the Creative Commons Attribution 4.0 License.
the Creative Commons Attribution 4.0 License.
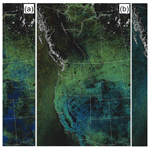
Forest-fire aerosol–weather feedbacks over western North America using a high-resolution, online coupled air-quality model
Paul A. Makar
Ayodeji Akingunola
Jack Chen
Balbir Pabla
Wanmin Gong
Craig Stroud
Christopher Sioris
Kerry Anderson
Philip Cheung
Junhua Zhang
Jason Milbrandt
The influence of both anthropogenic and forest-fire emissions, and their subsequent chemical and physical processing, on the accuracy of weather and air-quality forecasts, was studied using a high-resolution, online coupled air-quality model. Simulations were carried out for the period 4 July through 5 August 2019, at 2.5 km horizontal grid cell size, over a 2250×3425 km2 domain covering western Canada and USA, prior to the use of the forecast system as part of the FIREX-AQ ensemble forecast. Several large forest fires took place in the Canadian portion of the domain during the study period. A feature of the implementation was the incorporation of a new online version of the Canadian Forest Fire Emissions Prediction System (CFFEPSv4.0). This inclusion of thermodynamic forest-fire plume-rise calculations directly into the online air-quality model allowed us to simulate the interactions between forest-fire plume development and weather.
Incorporating feedbacks resulted in weather forecast performance that exceeded or matched the no-feedback forecast, at greater than 90 % confidence, at most times and heights in the atmosphere. The feedback forecast outperformed the feedback forecast at 35 out of 48 statistical evaluation scores, for PM2.5, NO2, and O3. Relative to the climatological cloud condensation nuclei (CCN) and aerosol optical properties used in the no-feedback simulations, the online coupled model's aerosol indirect and direct effects were shown to result in feedback loops characterized by decreased surface temperatures in regions affected by forest-fire plumes, decreases in stability within the smoke plume, increases in stability further aloft, and increased lower troposphere cloud droplet and raindrop number densities. The aerosol direct and indirect effect reduced oceanic cloud droplet number densities and increased oceanic raindrop number densities, relative to the no-feedback climatological simulation. The aerosol direct and indirect effects were responsible for changes to the near-surface PM2.5 and NO2 concentrations at greater than the 90 % confidence level near the forest fires, with O3 changes remaining below the 90 % confidence level.
The simulations show that incorporating aerosol direct and indirect effect feedbacks can significantly improve the accuracy of weather and air-quality forecasts and that forest-fire plume-rise calculations within an online coupled model change the predicted fire plume dispersion and emissions, the latter through changing the meteorology driving fire intensity and fuel consumption.
- Article
(49180 KB) -
Supplement
(514 KB) - BibTeX
- EndNote
Atmospheric aerosol particles may be emitted (primary particles) or result from the condensation of the products of gas-phase oxidation reactions (secondary aerosol). With increasing transport time from emission sources, the processes of coagulation (colliding particles stick/adhere, creating larger particles) and condensation (low-volatility gases condense to particle surfaces) tend to result in particles which have a greater degree of internal mixing (internal homogeneous mixtures). Primary and near-source particles are more likely to have a single or a smaller number of chemical constituents (external mixtures).
Atmospheric particles also modify weather through well-established pathways. Under clear-sky conditions, the particles may absorb and/or scatter incoming light, depending on their size, shape, mixing state (internal, external, or combinations), and composition. The presence of the particles themselves may thus affect the radiative budget of the atmosphere, resulting in either positive or negative climate forcing (i.e., the absorption of a greater amount of incoming solar radiation versus increased scattering reflection of that radiation back out into space, a process known as the aerosol direct effect, ADE). Aerosols can also alter the atmospheric radiative balance through interactions with clouds, this influence being referred to as the aerosol indirect effect (AIE). Three broad classes of categories by which cloud–aerosol interactions take place (Oreopoulos et al., 2020) include the first indirect effect, whereby higher aerosol loadings result in increasing numbers of cloud droplets with smaller sizes, hence increasing cloud albedo (Twomey, 1977); the second indirect effect, whereby higher aerosol loadings suppress the collision–coalescence activity of the smaller droplets, reducing precipitation/drizzle, changing cloud heights, and changing cloud lifetime in warm clouds (Albrecht, 1989); and aerosol “invigoration” of storm clouds, whereby higher aerosol loadings may result in delayed glaciation of cloud droplets, in turn leading to greater latent heat release and stronger convection (Rosenfeld et al., 2008).
The uncertainties associated with the ADE and particularly AIE account for a large portion of the uncertainties in current climate model predictions for radiative forcing between 1750 and 2011 (Mhyre et al., 2013). Carbon dioxide is believed to have a positive (warming) global radiative forcing of approximately 1.88±0.20 W m−2, while the direct and indirect effects both have nominal values of approximately −0.45 W m−2, with uncertainty ranges encompassing −0.94 to +0.07 and −1.22 to 0.0 W m−2 respectively. These uncertainties have spurred research designed to better characterize the ADE and AIE and reduce these uncertainties, through both observations and atmospheric modelling.
Observational studies of the ADE have established its large impact; for example, high aerosol loading over Eurasian boreal forests has been found to double the diffuse fraction of global radiation (i.e., increased scattering), a change sufficient to affect plant growth characterized via gross primary production (Ezhova et al., 2018). Aerosol assimilation of Geostationary Ocean Color Imager aerosol optical depth (AOD) observations into a coupled meteorology–chemistry model showed that South Korean AOD values increased by as much as 0.15 with the use of assimilation; these increases corresponded to a local −31.39 W m−2 reduction in solar radiation received at the surface, and reductions in planetary boundary layer height, air temperature, and surface wind speed over land, and a deceleration of vertical transport (Jung et al., 2019). Other studies in East Asia have shown ADE decreasing local shortwave radiation reaching the surface by −20 W m−2 (Wang et al., 2016), as well as significant changes in surface particulate matter and gas concentrations in response to these radiation changes.
However, one commonality amongst the recent studies of the ADE for air-quality models is a tendency towards negative biases in predicted aerosol optical depths, potentially indicating systematic underpredictions in aerosol mass, aerosol size, and/or inaccuracies in the assumptions for shape and/or mixing state. Mallet et al. (2017) noted this negative bias for regional climate model AOD predictions associated with large California forest fires compared to OMI and MISR satellite observations. Palacios-Peña et al. (2018) noted that high AOD events associated with forest fires were underpredicted by most models in a study employing a multi-regional model ensemble. The chosen AOD calculation methodology and mixing state assumptions employed in models also play a role in systematic biases: Curci et al. (2015) compared aerosol optical depths, single scattering albedos, and asymmetry factors at different locations to observations, varying the source model for the aerosol composition, as well as the mixing state assumptions used in generating aerosol optical properties, for Europe and North America. AODs were biased low by a factor of 2 or more, regardless of model aerosol inputs or mixing state assumptions at 440 nm, and single scattering albedos were biased low by up to a factor of 2, with the poorest performance for “core-shell” approaches, while asymmetry factor estimates showed no consistent bias relative to observations. However, the assumed mixing state was clearly a controlling factor in the negative biases; the AOD predictions closest to the observations at 440 nm assumed an external mixture with particle sulfate and nitrate assumed to grow hygroscopically as pure sulfuric acid, lowering their refractive index with increasing aerosol size. This mixing state assumption and the different homogeneous mixture assumptions gave the best fit for single scattering albedo relative to observations. While not commenting on aerosol direct effect implications, Takeishi et al. (2020) noted that forest-fire aerosols increase particle number concentrations but reduce their water uptake (hygroscopicity) relative to anthropogenic aerosols, with the latter effect reducing the resulting cloud droplet numbers by up to 37 %. Mixing state and hygroscopicity properties of aerosols were thus shown to have a controlling influence on the ADE.
The AIE has often been shown to be locally more important for the radiative balance than ADE in terms of magnitude of the radiative forcing and response of predicted weather to AIE and ADE (Makar et al., 2015a; Jiang et al., 2015; Nazarenko et al., 2017). Several recent studies have attempted to characterize the relative importance of the AIE with the use of multi-year satellite observations, sometimes making use of models and data assimilation. Saponaro et al. (2017) used MODIS–Aqua-linked observations of aerosol optical depth and Ångström exponent to various cloud properties, noting that the cloud fraction, cloud optical thickness, liquid water path, and cloud top height all increased with increasing aerosol loading, while cloud droplet effective radius decreased, with the effects dominating at low levels (between 900 to 700 hPa). Zhao et al. (2018) examined 30 years of cloud and aerosol data (1981–2011) and found that increasing aerosol loading up to AOD < 0.08 increased cloud cover fraction and cloud top height, while further increases in aerosol loading (AOD from 0.08 to 0.13) resulted in higher cloud tops and larger cloud droplets. In polluted environments (AOD > 0.30), cloud droplet effective radius, optical depth, and water path increase; and cloud droplet effective radius increased with increasing AOD. The first ADE was most sensitive to AOD in the AOD range 0.13 to 0.30, and the reduction of precipitation efficiency associated with the second aerosol indirect effect occurred for AODs between 0.08 and 0.4, in oceanic areas downwind of continental sources.
However, sources of uncertainty in AIE estimates persist, in part due to the number of poorly understood processes contributing to the atmospheric response to the presence of aerosols. Nazerenko et al. (2017) showed that short-term atmospheric radiative changes were reduced in magnitude when sea-surface temperature and sea-ice coupling was included in climate change simulations. Suzuki and Takemura (2019) showed that the vertical structure of atmospheric aerosols, as well as their composition, had a significant influence on radiative forcing. Penner et al. (2018) and Zhu and Penner (2020) examined the impact of aerosol composition on cirrus clouds via ice nucleation, finding negative forcings for most forms of soot but a contrary impact of secondary organic aerosols. Rothenburg et al. (2018) noted that tests of aerosol activation schemes carried out under current climate conditions had little variability but had much greater variability for pre-industrial simulations, implying that the available data for evaluation using current conditions may poorly constrain ADE and AIE parameterizations used in simulating in past climates.
Forest fires are of key interest for improving the understanding and representation of ADE and AIE in models, due to the large amount of aerosols released during these biomass burning events. Forest-fire emissions and interactions with weather are also of interest due to the expectation that the meteorological conditions resulting in forest fires may become more prevalent in the future under climate change (Hoegh-Guldberg et al., 2018). Observations of aerosol optical properties during long-range transport events of North American forest-fire plumes to Europe showed 500 nm AOD values of 0.7 to 1.2 over Norway, with Ångström exponents exceeding 1.4 and absorbing Ångström exponents ranging from 1.0 to 1.25, along with single scattering albedos greater than 0.9 at the surface and up to 0.99 in the column over these sites (Markowicz et al., 2016). Biomass burning was shown to have a specific set of optical properties relatively independent of fuel type for three different types of biomass burning in China (cropland), Siberia (mixed forest), and California (needleleaf forest). The increase in upward radiative forcing at the top of the atmosphere was due to fires being linearly correlated to AOD (R from 0.48 to 0.68), with slopes covering a relatively small range from 20 to 23 W m−2 per unit of AOD. O'Neill et al. (2002) showed that forest fires have a profound impact on aerosol optical depth in western Canada, accounting for 80 % of the summer AOD variability in that region, with a factor of 3 increase in AOD levels from clear-sky to forest-fire plume conditions. The analysis of TOMS AVHRR and GOES imagery by O'Neill et al. (2001) suggested that forest-fire aerosols increase in size with increasing downwind distance, due to secondary aerosol ageing and condensation chemistry. We note here that reanalyzing the data presented in O'Neill et al. (2001) results in a linear relationship between fine-mode particle effective radius (reff, µm) and the base 10 logarithm of distance from the fires (D, km) of R2=0.18). Mallet et al. (2017) simulated AODs in the range 1 to 2 for biomass burning events and also noted changes in direct radiative forcing at the top of the atmosphere from positive to negative in both model results and simulations, with increasing downwind distance from the sources. Lu and Sokolik (2017) carried out simulations with 5 km horizontal grid spacings for the eastern Russia forest fires of 2002, assuming an internal mixture for emitted aerosols with the WRF-CHEM model, and noted impacts on cloud formation for two different periods. The first period was characterized by high cloud droplet and small ice nuclei numbers, where the fire plumes reduced cloud rain and snow water content, large-scale frontal system dynamics were altered by smoke, and precipitation was delayed by a day. The second period was characterized by high numbers for cloud droplets and ice nuclei, where the fire plumes reduced rain water content and increased snow water content, and precipitation locations changed locally across the simulation domain. Russian forest-fire simulations for 2010 with suites of online coupled air-quality models (Makar et al. (2015a, b); Palacios-Peña et al., 2018; Baró et al., 2017) showed substantial local impacts, such as reductions in average downward shortwave radiation of up to 80 W m−2 and in temperature of −0.8 ∘C (Makar et al., 2015a).
Given the above developments in direct and indirect parameterizations, and the increasing amount of information available for estimating forest-fire emissions, the impact of forest fires on weather, in the context of weather forecasting, is worthy of consideration. Air-quality model predictions of forest-fire plumes have been provided to the public under operational forecast conditions of time- and memory-space-limited computer resources (e.g., Chen et al., 2019; James et al., 2018; Ahmadov et al., 2019; Pan et al., 2017). These simulations make use of satellite retrievals of forest-fire hotspots, climatological data on the extent of area burned by land use type, databases of fuel type linked to emission factors, and an a priori weather forecast to provide the meteorological inputs required to predict forest-fire plume rise. The latter point is worthy of note in the context of the direct and indirect feedback studies noted above – both climate and weather simulations with prescribed forest-fire emissions have consistently resulted in large perturbations of weather patterns in the vicinity of the forest fires. However, their approaches for predicting forest-fire plume rise and fire intensity and fuel consumption in operational regional-scale forecasts up until now have relied on weather forecast information provided a priori and are hence lacking those meteorological feedback effects.
The connection of the ADE and AIE within a regional air-quality and weather forecast model context is referred to as “coupling”, with such a model being described in that body of literature as “online coupled” (Galmarini et al., 2015) or “aerosol-aware” (Grell and Freitas, 2014). However, several researchers have examined aerosol–radiative coupling along with fire spread and growth (as opposed to fire intensity and fuel consumption). The latter work employs very high-resolution forest-fire spread and growth models, and due to their very high resolution, an additional level of coupling, that of interaction of dynamic meteorology with the heat released by the fire, may be included. However, the resolution requirements for these models (and their need for a relatively small computational time step) constrain their application to a relatively small region. A requirement for these approaches is the use of a very high-resolution fire growth model imbedded within the air-quality model. At these resolutions, the simulated local-scale meteorology determines fire spread on the landscape, which in turn modifies the temperature and wind fields, in turn affecting future fire spread. The seminal work on this topic was carried out by Clark et al. (1996) and Linn et al. (2002). More recent work includes the development of the WRF-FIRE model (Mandel et al., 2011; Coen et al., 2013), with full chemistry added in the WRFSC model (Kochanski et al., 2016). Examples of the resolution required for these models include inner domain resolutions of 444 m, with an imbedded fire model mesh of 22.2 m resolution and a time step of 3.3 s (Kochanski et al., 2016); 1.33 km, with an imbedded fire model mesh of 67.7 m and a time step of 2 s (Kochanski et al., 2019), and 222 m, with a fire model mesh of 22 m and a time step of 2 s (Peace et al., 2015). Kochanski et al. (2016) also noted a 13 to 30 h computational time requirement to run their high-resolution modelling system. These modelling efforts allow for this additional level of coupling – but at the expense of additional computation time, preventing, at the current state of supercomputer processing, their application on synoptic-scale forecast domains combined with a full gas chemistry and size-resolved multi-component particle chemistry representation. Here we explore the effects of fire emissions characterized by fire intensity and fuel consumption modelling on the aerosol direct and indirect effects over synoptic-scale domain. Our coupling refers to that between the aerosols released by fires and other sources to meteorology through the ADE and AIE, with the resulting changes in meteorology in turn influencing fire intensity and fuel consumption, which in turn influence plume rise, emissions height, and distribution, closing this feedback loop. We do not implement a very high-resolution growth model, noting that this is impractical for operational forecasts at the current time, while showing that synoptic-scale 2.5 km simulations incorporating fire feedbacks may be carried out within an operational window with currently available supercomputers. As shown below, we find that a sufficiently substantial feedback between the aerosol direct and indirect effects can be discerned to change the vertical distribution of emitted pollutants.
