the Creative Commons Attribution 4.0 License.
the Creative Commons Attribution 4.0 License.
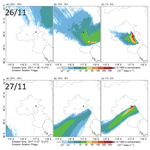
Simultaneous measurements of urban and rural particles in Beijing – Part 2: Case studies of haze events and regional transport
Yang Chen
Guangming Shi
Jing Cai
Zongbo Shi
Zhichao Wang
Xiaojiang Yao
Mi Tian
Chao Peng
Yiqun Han
Yue Liu
Xi Yang
Mei Zheng
Fumo Yang
Qiang Zhang
Kebin He
Two parallel field studies were conducted simultaneously at both urban and rural sites in Beijing from 1 to 29 November 2016. An online single-particle chemical composition analysis was used as a tracer system to investigate the impact of heating activities and the formation of haze events. Central heating elevated EC-Nit (elemental carbon-nitrate), EC-Nit-Sul (EC-nitrate-sulfate), and ECOC-Nit (ECOC: internal-mixed elemental carbon and organic carbon) levels by 1.5–2.0 times due to the increased use of coal in the urban areas. However, in the rural areas, residential heating, which mainly consumes low-quality coal, and biomass burning elevated ECOC-Nit-Sul, NaK-Nit, and OC-Sul levels by 1.2–1.5 times. Four severe haze events (hourly PM2.5 > 200 µg m−3) occurred at both sites during the studies. In each event, a pattern of transport and accumulation was found. In the first stage of the pattern, particles were regionally transported from the south and southwest and accumulated under air stagnation, creating significant secondary formation, then PM2.5 was elevated to 300 µg m−3. At both sites, the severe haze occurred due to different patterns of local emission, transport, and secondary processes. At Pinggu (PG), the sulfate-rich residential coal burning particles were dominant. The regional transport between PG and Peking University (PKU) was simulated using the Weather Research and Forecasting HYbrid Single-Particle Lagrangian Integrated Trajectory (WRF-HYSPLIT) model, confirming that the transport from PG to PKU was significant, but PKU to PG occurred occasionally. These cases can explain the serious air pollution in the urban areas of Beijing and the interaction between urban and rural areas. This study can provide references for enhancing our understanding of haze formation in Beijing.
- Article
(8226 KB) - Full-text XML
- Companion paper
-
Supplement
(937 KB) - BibTeX
- EndNote
The Beijing–Tianjin–Hebei (BTH) area in China has been suffering from extreme haze events caused by high concentrations of PM2.5 (> 200 µg m−3) since 2013 (Guo et al., 2014). Studies have been performed to understand the formation of such massive haze events in Beijing (Tian et al., 2014; Quan et al., 2013; Che et al., 2014; He et al., 2015). Traffic, cooking, and coal combustion emissions accounted for 41 %–59 % of the total submicron organic aerosols, and the remainder was secondary organic aerosols (Sun et al., 2014). Model studies suggest that temperature inversion, low boundary layer, and transported pollutants cause the local accumulation of PM2.5 in urban areas (Zhang et al., 2015). In short, significant local emissions, unfavorable meteorological conditions, and regional transport play essential roles in accumulating PM2.5.
There are unresolved issues surrounding whether the rapid boosting of PM in Beijing is due to local secondary aerosol formation or transport. Wang et al. (2016) have proposed that the accumulation of nitrates is dominant at the beginning of haze events, and then sulfate increases because SO2 is oxidized into sulfate in ammonium-rich conditions. Moreover, Cheng et al. (2016) have suggested that NO2 could oxidize SO2 to sulfate on the surface of alkali aerosols. However, Li et al. (2015) have argued that regionally transported PM2.5 is a significant cause of severe haze. Last but not least, Sun et al. (2013, 2014) have proposed that both local formation and regional transport are causal factors. Except for model studies, most field studies have focused on urban areas in Beijing with limited attention to rural areas. The characterization of rural PM is also essential to understanding the evolution of particulate haze events.
The cold winter results in the necessity of heating, consequently impacting the air quality in BTH (Sun et al., 2014). In urban areas, central heating systems use coal or natural gas, while rural households use coal or biofuel for heating and cooking. Residential emissions in Beijing reach about 4 million tons, mainly caused by low-efficiency coal combustion (Li et al., 2015). Coal combustion organic aerosols (CCOAs) account for 20 %–32 % of total submicron organic aerosols (OAs) in Beijing (Sun et al., 2013, 2014). However, whether CCOA is contributed by central or household heating remains unclear. Notably, central and household heating releases distinct particles due to different burning conditions (Lee et al., 2005; Chagger et al., 1999). Therefore, analyzing household heating and cooking emissions in rural areas is also beneficial for understanding the source of urban PM2.5 in Beijing.
The single-particle aerosol mass spectrometer (SPAMS) has proven to be a useful tool for characterizing the single-particle chemical composition, mixing state, and processing of atmospheric particles (Chen et al., 2019a). Single-particle chemical composition and mixing state can be used as a tracing system to explore the sources and origins of unique particle types (Chen et al., 2019b; Li et al., 2016). For example, by combining meteorological parameters, we can determine the sources and transport conditions of specific particle types (Chen et al., 2018, 2020).
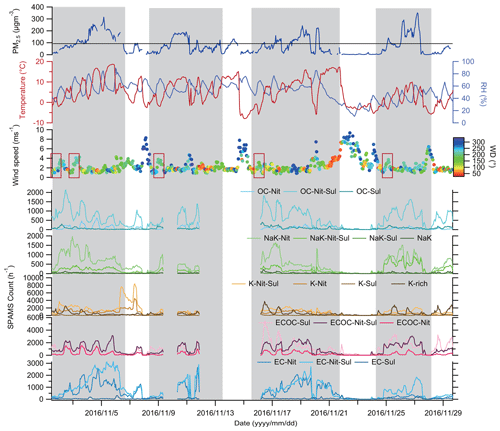
Figure 3Time trends of PM2.5, temperature, relative humidity, wind direction, wind speed, and single-particle types at PKU. The rectangles indicate the transport of regional particles.
