the Creative Commons Attribution 4.0 License.
the Creative Commons Attribution 4.0 License.
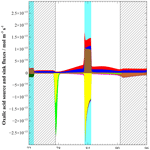
Multiphase MCM–CAPRAM modeling of the formation and processing of secondary aerosol constituents observed during the Mt. Tai summer campaign in 2014
Yanhong Zhu
Andreas Tilgner
Erik Hans Hoffmann
Kimitaka Kawamura
Lingxiao Yang
Likun Xue
Wenxing Wang
Despite the high abundance of secondary aerosols in the atmosphere, their formation mechanisms remain poorly understood. In this study, the Master Chemical Mechanism (MCM) and the Chemical Aqueous-Phase Radical Mechanism (CAPRAM) are used to investigate the multiphase formation and processing of secondary aerosol constituents during the advection of air masses towards the measurement site of Mt. Tai in northern China. Trajectories with and without chemical–cloud interaction are modeled. Modeled radical and non-radical concentrations demonstrate that the summit of Mt. Tai, with an altitude of ∼1.5 km a.m.s.l., is characterized by a suburban oxidants budget. The modeled maximum gas-phase concentrations of the OH radical are 3.2×106 and 3.5×106 molec. cm−3 in simulations with and without cloud passages in the air parcel, respectively. In contrast with previous studies at Mt. Tai, this study has modeled chemical formation processes of secondary aerosol constituents under day vs. night and cloud vs. non-cloud cases along the trajectories towards Mt. Tai in detail. The model studies show that sulfate is mainly produced in simulations where the air parcel is influenced by cloud chemistry. Under the simulated conditions, the aqueous reaction of with H2O2 is the major contributor to sulfate formation, contributing 67 % and 60 % in the simulations with cloud and non-cloud passages, respectively. The modeled nitrate formation is higher at nighttime than during daytime. The major pathway is aqueous-phase N2O5 hydrolysis, with a contribution of 72 % when cloud passages are considered and 70 % when they are not. Secondary organic aerosol (SOA) compounds, e.g., glyoxylic, oxalic, pyruvic and malonic acid, are found to be mostly produced from the aqueous oxidations of hydrated glyoxal, hydrated glyoxylic acid, nitro-2-oxopropanoate and hydrated 3-oxopropanoic acid, respectively. Sensitivity studies reveal that gaseous volatile organic compound (VOC) emissions have a huge impact on the concentrations of modeled secondary aerosol compounds. Increasing the VOC emissions by a factor of 2 leads to linearly increased concentrations of the corresponding SOA compounds. Studies using the relative incremental reactivity (RIR) method have identified isoprene, 1,3-butadiene and toluene as the key precursors for glyoxylic and oxalic acid, but only isoprene is found to be a key precursor for pyruvic acid. Additionally, the model investigations demonstrate that an increased aerosol partitioning of glyoxal can play an important role in the aqueous-phase formation of glyoxylic and oxalic acid. Overall, the present study is the first that provides more detailed insights in the formation pathways of secondary aerosol constituents at Mt. Tai and clearly emphasizes the importance of aqueous-phase chemical processes on the production of multifunctional carboxylic acids.
- Article
(6911 KB) - Full-text XML
-
Supplement
(6849 KB) - BibTeX
- EndNote
Secondary aerosols are more abundant than primary aerosols (Volkamer et al., 2006). Their constituents are formed on a regional scale and transported over long distances and thus have a direct impact on the air quality of a wider area (Kim et al., 2007; Matsui et al., 2009; DeCarlo et al., 2010). Secondary aerosols are usually divided into two classes: secondary inorganic aerosol (SIA) and secondary organic aerosol (SOA). A number of studies have been conducted that aimed to investigate their formation mechanisms (Yao et al., 2002; Duan et al., 2006; Wang et al., 2006; Guo et al., 2010; Zhao et al., 2013). The SIA components, including sulfate, nitrate and ammonium, are important contributors to fine particulate matter (PM2.5) and play an important role in haze formation (Volkamer et al., 2006; Sun et al., 2014; Wang et al., 2014; Zhang et al., 2014). The SIA formation processes are relatively well understood, but some indefiniteness still remains, such as multiphase formation, particularly under highly polluted conditions such as in those China (Wang et al., 2014; G. Wang et al., 2016). SOA is also a key component of PM2.5 and linked to adverse health effects, visibility reduction and climate change (Tabazadeh, 2005; Seagrave et al., 2006; De Gouw and Jimenez, 2009; Shrivastava et al., 2017). However, their formation mechanisms are still not well understood (Huang et al., 2014).
Dicarboxylic acids and related compounds (oxo-carboxylic acids and α-dicarbonyls) (DCRCs) are ubiquitous water-soluble components of SOA (Kawamura and Sakaguchi, 1999; Kawamura and Yasui, 2005; Pavuluri et al., 2010). They are mainly produced by secondary processes of precursors via gas-phase and subsequent aqueous-phase reactions (Glasius et al., 2000; Legrand et al., 2007; Kundu et al., 2010; Tilgner and Herrmann, 2010). A detailed knowledge of the formation processes of DCRCs is helpful to better understand the fate of SOAs in the troposphere. A number of studies have proposed that aromatic hydrocarbons, isoprene and ethene are important precursors for DCRCs (Warneck, 2003; Ervens et al., 2004; Bikkina et al., 2014; Tilgner and Herrmann, 2010). However, modeling studies that focus on understanding of DCRC formation pathways based on field measurements are limited. Additionally, model studies show growing evidence that substantial amounts of DCRCs are formed by aqueous-phase reactions within aerosol particles, clouds and fog droplets (Sorooshian et al., 2006; Carlton et al., 2007, 2009; Ervens et al., 2008, 2011; Ervens, 2015; Tilgner and Herrmann, 2010; Tilgner et al., 2013; Mouchel-Vallon et al., 2017). Nevertheless, the applied mechanisms are still incomplete and the formation processes are therefore not completely understood. Hence, in this study, a near-explicit multiphase model is applied to investigate the chemical processing of DCRCs in both gas and aqueous phases in order to understand the formation processes and the fate of DCRCs in the atmosphere.
The present study focuses on the multiphase formation mechanism of key secondary aerosol constituents measured in June 2014 at Mt. Tai, which is the highest mountain on the North China Plain (NCP). Mt. Tai is located in Shandong province on the NCP and between the Bohai Rim (BHR) and the Yangtze River Delta (YRD) regions. Together, the BHR and YRD regions had a population of more than 450 million in 2018 (China Statistical Yearbook in 2019). In summer, clouds frequently occur over the summit of Mt. Tai. Despite a small amount of emissions from temples and small restaurants at Mt. Tai's peak, the sampling site on top of Air Force Hotel, Houshiwu, was typically not influenced much by tourists and temples (Sun et al., 2016). The special altitude and geographical location of Mt. Tai provide a suitable site to measure regional secondary aerosol constituents and to investigate their formation pathways along the advection to the measurement site.
The detailed objectives of the present study are as follows: (i) characterization of modeled radical and non-radical oxidant concentrations; (ii) assessment of modeled concentrations and formation processes of key secondary inorganic compounds; (iii) study of modeled concentrations of DCRCs and a comparison with field observations to assess the model predictions; (iv) investigation of source and sink pathways of selected DCRCs; (v) examination of the impact of emission data on modeled secondary aerosol concentrations; (vi) identification of the key precursors of selected DCRCs; and (vii) the impact of higher glyoxal (Gly) partitioning constants on the modeled concentrations of Gly, glyoxylic acid (ωC2), and oxalic acid (C2).
Detailed descriptions about the sampling site, the sampling instruments and the analysis methods can be found in a previous publication (Zhu et al., 2018). Campaign observation data, meteorological conditions and corresponding findings are also given there. The sampling period was from 4 June to 4 July 2014. The meteorological data during the campaign were as follows: temperatures ranged from 10 to 25 ∘C, with an average of 17±6.2 ∘C; relative humidity (RH) ranged from 58 % to 100 %, with an average of 87±13 %; the prevailing wind direction was northwest; and wind speeds ranged from 1 to 7 m s−1. The weather conditions were mostly cloudy and occasionally foggy. Source identification indicated that DCRCs were mainly derived from anthropogenic activities followed by photochemical aging. Secondary sources, fuel combustion, photooxidation of unsaturated fatty acids and waste burning were also significant sources. DCRCs exhibited mostly similar concentrations during daytime and nighttime, e.g., C2, pyruvic acid (Pyr) and ωC2 (Zhu et al., 2018). These results differed from previous studies at urban (New Delhi, India) (Miyazaki et al., 2009), suburban (the northern vicinity of Beijing) (He et al., 2013) and mountain sites (Central Himalayan) (Hegde and Kawamura, 2012), where diurnal variations were observed. Therefore, this study focused on the formation processes of these compounds to better understand the similar concentrations during daytime and nighttime summer conditions at Mt. Tai.
2.1 Model and mechanism description
In this study, we applied the air parcel model SPACCIM (SPectral Aerosol Cloud Chemistry Interaction Model, Wolke et al., 2005) to simulate multiphase chemistry along main trajectories during a simulated campaign period. SPACCIM combines a multiphase chemical model with a cloud microphysical model, simulating aqueous-phase chemistry in deliquesced particles and cloud droplets. The cloud microphysical model applied in SPACCIM is based on the work of Simmel and Wurzler (2006) and Simmel et al. (2005). Droplet formation, evolution and evaporation are realized by a one-dimensional sectional microphysics considering deliquesced particles and cloud droplets. In the present study, the moving bin version of SPACCIM has been applied. In the model, the growth and shrinking of aerosol particles by water vapor diffusion and nucleation and the growth and evaporation of cloud droplets is considered. The dynamic growth rate in the condensation and evaporation process and the droplet activation is based on the Köhler theory. Due to the emphasis on complex multiphase chemistry, other microphysical processes, such as impaction of aerosol particles and collision and coalescence of droplets and thus precipitation, were not considered in the present study. Moreover, the air parcel model SPACCIM is not able to reflect the complexity of tropospheric mixing processes. Nevertheless, the complex model enables detailed investigations of the multiphase chemical processing of gases, deliquescent particles and cloud droplets. More detailed descriptions of SPACCIM can be found in Wolke et al. (2005), Sehili et al. (2005) and Tilgner et al. (2013). However, SPACCIM cannot assess the complexity of (i) the tropospheric mixing processes along the transport, (ii) the occurring aerosol particle microphysical processes (e.g., nucleation, aggregation, etc.) or (iii) the effects of nonideal solutions on the occurring multiphase chemistry. These limitations have to be kept in mind when studying deliquesced particles and comparing predicted and observed concentrations at Mt. Tai. The potential limitations of an ideal solution assumption compared to a nonideal treatment are discussed in a recent paper by Rusumdar et al. (2020).
The applied multiphase chemistry mechanism is comprised of the Master Chemical Mechanism (MCM3.2 scheme with 13 927 reactions, http://mcm.leeds.ac.uk/MCM/, last access: 16 August 2019; Jenkin et al., 2003; Saunders et al., 2003) and the Chemical Aqueous-Phase Radical Mechanism (CAPRAM4.0 scheme with 7129 reactions; Bräuer et al., 2019). MCM3.2 is a near-explicit gas-phase chemistry mechanism, while CAPRAM4.0 explicitly describes the aqueous-phase chemical and phase transfer processes of inorganic compounds and organic compounds of up to 4 carbon atoms. Phase exchange processes (in total 275) are implemented based on the kinetic resistance model of Schwartz (1986), in which the mass accommodation coefficient, the gas-phase diffusion coefficient and the Henry's law constant are considered.
2.2 Trajectories and performed model simulations
Zhu et al. (2018) have shown that during the sampling period (4 June–4 July 2014) air masses arriving at Mt. Tai mainly came from the north (named cluster 2) and the south (named cluster 4) (Fig. S1 in the Supplement). The two clusters accounted for 79 % of the total trajectories. Moreover, the sum of DCRC concentrations in clusters 2 and 4 amounted to 73 % of total DCRC concentration during the sampling period. Therefore, in this study, we selected clusters 2 and 4 to simulate and investigate the formation processes and the fate of DCRCs. Additionally, Zhu et al. (2018) have clearly shown that biomass burning was only an important source during the first half of the sampling period (4–19 June). The aim of the study was to investigate the secondary formation of aerosol constituents along the trajectories towards Mt. Tai. However, biomass burning can be an important primary source of compounds that are often of secondary origin. Therefore, in this study, we focused on the period that was less impacted by biomass burning. In addition, both clusters 2 and 4 exhibited a rather stable transport above the mixing layer to the Mt. Tai site.
A total simulation time of 96 h is chosen (4 d), representing a typical aerosol lifespan (Willams et al., 2002). The first 24 h are considered a model initialization day. Thus, only the model results from 24 to 96 h are presented in this study. With the help of measured RH at Mt. Tai and meteorological values of clusters 2 and 4 that are obtained by HYSPLIT4.9 (Draxler and Rolph, 2003) and MODIS satellite pictures (Li et al., 2005), we have ascertained that clouds most likely occurred at the Mt. Tai top and advected to Mt. Tai at the altitude of the trajectories (Zhu et al., 2018). Radiosonde data (http://weather.uwyo.edu/upperair/sounding.html, last access: 28 August 2019) also show that clouds could occur in the trajectories of clusters 2 and 4. Cloud interactions are considered on the basis of the measured RH values at Mt. Tai and RH values in radiosonde data at about 1500 m. The fractions of RH values above 90 % are calculated and used as a representative for the time possibly spent by a trajectory inside the clouds. For cluster 2, three 1.28 h daytime and three 1.92 h nighttime cloud cycles are repeated every 24 h. For cluster 4, daytime and nighttime cloud durations are 1.28 and 1.68 h, respectively, and are also repeated every 24 h. In order to better identify the impact of cloud droplet chemistry, we have also considered a model scenario without clouds. During the non-cloud period, RH is 70 % from 24 to 96 h. Acronyms for the different model simulations performed in this study can be found in Table 1.
