the Creative Commons Attribution 4.0 License.
the Creative Commons Attribution 4.0 License.
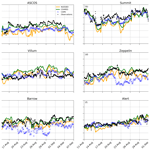
Role of oceanic ozone deposition in explaining temporal variability in surface ozone at High Arctic sites
Johannes G. M. Barten
Laurens N. Ganzeveld
Gert-Jan Steeneveld
Maarten C. Krol
Dry deposition is an important removal mechanism for tropospheric ozone (O3). Currently, O3 deposition to oceans in atmospheric chemistry and transport models (ACTMs) is generally represented using constant surface uptake resistances. This occurs despite the role of solubility, waterside turbulence and O3 reacting with ocean water reactants such as iodide resulting in substantial spatiotemporal variability in O3 deposition and concentrations in marine boundary layers. We hypothesize that O3 deposition to the Arctic Ocean, having a relatively low reactivity, is overestimated in current models with consequences for the tropospheric concentrations, lifetime and long-range transport of O3. We investigate the impact of the representation of oceanic O3 deposition to the simulated magnitude and spatiotemporal variability in Arctic surface O3.
We have integrated the Coupled Ocean-Atmosphere Response Experiment Gas transfer algorithm (COAREG) into the mesoscale meteorology and atmospheric chemistry model Polar-WRF-Chem (WRF) which introduces a dependence of O3 deposition on physical and biogeochemical drivers of oceanic O3 deposition. Also, we reduced the O3 deposition to sea ice and snow. Here, we evaluate WRF and CAMS reanalysis data against hourly averaged surface O3 observations at 25 sites (latitudes > 60∘ N). This is the first time such a coupled modeling system has been evaluated against hourly observations at pan-Arctic sites to study the sensitivity of the magnitude and temporal variability in Arctic surface O3 on the deposition scheme. We find that it is important to nudge WRF to the ECMWF ERA5 reanalysis data to ensure adequate meteorological conditions to evaluate surface O3.
We show that the mechanistic representation of O3 deposition over oceans and reduced snow/ice deposition improves simulated Arctic O3 mixing ratios both in magnitude and temporal variability compared to the constant resistance approach. Using COAREG, O3 deposition velocities are in the order of 0.01 cm s−1 compared to ∼ 0.05 cm s−1 in the constant resistance approach. The simulated monthly mean spatial variability in the mechanistic approach (0.01 to 0.018 cm s−1) expresses the sensitivity to chemical enhancement with dissolved iodide, whereas the temporal variability (up to ±20 % around the mean) expresses mainly differences in waterside turbulent transport. The mean bias for six sites above 70∘ N reduced from −3.8 to 0.3 ppb with the revision to ocean and snow/ice deposition. Our study confirms that O3 deposition to high-latitude oceans and snow/ice is generally overestimated in ACTMs. We recommend that a mechanistic representation of oceanic O3 deposition is preferred in ACTMs to improve the modeled Arctic surface O3 concentrations in terms of magnitude and temporal variability.
- Article
(6915 KB) - Full-text XML
- BibTeX
- EndNote
Tropospheric ozone (O3) is the third most important greenhouse gas and a secondary air pollutant negatively affecting human health (Nuvolone et al., 2018) and plant growth (Ainsworth et al., 2012) due to its oxidative character. O3 shows a large spatiotemporal variability due to its relatively short lifetime (3–4 weeks) in the free troposphere compared to other greenhouse gases. Its main sources are chemical production and entrainment from the stratosphere. Its main sinks are chemical destruction and deposition to the Earth's surface (Young et al., 2018; Tarasick et al., 2019). Understanding the Arctic O3 budget is of particular interest because its remote location implies that anthropogenic sources and sinks are generally absent. This implies that these Arctic O3 observations allow us to determine large-scale trends in tropospheric O3 (Helmig et al., 2007b; Gaudel et al., 2020; Cooper et al., 2020). In the Arctic, routine tropospheric O3 observations indicate an increasing trend up to the early 2000s which has been leveling off (Oltmans et al., 2013; Cooper et al., 2014) or decreasing at individual sites (Cooper et al., 2020) in the last decade. This upward trend can be attributed to increased emissions of precursors in the midlatitudes (Cooper et al., 2014; Lin et al., 2017), but stratosphere-to-troposphere transport may also have played a role (Pausata et al., 2012). Local emissions of precursors are expected to become an important source of Arctic O3 concentrations due to the warming Arctic climate and increasing local economic activity (Marelle et al., 2016; Law et al., 2017). This underlines the need for understanding the sources and sinks of Arctic tropospheric O3 and to accurately representing them in atmospheric chemistry and transport models (ACTMs).
On the global scale, dry deposition accounts for ∼ 25 % of the total sink term (Lelieveld and Dentener, 2000) in ACTM simulations and is especially important for the O3 budget in the atmospheric boundary layer (ABL). Dry deposition in ACTMs is often represented as a resistance in series approach (Wesely, 1989). Herein, the total resistance rt consists of three serial resistances: the aerodynamic resistance (ra) representing turbulent transport to the surface, the quasi-laminar sublayer resistance (rb) representing diffusion close to the surface and the surface resistance (rs) expressing the efficiency of removal by the surface. The dry-deposition velocity (Vd) is then evaluated as the reciprocal of rt. The ra term mainly depends on the stability of the atmosphere and friction velocity (u*) (Padro, 1996; Toyota et al., 2016). The rb term also scales with u* and varies with the diffusivity of the chemical species (Wesely and Hicks, 2000). Low-solubility gases like O3 have a high rs, in comparison to the relatively small ra+rb term, which dominates the magnitude of the O3 dry-deposition velocity (). Thus, accurately representing the surface uptake efficiency of O3 is crucial. During episodes of low wind speeds, the ra+rb term can pose an additional restriction on the exchange of O3 with oceans (Fairall et al., 2007).
Observed O3 deposition to oceans (e.g., Chang et al., 2004; Clifford et al., 2008; Helmig et al., 2012) and coastal waters (e.g., Gallagher et al., 2001) is relatively slow (∼ 0.01–0.1 cm s−1). However, oceanic O3 is relevant for the global O3 deposition budget due to the large surface area of water bodies (Ganzeveld et al., 2009; Hardacre et al., 2015). Recent experimental and modeling studies indicate the spatiotemporal variability in oceanic O3 uptake efficiency (Ganzeveld et al., 2009; Helmig et al., 2012; Luhar et al., 2018). However, most ACTMs often use a constant O3 surface uptake efficiency of 2000 cm s−1 to water bodies, proposed by Wesely (1989), resulting in a simulated ocean of ∼ 0.05 cm s−1. The observed shows a larger variability including also a dependency on wind speed and sea surface temperature (SST) (Helmig et al., 2012). The turbulence-driven enhancement by wind speed (Fairall et al., 2007) is complemented by a strong chemical enhancement of oceanic O3 deposition associated with its chemical destruction through the oxidation of ocean water reactants such as dissolved iodide and dissolved organic matter (DOM) (Chang et al., 2004). Mechanistic O3 deposition representations in models include the physical and biogeochemical drivers of the exchange of O3 in surface waters (Fairall et al., 2007, 2011; Ganzeveld et al., 2009; Luhar et al., 2017, 2018). Dissolved iodide is deemed to be the main reactant of O3 in surface waters (Chang et al., 2004) and therefore often applied in these representations. Some studies only consider dissolved iodide as a reactant (Luhar et al., 2017; Pound et al., 2020), whereas Ganzeveld et al. (2009) also included DOM as one reactant contributing to the chemical enhancement of oceanic O3 deposition. These mechanistic deposition representations appeared to be crucial for O3 dry-deposition modeling, the marine ABL O3 concentrations and the potentially involved feedback mechanisms such as the release of halogen compounds as a function of O3 deposition (Prados Roman et al., 2015).
Up until now, earlier studies on global-scale oceanic O3 deposition (Ganzeveld et al., 2009; Luhar et al., 2017) evaluated monthly mean surface O3 observations (Pound et al., 2020). The implementation of these mechanistic exchange methods in ACTMs, in particular the method proposed by Luhar et al. (2018) using a two-layer model representation (compared to a bulk layer version by Ganzeveld et al., 2009), results in a ∼ 50 % reduction in the global mean which affects the tropospheric O3 burden (Pound et al., 2020). The mechanistic representation in Pound et al. (2020) especially results in a simulated decrease in to cold polar waters with relatively low reactivity. Simulated can be as low as 0.01 cm s−1 compared to the commonly applied of 0.05 cm s−1 in the constant surface uptake resistance approach (Pound et al., 2020). However, the hypothesized deposition reduction to cold waters is expected to substantially affect Arctic ABL O3 concentrations on relatively short timescales (sub-monthly) and potentially improve operational Arctic O3 forecasts, e.g., the air quality forecasts by the Copernicus Atmosphere Monitoring Service (CAMS) (Inness et al., 2019).
The evaluation of simulated oceanic O3 deposition in the Arctic is hampered by a lack of O3 ocean–atmosphere flux observations and consequently relies on a comparison of simulated and observed surface O3 concentrations not only regarding the magnitude but in particular the temporal variability. We hypothesize that on synoptic timescales these concentrations are controlled by temporal variability in the main physical drivers of oceanic O3 deposition, e.g., atmospheric and waterside turbulence mainly as a function of wind speed. Chemical enhancement of, e.g., iodide to O3 deposition is anticipated to control the long-term (months) baseline level of more associated with anticipated long-term (e.g., seasonal) changes in ocean water biogeochemical conditions (Sherwen et al., 2019). This evaluation of Arctic spatiotemporal O3 concentrations aims to better understand the role of ocean and sea ice deposition as a potentially important but also uncertain sink impacting Arctic air pollution (Arnold et al., 2016). Furthermore, the projected opening of the Arctic Ocean, as a result of climate change, urges us to improve our understanding of Arctic Ocean–atmosphere exchange.
We aim to identify and quantify the impact of a mechanistic representation of O3 deposition in explaining observed hourly Arctic surface O3 concentrations, both in terms of magnitude and temporal variability. A mesoscale coupled meteorology–atmospheric chemistry model is evaluated against a large dataset of pan-Arctic O3 observations at a high-resolution (hourly) timescale for the end of summer 2008. Using a much higher spatial and temporal resolutions compared to other global modeling studies, we aim to evaluate to what extent the role of spatiotemporal variability in O3 deposition explains observed surface O3 concentrations particularly regarding temporal variability. We also indicate the role of meteorology in simulating these O3 concentrations by nudging the simulated synoptic conditions towards an atmospheric reanalysis dataset.
2.1 Regional coupled meteorology–chemistry model
We use the Weather Research and Forecasting model (v4.1.1) coupled to chemistry (Chem) (Grell et al., 2005) and optimized for Polar regions (Hines and Bromwich, 2008). Polar-WRF-Chem (hereafter WRF) is a non-hydrostatic mesoscale numerical weather prediction and atmospheric chemistry model used for operational and research purposes. Figure 1 shows the selected study area including the locations of surface O3 observational sites selected for this study (more information in Sect. 2.5). WRF is set up with a polar projection centered at 90∘ N, 250 × 250 horizontal grid points (30 × 30 km resolution) and 44 vertical levels up to 100 hPa, with a finer vertical grid spacing in the ABL and lower troposphere. The simulation period is 8 August to 7 September 2008 including 3 d of spin-up. This end-of-summer 2008 period is chosen (1) to limit the role of active halogen chemistry during springtime (Pratt et al., 2013; Thompson et al., 2017; Yang et al., 2020) and (2) the additional availability of O3 observations in the High Arctic over sea ice from the Arctic Summer Cloud Ocean Study (ASCOS) campaign (Paatero et al., 2009). The ECMWF ERA5 meteorology (0.25∘ × 0.25∘) (Hersbach et al., 2020) and CAMS reanalysis chemistry (0.75∘ × 0.75∘) (Inness et al., 2019) products are used for the initial and boundary conditions. Boundary conditions, SSTs and sea ice fractions are updated every 3 h to these reanalysis products to allow for the sea ice retreat during the simulation. Other relevant parameterization schemes and emission datasets have been listed in Table A1 and are mostly based on Bromwich et al. (2013).