A key consideration in parameterizing the AIE (via aerosol–cloud interaction) is the manner in which the cloud condensation process is represented in the meteorological component of the modelling system. In numerical weather prediction (NWP) models, clouds and precipitation are represented by a combination of physical parameterizations that are each targeted at a specific subset of moist processes. These include “implicit” (subgrid-scale) clouds generated by the boundary layer and the convection parameterization schemes (e.g., Sundqvist, 1988) and “explicit” clouds from the grid-scale condensation scheme (Milbrandt and Yau, 2005a, b; Morrison and Milbrandt, 2015; Milbrandt and Morrison, 2016). Depending on the model grid these “moist physics” schemes vary in their relative importance.
However, regardless of the horizontal grid cell size, the grid-scale condensation scheme plays a crucial role in atmospheric models, though to different degrees and using different methods, depending on the grid spacing and the corresponding relative contributions of the implicit schemes. A grid-scale condensation scheme will in general consist of the following three components: (1) a subgrid cloud fraction parameterization (CF, or cloud “macrophysics” scheme); (2) a microphysics scheme; and (3) a precipitation scheme (Jouan et al., 2020). The cloud fraction (CF) is the percentage of the grid element that is covered by cloud (and is saturated), even though the grid-scale relative humidity may be less than 100 %. The microphysics parameterization computes the bulk effects of a complex set of cloud microphysical processes. If precipitating hydrometeors are advected by the model dynamics, the precipitation is said to be prognostic; if precipitation is assumed to fall instantly to the surface upon production, it is considered diagnostic. The precipitation “scheme” is not a separate component per se, since it simply reflects the level of detail in the microphysics parameterization, but it is a useful concept to facilitate the comparison of different grid-scale condensation parameterizations.
With a wide range of grid cell sizes in current NWP models, there is a wide variety of types of condensation schemes and degrees of complexity in their various components. For example, cloud-resolving models (with grid spacing on the order of 1 km or less) have typically used detailed bulk microphysics schemes (BMSs), with prognostic precipitation, and no diagnostic or prognostic CF component (i.e., the CF is either 0 or 1). Large-scale global models use condensation parameterizations, sometimes referred to as “stratiform” cloud schemes, typically with much simpler microphysics and diagnostic precipitation but with more emphasis on the details of the CF. However, with continually increasing computer resources and decreasing grid spacing (both in research and operational prediction systems), the distinction between schemes designed for specific ranges of model resolutions is disappearing, and condensation schemes are being designed or modified to be more versatile and usable across a wider range of model resolutions (e.g., Milbrandt and Morrison, 2016).
Aerosol–cloud interactions and feedback mechanisms are difficult to represent in grid-scale condensation schemes with very simple microphysics components. For example, to benefit from the predicted number concentrations of cloud condensation nuclei (CCN) and ice nuclei, the microphysics needs to be double-moment (predicting both mass and number) for at least cloud droplets and ice crystals, respectively. Until recently, detailed BMSs were only used at cloud resolving scales, hence requiring these relatively high resolutions to be recommended in feedback modelling. In recent years, multi-moment BMSs have been used in operational NWP for model grid spacings of 2–4 km (e.g., Seity et al., 2010; Pinto et al., 2015; Milbrandt et al., 2016). Further, condensation schemes with detailed microphysics are starting to use non-binary CF components (e.g., Chosson et al., 2014; Jouan et al., 2020), thereby allowing detailed microphysics to be used at larger scales and hence allowing the same indirect feedback parameterizations to be used at multiple scales. Nevertheless, the expectation is that detailed parameterization will provide a more accurate representation of cloud formation at the near cloud-resolving scales, without the complicating aspect of a diagnostic CF, motivating the use of kilometre-scale grid spacing for feedback studies.
The formation of secondary aerosols from complex chemical reactions is another key consideration in feedback forecast implementation, given the impact of aerosol composition on aerosol optical and cloud formation properties, as described above.
In the sections which follow, we describe our high-resolution, online coupled air-quality model with online forest-fire plume-rise calculations, which was created as part of the FIREX-AQ air-quality forecast ensemble (https://www.esrl.noaa.gov/csl/projects/firex-aq/, 17 June 2021), to address the following questions:
- 1.
Will an online coupled model of this nature provide improved forecasts of both weather and air quality, using standard operational forecast evaluation tools, techniques, and metrics of forecast confidence? That is, despite the uncertainties in the literature as described above, are these processes sufficiently well described in our model that their use results in a formal improvement in forecast accuracy?
- 2.
Are the changes in forest-fire plume rise associated with implementing this process directly within an online coupled model sufficient to result in significant perturbations to weather predictions and to chemistry? What are these perturbations?
We employ our online coupled model with a 2.5 km grid cell size domain covering most of western North America and compare model results to surface meteorological and chemical observations and to vertical column observations of temperature and aerosol optical depth (AOD), in order to quantitatively evaluate the effect of feedback coupling of the ADE and AIE on model performance. We then compare feedback and no-feedback simulations to show the impacts of the ADE and AIE feedbacks on cloud and other meteorological predictions and on key air-quality variables (particulate matter, nitrogen dioxide, and ozone). We begin our analysis with a description of our modelling platform.
2.1 GEM-MACH
The Global Environmental Multiscale – Modelling Air-quality and CHemistry (GEM-MACH) model in its online coupled configuration has been described elsewhere (Makar et al., 2015a, b; Gong et al., 2015, 2016). The model combines the Environment and Climate Change Canada Global Environmental Multiscale weather numerical weather prediction model (GEM; Cote et al., 1998; Girard et al., 2014) with gas and particle process representation using the online paradigm, with options for climatological versus full coupling between meteorology and chemistry. GEM-MACH's main processes for the two configurations employed here are described in Table 1.
Simulations were carried out with a 2.5 km horizontal grid cell spacing over a 900×1370 grid cell domain, covering most of western Canada and the USA (Fig. 1). The meteorological boundary conditions for the simulation were a combination of 10 km resolution GEM forecasts updated hourly (themselves originating in data assimilation analyses of real-time weather information; Fig. 1a) and 2.5 km GEM simulations (Fig. 1c) employing, in the northern portion of this 2.5 km domain, the Canadian Land Data Assimilation System (Carrera et al., 2015), to better simulate surface conditions. Both “feedback” and “no-feedback” simulations were carried out on a 30 h forecast cycle (Fig. 2). Following the usual practice for weather forecasts, the analysis-driven meteorological forecasts at 10 km resolution were updated operationally every 24 h at 12:00 UT (Fig. 2a). These 10 km resolution weather forecasts were used to drive a 30 h, 10 km resolution GEM-MACH forecast (Figs. 1b and 2b), which employed ECMWF reanalysis data for North American chemical lateral conditions (Innes et al., 2019). The 10 km resolution weather forecasts were also used to drive a 30 h meteorology-only forecast at 2.5 km resolution on the high-resolution domain (Figs. 1c and 2c). The last 24 h of the 10 km resolution GEM-MACH forecast was also used to provide chemical lateral boundary conditions for the 24 h 2.5 km online coupled GEM-MACH simulation (Figs. 1c and 2d). The last 24 h of the 2.5 km GEM simulation was used as meteorological initial and boundary conditions for the 24 h 2.5 km online coupled GEM-MACH simulation (Figs. 1c and 2d). The two stages of meteorology-only simulations were carried out to prevent chaotic drift from the observed meteorology and to allow spin-up time for the cloud fields of that meteorology to reach equilibrium (6 h timeframe). Chemical initial concentrations for each consecutive forecast within the 2.5 km GEM-MACH model domain were “rolled over” or “daisy-chained” between subsequent forecasts without chemical data assimilation. Forecast performance scores presented here are for the inner 2.5 km domain from this set of linked 24 forecast simulations, mimicking operational forecast conditions.
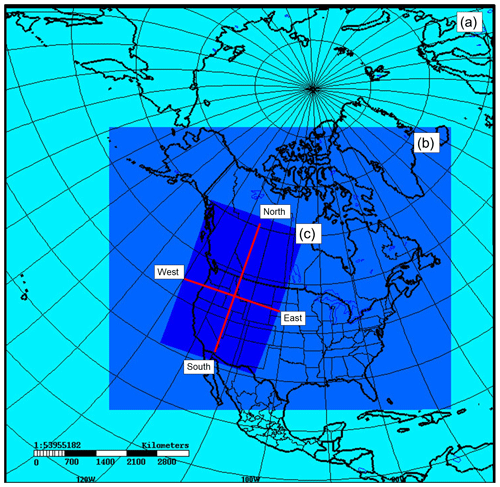
Figure 1GEM-MACH domains: (a) GEM meteorology 10 km resolution forecast domain. (b) GEM-MACH 10 km resolution forecast domain. (c) GEM-MACH inner 2.5 km grid resolution forecast domain for comparison to observations. Red lines indicate locations of illustrative south–north and west–east cross sections appearing in subsequent analysis in the text.
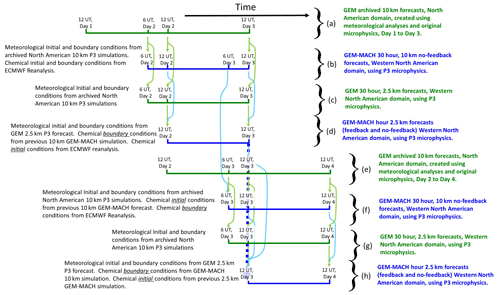
Figure 2Example time sequencing of model simulations used to generate the 2.5 km GEM-MACH simulations carried out here. Green lines and print indicate GEM (weather forecast only) simulations), and blue lines and print indicate 2.5 km GEM-MACH simulations. Arrows indicate data flow (light green: meteorological information; light blue: chemical information). Steps (a) through (h) illustrate the sequence of forecasts used to generate 2 consecutive days of 2.5 km GEM-MACH simulations. Note that online coupling occurs only at the 2.5 km GEM-MACH forecast level, in this sequencing.
2.2 CFFEPS version 4.0: online forest-fire plume-rise calculations
In addition to the above algorithm improvements relative to GEM-MACH implementations, this model system setup has incorporated the first online calculation of forest-fire plume rise by energy balance driven using online meteorology, in a new version of the Canadian Forest Fire Emissions Prediction System (CFFEPS). The algorithms of CFFEPSv2.03 are described in detail and evaluated elsewhere (Chen et al., 2019) but will be outlined briefly here, as well as subsequent modifications to this forest-fire emissions processing module.
CFFEPS combines near-real-time satellite detection of forest-fire hotspots with national statistics of burn areas by Canadian province and by specific fuel type across North America. CFFEPS assumes persistence fire growth in the subsequent 24 to 72 h forecasts with hourly fuel consumed calculated (kg m−2), based on GEM forecast meteorology and predicted fire intensity and fuel consumption in grid cells representing fire locations. The modelled fire fuel consumption is then linked with combustion-phase specific emission factors (g kg−1) for fire specific emissions and chemical speciation. Fire energy associated with the modelled combustion process is also estimated and is used in conjunction with a priori forecasts of meteorology within the column to determine plume rise. In its offline/non-coupled configuration (Chen et al., 2019), CFFEPS carries out residual buoyancy calculations at five preset pressure levels (surface, 850, 700, 500, 250 mb). CFFEPS predicts plume injection heights, which are in turn used to redistribute the mass emissions below the plume top to the model hybrid levels. This approach employed in CFFEPSv2.03 provided a substantial improvement in forecast accuracy relative to the previous approach employing modified Briggs (Briggs, 1965, 1984; Pavlovic et al., 2016) plume-rise formulae in the offline GEM-MACH forecast system (Chen et al., 2019). A recent evaluation of the plume heights predicted by CFFEPS was carried out utilizing MISR and TROPOMI satellite retrieval data (Griffin et al., 2020). A total of 70 cases studied using MISR data showed good agreement between satellite and CFFEPS-predicted maximum and mean plume heights (maximum plume height observed versus predicted values and standard deviations: 1.7±0.9 versus 2.0±1.0 km; mean plume height observed versus predicted: 1.3±0.6 versus 1.3±0.4 km). A larger number of case studies using TROPOMI data (671 in total) also showed a reasonable agreement, with CFFEPS showing a small tendency to overpredict heights (maximum observed versus predicted plume heights 2.2±1.6 versus 2.5±1.2 km; mean observed versus predicted plume heights 0.7±0.5 versus 1.1±0.6 km).
However, other work has shown the substantial impact of large forest fires on regional weather (Makar et al., 2015a; Palacios-Peña et al., 2018; Baró et al., 2017), including changes to the surface radiative balance and atmospheric stability. These findings imply that plume-rise calculations employing an a priori weather forecast lacking the impact of fire plumes via the ADE and AIE may not accurately predict the weather conditions critical to subsequent forest-fire plume-rise prediction. In order to study this possibility, and to allow forest-fire plumes to influence weather and hence subsequent fire spread/growth, several changes were made to the CFFEPS implementation, resulting in version 4.0 of CFFEPS, used here. The process flow within CFFEPSv2.03 versus CFFEPSv4.0 is compared in Fig. 3. The original C language CFFEPSv2.03 code was converted to FORTRAN90 and, following successful offline comparisons to the original code, was then integrated as an online subroutine package within GEM-MACH itself, with the near-real-time satellite hotspot data and location fuel parameters being read into GEM-MACH directly (CFFEPSv4.0 is this new online package). A key advantage of the CFFEPSv4.0 subroutine integration within GEM-MACH is that the residual buoyancy calculations for plume injection heights are now carried out over the model hybrid model layers, rather than the five coarse-resolution, prescribed pressure levels of CFFEPSv2.03, making complete use of GEM-MACH's detailed vertical structure. Additionally, CFFEPSv4.0 allows plume-rise calculations to be updated during model runtime. When GEM-MACH is run in online coupled mode, the ADE and AIE implementations allow model-generated aerosols to modify the predicted meteorology, in turn influencing predicted fire emissions and plume rise, closing these feedback loops. The online implementation of CFFEPSv4.0 thus allows us to investigate the effects of meteorology on subsequent forest-fire plume development, the changes to modelled aerosol compositions, and, ultimately, the feedbacks to weather.
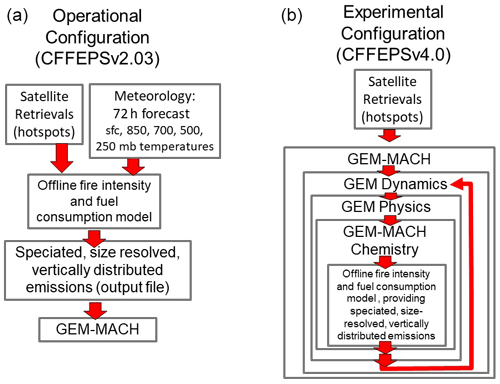
Figure 3Process comparison between original (CFFEPSv2.03, a) and online (CFFEPSv4.0, b) forest-fire emissions and vertical plume distribution algorithms.