We have also carried out sensitivity runs in this study, investigating the following three aspects: (i) the impact of considered emission data on modeled secondary aerosol concentrations; (ii) the identification of key precursors of C2, Pyr, and ωC2 by relative incremental reactivity (RIR) (Xue et al., 2014); and (iii) the assessment of impact of increased Gly aerosol partitioning on concentrations of Gly, ωC2, and C2. RIR is defined as the ratio of the decrease in the maximum concentrations of the DCRCs and the decrease in the emission data of the corresponding precursor (a 20 % reduction is adopted in this study).
2.3 Chemical and physical model initialization
Zhu et al. (2018) have reported that the pollutant concentrations during the campaign at Mt. Tai were largely controlled by long-range transport. The formation processes of secondary aerosols during long-range transport strongly depend on the emission of precursors. Therefore, emission data passed over in clusters 2 and 4 are implemented in the model. Biogenic emission data (isoprene, α- and β-pinenes) are obtained from Emissions of atmospheric Compounds and Compilation of Ancillary Data (ECCAD) MEGAN-MACC dataset (https://eccad.aeris-data.fr/, last access: 19 September 2019), while other input emission data, i.e., volatile organic compounds (VOCs), including alkanes, alkenes, aromatics, aldehydes, and alcohols; CO; CO2; NH3; NO; NO2; and SO2, are obtained from a new anthropogenic emission inventory in Asia (http://www.meicmodel.org/dataset-mix.html, last access: 15 September 2019) (M. Li et al., 2017). Emission data applied in the model can be found in Table S1 in the Supplement. The deposition velocities used in SPACCIM were taken from Ganzeveld et al. (1998) and are presented in Table S2. Additionally, the multiphase chemistry mechanism is also constrained by the initial concentrations of key species at the corresponding originated areas. The initial chemical data include gas-phase concentrations of inorganic gases (NO, NO2, O3, SO2, HNO3, NH3, H2O2), VOCs (including alkanes, alkenes, aromatics, aldehydes, alcohols and ketones) (Barletta et al., 2005; Duan et al., 2008; An et al., 2009; Liu et al., 2009; He et al., 2010; Ianniello et al., 2011; Meng et al., 2011; Li et al., 2011; Liu et al., 2012b; P. S. Zhao et al., 2013; Y. H. Wang et al., 2014; J. Li et al., 2015; L. Y. Li et al., 2015; Rao, et al., 2016; Y. Wang et al., 2016) and particle-phase data (Hu et al., 2015; Wang et al., 2015; Sun et al., 2013, 2015; Liu et al., 2014). In cases with missing values, values are taken from the CAPRAM urban scenario (http://projects.tropos.de/capram, last access: 15 July 2019, Herrmann et al., 2005). These initial model data and the aerosol parameters are both given in Table S3 and Table S4. The meteorological scenarios are illustrated in Fig. S2.
3.1 Modeled concentrations of important oxidants
Due to the key role of radical and non-radical oxidation in the formation processes of secondary aerosol constituents, their concentration variations and corresponding reasons are investigated. Several publications have already focused on the oxidant budget in China. Kanaya et al. (2009) modeled gas-phase concentrations of OH, HO2 and O3 oxidants at Mt. Tai using the Regional Atmospheric Chemistry Mechanism (RACM), but they did not consider the effect of aqueous-phase conditions, such as cloud cases. Kanaya et al. (2013) only observed O3 gas-phase concentration at Mt. Tai and modeled photochemical O3 production processes by RACM. Suhail et al. (2019) only observed gas-phase NO3 concentration at the Wangdu site in the NCP. Ren et al. (2009) and Y. Wang et al. (2016) reported measurement of gas-phase peroxides at the Mt. Tai and Wangdu site in the NCP, respectively, but no other radical or non-radical oxidants. Liu et al. (2012a) modeled gas-phase concentrations of OH, HO2, and RO2 and investigated ROx budgets and O3 formation in Beijing in the NCP using a 1-D photochemical model. These studies partly investigated the radical or non-radical oxidants and lacked investigation of aqueous-phase reactions. To our knowledge, this is the first detailed multiphase chemical modeling study examining radical and non-radical oxidant concentrations along the trajectory to the Mt. Tai under day vs. night and cloud vs. non-cloud cases.
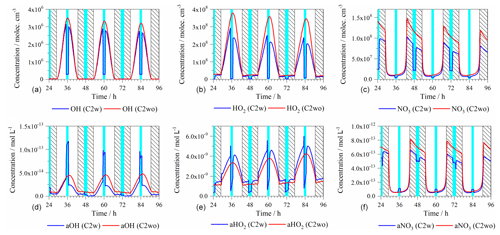
Figure 1Time series of the modeled gas-phase (a, b, c: molec. cm−3) and aqueous-phase (d, e, f: mol L−1) radical oxidant concentrations of the C2w and C2wo cases, respectively (light blue column: cloud; shadow: night; a: aqueous phase). For acronyms, see Table 1.
3.1.1 Key radical oxidants
Figure 1 shows the modeled gas- and aqueous-phase concentrations of important radical oxidants in the C2w and C2wo cases. The gas- and aqueous-phase OH, HO2 and NO3 radical concentrations and the corresponding time profiles in the C4w and C4wo cases are quite similar to those in the C2w and C2wo cases. Therefore, the corresponding plots of the C4w and C4wo cases are presented in Fig. S3.
Due to photochemistry, the gas-phase OH and HO2 oxidants showed a distinct diurnal profile, but they are significantly influenced by cloud occurrences. Under daytime cloud droplet conditions, OH and HO2 concentrations in the gas phase decreased by about 90 % and 98 %, respectively. The reduction of gas-phase OH radical concentrations in daytime cloud is mainly caused by the reduction of the gas-phase formation pathway of the HO2+NO reaction. Due to high water solubility, a direct phase transfer of HO2 into cloud droplets contributed significantly to its gas-phase concentration reduction.
The NO3 radical exhibited higher gas-phase concentrations during the night than during the day. The levels are also substantially lower during in-cloud conditions. Under nighttime cloud droplet conditions, gas-phase NO3 concentrations decreased by about 72 %. The decreased NO3 radical concentration mainly resulted from the increased N2O5 uptake during in-cloud conditions.
Unfortunately, we did not perform measurements of key radicals during the campaign. However, the simulated maxima of the gas-phase concentrations of OH (C2w: 3.2×106; C2wo: 3.5×106 molec. cm−3) and HO2 (C2w: 2.9×108; C2wo: 3.8×108 molec. cm−3) for Mt. Tai in this study are discussed in comparison to both the measurements and previous modeling studies. Compared with the modeled maximum OH (6.0×106 molec. cm−3) and HO2 (7.0×108 molec. cm−3) concentrations at Mt. Tai in June 2006 taken using a photochemical box model that was based on the Regional Atmospheric Chemistry Mechanism (RACM) (Kanaya et al., 2009), the OH and HO2 concentrations reported here are only slightly lower. Moreover, the modeled OH and HO2 concentrations in this study are lower than those of simulated results over the Chinese megacity of Beijing in August 2007 using a 1-D photochemical model (Regional chEmical and trAnsport Model, REAM-1D), whose chemistry was driven by the standard GEOS-Chem gas-phase chemistry mechanism (OH: 9×106; HO2: 6.8×108 molec. cm−3) (Liu et al., 2012a), and much lower than the measured data by laser-induced fluorescence (LIF) at a rural site downwind of the megacity of Guangzhou, China, on 3–30 July 2006 (OH: 15–26×106; HO2: 3–25×108 molec. cm−3) (Lu et al., 2012). Additionally, the simulated NO3 radical maxima (C2w: 1.0×108; C2wo: 1.5×108 molec. cm−3) are much lower than those observed at the urban site of Shanghai, China, by Differential Optical Absorption Spectroscopy (DOAS) from 15 August to 7 October 2011 (2.5×109 molec. cm−3) (S. Wang et al., 2013). These comparisons suggest that the predicted model data represented a suburban oxidant budget along the trajectory above the boundary layer to Mt. Tai.
Similar to the gas phase, aqueous-phase concentrations of OH and HO2 also display a distinct diurnal profile with peak concentration around noon and are largely impacted by cloud interactions. Under daytime cloud droplet conditions, OH aqueous-phase concentrations are increased by a factor of 3, mainly due to the increased direct transfer of OH from the gas phase. Compared with OH concentrations measured in remote clouds from laboratory studies (average: mol L−1; Arakaki et al., 2013), the modeled average aqueous-phase OH concentration in daytime clouds ( mol L−1) is much higher. The difference between measured and modeled OH concentrations is comprehensively discussed in Tilgner and Herrmann (2018). The chapter outlined that both model results and laboratory investigations of field samples are biased. However, it should be mentioned that a more comprehensive aqueous-phase mechanism tends to lower OH predictions due to the higher number of possible OH sinks. On the other hand, laboratory investigations of field samples most likely tend to underestimate the OH sources due to the limitation of present offline methods. For instance, during the time period on the way from the measurement site to the laboratory, the OH radical can still be consumed by oxidation processes that cannot be resolved by the laboratory protocol, and OH sources related to the uptake of OH precursors (H2O2, ROOHs, etc.) are also excluded. Therefore, an adequate comparison is rather difficult at present. On the other hand, HO2 aqueous-phase concentrations are decreased by a factor of 0.9 due to aqueous-phase reactions of HO2 with Cu2+ or Cu+. However, higher HO2 aqueous-phase concentrations appear at the beginning of the daytime cloud. This is caused by the increased H2O2 aqueous concentrations due to uptake at the beginning of the daytime cloud period. In the aqueous-phase, H2O2 reactions with Mn3+ or FeO2+ led to a formation of HO2.
Table 2Average aqueous-phase concentrations (mol L−1) of modeled radical and non-radical oxidants in different simulations.
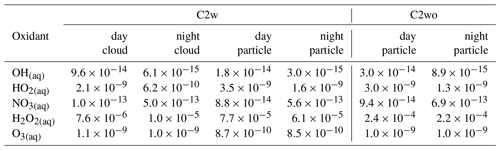
The NO3 radical presents higher aqueous-phase concentrations during the night, with a minor cloud impact. Under nighttime in-cloud conditions, the NO3 radical concentration decreases by about 13 %. In Table 2, average aqueous-phase concentrations of OH, HO2 and NO3 under different conditions are presented. Aqueous-phase NO3 concentrations are much higher than those of aqueous-phase OH. Considering the normally lower reaction rate constant of the aqueous NO3 radical compared to aqueous OH (Herrmann et al., 2010, 2015; Ng et al., 2017), both OH and NO3 radicals are able to initiate aqueous-phase oxidations under the simulated conditions, contributing to the chemical processing of SOA constituents.
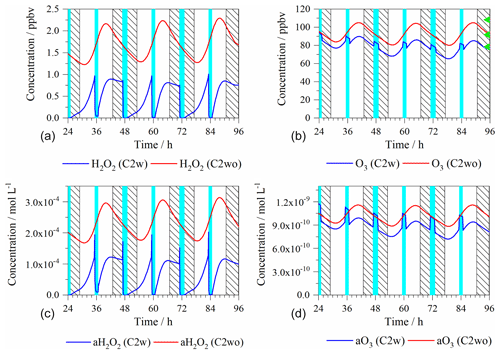
Figure 2Time series of modeled gas-phase (a, b ppbv) and aqueous-phase (c, d mol L−1) non-radical oxidant concentrations in the C2w and C2wo cases. Light blue column: cloud; shadow: night; a: aqueous phase; green triangle: the maximum (above), average (middle), and minimum (below) value of measured concentration at Mt. Tai.
3.1.2 Key non-radical oxidants
Figure 2 depicts the modeled gas- and aqueous-phase concentrations of H2O2 and O3 for the C2w and C2wo cases. The corresponding plots for the C4w and C4wo cases can be found in the Supplement because of their similar concentration levels and patterns to C2w and C2wo, respectively (Fig. S4).
Figure 2 shows that, due to active photochemistry, gas-phase concentrations of H2O2 and O3 mainly increased during the day and decreased during the night. During cloud periods, H2O2 gas-phase concentrations are decreased by 96 % due to direct phase transfer and corresponding aqueous-phase chemistry. The predicted cloud impact is minor for gas-phase O3 concentrations, which is consistent with former studies (Tilgner et al., 2013). During daytime cloud periods, O3 gas-phase concentrations are decreased by about 4 %. In the C2wo case, H2O2 concentrations are substantially higher than those in C2w because of the missing cloud-phase sinks (e.g., hydrogen sulfide oxidation). However, O3 concentrations in C2wo and C2w are similar because of the minor cloud impact on O3 in C2w.
In the C2wo case, measured gas-phase O3 concentrations at Mt. Tai ranged from 78.6 to 108.3 ppbv (Fig. 2), which is typical in a Chinese suburban regime (Y. Wang et al., 2013). However, these concentrations are reached even at the high altitude of Mt. Tai. Additionally, the simulated maxima gas-phase H2O2 concentrations (C2w: 1.0; C2wo: 2.3 ppbv) are lower than those observed at a rural site downwind of the more polluted area of Hebei, China (11.3 ppbv) (Y. Wang et al., 2016). The simulated O3 maxima (C2w: 94.2; C2wo: 105.1 ppbv) are slightly lower than those observed at the Nanjing urban area in China (133.9 ppbv) (An et al., 2015).
The aqueous-phase H2O2 presents a similar concentration pattern as the gas-phase H2O2. Higher H2O2 aqueous-phase concentrations at the beginning of the daytime cloud are caused by the fast phase transfer. The subsequent sharp decrease during the first minute is connected to the efficient hydrogen sulfide oxidation. In the C2wo case, aqueous-phase O3 concentrations increase during the day and decrease throughout the night. In both daytime and nighttime clouds, O3 aqueous-phase concentrations are increased by about 18 %. The average aqueous-phase concentrations of H2O2 and O3 in the different cases can be found in Table 2.