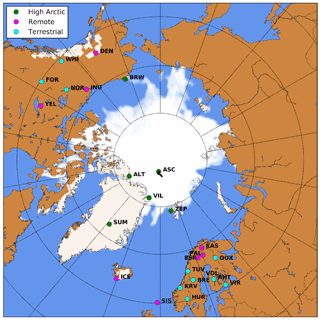
Figure 1WRF domain including sea ice and snow cover at the start of the simulation. Locations with surface observations O3 are indicated in green (High Arctic), magenta (Remote) and cyan (Terrestrial) (see Sect. 2.5). The drifting path of the ASCOS campaign during the simulation is indicated with the black line.
2.2 Nudging to ECMWF ERA5
The first WRF simulation, without any adjustments to O3 deposition, indicated that WRF was misrepresenting the temporal variability in surface O3 observations, most prominently starting from a few days into the simulation. We hypothesize that this misrepresentation is caused by deviations in the synoptic conditions in the free-running WRF simulation. This was confirmed with a comparison of simulated and satellite observed wind speeds above oceans at a spatial resolution of 0.25∘ × 0.25∘ (Wentz and Meissner, 2004). To overcome the impact of this deficiency on our O3 study, nudging is applied to ensure an optimal model evaluation with observations. Hence, WRF is nudged every 3 h to the ECMWF ERA5 specific humidity, temperature and wind fields in the free troposphere with nudging coefficients of 1 × 10−5, 3 × 10−4 and 3 × 10−4 s−1, respectively.
2.3 Representation of ocean–atmosphere gas exchange
The Coupled Ocean-Atmosphere Response Experiment (COARE) (Fairall et al., 1996) has been developed to study physical exchange processes (sensible heat, latent heat and momentum) at the ocean–atmosphere interface. Later, COARE has been extended to include the exchange of gaseous species such as O3, dimethyl sulfide (DMS) and carbon dioxide (CO2) (Fairall et al., 2011). Many studies have used the COARE Gas transfer algorithm (COAREG) in combination with eddy-covariance measurements to study the effects of wind speed and sea state on ocean–atmosphere gas exchange (e.g., Helmig et al., 2012; Blomquist et al., 2017; Bell et al., 2017; Porter et al., 2020). Furthermore, the COAREG algorithm has also been previously used in global O3 modeling studies (Ganzeveld et al., 2009). The choice for COAREG is further motivated by the consistent coupling with other species such as DMS.
Here we use COAREG version 3.6, which is extended with a two-layer scheme for surface resistance compared to the previous version described by Fairall et al. (2007, 2011). The two-layer scheme is similar to Luhar et al. (2018) building upon a first application of a one-layer version of COAREG by Ganzeveld et al. (2009). In that study, chemical enhancement of ocean O3 deposition by its reaction with iodide was considered using a global climatology of ocean surface water concentrations of nitrate serving as a proxy for oceanic iodide concentrations (I). Besides nitrate, satellite-derived chlorophyll-α concentrations have been used as a proxy for I (Oh et al., 2008). Since then, alternative parameterizations of oceanic I have been proposed (e.g., MacDonald et al., 2014) using SST as a proxy for this reactant. In COAREG, chemical reactivity of O3 with I is present through the depth of the oceanic mixing layer. O3 loss by waterside turbulent transfer is negligible in the top water layer (few micrometers), but is accounted for in the underlying water column. The waterside turbulent transfer term is especially relevant for relatively cold waters because the chemical enhancement term is then relatively low (Fairall et al., 2007; Ganzeveld et al., 2009; Luhar et al., 2017). The last two important waterside processes that determine the total O3 deposition are molecular diffusion and solubility of O3 in seawater which both depend on the SST. In Appendix B we list the formulation of the air side and waterside resistance terms in the COAREG routine applied in this study and show the sensitivity to the environmental factors wind speed, SST and I for typical Arctic conditions.
The COAREG algorithm is coupled such that WRF provides the meteorological and SST input for the COAREG routine. In turn, the COAREG calculated ocean–atmosphere exchange velocities are used in the WRF model to calculate the oceanic O3 deposition flux replacing the default oceanic O3 deposition fluxes calculated by the Wesely (1989) scheme reflecting use of the default constant rs of 2000 s m−1. For grid boxes with fractional sea ice cover, COAREG replaces the Wesely deposition scheme for the fraction that is ice free. Note that in this study, only O3 ocean–atmosphere exchange is represented by COAREG not having modified simulations of ocean–atmosphere exchange of other compounds (e.g., DMS).
Moreover, we apply the monthly mean I distribution by Sherwen et al. (2019) (0.125∘ × 0.125∘ resolution) which applies a machine learning approach, namely the random forest regressor algorithm (Pedregosa et al., 2011), using various physical and chemical variables such as SST, nitrate, salinity and mixed layer depth. This distribution replaces the previously applied I estimations only using SST (Chance et al., 2014; MacDonald et al., 2014). At high latitudes, these I distributions are highly uncertain due to the limited number of observations. The choice for Sherwen et al. (2019) is motivated by the most accurate representation of observed I by the introduction of other predictors besides SST. Furthermore, this product will be further updated with newly available measurements. Figure C1 shows the spatial distribution of I used in the calculation of the O3 deposition velocities. Using the Sherwen et al. (2019) distribution for August/September we found I concentrations ranging between 30 and 80 nM for the open oceans up to 130 nM in coastal waters. In MacDonald et al. (2014) and Chance et al. (2014), I is solely a function of SST which leads to I in the order of 5 to 50 nM and thus low reactivity and O3 deposition velocities.
2.4 Deposition to snow and ice
Reported atmosphere–snow gas exchange spans a wide range of observed O3 deposition velocities. Some studies even report episodes of negative deposition fluxes (emissions) over snow or sea ice (Zeller, 2000; Helmig et al., 2009; Muller et al., 2012). Clifton et al. (2020a) recently summarized observed O3 deposition velocities to snow having a range of −3.6 to 1.8 cm s−1 with most of the observations indicating a deposition velocity between 0 and 0.1 cm s−1 for multiple snow-covered surfaces (e.g., grass, forest and sea ice). Generally, O3 concentrations in the interstitial air of the snowpack are lower than in the air above making the snowpack not a direct source of O3 in terms of emissions (Clifton et al., 2020a). However, the emissions of O3 precursors from the snowpack can enhance O3 production in the very stable atmosphere above the snowpack (Clifton et al., 2020a). Helmig et al. (2007a) investigated the sensitivity of a global chemistry and tracer transport model to the prescribed O3 deposition velocity and found the best agreement between modeled and observed O3 concentrations at four Arctic sites by applying deposition velocities in the order of 0.00–0.01 cm s−1. Following Helmig et al. (2007a) we have increased the O3 surface uptake resistance (rs) for snow and ice land use classes to 104 s m−1. This corresponds to total deposition velocities of ≤ 0.01 cm s−1, which is a reduction of ∼ 66 % compared to the Wesely deposition routine that is the default being applied in WRF (Grell et al., 2005).
2.5 Observational data of surface ozone
The new modeling setup, including nudging to ECMWF ERA5 and the revised O3 deposition to snow, ice and oceans, is evaluated against observational data of pan-Arctic surface O3 concentrations. We expect that the different representation of O3 deposition mostly affects O3 concentrations in the ABL. Therefore, we evaluate our simulations against hourly averaged surface O3 observations from 25 measurement sites above 60∘ N. These sites are further categorized in three site selections: “High Arctic”, “Terrestrial” and “Remote”. High Arctic refers to sites having latitudes > 70∘ N and for which we expect that the deposition footprint is a combination of ocean and sea ice (e.g., Helmig et al., 2007b). The Terrestrial sites are located below 70∘ N and show a clear diurnal cycle in observed O3. Sites are characterized as Terrestrial when the average observed minimum nighttime mixing ratio is > 8 ppb smaller than the average observed maximum daytime mixing ratio during the ∼ 1 month of simulation. This criterion is based on a preparatory analysis of the observational data, footprint and site characteristics. The Remote sites have been identified as such based on their location below 70∘ N and showing no clear diurnal cycle in O3 concentrations. The analysis also includes the observations during the Arctic Summer Cloud Ocean Study (ASCOS) campaign, when the icebreaker Oden was located in the Arctic sea ice (Tjernström et al., 2012). In total, 25 surface O3 measurement sites are included (Fig. 1), of which 6, 8 and 11 sites are characterized as High Arctic, Remote and Terrestrial sites, respectively. A full list of available measurement sites is available in Table D1.
2.6 Overview of performed simulations
In total, we perform two simulations. The first WRF simulation (NUDGED) is a run with the setup described in Sect. 2.1 and nudged with the synoptic conditions to the ECMWF ERA5 product as described in Sect. 2.2. The second simulation (COAREG) includes also includes the adjustments to the O3 deposition to oceans as described in Sect. 2.3 and the O3 deposition to snow and ice as described in Sect. 2.4. Furthermore, we also compare our results with the state-of-the-art CAMS global reanalysis data product (Inness et al., 2019). This product has a temporal resolution of 3 h, a spatial resolution of 0.75∘ × 0.75∘ and does not include a mechanistic representation of ocean–atmosphere O3 exchange. CAMS assimilates satellite observations of O3 but it does not assimilate O3 observations from radiosondes or in situ measurement sites such as the 25 sites used in the evaluation presented here. This implies that lower-tropospheric O3 is weakly constrained by observations in this CAMS product making an accurate model representation of the sources and sinks important. We opted to include the CAMS reanalysis data as another tool to study Arctic surface O3 and to address potential limitations in its model setup. Moreover, CAMS is being widely used for air quality forecasts and assessments but also to constrain regional-scale modeling experiments such as presented in this study. Therefore, an analysis of the performance of the CAMS reanalysis data might also benefit future Arctic air quality assessments.
3.1 Dry-deposition budgets and distribution
Figure 2a and b show the mean deposition velocities for the NUDGED and COAREG runs, respectively. As expected, in the NUDGED run (Fig. 2a) the mean to oceans is in the order of 0.05 cm s−1. Furthermore, the spatial distribution shows a relatively low heterogeneity and no increase in deposition velocities towards the warmer waters. The COAREG run (Fig. 2b) provides a mean in the order of 0.01 cm s−1 for the Arctic Ocean > 70∘ N up to 0.018 cm s−1 for oceans with high I concentrations (Fig. C1). Simulated oceanic O3 deposition is elevated in coastal waters (e.g., Baltic Sea and around the Bering Strait) with I concentrations reaching up to 130 nM compared to 30–50 nM for the open Arctic Ocean waters (Fig. C1). This highlights the sensitivity of the COAREG scheme to chemical enhancement with dissolved iodide.
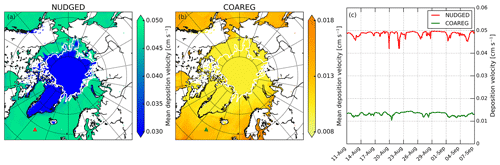
Figure 2Spatial distribution of the mean simulated O3 deposition velocity to snow/ice and oceans (cm s−1) for the (a) NUDGED and (b) COAREG simulations and (c) temporal variation in O3 deposition velocity (cm s−1) for the NUDGED (red) and COAREG (green) simulations. The red and green markers in (a) and (b) indicate the location of the time series shown in (c). To give an indication of the sea ice extent, the white contours show the sea ice fraction of 0.5 at the start of the simulation.