The formation of particles from forest fires affects meteorology on the larger scale via the ADE and AIE, in turn modifying the regional-scale atmospheric features affecting fire growth, such as the temperature profiles below forest-fire plumes. However, we note that CFFEPSv4.0 employs forest fire heat to determine plume rise as a subgrid-scale thermodynamic process parameterization rather than a very high-resolution explicit fire growth parameterization; the very local-scale weather modifications due to the addition of forest-fire heat to the atmosphere are not incorporated into fire spread or GEM microphysics. Specifically, when the feedback version of GEM-MACH incorporating CFFEPSv4.0 is used in its online coupled configuration, CFFEPSv4.0 uses estimates of the heat released to calculate forest-fire plume rise. These calculations employ lapse rates at the fire locations, that with feedbacks enabled, include the ADE and AIE generated by forest-fire aerosols on atmospheric stability within the current online coupled model time step. This is in contrast to earlier offline implementations of CFFEPS, which made use of a priori non-feedback weather forecast lapse rates. To the best of our knowledge, this is the first implementation of a dynamic forest-fire plume injection height scheme incorporated into an online coupled high-resolution, operational air-quality forecast modelling system. The impact of this feedback on both weather and air-quality can be substantial, as we show in the following sections.
The locations of the daily forest hotspots detected during the study period and the corresponding magnitude of the daily PM2.5 emissions generated by CFFEPS for each hotspot are shown in Fig. 4. Individual hotspots with the highest magnitude emissions are located in the state of Nevada (Fig. 4a, southern boxed region). However, the largest ensemble emissions from a suite of hotspots occur in northern Alberta (Fig. 4a, northern boxed region). Expanded views of the northern Alberta and Nevada hotspots are shown in Fig. 4b and c respectively – the use of smaller symbols shows that the Alberta hotspots are groups representing large spreading fires, which are overplotted in Fig. 4a, while the Nevada hotspots indicate single fires of small spatial extent and duration rather than larger spreading fires. The Alberta fires are thus the most significant sources of forest-fire emissions in the study domain for the period analyzed here.
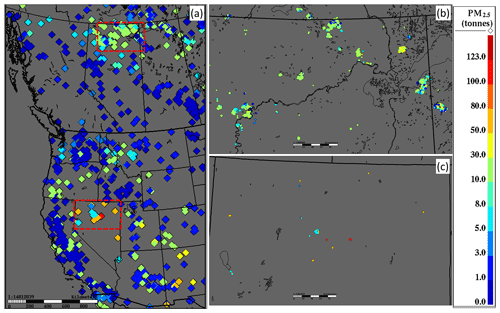
Figure 4Hotspot locations during the study period, colour-coded by daily total tonnes PM2.5 emitted. (a) Entire model 2.5 km domain, with northern Alberta and northern Nevada subregions as dashed red boxes; (b) close-up of northern Alberta, with smaller symbols for individual hotspots showing the large fire regions; (c) close-up of northern Nevada, to the same scale as (b), showing isolated hotspots with high emissions.
2.3 Feedback and no-feedback simulations
Two simulations were carried out for the period 4 July through 5 August 2019; a feedback (ADE and AIE feedbacks enabled – online coupled model) and a no-feedback simulation (ADE and AIE make use of GEM's climatological aerosol radiative and CCN properties – the one-way coupled model). During this period, five large forest fires took place in the northern portion of the modelling domain. The two parallel combined meteorology and air-quality forecasts in the online coupled model with/without ADE and AIE coupling were evaluated for meteorological and air-quality variables. Following evaluation, the simulation mean values of hourly meteorological and chemical tracer predictions were compared to analyze the impact of online coupled ADE and AIE feedbacks on both sets of fields.
3.1 Meteorology evaluation
Surface meteorological conditions were evaluated at 3 h intervals from the start of both of the two sets of paired 24 h forecasts using standard metrics of weather forecast performance, including mean bias (MB), mean absolute error (MAE), root mean square error (RMSE), correlation coefficient (R), and standard deviation (σ). In all comparisons, a 90 % confidence level assuming a normal distribution was used to identify statistically different results between forecast simulations. Note that 90 % confidence levels are commonly used in meteorological forecast evaluation, with values of 80 % to 85 % recommended (Pinson and Kariniotakis, 2004) and up to 90 % used (Luig et al., 2001) for variables such as wind speed, rather than the 95 % or 99 % confidence levels in other fields, in recognition of the difficulties inherent in prognostic forecasts of the chaotic weather system. Here, the confidence range formulation of Geer (2016) has been applied using a 90 % confidence level in model predictions, with the statistical measures considered different at the 90 % confidence level when the 90 % confidence ranges do not overlap. The surface meteorological evaluations shown here only include those variables and metrics for which results were significantly different at the 90 % confidence level.
Several model forecast output variables were evaluated, and the surface variables showing statistically significant differences relative to observations at the 90 % confidence level included 2 m temperature, surface pressure, 2 m dew-point temperature, 10 m wind speed, sea-level pressure, and accumulated precipitation (the latter in three different metrics). The comparisons are shown as time series in 3-hourly intervals as a function of forecast hour prediction time forward from forecast hour 0, for grid cells corresponding to measurement locations in Figs. 5–11 for each of these quantities, respectively. Note that these statistics measure domain-wide performance, across all of the reporting stations within the model domain, during the sequence of 24 h forecasts comprising the simulation period. The duration of the time series in these comparison figures is thus a function of the duration of the contributing forecasts.
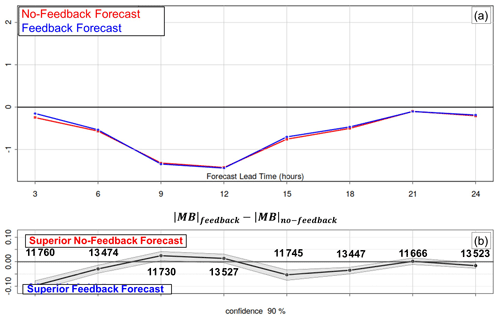
Figure 5Mean bias in surface temperature (∘C) at forecast hours starting at 00:00 UT. (a) Red line: no-feedback forecast values; blue line: feedback forecast values. (b) Difference in absolute value of mean bias between the two forecasts , with the region below 90 % confidence level shown shaded grey. Mean values above and below the “0” line and outside of the shaded region thus indicate differences in the mean between the two forecasts which differ at or above the 90 % confidence level. Values of the difference which appear below and above the zero line and outside of the grey area thus indicate superior domain average performance for the feedback/no-feedback forecasts at each of the 3-hourly intervals, respectively. Numbers appearing above the metric differences are the number of observations contributing to the calculated metrics.
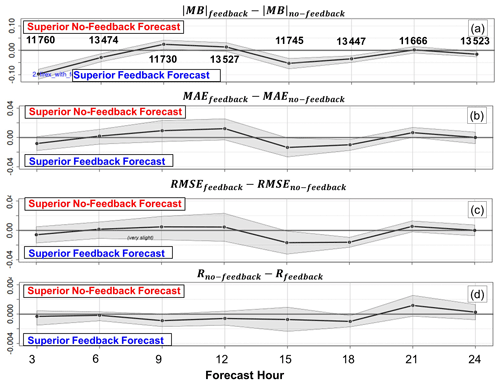
Figure 6Summary meteorological performance comparison for surface temperature (∘C). (a) Mean bias, (b) mean absolute error, (c) root mean square error, and (d) Pearson correlation coefficient. The 90 % confidence level is shown in grey. Numbers appearing above the absolute mean bias differences are the number of stations contributing to the calculated metrics.
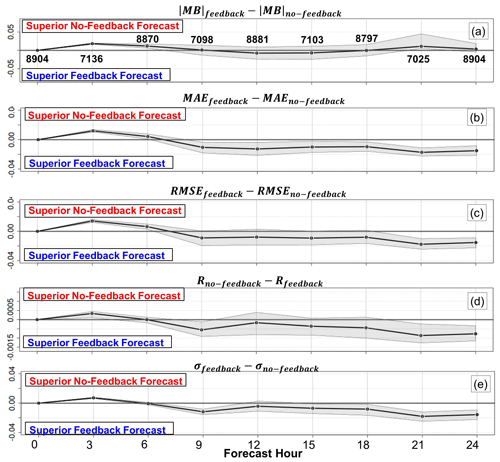
Figure 7Summary meteorological performance comparison for surface pressure (hPa). (a) Mean bias, (b) mean absolute error, (c) root mean square error, (d) Pearson correlation coefficient, and (e) standard deviation. The 90 % confidence level is shown in grey. Numbers appearing above the absolute mean bias differences are the number of stations contributing to the calculated metrics.
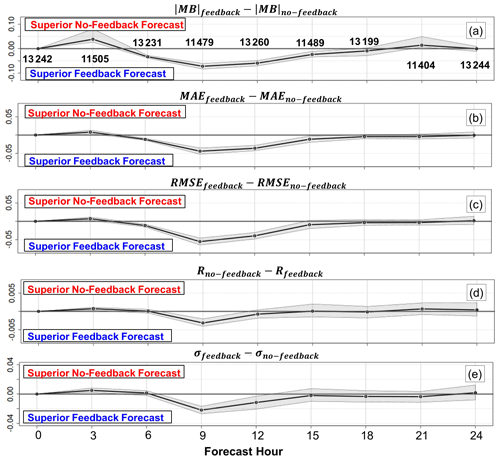
Figure 8Summary meteorological performance comparison for dew-point temperature (∘C). (a) Mean bias, (b) mean absolute error, (c) root mean square error, (d) Pearson correlation coefficient, and (e) standard deviation. The 90 % confidence level is shown in grey. Numbers appearing above the absolute mean bias differences are the number of stations contributing to the calculated metrics.
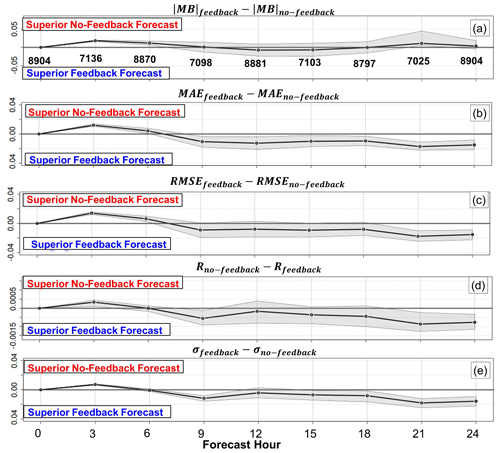
Figure 9Summary meteorological performance comparison for sea-level pressure (hPa). (a) Mean bias, (b) mean absolute error, (c) root mean square error, (d) Pearson correlation coefficient, and (e) standard deviation. The 90 % confidence level is shown in grey. Numbers appearing above the absolute mean bias differences are the number of stations contributing to the calculated metrics.
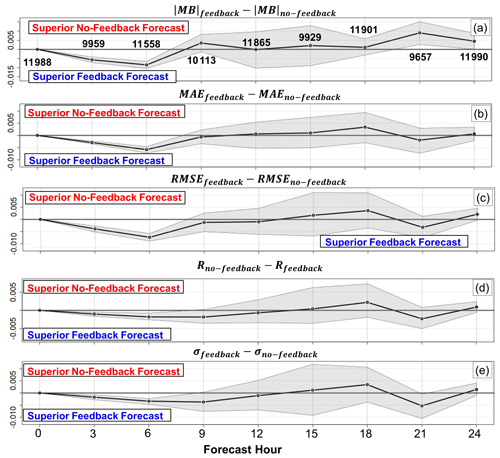
Figure 10Summary meteorological performance comparison for 10 m wind speed (m s−1). (a) Mean bias, (b) mean absolute error, (c) root mean square error, (d) Pearson correlation coefficient, and (e) standard deviation. The 90 % confidence level is shown in grey. Numbers appearing above the absolute mean bias differences are the number of stations contributing to the calculated metrics.
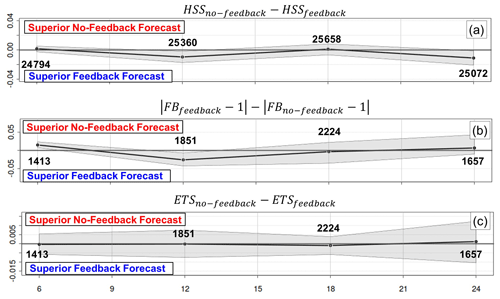
Figure 11Precipitation performance evaluation (mm precipitation). (a) Heidke skill score of 6 h accumulated precipitation (no-feedback–feedback). (b) Frequency bias index of 6 h accumulated precipitation (threshold of 2 mm, no-feedback–feedback). (c) Equitable threat score of 6 h accumulated precipitation (threshold of 2 mm, no-feedback–feedback).
Figure 5 shows an example analysis for surface temperature bias for the entire model domain. Figure 5a shows the average model mean bias (MB) time series across all stations and all forecasts at the given forecast hours, while Fig. 5b shows the corresponding difference in the MB absolute values. The difference plot in Fig. 5b shows the feedback–no-feedback scores, such that scores below the zero line indicate superior performance of the feedback forecast, while those above the zero line indicate superior performance of the no-feedback forecast. Here, the feedback forecast was statistically superior at forecast hours 3, 6, 15, 18, and 24 at the 90 % confidence level at these forecast hours, and both simulations were at par (differences below the 90 % confidence level) at hours 12 and 21, with the no-feedback forecast being superior at 90 % confidence at hour 9. The feedback forecast thus has superior performance, at greater than 90 % confidence, over half of the forecast hours evaluated within the domain, equivalent performance at 2 h (hours 12 and 21, both within 90 % confidence limits), and inferior performance at 1 h (hour 9), during the simulation period.
All of the metrics for which surface temperature forecast performance differed at the 90 % confidence level are shown in Fig. 6. In addition to MB, the scores for MAE and RMSE showed superior forecast performance for the feedback relative to the no-feedback case at the 90 % confidence level for hours 15 and 18, while the improvement for the correlation coefficient only reached the 90 % confidence level at hour 18.
The meteorological forecast performance metrics with statistically significant differences for surface pressure, dew-point temperature, and sea-level pressure are shown in Figs. 7–9 respectively. The model performance differences in these three figures show a similar pattern: a degradation in performance with the use of feedbacks at hour 3, with the differences between the two forecasts either dropping below the 90 % confidence level, or the feedback forecast showing an improvement by hour 9, followed by several hours in which the feedback forecast has a superior performance, usually at greater than 90 % confidence. The duration of this latter period varies between the metrics, from up to 18 h for MAE for surface pressure (Fig. 7b) to 3 h for the correlation coefficient of dew-point temperature (Fig. 8d).
The initial loss of performance for the feedback forecast may represent a form of “model spin-up” that may be unique to online coupled models but may be affected or improved with further adjustments to the forecast cycling setup for the chemical species. As noted earlier (Fig. 2), in order to prevent chaotic drift from observed meteorology, we made use of a 30 h 2.5 km resolution analysis-driven weather forecast to update our online coupled model's initial meteorology at hour zero of each 24 h forecast. The cloud fields provided as initial conditions at hour zero include observation analysis for the 6 h prior to hour zero – these have reached a quasi-equilibrium in the high-resolution weather forecast (Fig. 2b and e) by the time they are used as initial and boundary conditions in the online coupled model (Fig. 2c and f). However, the online coupled model's aerosol fields at hour zero, used to initialize the subsequent forecast (Fig. 2, dashed blue arrow), still reflect the locations of aerosol–cloud interactions in the previous online coupled simulation. The initial 3 to 6 h of feedback forecast degradation represents the time required for the online coupled model to reach a new equilibrium consistent between both its aerosol and the cloud fields.