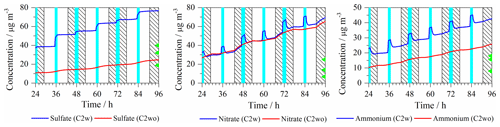
Figure 3Time series of the modeled aerosol mass concentrations (µg m−3) of key secondary inorganic aerosol constituents (sulfate, nitrate and ammonium) in the C2w and C2wo cases. Light blue column: cloud; shadow: night; green triangle: the maximum (above), average (middle), and minimum (below) value of measured concentration at Mt. Tai.
3.2 Modeled concentrations and formation of key secondary inorganic aerosol constituents
In Fig. 3, modeled concentrations of the most important SIA constituents are plotted, including (i) sulfate (sum of all sulfur compounds with oxidation state +6, mainly ), (ii) nitrate (sum of all nitrogen compounds with oxidation state +5, mainly ) and (iii) ammonium (sum of all nitrogen compounds with oxidation state −3, mainly ). The corresponding plots for the C4w and C4wo cases are presented in Fig. S5.
3.2.1 Sulfate
Conducted field observations, together with estimated sulfur oxidation rates using a tracer method in previous studies at Mt. Tai, have suggested that sulfate formation is closely related to cloud chemistry (Zhou et al., 2009; Shen et al., 2012; Guo et al., 2012). However, these studies are not able to comprehensively quantify the impact of cloud chemistry on sulfate concentration and have not performed detailed investigations on chemical formation pathways of sulfate during the transport to Mt. Tai. In this study, we primarily present modeled concentrations of sulfate and discuss the differences between the different day vs. night and cloud vs. non-cloud cases using a multiphase chemistry model. Moreover, findings of sulfate source and sink chemical reactions are presented for the different model cases.
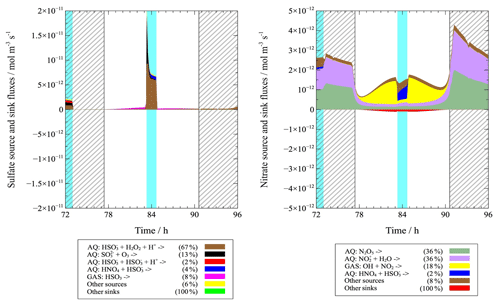
Figure 4Modeled multiphase (gas + aqueous phase) source and sink fluxes of sulfate and nitrate (light blue column: cloud; shadow: night). Percentage is given for the third model day.
Figure 3 shows that sulfate concentrations mainly increase under in-cloud conditions throughout the whole simulation due to active in-cloud chemical sulfur oxidation pathways. Although in-cloud residence time is slightly higher during the night, sulfate concentrations increase more in the daytime clouds (35 %) than the nighttime clouds (15 %) because of the increased aqueous reaction of with H2O2 in daytime cloud droplets (Fig. 4). As shown in Fig. 4, about 97 % of sulfate formation fluxes are related to in-cloud conditions, and they mostly occurred in daytime clouds. The aqueous-phase reaction of with H2O2 represents the most important sulfate source in daytime cloud with a contribution of 78 %. In the nighttime cloud, aqueous-phase reaction of with H2O2 (42 %) and aqueous reaction of bisulfite with O3 (28 %) are dominant pathways for sulfate formation.
In the C2wo case, sulfate concentrations gradually increase over time (Fig. 3). The highest increase occurs during the day as a consequence of the gas-phase SO2 oxidation by OH (Fig. S6). However, the formed sulfate mass in the C2wo case is substantially lower than in the C2w case. At the end of the simulation, the modeled sulfate concentrations are 76.7 and 24.7 µg m−3 in the C2w and C2wo cases, respectively. Compared to the maximum (40.0 µg m−3), average (32.0 µg m−3) and minimum (18.8 µg m−3) values of the measured sulfate concentrations at Mt. Tai (Fig. 3), SPACCIM overestimates measured concentrations of sulfate in the C2w case but slightly underestimates the mean sulfate concentration in the C2wo case.
3.2.2 Nitrate
Studies at Mt. Tai focused on nitrate suggested that photochemical formation of HNO3 has important contribution to nitrate formation (Zhou et al., 2009; Guo et al., 2012). Wen et al. (2018) found that partitioning of HNO3 from gas to particulate phase and hydrolysis of N2O5 is the predominant daytime and nighttime formation pathway of nitrate at Mt. Tai, respectively. However, these studies did not consider nitrate formation processes related to in-cloud conditions. In this study, we not only focus on the concentration time profiles of nitrate under day vs. night but also compare cloud vs. non-cloud cases. Furthermore, we have analyzed nitrate source and sink reaction rates and their contributions in different cases.
As can be seen in Fig. 3, nitrate concentrations are increased throughout the simulation. Under in-cloud conditions, nitrate concentrations are increased by about 10 % and 24 % during the day and the night, respectively. The concentration time profiles in the C2w and C2wo cases show only small differences, indicating that most of the nitrate formation occurs during non-cloud periods. Therefore, the end concentrations of C2w and C2wo do not differ significantly. An analysis of chemical sink and source in the C2w case (Fig. 4) has revealed that nitrate is mainly produced by aqueous-phase N2O5 hydrolysis during the night. During the day, nitrate is mainly produced by the aqueous-phase reaction of HNO4 and , gas-phase reaction of OH and NO2, and aqueous-phase N2O5 hydrolysis.
A comparison of daytime and nighttime fluxes in the C2wo case has revealed that 31 % and 69 % of nitrate formation fluxes occur during the day and at night, respectively. In the C2wo case, nighttime nitrate is mainly produced by aqueous-phase N2O5 hydrolysis with a contribution of 92 % (Fig. S6), which agrees with the result in Wen et al. (2018). However, gas-phase reaction of OH+NO2 to gaseous HNO3 and further partitioning into the aerosols is the most important source for daytime nitrate, with a contribution of 56 % (Fig. S6). Aqueous-phase N2O5 hydrolysis is also significant for daytime nitrate formation with a contribution of 30 %. In comparison, Wen et al. (2018) have suggested the partitioning of HNO3 from gas to the particulate phase to be the most important formation pathway for daytime nitrate with a contribution of 94 %. The N2O5 hydrolysis has been identified as the second most important pathway with a contribution of 4 %–6 %.
The modeled nitrate concentrations are 69.5 and 65.3 µg m−3 at 96 h in the C2w and C2wo cases, respectively. Compared to the maximum (25.0 µg m−3), average (14.0 µg m−3) and minimum (6.8 µg m−3) values of the measured nitrate concentrations at Mt. Tai (Fig. 3), the model simulations overestimate the measured concentrations in both cases. Potential reasons are discussed in Sect. 3.3.2.
3.2.3 Ammonium
Measured ammonium concentrations at Mt. Tai can be strongly impacted by acidification and cloud chemistry (Guo et al., 2012; J. Li et al., 2017). Still, a detailed analysis of the occurring processes is missing. Therefore, we provide a detailed insight into the ammonium concentration variation trends and the impact of acidification and cloud processing along the simulated trajectories towards Mt. Tai.
Similar to sulfate and nitrate, ammonium concentrations also gradually increased throughout the simulation due to the included emissions rates and the followed uptake of gaseous NH3 into the aqueous phase. Ammonium concentrations rose in cloud droplets both during the day and night because a more efficient uptake into the larger cloud water volume. After cloud evaporation, ammonium aerosol concentrations are increased due to stronger partitioning into more acidified deliquesced particles. In the C2wo case, ammonium concentrations are also increased during both the day and night. However, modeled aerosol mass of ammonium in the C2wo case is lower than that in the simulation case with cloud interaction (C2w case). In the C2wo case, less sulfate is formed. Consequently, deliquesced aerosol particles are less acidified and a larger fraction of ammonium stays in the gas phase as gaseous NH3. The simulated ammonium concentrations after 96 h are 42.7 and 25.9 µg m−3 in the C2w and C2wo cases, respectively, which are higher than the measured concentrations (maximum: 18.0, average: 15.6, minimum: 7.9 µg m−3).
The differences between the modeled and measured concentrations of sulfate, nitrate and ammonium can be attributed to several issues such as (i) the indefiniteness of the input emission data, (ii) the initial concentrations, (iii) the missing entrainment–detrainment processes and (iv) the performed heating of the inlet during the sampling of wet aerosol (see Sect. 3.3.2 for further details).
3.3 Modeled concentrations of DCRCs and comparison with field data
In recent years, a number of field observations on DCRCs have been conducted in the NCP. For example, He et al. (2013), Ho et al. (2015), Zhao et al. (2018) and Yu et al. (2019) observed DCRCs in Beijing, and Wang et al. (2009), Kawamura et al. (2013), Meng et al. (2018) and Zhao et al. (2019) measured DCRCs at Mt. Tai. Our field observation about DCRCs at Mt. Tai has been reported in Zhu et al. (2018). However, these studies are focused on DCRC concentrations, molecular compositions, temporal variations, size distributions, source implications and stable carbon isotopic composition. They have not investigated the chemical formation of DCRC concentrations along the trajectory or the impact of in-cloud and non-cloudy conditions on DCRC concentrations. To our knowledge, a multiphase chemical model study investigating the DCRCs concentration variations and their chemical processing along the trajectory to Mt. Tai considering day vs. night and cloud vs. non-cloud cases has not been yet reported.
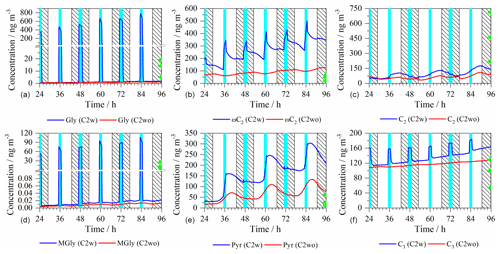
Figure 5Time series of the modeled aerosol mass concentrations of selected DCRCs (a, b, c Gly, ωC2, and C2; d, e, f MGly, Pyr, and C3) in the C2w and C2wo cases. Light blue column: cloud; shadow: night; green triangle: the maximum (above), average (middle), and minimum (below) value of measured concentrations at Mt. Tai.
3.3.1 Modeled concentrations of DCRCs
Figure 5 shows the modeled aerosol mass concentrations of Gly, ωC2, C2, methylglyoxal (MGly), Pyr and malonic acid (C3), both in the C2w and C2wo cases and the values measured at Mt. Tai. In the C4w and C4wo cases, the modeled Gly, ωC2, C2, MGly, Pyr and C3 concentrations show similar patterns to those in the C2w and C2wo cases, respectively (Fig. S8).
Dicarbonyl compounds
In the C2w case, Gly and MGly concentration patterns show a substantial uptake into cloud droplets. Gly concentrations decreased during the daytime and nighttime cloud droplet periods due to in-cloud oxidation processes. On the other hand, MGly concentrations display a decrease in the daytime cloud droplets but an increase under nighttime in-cloud conditions. This might have been caused by the fact that the aqueous oxidation fluxes under nighttime in-cloud conditions are lower than the ones under daytime. This might have been caused by the fact that the aqueous oxidation fluxes under nighttime in-cloud conditions are lower than the ones under daytime conditions because of the much lower OH radical concentrations under nighttime in-cloud conditions (Fig. S3). In the C2wo case, Gly and MGly concentrations are very low due to the low partitioning towards aqueous particles that has been predicted by the model. The effect of a potentially higher partitioning constant of Gly (Volkamer et al., 2009; Ip et al., 2009) is investigated in Sect. 3.5.3. It is worth noting that Gly or MGly have similar concentrations at the end of the simulation with or without cloud chemistry.
C2 carboxylic acids
In the C2w case, aqueous-phase concentrations of ωC2 are increased under both daytime and nighttime in-cloud conditions and early in the night of non-cloud periods. On the other hand, ωC2 concentrations are lowered during the day and later in the night under non-cloudy conditions. In the C2wo case, ωC2 is decreased during the morning periods but is increased in the late afternoon and at night. Compared with the C2wo case, the C2w case shows higher ωC2 concentrations, which suggests that cloud processes play important roles in ωC2 formation and oxidation.
In the C2w case, modeled aqueous-phase concentrations of C2 are increased under daytime in-cloud conditions and daytime aqueous particle conditions but are lowered during nighttime cloud periods and under nighttime aqueous particle conditions. In the C2wo case, C2 is increased during the day but is decreased during the night.
C3 carboxylic acids
In the C2w case, Pyr concentrations are raised during the daytime and nighttime in-cloud conditions as well as in the late mornings of the non-cloud periods. Pyr concentrations are decreased in the early morning, afternoon and nighttime non-cloudy conditions (caused by the efficient degradation from the reaction with aqueous-phase H2O2). In the C2wo case, aqueous-phase concentration profile of Pyr is characterized by an increase during the morning and early afternoon period and by a strong decrease during the late afternoon and night. Pyr shows a high correlation with aqueous-phase H2O2 in the C2wo case due to its efficient H2O2 decay (Fig. S7).
The aqueous-phase C3 concentrations are increased during cloud formation due to the uptake of gaseous C3 into cloud droplets. Moreover, C3 concentrations are increased during non-cloud periods. In the C2wo case, C3 concentrations only increased slightly during the night and even less so during the day. In comparison to the C2wo case, the C2w case shows higher concentrations of C3, indicating that cloud oxidation processes are very important for C3 aqueous-phase formation under the simulated conditions. The production of C3 is 27 % higher in the C2w case than in the C2wo case.
3.3.2 Comparison to field observations
The ratios of the average concentration of modeled and measured DCRCs can be found in Table 3. The results show that model predictions are higher than the measured concentrations of C3, Pyr and ωC2 in both the C2w and C2wo cases. Moreover, the concentration ratios of Pyr and ωC2 in the C2w case are much higher than in the C2wo case. On the other hand, model results underpredict the C2, Gly and MGly concentrations in both cases.
