org/10.1016/0045-7825(74)90029-2, 1974.
Lauwaet, D., Viaene, P., Brisson, E., van Noije, T., Strunk, A., van Looy, S., Maiheu, B., Veldeman, N., Blyth, L., de Ridder, K., and Janssen, S.: Impact of nesting resolution jump on dynamical downscaling ozone concentrations over Belgium, Atmos. Environ., 67, 46–52, https://doi.org/10.1016/j.atmosenv.2012.10.034, 2013.
Lindskog, M., Gustafsson, N., Navascués, B., Mogensen, K. S., Huang, X.-Y., Yang, X., Andræ, U., Berre, L., Thorsteinsson, S., and Rantakokko, J.: Three-dimensional variational data assimilation for a limited area model. Part II: Observation handling and assimilation experiments, Tellus A, 53, 447–468, https://doi.org/10.3402/tellusa.v53i4.14578, 2001.
Lynch, P. and Huang, X.-Y.: Initialization of the HIRLAM Model Using a Digital Filter, Mon. Weather Rev., 120, 1019–1034, https://doi.org/10.1175/1520-0493(1992)120<1019:IOTHMU>2.0.CO;2, 1992.
Madronich, S. and Focke, S.: The role of solar radiation in atmospheric chemistry, in: Handbook of Environmental Chemistry, edited by: Boule P., Springer-Verlag, New York, 1–26, 1999.
Mahura, A., Leroyer, S., Baklanov, A., Mestayer, P., Korsholm, U., and Calmet, I.: Temporal and Spatial Variability of Fluxes in Urbanized Areas, in: Urban Climate and Bioclimate, University of Lodz, Lodz, Poland, 219–232, ISBN:978-83-7525-243-9, 2008a.
Mahura, A., Petersen, C., Baklanov, A., and Amstrup, B.: Evaluation of Building Effect Parameterization Module for Urbanized Numerical Weather Prediction Modelling, in: Urban Climate and Bioclimate, University of Lodz, Lodz, Poland, 371–380, ISBN 978-83-7525-243-9, 2008b.
Martilli, A., Clappier, A., and Rotach, M.: An urban surface exchange parameterization for mesoscale models, Bound.-Lay. Meteorol., 104, 261–304, https://doi.org/10.1023/A:1016099921195, 2002.
Noilhan, J. and Planton, S.: A simple parameterization of land surface processes for meteorological models, Mon. Weather Rev., 117, 536–549, https://doi.org/10.1175/1520-0493(1989)117<0536:ASPOLS>2.0.CO;2, 1989.
Nuterman, R., Baklanov, A., and Starchenko, A.: Modeling of aerodynamics and pollution dispersion from traffic in the urban sublayer, Math. Models Comput. Simul., 2, 738–752, https://doi.org/10.1134/S2070048210060098, 2010.
Nuterman, R., Starchenko, A. V., and Baklanov, A.: Numerical model of urban aerodynamics and pollution dispersion. Int. J. of Environment and Pollution, 44, 385–393, https://doi.org/10.1504/IJEP.2011.038440, 2011.
Patankar, S.: Numerical Heat Transfer and Fluid Flow (1st edn.), CRC Press, Boca Raton, FL, USA, 214 pp., 1980.
Pepe, N., Pirovano, G., Lonati, G., Balzarini, A., Toppetti, A., Riva, G. M., and Bedogni, M.: Development and application of a high-resolution hybrid modelling system for the evaluation of urban air quality, Atmos. Environ., 141, 297–311, https://doi.org/10.1016/j.atmosenv.2016.06.071, 2016.
Petersen, C., Kmit, M., Nielsen, N., Amstrup, B., and Huess, V.: Performance of DMI-HIRLAM-T15 and DMI-HIRLAM-S05 and the storm surge model in winter storms, Technical Report 05-13, DMI, Copenhagen, 31 pp., ISSN 1399-1388, 2005.