Figure 2c shows the temporal variability in for one of the grid boxes, which is in terms of temporal variability representative of the whole domain. The temporal variability in the NUDGED run is mainly governed by temporal variability in ra. During episodes with high wind speeds (> 10 m s−1), ra becomes so small that it is negligible over the constant surface uptake resistance of 2000 s m−1, corresponding to a maximum of 0.05 cm s−1. During episodes with low wind speeds (< 5 m s−1), reduced turbulent transport poses some additional restriction on O3 removal with increasing ra, which reduces the to ∼ 0.04 cm s−1. In the COAREG run, temporal variability in is also governed by wind speeds that control the waterside turbulent transport of O3 in seawater besides atmospheric turbulent transport. For high wind speeds, the waterside turbulent transport increases (Fig. B1) and more O3 is transported through the turbulent layers. For our simulation, we found that the temporal variability in O3 deposition due to waterside turbulent transport can be up to ±20 % around the mean. Only during episodes of very low wind speeds (< 2.5 m s−1) does the ra+rb term pose an additional restriction on O3 deposition in the COAREG run. Overall, the to oceans in the COAREG run is reduced by ∼ 60 %–80 % compared to the NUDGED run. The mean to snow and ice is reduced by ∼ 66 %, from ∼ 0.03 cm s−1 in the NUDGED run to ∼ 0.01 cm s−1 in the COAREG run.
Table 1Mean simulated O3 deposition velocity (±standard deviation) (cm s−1) and total simulated deposition budget (Tg O3 yr−1) for the NUDGED and COAREG runs to water, snow/ice and land each representing 37 %, 15 % and 48 % of the total surface area, respectively. The standard deviation gives an indication of the spatiotemporal variability in simulated O3 deposition velocities.

The temporal evolution in oceanic O3 deposition velocities simulated by the COAREG run appears to be on the low side of observed and of that simulated elsewhere (e.g., Chang et al., 2004; Oh et al., 2008; Ganzeveld et al., 2009). Chang et al. (2004) showed that can increase by a factor of 5 with wind speed increasing from 0 to 20 m s−1. Luhar et al. (2017) (their Fig. 7) shows a wide range of observed and simulated sensitivities to wind speed. Observations from the TexAQS06 summer campaign in the Gulf of Mexico show a large sensitivity to 10 m wind speeds even though the model seems unable to capture these high deposition velocities at high wind speeds (Luhar et al., 2017). However, Luhar et al. (2017) also shows that for the GasEx08 campaign in the cold Southern Ocean the sensitivity of observed and simulated to 10 m wind speeds is very limited. This limited sensitivity is most accurately represented by the modified two-layer reactivity scheme compared to the older one-layer scheme due to a more limited interaction between chemical reactivity and waterside turbulent transport (Luhar et al., 2017). Furthermore, the variability around the mean presented in Table 1 (0.012 ± 0.002 cm s−1) seems to correspond to the Oh et al. (2008) (0.016 ± 0.0015 cm s−1) 1-month simulation including O3 removal by I. In this study we show the intra-monthly variability in oceanic O3 deposition, which is expected to be relatively low compared to the seasonal variability which will also be driven by temporal changes in solubility and reactivity due to the seasonal changes in SST and I.
By estimating the total deposition flux for the water, snow/ice and land surfaces we can quantify the total simulated O3 deposition budget (Table 1) for the Arctic modeling domain. Land, not covered with snow or ice, is the dominant surface type for this specific domain setup in summer with 48 %. Combined with a relatively high simulated of ∼ 0.45 cm s−1, this is the most important sink, in terms of deposition, of simulated O3 with ∼ 135 Tg O3 yr−1. The simulated O3 deposition budget to water bodies, covering 37 % of the total surface area, contributes ∼ 10 % in the NUDGED run (15.4 Tg O3 yr−1) to the total O3 deposition sink. In the COAREG run, this reduces to only ∼ 3 % (4.6 Tg O3 yr−1) of the total O3 deposition sink. Simulated O3 deposition to snow and ice, covering 15 % of the total surface area, is the least important deposition sink removing 4.1 and 1.7 Tg O3 yr−1 in the NUDGED and COAREG runs, respectively.
3.2 Simulated and observed monthly mean surface ozone
Figure 3 shows the spatial distribution in the simulated mean surface O3 mixing ratios overlain with the observed mean surface O3 mixing ratios. In the NUDGED and COAREG runs (Fig. 3a and b, respectively) we find similar surface O3 mixing ratios of ∼ 15–20 ppb over the Russian, Canadian and Alaskan landmasses. Over Scandinavia, slightly higher surface O3 mixing ratios of ∼ 20–25 ppb are simulated due to more anthropogenic emissions of precursors in the EDGAR emission inventory and advection of O3 and its precursors from outside the domain. As expected, we find a limited effect of reduced deposition to water and snow/ice to the simulated mean O3 mixing ratios over land. In general, the model appears to simulate the mean observed surface O3 mixing ratios for the Remote and Terrestrial sites (all sites < 70∘ N) generally well without clear positive or negative bias. Due to the altitude effect, relatively high surface O3 concentrations are simulated over Greenland even though the deposition velocity to snow and the surrounding oceans is of similar magnitude (∼ 0.01 cm s−1).
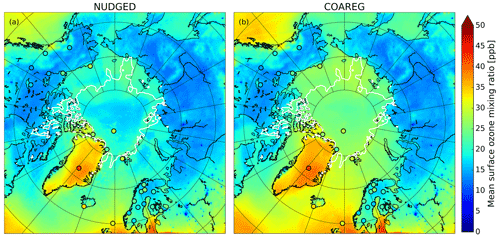
Figure 3Spatial distribution of the simulated mean surface O3 mixing ratio (ppb) for the (a) NUDGED and (b) COAREG runs. The filled circles indicate the mean observed ozone mixing ratios (ppb) for the simulated period. To indicate the sea ice extent, the white contours show the sea ice fraction of 0.5 at the start of the simulation.
The reduced O3 deposition to water and snow/ice surfaces, comparing the NUDGED and COAREG simulation results (Sect. 3.1, Table 1), appears to be limited in terms of relative changes in and the total simulated O3 deposition budget. However, these relatively small changes do substantially affect the simulated spatial distribution of surface O3 mixing ratios over oceans and sea ice as indicated in Fig. 3. We find that the NUDGED run (Fig. 3a) systematically underestimates the mean observed surface O3 mixing ratios for the High Arctic sites (all sites > 70∘ N) by ∼ 5–10 ppb, which appears to be caused by an overestimated deposition to ocean, snow and ice surfaces, also further substantiated by the following analysis of temporal variability in O3 concentrations (Sect. 3.3). Over the Arctic sea ice and oceans the ABL is typically very shallow and atmospheric turbulence is relatively weak. This suppresses vertical mixing and entrainment of O3-rich air from the free troposphere. Dry deposition of O3 to the ocean or snow/ice surfaces appears to be an important removal mechanism that has a large impact on O3 concentrations in these shallow ABLs (Clifton et al., 2020b) both in terms of magnitude but also temporal variability (see Sect. 3.4). In the COAREG run, surface O3 mixing ratios over oceans and Arctic sea ice have increased by up to 50 %. Furthermore, the reduced deposition to snow/ice has also clearly affected simulated surface O3 mixing ratios over Greenland. Most importantly, the negative bias in simulated surface O3 mixing ratios is reduced in the COAREG run with respect to the NUDGED run (see Sect. 3.3).
3.3 Simulated and observed hourly surface ozone
In this section we show how the application of the revised deposition scheme improves the model prediction scores of surface O3 concentrations reflected in a comparison of the simulated and observed hourly surface O3 mixing ratios at the three site selections (High Arctic, Remote and Terrestrial). To our knowledge, this is the first time such an oceanic O3 deposition scheme coupled to a meteorology–chemistry model has been evaluated against a large dataset of hourly surface O3 observations. Figure 4 shows a comparison between observed and simulated hourly surface O3 mixing ratios subdivided into the three site selections: High Arctic, Remote and Terrestrial. As expected, for the High Arctic sites (Fig. 4, top row) we find that the NUDGED run is underestimating the observed surface O3 mixing ratios with a mean bias of −3.8 ppb, which is also consistent with the findings in Fig. 3, where the NUDGED run appears to underestimate surface O3 mixing ratios in the High Arctic region. The COAREG run, having a reduced O3 deposition sink to oceans and snow/ice appears to better represent the surface O3 observations with a slight positive bias of 0.3 ppb. The mean absolute error (MAE) in the COAREG run is reduced to 4.7 ppb from 6.4 ppb for the NUDGED run. Furthermore, we find that the CAMS reanalysis data also underestimate surface O3 in the High Arctic with a bias of −5.0 ppb and an MAE of 6.8 ppb. Note that the performance for the WRF runs and CAMS reanalysis product varies for each observational site, which is further examined in Sect. 3.4.
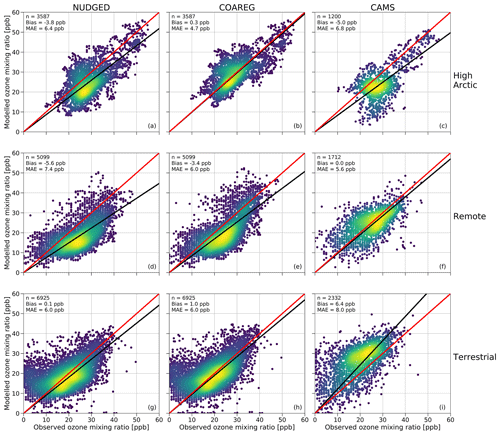
Figure 4Comparison of the hourly observed and simulated ozone mixing ratios (ppb) for the NUDGED (a, d, g) and COAREG (b, e, h) runs and CAMS data (c, f, i) for the High Arctic (a–c), Remote (d–f) and Terrestrial (TE) (g–i) sites. The red line indicates the 1 : 1 line and the black line indicates the ordinary least squares regression line through the origin. The number of data points (n), bias (ppb) and mean absolute error (MAE) (ppb) are shown in the top left corner. The colors represent the multivariate kernel density estimation with yellow colors having a higher density.
For the Remote sites (Fig. 4, middle row), having no clear diurnal cycle in surface O3, we again find an improvement by including the mechanistic ocean deposition routine and reduced snow/ice deposition. This improvement appears to be most pronounced for coastal sites like Stórhöfði (63.4∘ N, 20.3∘ W) and Inuvik (68.4∘ N, 133.7∘ W) with a reduction in the MAE of 32 % and 19 %, respectively (not shown here). Overall, the improvement for the COAREG compared to the NUDGED run in the Remote site selection is not as significant compared to the High Arctic sites, also because of the larger role of O3 deposition to land and vegetation, which remained unchanged in this study. We find that the CAMS data show the best performance for the Remote sites with no bias and with an MAE of 5.6 ppb.
For the Terrestrial sites (Fig. 4, bottom row), having a clear diurnal cycle in surface O3, the WRF runs slightly overestimate the observed surface O3 mixing ratios with mean biases of 0.1 and 1.0 ppb for the NUDGED and COAREG runs, respectively. Reducing the O3 deposition to oceans and snow/ice increases the bias, but the MAE of 6.0 ppb remains unchanged. The CAMS reanalysis data appear to perform worst for the Terrestrial sites with a bias of 6.4 ppb and an MAE of 8.0 ppb. This might be explained by the lower spatial and temporal resolution of CAMS specifically at these sites having a relatively strong diurnal cycle in ABL dynamics, O3 deposition to vegetation and O3 concentrations. Also a misrepresentation of emissions of precursor emissions and concentrations and the O3 deposition to vegetation (Michou et al., 2005; Val Martin et al., 2014) might explain some of the differences.