SPACCIM overestimates the measured ωC2 concentrations but underestimates the measured C2 ones, suggesting the conversion of ωC2 is implemented less efficiently into CAPRAM than is seen in the field. Phase partitioning between gas and aqueous phase, a key process for modeled MGly concentration, may be not sufficient to predict the measured MGly aerosol concentrations in the field because of model simplicity. Kroll et al. (2017) have found that a possible hydration of MGly in the gas phase might lead to an enhanced uptake into aqueous particles. Thus, the MGly uptake may be underestimated. Additionally, other important processes that can effectively enhance partitioning of MGly are not yet considered, such as reversible oligomerizations (Ervens and Volkamer, 2010). As a result, the modeled aqueous-phase MGly concentration is rather low and about 3 orders of magnitude lower than the measured data. Based on this finding, a sensitivity study has been performed (see Sect. 3.5 for details).
Table 3Ratios of the concentrations of the modeled and measured DCRC compounds in the different model trajectories at Mt. Tai.
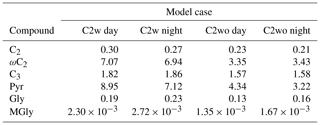
The overestimation and underestimation of the measured concentrations of inorganic and organic aerosol constituents could have the following reasons.
-
Input data. Indefiniteness of emission data and initial concentrations. The emission data are obtained through a new anthropogenic emission inventory in Asia, which provides monthly emissions in 2010 by sector at resolution. However, the model simulation period in this study is in 2014, and the spatial resolution is less than . Therefore, errors in conversion of the emission data can occur. The height of Mt. Tai (about 1500 m) also causes its input to be indefinite. Furthermore, initial concentrations in the areas of origin are obtained through related references rather than field measurements, which also leads to indefiniteness.
-
Field measurement. PM2.5 samples are the only ones available, so a possible contribution of larger particles might have been missed. Moreover, heating the inlet during the sampling of wet aerosol has definitely lowered the measured concentrations of more volatile compounds such as ammonium nitrate.
-
MCM. Some species' crucial gaseous precursors are efficiently destroyed by MCM. For example, it is recommended that gas-phase oxidation of acetic acid by OH proceed via both an H atom abstraction from the OH group and the CH3 group (Sun et al., 2009). Nonetheless, only an H abstraction for the OH group is implemented in the MCM. This oxidation scheme is implemented for all carboxylic acids. The disadvantage of the MCM probably leads to an underestimation of the functionalized acids and need to be resolved in more detail. However, the development of an improved gas-phase acid oxidation scheme for the MCM goes beyond the scope of this study.
-
CAPRAM. Missing sources of selected DCRCs from higher organic chemistry, such as the aqueous-phase chemistry of aromatic compounds (Hoffmann et al., 2018).
-
SPACCIM. The model neglects nonideal solution effects and do not consider organic salt formation. These factors possibly result in overestimated or underestimated results. Recent studies by Rusumdar et al. (2020) have demonstrated that nonideal chemistry treatment led to much higher concentrations of C2 and ωC2.
Apart from MGly, the concentration ratios of the modeled and measured species ranged from 0.1 to 8.3. Interestingly, the ratio of C3 is close to 1, which might be related to a good representation of the concentrations of C3 precursors. The comparison indicates that formation pathways of DCRCs implemented in CAPRAM4.0 are realistic but highly dependent on the input data of precursors. SPACCIM, with MCM3.2 and CAPRAM4.0 implemented, is a numerical tool that can help us better understand the complexity of the multiphase processing of DCRCs . However, the present study also demonstrates that there are still huge gaps in knowledge about the formation and degradation of secondary aerosol constituents. Hence, further laboratory investigations and modeling work are necessary.
3.4 Model investigations of chemical sources and sinks of selected DCRCs
Although field observations have speculated about several potential formation pathways of some DCRCs species by correlations or ratios analyses (Hegde and Kawamura, 2012; Kawamura et al., 2013; Zhao et al., 2019), the detailed pathways of DCRCs need to be studied.
Multiphase model simulations are a suitable tool to investigate DCRC formation processes. In recent years, DCRC formation processes have been examined by several model studies. For example, Tilgner and Herrmann (2010) have modeled gas- and aqueous-phase processing of C2–C4 carbonyl compounds and carboxylic acids by SPACCIM; Ervens et al. (2004) have discussed formation pathways of Pyr and C2–C6 dicarboxylic acids in gas and aqueous phases; Lim et al. (2005) have reported the formation pathways of Gly, MGly, Pyr, and C2 by isoprene oxidation in gas and aqueous phases using a photochemical box model; and Huisman et al. (2011) have investigated the driving processes of Gly chemistry using the Master Chemical Mechanism (MCM, v3.1). These studies have suggested that DCRC formations are related to the oxidations of anthropogenic (e.g., toluene and ethylene) and biogenic (e.g., isoprene) gas-phase VOC precursors. The emissions of these anthropogenic and biogenic VOC in China are much higher than those reported in above references (Sindelarova et al., 2014; Bauwens et al., 2016). However, multiphase model simulations are sparsely used to study DCRC formations in China. Therefore, the present study aims to undertake a detailed investigation of the formation pathways of selected DCRCs under day vs. night and cloud vs. non-cloud cases along the trajectories approaching Mt. Tai.
Due to the similar concentration levels and corresponding variation trends of ωC2, C2, Pyr and C3 in clusters 2 and 4, the source and sink flux analyses are only presented and discussed for the C2 case. Additionally, the corresponding plots of the four compounds in the C2wo case are presented in the Supplement (Fig. S9).
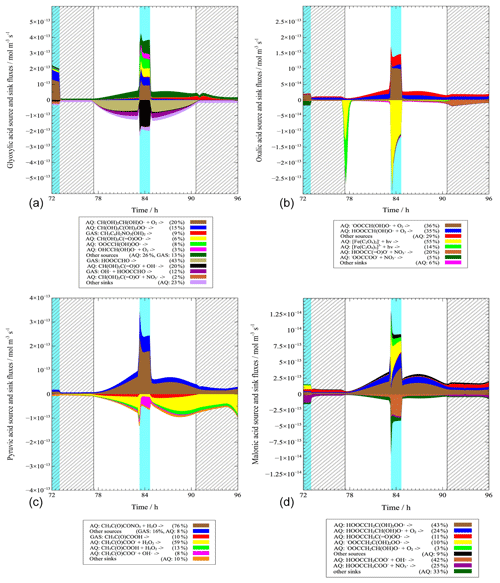
Figure 6Modeled multiphase (gas phase + aqueous phase) source and sink fluxes of ωC2 (a), C2 (b), Pyr (c) and C3 (d) along the trajectory of the third model day (light blue column: cloud; shadow: night). Percentage is given for the third model day.
3.4.1 Glyoxylic acid (ωC2)
In Fig. 6, the multiphase source and sink fluxes of ωC2 (C2w case) on the third model day are plotted. The results reveal a net formation flux that mainly occurs during in-cloud conditions as well as a net degradation that mainly occurs during non-cloud periods. About 94 % of the net formation flux of ωC2 is simulated under in-cloud conditions. However, non-cloudy conditions represent 99 % of the net sink flux of ωC2.
Under daytime and nighttime in-cloud conditions, the major formation pathways of ωC2 are aqueous-phase reactions of hydrated Gly with the OH and radical (contribution of 60 % during the day and 86 % during the night), which is similar to the results in Tilgner and Herrmann (2010), and Tilgner et al. (2013). The aqueous-phase oxidation of glycolate by OH is also significant under daytime in-cloud conditions with a contribution of 18 %. Under daytime and nighttime non-cloudy conditions, aqueous reactions of hydrated Gly (day: 20 %; night: 20 %) and gas-phase decay of 3-methyl-6-nitrocatechol (day: 14 %; night: 24 %) are significant for ωC2 formation. Other reactions contributed less than 5 % to the overall source flux.
Under daytime clouds, ωC2 sink is dominated by aqueous-phase reaction of hydrated glyoxylate with OH (contribution of 88 %), which is consistent with Ervens et al. (2004). During nighttime clouds, however, aqueous-phase reactions of hydrated glyoxylate with NO3 (45 %) and OH (28 %) are the most important sinks. In contrast to those under in-cloud conditions, gas-phase ωC2 photolysis (57 %) and gas-phase reaction of ωC2 with OH (18 %) act as key sinks of ωC2 under daytime non-cloudy conditions. Under nighttime non-cloudy conditions, the sink fluxes of ωC2 are low and therefore unimportant.
3.4.2 Oxalic acid (C2)
Figure 6 also depicts the source and sink fluxes of C2 in the C2w case. The model simulated a net formation flux during the non-cloud periods and a net degradation in the early morning hours when non-clouds are present but the photolysis of iron oxalate complexes is efficient. A net formation of about 94 % C2 is simulated under non-cloudy conditions. About 74 % of the net C2 sink fluxes are predicted during the early morning non-cloud period, and 26 % are related to the cloud oxidation fluxes.
The most important source of C2 in the aqueous phase under in-cloud conditions is aqueous oxidations of hydrated glyoxylate with the OH radical, which agrees with other model results (Tilgner and Herrmann, 2010; Ervens et al., 2004). This formation pathway contributes to C2 formation with about 72 % during the day and 87 % during the night. In contrast to that under in-cloud conditions, the most important C2 formation pathway during daytime and nighttime non-cloudy conditions is the aqueous-phase oxidation of hydrated ωC2 with the OH radical (day: 39 %; night: 52 %). The field observations also suggested that aqueous-phase oxidation of ωC2 is the most important formation pathway of C2 (Kundu et al., 2010; Kawamura et al., 2013; He and Kawamura, 2010), but they are not able to quantify contribution and the responsible specific oxidation pathways. Other reactions contribute less than 5 % to the overall source flux.
The most important sink of C2 under daytime in-cloud conditions is the photolysis of iron oxalate complexes, with a contribution of about 93 %. The result is similar to reported laboratory experiment findings (Pavuluri and Kawamura, 2012) and aqueous model simulations (Tilgner and Herrmann, 2010). On the other hand, aqueous reaction of oxalate with NO3 (80 %) is the most important sink in the nighttime cloud case. Under nighttime non-cloudy conditions, C2 sink is dominated by the reaction of the oxalate monoanion with NO3 (81 %). Under daytime non-cloudy conditions, the only significant C2 sink is the photolysis of iron oxalate complexes. However, photolysis of iron oxalate complexes under aqueous particle conditions is most likely overestimated in the present SPACCIM studies. A recent study by Rusumdar et al. (2020), using an advanced SPACCIM version with a nonideal treatment but which has a more reduced chemical CAPRAM scheme, revealed that the formation and consequently the photolysis of iron oxalate complexes is inhibited under aqueous particle conditions. The possible overestimation of the photolytic decay leads to a significantly low C2 concentration and thus might be one of the reasons for the underestimated C2 concentration.
3.4.3 Pyruvic acid (Pyr)
The modeled source and sink fluxes of Pyr in the C2w case on the third model day can be found in Fig. 6. A net formation flux is modeled mainly under in-cloud conditions, especially during the day, along with a net degradation during non-cloud periods. About 72 % of the net Pyr flux occurs in clouds, whereas 28 % is formed under non-cloudy conditions. However, 100 % of the multiphase Pyr net sink fluxes are related to non-cloud oxidation.
Under in-cloud conditions, the dominant source for Pyr is hydrolysis of the aqueous-phase oxidation product of nitro-2-oxopropanoate, with a contribution of 89 % during the day and 70 % during the night. The result is different from former model studies, e.g., Ervens et al. (2004), Lim et al. (2005), Tilgner and Herrmann (2010) and Tilgner et al. (2013), which modeled the aqueous oxidations of MGly as the major formation pathway of Pyr. However, these model studies have also modeled different environmental conditions with much lower anthropogenic pollution, including lower NOx and NO3 radical budgets compared to the Chinese conditions. Furthermore, the former studies have also used rather simple gas-phase mechanisms and lack potential production pathways from higher organic compounds. Similarly, the aqueous oxidation of nitro-2-oxopropanoate is identified as a major source under non-cloudy conditions, with a contribution of 87 % during the day and 74 % during the night.
The key sinks of Pyr under daytime in-cloud conditions are aqueous-phase reactions of pyruvate with OH (58 %) and H2O2 (29 %). This finding is consistent with results from laboratory experiments (Carlton et al., 2006). Under nighttime in-cloud conditions, the sink fluxes are very low and therefore can be ignored. Under daytime and nighttime non-cloudy conditions, dominant sinks are aqueous-phase reactions of pyruvate with H2O2 (day: 57 %; night: 72 %) and free Pyr with H2O2 (day: 13 %; night: 15 %). Additionally, gas-phase Pyr photolysis (15 %) is also important under daytime non-cloudy conditions.
3.4.4 Malonic acid (C3)
In Fig. 6, the modeled source and sink fluxes of C3 in the C2w case are presented for the third model day. A net formation flux can be seen under daytime in-cloud conditions and both daytime and nighttime non-cloudy conditions. A net degradation is only found under nighttime in-cloud conditions. A C3 net formation flux is about 82 % under non-cloudy conditions and 18 % under daytime in-cloud conditions.
The major modeled sources of C3 under daytime and nighttime in-cloud conditions are aqueous oxidation reactions of hydrated 3-oxopropanoic acid (day: 48 %; night: 50 %) and hydrated 3-oxopropanoate (day: 45 %; night: 47 %). However, under non-cloudy conditions, aqueous-phase oxidation of hydrated 3-oxopropanoic acid is dominant, with a contribution of 79 % during the day and 88 % during the night.
Differences between the sink fluxes under in-cloud and non-cloudy conditions are modeled. The C3 sinks under daytime clouds are dominated by aqueous-phase reaction of malonate monoanion with OH. Its contribution to total sink flux under the daytime cloud is 70 %. In contrast, the aqueous-phase reaction of malonate monoanion with NO3 is the most important sink under nighttime in-cloud conditions, with a contribution of 75 %. The predominant sink pathway of C3 in daytime cloud is consistent with Ervens et al. (2004), but the sink pathway in nighttime cloud is different due to the missing NO3 radical pathways in their mechanism. The modeled C3 sinks under non-cloudy conditions are much lower than the sinks under in-cloud conditions and thus are unimportant.