As mentioned in Part 1 (Chen et al., 2020), two SPAMSs were deployed simultaneously at Peking University (PKU) and Pinggu (PG) to monitor urban and rural particles in the Beijing region. In Part 2, the resolved particle types are used to trace the evolution, transport, and formation of pollution events. The detailed analysis of haze events and effects of heating activities are addressed. Combining field measurements and model studies, the interactions between the two sampling sites, representing urban and rural eastern areas, are systematically analyzed.
2.1 Sampling sites, instrumentation, and data analysis
Please refer to Part 1 for details (Chen et al., 2020). Briefly, the field studies were performed simultaneously at Peking University (116.32∘ E, 39.99∘ N) and Pinggu (117.05∘ E, 40.17∘ N) from 1 to 29 November 2016 (Fig. 1). The detailed description of these two sites is available from Chen et al. (2020). The two sites represent both typical urban and rural areas respectively. The local meteorological data are retrieved from the local meteorological offices. Two SPAMSs (0515, Hexin Inc., Guangzhou, China) were deployed at both sites for parallel measurements. SPAMS generates single-particle mass spectra from the captured individual particles. The technical description of SPAMS is available in the literature (Li et al., 2011). A neural network algorithm based on adaptive resonance theory (ART-2a) was applied for clustering particle types in the datasets (Song et al., 1999). During the clustering procedure, the relative peak areas (RPAs) of sulfate and nitrate are considered. A criterion of RPA > 0.1 is used to identify whether they are nitrate-rich (-Nit), sulfate-rich (-Sul), or both. Based on the strategy, 20 and 19 particle types were identified at PKU and PG respectively.
Table 1Particle types and their relative fractions and chemical composition.
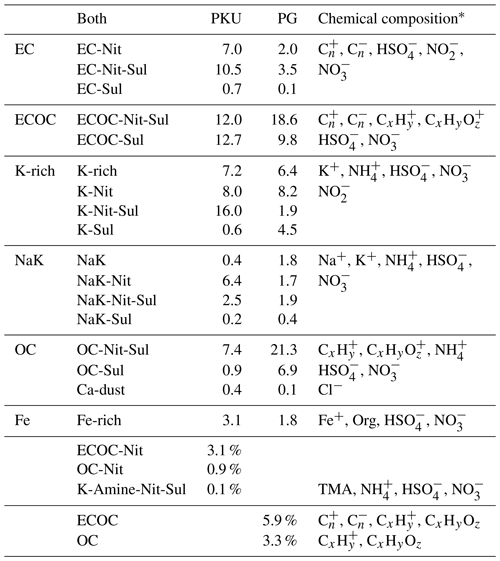
* Chemical species with ionic relative peak area > 0.1.
2.2 Dispersion model
A WRF-HYSPLIT (Weather Research and Forecasting HYbrid Single-Particle Lagrangian Integrated Trajectory) coupling model was used to describe the air parcel movement between PKU and PG. The description of the model is available at https://www.arl.noaa.gov/hysplit/inline-wrf-hysplit-coupling/ (last access: 20 June 2020). The HYSPLIT dispersion simulations were driven by the meteorological data fields from the WRF model version 3.8. The WRF domains are shown in Fig. 2. The innermost domain was configured to cover northern China with a horizontal resolution of 3 km and 35 vertical layers. The longwave and shortwave radiation schemes were set according to the RRTMG (Rapid Radiative Transfer Model for General Circulation Models) and Dudhia schemes respectively. The Yonsei University (YSU) scheme was used for the planetary boundary layer (PBL) parameterization. For the microphysics, the Morrison 2-moment scheme was adopted. NCEP FNL (National Centers for Environmental Prediction final) data with a resolution of was employed as initial and boundary conditions. The WRF simulation was initialized as a “cold start” at 00:00 UTC each day and ran for 36 h. The first 12 h were discarded as model spin-up time, and the output for the following 24 h was retained. This process was repeated to produce continuous meteorological data fields for the whole experimental period. The HYSPLIT was set to release 10 000 Lagrangian particles within 1 h at PKU and PG 10 m above ground level. The concentration of released particles was simulated with one vertical layer extending from 0 to 1000 m above ground level.
3.1 Particle type description
We observed five particle categories at both sites: elemental carbon (EC), organic carbon (OC), internal-mixed EC and OC (ECOC), potassium-rich (K-rich), and metals. According to their different stages of atmospheric processing, the five categories can be divided into up to 20 particle types, as shown in Table 1. Particles with relative peak areas of sulfate and nitrate greater than 0.1 were marked with sulfate (-Sul) and nitrate (-Nit), respectively, or both (-Nit-Sul). The typical single-particle mass spectra of all particle types are available in the Supplement. Moreover, the suffixes “_PKU” and “_PG” are used when the same particles appear. The higher relative abundance of secondary species indicates the particles are more aged (Chen et al., 2020).
As described in Part 1, we performed a responding analysis of meteorological factors (e.g., wind speed and wind direction) and hourly number counts of observed particles at both sites. At PKU, the following particle types were local: EC-Nit, EC-Nit-Sul, ECOC-Nit-Sul, Ca-rich, and ECOC-Nit. These particles arrived at PKU with no unique orientations, at low wind speed (commonly < 2 m s−1), and with clear diurnal patterns. In contrast, parts of OC-Nit, OC-Sul, NaK-Nit, and NaK-Nit-Sul responded to unique wind directions, implying that these particle types were regionally transported. At PG, all particle types showed patterns that were both local and regional. For example, OC, ECOC, OC-Nit-Sul, and ECOC-Nit-Sul came from the local area, northeast, and southwest. Universal patterns can be used to determine the mechanisms of pollution event formation when combined with unique cases.