One possible solution for this model spin-up inconsistency would be to eliminate the intermediate driving 2.5 km meteorological simulation in favour of a longer 30 h online coupled forecast with the first 6 h removed as spin-up (i.e., extend the duration of steps c and f in Fig. 2 to 30 h, starting at UT hour 6). The duration of the forecast experiments carried out here was limited to 24 h due to limited computational resources and, more importantly, to the operational requirement for an on-time forecast delivery for the purpose of the FIREX-AQ field campaign. The 24 h forecast simulations carried out in Fig. 2c and f each required nearly 3 h of supercomputer processing time; longer simulation periods were not possible within the operational window available for forecasting.
Model 10 m wind speed forecasts were also improved with the incorporation of feedbacks for hours 3 and 6, for all metrics (Fig. 10). A decrease in MB performance at hours 21 and 24 can also be seen in this figure.
Precipitation forecast performance from the two simulations varied depending on the metric chosen (Fig. 11). The metrics in this case were based on the number of coincident precipitation “events” versus “non-events” as shown in Table 2.
Table 2Event versus non-event contingency table. A is the number of events forecast and observed; B is the number of events forecast but not observed; C is the number of events observed but not forecast; D is the number of cases where events were neither forecast nor observed.
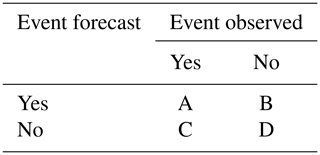
The Heidke skill score measures the fractional improvement of the forecast over the number correct by chance. The frequency bias measures the frequency of event over-forecasts (FB > 1) versus event under-forecasts (FB < 1). The equitable threat score , where measures the observed and/or forecast events that were correctly predicted. Following standard practice at Environment and Climate Change Canada, the HSS is used as a measure of total precipitation accumulated over a 6 h interval, with no lower limit on the amount of precipitation defining an event, while FB and ETS define precipitation events as being those with greater than 2 mm per 6 h interval – consequently FB and ETS have a smaller number of data points for comparison than HSS.
Figure 11 shows that improvements to the online coupled precipitation forecast at the 90 % confidence level were seen for the HSS 6 h accumulated metric at hours 12 and 24, while the frequency bias index of 6 h accumulated precipitation showed degradation at hours 6 and improved performance at hour 12, and the equitable threat score of 6 h accumulated precipitation showed significant differences at 90 % confidence between the two simulations. As is noted above, the latter two metrics employed a minimum 6 h precipitation threshold of 2 mm prior to comparisons (this is the reason for the reduced number of points available for comparison in Fig. 11b and c relative to Fig. 11a). These findings suggest that the online coupled model's improvements for total precipitation (Fig. 11a) are the result of slightly improved performance for relatively light precipitation events (<2 mm 6 h−1).
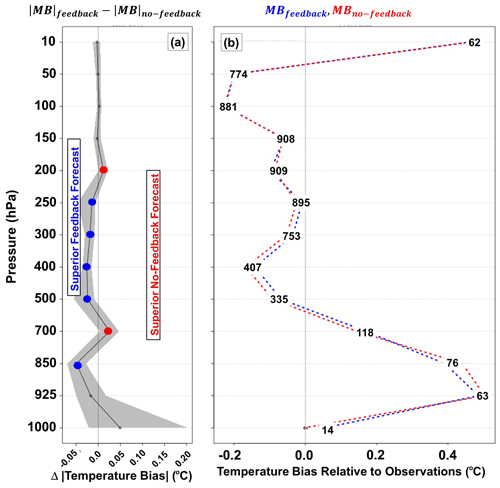
Figure 12Forecast hour 12 (00:00 UT) summary upper air temperature performance comparison for air temperature (mean bias, ∘C). (a) Difference in absolute value of mean bias in temperature (feedback forecast–no-feedback forecast). Grey regions represent 90 % confidence levels, and blue symbols show pressure levels at which the feedback mean bias outperforms the no-feedback mean-bias at >90 % confidence. Red symbols show pressure levels at which the no-feedback mean bias outperforms the feedback mean bias at >90 % confidence. The 90 % confidence level is shown in grey. (b) Mean bias in upper air temperature for feedback (blue) and no-feedback (red) (∘C). Numbered values on the profiles indicate the number of observed data–model pairs at each pressure level.
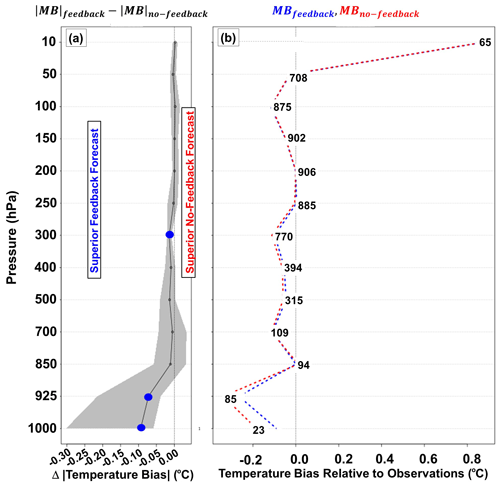
Figure 13Forecast hour 24 (12:00 UT) summary upper air temperature performance comparison for air temperature (mean bias, ∘C). (a, b) as in Fig. 12.
The amalgamated observations and model pairs of vertical temperature profile data from 39 radiosonde sites in western North America are shown in Figs. 12 and 13. Improvements in the forecasted temperature vertical profile with increasing forecast time are evident at 250, 300, 400, 500, and 850 hPa in the 12th hour forecast, with degradations at 200 and 700 hPa (Fig. 12). Improvements at 300, 925, and 1000 hPa may be seen in the 24th hour (Fig. 13) forecast; it is also worth noting that the entire region at and below 300 hPa has improved temperature forecasts (mean values to the left of the vertical line), albeit not always at >90 % confidence. There are larger differences between the 1000 hPa forecasts, though these also have the least number of contributing stations (i.e., only those located close to sea level contribute to the lowest level temperature biases). Other levels of the atmosphere showed no statistically significant change at the 90 % confidence level in temperature profile forecast performance with the use of feedbacks.
3.2 Chemistry evaluation
Improvements to air-quality model performance metrics have been a focus for research since the 1980s, starting with dispersion model evaluation (Fox, 1981) and the identification of mean bias and normalized mean square error as potentially useful metrics to complement the Pearson correlation coefficient (Hanna, 1988). More recently, the Pearson correlation coefficient has been noted as being capable of producing high values for relatively poor model results (Krause et al., 2005), as well as being unable to distinguish systematic model underestimation (Yu et al., 2006), unable to provide information on whether data series have a similar magnitude, and capable of providing a false sense of relationship where none exists due to outliers (Duveiller et al., 2016) and clusters of model–observation pairs (Aggarwal and Ranganathan, 2016). More recently, model evaluation has focused on metrics which do not have the tendency to weight the higher magnitude values unduly (a particularly useful property with air-quality variables, which may vary by several orders of magnitude), which are dimensionless (allowing a comparison across different evaluated variables) and which are bounded and symmetric (properties allowing comparisons to be made and equally valued across the entire range of possible concentrations; e.g., Yu et al., 2006). Metrics such as the modified coefficient of efficiency (Legates and McCabe, 1999) and the more recent incarnations of the index of agreement (Willmott et al., 2012) are examples of the more recent metrics used for air-quality model evaluation. Here, we have made use of a range of metrics spanning the literature on this topic, with the understanding that the properties of different metrics vary, that no single metric provides a perfect means of evaluating model performance, and that a variety of metrics should be applied. The metrics used here span the variety that have appeared in the literature since the early 1980s and include the factor of 2, mean bias, mean gross error, normalized mean gross error, correlation coefficient, root mean square error, coefficient of efficiency, and index of agreement. The formulae for these metrics and a brief description of their relative advantages and disadvantages appear in Sect. S1 and Table S1 in the Supplement.
Table 3Summary performance metrics for ozone, nitrogen dioxide, and PM2.5. Boldface indicates the simulation with the better performance score for the given metric, chemical species, and subregion, italics indicate a tied score, and regular font indicates the simulation with the lower performance score. FO2: fraction of scores within a factor of 2. MB: mean bias. MGE: mean gross error. NMGE: normalized mean gross error. R: correlation coefficient. RMSE: root mean square error. COE: coefficient of error. IOA: index of agreement.
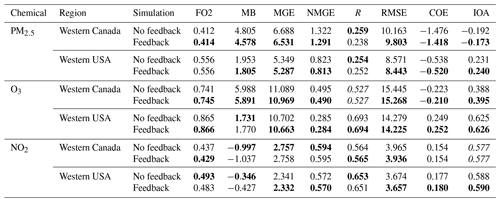
Both simulations' performance for ozone (O3), nitrogen dioxide (NO2), and particulate matter with diameters less than 2.5 µm (PM2.5) were evaluated using the above metrics, employing hourly AIRNOW data (for USA, AQS network: https://www.epa.gov/aqs, 17 June 2021; for Canada, NAPS network: http://maps-cartes.ec.gc.ca/rnspa-naps/data.aspx, 17 June 2021) and the openair package (Carslaw and Ropkins, 2012). The summary performance metric scores for the two simulations grouped, according to contributing measurement network, are shown in Table 3, with boldfaced values indicating the better score for the given simulation case. With respect to this table, we note the following:
- a.
The feedback simulation generally outperforms the no-feedback simulation (more boldfaced scores in the feedback rows, for 35 out of 48 metric comparisons).
- b.
Feedback forecast score improvements occurred were more noticeable for PM2.5 (usually first to second digit), followed by O3, with the NO2 scores often being the same for the first few digits.
- c.
We note that the boundary conditions employed for our 2.5 km simulations had a strong impact on model air-quality performance. As described above, these boundary conditions originated in a 10 km resolution simulation making use of ECMWF global reanalysis values on its own lateral boundaries. The magnitudes of the statistics of Table 3 may be compared to the magnitudes of the statistics from our initial ACPD submission (which made use of a MOZART 2009 reanalysis for chemical lateral boundary conditions for the 2.5 km GEM-MACH domain); these earlier results are shown in the Supplement, Table S2. The use of feedbacks had a similar relative impact on forecast performance (34 out of 48 statistics improving in the feedback forecast in the initial simulation, compared to 35 out of 48 statistics in the current work). However, the net impact of the ECMWF-driven 10 km GEM-MACH values being used for chemical lateral boundary conditions, rather than the MOZART climatology, was a degradation of performance: comparing the equivalent entries in Tables 3 and S2, it can be seen that 71 out of 96 scores were better with the earlier use of the MOZART reanalysis. As we show below, however, the revised boundary conditions led to improvements in model aerosol optical depth performance relative to observations.
The impact of lateral boundary conditions on model predictions can be seen when comparing MODIS retrievals of aerosol optical depth (AOD) with model predictions (Fig. 14). AOD is a function of both the particle's abundance and optical properties, integrated throughout the vertical column. However, direct comparisons between satellite and model-predicted AOD values must be undertaken with some care, due to the nature of the satellite retrieval quality assurance and control procedures, the motion of the orbiting spacecraft, and the scan time of the instrument. The manner in which AOD is calculated introduces additional uncertainty due to the range of values which may be derived from the same aerosol speciation using different methodologies (Curci et al., 2015). For a polar-orbiting instrument such as MODIS, the time at which overpasses occur varies with location, and valid satellite retrievals may not occur when the location being scanned is obscured by clouds. Observed averages may be built up over multiple valid scans over time, but the number of valid scans contributing to the local average at any given location will vary, due to the time and space variation in cloud cover. Here, individual valid Collection 6.1 MODIS/Aqua (MYD04_L2 AOD_550_Dark_Target_Deep_Blue_Combined) 10 km resolution 550 nm AODs were matched in time and space to the nearest model 2.5 km grid cell and output frequency hour. The paper by Levy et al. (2013) contains details on the MODIS combined AOD product. No averaging was employed in our comparison (Fig. 14); all satellite overpass AOD pixels and matching model AOD pixels are shown. Noting that the AOD colour scale is logarithmic, the model simulation driven using the ECMWF + 10 km resolution GEM-MACH for boundary conditions (Fig. 14b) is a much better match to observations (Fig. 14a) than the model simulation driven by MOZART climatological boundary conditions (Fig. 14c). The slope of the linear best fit line between all observation and model pairs in each case mirrors this finding, with the original (MOZART climatology) boundary conditions having a slope of 0.15 and R2 of 0.0382 and the revised ECMWF + GEM-MACH 10 km boundary conditions having a slope of 0.56 and an R2 of 0.067.
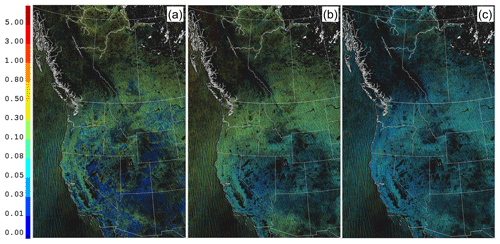
Figure 14550 nm AOD comparison. (a) All MODIS observations sampled over the model domain and forecast duration and (b) GEM-MACH 2.5 km simulation, driven by 10 km GEM-MACH simulations, in turn driven by ECMWF reanalysis for 2.5 km domain boundary conditions. (c) GEM-MACH 2.5 km simulation, driven by MOZART climatological boundary conditions.
Previous work with CFFEPS by Chen et al. (2019) for the 2017 fire season has shown similar PM2.5 positive biases for western Canada, with MB of +5.8 µg m−3 (88 stations) and for western USA with MB of +8.6 µg m−3 (221 stations). These positive biases (Chen et al., 2019) were higher specific to subregions closer to areas of active fires (MB of +12 µg m−3 for the subregion including the provinces of Alberta and British Columbia and +29 µg m−3 for the subregion comprising the states of Idaho, Montana, Oregon, and Washington, respectively). At least part of the positive biases may be due to 10 km GEM-MACH forest-fire emissions occurring in the state of Alaska being overestimated during the study period. However, the ECMWF reanalysis also captures significant particulate mass crossing the Bering Strait from fires in Siberia during this period, so the relative contributions of fires within the low-resolution GEM-MACH domain and the ECMWF boundary conditions driving that domain are combined and cannot be separated in the runs carried out here.
The local AOD positive biases associated with fires could also be the result of the mixing state assumptions of the Mie code used here for generating aerosol optical properties. These assumptions may also account for negative AOD biases over much of the remainder of the model domain. As noted earlier, this overall negative bias of AOD predictions (both boundary condition configurations result in observation:model slopes less than unity) is a common problem in air-quality models and may be due to assumptions regarding the model mixing state (Curci et al., 2015). That comparison of multiple mixing state assumptions on AOD with observations for European and North American modelling domains (Curci et al., 2015) showed a typical factor of 2 model underprediction of 440 nm North American AOD across all mixing state assumptions, with European AOD negative biases ranging from unbiased to a factor of 2. These earlier findings along with overestimates at forest-fire plumes with our current homogeneous mixture approach at 550 nm suggest that the hygroscopic growth may be overestimated for forest-fire particles, in turn overestimating forest-fire AODs locally, while external mixing assumptions may be required to improve model AOD performance elsewhere in the model domain.
We note that the combined use of the ECMWF global reanalysis and a 10 km resolution GEM-MACH simulation to provide boundary conditions for our 2.5 km domain resulted in a degradation of model performance for surface PM2.5, O3, and NO2, for 71 out of 96 statistical scores, compared to the use of a MOZART2009 reanalysis for 2.5 km domain boundary conditions. The improvement associated with the use of feedbacks was maintained, showing that the impact of feedbacks is a robust finding. However, the performance degradation associated with the change of boundary conditions is a source of concern.