3.4 Temporal variability of surface ozone in the High Arctic
In Sect. 3.3 we have shown how revising the O3 deposition scheme to oceans and snow/ice can improve the model's capability to represent the observed hourly surface O3 mixing ratios, especially for the High Arctic sites. In this section we show how the NUDGED and COAREG runs and CAMS represent the temporal variation in High Arctic surface O3 observations, focusing on 6 out of the 25 measurement sites. These six High Arctic sites have been selected due to their deposition footprint being dominated by transport over, and deposition to, ocean and sea-ice-covered surfaces. Figure 5 shows the observed and simulated surface O3 time series for ASCOS, Summit, Villum, Zeppelin, Barrow and Alert. Furthermore, Table 2 shows the model skill indicators for the High Arctic sites. These skill indicators include the mean absolute error (MAE) that represents the systematic error, the standard deviation of observation minus model prediction σo−p that represents the random error, and the Pearson R correlation coefficient (R) that represents the degree of correlation.
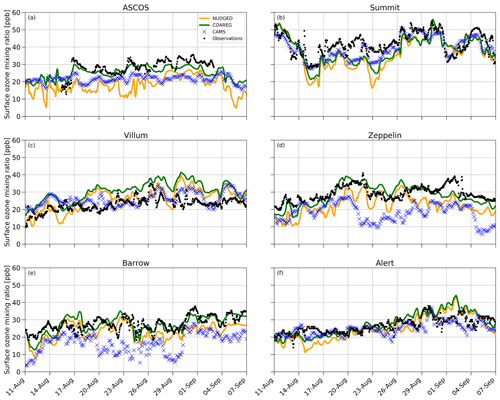
Figure 5Temporal evolution of hourly surface O3 mixing ratios (ppb) for the NUDGED (yellow) and COAREG (green) runs, CAMS data (blue crosses) and observations (black dots) at ASCOS (∼ 87.4∘ N, ∼ 6.0∘ W), Summit (72.6∘ N, 38.5∘ W), Villum (81.6∘ N, 16.7∘ W), Zeppelin (78.9∘ N, 11.9∘ E), Barrow (71.3∘ N, 156.6∘ W) and Alert (82.5∘ N, 62.3∘ W).
Table 2MAE (ppb), σo−p (ppb) and Pearson R correlation coefficient (R) (–) for the NUDGED and COAREG runs and CAMS reanalysis data at the ASCOS, Summit, Villum, Zeppelin, Barrow and Alert observational sites. The lowest model error and highest correlation have been made bold for every site.

The observations at ASCOS (Fig. 5a) show a sudden increase in surface O3 mixing ratios from 20 to over 30 ppb around the 17 August due to advection of relatively O3-rich air during a synoptically active period (Tjernström et al., 2012). Only the COAREG run appears to be able to simulate a similar increase in surface O3, while NUDGED and CAMS show a minor increase in simulated surface O3. From the 17 August onwards, the observations show mixing ratios between 25 and 35 ppb. The WRF simulations indicate advection of air over ocean and ice surfaces during this time period (not shown here). In the COAREG simulation, with less deposition to these surfaces, surface O3 mixing ratios are less depleted. Only the COAREG run is able to represent these observed mixing ratios with a bias of −2.0 ppb, whereas NUDGED and CAMS are clearly biased towards lower mixing ratios.
At Summit (Fig. 5b), we find a large temporal variability in observed surface O3 between 30 and 55 ppb. From the 11 August onwards we find a decreasing trend in observed surface O3 down to 30 ppb before increasing to 40 ppb around the 17 August. All models capture this specific event in terms of temporal variability even though NUDGED and COAREG are still biased at the observed minimum of 30 ppb. Furthermore, we find that the CAMS reanalysis data represent this specific period very well, also in terms of magnitude. At Summit, the increase in surface O3 in the COAREG run relative to the NUDGED run mostly reflects the reduction in deposition to snow and ice due to the prevailing katabatic wind flow (Gorter et al., 2014). During episodes with low wind speeds the ABL becomes very stable and shallow during which deposition to snow and ice becomes an important process in removing O3 in the ABL. In the period between the 14 and 26 August this reduction in deposition can increase the surface O3 mixing ratios of up to 10 ppb (e.g., 23 August). In contrast, during episodes with higher wind speeds and deeper ABLs the reduced O3 deposition to snow hardly affects the simulated surface O3 concentrations. Interestingly, we find that the NUDGED and COAREG simulations show a larger negative bias (∼ 5–10 ppb) during the period with low wind speeds and shallow ABLs. Over the entire simulated period, CAMS performs best at Summit, with an MAE of 3.9 ppb, followed by COAREG, with an MAE of 6.1 ppb.
Villum (Fig. 5c) is the only site for which the NUDGED and COAREG runs as well as the CAMS reanalysis data all systematically overestimate the observed mixing ratios, especially later into the simulation. The observations show an increase in O3 mixing ratios from 10 to 20 ppb in the first 3 d of the simulation, whereafter it remains between 20 and 30 ppb with relatively low temporal variability compared to some of the other sites (e.g., Summit, Barrow). Both the NUDGED and COAREG runs simulate mixing ratios of up to 40 ppb, and CAMS simulates maximum surface O3 mixing ratios of 35 ppb. In terms of representing the magnitude of surface O3 mixing ratios CAMS performs best with an MAE of 4.5.
Zeppelin (Fig. 5d) and Barrow (Fig. 5e) show similar behavior in terms of observation–model comparison. For both locations the CAMS reanalysis data systematically underestimate observed O3 mixing ratios with a biases > 10 ppb. In the NUDGED run the bias equals −6.9 and −4.6 ppb for Zeppelin and Barrow, respectively. In the COAREG run the bias is reduced to −1.0 and −0.2 ppb for Zeppelin and Barrow, respectively. This reduction in bias is, together with ASCOS, the largest among the six High Arctic sites and shows the large sensitivity to the representation of O3 deposition. At Barrow, the dominant wind directions during the simulation period are NW–NE reflecting a footprint mostly from the Arctic sea ice and ocean. Especially in the period from the 23 August onward, the COAREG run is very accurate in representing the magnitude as well as the temporal variability in observed surface O3. During this period, the NUDGED run simulates surface O3 mixing ratios of up to 5 ppb lower due to the overestimated deposition to oceans and sea ice. At both sites, the model performance of COAREG is in the same order of magnitude, with an MAE, σo−p and R of 3.5 ppb, 4.2 ppb and 0.65, respectively.
At Alert (Fig. 5f), we find a relatively steady increase in observed surface O3 from 20 ppb at the start of the simulation to 30 ppb at the end of the simulation. The temporal variability, both in observed and simulated surface O3, appears to be lower compared to some of the other High Arctic sites. Again, the statistical parameters such as MAE, σo−p and R improve in the COAREG run with respect to the NUDGED run. At Alert, we find that CAMS has the lowest MAE and σo−p of 3.0 and 3.4 ppb, respectively.
The model performance in terms of temporal variability in surface O3 observations is diagnosed by using the Pearson R correlation coefficient. The model performance improved for all six sites in the COAREG run with respect to the NUDGED run. The COAREG simulation performs best for five out of the six observational sites in terms of Pearson R correlation coefficient and is only outperformed by CAMS at Summit. Overall, we find that coupling the WRF model to the mechanistic COAREG ocean–atmosphere exchange representation decreases the MAE and σo−p for all High Arctic sites except for Villum by better representing the magnitude of, but also temporal variability in observed surface O3. The CAMS reanalysis data perform well for some locations (e.g., Summit, Alert), while for Zeppelin and Barrow the discrepancy is among the largest we found in the observation–model comparison.
This study demonstrates the impact of a mechanistic representation of ocean–atmosphere O3 exchange to simulate the magnitude and temporal variability of hourly surface O3 concentrations in the Arctic at 25 sites. We show that the modeled sensitivity of the surface O3 concentrations to the representation of O3 to ocean, ice and snow surfaces is high, even though the total deposition budget is an order of magnitude smaller than the deposition budget to land and vegetation. Using a mechanistic oceanic O3 deposition representation and reduced O3 deposition to snow and ice greatly reduced the negative bias in surface O3, especially in the High Arctic. Furthermore, the temporal variability in surface O3 was also better represented by the mechanistic representation of oceanic O3 deposition also accounting for temporal variations in the driving processes of oceanic O3 deposition such as waterside turbulent transport. This analysis also shows a discrepancy in the representation of simulated O3 at sites having a terrestrial footprint (e.g., Norway, Sweden, Finland). However, the model representation of O3 deposition to vegetation and land, including diurnal and seasonal variability (Lin et al., 2019), is beyond the scope of this study. To find out whether the implementation of a mechanistic representation of oceanic O3 deposition specifically affects the variability of surface O3 at certain timescales, we have performed an additional wavelet analysis (Torrence and Compo, 1998). For the six High Arctic sites we found that ∼ 55 %–70 % of the simulated and observed signal is present at timescales > 4 d representing the longer timescales and synoptic variability in wind speeds and vertical and horizontal mixing conditions. Interestingly, we found that the observations show more variability compared to the model simulations at timescales of hours, arguably due to the misrepresentation of some sub-grid processes. We do not find any clear indication that the implementation of COAREG significantly affects the variability of surface O3 at High Arctic sites at a specific timescale.
The COAREG scheme has been developed and validated against eddy-covariance measurements over mostly subtropical waters (Bariteau et al., 2010; Helmig et al., 2012) and has been applied to study the effects of wind speed and sea state on ocean–atmosphere gas transfer (Blomquist et al., 2017; Bell et al., 2017; Porter et al., 2020). We do expect that these main drivers, i.e., waterside turbulent transfer and chemical enhancement with dissolved iodide, also control oceanic O3 deposition at high latitudes. Indirect evaluation of oceanic O3 deposition through a comparison of surface O3 observations instead of direct oceanic O3 flux measurements indicates that including this mechanistic representation of O3 deposition improves both the modeled magnitude and temporal variability in surface O3 observations. However, a lack of oceanic O3 deposition flux measurements hampers the direct model evaluation of the high-latitude O3 deposition flux. This is expected to be soon resolved by getting access to O3 flux observations collected in the Multidisciplinary drifting Observatory for the Study of Arctic Climate (MOSAiC) 1-year field campaign.
Furthermore, we have reduced the deposition to snow and ice following Helmig et al. (2007a) and Clifton et al. (2020a). The results of Helmig et al. (2007a) also motivated follow-up observational and modeling studies aiming at the development of more mechanistic representations of O3 deposition to snow-/ice-covered surfaces. For example, efforts have been made to simulate O3 dynamics in and above the snowpack using a 1D model setup to explain observations of O3 and NOx concentrations measured above and inside the Summit snowpack (Van Dam et al., 2015). This 1D modeling study suggested the role of aqueous-phase oxidation of O3 with formic acid in the snowpack (Murray et al., 2015). Comparable 1D modeling studies focused on assessing the role of catalytic O3 loss via bromine radical chemistry in the snowpack interstitial air (Thomas et al., 2011; Toyota et al., 2014). However, these studies mainly addressed the role of some of this snowpack chemistry in explaining, partly observed, O3 concentrations and not so much on snow–atmosphere O3 fluxes and derived deposition rates that would corroborate the inferred very small O3 deposition rates by Helmig et al. (2007a). Clifton et al. (2020a) summarized that accurate process-based modeling of O3 deposition to snow requires a better understanding of the underlying processes and dependencies. An eddy-covariance system that has been deployed as part of the MOSAiC campaign will further enhance our understanding of O3 deposition in shallow ABLs at high latitudes (Clifton et al., 2020b).