3.2 Overview of haze events
Figures 3 and 4 show the overview of PM2.5, meteorology parameters, and time trends of particles at PKU and PG respectively. There were four parallel haze events during the observation period: 1–7 November (E1), 9–15 November (E2), 15–22 November (E3), and 25–28 November 2016 (E4).
The pattern of single-particle chemical composition, represented by normalized number fractions of particle types in different periods, is used to describe PM characteristics. The correlations of normalized number fractions during events at PKU and PG are shown in Tables 2 and S3 in the Supplement. E1_PKU was well correlated with Clear1 (R=0.90) and E2_PKU (R=0.86) but poorly correlated with Clear2 (R=0.38) and E4 (R=0.64). This is because E1_PKU and E2_PKU occurred before the heating period, but E4_PKU occurred after (15 November 2016). The chemical compositions of the four events at PG are highly correlated with each other (all Rs > 0.90; Table S3). These results indicate that the chemical composition patterns changed significantly at PKU but insignificantly at PG.
3.3 Influence of heating activities
Central heating began on 15 November 2016 in the urban area, while residential heating in the rural area had no distinct starting day. As such, the shift in emissions due to the increased use of solid fuel directly affected the particulate chemical composition. As shown in Fig. 5, the normalized fractions of EC-Nit_PKU, EC-Nit-Sul_PKU, and OC-Nit_PKU increased by about 1.5 times. EC-Nit_PKU and EC-Nit-Sul_PKU came from multiple local sources, one of which was coal burning in boilers (Xu et al., 2018). In addition, high EC concentrations have been observed during the heating period each year for decades (Chen et al., 2016b). The mass spectra of OC-Nit particles were composed of a series of ion fragments of polycyclic aromatic hydrocarbons (PAHs). The results are consistent with organic aerosols from coal burning in AMS-related studies (Wang et al., 2019). Additionally, PM2.5-bound PAHs increased by 3 times when the heating period began in Beijing (Zhang et al., 2017). The results also suggest the potential health risks of coal burning in wintertime in Beijing (Linak et al., 2007; Chen et al., 2013).
Biomass burning (BB) has been proven to be a significant source of PM2.5 in Beijing (Sun et al., 2014), accounting for 9 %–12 % (Liu et al., 2019). Anthropogenic BB, e.g., burning household biofuel, is prohibited in urban areas but common in the areas surrounding Beijing. Most BB-related particles such as K-rich, K-Nit, and K-Nit-Sul at PKU were regional (Part 1) (Chen et al., 2020). Not surprisingly, K-Nit_PKU and K-Nit-Sul_PKU both increased by 1.7 times after 15 November 2016. Interestingly, K-Amine-Nit_PKU increased by 2.3 times after the heating period began, suggesting that BB is also a source of particulate amines in Beijing (Chen et al., 2019b).
After 15 November 2016, NaK-Nit-Sul_PG, Ca-rich_PG, and OC-Sul_PG increased by 1.96, 1.30, and 1.47 times respectively. As described above, in rural areas, low-quality coal is commonly used for residential heating and cooking, resulting in abundant EC-Sul, OC-Sul, and NaK-Nit-Sul (Xu et al., 2018; Chen et al., 2016a). Interestingly, Ca-rich particles that were well correlated with OC-Sul (R=0.79) also increased, possibly due to fly ash from coal stoves.
A number of studies have reported contributions of coal burning to the submicron PM in urban areas of Beijing. According to these mass-based studies, PM-bound PAHs, chloride, sulfate, nitrate, and lead were markers for emissions of coal burning (Xu et al., 2018; Sun et al., 2014; Ma et al., 2016; Zhang et al., 2019). Our result shows that these species were internally mixed as the ECOC particles. In particular, the household heating in PG released significant fractions of ECOC particles that arrived in the urban areas of Beijing. Likewise, K-rich particles from BB were also transported to the urban areas of Beijing. Conclusively, the control of emissions from household heating is also key to improving the air quality in Beijing.
3.4 Case studies: haze events at PKU
As shown in Fig. 3, before PM2.5 increased to 100 µg m−3 during E1_PKU, two processes of PM2.5 transport were observed. The first process was from 12:00 on 1 November to 02:00 on 2 November 2016, in which OC-Nit-Sul, K-Nit-Sul, K-Nit, NaK-Nit, and K-Nit-Sul increased dramatically as the southerly wind speed increased from 1.3 to 3.7 m s−1. The wind speed then decreased to 1.2 m s−1 until 16:00 on 2 November 2016, and the accumulation of PM2.5 resulted in a concentration of 67 µg m−3. The second process occurred from 17:00 on 2 November to 16:00 on 3 November 2016. Severe accumulation then started at 01:00 on 4 November 2016 with an elevating trend in relative humidity (RH), reaching the highest PM2.5 level of 314 µg m−3 at 03:00 on 5 November 2016. After that, the wind dispersed the PM2.5 to 11 µg m−3 at 17:00 on 6 November 2016. In short, regional particles were transported from the south and southwest, and then the accumulation of PM2.5 began. The accumulation of pollutants was accompanied by secondary aerosol formation, causing severe haze events.
During the events at PKU (Fig. 3), particles transported from the south and southwest were observed and labeled with red rectangles. During E4_PKU, the PM2.5 concentration increased from 6 to 122 µg m−3 between 15:00 on 24 November and 03:00 on 25 November 2016 due to the southerly wind, which brought abundant NaK-Nit, NaK-Nit-Sul, ECOC-Nit-Sul, and EC-Nit-Sul. Notably, regional particles were dramatically different from those of E1_PKU due to the heating period. Then, under stagnant air conditions, the accumulation began at 22:00 on 25 November 2016 and lasted until 03:00 on 26 November 2016, with PM2.5 levels reaching 281 µg m−3. At this stage, such local particles as OC-Nit-Sul, ECOC-Nit-Sul, and ECOC-Nit also showed accumulation and local emissions, while both the K-rich and NaK families showed a pattern of transport and accumulation (Figs. 6 and 7).