3.5 Sensitivity studies
Due to the similarity between clusters 2 and 4, as mentioned above, sensitivity tests are only performed under cluster 2 conditions. The present study investigated the (i) impact of emissions on modeled compound concentrations; the (ii) key precursors of DCRCs; and the (iii) impact of increased Gly aerosol partitioning on Gly, ωC2, and C2.
3.5.1 Impact of emissions
First, sensitivity tests are performed to evaluate the effect of different emission strengths on the concentrations of key secondary inorganic compounds and selected DCRCs during the transport. The emission sensitivities of sulfate, nitrate, ammonium, Gly, ωC2, C2, MGly, Pyr and C3 are investigated by doubling the applied emission fluxes of the base case. The results of the sensitivity tests can be found in Fig. 7. The modeled concentrations of sulfate, nitrate, ammonium, Gly, ωC2, C2, MGly, Pyr and C3 are increased by a factor of about 2 when the emissions doubled, which suggests an almost linear dependency. The results indicate that the modeled concentrations of secondary aerosol are very sensitive to the emissions in the model.
3.5.2 Identification of the key precursors of C2, Pyr and ωC2
Further sensitivity tests are conducted to identify key primary precursors of DCRCs during atmospheric transport. We have adopted the relative incremental reactivity (RIR) method by Carter and Atkinson (1989) for the sensitivity tests. The positive or negative RIR value reveals that reducing precursor emissions would weaken or aggravate DCRC formation, respectively. The RIR method has already been applied in a former study to investigate the precursors of peroxy acetyl nitrate in urban plume in Beijing (Xue et al., 2014).
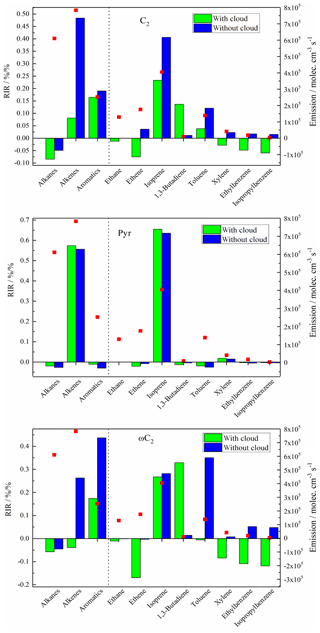
Figure 8The calculated RIRs for C2, Pyr and ωC2 in both the C2w (green bars) and C2wo (blue bars) cases at Mt. Tai (column: RIR values; red dots: emission data).
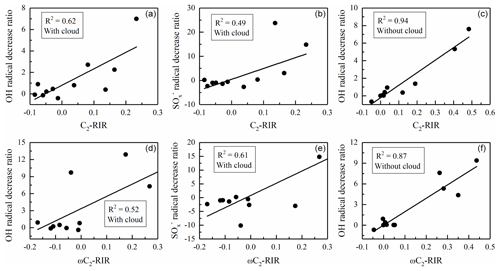
Figure 9Correlations between the decreasing ratios of radical oxidants and C2-RIR (a, b, c) and ωC2-RIR (d, e, f) under C2w and C2wo conditions, respectively.
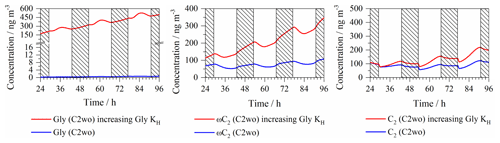
Figure 10Concentration variations in modeled Gly, ωC2 and C2 when increasing the Gly Henry's law constant by 2 orders of magnitude (shadow: night; KH: Henry's law constant).
As can be seen in Fig. 8, C2 formation in the C2w case is mostly sensitive to aromatics and alkenes. Among the aromatic compounds, toluene is the most important one for C2 formation. However, other aromatic species (such as xylene, ethylbenzene, isopropylbenzene) present negative RIRs. Among the alkenes, isoprene and 1,3-butadiene are dominant, but ethene shows negative RIR. The alkane RIRs are all negative. Positive and negative RIRs probably depend on oxidant variations. As shown in Fig. S10, the important sources of C2 in the C2w case are the oxidation of hydrated ωC2 by the OH radical and sulfur-containing radicals (). A reduced concentration ratio of the OH or is calculated in cases with a 20 % decrease in emission data. After the calculation, a correlation with RIR values has been performed. Figure 9 shows that OH and radicals have high and moderate correlations with C2-RIRs in C2w case, respectively, suggesting that the concentration variations in OH and radicals are the reason for the positive and negative C2-RIRs in the C2w case.
In the C2wo case, alkenes account for the highest RIR. The RIR of alkenes is more than 2 times higher than that of the second highest group (aromatic compounds). Among the alkenes, the dominant compound is isoprene. Contrary to the C2w case, 1,3-butadiene reveals very low RIR under C2wo conditions. In the C2wo case, ethene exhibits a positive but low RIR. Among aromatic compounds, toluene shows the highest RIR. Xylene, ethylbenzene and isopropylbenzene also present significantly positive RIR values in the C2wo case. Alkanes again have negative RIRs. As shown in Fig. S10, in the C2wo case, the reactions of dissolved ωC2 with OH radicals represent the major pathways. Strong correlations between C2-RIRs and OH radical in the C2wo case (Fig. 9) indicate that the calculated positive and negative RIRs in the C2wo case are due to OH radical concentration variations.
For Pyr, in both C2w and C2wo cases, alkenes are the dominant precursor group with the largest RIRs. The major compound is isoprene. The absolute RIR values for other selected species are less than 0.05. These results indicated that Pyr formation during atmospheric transport is highly sensitive to isoprene.
In the C2w case, aromatic compounds are the most significant precursors of ωC2 with high positive RIR. However, individual aromatic species listed in Fig. 8 are characterized by negative RIRs. Alkanes and alkenes show negative values. However, isoprene and 1,3-butadiene have positive RIRs, and their high levels suggest that they are key species controlling ωC2 formation during the modeled summer conditions. As shown in Fig. S10, oxidations of dissolved Gly by OH and radicals are the most important sources for ωC2 formation in the C2w case. High correlations between OH and radicals with ωC2-RIR values (Fig. 9) suggest that positive and negative ωC2-RIRs in the C2w case are a result of variations in the two oxidants.
Figure 8 shows that aromatic compounds account for the highest RIR under C2wo conditions and that toluene is a major contributor. Ethylbenzene and isopropylbenzene also made significant contributions. The alkene RIRs are the next highest. Isoprene is the most abundant compound during ωC2 formation. As in the cases of C2 and Pyr, alkanes also have negative ωC2-RIRs. ωC2 production mainly depends on the oxidation of dissolved Gly by OH radical in the C2wo case, and the variation trends of the OH radical are the reason for the positive and negative ωC2-RIRs (see Figs. S10 and 9).
3.5.3 Identifying the impact of increased Gly aerosol partitioning
Phase partitioning between gas and aqueous phase in a multiphase model can be affected, e.g., by salting-in and salting-out effects and other reversible accretion reactions (Herrmann et al., 2015). For example, Ip et al. (2009) and Kampf et al. (2013) have reported that and ammonium sulfate can have a significant effect on the uptake of Gly into an aqueous solution. Therefore, a sensitivity study considering increased Gly aerosol partitioning has been done to evaluate the changes of Gly, ωC2 and C2 concentrations. This has been realized by increasing the CAPRAM Gly Henry's law constant (1.4 mol L−1 atm−1, Betterton and Hoffmann, 1988). An increased Gly Henry's law constant (raised by 2 orders of magnitude) have been applied, which is close to the value reported in Volkamer et al. (2009). As can be seen in Fig. 10, compared to the base C2wo case performed without an increased Gly Henry's law constant, the modeled ωC2 and C2 aerosol concentrations increase by 3 and 2 times, respectively. This result suggested that an increased Gly aerosol partitioning might play an important role in ωC2 and C2 aqueous-phase formation.
The present study focuses on the formation processes of secondary aerosols constituents along trajectories towards Mt. Tai using the multiphase chemistry air parcel model SPACCIM. The modeled radical and non-radical concentrations (e.g., gas-phase OH concentration of 3.2×106 in C2w and 3.5×106 molec. cm−3 in C2wo) suggest that the atmospheric environment of Mt. Tai (∼1.5 km a.m.s.l.) is still characterized by a suburban oxidants budget at the altitude of about 1.5 km. Compared to previous studies at Mt. Tai, this study is the first that investigates the formation processes of secondary aerosols constituents along different trajectories towards Mt. Tai under day vs. night and cloud vs. non-cloudy conditions in detail. The aqueous reaction of with H2O2 has been identified as the major contributor to formation (contribution of 67 % in C2w and 60 % in C2wo). formation is higher during the night than during the day. The major pathways are aqueous-phase N2O5 hydrolysis (contribution of 72 % in C2w and 70 % in C2wo) and gas-phase reaction of OH+NO2 (contribution of 18 % in C2w and 21 % in C2wo). Aqueous-phase reactions of hydrated Gly, hydrated ωC2, nitro-2-oxopropanoate and hydrated 3-oxopropanoic acid are dominant formation pathways of ωC2, C2, Pyr and C3, respectively. Sensitivity tests indicate that isoprene, 1,3-butadiene and toluene are the key precursors of ωC2 and C2. The model data analyses show that isoprene is the predominant precursor for Pyr. When emissions are doubled, the modeled SOA compound concentrations increase by about 2 times, suggesting that gaseous VOC emissions are a driving factor for the modeled SOA compound concentrations. The results indicate the importance of further emission reduction efforts to achieve a better standard of air quality in this part of China.
Additionally, the simulations show that increased Gly aerosol partitioning plays an important role in ωC2 and C2 aqueous-phase formation. Finally, the present study reveals that, in order to better understand the presence, formation, chemical fate and phase partitioning of DCRCs in the troposphere in the future, comprehensive aerosol and cloud field studies, advanced mechanistic laboratory studies, and more chemical processes model studies are necessary. In cases with field investigations, advanced measurements characterizing chemical gas and aerosol compositions with a high time resolution are needed to enable better comparison with and evaluations of present multiphase models. On the other hand, for future model comparisons more advanced models are also required. Those should include a more detailed chemistry description, a detailed treatment of nonideal solution effects and an improved treatment of the phase partitioning of organic compounds, e.g., considering salting-in and salting-out effects and other reversible accretion reactions. Thus, the observed differences between modeled data and measurements could be fixed.
The input data used in the paper are given in the Supplement. The output data of all figures given in this study are publicly available at https://github.com/Yanhong-Zhu/acp-2019-982-data (Zhu, 2020).
The supplement related to this article is available online at: https://doi.org/10.5194/acp-20-6725-2020-supplement.
YZ, AT and HH designed the SPACCIM modeling work. YZ, AT and EHH performed the different SPACCIM simulations. YZ, AT, EHH and HH analyzed the SPACCIM simulation results. YZ and LX performed and interpreted the RIR analysis. YZ, AT, KK, LY and WW compared the model results with field data. YZ, AT, EHH, HH and LX wrote the paper and prepared the manuscript material with contributions from all the co-authors.
The authors declare that they have no conflict of interest.
We thank the European Commission for support of the MARSU project (contract no. 69089). The authors acknowledge the Emissions of atmospheric Compounds and Compilation of Ancillary Data (ECCAD) MEGAN-MACC dataset. The authors also acknowledge the China Scholarship Council for supporting Yanhong Zhu to study in the project at the Atmospheric Chemistry Department (ACD) of the Leibniz Institute for Tropospheric Research (TROPOS), Germany.
This research has been supported by the National Key Research and Development Program of China (grant no. 2016YFC0200500), the National Natural Science Foundation of China (grant nos. 21577079 and 41922051), the Japan Society for the Promotion of Science through Grant-in-Aid (grant no. 24221001), and the European Commission for support of the MARSU project (grant no. 69089).
This paper was edited by Holger Tost and reviewed by two anonymous referees.
An, J., Zou, J., Wang, J., Lin, X., and Zhu, B.: Differences in ozone photochemical characteristics between the megacity Nanjing and its suburban surroundings, Yangtze River Delta, China, Environ. Sci. Pollut. Res., 22, 19607–19617, https://doi.org/10.1007/s11356-015-5177-0, 2015.
An, J. L., Zhang, W., and Qu, Y.: Impacts of a strong cold front on concentrations of HONO, HCHO, O3, and NO2 in the heavy traffic urban area of Beijing, Atmos. Environ., 43, 3454–3459, https://doi.org/10.1016/j.atmosenv.2009.04.052, 2009.
Arakaki, T., Anastasio, C., Kuroki, Y., Nakajima, H., Okada, K., Kotani, Y., Handa, D., Azechi, S., Kimura, T., Tsuhako, A., and Miyagi, Y.: A General Scavenging Rate Constant for Reaction of Hydroxyl Radical with Organic Carbon in Atmospheric Waters, Environ. Sci. Technol., 47, 8196–8203, https://doi.org/10.1021/es401927b, 2013.
Barletta, B., Meinardi, S., Rowland, F. S., Chan, C. Y., Wang, X. M., Zou, S. C., Chan, L. Y., and Blake, D. R.: Volatile organic compounds in 43 Chinese cities, Atmos. Environ., 39, 5979–5990, https://doi.org/10.1016/j.atmosenv.2005.06.029, 2005.
Bauwens, M., Stavrakou, T., Müller, J.-F., De Smedt, I., Van Roozendael, M., van der Werf, G. R., Wiedinmyer, C., Kaiser, J. W., Sindelarova, K., and Guenther, A.: Nine years of global hydrocarbon emissions based on source inversion of OMI formaldehyde observations, Atmos. Chem. Phys., 16, 10133–10158, https://doi.org/10.5194/acp-16-10133-2016, 2016.
Betterton, E. A. and Hoffmann, M. R.: Henry's law constants of some environmentally important aldehydes, Environ. Sci. Technol., 22, 1415–1418, https://doi.org/10.1021/es00177a004, 1988.