Peuch, V.-H., Engelen, R., Calnan, R., Lambert, J.-C., and de Rudder, A., and The MACC-II Consortium: Monitoring Atmospheric Composition and Climate II – Interim Implementation, Final Report, ECMWF, Reading, UK, 137 pp., 2014.
Peuch, V.-H., Engelen, R., Calnan, R., Lambert, J.-C., and de Rudder, A., and The MACC-III Consortium: Monitoring Atmospheric Composition and Climate 3, Final Report, ECMWF, Reading, UK, 148 pp., 2016.
Ramboll Environ: User's Guide, Comprehensive air quality model with extensions, Version 6.40, Ramboll Environ, Novato, California, 283 pp., 2016 (data available at: https://camx-wp.azurewebsites.net/getmedia/CAMx_v6.40.src.161223.tgz, last access: 20 June 2021).
Richards, P. J. and Hoxey, R. P.: Appropriate boundary conditions for computational wind engineering models using the k-ε turbulence model, J. Wind Eng. Ind. Aerod., 46–47, 145–153, https://doi.org/10.1016/0167-6105(93)90124-7, 1993.
Sabatino, S. D., Buccolieri, R., Olesen, H., Rørdam, H., Ketzel, M., Berkowicz, R., Franke, J., Schatzmann, M., Schlünzen, K. H., Leitl, B., Britter, R., Borrego, C., Alexandre, C., Castelli, S. T., Reisin, T. G., Hellsten, A., Saloranta, J., Moussiopoulos, N., Barmpas, F., Brzozowski, K., Goricsán, I., Balczò, M., Bartzis, J. G., Efthimiou, G., Nuterman, R., and Starchenko, A. V.: COST 732 in practice: the MUST model evaluation exercise, Int. J. Environ. Pollut., 44, 403–418, https://doi.org/10.1504/IJEP.2011.038442, 2011.
Sander, S. P., Friedl, R. R., Golden, D. M., Kurylo, M. J., Moortgat, G. K., Keller-Rudek, H., Wine, P. H., Ravishankara, A. R., Kolb, C. E., Molina, M. J., Finlayson-Pitts, B. J., Huie, R. E., and Orkin, V. L.: Chemical Kinetics and Photochemical Data for Use in Atmospheric Studies, Evaluation Number 15, JPL Publication, Jet Propulsion Laboratory, National Aeronautics and Space Administration, Pasadena, CA, USA, 2006.
San José, R., Pérez, J. L., and Gonzalez-Barras, R. M.: Assessment of mesoscale and microscale simulations of a NO2 episode supported by traffic modelling at microscopic level, Sci. Total Environ., 752, 141992, https://doi.org/10.1016/j.scitotenv.2020.141992, 2021.
Sass, B. H.: A research version of the STRACO cloud scheme, DMI Tech. Rep., 02-10, Danish Meteorological Institute, Copenhagen, 25 pp., ISSN 1399-1388, 2002.
Savijärvi, H.: Fast radiation parameterization schemes for mesoscale and short-range forecast models, J. Appl. Meteor., 29, 437–447, https://doi.org/10.1175/1520-0450(1990)029<0437:FRPSFM>2.0.CO;2, 1990.
Schaap, M., Cuvelier, C., Hendriks, C., Bessagnet, B., Baldasano, J. M., Colette, A., Thunis, P., Karam, D., Fagerli, H., Graff, A., Kranenburg, R., Nyiri, A., Pay, M. T., Rouïl, L., Schulz, M., Simpson, D., Stern, R., Terrenoire, E., and Wind, P.: Performance of European chemistry transport models as function of horizontal resolution, Atmos. Environ., 112, 90–105, https://doi.org/10.1016/j.atmosenv.2015.04.003, 2015.
Schatzmann, M., Olesen, H., and Franke, J. (Eds.): COST 732 Model Evaluation Case Studies: Approach and Results, COST Office, Hamburg, Germany, ISBN 3-00-018312-4, 2010.