As shown in Fig. 6, which gives an integrated view of related particle types in urban Beijing, three types of particle evolution are distinguished during E1. First, EC particles, including EC-Nit, EC-Nit-Sul, and EC-Sul, show trends of accumulation but with clear patterns of emissions, suggesting a pattern of emission and accumulation. Second, for regional particles such as the K-rich and NaK families, the processes of transport and accumulation were identified with significant accumulation but unclear diurnal patterns. Third, the OC and ECOC families illustrated clear diurnal patterns of local emission and evolution. Notably, during the development of E1, the fractions of aged ECOC-Nit-Sul increased from 20 % to 83 %, suggesting that significant secondary processing occurred.
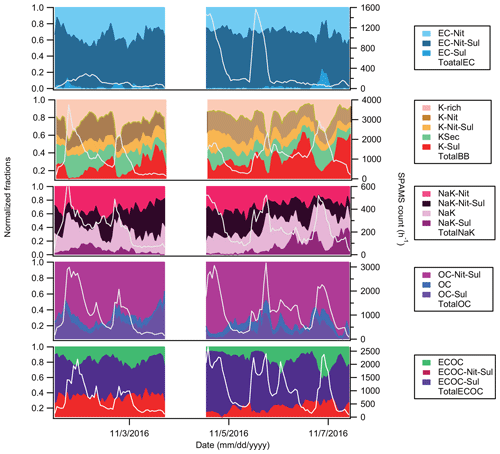
Figure 8Time trends of number fractions of particle types (left) and hourly counts of particle families (EC, BB, NaK, OC, and ECOC; right) during Pollution Event 1 (E1; 1–8 November) at PG.
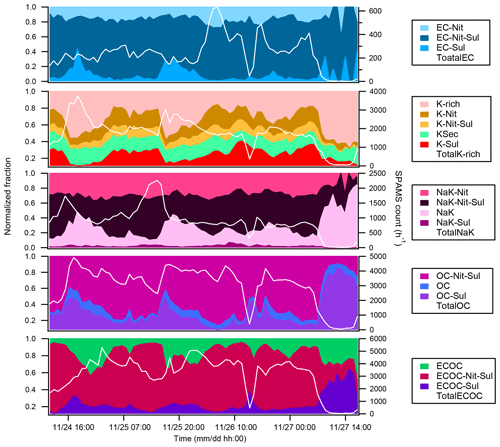
Figure 9Time trends of number fractions of particle types (left) and hourly counts of particle families (EC, BB, NaK, OC, and ECOC; right) during Pollution Event 4 (E4) at PG.
Due to the nature of SPAMS, the quantitative measurement of secondary formation is unavailable. Fortunately, as an integrated and extensive project, the APHH-Beijing (Air Pollution and Human Health) project also included the online monitoring of the chemical composition of PM2.5. For example, during the transport stage of E4_PKU, PM2.5 was composed of 60 % organic matter (OM) and 40 % total nitrate, sulfate, and ammonium. During the accumulation stage, sulfate, nitrate, and ammonium levels were elevated up to 123 µg m−3 (63 %) together (Liu et al., 2019). Wang et al. (2019) also reported that, during the accumulation stage of E4_PKU, the elevation of secondary oxygenated organic aerosol (OOA1 and OOA2) was significant.
In the most recent study of aerosol–radiation feedback deterioration in Beijing during wintertime, Wu et al. (2019) proposed that the increase of near-surface PM2.5 from 10 to 200 µg m−3 can result in a decrease in the PBL from 1500 to 400 m; the decrease consequently contributes to PM2.5 concentration by 20 %. However, a 20 % difference cannot explain the fact that PM2.5 concentration increased from 100 to 300 µg m−3. Moreover, when PM2.5 exceeded 200 µg m−3, the height of the PBL remained at 400–500 m, and air stagnation occurred with weak horizontal wind and inactive advection. Zhong et al. (2017) observed that weak temperature inversion occurred at the same period, and near-surface RH increased after southerly transport along with decreased vertical wind speed and increased RH during winter. Air stagnation was also observed in this study with low wind speed and high RH (Fig. 2). Based on the evidence of chemical evolution, the southerly transport of PM was strongly connected to pollution events at PKU.
3.5 Case studies: haze events at PG
A pollution event occurred at PG (E1_PG) from 1 to 8 November . During this period, a similar pattern of transport and accumulation as E1_PKU was also observed. At the beginning of each pollution event, there was also a transport process of particles from the southwest (Fig. 4); when the wind speed reached < 2 m s−1, accumulations began, and the haze dispersed with the elevating wind speed. The development of haze events was similar, and Fig. 4 lists all the favorable wind directions for transport marked with red rectangles. As shown in Fig. 9, EC-Nit and EC-Nit-Sul showed unclear diurnal patterns, indicating that both particle types were transported regionally. K-rich, NaK, OC, and ECOC had clear diurnal heating and cooking patterns, suggesting that local sources were dominant. Such aged particle types as OC-Nit-Sul and ECOC-Nit-Sul increased due to local aging processes during E1_PG. Therefore, E1_PG was mainly driven by the input of particles, local emissions, and accumulation. Moreover, the relative abundance of ECOC-Nit-Sul increased 2-fold from 02:00 on 3 November to 12:00 on 3 November 2016, suggesting the contribution of secondary formation (Fig. 8).
Both E1_PG and E1_PKU had patterns of transport and accumulation, but the transported particles were different; for example, at the PG site, the appearance of EC-Nit and EC-Nit-Sul, which came from the west, i.e., urban Beijing, was pronounced, while at PKU aged particle types such as OC-Nit-Sul, K-Nit-Sul, K-Nit, NaK-Nit, and K-Nit-Sul increased dramatically due to transport. These particle types were emitted from residential heating in rural areas. In the accumulation stages at both sites, the concentrations of local particles rose, such as EC-Nit-Sul at PKU and NaK-Nit-Sul at PG. In short, the evolution of particles, including both transport and accumulation at both PKU and PG, was affected by the movement of air mass and local emissions.