In this study we used the COAREG transfer algorithm version 3.6, which is extended with a two-layer scheme for surface resistance compared to the previous versions (Fairall et al., 2007, 2011) and is similar to Luhar et al. (2018). Our WRF simulations excluded the additional role of chlorophyll, dissolved organic matter (DOM) or other species such as DMS on chemical enhancement of O3 in surface waters. Experimental studies have shown that DMS, chlorophyll or other reactive organics may enhance the removal of O3 at the sea surface (Chang et al., 2004; Clifford et al., 2008; Reeser et al., 2009; Martino et al., 2012). The global modeling study by Ganzeveld et al. (2009) included a chlorophyll–O3 reactivity that increased linearly with chlorophyll concentration as a proxy for the role of DOM in oceanic O3 deposition. Including this reaction substantially enhances O3 deposition to coastal waters such that actually observed O3 deposition to these coastal waters is well reproduced (Ganzeveld et al., 2009). Other studies such as Luhar et al. (2017) and Pound et al. (2020) ignored the potential role of DOM–O3 chemistry in oceanic O3 deposition. Luhar et al. (2018), who did not explicitly consider coastal waters, even suggested that including such a reaction deteriorates the comparison with O3 flux observations above open oceans. To test the sensitivity of our model setup to other reactants in the surface water we have performed an additional sensitivity analysis including the chlorophyll–O3 and DMS–O3 reactions from Ganzeveld et al. (2009). Oceanic chlorophyll concentrations have been retrieved from the 9 × 9 km resolution MODIS chlorophyll-α dataset available at https://modis.gsfc.nasa.gov/data/dataprod/chlor_a.php (last access: 14 August 2020). Chlorophyll-α concentrations are typically < 3 mg m−3 for open oceans and up to 25 mg m−3 for coastal waters. For oceanic DMS concentrations, we use the monthly climatology from Lana et al. (2011). The sensitivity study with chlorophyll as an additional reactant indicated a slight increase (up to 5 %) in deposition to coastal waters with chlorophyll concentrations of up to 25 mg m−3. However, the resulting effect on surface O3 concentrations was not significant due to the large fraction of oceans with very low (< 3 mg m−3) chlorophyll-α concentrations. Also, the reactions with oceanic DMS appear to be weak due to relatively low DMS concentrations in August/September. These sensitivity studies indicate that I is the main driver of chemical reactivity of O3 in the Arctic Ocean in summer. However a potential sensitivity of these reactants on Arctic O3 deposition could be expected especially in the spring to summer transition following algal blooms (Stefels et al., 2007; Riedel et al., 2008).
We nudged the WRF model to the ECMWF ERA5 reanalysis product to ensure a fair model evaluation with observations due to a better representation of the synoptic conditions. This indicated the important role of the model representation of meteorology, e.g., the advection of polluted air and mixing/entrainment of O3 in the ABL, in representing the observed surface O3 concentrations. The model evaluation was set up at a resolution of 30 × 30 km, which is in the order of the ERA5 reanalysis data (0.25∘ × 0.25∘) used for initial conditions, boundary conditions and nudging. Here, we opted for a 30 km grid spacing because we expect that the main drivers of tropospheric O3 (chemical production and destruction, stratosphere–troposphere transport, dry deposition, mixing and advection processes) can be sufficiently resolved at this grid spacing especially over the relatively homogeneous ocean, ice and snow surfaces. However, we do realize that such a coarse grid spacing may have hampered representing local air flow phenomena such as katabatic winds (Klein et al., 2001), which could explain some of the mismatch at sites like Villum (Nguyen et al., 2016). Another justification for the 30 km grid spacing was to limit computational time and to have a large enough domain to cover the entire region above 60∘ N to conduct a large pan-Arctic evaluation while at the same time having all observational sites far enough from the domain boundaries to limit the effect of the imposed meteorological and chemical boundary conditions.
In general, the relatively scarce Arctic observations limit evaluation of modeling studies and extrapolation of these results for the Arctic summer to other seasons and lower latitudes. In this case, this includes the uncertainty in the magnitude and distribution of driving factors of oceanic O3 deposition such as I or DOM. New I measurements at high latitudes, for example those performed during the year-round MOSAiC expedition, will be very useful to better constrain the global I distributions as well as mechanistic oceanic O3 deposition representations. Measurements of O3 concentrations and deposition fluxes to the Arctic Ocean can assist us to better constrain these modeling setups in terms of magnitude and temporal variability and can potentially indicate the sensitivity to other environmental factors such as wind speed in waters with low reactivity. Furthermore, including the role of halogen chemistry (Pratt et al., 2013; Thompson et al., 2017) might give an indication of the combined role of halogens and oceanic deposition in removing O3 and explaining the magnitude and temporal variability of O3 concentrations in the High Arctic.
The mesoscale meteorology–chemistry model Polar-WRF-Chem was coupled to the Coupled Ocean-Atmosphere Response Experiment Gas transfer algorithm (COAREG) to allow for a mechanistic representation of ocean–atmosphere exchange of O3. This scheme represents the effects of molecular diffusion, solubility, waterside turbulent transfer and chemical enhancement of O3 uptake through its reactions with dissolved iodide. The COAREG scheme replaces the constant surface uptake resistance approach often applied in ACTMs. Furthermore, we have increased the modeled O3 surface uptake resistance to snow and ice. In total, two simulations were performed: (1) a default WRF setup nudged to ERA5 synoptic conditions (NUDGED) and (2) a WRF setup with adjustments to O3 surface uptake resistance as described above (COAREG). Furthermore, the CAMS global reanalysis data product has also been included in the presented evaluation of High Arctic surface O3. This CAMS product is widely used in air quality assessments and to constrain regional-scale modeling experiments. This provides additional information on the quality of the CAMS data products but also on potential issues in the representation of O3 sources and sinks, e.g., oceanic and snow/sea ice deposition, for the High Arctic. The modeling approach was set up for 1 month at the end of summer 2008 and evaluated against hourly surface O3 at 25 sites for latitudes > 60∘ N including observations over the Arctic sea ice as part of the ASCOS campaign.
Using the mechanistic representation of ocean–atmosphere exchange, O3 deposition velocities were simulated in the order of 0.01 cm s−1 compared to ∼ 0.05 cm s−1 in the constant surface uptake resistance approach. In the COAREG run, the spatial variability (0.01 to 0.018 cm s−1) in the mean O3 deposition velocities expressed the sensitivity to chemical enhancement with dissolved iodide. The temporal variability of O3 deposition velocities (up to ±20 % around the mean) is governed by surface wind speeds and expressed differences in waterside turbulent transport. Using the mechanistic representation of ocean–atmosphere exchange reduced the total simulated O3 deposition budget to water bodies by a factor of 3.3 compared to the default constant ocean uptake rate approach and the increase in surface uptake resistance to snow and ice reduced the deposition budget by a factor of 2.4.
Despite the fact that O3 deposition to oceans, snow and ice surfaces only constitutes a small term in the total O3 deposition budget (> 90 % of the deposition is to land), we find a substantial sensitivity to the simulated surface O3 mixing ratios. In the COAREG run, the simulated mean monthly surface O3 mixing ratios have increased by up to 50 % in the typically shallow Arctic ABL above the oceans and sea ice relative to the NUDGED run. The mechanistic representation of O3 deposition to oceans resulted in a substantially improved representation of surface O3 observations, especially for the High Arctic sites with latitudes > 70∘ N. The NUDGED run underestimated the observed surface O3 mixing ratios with a bias of −3.8 ppb, whereas the COAREG run had a bias of 0.3 ppb. The evaluation of the WRF runs at individual High Arctic sites showed that using the mechanistic representation of O3 deposition to oceans results in a better representation of surface O3 observations both in terms of magnitude and temporal variability. Similar to the NUDGED run, CAMS underestimated High Arctic observed surface O3 with a bias of −5.0 ppb indicating that the representation of the deposition removal mechanism to oceans and snow/ice in CAMS might also be overestimated and should be reconsidered.
This study highlights the impact of a mechanistic representation of oceanic O3 deposition on Arctic surface O3 concentrations at a high (hourly) temporal resolution. It mostly corroborates the findings of global-scale studies (e.g., Ganzeveld et al., 2009; Luhar et al., 2017; Pound et al., 2020) and recommends that the representation of O3 deposition to oceans and snow/ice in global- and regional-scale ACTMs should be revised. This revision is needed not only to better quantify the O3 budget at the global scale, but also to better represent the observed magnitude and temporal variability of surface O3 at the regional scale. In addition, explicit consideration of the mechanisms involved in O3 removal by the oceans (and sea ice/snowpack) are essential to also evaluate the role of potentially important feedback mechanisms and future trends in and the role of O3 in Arctic climate change as a function of declining sea ice cover, increasing emissions and changes in oceanic biogeochemical conditions. On the regional scale, this study also has implications for methods to quantify future trends in Arctic tropospheric O3, Arctic air pollution and climate in a period of declining sea ice and increasing local emissions of precursors.
The exchange velocity, in this case deposition, of ozone () (m s−1) is calculated from the waterside resistance (rw) (s m−1) and air side resistance terms (ra+rb) (s m−1) as follows:
Here, α (–) is the dimensionless solubility of O3 in sea water calculated from SST (K) following Morris (1988) as
and the waterside resistance term (rw) is calculated as
Here, a (s−1) is the chemical reactivity of O3 with I calculated with the second-order rate coefficient (M−1 s−1) from Magi et al. (1997) and the I concentrations (M) from Sherwen et al. (2019):
In Eq. (B3), D (m2 s−1) is the molecular diffusivity of O3 in ocean water and is calculated from the kinematic viscosity ν (m2 s−1) and the waterside Schmidt number (Scw) (–) as
where μ (kg m−1 s−1) is the dynamic viscosity of seawater and ρ (kg m−3) is the density of seawater.
Finally, the air side resistance terms (ra+rb) (s m−1) of the deposition velocity in Eq. (B1) are calculated as
where Cd (–) is the momentum drag coefficient, Sca (–) is the Schmidt number for ozone in the atmosphere, κ is the von Kármán constant (0.4) and (m s−1) is the friction velocity in the atmosphere. The ra+rb term is typically in the order of 100 s m−1 (Fairall et al., 2011).
Compared to COAREG version 3.1 (Fairall et al., 2007, 2011), COAREGv3.6 is extended with a two-layer scheme based on Luhar et al. (2018). This extension is included in the second term of the waterside resistance term (Eq. B3). Here, , and with . This part of the equation is a function of the chemical reactivity a (s−1) (Eq. B4), the waterside friction velocity (m s−1), the molecular diffusivity of O3 in ocean water (Eq. B5) and δm (m) representing the depth of the interface between the top water layer and the underlying turbulent layer. In this study we have applied with c0=0.4 based on Luhar et al. (2018). K0(ξδ) and K1(ξδ) are the modified Bessel functions of the second kind of order 0 and 1, respectively. For more information on the derivation of the formulas, please visit Fairall et al. (2007, 2011) and Luhar et al. (2018).
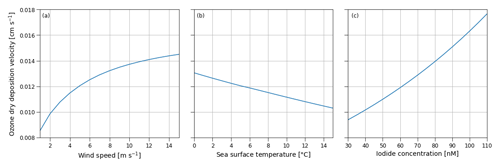
Figure B1Sensitivity of the ozone dry-deposition velocity from COAREG to the environmental factors 10 m wind speed (m s−1) (a), sea surface temperature (∘C) (b) and sea surface iodide concentration (nM) (c) using typical values of 10 m wind speed, sea surface temperature and iodide concentration of 5 m s−1, 5 ∘C and 60 nM, respectively. Note that the sensitivity to sea surface temperature does not include effects of increasing reactivity but mostly represents the effect of reduced solubility (Eq. B2).