Bikkina, S., Kawamura, K., Miyazaki, Y., and Fu, P.: High abundances of oxalic, azelaic, and glyoxylic acids and methylglyoxal in the open ocean with high biological activity: Implication for secondary OA formation from isoprene, Geophys. Res. Lett., 41, 3649–3657, https://doi.org/10.1002/2014GL059913, 2014.
Bräuer, P., Mouchel-Vallon, C., Tilgner, A., Mutzel, A., Böge, O., Rodigast, M., Poulain, L., van Pinxteren, D., Wolke, R., Aumont, B., and Herrmann, H.: Development of a protocol for the auto-generation of explicit aqueous-phase oxidation schemes of organic compounds, Atmos. Chem. Phys., 19, 9209–9239, https://doi.org/10.5194/acp-19-9209-2019, 2019.
Carlton, A. G., Turpin, B. J., Lim, H. J., Altieri, K. E., and Seitzinger, S.: Link between isoprene and secondary organic aerosol (SOA): Pyruvic acid oxidation yields low volatility organic acids in clouds, Geophys. Res. Lett., 33, L06822, https://doi.org/10.1029/2005GL025374, 2006.
Carlton, A. G., Turpin, B. J., Altieri, K. E., Seitzinger, S., Reff, A., Lim, H. J., and Ervens, B.: Atmospheric oxalic acid and SOA production from glyoxal: Results of aqueous photooxidation experiments, Atmos. Environ., 41, 7588–7602, https://doi.org/10.1016/j.atmosenv.2007.05.035, 2007.
Carlton, A. G., Wiedinmyer, C., and Kroll, J. H.: A review of Secondary Organic Aerosol (SOA) formation from isoprene, Atmos. Chem. Phys., 9, 4987–5005, https://doi.org/10.5194/acp-9-4987-2009, 2009.
Carter, W. P. and Atkinson, R.: Computer modeling study of incremental hydrocarbon reactivity, Environ. Sci. Technol., 23, 864–880, https://doi.org/10.1021/es00065a017, 1989.
DeCarlo, P. F., Ulbrich, I. M., Crounse, J., de Foy, B., Dunlea, E. J., Aiken, A. C., Knapp, D., Weinheimer, A. J., Campos, T., Wennberg, P. O., and Jimenez, J. L.: Investigation of the sources and processing of organic aerosol over the Central Mexican Plateau from aircraft measurements during MILAGRO, Atmos. Chem. Phys., 10, 5257–5280, https://doi.org/10.5194/acp-10-5257-2010, 2010.
De Gouw, J. and Jimenez, J. L.: Organic aerosols in the Earth's atmosphere, Environ. Sci. Technol., 43, 7614–7618, https://doi.org/10.1021/es9006004, 2009.
Draxler, R. and Rolph, G.: HYSPLIT (HYbrid Single-Particle Lagrangian Integrated Trajectory) model access via NOAA ARL READY, Silver Spring, Md: NOAA Air Resources Laboratory, available at: http://www.arl.noaa.gov/ready/hysplit4.html (last access: 20 August 2019), 2003.
Duan, F., He, K., Ma, Y., Yang, F., Yu, X., Cadle, S., Chan, T., and Mulawa, P.: Concentration and chemical characteristics of PM2.5 in Beijing, China: 2001–2002, Sci. Total Environ., 355, 264–275, https://doi.org/10.1016/j.scitotenv.2005.03.001, 2006.
Duan, J. C., Tan, J. H., Yang, L., Wu, S., and Hao, J. M.: Concentration, sources and ozone formation potential of volatile organic compounds (VOCs) during ozone episode in Beijing, Atmos. Res., 88, 25–35, https://doi.org/10.1016/j.atmosres.2007.09.004, 2008.
Ervens, B.: Modeling the processing of aerosol and trace gases in clouds and fogs, Chem. Rev., 115, 4157–4198, https://doi.org/10.1021/cr5005887, 2015.
Ervens, B. and Volkamer, R.: Glyoxal processing by aerosol multiphase chemistry: towards a kinetic modeling framework of secondary organic aerosol formation in aqueous particles, Atmos. Chem. Phys., 10, 8219–8244, https://doi.org/10.5194/acp-10-8219-2010, 2010.
Ervens, B., Feingold, G., Frost, G. J., and Kreidenweis, S. M.: A modeling study of aqueous production of dicarboxylic acids: 1. Chemical pathways and speciated organic mass production, J. Geophys. Res.-Atmos., 109, D15205, https://doi.org/10.1029/2003JD004387, 2004.
Ervens, B., Carlton, A. G., Turpin, B. J., Altieri, K. E., Kreidenweis, S. M., and Feingold, G.: Secondary organic aerosol yields from cloud-processing of isoprene oxidation products, Geophys. Res. Lett., 35, L02816, https://doi.org/10.1029/2007GL031828, 2008.
Ervens, B., Turpin, B. J., and Weber, R. J.: Secondary organic aerosol formation in cloud droplets and aqueous particles (aqSOA): a review of laboratory, field and model studies, Atmos. Chem. Phys., 11, 11069–11102, https://doi.org/10.5194/acp-11-11069-2011, 2011.
Ganzeveld, L., Lelieveld, J., and Roelofs, G.: A dry deposition parameterization for sulfur oxides in a chemistry and general circulation model, J. Geophys. Res.-Atmos., 103, 5679–5694, https://doi.org/10.1029/97JD03077, 1998.
Glasius, M., Lahaniati, M., Calogirou, A., Di Bella, D., Jensen, N. R., Hjorth, J., Kotzias, D., and Larsen, B. R.: Carboxylic acids in secondary aerosols from oxidation of cyclic monoterpenes by ozone, Environ. Sci. Technol., 34, 1001–1010, https://doi.org/10.1021/es990445r, 2000.
Guo, J., Wang, Y., Shen, X. H., Wang, Z., Lee, T., Wang, X. F., Li, P. H., Sun, M. H., Collett Jr., J. L., Wang, W. X., and Wang, T.: Characterization of cloud water chemistry at Mount Tai, China: Seasonal variation, anthropogenic impact, and cloud processing, Atmos. Environ., 60, 467–476, https://doi.org/10.1016/j.atmosenv.2012.07.016, 2012.
Guo, S., Hu, M., Wang, Z. B., Slanina, J., and Zhao, Y. L.: Size-resolved aerosol water-soluble ionic compositions in the summer of Beijing: implication of regional secondary formation, Atmos. Chem. Phys., 10, 947–959, https://doi.org/10.5194/acp-10-947-2010, 2010.
He, N. and Kawamura, K.: Distributions and diurnal changes of low molecular weight organic acids and α-dicarbonyls in suburban aerosols collected at Mangshan, North China, Geochem. J., 44, 17–22, https://doi.org/10.2343/geochemj.1.0093, 2010.
He, N., Kawamura, K., Okuzawa, K., Kanaya, Y., and Wang, Z. F.: Diurnal variations of total carbon, dicarboxylic acids, ketoacids and α-dicarbonyls in aerosols in the northern vicinity of Beijing, Atmos. Chem. Phys. Discuss., 13, 16699–16731, https://doi.org/10.5194/acpd-13-16699-2013, 2013.
He, S. Z., Chen, Z. M., Zhang, X., Zhao, Y., Huang, D. M., Zhao, J. N., Zhu, T., Hu, M., and Zeng, L. M.: Measurement of atmospheric hydrogen peroxide and organic peroxides in Beijing before and during the 2008 Olympic Games: Chemical and physical factors influencing their concentrations, J. Geophys. Res., 115, D17307, https://doi.org/10.1029/2009JD013544, 2010.
Hegde, P. and Kawamura, K.: Seasonal variations of water-soluble organic carbon, dicarboxylic acids, ketocarboxylic acids, and α-dicarbonyls in Central Himalayan aerosols, Atmos. Chem. Phys., 12, 6645–6665, https://doi.org/10.5194/acp-12-6645-2012, 2012.
Herrmann, H., Tilgner, A., Barzaghi, P., Majdik, Z., Gligorovski, S., Poulain, L., and Monod, A.: Towards a more detailed description of tropospheric aqueous phase organic chemistry: CAPRAM 3.0, Atmos. Environ., 39, 4351–4363, https://doi.org/10.1016/j.atmosenv.2005.02.016, 2005.
Herrmann, H., Hoffmann, D., Schaefer, T., Bräuer, P., and Tilgner, A.: Tropospheric aqueous-phase free-radical chemistry: Radical sources, spectra, reaction kinetics and prediction tools, Chem. Phys. Chem., 11, 3796–3822, https://doi.org/10.1002/cphc.201000533, 2010.
Herrmann, H., Schaefer, T., Tilgner, A., Styler, S. A., Weller, C., Teich, M., and Otto, T.: Tropospheric aqueous-phase chemistry: kinetics, mechanisms, and its coupling to a changing gas phase, Chem. Rev., 115, 4259–4334, https://doi.org/10.1021/cr500447k, 2015.
Ho, K. F., Huang, R.-J., Kawamura, K., Tachibana, E., Lee, S. C., Ho, S. S. H., Zhu, T., and Tian, L.: Dicarboxylic acids, ketocarboxylic acids, α-dicarbonyls, fatty acids and benzoic acid in PM2.5 aerosol collected during CAREBeijing-2007: an effect of traffic restriction on air quality, Atmos. Chem. Phys., 15, 3111–3123, https://doi.org/10.5194/acp-15-3111-2015, 2015.
Hoffmann, E. H., Tilgner, A., Wolke, R., Böge, O., Walter, A., and Herrmann, H.: Oxidation of substituted aromatic hydrocarbons in the tropospheric aqueous phase: kinetic mechanism development and modelling, Phys. Chem. Chem. Phys., 20, 10960–10977, https://doi.org/10.1039/C7CP08576A, 2018.
Hu, G. Y., Sun, J. Y., Zhang, Y. M., Shen, X. J., and Yang, Y.: Chemical Composition of PM2.5 Based on Two-Year Measurements at an Urban Site in Beijing, Aerosol Air Qual. Res., 15, 1748–1759, https://doi.org/10.4209/aaqr.2014.11.0284, 2015.
Huang, R. J., Zhang, Y., Bozzetti, C., Ho, K. F., Cao, J. J., Han, Y., Daellenbach, K. R., Slowik, J. G., Platt, S. M., and Canonaco, F.: High secondary aerosol contribution to particulate pollution during haze events in China, Nature, 514, 218–222, https://doi.org/10.1038/nature13774, 2014.
Huisman, A. J., Hottle, J. R., Galloway, M. M., DiGangi, J. P., Coens, K. L., Choi, W., Faloona, I. C., Gilman, J. B., Kuster, W. C., de Gouw, J., Bouvier-Brown, N. C., Goldstein, A. H., LaFranchi, B. W., Cohen, R. C., Wolfe, G. M., Thornton, J. A., Docherty, K. S., Farmer, D. K., Cubison, M. J., Jimenez, J. L., Mao, J., Brune, W. H., and Keutsch, F. N.: Photochemical modeling of glyoxal at a rural site: observations and analysis from BEARPEX 2007, Atmos. Chem. Phys., 11, 8883–8897, https://doi.org/10.5194/acp-11-8883-2011, 2011.
Ianniello, A., Spataro, F., Esposito, G., Allegrini, I., Hu, M., and Zhu, T.: Chemical characteristics of inorganic ammonium salts in PM2.5 in the atmosphere of Beijing (China), Atmos. Chem. Phys., 11, 10803–10822, https://doi.org/10.5194/acp-11-10803-2011, 2011.
Ip, H., Huang, X., and Yu, J. Z.: Effective Henry's law constants of glyoxal, glyoxylic acid, and glycolic acid, Geophys. Res. Lett., 36, L01802, https://doi.org/10.1029/2008GL036212, 2009.
Jenkin, M. E., Saunders, S. M., Wagner, V., and Pilling, M. J.: Protocol for the development of the Master Chemical Mechanism, MCM v3 (Part B): tropospheric degradation of aromatic volatile organic compounds, Atmos. Chem. Phys., 3, 181–193, https://doi.org/10.5194/acp-3-181-2003, 2003.
Kampf, C. J., Waxman, E. M., Slowik, J. G., Dommen, J., Pfaffenberger, L., Praplan, A. P., Prevot, A. S., Baltensperger, U., Hoffmann, T., and Volkamer, R.: Effective Henry's law partitioning and the salting constant of glyoxal in aerosols containing sulfate, Environ. Sci. Technol., 47, 4236–4244, https://doi.org/10.1021/es400083d, 2013.
Kanaya, Y., Pochanart, P., Liu, Y., Li, J., Tanimoto, H., Kato, S., Suthawaree, J., Inomata, S., Taketani, F., Okuzawa, K., Kawamura, K., Akimoto, H., and Wang, Z. F.: Rates and regimes of photochemical ozone production over Central East China in June 2006: a box model analysis using comprehensive measurements of ozone precursors, Atmos. Chem. Phys., 9, 7711–7723, https://doi.org/10.5194/acp-9-7711-2009, 2009.
Kanaya, Y., Akimoto, H., Wang, Z.-F., Pochanart, P., Kawamura, K., Liu, Y., Li, J., Komazaki, Y., Irie, H., Pan, X.-L., Taketani, F., Yamaji, K., Tanimoto, H., Inomata, S., Kato, S., Suthawaree, J., Okuzawa, K., Wang, G., Aggarwal, S. G., Fu, P. Q., Wang, T., Gao, J., Wang, Y., and Zhuang, G.: Overview of the Mount Tai Experiment (MTX2006) in central East China in June 2006: studies of significant regional air pollution, Atmos. Chem. Phys., 13, 8265–8283, https://doi.org/10.5194/acp-13-8265-2013, 2013.
Kawamura, K. and Sakaguchi, F.: Molecular distributions of water soluble dicarboxylic acids in marine aerosols over the Pacific Ocean including tropics, J. Geophys. Res.-Atmos., 104, 3501–3509, https://doi.org/10.1029/1998JD100041, 1999.
Kawamura, K. and Yasui, O.: Diurnal changes in the distribution of dicarboxylic acids, ketocarboxylic acids and dicarbonyls in the urban Tokyo atmosphere, Atmos. Environ., 39, 1945–1960, https://doi.org/10.1016/j.atmosenv.2004.12.014, 2005.