When E4_PG occurred, transport from the southwest was identified along with the transport of EC-Sul and EC-Nit-Sul, resulting in a PM2.5 concentration of 176 µg m−3 at 10:00 on 24 November 2016. The average wind speed was 1.5 m s−1 at the time, representing a typical stagnant-air condition. All particle families showed accumulation trends after that (Fig. 4). The sharp decrease in all particle families was due to the high westerly wind speed (> 4 m s−1) at 12:00 on 26 November 2016. During particulate accumulation at PG, such local particle types as ECOC, OC, and NaK still had diurnal patterns, but the aged -Nit-Sul particle types were predominant (> 50 % in all particle families). Thus, the local accumulation of pollutants was the major driver of E4_PG (Fig. 8).
3.6 Interaction of PM between PKU and PG
Since PKU and PG share 17 common particle types, possible transport between the two sites was validated using the HYSPLIT model. All cases of transport are available in the Supplement (Figs. S11 and S12). Figures 10 and 11 only illustrate the examples of transport during each pollution event. The PKU site is located on the edge of plumes originating from PG during E1, which implies that the particulate transport was partially from PG (Fig. 10). Moreover, the PKU site lies in the high concentration zone of PG plumes from E3 and E4. Therefore, E3_PKU and E4_PKU were confidently considered input haze events. In contrast, the relatively low transport of air mass from PKU to PG was observed during these events. As shown in Fig. 11, the air mass passing through the PKU site mainly influenced the areas in the south and east. Consequently, the PG site was seldom in the high concentration zone of plumes originating from PKU.
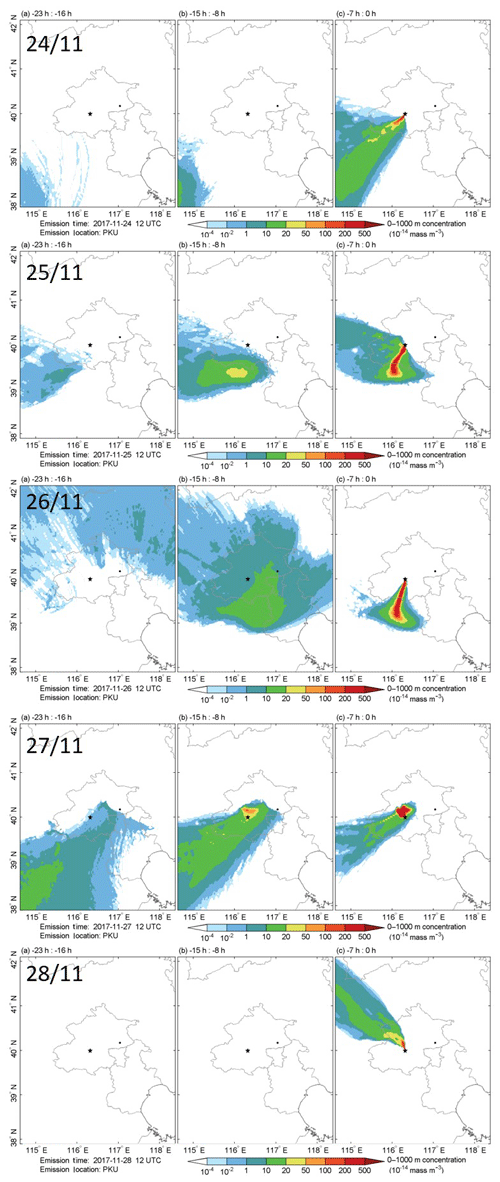
Figure 11Typical dispersion of air mass from PKU (star on the left) to PG (dot on the right) in E1 (1 November), E2 (11 November), E3 (19 November), and E4 (29 November).
Figures 10 and 11 suggest that pollutants were transported significantly from PG to PKU during stagnant air conditions when dense haze occurred. These results are consistent with the analysis of particle categories. As shown in Fig. 3, when the transport occurred on 4, 19, and 26 November, the regional particle types, such as K-Nit-Sul, NaK-Nit-Sul, ECOC-Nit-Sul, and OC-Nit-Sul, increased due to the transport from the east (Part 1). In an urban area such as PKU, the local EC particles were associated with the ECOC and OC families, causing severe pollution in the urban area. On the other hand, in the rural area, the aged particles were dominant under stagnant air conditions and transported to PKU, leading to extreme urban particulate pollution. Moreover, our results are consistent with other studies in the APHH-Beijing project. For example, Du et al. (2019) have confirmed that regional transport plays a non-negligible role in haze episodes with contributions of 14 %–31 % to the surface PM2.5 mass concentration.
3.7 Implications
This study provides the polar plots that are used to explain the interaction of pollutants and wind. Such regional pollution sources as BB, coal, and steel industries have a strong impact on the particulate chemical composition of the air in urban Beijing. Moreover, according to model studies, air pollutants in Hebei, Henan, and Shandong provinces are transported to Beijing (Shi et al., 2019; Du et al., 2019). In these provinces, efforts have been made to abate emissions from the steel industry, power plants, and traffic. However, BB accounted for 10 %–20 % of the PM2.5 in the study period (Liu et al., 2019). In particular, household biofuel combustion is a primary BB source during winter, impacting both outdoor and indoor air quality (Zhang and Cao, 2015). Therefore, more attention should be paid to tackling BB emissions.