Figure B1 shows the sensitivity of the COAREG routine coupled to WRF to the environmental factors wind speed, SST and iodide concentration. The sensitivity to wind speeds (Fig. B1a) expresses the role of waterside turbulent transport and aerodynamic resistance. For low wind speeds waterside turbulent transport is limited and therefore limits the exchange of O3 from the atmosphere to the ocean. At high wind speeds, the dry deposition of O3 is limited by chemical reactivity of O3 with I at typical Arctic SSTs of 5 ∘C and I concentrations of 60 nM (see also Fig. C1). At very low wind speeds (< 2.5 m s−1) the aerodynamic resistance poses an extra restriction on the ocean–atmosphere exchange of O3. The sensitivity to SST (Fig. B1b) mostly represents the role of solubility (Eq. B2) with warmer waters having a lower solubility. In contrast to Luhar et al. (2018), the SST is not used to calculate the I concentrations and does therefore not show a positive correlation. The sensitivity to I (Fig. B1c) represents the role of chemical enhancement which is stronger than the generally compensating effect of solubility in warmer waters for typical Arctic conditions.
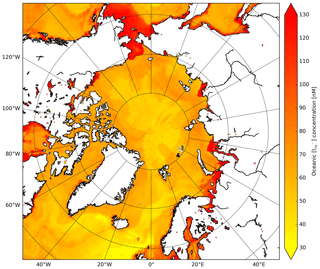
Figure C1Spatial distribution of Sherwen et al. (2019) oceanic iodide concentrations (nM) at the start of the simulation.
The COAREG algorithm is available at ftp://ftp1.esrl.noaa.gov/BLO/Air-Sea/bulkalg/cor3_6/gasflux36/ (Fairall et al., 2007). The coupled Polar-WRF-Chem model, model output and post-processing scripts are available upon request.
Third party data products used in this paper can be best accessed through their corresponding publications (CAMS, Inness et al., 2019; ERA5, Hersbach et al., 2020; Oceanic Iodide, Sherwen et al., 2019; Oceanic DMS, Lana et al., 2011) but are also available upon request. MODIS chlorophyll-α data are available through https://doi.org/10.5067/AQUA/MODIS/L3M/CHL/2014 (NASA Goddard Space Flight Center et al., 2014). AMSR-E wind speed data are available through https://doi.org/10.5067/AMSR-E/AE_DYOCN.002 (Wentz and Meissner, 2004).
JGMB, LNG and GJS designed the experiment. JGMB performed the Polar-WRF-Chem simulations and performed the analysis. JGMB, LNG, GJS and MCK wrote the paper.
The authors declare that they have no conflict of interest.
Publisher's note: Copernicus Publications remains neutral with regard to jurisdictional claims in published maps and institutional affiliations.
The authors acknowledge the Polar-WRF-Chem developers and support as well as the COAREG developers and in particular Chris Fairall. Furthermore, the authors thank the three anonymous reviewers for their extensive reviews as well as Owen Cooper and Ashok Luhar for providing short comments on the paper.
This research has been supported by the Nederlandse Organisatie voor Wetenschappelijk Onderzoek (grant no. 866.18.004).
This paper was edited by Leiming Zhang and reviewed by three anonymous referees.
Ainsworth, E. A., Yendrek, C. R., Sitch, S., Collins, W. J., and Emberson, L. D.: The effects of tropospheric ozone on net primary productivity and implications for climate change, Annu. Rev. Plant Biol., 63, 637–661, 2012. a
Arnold, S. R., Law, K. S., Brock, C. A., Thomas, J. L., Starkweather, S. M., von Salzen, K., Stohl, A., Sharma, S., Lund, M. T., Flanner, M. G., Petäjä, T., Tanimoto, H., Gamble, J., Dibb, J. E., Melamed, M., Johnson, N., Fidel, M., Tynkkynen, V.-P., Baklanov, A., Eckhardt, S., Monks, S. A., Browse, J., and Bozem, H.: Arctic air pollution: Challenges and opportunities for the next decade, Elementa: Science of the Anthropocene, 4, 000104, https://doi.org/10.12952/journal.elementa.000104, 2016. a
Bariteau, L., Helmig, D., Fairall, C. W., Hare, J. E., Hueber, J., and Lang, E. K.: Determination of oceanic ozone deposition by ship-borne eddy covariance flux measurements, Atmos. Meas. Tech., 3, 441–455, https://doi.org/10.5194/amt-3-441-2010, 2010. a
Bell, T. G., Landwehr, S., Miller, S. D., de Bruyn, W. J., Callaghan, A. H., Scanlon, B., Ward, B., Yang, M., and Saltzman, E. S.: Estimation of bubble-mediated air–sea gas exchange from concurrent DMS and CO2 transfer velocities at intermediate–high wind speeds, Atmos. Chem. Phys., 17, 9019–9033, https://doi.org/10.5194/acp-17-9019-2017, 2017. a, b
Blomquist, B., Brumer, S., Fairall, C., Huebert, B., Zappa, C., Brooks, I., Yang, M., Bariteau, L., Prytherch, J., Hare, J., Czerski, H., and Pascal, R. W.: Wind speed and sea state dependencies of air-sea gas transfer: Results from the high wind speed gas exchange study (HiWinGS), J. Geophys. Res.-Oceans, 122, 8034–8062, 2017. a, b
Bromwich, D. H., Otieno, F. O., Hines, K. M., Manning, K. W., and Shilo, E.: Comprehensive evaluation of polar weather research and forecasting model performance in the Antarctic, J. Geophys. Res.-Atmos., 118, 274–292, 2013. a
Chance, R., Baker, A. R., Carpenter, L., and Jickells, T. D.: The distribution of iodide at the sea surface, Environ. Sci.-Proc. Imp., 16, 1841–1859, 2014. a, b
Chang, W., Heikes, B. G., and Lee, M.: Ozone deposition to the sea surface: chemical enhancement and wind speed dependence, Atmos. Environ., 38, 1053–1059, 2004. a, b, c, d, e, f
Chen, F. and Dudhia, J.: Coupling an advanced land surface–hydrology model with the Penn State–NCAR MM5 modeling system. Part I: Model implementation and sensitivity, Mon. Weather Rev., 129, 569–585, 2001. a
Clifford, D., Donaldson, D., Brigante, M., D'Anna, B., and George, C.: Reactive uptake of ozone by chlorophyll at aqueous surfaces, Environ. Sci. Technol., 42, 1138–1143, 2008. a, b
Clifton, O. E., Fiore, A. M., Massman, W. J., Baublitz, C. B., Coyle, M., Emberson, L., Fares, S., Farmer, D. K., Gentine, P., Gerosa, G., Guenther, A. B.., Helmig, D., Lombardozzi, D. L.., Munger, J. W., Patton, E. G., Pusede, S. E., Schwede, D. B., Silva, S. J., Sörgel, M., Steiner, A. L., and Tai, A. P. K.: Dry deposition of ozone over land: processes, measurement, and modeling, Rev. Geophys., 58, e2019RG000670, https://doi.org/10.1029/2019RG000670, 2020a. a, b, c, d, e
Clifton, O. E., Paulot, F., Fiore, A., Horowitz, L., Correa, G., Baublitz, C., Fares, S., Goded, I., Goldstein, A., Gruening, C., Hogg, A. J., Loubet, B., Mammarella, I., Munger, J. W., Neil, L., Stella, P., Uddling, J., Vesala, T., and Weng, E.: Influence of dynamic ozone dry deposition on ozone pollution, J. Geophys. Res.-Atmos., 125, e2020JD032398, https://doi.org/10.1029/2020JD032398, 2020b. a, b
Cooper, O. R., Parrish, D., Ziemke, J., Cupeiro, M., Galbally, I., Gilge, S., Horowitz, L., Jensen, N., Lamarque, J.-F., Naik, V., Oltmans, S. J., Schwab, J., Shindell, D. T., Thompson, A. M., Wang, Y., and Zbinden, R. M.: Global distribution and trends of tropospheric ozone: An observation-based review, Elementa: Science of the Anthropocene, 2, 000029, https://doi.org/10.12952/journal.elementa.000029, 2014. a, b
Cooper, O. R., Schultz, M. G., Schröder, S., Chang, K.-L., Gaudel, A., Benítez, G. C., Cuevas, E., Fröhlich, M., Galbally, I. E., Molloy, S., Molloy, S., Kubistin, D., Lu, X., McClure-Begley, A., Nédélec, P., O'Brien, J., Oltmans, S. J., Petropavlovskikh, I., Ries, L., Senik, I., Sjöberg, K., Solberg, S., Spain, G. T., Spangl, W., Steinbacher, M., Tarasick, D., Thouret, V., and Xu, X.: Multi-decadal surface ozone trends at globally distributed remote locations, Elementa: Science of the Anthropocene, 8, 23, https://doi.org/10.1525/elementa.420, 2020. a, b
Fairall, C., Yang, M., Bariteau, L., Edson, J., Helmig, D., McGillis, W., Pezoa, S., Hare, J., Huebert, B., and Blomquist, B.: Implementation of the Coupled Ocean-Atmosphere Response Experiment flux algorithm with CO2, dimethyl sulfide, and O3, J. Geophys. Res.-Oceans, 116, C00F09, https://doi.org/10.1029/2010JC006884, 2011. a, b, c, d, e, f, g
Fairall, C. W., Bradley, E. F., Rogers, D. P., Edson, J. B., and Young, G. S.: Bulk parameterization of air-sea fluxes for tropical ocean-global atmosphere coupled-ocean atmosphere response experiment, J. Geophys. Res.-Oceans, 101, 3747–3764, 1996. a
Fairall, C. W., Helmig, D., Ganzeveld, L., and Hare, J.: Water-side turbulence enhancement of ozone deposition to the ocean, Atmos. Chem. Phys., 7, 443–451, https://doi.org/10.5194/acp-7-443-2007, 2007 (code available at: ftp://ftp1.esrl.noaa.gov/BLO/Air-Sea/bulkalg/cor3_6/gasflux36/, last access: 10 September 2020). a, b, c, d, e, f, g, h, i
Gallagher, M., Beswick, K., and Coe, H.: Ozone deposition to coastal waters, Q. J. Roy. Meteor. Soc., 127, 539–558, 2001. a
Ganzeveld, L., Helmig, D., Fairall, C., Hare, J., and Pozzer, A.: Atmosphere-ocean ozone exchange: A global modeling study of biogeochemical, atmospheric, and waterside turbulence dependencies, Global Biogeochem. Cy., 23, GB4021, https://doi.org/10.1029/2008GB003301, 2009. a, b, c, d, e, f, g, h, i, j, k, l, m, n
Gaudel, A., Cooper, O. R., Chang, K.-L., Bourgeois, I., Ziemke, J. R., Strode, S. A., Oman, L. D., Sellitto, P., Nédélec, P., Blot, R., Thouret, V., and Granier, C.: Aircraft observations since the 1990s reveal increases of tropospheric ozone at multiple locations across the Northern Hemisphere, Sci. Adv., 6, eaba8272, https://doi.org/10.1126/sciadv.aba8272, 2020. a
Gery, M. W., Whitten, G. Z., Killus, J. P., and Dodge, M. C.: A photochemical kinetics mechanism for urban and regional scale computer modeling, J. Geophys. Res.-Atmos., 94, 12925–12956, 1989. a