We also note that, while AOD performance has improved with the use of the ECMWF + GEM-MACH 10 km boundary conditions in Fig. 14, significant overestimates of AOD occur with the use of these boundary conditions, in several regions in the USA. This may be seen by comparing Fig. 14a and b for the states of Montana, Wyoming, Nevada, New Mexico, and Utah, where the dark blue colours in the observations (Fig. 14a) indicate observed AODs less than 0.01, whereas the 10 km simulations driven by ECMWF + GEM-MACH (Fig. 14b) indicate values of 0.03 to 0.05). This is consistent with the increase in positive surface bias in PM2.5 associated with the use of the ECMWF + GEM-MACH 10 km boundary conditions (e.g., feedback runs having western Canada and western USA positive biases of PM2.5 of 4.578 and 1.805 µg m−3 (Table 1), compared to the MOZART2009 reanalysis driven run values of 0.236 and −1.786 µg m−3 (Table S2), respectively). The boundary condition setup thus accounts for a substantial increase in overall surface PM2.5 mass, with the use of ECMWF + GEM-MACH 10 km increasing mean PM2.5 by 4.34 and 3.59 µg m−3 in western Canada and USA, respectively, relative to the use of MOZART2009.
The reduction in performance may thus be due to two possible causes (or their combination): (1) the domain within the GEM-MACH 10 km simulation might be sufficiently large, and the emissions in the regions between the 10 km boundaries and the 2.5 km domain sufficiently in error, that the 2.5 km simulation accuracy is adversely affected; (2) the ECMWF reanalysis employed on the outermost boundary of the 10 km domain contributes sufficient PM2.5, NO2, and O3 to the simulations that the innermost domain model performance at the surface is adversely affected. That is, the degradation in performance may be associated with the GEM-MACH 10 km simulation, the ECMWF reanalysis, or a combination of both factors.
In order to examine the potential impact of the ECMWF reanalysis used as the outermost domain boundary conditions, we evaluated the ECMWF reanalysis using the same observation data, station locations, and performance metrics as were used for our 2.5 km simulations – the results of this analysis are shown in Tables S3 (PM2.5), S4 (O3), and S5 (NO2). This additional analysis shows that the ECMWF reanalysis values during the study period have higher positive biases for PM2.5 and O3 and lower negative biases for NO2 than the corresponding 2.5 km GEM-MACH simulations. A similar pattern occurs for the other statistical metrics, with the ECMWF reanalysis usually having a reduced performance for most statistical scores for PM2.5, O3, and NO2 over the study region in comparison to the high-resolution model simulations carried out here. The ECMWF reanalysis was also evaluated for the same time period for the entire North American domain, in Tables S3 to S5; the scores for this last analysis suggest that the performance of the reanalysis relative to observations is similar over the continent and is not limited to our study area. We note that the ECMWF reanalysis has relatively low spatial and time resolution compared to the 2.5 km simulations carried out here ( versus 2.5 km×2.5 km, 3-hourly output values compared to 1 h output values) and relatively coarse resolution for particle sizes (e.g., 3 size bins compared to the 12 bins used within GEM-MACH; these issues may factor into the performance scores). The comparison does not rule out the possibility that GEM-MACH's 10 km resolution simulations may also contribute adversely to our 2.5 km model performance. However, our analysis suggests that our use of the ECMWF reanalysis for boundary conditions on our outermost domain likely accounts for at least some of the performance degradation in our modelling system, compared to the MOZART2009 boundary condition simulation carried out earlier.
3.3 Model evaluation summary
Overall, the incorporation of feedbacks in this study has resulted in improvements in weather and air-quality forecast accuracy, albeit with some caveats. Weather forecast variables showed improvements at the 90 % confidence level for several fields, and vertical profiles showed a matching performance or improvements at most levels and times. Total precipitation scores also showed minor improvements or matching performance at the 90 % confidence level. A previously unexpected spin-up issue specific to online coupled models was noted: the impact of online coupled particulate matter on cloud variables was sufficiently strong that cloud field adjustment in the first 6 h of the forecast was required prior to some weather forecast variable improvements to be apparent (surface pressure, dew-point temperature, sea-level pressure). While the current forecast cycling duration was constrained by operational requirements, this suggests that forecast cycling should include both air-quality and meteorological variables during online coupled forecast spin-up periods. That is, the model tracer concentrations 6 h prior to the current forecast start-up could also be used during the initial meteorological spin-up period, thus allowing for the simultaneous spin-up of chemistry and cloud formation. Scores for surface PM2.5, NO2, and O3 also generally improved with the incorporation of feedbacks (35 out of 48 comparisons showed improvements). The choice of lateral boundary conditions was shown to have a significant impact on chemical performance within the model domain. In comparison to satellite-based AOD values, the current model's AOD values were generally biased low, with smaller magnitude biases being associated with the ECMWF + 10 km GEM-MACH boundary conditions. The latter comparison also showed that large fires off-domain in Alaska and Siberia likely had a large impact on AODs in the eastern and northern section of the model domain, through comparison with our initial simulations.
In this section, we compare time averages of the entire study period for the two simulations, both at the surface and in vertical cross sections through the model domain, to illustrate some of the changes in both weather and air-quality associated with the incorporation of feedbacks. We have found differences at greater than 90 % confidence between the predicted meteorological and chemical forecasts in the vicinity of the Alberta–Saskatchewan forest fires, as well as in contrasting changes between land and sea. We note again here that the no-feedback simulation makes use of time-invariant and spatially invariant aerosol CCN and optical properties, within the meteorological portion of the model. The comparisons thus show the differences associated with the use of climatological constant aerosol properties and the online coupled model-generated aerosols.
As in the meteorological evaluation, we have made use of 90 % confidence levels in order to gauge the level of significance of the differences between the feedback and no-feedback simulations in the following analysis.
The approach for representing model grid value 90 % confidence levels is described in detail in Sect. S2. The differences in the mean grid cell values between the simulations for which the above quantity is greater than unity differ at or greater than the 90 % confidence level. Differences in the mean values, as well as the value of the above ratio, are thus reported in the following section.
4.1 Effects of feedbacks on time-averaged meteorology
The feedback–no-feedback differences in the simulation-period average cloud droplet number density (number per kilogram of air) and mass density (grams of water per kilogram of air) along centered cross sections spanning the length and width of the 2.5 km resolution model domain are shown in Fig. 15 (cross section locations are shown in Fig. 1). The “ocean”, “land”, and “forest fire” regions identified are with reference to the approximate locations of these features along these cross sections. Figure 15 also shows the confidence ratio values as described above – regions where the predicted mean values differ at or above the 90 % confidence level are shown in red, while those differences below the 90 % confidence interval are shown in blue. Feedbacks increase the cloud droplet number density over the northern part of the domain, including the region impacted by the Alberta–Saskatchewan forest fires, from the surface up to about 500 mb (roughly equivalent to hybrid level 0.500), and decrease at higher elevations further to the south and along the length of the model domain into the western USA (Fig. 15a). Cloud droplet numbers also decrease over the ocean but increase eastwards over the land (Fig. 15b). The latter is unrelated to the forest fires; this is an indication that the modelled aerosol number concentration over the ocean is much lower than the single climatological aerosol population assumed in the no-feedback run, resulting in lower cloud droplet number concentrations. The changes are significant at the 90 % confidence level from the surface up to hybrid level 0.60 in the northern region which is most impacted by forest-fire smoke, and in isolated regions further aloft along the south–north cross section (Fig. 15c), and over the regions of the ocean in the west–east cross section (Fig. 15d). Aerosol loadings that are higher than climatology, a large portion of which are due to the forest fires, resulted in increased cloud droplet number densities in the lower troposphere while decreasing them in the middle–upper troposphere (Fig. 15a). This impact of feedbacks is in accord with the satellite observations of Saponaro et al. (2017) and was also seen in Takeishi et al. (2020). In contrast, cloud droplet mass density (i.e., cloud liquid water content) largely decreases across the domain along the north–south cross section (Fig. 15e), as well as over the ocean, with a varying pattern over the land in the east–west cross section (Fig. 15f). The magnitudes and significance levels for the average change in cloud droplet mass are lower than for cloud droplet number, with the most significant differences occurring over the ocean (Fig. 15g and h).
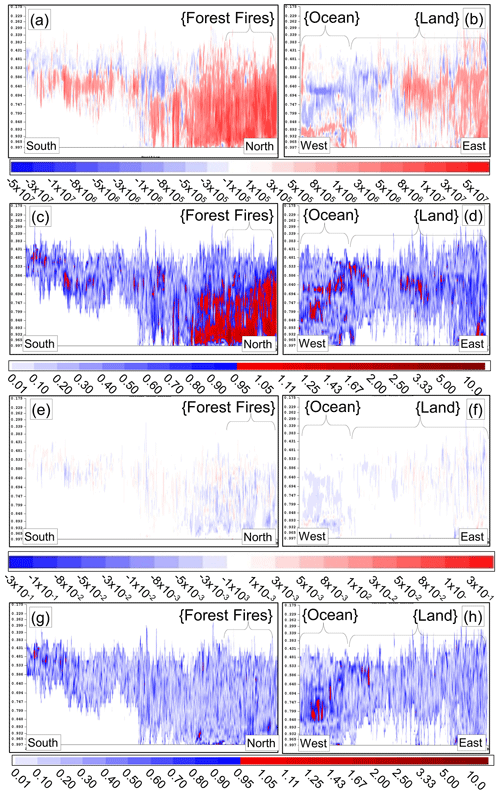
Figure 15(a, b) Difference in mean (feedback–no-feedback) cloud droplet number simulations along south–north and east–west cross sections through the middle of the model domain. (c, d) Corresponding significance level of mean cloud droplet number differences using the confidence ratio defined in Eq. (S1) in Sect. S2 – red areas indicate ratio values greater than unity, i.e., significance at or above the 90 % confidence level. (e, f) Difference in mean cloud droplet mass (g kg−1). (g, h) Corresponding significance level of mean cloud droplet mass difference. Note: the vertical axis in hybrid coordinates does not show all model levels for clarity; the model has much finer resolution in the lower part of the atmosphere than shown, and the portion of the vertical domain shown encompasses only the lower half of the levels in the model.
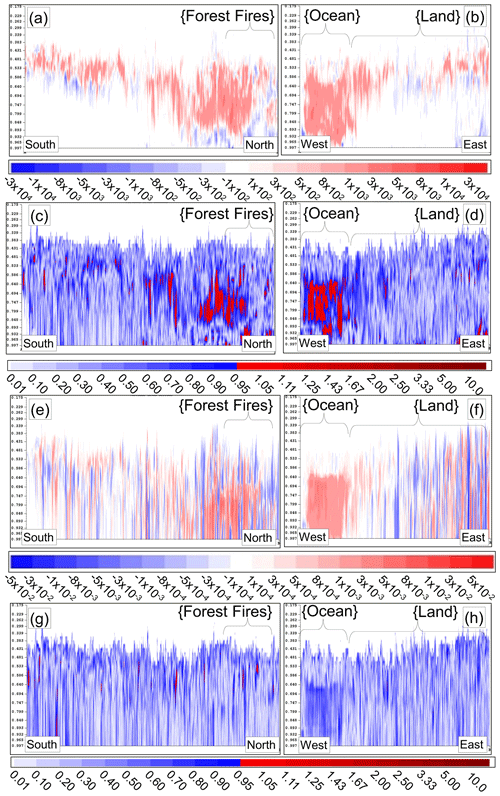
Figure 16(a, b) Difference in mean (feedback–no-feedback) raindrop number simulations along south–north and east–west cross sections through the middle of the model domain. (c, d) Corresponding significance level of mean raindrop number differences using the confidence ratio defined in Eq. (S1) in Sect. S2 – red areas indicate ratio values greater than unity, i.e., significance at or above the 90 % confidence level. (e, f) Difference in rain cloud drop mass (g kg−1). (g, h) Corresponding significance level of mean raindrop mass difference.
Consistent with the cloud droplet number changes, rain droplet numbers and mass mixing ratios increase aloft with the feedback simulation, over both the forest region impacted by the forest fires (Fig. 16a and e) and over the ocean (Fig. 16b and f), with a varying impact over the land and more distant from the forest-fire sources (Fig. 16f). The changes are significant at the 90 % confidence level for rain droplet number in these regions (compare Fig. 16a with c; 16b with d), while the rain droplet mass changes sometimes reach but are usually below the 90 % confidence level (Fig. 16g and h).
These results suggest that relative to the no-feedback simulation, which employs climatological aerosol CCN properties, the AIE in the feedback simulation is causing significant change in hydrometeor numbers and a less significant increase in hydrometeor mass. In the forest-fire-impacted region, the ADE and AIE in the feedback simulation significantly increase the number of cloud droplets near the surface and throughout the middle to upper troposphere (Fig. 15a and c). The raindrop number in the middle troposphere (Fig. 16a and c) also increases significantly between hybrid levels 0.90 and 0.70 (Fig. 16e and g). Near-surface raindrop number and raindrop mass differences throughout the cross sections (Fig. 16e and f) fall below the 90 % confidence level (Fig. 16g and h).
Over the oceans, water droplet number and mass both decrease (Fig. 15b and f), and raindrop number and mass increase (Fig. 16b and f); more atmospheric water is converted to raindrops as a result of the feedbacks, relative to the climatology in the no-feedback simulation. However, these changes are more significant aloft than at the surface, with the difference in both raindrop number and mass falling below the 90 % confidence level near the surface. We interpret these changes as a shift in over-ocean liquid hydrometeor numbers and to a lesser degree the water mass aloft from cloud droplets to raindrops due to the AIE in the feedback setup relative to the climatology of the no-feedback simulation. The changes occur at the 90 % confidence level aloft, but the near-surface changes are smaller and are usually below the 90 % confidence level.
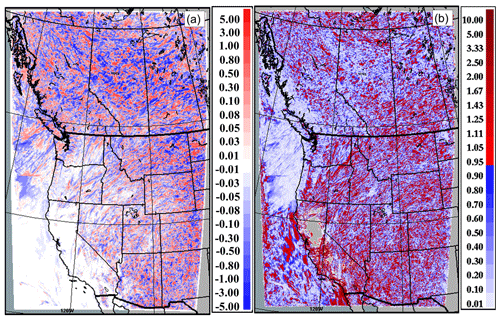
Figure 17(a) Average (feedback–no-feedback) total surface precipitation during the simulation period. (b) The 90 % confidence ratio – values greater than 1 indicate significantly different results at the 90 % confidence level.
Differences in the average surface precipitation flux and the confidence ratio values are shown in Fig. 17. Changes in average precipitation (Fig. 17a) appear random, though locally these differences are significant at the 90 % confidence level (Fig. 17b). Both the magnitude of the differences and the frequency in their reaching the 90 % confidence level increase south-westwards. Given the local and episodic nature of rainfall events, the high level of significance in this case probably results from the presence or absence of individual rainfall events between the two simulations affecting the local average and standard deviations.
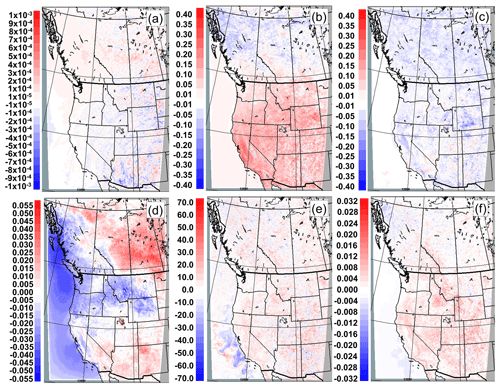
Figure 18Differences in average meteorological fields (feedback–no-feedback; red values indicate more positive values in the feedback simulation than in the no-feedback simulation). Panels show average difference in (a) specific humidity (g kg−1); (b) air temperature (∘C), (c) dew-point temperature (∘C), (d) surface pressure (mb), (e) planetary boundary layer height (m), and (f) friction velocity (m s−1).