This study improves our general understanding of the sources of sulfates in Beijing. Particles that only increased with the uptake of sulfate, such as OC-Sul_PKU, K-Sul_PKU, and NaK-Sul_PKU, were transported regionally and arrived at the sampling site during high wind speeds (> 4 m s−1). The results are consistent with the findings of Duan et al. (2019) and Du et al. (2019), which are that sulfates in Beijing during winter are formed regionally. Nitrate-containing particles could be found after processing in the NOx-rich urban and rural plumes of Beijing. Since SPAMS is limited in tracking such partial organics as hydrocarbons and PAHs, the evolution of secondary organics is unavailable in this study.
The wintertime haze events that occurred in Beijing from 1 to 29 November 2016 have been investigated. The heating period, including central and residential heating in both urban and rural areas, severely impacted the particulate chemical composition in the region. In Beijing, a pattern of the transport and accumulation of particles was found in both the urban and rural areas. The input of regional particles was strongly connected to stagnation of the air which provided favorable conditions for the accumulation of pollutants, ultimately leading to severe haze events. In the rural area, the heavy haze was mainly controlled by air stagnation and local emissions, but regional transport was also observed. We also discussed the influence of regional transport using the dispersion model. The air masses between PKU and PG interacted with each other whenever heavy haze occurred. Parts 1 and 2 of this study are useful for understanding the formation mechanism of winter haze in both the urban and rural areas of Beijing. This study also implies that the mitigation of PM is dependent on emissions from both urban and rural areas.
All the data described in this study are available upon request from the corresponding authors.
The supplement related to this article is available online at: https://doi.org/10.5194/acp-20-9249-2020-supplement.
YC, FY, MZ, TZ, QZ, and KH designed the experiments; YC, JC, ZW, MT, CP, and YH carried them out; XY, GS, and ZS analyzed the experimental data; and YC prepared the paper with contributions from all coauthors.
The authors declare that they have no conflict of interest.
This article is part of the special issue “In-depth study of air pollution sources and processes within Beijing and its surrounding region (APHH-Beijing) (ACP/AMT inter-journal SI)”. It is not associated with a conference.
We are grateful for the financial support from the National Natural Science Foundation of China (Grant No. 41703136 and 81571130100).
This research has been supported by the National Natural Science Foundation of China (grant nos. 41703136 and 81571130100).
This paper was edited by James Allan and reviewed by two anonymous referees.
Chagger, H., Jones, J., Pourkashanian, M., Williams, A., Owen, A., and Fynes, G.: Emission of volatile organic compounds from coal combustion, Fuel, 78, 1527–1538, 1999.
Che, H., Xia, X., Zhu, J., Li, Z., Dubovik, O., Holben, B., Goloub, P., Chen, H., Estelles, V., Cuevas-Agulló, E., Blarel, L., Wang, H., Zhao, H., Zhang, X., Wang, Y., Sun, J., Tao, R., Zhang, X., and Shi, G.: Column aerosol optical properties and aerosol radiative forcing during a serious haze-fog month over North China Plain in 2013 based on ground-based sunphotometer measurements, Atmos. Chem. Phys., 14, 2125–2138, https://doi.org/10.5194/acp-14-2125-2014, 2014.
Chen, Y., Ebenstein, A., Greenstone, M., and Li, H.: Evidence on the impact of sustained exposure to air pollution on life expectancy from China's Huai River policy, P. Natl. Acad. Sci. USA, 110, 12936–12941, 2013.
Chen, Y., Cao, J., Huang, R., Yang, F., Wang, Q., and Wang, Y.: Characterization, mixing state, and evolution of urban single particles in Xi'an (China) during wintertime haze days, Sci. Total Environ., 573, 937–945, https://doi.org/10.1016/j.scitotenv.2016.08.151, 2016a.
Chen, Y., Schleicher, N., Fricker, M., Cen, K., Liu, X. L., Kaminski, U., Yu, Y., Wu, X. F., and Norra, S.: Long-term variation of black carbon and PM2.5 in Beijing, China with respect to meteorological conditions and governmental measures, Environ. Pollut., 212, 269–278, https://doi.org/10.1016/j.envpol.2016.01.008, 2016b.
Chen, Y., Liu, H., Yang, F., Zhang, S., Li, W., Shi, G., Wang, H., Tian, M., Liu, S., Huang, R., Wang, Q., Wang, P., and Cao, J.: Single particle characterization of summertime particles in Xi'an (China), Sci. Total Environ., 636, 1279–1290, https://doi.org/10.1016/j.scitotenv.2018.04.388, 2018.
Chen, Y., Liu, H., Huang, R. J., Yang, F., Tian, M., Yao, X., Shen, Z., Yan, L., and Cao, J.: Atmospheric Processing of Loess Particles in a Polluted Urban Area of Northwestern China, J. Geophys. Res.-Atmos., 124, 7919–7929, https://doi.org/10.1029/2018jd029956, 2019a.
Chen, Y., Tian, M., Huang, R.-J., Shi, G., Wang, H., Peng, C., Cao, J., Wang, Q., Zhang, S., Guo, D., Zhang, L., and Yang, F.: Characterization of urban amine-containing particles in southwestern China: seasonal variation, source, and processing, Atmos. Chem. Phys., 19, 3245–3255, https://doi.org/10.5194/acp-19-3245-2019, 2019b.
Chen, Y., Cai, J., Wang, Z., Peng, C., Yao, X., Tian, M., Han, Y., Shi, G., Shi, Z., Liu, Y., Yang, X., Zheng, M., Zhu, T., He, K., Zhang, Q., and Yang, F.: Simultaneous measurements of urban and rural particles in Beijing – Part 1: Chemical composition and mixing state, Atmos. Chem. Phys., 20, 9231–9247, https://doi.org/10.5194/acp-20-9231-2020, 2020.
Cheng, Y., Zheng, G., Wei, C., Mu, Q., Zheng, B., Wang, Z., Gao, M., Zhang, Q., He, K., and Carmichael, G.: Reactive nitrogen chemistry in aerosol water as a source of sulfate during haze events in China, Sci. Adv., 2, e1601530, https://doi.org/10.1126/sciadv.1601530, 2016.