Gorter, W., Van Angelen, J., Lenaerts, J., and Van den Broeke, M.: Present and future near-surface wind climate of Greenland from high resolution regional climate modelling, Clim. Dynam., 42, 1595–1611, 2014. a
Grell, G. A., Peckham, S. E., Schmitz, R., McKeen, S. A., Frost, G., Skamarock, W. C., and Eder, B.: Fully coupled “online” chemistry within the WRF model, Atmos. Environ., 39, 6957–6975, 2005. a, b
Guenther, A. B., Jiang, X., Heald, C. L., Sakulyanontvittaya, T., Duhl, T., Emmons, L. K., and Wang, X.: The Model of Emissions of Gases and Aerosols from Nature version 2.1 (MEGAN2.1): an extended and updated framework for modeling biogenic emissions, Geosci. Model Dev., 5, 1471–1492, https://doi.org/10.5194/gmd-5-1471-2012, 2012. a
Hardacre, C., Wild, O., and Emberson, L.: An evaluation of ozone dry deposition in global scale chemistry climate models, Atmos. Chem. Phys., 15, 6419–6436, https://doi.org/10.5194/acp-15-6419-2015, 2015. a
Helmig, D., Ganzeveld, L., Butler, T., and Oltmans, S. J.: The role of ozone atmosphere-snow gas exchange on polar, boundary-layer tropospheric ozone – a review and sensitivity analysis, Atmos. Chem. Phys., 7, 15–30, https://doi.org/10.5194/acp-7-15-2007, 2007a. a, b, c, d, e
Helmig, D., Oltmans, S. J., Carlson, D., Lamarque, J.-F., Jones, A., Labuschagne, C., Anlauf, K., and Hayden, K.: A review of surface ozone in the polar regions, Atmos. Environ., 41, 5138–5161, 2007b. a, b
Helmig, D., Cohen, L. D., Bocquet, F., Oltmans, S., Grachev, A., and Neff, W.: Spring and summertime diurnal surface ozone fluxes over the polar snow at Summit, Greenland, Geophys. Res. Lett., 36, L08809, https://doi.org/10.1029/2008GL036549, 2009. a
Helmig, D., Lang, E., Bariteau, L., Boylan, P., Fairall, C., Ganzeveld, L., Hare, J., Hueber, J., and Pallandt, M.: Atmosphere-ocean ozone fluxes during the TexAQS 2006, STRATUS 2006, GOMECC 2007, GasEx 2008, and AMMA 2008 cruises, J. Geophys. Res.-Atmos., 117, D04305, https://doi.org/10.1029/2011JD015955, 2012. a, b, c, d, e
Hersbach, H., Bell, B., Berrisford, P., Hirahara, S., Horányi, A., Muñoz-Sabater, J., Nicolas, J., Peubey, C., Radu, R., Schepers, D., Simmons, A., Soci, C., Abdalla, S., Abellan, X., Balsamo, G., Bechtold, P., Biavati, G., Bidlot, J., Bonavita, M., De Chiara, G., Dahlgren, P., Dee, D., Diamantakis, M., Dragani, R., Flemming, J., Forbes, R., Fuentes, M., Geer, A., Haimberger, L., Healy, S., Hogan, R. J., Hólm, E., Janisková, M., Keeley, S., Laloyaux, P., Lopez, P., Lupu, C., Radnoti, G., de Rosnay, P., Rozum, I., Vamborg, F., Villaume, S., Thépaut, J.-N.: The ERA5 global reanalysis, Q. J. Roy. Meteor. Soc., 146, 1999–2049, 2020. a, b, c
Hines, K. M. and Bromwich, D. H.: Development and testing of Polar Weather Research and Forecasting (WRF) model. Part I: Greenland ice sheet meteorology, Mon. Weather Rev., 136, 1971–1989, 2008. a
Hong, S.-Y., Dudhia, J., and Chen, S.-H.: A revised approach to ice microphysical processes for the bulk parameterization of clouds and precipitation, Mon. Weather Rev., 132, 103–120, 2004. a
Iacono, M. J., Delamere, J. S., Mlawer, E. J., Shephard, M. W., Clough, S. A., and Collins, W. D.: Radiative forcing by long-lived greenhouse gases: Calculations with the AER radiative transfer models, J. Geophys. Res.-Atmos., 113, D13103, https://doi.org/10.1029/2008JD009944, 2008. a, b
Inness, A., Ades, M., Agustí-Panareda, A., Barré, J., Benedictow, A., Blechschmidt, A.-M., Dominguez, J. J., Engelen, R., Eskes, H., Flemming, J., Huijnen, V., Jones, L., Kipling, Z., Massart, S., Parrington, M., Peuch, V.-H., Razinger, M., Remy, S., Schulz, M., and Suttie, M.: The CAMS reanalysis of atmospheric composition, Atmos. Chem. Phys., 19, 3515–3556, https://doi.org/10.5194/acp-19-3515-2019, 2019. a, b, c, d, e
Janjić, Z. I.: The step-mountain eta coordinate model: Further developments of the convection, viscous sublayer, and turbulence closure schemes, Mon. Weather Rev., 122, 927–945, 1994. a
Janjić, Z. I.: Nonsingular implementation of the Mellor–Yamada level 2.5 scheme in the NCEP Meso model, Office Note no. 437, National Center for Environmental Prediction, available at: https://www.researchgate.net/publication/228749162_Nonsingular_Implementation_of_the_Mellor-Yamada_Level_25_Scheme_in_the_NCEP_Meso_Model (last access: 7 July 2021), 2001. a
Janssens-Maenhout, G., Crippa, M., Guizzardi, D., Muntean, M., Schaaf, E., Dentener, F., Bergamaschi, P., Pagliari, V., Olivier, J. G. J., Peters, J. A. H. W., van Aardenne, J. A., Monni, S., Doering, U., Petrescu, A. M. R., Solazzo, E., and Oreggioni, G. D.: EDGAR v4.3.2 Global Atlas of the three major greenhouse gas emissions for the period 1970–2012, Earth Syst. Sci. Data, 11, 959–1002, https://doi.org/10.5194/essd-11-959-2019, 2019. a
Kain, J. S.: The Kain–Fritsch convective parameterization: an update, J. Appl. Meteorol., 43, 170–181, 2004. a
Klein, T., Heinemann, G., Bromwich, D. H., Cassano, J. J., and Hines, K. M.: Mesoscale modeling of katabatic winds over Greenland and comparisons with AWS and aircraft data, Meteorol. Atmos. Phys., 78, 115–132, 2001. a
Lana, A., Bell, T., Simó, R., Vallina, S., Ballabrera-Poy, J., Kettle, A., Dachs, J., Bopp, L., Saltzman, E., Stefels, J., Johnson, J. E., and Liss, P. S.: An updated climatology of surface dimethlysulfide concentrations and emission fluxes in the global ocean, Global Biogeochem. Cy., 25, GB1004, https://doi.org/10.1029/2010GB003850, 2011. a, b
Law, K. S., Roiger, A., Thomas, J. L., Marelle, L., Raut, J.-C., Dalsøren, S., Fuglestvedt, J., Tuccella, P., Weinzierl, B., and Schlager, H.: Local Arctic air pollution: Sources and impacts, Ambio, 46, 453–463, 2017. a
Lelieveld, J. and Dentener, F. J.: What controls tropospheric ozone?, J. Geophys. Res.-Atmos., 105, 3531–3551, 2000. a
Lin, M., Horowitz, L. W., Payton, R., Fiore, A. M., and Tonnesen, G.: US surface ozone trends and extremes from 1980 to 2014: quantifying the roles of rising Asian emissions, domestic controls, wildfires, and climate, Atmos. Chem. Phys., 17, 2943–2970, https://doi.org/10.5194/acp-17-2943-2017, 2017. a
Lin, M., Malyshev, S., Shevliakova, E., Paulot, F., Horowitz, L. W., Fares, S., Mikkelsen, T. N., and Zhang, L.: Sensitivity of ozone dry deposition to ecosystem-atmosphere interactions: A critical appraisal of observations and simulations, Global Biogeochem. Cy., 33, 1264–1288, 2019. a
Luhar, A. K., Galbally, I. E., Woodhouse, M. T., and Thatcher, M.: An improved parameterisation of ozone dry deposition to the ocean and its impact in a global climate–chemistry model, Atmos. Chem. Phys., 17, 3749–3767, https://doi.org/10.5194/acp-17-3749-2017, 2017. a, b, c, d, e, f, g, h, i, j
Luhar, A. K., Woodhouse, M. T., and Galbally, I. E.: A revised global ozone dry deposition estimate based on a new two-layer parameterisation for air–sea exchange and the multi-year MACC composition reanalysis, Atmos. Chem. Phys., 18, 4329–4348, https://doi.org/10.5194/acp-18-4329-2018, 2018. a, b, c, d, e, f, g, h, i, j
MacDonald, S. M., Gómez Martín, J. C., Chance, R., Warriner, S., Saiz-Lopez, A., Carpenter, L. J., and Plane, J. M. C.: A laboratory characterisation of inorganic iodine emissions from the sea surface: dependence on oceanic variables and parameterisation for global modelling, Atmos. Chem. Phys., 14, 5841–5852, https://doi.org/10.5194/acp-14-5841-2014, 2014. a, b, c
Magi, L., Schweitzer, F., Pallares, C., Cherif, S., Mirabel, P., and George, C.: Investigation of the uptake rate of ozone and methyl hydroperoxide by water surfaces, J. Phys. Chem. A, 101, 4943–4949, 1997. a
Marelle, L., Thomas, J. L., Raut, J.-C., Law, K. S., Jalkanen, J.-P., Johansson, L., Roiger, A., Schlager, H., Kim, J., Reiter, A., and Weinzierl, B.: Air quality and radiative impacts of Arctic shipping emissions in the summertime in northern Norway: from the local to the regional scale, Atmos. Chem. Phys., 16, 2359–2379, https://doi.org/10.5194/acp-16-2359-2016, 2016. a
Martino, M., Lézé, B., Baker, A. R., and Liss, P. S.: Chemical controls on ozone deposition to water, Geophys. Res. Lett., 39, L05809, https://doi.org/10.1029/2011GL050282, 2012. a
Michou, M., Laville, P., Serça, D., Fotiadi, A., Bouchou, P., and Peuch, V.-H.: Measured and modeled dry deposition velocities over the ESCOMPTE area, Atmos. Res., 74, 89–116, 2005. a
Morris, J.: The aqueous solubility of ozone – A review, Ozone news, 1, 14–16, 1988. a
Muller, J. B., Dorsey, J. R., Flynn, M., Gallagher, M. W., Percival, C. J., Shallcross, D. E., Archibald, A., Roscoe, H. K., Obbard, R. W., Atkinson, H. M., Lee, J. D., Moller, S. J., and Carpenter, L. J.: Energy and ozone fluxes over sea ice, Atmos. Environ., 47, 218–225, 2012. a
Murray, K. A., Kramer, L. J., Doskey, P. V., Ganzeveld, L., Seok, B., Van Dam, B., and Helmig, D.: Dynamics of ozone and nitrogen oxides at Summit, Greenland. II. Simulating snowpack chemistry during a spring high ozone event with a 1-D process-scale model, Atmos. Environ., 117, 110–123, 2015. a
NASA Goddard Space Flight Center, Ocean Ecology Laboratory, Ocean Biology Processing Group: Moderate-resolution Imaging Spectroradiometer (MODIS) Aqua Chlorophyll Data, 2014 Reprocessing, NASA OB.DAAC, Greenbelt, MD, USA [data set], https://doi.org/10.5067/AQUA/MODIS/L3M/CHL/2014, 2014. a
Nguyen, Q. T., Glasius, M., Sørensen, L. L., Jensen, B., Skov, H., Birmili, W., Wiedensohler, A., Kristensson, A., Nøjgaard, J. K., and Massling, A.: Seasonal variation of atmospheric particle number concentrations, new particle formation and atmospheric oxidation capacity at the high Arctic site Villum Research Station, Station Nord, Atmos. Chem. Phys., 16, 11319–11336, https://doi.org/10.5194/acp-16-11319-2016, 2016. a