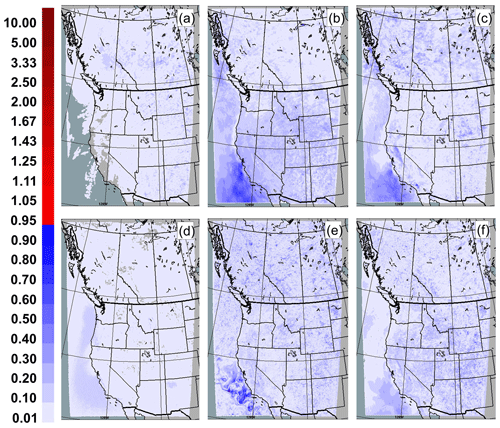
Figure 1990 % confidence ratios, with the same fields as Fig. 18. Values greater than 1 indicate significantly different results at or greater than the 90 % confidence level.
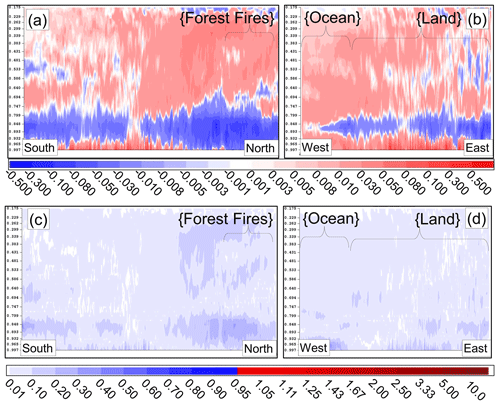
Figure 20(a, b) Difference in mean (feedback–no-feedback) temperature simulations along south–north and east–west cross sections through the middle of the model domain. (c, d) Corresponding confidence ratio of mean temperature differences – red areas indicate ratio values greater than unity, i.e., significance at or above the 90 % confidence level.
Several systematic changes in the average values of the model's meteorological output fields were noted due to the use of feedbacks relative to aerosol property climatologies (Fig. 18), although all fall below the 90 % confidence level for the difference in the mean values between the two simulations (Fig. 19). Specific humidity increased in the region most affected by fires (Fig. 18a), and surface air temperature decreased below the smoke plumes while increasing further south (Fig. 18b), while dew-point temperature decreased (Fig. 18c), implying a decrease in relative humidity with feedbacks. Surface pressure increased over the land (mostly east of the Rockies), particularly in the region downwind of the Alberta–Saskatchewan fires while decreasing over the ocean (Fig. 18d). Planetary boundary layer height increased over the land (Fig. 18e) except in the immediate vicinity of the Alberta–Saskatchewan fires, consistent with decreased atmospheric stability in the lowest part of the atmosphere. The friction velocity also increased with the use of feedbacks (Fig. 18f); this is consistent with a decrease in stability and an increase in turbulent energy. The air temperature increases occur at the surface south of the forest-fire-impacted region and above roughly 750 mb, decreasing temperatures from the surface in the forest-fire-impacted region up to 750 mb (Fig. 20a and b). Feedbacks thus increase near-surface temperatures, relative to the no-feedback meteorological model's simple aerosol climatology, in regions far from the fires, decreasing them near the fires, decrease temperatures in the lower free troposphere, and increase temperatures further aloft. All of these differences between feedback and no-feedback simulations, despite their large geographic range, fall below the local 90 % confidence ratio. However, when the differences in air temperature associated with feedback and no-feedback forecasts are compared to observations across the entire domain (as opposed to at grid point locations as in Figs. 18 and 19), the 90 % confidence level is exceeded, both at the surface at specific forecast times (Fig. 6a), and at multiple heights aloft at the 12th and 24th forecast hours (Figs. 12 and 13).
4.2 Effects of feedbacks on time-averaged chemistry
In the previous meteorological impacts section, changes in aerosol loading relative to the climatology, dominated by forest fires, were shown to have a significant impact on cloud formation and atmospheric temperatures through ADE and AIE. These might be expected to in turn influence and be influenced by particulate matter emitted by the forest fires, with the plume rise of the forest fires dependent on the meteorological changes. Air temperatures increase slightly in the model surface layer south of the fires (Fig. 18b, +0.01 to +0.05 ∘C) but decrease at greater magnitudes through the rest of the lower troposphere (surface near the fires to hybrid level 0.749, Fig. 20a), with a maximum decrease of −0.5 ∘C between hybrid levels 0.893 and 0.848. The reduction in temperatures between hybrid levels 0.90 to 0.70 from the impact of the smoke plumes is similar to the findings of Saponaro et al. (2017). These changes in air temperature imply a decrease in near-surface atmospheric stability associated with feedbacks, given that the overall temperature gradient from the surface has become more negative (that is, the ambient lapse rate has increased). Rising air parcels will follow an adiabatic lapse rate; these increases in the ambient lapse rate imply that rising air parcels will have an increasing tendency to be warmer than their environment. Feedbacks have thus reduced atmospheric stability within the forest-fire smoke in the lowest part of the atmosphere; the atmosphere there has become more unstable. Meanwhile, the feedbacks decrease the environmental lapse rate further aloft above the forest-fire smoke, between hybrid levels 0.848 and 0.339. Rising air parcels in this region following an adiabatic lapse rate will thus have an increasing tendency to be colder than their environment – the atmosphere above the smoke plumes has become more stable. This is echoed by the response of the concentration fields to the near-surface stability change, as can be seen through comparisons of the PM2.5, NO2, and O3 surface concentration changes (Fig. 21) and the vertical cross sections (Figs. 22–24), respectively.
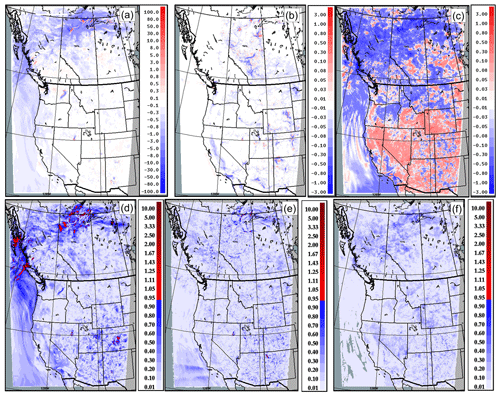
Figure 21(a–c) Difference (feedback–no-feedback) in surface mean PM2.5 (µg m−3), NO2 (ppbv), and O3 (ppbv), respectively. (d–f) Corresponding confidence ratio of mean differences – red areas indicate ratio values greater than unity, i.e., significance at or above the 90 % confidence level.
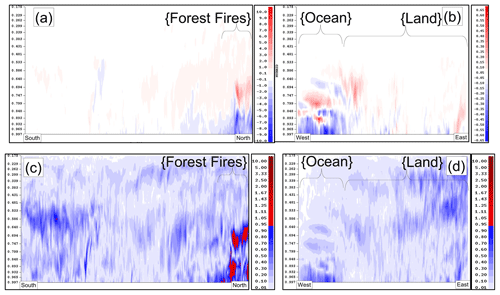
Figure 22(a, b) Difference (feedback–no-feedback) in predicted mean PM2.5 (µg m−3), along domain-center south–north and west–east cross sections. (c, d) Corresponding confidence ratio of mean differences – red areas indicate ratio values greater than unity, i.e., significance at or above the 90 % confidence level. Note that colour bar scales differ between (a) and (b).
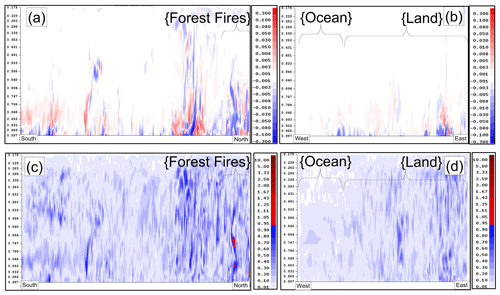
Figure 23(a, b) Difference (feedback–no-feedback) in predicted mean NO2 (ppbv), along domain-center south–north and west–east cross sections. (c, d) Corresponding confidence ratio of mean differences – red areas indicate ratio values greater than unity, i.e., significance at or above the 90 % confidence level.
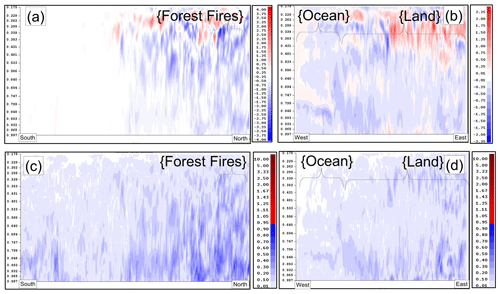
Figure 24(a, b) Difference (feedback–no-feedback) in predicted mean O3 (ppbv), along domain-center south–north and west–east cross sections. (c, d) Corresponding confidence ratio of mean differences – red areas indicate ratio values greater than unity, i.e., significance at or above the 90 % confidence level. Note that colour bar scales differ between (a) and (b).
Changes above the 90 % confidence level for PM2.5 and NO2 occur near the forest fires themselves (red regions, near top of model domain; Fig. 21a and b), though they remain below 90 % confidence for O3 (Fig. 21c).
Feedbacks result in near-surface PM2.5 decreases in the regions downwind of the forest fires (Figs. 21a and 22a; note the large blue region and more intense blue region near surface in Fig. 22a), suggesting less PM2.5 mass is present near the surface due to the feedbacks. Given the increase in near-surface stability below the fire plumes noted above, this change in the vertical distribution probably reflects a decrease in downward diffusive mixing of the forest-fire plumes once aloft – the feedbacks thus have a tendency to increase the smoke plume concentrations aloft, by preventing the downward mixing of smoke injected by the fires. These PM2.5 concentration effects rise above the 90 % confidence level within the region closest to the fires.
Feedbacks result in an increase in near-surface NO2 in several inland urban centers and less NO2 at surface level downwind (Fig. 21b; though these differences are only significant at the 90 % confidence level within the forest-fire plumes (Figs. 21e and 23c). Ocean versus land NO2 differences remain below the 90 % confidence level.
Feedbacks decreased lower troposphere O3 near the forest fires (Figs. 21c and 24a), while increasing O3 near and above hybrid level 0.383. The forest fires are also the only area where the differences between mean ozone forecasts approach 90 % confidence.
Overall, the most significant effects of the feedbacks were (1) increases in PM2.5 aloft and decreases near the surface in areas impacted by the fires; (2) increases in NO2 aloft and decreases near the surface near the fires, to a lesser extent than PM2.5; and (3) decreases in lower troposphere O3, particularly near the surface in the region impacted by the fires.
The feedback-induced changes in primary and secondary pollutants in the forest-fire regions are consistent with the decrease in atmospheric stability noted above – a greater proportion of the primary particulate matter and NO2 resulting from near-surface forest-fire emissions of NO remain aloft with the addition of feedbacks. The decrease in surface ozone and increase further aloft in the fire region (Fig. 24a) spatially match the decrease in surface NO2 (Fig. 22a). Chemically, this may imply that the changes associated with feedbacks occur in NOx-limited environments, i.e., with relatively high ratios, since in these environments, decreases in NOx emissions may lead to decreases in the rate of secondary O3 formation. Alternatively, the reduction in near-surface O3 concentrations may reflect a decrease in light levels reaching the surface due to cloud attenuation (aerosol indirect effect), with the resultant lower photolysis rates resulting in a reduction in surface photochemical ozone production.
Our analysis thus suggests that a net enhanced upward transport occurs in forest-fire plumes due to feedbacks and that this transport is linked to the following feedback-induced changes:
- 1.
increases in local near-surface atmospheric stability, reducing downward mixing of particulate plumes once aloft (Fig. 22a);
- 2.
increases in cloud droplet numbers throughout the lower troposphere (Fig. 15a); and
- 3.
increases in raindrop numbers aloft (Fig. 16a).
This combination suggests the presence of an AIE feedback loop – increased lower atmosphere stability results in a greater proportion of particulate matter remaining aloft, in turn resulting in more particles remaining at higher levels in the atmosphere where they may act as cloud condensation nuclei, increasing cloud droplets aloft (Fig. 15a). This in turn results in increased lower middle troposphere cooling, through the first AIE (increase in cloud droplet numbers aloft, leading to increased cloud albedo and cooling of the atmosphere below the cloud tops), while the corresponding decreases in particles and cloud condensation nuclei at lower levels result in a smaller near-surface impact on the AIE and ADE, hence relatively minor changes in near-surface temperatures (Fig. 20a). This combination maintains a feedback-induced near-surface unstable temperature gradient, relative to the no-feedback simulation employing aerosol property climatologies. We acknowledge that these changes in temperature fall below the 90 % confidence level for the averages over all times, though note that differences in mean bias relative to observations for the two simulations became significantly different at specific times of day in the forecasts (Fig. 6a; hours 3, 6, 15, and 18, corresponding to 15:00, 18:00, 03:00, and 06:00 UT or 09:00, 12:00, 21:00, and 00:00 MDT), implying that the temperature changes at these specific times reach a higher level of significance. Similarly, Figs. 12 and 13 show reductions in the near-surface temperature biases with the use of feedbacks.
4.3 Summary: differences in forecast simulation-period averages
Relative to the no-feedback simulation employing an aerosol climatology, the AIE feedback as simulated here is associated with increases in near-surface stability over both ocean- and forest-fire-influenced land areas. Over oceans, near-surface particulate matter is removed as cloud condensation nuclei, resulting in increased cloud droplet numbers, maintaining the temperature gradient through the first aerosol indirect effect. In the vicinity of forest fires, increases in near-surface stability result in more PM2.5 remaining aloft, increasing the availability of cloud condensation nuclei aloft, increasing cloud droplet numbers aloft, hence also maintaining the less stable near-surface temperature gradient through the first aerosol indirect effect. We note that the ADE may also play a weak role, particularly in the southern part of the domain, where lower atmosphere temperature gradient increases are not accompanied by significant changes in cloud droplet numbers (Fig. 15a, southern half of the cross section) but are accompanied by significant though small magnitude increases in PM2.5 in the lower atmosphere (Fig. 22a, southern half of cross section) and temperature profile changes (Fig. 20) below the 90 % confidence level.
The work carried out here suggests that the answers to our two research questions (“can online coupled models improve both air-quality and meteorological forecasts?” and “are the changes in forest-fire forecasts associated with implementing forest-fire emissions within an online coupled model sufficient to significantly perturb weather and chemistry?”) are both a qualified “yes”. Within the high-resolution domain size employed here, improvements or matching weather forecast performance were seen for most times and heights in the atmosphere, at greater than 90 % confidence. Improvements in model performance for surface PM2.5, NO2, and O3 were also found, across most statistical measures (35 out of 48 statistical evaluation scores showed improvements). Comparing average vertical cross sections, the chemical concentration changes associated with feedbacks were the most significant close to the forest fires in the northern portion of the domain. There, increased net vertical transport associated with decreased near-surface stability lowered near-surface PM2.5 and NO2 concentrations and increased them aloft and resulted in reduced surface O3.
Our simulations suggest that aerosol optical depth in the region, as well as the overall chemical performance of the model, was strongly influenced by upwind boundary conditions. AODs were biased low despite PM2.5 positive biases, suggesting that the homogeneous mixture approach for aerosol optical properties results in a general underprediction of aerosol optical depths, in accord with Curci et al. (2015), and that obtaining better data for forest-fire aerosol optical properties should be a priority for future study, as well as an examination of external mixture approaches. Positive AOD biases in the region affected by fires suggest that forest-fire plumes have significantly different optical properties and may be less hygroscopic than industrial aerosols of comparable size. Special/separate treatment of forest-fire CCN and optical properties is therefore also recommended in future work.
Online coupling forest-fire plume-rise calculations with the weather parameters were shown to have a significant impact on the height of primary pollutants reached by forest fires, on the formation of near-surface ozone near the forest fires, and on particulate matter. These changes were largely driven by the AIE, which maintains an increased lapse rate (decreased near-surface stability) over the forest-fire-influenced and oceanic portions of the region studied. Weak evidence for the influence of the ADE was shown in the southern part of the domain, where increases in particulate matter were also accompanied by decreases in stability between the surface and the lower–middle troposphere (the differences were at a confidence level lower than 90 % for these comparisons of temperatures averaged over all model times).