Du, H., Li, J., Chen, X., Wang, Z., Sun, Y., Fu, P., Li, J., Gao, J., and Wei, Y.: Modeling of aerosol property evolution during winter haze episodes over a megacity cluster in northern China: roles of regional transport and heterogeneous reactions of SO2, Atmos. Chem. Phys., 19, 9351–9370, https://doi.org/10.5194/acp-19-9351-2019, 2019.
Duan, J., Huang, R.-J., Lin, C., Dai, W., Wang, M., Gu, Y., Wang, Y., Zhong, H., Zheng, Y., Ni, H., Dusek, U., Chen, Y., Li, Y., Chen, Q., Worsnop, D. R., O'Dowd, C. D., and Cao, J.: Distinctions in source regions and formation mechanisms of secondary aerosol in Beijing from summer to winter, Atmos. Chem. Phys., 19, 10319–10334, https://doi.org/10.5194/acp-19-10319-2019, 2019.
Guo, S., Hu, M., Zamora, M. L., Peng, J., Shang, D., Zheng, J., Du, Z., Wu, Z., Shao, M., Zeng, L., Molina, M. J., and Zhang, R.: Elucidating severe urban haze formation in China, P. Natl. Acad. Sci. USA, 111, 17373–17378, https://doi.org/10.1073/pnas.1419604111, 2014.
He, H., Tie, X., Zhang, Q., Liu, X., Gao, Q., Li, X., and Gao, Y.: Analysis of the causes of heavy aerosol pollution in Beijing, China: A case study with the WRF-Chem model, Particuology, 20, 32–40, 2015.
Lee, R. G., Coleman, P., Jones, J. L., Jones, K. C., and Lohmann, R.: Emission factors and importance of PCDD/Fs, PCBs, PCNs, PAHs and PM10 from the domestic burning of coal and wood in the UK, Environ. Sci. Technol., 39, 1436–1447, 2005.
Li, L., Huang, Z., Dong, J., Li, M., Gao, W., Nian, H., Fu, Z., Zhang, G., Bi, X., Cheng, P., and Zhou, Z.: Real time bipolar time-of-flight mass spectrometer for analyzing single aerosol particles, Int. J. Mass Spectrom., 303, 118–124, https://doi.org/10.1016/j.ijms.2011.01.017, 2011.
Li, P., Yan, R., Yu, S., Wang, S., Liu, W., and Bao, H.: Reinstate regional transport of PM2.5 as a major cause of severe haze in Beijing, P. Natl. Acad. Sci. USA, 112, E2739–E2740, https://doi.org/https://doi.org/10.1073/pnas.1502596112, 2015.
Li, W., Shao, L., Zhang, D., Ro, C.-U., Hu, M., Bi, X., Geng, H., Matsuki, A., Niu, H., and Chen, J.: A review of single aerosol particle studies in the atmosphere of East Asia: morphology, mixing state, source, and heterogeneous reactions, J. Clean. Prod., 112, 1330–1349, https://doi.org/10.1016/j.jclepro.2015.04.050, 2016.
Linak, W. P., Yoo, J.-I., Wasson, S. J., Zhu, W., Wendt, J. O. L., Huggins, F. E., Chen, Y., Shah, N., Huffman, G. P., and Gilmour, M. I.: Ultrafine ash aerosols from coal combustion: Characterization and health effects, P. Combust. Inst., 31, 1929–1937, https://doi.org/10.1016/j.proci.2006.08.086, 2007.
Liu, Y., Zheng, M., Yu, M., Cai, X., Du, H., Li, J., Zhou, T., Yan, C., Wang, X., Shi, Z., Harrison, R. M., Zhang, Q., and He, K.: High-time-resolution source apportionment of PM2.5 in Beijing with multiple models, Atmos. Chem. Phys., 19, 6595–6609, https://doi.org/10.5194/acp-19-6595-2019, 2019.
Ma, L., Li, M., Huang, Z., Li, L., Gao, W., Nian, H., Zou, L., Fu, Z., Gao, J., Chai, F., and Zhou, Z.: Real time analysis of lead-containing atmospheric particles in Beijing during springtime by single particle aerosol mass spectrometry, Chemosphere, 154, 454–462, https://doi.org/10.1016/j.chemosphere.2016.04.001, 2016.
Quan, J., Gao, Y., Zhang, Q., Tie, X., Cao, J., Han, S., Meng, J., Chen, P., and Zhao, D.: Evolution of planetary boundary layer under different weather conditions, and its impact on aerosol concentrations, Particuology, 11, 34–40, https://doi.org/10.1016/j.partic.2012.04.005, 2013.
Shi, Z., Vu, T., Kotthaus, S., Harrison, R. M., Grimmond, S., Yue, S., Zhu, T., Lee, J., Han, Y., Demuzere, M., Dunmore, R. E., Ren, L., Liu, D., Wang, Y., Wild, O., Allan, J., Acton, W. J., Barlow, J., Barratt, B., Beddows, D., Bloss, W. J., Calzolai, G., Carruthers, D., Carslaw, D. C., Chan, Q., Chatzidiakou, L., Chen, Y., Crilley, L., Coe, H., Dai, T., Doherty, R., Duan, F., Fu, P., Ge, B., Ge, M., Guan, D., Hamilton, J. F., He, K., Heal, M., Heard, D., Hewitt, C. N., Hollaway, M., Hu, M., Ji, D., Jiang, X., Jones, R., Kalberer, M., Kelly, F. J., Kramer, L., Langford, B., Lin, C., Lewis, A. C., Li, J., Li, W., Liu, H., Liu, J., Loh, M., Lu, K., Lucarelli, F., Mann, G., McFiggans, G., Miller, M. R., Mills, G., Monk, P., Nemitz, E., O'Connor, F., Ouyang, B., Palmer, P. I., Percival, C., Popoola, O., Reeves, C., Rickard, A. R., Shao, L., Shi, G., Spracklen, D., Stevenson, D., Sun, Y., Sun, Z., Tao, S., Tong, S., Wang, Q., Wang, W., Wang, X., Wang, X., Wang, Z., Wei, L., Whalley, L., Wu, X., Wu, Z., Xie, P., Yang, F., Zhang, Q., Zhang, Y., Zhang, Y., and Zheng, M.: Introduction to the special issue “In-depth study of air pollution sources and processes within Beijing and its surrounding region (APHH-Beijing)”, Atmos. Chem. Phys., 19, 7519–7546, https://doi.org/10.5194/acp-19-7519-2019, 2019.