Kawamura, K., Tachibana, E., Okuzawa, K., Aggarwal, S. G., Kanaya, Y., and Wang, Z. F.: High abundances of water-soluble dicarboxylic acids, ketocarboxylic acids and α-dicarbonyls in the mountaintop aerosols over the North China Plain during wheat burning season, Atmos. Chem. Phys., 13, 8285–8302, https://doi.org/10.5194/acp-13-8285-2013, 2013.
Kim, H. S., Huh, J. B., Hopke, P. K., Holsen, T. M., and Yi, S. M.: Characteristics of the major chemical constituents of PM2.5 and smog events in Seoul, Korea in 2003 and 2004, Atmos. Environ., 41, 6762–6770, https://doi.org/10.1016/j.atmosenv.2007.04.060, 2007.
Kroll, J. A., Hansen, A. S., Møller, K. H., Axson, J. L., Kjaergaard, H. G., and Vaida, V.: Ultraviolet Spectroscopy of the Gas Phase Hydration of Methylglyoxal, ACS Earth Space Chem., 1, 345–352, https://doi.org/10.1021/acsearthspacechem.7b00054, 2017.
Kundu, S., Kawamura, K., and Lee, M.: Seasonal variations of diacids, ketoacids, and α-dicarbonyls in aerosols at Gosan, Jeju Island, South Korea: Implications for sources, formation, and degradation during long-range transport, J. Geophys. Res.-Atmos., 115, D19307, https://doi.org/10.1029/2010JD013973, 2010.
Legrand, M., Preunkert, S., Oliveira, T., Pio, C. A., Hammer, S., Gelencsér, A., Kasper-Giebl, A., and Laj, P.: Origin of C2-C5 dicarboxylic acids in the European atmosphere inferred from year-round aerosol study conducted at a west-east transect, J. Geophys. Res.-Atmos., 112, D23S07, https://doi.org/10.1029/2006JD008019, 2007.
Li, C. C., Mao, J. T., Alexis, K. H. L., Yuan, Z. B., Wang, M. H., and Liu, X. Y.: Application of MODIS satellite products to the air pollution research in Beijing, Sci. China Ser. D., 48. 209–219, 2005.
Li, J., Xie, S. D., Zeng, L. M., Li, L. Y., Li, Y. Q., and Wu, R. R.: Characterization of ambient volatile organic compounds and their sources in Beijing, before, during, and after Asia-Pacific Economic Cooperation China 2014, Atmos. Chem. Phys., 15, 7945–7959, https://doi.org/10.5194/acp-15-7945-2015, 2015.
Li, J., Wang, X., Chen, J., Zhu, C., Li, W., Li, C., Liu, L., Xu, C., Wen, L., Xue, L., Wang, W., Ding, A., and Herrmann, H.: Chemical composition and droplet size distribution of cloud at the summit of Mount Tai, China, Atmos. Chem. Phys., 17, 9885–9896, https://doi.org/10.5194/acp-17-9885-2017, 2017.
Li, L. Y., Xie, S. D., Zeng, L. M., Wu, R. R., and Li, J.: Characteristics of volatile organic compounds and their role in ground-level ozone formation in the Beijing-Tianjin-Hebei region, China, Atmos. Environ., 113, 247–254, https://doi.org/10.1016/j.atmosenv.2015.05.021, 2015.
Li, M., Zhang, Q., Kurokawa, J.-I., Woo, J.-H., He, K., Lu, Z., Ohara, T., Song, Y., Streets, D. G., Carmichael, G. R., Cheng, Y., Hong, C., Huo, H., Jiang, X., Kang, S., Liu, F., Su, H., and Zheng, B.: MIX: a mosaic Asian anthropogenic emission inventory under the international collaboration framework of the MICS-Asia and HTAP, Atmos. Chem. Phys., 17, 935–963, https://doi.org/10.5194/acp-17-935-2017, 2017.
Li, Y., An, J. L., Min, M., Zhang, W., Wang, F., and Xie, P. H.: Impacts of HONO sources on the air quality in Beijing, Tianjin and Hebei Province of China, Atmos. Environ., 45, 4735–4744, https://doi.org/10.1016/j.atmosenv.2011.04.086, 2011.
Lim, H. J., Carlton, A. G., and Turpin, B. J.: Isoprene forms secondary organic aerosol through cloud processing: model simulations, Environ. Sci. Technol., 39, 4441–4446, https://doi.org/10.1021/es048039h, 2005.
Liu, Y., Shao, M., Kuster, W. C., Goldan, P. D., Li, X. H., Lu, S. H., and De Gouw, J. A.: Source Identification of Reactive Hydrocarbons and Oxygenated VOCs in the Summertime in Beijing, Environ. Sci. Technol., 43, 75–81, https://doi.org/10.1021/es801716n, 2009.
Liu, Y. J., Zhang, T. T., Liu, Q. Y., Zhang, R. J., Sun, Z. Q., and Zhang, M. G.: Seasonal Variation of Physical and Chemical Properties in TSP, PM10 and PM2.5 at a Roadside Site in Beijing and Their Influence on Atmospheric Visibility, Aerosol Air Qual. Res., 14, 954–969, https://doi.org/10.4209/aaqr.2013.01.0023, 2014.
Liu, Z., Wang, Y., Gu, D., Zhao, C., Huey, L. G., Stickel, R., Liao, J., Shao, M., Zhu, T., Zeng, L., Amoroso, A., Costabile, F., Chang, C.-C., and Liu, S.-C.: Summertime photochemistry during CAREBeijing-2007: ROx budgets and O3 formation, Atmos. Chem. Phys., 12, 7737–7752, https://doi.org/10.5194/acp-12-7737-2012, 2012a.
Liu, Z., Wang, Y. H., Vrekoussis, M., Richter, A., Wittrock, F., Burrows, J. P., Shao, M., Chang, C. C., Liu, S. C., Wang, H. L., and Chen, C. H.: Exploring the missing source of glyoxal (CHOCHO) over China, Geophys. Res. Lett., 39, L10812, https://doi.org/10.1029/2012GL051645, 2012b.
Lu, K. D., Rohrer, F., Holland, F., Fuchs, H., Bohn, B., Brauers, T., Chang, C. C., Häseler, R., Hu, M., Kita, K., Kondo, Y., Li, X., Lou, S. R., Nehr, S., Shao, M., Zeng, L. M., Wahner, A., Zhang, Y. H., and Hofzumahaus, A.: Observation and modelling of OH and HO2 concentrations in the Pearl River Delta 2006: a missing OH source in a VOC rich atmosphere, Atmos. Chem. Phys., 12, 1541–1569, https://doi.org/10.5194/acp-12-1541-2012, 2012.
Matsui, H., Koike, M., Kondo, Y., Takegawa, N., Kita, K., Miyazaki, Y., Hu, M., Chang, S. Y., Blake, D., and Fast, J. D.: Spatial and temporal variations of aerosols around Beijing in summer 2006: Model evaluation and source apportionment, J. Geophys. Res.-Atmos., 114, D00G13, https://doi.org/10.1029/2008JD010906, 2009.
Meng, J., Wang, G., Hou, Z., Liu, X., Wei, B., Wu, C., Cao, C., Wang, J., Li, J., Cao, J., Zhang, E., Dong, J., Liu, J., Ge, S., and Xie, Y.: Molecular distribution and stable carbon isotopic compositions of dicarboxylic acids and related SOA from biogenic sources in the summertime atmosphere of Mt. Tai in the North China Plain, Atmos. Chem. Phys., 18, 15069–15086, https://doi.org/10.5194/acp-18-15069-2018, 2018.
Meng, Z. Y., Lin, W. L., Jiang, X. M., Yan, P., Wang, Y., Zhang, Y. M., Jia, X. F., and Yu, X. L.: Characteristics of atmospheric ammonia over Beijing, China, Atmos. Chem. Phys., 11, 6139–6151, https://doi.org/10.5194/acp-11-6139-2011, 2011.
Miyazaki, Y., Aggarwal, S. G., Singh, K., Gupta, P. K., and Kawamura, K.: Dicarboxylic acids and water-soluble organic carbon in aerosols in New Delhi, India, in winter: Characteristics and formation processes, J. Geophys. Res.-Atmos., 114, D19206, https://doi.org/10.1029/2009JD011790, 2009.
Mouchel-Vallon, C., Deguillaume, L., Monod, A., Perroux, H., Rose, C., Ghigo, G., Long, Y., Leriche, M., Aumont, B., Patryl, L., Armand, P., and Chaumerliac, N.: CLEPS 1.0: A new protocol for cloud aqueous phase oxidation of VOC mechanisms, Geosci. Model Dev., 10, 1339–1362, https://doi.org/10.5194/gmd-10-1339-2017, 2017.
Ng, N. L., Brown, S. S., Archibald, A. T., Atlas, E., Cohen, R. C., Crowley, J. N., Day, D. A., Donahue, N. M., Fry, J. L., Fuchs, H., Griffin, R. J., Guzman, M. I., Herrmann, H., Hodzic, A., Iinuma, Y., Jimenez, J. L., Kiendler-Scharr, A., Lee, B. H., Luecken, D. J., Mao, J., McLaren, R., Mutzel, A., Osthoff, H. D., Ouyang, B., Picquet-Varrault, B., Platt, U., Pye, H. O. T., Rudich, Y., Schwantes, R. H., Shiraiwa, M., Stutz, J., Thornton, J. A., Tilgner, A., Williams, B. J., and Zaveri, R. A.: Nitrate radicals and biogenic volatile organic compounds: oxidation, mechanisms, and organic aerosol, Atmos. Chem. Phys., 17, 2103–2162, https://doi.org/10.5194/acp-17-2103-2017, 2017.
Pavuluri, C. M. and Kawamura, K.: Evidence for 13-carbon enrichment in oxalic acid via iron catalyzed photolysis in aqueous phase, Geophys. Res. Lett., 39, L03802, https://doi.org/10.1029/2011GL050398, 2012.
Pavuluri, C. M., Kawamura, K., and Swaminathan, T.: Water-soluble organic carbon, dicarboxylic acids, ketoacids, and α-dicarbonyls in the tropical Indian aerosols, J. Geophys. Res.-Atmos., 115, D11302, https://doi.org/10.1029/2009JD012661, 2010.
Rao, Z. H., Chen, Z. M., Liang, H., Huang, L. B., and Huang, D.: Carbonyl compounds over urban Beijing: Concentrations on haze and non-haze days and effects on radical chemistry, Atmos. Environ., 124, 207–216, https://doi.org/10.1016/j.atmosenv.2015.06.050, 2016.
Ren, Y., Ding, A. J., Wang, T., Shen, X. H., Guo, J., Zhang, J. M., Wang, Y., Xu, P. J., Wang, X. F., Gao, J., and Collett Jr., J. L.: Measurement of gas-phase total peroxides at the summit of Mount Tai in China, Atmos. Environ., 43, 1702–1711, https://doi.org/10.1016/j.atmosenv.2008.12.020, 2009.
Rusumdar, A. J., Tilgner, A., Wolke, R., and Herrmann, H.: Treatment of non-ideality in the multiphase model SPACCIM-Part2: Impacts on the multiphase chemical processing in deliquesced aerosol particles, Atmos. Chem. Phys. Discuss., https://doi.org/10.5194/acp-2019-819, in review, 2019.
Saunders, S. M., Jenkin, M. E., Derwent, R. G., and Pilling, M. J.: Protocol for the development of the Master Chemical Mechanism, MCM v3 (Part A): tropospheric degradation of non-aromatic volatile organic compounds, Atmos. Chem. Phys., 3, 161–180, https://doi.org/10.5194/acp-3-161-2003, 2003.
Schwartz, S. E.: Mass-transport considerations pertinent to aqueous phase reactions of gases in liquid-water clouds, in: Chemistry of multiphase atmospheric systems, Springer, 415–471, https://doi.org/10.1007/978-3-642-70627-1_16, 1986.
Seagrave, J., McDonald, J. D., Bedrick, E., Edgerton, E. S., Gigliotti, A. P., Jansen, J. J., Ke, L., Naeher, L. P., Seilkop, S. K., and Zheng, M.: Lung toxicity of ambient particulate matter from southeastern US sites with different contributing sources: relationships between composition and effects, Environ. Health Persp., 114, 1387–1393, https://doi.org/10.1289/ehp.9234, 2006.
Sehili, A., Wolke, R., Knoth, O., Simmel, M., Tilgner, A., and Herrmann, H.: Comparison of different model approaches for the simulation of multiphase processes, Atmos. Environ., 39, 4403–4417, https://doi.org/10.1016/j.atmosenv.2005.02.039, 2005.
Shen, X. H., Lee, T., Guo, J., Wang, X. F., Li, P. H., Xu, P. J., Wang, Y., Ren, Y., Wang, W. X., Wang, T., Li, Y., Carn, S. A., and Collett Jr., J. L.: Aqueous phase sulfate production in clouds in eastern China, Atmos. Environ., 62, 502–511, https://doi.org/10.1016/j.atmosenv.2012.07.079, 2012.
Shrivastava, M., Cappa, C. D., Fan, J. W., Goldstein, A. H., Guenther, A. B., Jimenez, J. L., Kuang, C., Laskin, A., Martin, S. T., Ng, N. L., Petaja, T., Pierce, J. R., Rasch, P. J., Roldin, P., Seinfeld, J. H., Shilling, J., Smith, J. N., Thornton, J. A., Volkamer, R., Wang, J., Worsnop, D. R., Zaveri, R. A., Zelenyuk, A., and Zhang, Q.: Recent advances in understanding secondary organic aerosol: Implications for global climate forcing, Rev. Geophys., 55, 509–559, https://doi.org/10.1002/2016RG000540, 2017.
Simmel, M. and Wurzler, S.: Condensation and activation in sectional cloud microphysical models, Atmos. Res., 80, 218–236, https://doi.org/10.1016/j.atmosres.2005.08.002, 2006.