Relative to the no-feedback aerosol climatology for CCN and aerosol optical properties, the simulations carried out here suggested that in the vicinity of forest fires, feedbacks significantly increase cloud droplet number densities near the surface and aloft and significantly increase raindrop number densities aloft, relative to forecasts driven by climatological aerosol properties. Over the oceans, feedbacks decreased cloud droplet number density and increased raindrop number density aloft, relative to the simulation employing invariant CCN properties. Oceanic cloud droplet mass increased to a lesser degree (with smaller regions above the 90 % confidence level), as did raindrop mass (the mean differences for which for the most part remained below the 90 % confidence level). This provides some evidence for a shift in atmospheric water mass associated with feedbacks from cloud water to rain over the oceans relative to the no-feedback climatology, though this shift occurred largely within the variability of the cloud fields within each simulation. Longer simulations may be needed to achieve higher confidence in this finding.
The datasets used here for model evaluation are available from the publicly accessible websites of the AQS network at https://www.epa.gov/aqs (last access: 17 June 2021, US EPA, 2021) and the NAPS network at http://maps-cartes.ec.gc.ca/rnspa-naps/data.aspx (last access: 17 June 2021, Government of Canada, 2021).
The supplement related to this article is available online at: https://doi.org/10.5194/acp-21-10557-2021-supplement.
PAM was responsible for experiment design, conceptualization, analysis, and writing of manuscript drafts. AA was responsible for model code and run script design and implementation, statistical analysis of model results, and model analysis graphics. JC was responsible for forest-fire emissions processing system design and coding, manuscript contributions, and manuscript draft review and assistance. BP was responsible for forecast system simulations and design. WG was responsible for indirect effect updates, advice on the P3 cloud microphysics implementation, manuscript contributions, and manuscript review. CrS was responsible for code version contributions and manuscript review. ChS was responsible for AOD analysis, manuscript contributions, and manuscript review. KA was responsible for forest-fire emissions processing system design and coding, manuscript contributions, and manuscript review. PC was responsible for forecast system simulations and design. JZ was responsible for emissions processing and input field assistance, manuscript review, and manuscript contributions. JM was responsible for indirect effect updates and advice on implementing AIE in the P3 scheme, manuscript review and manuscript contributions.
The authors declare that they have no conflict of interest.
Publisher’s note: Copernicus Publications remains neutral with regard to jurisdictional claims in published maps and institutional affiliations.
This paper was edited by Ari Laaksonen and reviewed by two anonymous referees.
Abdul-Razzak, H. and Ghan, S. J.: A parameterization of aerosol activation. 3. Sectional representation, J. Geophys. Res.-Atmos., 107, AAC 1-1–AAC 1-6, https://doi.org/10.1029/2001JD000483, 2002.
Aggarwal, R. and Ranganathan, P.: Common pitfalls in statistical analysis: the use of correlation techniques, Perspect. Clin. Res., 7, 187–190, 2016.
Ahmadov, R., James, E., Grell, G., Alexander, C., Benjamin. S., McKeen, S., Pereira, G., Freitas,S., Csiszar, I., Tsidulko, M., Kondragunta, S., Xu, C., Wong, K. Y., and Albers, S.: Forecasting smoke, visibility and smoke-weather interactions using a coupled meteorology-chemistry modeling system: Rapid Refresh and High-Resolution Rapid Refresh coupled with Smoke (RAP/HRRR-Smoke), EGU 2019 Conference presentation, Geophysical Research Abstracts, Vol. 21, EGU2019-18605, available at https://meetingorganizer.copernicus.org/EGU2019/EGU2019-18605.pdf (last access: 17 June 2021), 2019.
Akingunola, A., Makar, P. A., Zhang, J., Darlington, A., Li, S.-M., Gordon, M., Moran, M. D., and Zheng, Q.: A chemical transport model study of plume-rise and particle size distribution for the Athabasca oil sands, Atmos. Chem. Phys., 18, 8667–8688, https://doi.org/10.5194/acp-18-8667-2018, 2018.
Albrecht, B. A.: Aerosols, cloud microphysics, and fractional cloudiness, Science, 245, 1227–1230, https://doi.org/10.1126/science.245.4923.1227, 1989.
Baró, R., Palacios-Peña, L., Baklanov, A., Balzarini, A., Brunner, D., Forkel, R., Hirtl, M., Honzak, L., Pérez, J. L., Pirovano, G., San José, R., Schröder, W., Werhahn, J., Wolke, R., Žabkar, R., and Jiménez-Guerrero, P.: Regional effects of atmospheric aerosols on temperature: an evaluation of an ensemble of online coupled models, Atmos. Chem. Phys., 17, 9677–9696, https://doi.org/10.5194/acp-17-9677-2017, 2017.
Briggs, G. A.: A plume rise model compared with observations, JAPCA J. Air. Waste Ma., 15, 433–438, https://doi.org/10.1080/00022470.1965.10468404, 1965.
Briggs, G. A.: Plume rise and buoyancy effects, atmospheric sciences and power production, in: DOE/TIC-27601(DE84005177), edited by: Randerson, D., Technical Information Center, US Dept. of Energy, Oak Ridge, TN, USA, 327–366, 1984.
Carrera, M. L., Belair, S., and Bilodeau, B.: The Canadian Land Data Assimilation System (CalDAS): description and synthetic evaluation study, J. Hydrometeorol., 16, 1293–1314, 2015.
Carslaw, D. C. and Ropkins, K.: openair – An R package for air quality data analysis, Environ. Modell. Softw., 27–28, 52–61, 2012.
Chen, J., Anderson, K., Pavlovic, R., Moran, M. D., Englefield, P., Thompson, D. K., Munoz-Alpizar, R., and Landry, H.: The FireWork v2.0 air quality forecast system with biomass burning emissions from the Canadian Forest Fire Emissions Prediction System v2.03, Geosci. Model Dev., 12, 3283–3310, https://doi.org/10.5194/gmd-12-3283-2019, 2019.
Chosson, F., Vaillancourt, P., Milbrandt, J. A., Yau, M. K., and Zadra, A.: Adapting two-moment microphysics schemes across model resolutions: subgrid clouds and precipitation fraction and microphysical sub-time step, J. Atmos. Sci., 71, 2635–2653, 2014.
Clark, T. L., Jenkins, M. A., Coen, J. L., and Packham, D. R.: A coupled atmosphere-fire model: role of convective froude number and dynamic engineering at the fireline, Int. J. Wildland Fire, 6, 177–190, 1996.
Coen, J. Cameron, M., Michalakes, J., Patton, E., Riggan, P., and Yedinak, K.: WRF-Fire: coupled weather-wildfire modelling with the Weather Research and Forecasting Model, J. Appl. Meteorol. Clim., 52, 16–38, 2013.
Côté, J., Gravel, S., Méthot, A., Patoine, A., Roch, M., and Staniforth, A.: The operational CMC/MRB global environmental multiscale (GEM) model. Part 1: design considerations and formulation, Mon. Weather Rev., 126, 1373–1395, 1998.
Curci, G., Hogrefe, C., Bianconi, R., Im, U., Balzarini, A., Baro, R., Brunner, D., Forkel, R., Giordano, L., Hirtl, M., Honzak, L., Jimenez-Guerrero, P., Knote, C., Langer, M., Makar, P. A., Pirovano, G., Perez, J. L., San Jose, R., Syrakov, D., Tuccella, P., Werhahn, J., Wolke, R., Zabkar, R., Zhang, J., and Galmarini, S.: Uncertainties of simulated aerosol optical properties induced by assumptions on aerosol physical and chemical properties: an AQMEII2 perspective, Atmos. Environ., 115, 541–522, 2015.
Duveiller, G., Fasbender, D, and Meroni, M.: Revisiting the concept of a symmetric index of agreement for continuous datasets, Sci. Rep.-UK, 6, 19401, https://doi.org/10.1038/srep19401, 2016.
Ezhova, E., Ylivinkka, I., Kuusk, J., Komsaare, K., Vana, M., Krasnova, A., Noe, S., Arshinov, M., Belan, B., Park, S.-B., Lavrič, J. V., Heimann, M., Petäjä, T., Vesala, T., Mammarella, I., Kolari, P., Bäck, J., Rannik, Ü., Kerminen, V.-M., and Kulmala, M.: Direct effect of aerosols on solar radiation and gross primary production in boreal and hemiboreal forests, Atmos. Chem. Phys., 18, 17863–17881, https://doi.org/10.5194/acp-18-17863-2018, 2018.
Fox, D. G.: Judging air quality model performance – a summary of the AMS workshop on dispersion model performance, B. Am. Meteorol. Soc., 62, 599–609, 1981.
Galmarini, S., Hogrefe, C., Brunner, D., Makar, P., and Baklanov, A.: Preface, Atmos. Environ., 115, 340–344, 2015.
Geer, A. J.: Significance of changes in medium-range forecast scores, Tellus A, 68, 30229, https://doi.org/10.3402/tellusa.v68.30229, 2016.
Girard, C., Plante, A., Desgagne, M., McTaggart-Cowan, R., Cote, J., Charron, M., Gravel, S., Lee, V., Patoine, A., Qaddouri, A., Roch, M., Spacek, L., Tanguay, M., Vaillancourt, P. A., and Zadra, A.: Staggered vertical discretization of the Canadian Environmental Multiscale (GEM) model using a coordinate of the log-hydrostatic-pressure type, Mon. Weather Rev., 142, 1183–1196, 2014.
Gong, S. L. Barrie, L. A., Blanchet, J.-P., von Salzen, K., Lohmann, U., Lesins, G., Spacek, L., Zhang, L. M., Girard, E., Lin, H., Leaitch, R., Leighton, H., Chylek, P., and Huang, P.: Canadian Aerosol Module: a size-segregated simulation of atmospheric aerosol processes for climate and air quality models. 1. Module development, J. Geophys. Res., 108, 4007, https://doi.org/10.1029/2001JD002002, 2003.
Gong, W., Makar, P. A., Zhang, J., Milbrandt, J, Gravel, S., Hayden, K. L., Macdonald, A. M., and Leaitch, W. R.: Modelling aerosol-cloud-meteorology interaction: a case study with a fully coupled air quality model (GEM-MACH), Atmos. Environ., 115, 695–715, 2015.
Gong, W., Beagley, S. R., Zhang, J., Cousineau, S., Chen, J., Sassi, M., Munoz-Alpizar, R., Morrison, H., Lyons, L., and Bellavance, P.: Modelling regional air quality in the Canadian Arctic: impact of North American wildfire and Arctic shipping emissions, in: Air Pollution Modelling and Its Application, XXIV, edited by: Steyn, D. G. and Chaumerliac, N., Springer, Switzerland, 301–306, 2016.
Government of Canada: National Air Pollution Surveillance (NAPS) Program, Government of Canada Open Data Portal, open.canada.ca, available at: http://maps-cartes.ec.gc.ca/rnspa-naps/data.aspx, last access: 17 June 2021.
Grell, G. A. and Freitas, S. R.: A scale and aerosol aware stochastic convective parameterization for weather and air quality modeling, Atmos. Chem. Phys., 14, 5233–5250, https://doi.org/10.5194/acp-14-5233-2014, 2014.
Griffin, D., Sioris, C., Chen, J., Dickson, N., Kovachik, A., de Graaf, M., Nanda, S., Veefkind, P., Dammers, E., McLinden, C. A., Makar, P., and Akingunola, A.: The 2018 fire season in North America as seen by TROPOMI: aerosol layer height intercomparisons and evaluation of model-derived plume heights, Atmos. Meas. Tech., 13, 1427–1445, https://doi.org/10.5194/amt-13-1427-2020, 2020.
Hanna, S. R.: Air quality model evaluation and uncertainty, JAPCA J. Air. Waste Ma., 38, 406–412, 1988.
Hoegh-Guldberg, O., Jacob, D., Taylor, M., Bindi, Brown, S., Camilloni, I., Diedhiou, A., Djalante, R., Ebi, K. L., Engelbrecht, F., Guiot, J., Hijioka, Y., Mehrotra, S., Payne, A., Seneviratne, S. I., Thomas, A., Warren, R., and Zhou, G.: Impacts of 1.5 ∘C Global Warming on Natural and Human Systems, in: Global Warming of 1.5 ∘C. An IPCC Special Report on the impacts of global warming of 1.5 ∘C above pre-industrial levels and related global greenhouse gas emission pathways, in the context of strengthening the global response to the threat of climate change, sustainable development, and efforts to eradicate poverty, edited by: Masson-Delmotte, V., Zhai, P., Pörtner, H.-O., Roberts, D., Skea, J., Shukla, P. R., Pirani, A., Moufouma-Okia, W., Péan, C., Pidcock, R., Connors, S., Matthews, J. B. R., Chen, Y., Zhou, X., Gomis, M. I., Lonnoy, E., Maycock, T., Tignor, M., and Waterfield, T.: World Meteorological Organization, Geneva, Switzerland, in press, available at: https://www.ipcc.ch/sr15/ (last access: 17 June 2021), 2018.
Inness, A., Ades, M., Agustí-Panareda, A., Barré, J., Benedictow, A., Blechschmidt, A.-M., Dominguez, J. J., Engelen, R., Eskes, H., Flemming, J., Huijnen, V., Jones, L., Kipling, Z., Massart, S., Parrington, M., Peuch, V.-H., Razinger, M., Remy, S., Schulz, M., and Suttie, M.: The CAMS reanalysis of atmospheric composition, Atmos. Chem. Phys., 19, 3515–3556, https://doi.org/10.5194/acp-19-3515-2019, 2019.
James, E., Ahmadov, R., and Grell, G.: Realtime Wildfire Smoke Prediction in the United States: the HRRR-Smoke Model, in: EGU General Assembly Conference Abstracts, vol. 20 of EGU General Assembly Conference Abstracts, 19526, 2018.
Jiang, Y., Yang, X-Q., and Liu, X.: Seasonality in anthropogenic aerosol effects on East Asian climate simulated with CAM5, J. Geophys. Res.-Atmos., 120, 10837–10861, https://doi.org/10.1002/2015JD023451, 2015.
Jouan, C., Milbrandt, J. A., Vaillancourt, P., Chosson, F., and Morrison, H.: Adaptation of the Predicted Particles Properties (P3) microphysics scheme for large-scale numerical weather prediction, Weather Forecast., 35, 2541–2565, 2020.
Jung, J., Souri, A. H., Wong, D., Lee, S., Jeon, W., Kim, J., and Choi, Y.: The impact of the direct effect of aerosols on meteorology and air quality using aerosol optical depth simulation during the KORUS-AQ campaign, J. Geophys. Res.-Atmos., 124, 8303–8319, https://doi.org/10.1029/2019JD030641, 2019.
Kochanski, A. K., Jenkins, M. A., Yedinak, K., Mandel, J., Beezley, J., and Lamb, B.: Toward an integrated system for fire, smoke and air-quality simulations, Int. J. Wildland Fire, 25, 534–568, 2016.
Kochanski, A. K., Malia, D. V., Fearon, M. G., Mandel, J., Souri, A. H., and Brown, T.: Modelling wildfire smoke feedback mechanisms using a coupled fire-atmosphere model with a radiatively active aerosol scheme, J. Geophys. Res.-Atmos., 124, 9099–9116, 2019.
Krause, P., Boyle, D. P., and Bäse, F.: Comparison of different efficiency criteria for hydrological model assessment, Adv. Geosci., 5, 89–97, https://doi.org/10.5194/adgeo-5-89-2005, 2005.