Nuvolone, D., Petri, D., and Voller, F.: The effects of ozone on human health, Environ. Sci. Pollut. R., 25, 8074–8088, 2018. a
Oh, I.-B., Byun, D. W., Kim, H.-C., Kim, S., and Cameron, B.: Modeling the effect of iodide distribution on ozone deposition to seawater surface, Atmos. Environ., 42, 4453–4466, 2008. a, b, c
Oltmans, S., Lefohn, A., Shadwick, D., Harris, J., Scheel, H., Galbally, I., Tarasick, D., Johnson, B., Brunke, E.-G., Claude, H., Zeng, G., Nichol, S., Schmidlin, F., Davies, J., Cuevas, E., Redondas, A., Naoe, H., Nakano, T., and Kawasato, T.: Recent tropospheric ozone changes – A pattern dominated by slow or no growth, Atmos. Environ., 67, 331–351, 2013. a
Paatero, J., Vaattovaara, P., Vestenius, M., Meinander, O., Makkonen, U., Kivi, R., Hyvärinen, A., Asmi, E., Tjernström, M., and Leck, C.: Finnish contribution to the arctic summer cloud ocean study (ASCOS) expedition, Arctic Ocean 2008, Geophysica, 45, 119–146, 2009. a
Padro, J.: Summary of ozone dry deposition velocity measurements and model estimates over vineyard, cotton, grass and deciduous forest in summer, Atmos. Environ., 30, 2363–2369, 1996. a
Pausata, F. S. R., Pozzoli, L., Vignati, E., and Dentener, F. J.: North Atlantic Oscillation and tropospheric ozone variability in Europe: model analysis and measurements intercomparison, Atmos. Chem. Phys., 12, 6357–6376, https://doi.org/10.5194/acp-12-6357-2012, 2012. a
Pedregosa, F., Varoquaux, G., Gramfort, A., Michel, V., Thirion, B., Grisel, O., Blondel, M., Prettenhofer, P., Weiss, R., Dubourg, V., Vanderplas, J., Passos, A., Cournapeau, D., Brucher, M., Perrot, M., and Duchesnay, É.: Scikit-learn: Machine learning in Python, J. Mach. Learn. Res., 12, 2825–2830, 2011. a
Porter, J., de Bruyn, W., Miller, S., and Saltzman, E.: Air/sea transfer of highly soluble gases over coastal waters, Geophys. Res. Lett., 47, L085286, https://doi.org/10.1029/2019GL085286, 2020. a, b
Pound, R. J., Sherwen, T., Helmig, D., Carpenter, L. J., and Evans, M. J.: Influences of oceanic ozone deposition on tropospheric photochemistry, Atmos. Chem. Phys., 20, 4227–4239, https://doi.org/10.5194/acp-20-4227-2020, 2020. a, b, c, d, e, f, g
Prados-Roman, C., Cuevas, C. A., Fernandez, R. P., Kinnison, D. E., Lamarque, J.-F., and Saiz-Lopez, A.: A negative feedback between anthropogenic ozone pollution and enhanced ocean emissions of iodine, Atmos. Chem. Phys., 15, 2215–2224, https://doi.org/10.5194/acp-15-2215-2015, 2015. a
Pratt, K. A., Custard, K. D., Shepson, P. B., Douglas, T. A., Pöhler, D., General, S., Zielcke, J., Simpson, W. R., Platt, U., Tanner, D. J., Huey, L. G., Carlsen, M., and Stirm, B. H.: Photochemical production of molecular bromine in Arctic surface snowpacks, Nat. Geosci., 6, 351–356, 2013. a, b
Reeser, D. I., Jammoul, A., Clifford, D., Brigante, M., D'Anna, B., George, C., and Donaldson, D.: Photoenhanced reaction of ozone with chlorophyll at the seawater surface, J. Phys. Chem. C, 113, 2071–2077, 2009. a
Riedel, A., Michel, C., Gosselin, M., and LeBlanc, B.: Winter–spring dynamics in sea-ice carbon cycling in the coastal Arctic Ocean, J. Marine Syst., 74, 918–932, 2008. a
Sherwen, T., Chance, R. J., Tinel, L., Ellis, D., Evans, M. J., and Carpenter, L. J.: A machine-learning-based global sea-surface iodide distribution, Earth Syst. Sci. Data, 11, 1239–1262, https://doi.org/10.5194/essd-11-1239-2019, 2019. a, b, c, d, e, f, g
Stefels, J., Steinke, M., Turner, S., Malin, G., and Belviso, S.: Environmental constraints on the production and removal of the climatically active gas dimethylsulphide (DMS) and implications for ecosystem modelling, Biogeochemistry, 83, 245–275, 2007. a
Tarasick, D., Galbally, I. E., Cooper, O. R., Schultz, M. G., Ancellet, G., Leblanc, T., Wallington, T. J., Ziemke, J., Liu, X., Steinbacher, M., Staehelin, J., Vigouroux, C., Hannigan, J. W., García, O., Foret, G., Zanis, P., Weatherhead, E., Petropavlovskikh, I., Worden, H., Osman, M., Liu, J., Chang, K.-L., Gaudel, A., Lin, M., Granados-Muñoz, M., Thompson, A. M., Oltmans, S. J., Cuesta, J., Dufour, G., Thouret, V., Hassler, B ., Trickl, T., and Neu, J. L.: Tropospheric Ozone Assessment Report: Tropospheric ozone from 1877 to 2016, observed levels, trends and uncertainties, Elementa: Science of the Anthropocene, 7, 39, https://doi.org/10.1525/elementa.376, 2019. a
Thomas, J. L., Stutz, J., Lefer, B., Huey, L. G., Toyota, K., Dibb, J. E., and von Glasow, R.: Modeling chemistry in and above snow at Summit, Greenland – Part 1: Model description and results, Atmos. Chem. Phys., 11, 4899–4914, https://doi.org/10.5194/acp-11-4899-2011, 2011. a
Thompson, C. R., Shepson, P. B., Liao, J., Huey, L. G., Cantrell, C., Flocke, F., and Orlando, J.: Bromine atom production and chain propagation during springtime Arctic ozone depletion events in Barrow, Alaska, Atmos. Chem. Phys., 17, 3401–3421, https://doi.org/10.5194/acp-17-3401-2017, 2017. a, b
Tjernström, M., Birch, C. E., Brooks, I. M., Shupe, M. D., Persson, P. O. G., Sedlar, J., Mauritsen, T., Leck, C., Paatero, J., Szczodrak, M., and Wheeler, C. R.: Meteorological conditions in the central Arctic summer during the Arctic Summer Cloud Ocean Study (ASCOS), Atmos. Chem. Phys., 12, 6863–6889, https://doi.org/10.5194/acp-12-6863-2012, 2012. a, b
Torrence, C. and Compo, G. P.: A practical guide to wavelet analysis, B. Am. Meteorol. Soc., 79, 61–78, 1998. a
Toyota, K., Dastoor, A. P., and Ryzhkov, A.: Air–snowpack exchange of bromine, ozone and mercury in the springtime Arctic simulated by the 1-D model PHANTAS – Part 2: Mercury and its speciation, Atmos. Chem. Phys., 14, 4135–4167, https://doi.org/10.5194/acp-14-4135-2014, 2014. a
Toyota, K., Dastoor, A. P., and Ryzhkov, A.: Parameterization of gaseous dry deposition in atmospheric chemistry models: Sensitivity to aerodynamic resistance formulations under statically stable conditions, Atmos. Environ., 147, 409–422, 2016. a
Val Martin, M., Heald, C., and Arnold, S.: Coupling dry deposition to vegetation phenology in the Community Earth System Model: Implications for the simulation of surface O3, Geophys. Res. Lett., 41, 2988–2996, 2014. a
Van Dam, B., Helmig, D., Toro, C., Doskey, P., Kramer, L., Murray, K., Ganzeveld, L., and Seok, B.: Dynamics of ozone and nitrogen oxides at Summit, Greenland: I. Multi-year observations in the snowpack, Atmos. Environ., 123, 268–284, 2015. a
Wentz, F. J. and Meissner, T.: AMSR-E/Aqua Daily L3 Global Ascending/Descending .25x.25 deg Ocean Grids, Version 2, Boulder, Colorado USA, NASA National Snow and Ice Data Center Distributed Active Archive Center [data set], https://doi.org/10.5067/AMSR-E/AE_DYOCN.002, 2004. a, b
Wesely, M.: Parameterization of surface resistances to gaseous dry deposition in regional-scale numerical models, Atmos. Environ., 23, 1293–1304, 1989. a, b, c
Wesely, M. and Hicks, B.: A review of the current status of knowledge on dry deposition, Atmos. Environ., 34, 2261–2282, 2000. a
Wild, O., Zhu, X., and Prather, M. J.: Fast-J: Accurate simulation of in-and below-cloud photolysis in tropospheric chemical models, J. Atmos. Chem., 37, 245–282, 2000. a
Yang, X., Blechschmidt, A.-M., Bognar, K., McClure-Begley, A., Morris, S., Petropavlovskikh, I., Richter, A., Skov, H., Strong, K., Tarasick, D. W., Uttal, T., Vestenius, M., and Zhao, X.: Pan-Arctic surface ozone: modelling vs. measurements, Atmos. Chem. Phys., 20, 15937–15967, https://doi.org/10.5194/acp-20-15937-2020, 2020. a
Young, P. J., Naik, V., Fiore, A. M., Gaudel, A., Guo, J., Lin, M., Neu, J., Parrish, D., Rieder, H., Schnell, J., Tilmes, S., Wild, O., Zhang, L., Ziemke, J., Brandt, J., Delcloo, A., Doherty, R. M., Geels, C., Hegglin, M. I., Hu, L., Im, U., Kumar, R., Luhar, A., Murray, L., Plummer, D., Rodriguez, J., Saiz-Lopez, A., Schultz, M. G., Woodhouse, M. T., and Zeng, G.: Tropospheric Ozone Assessment Report: Assessment of global-scale model performance for global and regional ozone distributions, variability, and trends, Elementa: Science of the Anthropocene, 6, 10, https://doi.org/10.1525/elementa.265, 2018. a
Zaveri, R. A. and Peters, L. K.: A new lumped structure photochemical mechanism for large-scale applications, J. Geophys. Res.-Atmos., 104, 30387–30415, 1999. a
Zeller, K.: Wintertime ozone fluxes and profiles above a subalpine spruce–fir forest, J. Appl. Meteorol., 39, 92–101, 2000. a
- Abstract
- Introduction
- Methods
- Results
- Discussion
- Conclusions
- Appendix A: WRF physical and chemical parameterization schemes.
- Appendix B: Formulation of the air side and waterside resistance terms
- Appendix C: Spatial distribution of oceanic iodide
- Appendix D: Surface ozone measurement sites
- Code availability
- Data availability
- Author contributions
- Competing interests
- Disclaimer
- Acknowledgements
- Financial support
- Review statement
- References
- Abstract
- Introduction
- Methods
- Results
- Discussion
- Conclusions
- Appendix A: WRF physical and chemical parameterization schemes.
- Appendix B: Formulation of the air side and waterside resistance terms
- Appendix C: Spatial distribution of oceanic iodide
- Appendix D: Surface ozone measurement sites
- Code availability
- Data availability
- Author contributions
- Competing interests
- Disclaimer
- Acknowledgements
- Financial support
- Review statement
- References