Simmel, M., Diehl, K., and Wurzler, S.: Numerical simulation of the microphysics of an orographic cloud: comparison with measurements and sensitivity studies, Atmos. Environ., 39, 4365–4373, https://doi.org/10.1016/j.atmosenv.2005.02.017, 2005.
Sindelarova, K., Granier, C., Bouarar, I., Guenther, A., Tilmes, S., Stavrakou, T., Müller, J.-F., Kuhn, U., Stefani, P., and Knorr, W.: Global data set of biogenic VOC emissions calculated by the MEGAN model over the last 30 years, Atmos. Chem. Phys., 14, 9317–9341, https://doi.org/10.5194/acp-14-9317-2014, 2014.
Sorooshian, A., Varutbangkul, V., Brechtel, F. J., Ervens, B., Feingold, G., Bahreini, R., Murphy, S. M., Holloway, J. S., Atlas, E. L., and Buzorius, G.: Oxalic acid in clear and cloudy atmospheres: Analysis of data from International Consortium for Atmospheric Research on Transport and Transformation 2004, J. Geophys. Res.-Atmos., 111, D23S45, https://doi.org/10.1029/2005JD006880, 2006.
Suhail, K., George, M., Chandran, S., Varma, R., Venables, D. S., Wang, M., and Chen, J.: Open path incoherent broadband cavity-enhancedmeasurements of NO3 radical and aerosol extinction in the North China Plain, Spectrochim. Acta. A, 208, 24–31, https://doi.org/10.1016/j.saa.2018.09.023, 2019.
Sun, L., Xue, L., Wang, T., Gao, J., Ding, A., Cooper, O. R., Lin, M., Xu, P., Wang, Z., Wang, X., Wen, L., Zhu, Y., Chen, T., Yang, L., Wang, Y., Chen, J., and Wang, W.: Significant increase of summertime ozone at Mount Tai in Central Eastern China, Atmos. Chem. Phys., 16, 10637–10650, https://doi.org/10.5194/acp-16-10637-2016, 2016.
Sun, W. J., Yang, L. M., Yu, L. Y., and Saeys, M.: Ab Initio Reaction Path Analysis for the Initial Hydrogen Abstraction from Organic Acids by Hydroxyl Radicals, J. Phys. Chem. A., 113, 7852–7860, https://doi.org/10.1021/jp8090792, 2009.
Sun, Y., Jiang, Q., Wang, Z., Fu, P., Li, J., Yang, T., and Yin, Y.: Investigation of the sources and evolution processes of severe haze pollution in Beijing in January 2013, J. Geophys. Res.-Atmos., 119, 4380–4398, https://doi.org/10.1002/2014JD021641, 2014.
Sun, Y. L., Wang, Z. F., Fu, P. Q., Yang, T., Jiang, Q., Dong, H. B., Li, J., and Jia, J. J.: Aerosol composition, sources and processes during wintertime in Beijing, China, Atmos. Chem. Phys., 13, 4577–4592, https://doi.org/10.5194/acp-13-4577-2013, 2013.
Sun, Y. L., Wang, Z. F., Du, W., Zhang, Q., Wang, Q. Q., Fu, P. Q., Pan, X. L., Li, J., Jayne, J., and Worsnop, D. R.: Long-term real-time measurements of aerosol particle composition in Beijing, China: seasonal variations, meteorological effects, and source analysis, Atmos. Chem. Phys., 15, 10149–10165, https://doi.org/10.5194/acp-15-10149-2015, 2015.
Tabazadeh, A.: Organic aggregate formation in aerosols and its impact on the physicochemical properties of atmospheric particles, Atmos. Environ., 39, 5472–5480, https://doi.org/10.1016/j.atmosenv.2005.05.045, 2005.
Tilgner, A. and Herrmann, H.: Radical-driven carbonyl-to-acid conversion and acid degradation in tropospheric aqueous systems studied by CAPRAM, Atmos. Environ., 44, 5415–5422, https://doi.org/10.1016/j.atmosenv.2010.07.050, 2010.
Tilgner, A. and Herrmann, H.: Tropospheric Aqueous-Phase OH Oxidation Chemistry: Current Understanding, Uptake of Highly Oxidized Organics and Its Effects, Multiphase Environ. Chem. Atmos., 1299, 49–85, https://doi.org/10.1021/bk-2018-1299.ch004, 2018.
Tilgner, A., Bräuer, P., Wolke, R., and Herrmann, H.: Modelling multiphase chemistry in deliquescent aerosols and clouds using CAPRAM3.0i, J. Atmos. Chem., 70, 221–256, https://doi.org/10.1007/s10874-013-9267-4, 2013.
Volkamer, R., Jimenez, J. L., San Martini, F., Dzepina, K., Zhang, Q., Salcedo, D., Molina, L. T., Worsnop, D. R., and Molina, M. J.: Secondary organic aerosol formation from anthropogenic air pollution: Rapid and higher than expected, Geophys. Res. Lett., 33, L17811, https://doi.org/10.1029/2006GL026899, 2006.
Volkamer, R., Ziemann, P. J., and Molina, M. J.: Secondary Organic Aerosol Formation from Acetylene (C2H2): seed effect on SOA yields due to organic photochemistry in the aerosol aqueous phase, Atmos. Chem. Phys., 9, 1907–1928, https://doi.org/10.5194/acp-9-1907-2009, 2009.
Wang, G., Kawamura, K., Umemoto, N., Xie, M. J., Hu, S. Y., and Wang, Z. F.: Water-soluble organic compounds in PM2.5 and size-segregated aerosols over Mount Tai in North China Plain, J. Geophys. Res.-Atmos., 114, D19208, https://doi.org/10.1029/2008JD011390, 2009.
Wang, G., Zhang, R., Gomez, M. E., Yang, L., Zamora, M. L., Hu, M., Lin, Y., Peng, J., Guo, S., and Meng, J.: Persistent sulfate formation from London Fog to Chinese haze, P. Natl. Acad. Sci. USA, 113, 13630–13635, https://doi.org/10.1073/pnas.1616540113, 2016.
Wang, H. B., Tian, M., Li, X. H., Chang, Q., Cao, J. J., Yang, F. M., Ma, Y. L., and He, K. B.: Chemical Composition and Light Extinction Contribution of PM2.5 in Urban Beijing for a 1-Year Period, Aerosol Air Qual. Res., 15, 2200–2211, https://doi.org/10.4209/aaqr.2015.04.0257, 2015.
Wang, S., Shi, C., Zhou, B., Zhao, H., Wang, Z., Yang, S., and Chen, L.: Observation of NO3 radicals over Shanghai, China, Atmos. Environ., 70, 401–409, https://doi.org/10.1016/j.atmosenv.2013.01.022, 2013.
Wang, Y., Zhuang, G., Sun, Y., and An, Z.: The variation of characteristics and formation mechanisms of aerosols in dust, haze, and clear days in Beijing, Atmos. Environ., 40, 6579–6591, https://doi.org/10.1016/j.atmosenv.2006.05.066, 2006.
Wang, Y., Shen, L., Wu, S., Mickley, L., He, J., and Hao, J.: Sensitivity of surface ozone over China to 2000–2050 global changes of climate and emissions, Atmos. Environ., 75, 374–382, https://doi.org/10.1016/j.atmosenv.2013.04.045, 2013.
Wang, Y., Yao, L., Wang, L., Liu, Z., Ji, D., Tang, G., Zhang, J., Sun, Y., Hu, B., and Xin, J.: Mechanism for the formation of the January 2013 heavy haze pollution episode over central and eastern China, Sci. China Earth Sci., 57, 14–25, https://doi.org/10.1007/s11430-013-4773-4, 2014.
Wang, Y., Chen, Z., Wu, Q., Liang, H., Huang, L., Li, H., Lu, K., Wu, Y., Dong, H., Zeng, L., and Zhang, Y.: Observation of atmospheric peroxides during Wangdu Campaign 2014 at a rural site in the North China Plain, Atmos. Chem. Phys., 16, 10985–11000, https://doi.org/10.5194/acp-16-10985-2016, 2016.
Warneck, P.: In-cloud chemistry opens pathway to the formation of oxalic acid in the marine atmosphere, Atmos. Environ., 37, 2423–2427, https://doi.org/10.1016/S1352-2310(03)00136-5, 2003.
Wang, Y. H., Hu, B., Ji, D. S., Liu, Z. R., Tang, G. Q., Xin, J. Y., Zhang, H. X., Song, T., Wang, L. L., Gao, W. K., Wang, X. K., and Wang, Y. S.: Ozone weekend effects in the Beijing–Tianjin–Hebei metropolitan area, China, Atmos. Chem. Phys., 14, 2419–2429, https://doi.org/10.5194/acp-14-2419-2014, 2014.
Wen, L., Xue, L., Wang, X., Xu, C., Chen, T., Yang, L., Wang, T., Zhang, Q., and Wang, W.: Summertime fine particulate nitrate pollution in the North China Plain: increasing trends, formation mechanisms and implications for control policy, Atmos. Chem. Phys., 18, 11261–11275, https://doi.org/10.5194/acp-18-11261-2018, 2018.
Williams, J., de Reus, M., Krejci, R., Fischer, H., and Ström, J.: Application of the variability-size relationship to atmospheric aerosol studies: estimating aerosol lifetimes and ages, Atmos. Chem. Phys., 2, 133–145, https://doi.org/10.5194/acp-2-133-2002, 2002.
Wolke, R., Sehili, A., Simmel, M., Knoth, O., Tilgner, A., and Herrmann, H.: SPACCIM: A parcel model with detailed microphysics and complex multiphase chemistry, Atmos. Environ., 39, 4375–4388, https://doi.org/10.1016/j.atmosenv.2005.02.038, 2005.
Xue, L., Wang, T., Wang, X., Blake, D. R., Gao, J., Nie, W., Gao, R., Gao, X., Xu, Z., and Ding, A.: On the use of an explicit chemical mechanism to dissect peroxy acetyl nitrate formation, Environ. Pollut., 195, 39–47, https://doi.org/10.1016/j.envpol.2014.08.005, 2014.
Yao, X., Chan, C. K., Fang, M., Cadle, S., Chan, T., Mulawa, P., He, K., and Ye, B.: The water-soluble ionic composition of PM2.5 in Shanghai and Beijing, China, Atmos. Environ., 36, 4223–4234, https://doi.org/10.1016/S1352-2310(02)00342-4, 2002.
Yu, Q., Chen, J., Qin, W. H., Cheng, S. M., Zhang, Y. P., Ahmad, M., and Ouyang, W.: Characteristics and secondary formation of water-soluble organic acids in PM1, PM2.5 and PM10 in Beijing during haze episodes, Sci. Total Environ., 669, 175–184, https://doi.org/10.1016/j.scitotenv.2019.03.131, 2019.
Zhang, J., Chen, J., Yang, L., Sui, X., Yao, L., Zheng, L., Wen, L., Xu, C., and Wang, W.: Indoor PM2.5 and its chemical composition during a heavy haze–fog episode at Jinan, China, Atmos. Environ., 99, 641–649, https://doi.org/10.1016/j.atmosenv.2014.10.026, 2014.
Zhao, P. S., Dong, F., He, D., Zhao, X. J., Zhang, X. L., Zhang, W. Z., Yao, Q., and Liu, H. Y.: Characteristics of concentrations and chemical compositions for PM2.5 in the region of Beijing, Tianjin, and Hebei, China, Atmos. Chem. Phys., 13, 4631–4644, https://doi.org/10.5194/acp-13-4631-2013, 2013.
Zhao, W., Kawamura, K., Yue, S., Wei, L., Ren, H., Yan, Y., Kang, M., Li, L., Ren, L., Lai, S., Li, J., Sun, Y., Wang, Z., and Fu, P.: Molecular distribution and compound-specific stable carbon isotopic composition of dicarboxylic acids, oxocarboxylic acids and α-dicarbonyls in PM2.5 from Beijing, China, Atmos. Chem. Phys., 18, 2749–2767, https://doi.org/10.5194/acp-18-2749-2018, 2018.
Zhao, W., Fu, P., Yue, S., Li, L., Xie, Q., Zhu, C., Wei, L., Ren, H., Li, P., Li, W., Sun, Y., Wang, Z., Kawamura, K., and Chen, J.: Excitation-emission matrix fluorescence, molecular characterization and compound-specific stable carbon isotopic composition of dissolved organic matter in cloud water over Mt. Tai, Atmos. Environ., 213, 608–619, https://doi.org/10.1016/j.atmosenv.2019.06.034, 2019.
Zhao, X. J., Zhao, P. S., Xu, J., Meng,, W., Pu, W. W., Dong, F., He, D., and Shi, Q. F.: Analysis of a winter regional haze event and its formation mechanism in the North China Plain, Atmos. Chem. Phys., 13, 5685–5696, https://doi.org/10.5194/acp-13-5685-2013, 2013.
Zhou, Y., Wang, T., Gao, X. M., Xue, L. K., Wang, X. F., Wang, Z., Gao, J., Zhang, Q. Z., and Wang, W. X.: Continuous observations of water-soluble ions in PM2.5 at Mount Tai (1534 ma.s.l.) in central-eastern China, J. Atmos. Chem., 64, 107–127, https://doi.org/10.1007/s10874-010-9172-z, 2009.
Zhu, Y.: acp-2019-982-data, available at: https://github.com/Yanhong-Zhu/acp-2019-982-data, last access: 15 May 2020.
Zhu, Y., Yang, L., Chen, J., Kawamura, K., Sato, M., Tilgner, A., van Pinxteren, D., Chen, Y., Xue, L., Wang, X., Simpson, I. J., Herrmann, H., Blake, D. R., and Wang, W.: Molecular distributions of dicarboxylic acids, oxocarboxylic acids and α-dicarbonyls in PM2.5 collected at the top of Mt. Tai, North China, during the wheat burning season of 2014, Atmos. Chem. Phys., 18, 10741–10758, https://doi.org/10.5194/acp-18-10741-2018, 2018.