Legates, D. R. and McCabe Jr, G. J.: Evaluating the use of “goodness-of-fit” measures in hydrologic and hydroclimatic model validation, Water Resour. Res., 35, 233–241, 1999.
Levy, R. C., Mattoo, S., Munchak, L. A., Remer, L. A., Sayer, A. M., Patadia, F., and Hsu, N. C.: The Collection 6 MODIS aerosol products over land and ocean, Atmos. Meas. Tech., 6, 2989–3034, https://doi.org/10.5194/amt-6-2989-2013, 2013.
Linn, R., Reisner, J., Colman, J. J., and Winterkamp, J.: Studying wildfire behavior using FIRETEC, Int. J. Wildland Fire, 11, 233–246, 2002.
Lu, Z. and Sokolik, I. N.: Examining the impact of smoke on frontal clouds and precipitation during the 2002 Yakutsk wildfires using the WRF-Chem-SMOKE model and satellite data, J. Geophys, Res.-Atmos., 122, 12765–12785, https://doi.org/10.1002/2017JD027001, 2017.
Luig, A., Bofinger, S., and Beyer, H. G.: Analysis of confidence intervals for the prediction of regional wind power output, in: Proc. European Wind Energy Conference, Copenhagen, 725–728, available at: https://www.researchgate.net/publication/228793040_Analysis_of_confidence_intervals_for_the_prediction_of_regional_wind_power_output (last access: 23 August 2020), 2001.
Makar, P. A., Moran, M. D., Schultz, M. T., and Taylor, A.: Speciation of volatile organic compound emissions for regional air quality modeling of particulate matter and ozone, J. Geophys. Res., 108, 4041, https://doi.org/10.1029/2001JD000797, 2003.
Makar, P. A., Gong, W., Milbrandt, J., Hogrefe, C., Zhang, Y., Curci, G., Zabkar, R., Im, U., Balzarini, A., Baro, R., Bianconi, R., Cheung, P., Forkel, R., Gravel, S., Hirtl, H., Honzak, L., Hou, A., Jimenz-Guerrero, P., Langer, M., Moran, M. D., Pabla, B., Perez, J. L., Pirovano,G., San Jose,R., Tuccella, P., Werhahn, J., Zhang, J., and Galmarini, S.: Feedbacks between air pollution and weather, part 1: Effects on weather, Atmos. Environ., 115, 442–469, 2015a.
Makar, P. A., Gong, W., Hogrefe, C., Zhang, Y., Curci, G., Zabkar, R., Milbrandt, J., Im, U., Balzarini, A., Baro, R., Bianconi, R., Cheung, P., Forkel, R., Gravel, S., Hirtl, H., Honzak, L., Hou, A., Jimenz-Guerrero, P., Langer, M., Moran, M. D., Pabla, B., Perez, J. L., Pirovano, G., San Jose,R., Tuccella, P., Werhahn, J., Zhang, J., and Galmarini, S.: Feedbacks between air pollution and weather, part 2: Effects on chemistry, Atmos. Environ., 115, 499–526, 2015b.
Makar, P. A., Staebler, R. M., Akingunola, A., Zhang, J., McLinden, C., Kharol, S. K., Pabla, B., Cheung, P., and Zheng, Q.: The effects of forest canopy shading and turbulence on boundary layer ozone, Nat. Commun., 8, 15243, https://doi.org/10.1038/ncomms15243, 2017.
Makar, P. A., Stroud, C., Akingunola, A., Zhang, J., Ren, S., Cheung, P., and Zheng, Q.: Vehicle induced turbulence and atmospheric pollution, Atmos. Chem. Phys. Discuss. [preprint], https://doi.org/10.5194/acp-2020-1243, in review, 2021.
Mallet, M., Solmon, F., Roblou, L., Peers, F., Turquety, S., Waquet, F., Jethva, H., and Torres, O.: Simulation of optical properties and direct and indirect radiative effects of smoke aerosols over marine stratocumulus clouds during summer 2008 in California with the regional climate model RegCM, J. Geophys. Res.-Atmos., 122, 10312–10337, https://doi.org/10.1002/2017JD026905, 2017.
Mandel, J., Beezley, J. D., and Kochanski, A. K.: Coupled atmosphere-wildland fire modeling with WRF 3.3 and SFIRE 2011, Geosci. Model Dev., 4, 591–610, https://doi.org/10.5194/gmd-4-591-2011, 2011.
Markowicz, K. M., Pakszys, R., Ritter, C., Zielensi, T., Udisti, R., Cappelletti, D., Mazzola, M., Shobara, M., Xian, P., Zawadzka, O., Lisok, J., Petelski, T., Makuch, P., and Karasinksi, G.: Impact of North American intense fires on aerosol optical properties measured over the European Arctic in July 2015, J. Geophys. Res.-Atmos., 121, 14487–14512, https://doi.org/10.1002/2016JD025310, 2016.
Milbrandt, J. A. and Morrison, H.: Parameterization of Cloud Microphysics Based on the Prediction of Bulk Ice Particle Properties. Part III: Introduction of Multiple Free Categories, J. Atmos. Sci., 73, 975–995, https://doi.org/10.1175/JAS-D-15-0204.1, 2016.
Milbrandt, J. A. and Yau, M. K.: A multimoment bulk microphysics parameterization. Part 1: analysis of the role of the spectral shape parameter, J. Atmos. Sci., 62, 3051–3064, 2005a.
Milbrandt, J. A. and Yau, M. K.: A multimoment bulk microphysics parameterization. Part II: a proposed three-moment closure and scheme, J. Atmos. Sci., 62, 3065–3081, 2005b.
Milbrandt, J. A., Bélair, S., Faucher, M., Vallée, M., Carrera, M. L., and Glazer, A.: The pan-Canadian High Resolution (2.5 km) Deterministic Prediction System, Weather Forecast., 31, 1791–1816, 2016.
Moran, M. D., Pavlovic, R., and Anselmo, D.: Regional air quality deterministic prediction system (RAQDPS): update from version 019 to version 020, Environment and Climate Change Canada, Montreal, available at: https://collaboration.cmc.ec.gc.ca/cmc/CMOI/product_guide/docs/tech_notes/technote_raqdps-v20_20180918_e.pdf (last access: 17 June 2021), 2018.
Morrison, H. and Grabowski, W. W.: Modeling supersaturation and subgrid-scale mixing with two-moment warm bulk microphysics, J. Atmos. Sci., 65, 792–812, https://doi.org/10.1175/2007JAS2374.1, 2008.
Morrison, H. and Milbrandt, J. A.: Parameterization of Cloud Microphysics Based on the Prediction of Bulk Ice Particle Properties. Part I: Scheme Description and Idealized Tests, J. Atmos. Sci., 72, 287–311, https://doi.org/10.1175/JAS-D-14-0065.1, 2015.
Myhre, G., Shindell, D., Bréon, F.-M., Collins, W., Fuglestvedt, J., Huang, J., Koch, D., Lamarque, J.-F., Lee, D., Mendoza, B., Nakajima, T., Robock, A., Stephens, G., Takemura, T., and Zhang, H.: Anthropogenic and Natural Radiative Forcing, in: Climate Change 2013: The Physical Science Basis. Contribution of Working Group I to the Fifth Assessment Report of the Intergovernmental Panel on Climate Change, edited by: Stocker, T. F., Qin, D., Plattner, G.-K., Tignor, M., Allen, S. K., Boschung, J., Nauels, A., Xia, Y., Bex, V., and Midgley, P. M., Cambridge University Press, Cambridge, UK and New York, USA, 2013.
Nazarenko, L., Rind, D., Tsigaridis, K., Del Genio, A. D., Kelly, M., and Tausnev, N.: Interactive nature of climate change and aerosol forcing, J. Geophys. Res.-Atmos., 122, 3457–3480, https://doi.org/10.1002/2016JD025809, 2017.
Oeropoulis, L., Cho, N., and Lee, D.: A global survey of apparent aerosol-cloud interaction signals, J. Geophys. Res.-Atmos., 125, e2019JD031287, https://doi.org/10.1029/2019JD031287, 2020.
O'Neill, N. T., Eck, T. G., Holben, B. N., Smirnov, A., Royer, A., and Li, Z.: Optical properties of boreal forest fire smoke derived from Sun photometry, J. Goephys. Res., 107, 4125, https://doi.org/10.1029/2001JD000877, 2002.
Palacios-Peña, L., Baró, R., Baklanov, A., Balzarini, A., Brunner, D., Forkel, R., Hirtl, M., Honzak, L., López-Romero, J. M., Montávez, J. P., Pérez, J. L., Pirovano, G., San José, R., Schröder, W., Werhahn, J., Wolke, R., Žabkar, R., and Jiménez-Guerrero, P.: An assessment of aerosol optical properties from remote-sensing observations and regional chemistry–climate coupled models over Europe, Atmos. Chem. Phys., 18, 5021–5043, https://doi.org/10.5194/acp-18-5021-2018, 2018.
Pan, L., Kim, H. C., Lee, P., Saylor, R., Tang, Y., Tong, D., Baker, B., Kondragunta, S., Xu, C., Ruminski, M. G., Chen, W., Mcqueen, J., and Stajner, I.: Evaluating a fire smoke simulation algorithm in the National Air Quality Forecast Capability (NAQFC) by using multiple observation data sets during the Southeast Nexus (SENEX) field campaign, Geosci. Model Dev. Discuss. [preprint], https://doi.org/10.5194/gmd-2017-207, 2017.
Pavlovic, R., Chen, J., Anderson, K., Moran, M. D., Beaulieu, P. A., Davignon, D. and Cousineau, S.: The FireWork air quality forecast system with near-real-time biomass burning emissions: Recent developments and evaluation of performance for the 2015 North American wildfire season, J. Air Waste Manage., 66, 819–841, 2016.
Peace, M., Mattner, T., Mills, G., Kepert, J., and McCaw, L.: Fire-modified meteorology in a coupled fire-atmosphere model, J. Appl. Meteorol Clim., 54, 704–720, 2015.
Penner, J.E, Zhou, C., Garnier, A., and Mitchell, D. L.: Anthropogenic aerosol indirect effects in cirrus clouds, J. Geophys. Res.-Atmos., 123, 11652–11677, https://doi.org/10.1029/2018JD029204, 2018.
Pinson, P. and Kariniotakis, G.: On-line adaptation of confidence intervals based on weather stability for wind power forecasting, in: Proc. of the Global Wind Power Conference, Chicago, USA, available at: https://hal-mines-paristech.archives-ouvertes.fr/hal-00529488/document (last access: 23 August 2020), 2004.
Pinto, J. O., Grim, J. A., and Steinter, M.: Assessment of the High-Resolution Rapid Refresh model's ability to predict mesoscale convective systems using object-based evaluation, J. Atmos. Sci., 30, 892–913, 2015.
Rosenfeld, D., Lohmann, U., Raga, G. B., O'Dowd, C. D., Kulmala, M., Fuzzi, S., Reissel, A., and Andreae, M. O.: Flood or drought: How do aerosols affect precipitation? Science, 321, 1309–1313, 2008.
Rothenberg, D., Avramov, A., and Wang, C.: On the representation of aerosol activation and its influence on model-derived estimates of the aerosol indirect effect, Atmos. Chem. Phys., 18, 7961–7983, https://doi.org/10.5194/acp-18-7961-2018, 2018.
Sandu, A. and Sander, R.: Technical note: Simulating chemical systems in Fortran90 and Matlab with the Kinetic PreProcessor KPP-2.1, Atmos. Chem. Phys., 6, 187–195, https://doi.org/10.5194/acp-6-187-2006, 2006.
Saponaro, G., Kolmonen, P., Sogacheva, L., Rodriguez, E., Virtanen, T., and de Leeuw, G.: Estimates of the aerosol indirect effect over the Baltic Sea region derived from 12 years of MODIS observations, Atmos. Chem. Phys., 17, 3133–3143, https://doi.org/10.5194/acp-17-3133-2017, 2017.
Seity, Y., Brousseau, P., Malardel, S., Hello, G., Bénard, P., Bouttier, F., Lac, C., and Masson, V.: The AROME-France convective-scale operational model, Mon. Weathwer Rev., 139, 876–913, 2010.
Stockwell, W. R. and Lurmann, F. W.: Intercomparison of the ADOM and RADM gas-phase chemical mechanisms, Electric Power Institute Topical Report, Electric Power Institute, Palo Alto, California, 323 pp., 1989.
Stroud, C. A., Makar, P. A., Zhang, J., Moran, M. D., Akingunola, A., Li, S.-M., Leithead, A., Hayden, K., and Siu, M.: Improving air quality model predictions of organic species using measurement-derived organic gaseous and particle emissions in a petrochemical-dominated region, Atmos. Chem. Phys., 18, 13531–13545, https://doi.org/10.5194/acp-18-13531-2018, 2018.
Sundqvist, H.: Parameterization of condensation and associated clouds in models for weather prediction and general circulation simulation, in: Physically-Based Modelling and Simulation of Climate and Climatic Change, edited by: Schlesinger M. E., NATO ASI Series, Series C: Mathematical and Physical Sciences, vol. 243, Springer, Dordrecht, 1988.
Suzuki, K. and Takemura, T.: Perturbations to global energy budget due to absorbing and scattering aerosols, J. Geophys. Res.-Atmos., 124, 2194–2209, https://doi.org/10.1029/2018JD029808, 2019.
Takeishi, A., Strorelvmo, T., and Fierce, L.: Disentangling the microphysical effects of fire particles on convective clouds through a case study, J. Geophys. Res.-Atmos., 125, e2019JD031890, https://doi.org/10.1029/2019JD031890, 2020.
Twomey, S.: The influence of polution on the shortwave albedo of clouds, J. Atmos. Sci, 34, 1149–1152, 1977.
US EPA: Air Quality System (AQS), available at: https://www.epa.gov/aqs, last access: 17 June 2021.
Wang, J., Allen, D. J., Pickering, K. E., Li, Z., and He, H.: Impact of aerosol direct effect on East Asian air quality during the EAST-AIRE campaign, J. Geophys. Res.-Atmos., 121, 6534–6554, https://doi.org/10.1002/2016JD025108, 2016.
Whaley, C. H., Makar, P. A., Shephard, M. W., Zhang, L., Zhang, J., Zheng, Q., Akingunola, A., Wentworth, G. R., Murphy, J. G., Kharol, S. K., and Cady-Pereira, K. E.: Contributions of natural and anthropogenic sources to ambient ammonia in the Athabasca Oil Sands and north-western Canada, Atmos. Chem. Phys., 18, 2011–2034, https://doi.org/10.5194/acp-18-2011-2018, 2018.
Willmott, C. J., Robeson, S. M., and Matsuura, K.: A refined index of model performance, Int. J. Climatol., 32, 2088–2094, 2012.
Yu, S., Eder, B., Dennis, R., Chu, S.-H., and Schwartz, S. E.: New unbiased metrics for evaluation of air quality models, Atmos. Sci. Lett., 7, 26–34, 2006.
Zhang, L., Brook, J. R., and Vet, R.: A revised parameterization for gaseous dry deposition in air-quality models, Atmos. Chem. Phys., 3, 2067–2082, https://doi.org/10.5194/acp-3-2067-2003, 2003.
Zhao, X., Liu, Y., Yu, F., and Heidinger, A. K.: Using long-term satellite observations to identify sensitive regimes and active regions of aerosol indirect effects for liquid clouds over global oceans, J. Geophys. Res.-Atmos., 123, 457–472, https://doi.org/10.1002/2017JD027187, 2018.
Zhu, J. and Penner, J. E.: Indirect effects of secondary organic aerosol on cirrus clouds, J. Geophys., Res.-Atmos., 125, e2019JD032233, https://doi.org/10.1029/2019JD032233, 2020.