Schlünzen, K., Grawe, D., Bohnenstengel, S., Schlüter, I., and Koppmann, R.: Joint modelling of obstacle induced and mesoscale changes – Current limits and challenges, J. Wind Eng. Ind. Aerod., 99, 217–225, https://doi.org/10.1016/j.jweia.2011.01.009, 2011.
Seinfeld, J. and Pandis, S.: Atmospheric Chemistry and Physics, From Air Pollution to Climate Change (2nd edn.), John Wiley & Sons, New York, 1152 pp., 2016.
Simmons, A.: Monitoring Atmospheric Composition and Climate, Meteorology – ECMWF Newsletter No. 123, 10–13, https://doi.org/10.21957/vqcvmlyg, 2010.
Slinn, S. and Slinn, W.: Predictions for particle deposition on natural waters, Atmos. Environ., 24, 1013–1016, https://doi.org/10.1016/0004-6981(80)90032-3, 1980.
Sokhi, R., Baklanov, A., and Schlünzen, K. (Eds.): Mesoscale Modelling for Meteorological and Air Pollution Applications, Anthem Press, New York, 382 pp., available at: http://www.jstor.org/stable/j.ctv80cdh5 (last access: 20 June 2021), 2018.
Stockwell, W. R. and Goliff, W. S.: Comment on “Simulation of a reacting pollutant puff using an adaptive grid algorithm” by R. K. Srivastava et al., J. Geophys. Res., 107, 4643, https://doi.org/10.1029/2002JD002164, 2002.
Temel, O., Bricteux, L., and van Beeck, J.: Coupled WRF-OpenFOAM study of wind flow over complex terrain, J. Wind Eng. Ind. Aerod., 174, 152–169, https://doi.org/10.1016/j.jweia.2018.01.002, 2018.
Undén, P., Rontu, L., Järvinen, H., Lynch, P., Calvo, J., Cats, G., Cuxart, J., Eerola, K., Fortelius, C., Garcia-Moya, J. A., Jones, C., Lenderlink, G., McDonald, A., Mc-Grath, R., Navascues, B., Nielsen, N. W., Øidegaard, V., Rodriguez, E., Rummukainen, M., Rõõm, R., Sattler, K., Sass, B. H., Savijärvi, H., Schreur, B. W., Sigg, R., The, H., and Tijm, A.: HIRLAM-5 Scientific Documentation, Tech. rep., The HIRLAM project, Norrköping, Sweden, 2002 (data available at: http://hirlam.org, last access 20 June 2021).
Vabishchevich, P. N.: The Method of Fictitious Domains in Problems of Mathematical Physics [in Russian], Moscow University, Moskva, 1991.
van Leer, B.: Towards the Ultimate Conservative Difference Scheme. II. Monotonicity and Conservation Combined in a Second Order Scheme, J. Comput. Phys., 14, 361–370, https://doi.org/10.1016/0021-9991(74)90019-9, 1974.
Veratti, G., Fabbi, S., Bigi, A., Lupascu, A., Tinarelli, G., Teggi, S., Brusasca, G., Butler, T. M., and Ghermandi, G.: Towards the coupling of a chemical transport model with a micro-scale Lagrangian modelling system for evaluation of urban NOx levels in a European hotspot, Atmos. Environ., 223, 117285, https://doi.org/10.1016/j.atmosenv.2020.117285, 2020.
Wesely, M.: Parameterization of Surface Resistances to Gaseous Dry Deposition in Regional-Scale Numerical Models, Atmos. Environ., 23, 1293–1304, https://doi.org/10.1016/0004-6981(89)90153-4, 1989.
Yarwood, G., Rao, S., Yocke, M., and Whitten, G.: Updates to the Carbon Bond chemical mechanism: CB05, RT-04-00675, ENVIRON International Corporation, Yocke & Company, Novato, CA, USA, 161 pp., 2005.