ong, X. H., Hopke, P. K., Fergenson, D. P., and Prather, K. A.: Classification of single particles analyzed by ATOFMS using an artificial neural network, ART-2A, Anal. Chem., 71, 860–865, https://doi.org/10.1021/ac9809682, 1999.
Sun, Y., Wang, Z., Fu, P., Jiang, Q., Yang, T., Li, J., and Ge, X.: The impact of relative humidity on aerosol composition and evolution processes during wintertime in Beijing, China, Atmos. Environ., 77, 927–934, https://doi.org/10.1016/j.atmosenv.2013.06.019, 2013.
Sun, Y., Jiang, Q., Wang, Z., Fu, P., Li, J., Yang, T., and Yin, Y.: Investigation of the sources and evolution processes of severe haze pollution in Beijing in January 2013, J. Geophys. Res.-Atmos., 119, 4380–4398, https://doi.org/10.1002/2014jd021641, 2014.
Tian, S., Pan, Y., Liu, Z., Wen, T., and Wang, Y.: Size-resolved aerosol chemical analysis of extreme haze pollution events during early 2013 in urban Beijing, China, J. Hazard. Mater., 279, 452–460, 2014.
Wang, G., Zhang, R., Gomez, M. E., Yang, L., Levy Zamora, M., Hu, M., Lin, Y., Peng, J., Guo, S., Meng, J., Li, J., Cheng, C., Hu, T., Ren, Y., Wang, Y., Gao, J., Cao, J., An, Z., Zhou, W., Li, G., Wang, J., Tian, P., Marrero-Ortiz, W., Secrest, J., Du, Z., Zheng, J., Shang, D., Zeng, L., Shao, M., Wang, W., Huang, Y., Wang, Y., Zhu, Y., Li, Y., Hu, J., Pan, B., Cai, L., Cheng, Y., Ji, Y., Zhang, F., Rosenfeld, D., Liss, P. S., Duce, R. A., Kolb, C. E., and Molina, M. J.: Persistent sulfate formation from London Fog to Chinese haze, P. Natl. Acad. Sci. USA, 113, 13630–13635, https://doi.org/10.1073/pnas.1616540113, 2016.
Wang, J., Liu, D., Ge, X., Wu, Y., Shen, F., Chen, M., Zhao, J., Xie, C., Wang, Q., Xu, W., Zhang, J., Hu, J., Allan, J., Joshi, R., Fu, P., Coe, H., and Sun, Y.: Characterization of black carbon-containing fine particles in Beijing during wintertime, Atmos. Chem. Phys., 19, 447–458, https://doi.org/10.5194/acp-19-447-2019, 2019.
Wu, J., Bei, N., Hu, B., Liu, S., Zhou, M., Wang, Q., Li, X., Liu, L., Feng, T., Liu, Z., Wang, Y., Cao, J., Tie, X., Wang, J., Molina, L. T., and Li, G.: Aerosol–radiation feedback deteriorates the wintertime haze in the North China Plain, Atmos. Chem. Phys., 19, 8703–8719, https://doi.org/10.5194/acp-19-8703-2019, 2019.
Xu, J., Wang, H., Li, X., Li, Y., Wen, J., Zhang, J., Shi, X., Li, M., Wang, W., Shi, G., and Feng, Y.: Refined source apportionment of coal combustion sources by using single particle mass spectrometry, Sci. Total Environ., 627, 633–646, https://doi.org/10.1016/j.scitotenv.2018.01.269, 2018.
Zhang, J., Huang, X., Chen, Y., Luo, B., Luo, J., Zhang, W., Rao, Z., and Yang, F.: Characterization of lead-containing atmospheric particles in a typical basin city of China: Seasonal variations, potential source areas, and responses to fireworks, Sci. Total Environ., 661, 354–363, https://doi.org/10.1016/j.scitotenv.2019.01.079, 2019.
Zhang, L., Wang, T., Lv, M., and Zhang, Q.: On the severe haze in Beijing during January 2013: Unraveling the effects of meteorological anomalies with WRF-Chem, Atmos. Environ., 104, 11–21, https://doi.org/10.1016/j.atmosenv.2015.01.001, 2015.
Zhang, Y., Chen, J., Yang, H., Li, R., and Yu, Q.: Seasonal variation and potential source regions of PM2.5-bound PAHs in the megacity Beijing, China: Impact of regional transport, Environ. Pollut., 231, 329–338, https://doi.org/10.1016/j.envpol.2017.08.025, 2017.
Zhang, Y. L. and Cao, F.: Is it time to tackle PM2.5 air pollutions in China from biomass-burning emissions?, Environ. Pollut., 202, 217–219, https://doi.org/10.1016/j.envpol.2015.02.005, 2015.
Zhong, J., Zhang, X., Wang, Y., Sun, J., Zhang, Y., Wang, J., Tan, K., Shen, X., Che, H., Zhang, L., Zhang, Z., Qi, X., Zhao, H., Ren, S., and Li, Y.: Relative contributions of boundary-layer meteorological factors to the explosive growth of PM2.5 during the red-alert heavy pollution episodes in Beijing in December 2016, J. Meteorol. Res., 31, 809–819, https://doi.org/10.1007/s13351-017-7088-0, 2017.