the Creative Commons Attribution 4.0 License.
the Creative Commons Attribution 4.0 License.
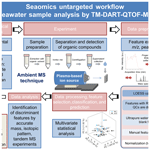
Seawater analysis by ambient mass-spectrometry-based seaomics
Nicolás Zabalegui
Malena Manzi
Antoine Depoorter
Nathalie Hayeck
Marie Roveretto
Chunlin Li
Manuela van Pinxteren
Hartmut Herrmann
Christian George
María Eugenia Monge
An analytical method coupled to multivariate statistical analysis was developed based on transmission-mode direct analysis in real-time quadrupole time-of-flight mass spectrometry (TM-DART-QTOF-MS) to interrogate lipophilic compounds in seawater samples without the need for desalinization. An untargeted metabolomics approach is addressed here as seaomics and was successfully implemented to discriminate the sea surface microlayer (SML) from the underlying water (ULW) samples (n=22, 10 paired samples) collected during a field campaign at the Cabo Verde islands during September–October 2017. A panel of 11 ionic species detected in all samples allowed sample class discrimination by means of supervised multivariate statistical models. Tentative identification of the species enriched in the SML samples suggests that fatty alcohols, halogenated compounds, and oxygenated boron-containing organic compounds are available at the surface for air–water transfer processes. A subset of SML samples (n=5) were subjected to on-site experiments during the campaign by using a lab-to-field approach to test their secondary organic aerosol (SOA) formation potency. The results from these experiments and the analytical seaomics strategy provide a proof of a concept that can be used for an approach to identifying organic molecules involved in aerosol formation processes at the air–water interface.
- Article
(2307 KB) - Full-text XML
-
Supplement
(1761 KB) - BibTeX
- EndNote
Oceans act as sinks and sources for gases and aerosol particles. The ocean surface chemical composition influences the physicochemical processes occurring at the air–water interface by connecting the ocean biogeochemistry with the atmospheric chemistry in the marine boundary layer (MBL; Donaldson and George, 2012). Therefore, understanding how the organic compounds of marine origin are influencing the formation of aerosols in the MBL with potential impacts on the radiative fluxes, aerosol hygroscopicity, and subsequent cloud condensation nuclei properties is important. It has been suggested that complex photoactive compounds are enhanced at the air–sea interface (Reeser et al., 2009a, b), thus inducing the abiotic production of volatile organic compounds. For instance, experimental photosensitized reactions at the air–water interface by using humic acids as a proxy of dissolved organic matter (DOM) have led to the chemical conversion of linear saturated fatty acids into unsaturated functionalized gas-phase products (Ciuraru et al., 2015). Atmospheric photochemistry can even take place in the absence of photosensitizers if the air–water interface is coated with a fatty acid (Rossignol et al., 2016). On a global scale, interfacial photochemistry has recently been suggested to serve as an abiotic source of volatile organic compounds, which is comparable to marine biological emissions (Brüggemann et al., 2018).
The sea surface microlayer (SML) covers up to 70 % of the Earth's surface and is enriched with DOM, including organic compounds such as fatty acids, fatty alcohols, sterols, amines, amino acids, proteins, lipids, phenolic compounds, and UV-absorbing humic-like substances derived from oceanic biota (Liss and Duce, 1997). In addition, particulate matter, microorganisms (Donaldson and George, 2012), and colloids and phytoplankton-exuded aggregates mainly constituted by lipopolysaccharides can also be found (Liss and Duce, 1997; Hunter and Liss, 1977; Bayliss and Bucat, 1975; Liss, 1986; Hardy, 1982; Garabetian et al., 1993; Williams et al., 1986; Schneider and Gagosian, 1985; Gershy, 1983; Guitart et al., 2004; Facchini et al., 2008; Kovac et al., 2002). While the identification of these classes of compounds has been achieved in the past, an improved chemical characterization of the SML and its chemical processing is highly desirable to better understand its contribution to atmospheric composition, air quality, and climate change (Liss and Duce, 1997).
Metabolomics is the comprehensive analysis and characterization of all small molecules (MW<1500) in a biological system (Fiehn et al., 2000; Nicholson and Lindon, 2008), such as the marine metabolome. Mass spectrometry (MS) is one of the primary analytical techniques used to explore the metabolome, as it is highly sensitive and versatile for conducting chemical analyses in targeted and untargeted studies (Clendinen et al., 2017; Weckwerth and Morgenthal, 2005). Targeted metabolomics focuses on detecting and quantifying a preselected set of metabolites. Conversely, untargeted metabolomics attempts to cover the broadest range of detectable compounds in a biological system (Viant et al., 2019), in order to subsequently extract chemical patterns or class fingerprints that can allow for sample classification based on metabolite panels without any a priori hypotheses. Multivariate statistical techniques compute all of the compound features (variables) simultaneously with the aim of reducing data dimensionality, finding underlying trends, and isolating feature panels relevant to class discrimination (Saccenti et al., 2014). Following compound identification, the relative changes of abundances can be analyzed for biological interpretation.
The advancements in the new, soft ambient ion generation techniques offer alternative MS-based applications for surface analysis, with little to no sample preparation, and address high-throughput analytical challenges in untargeted metabolomics workflows (Monge et al., 2013; Harris et al., 2011; Clendinen et al., 2017). In particular, direct analysis in real time (DART) (Cody et al., 2005; Gross, 2014; Jones et al., 2014; Monge and Fernández, 2014), which is a plasma-based ambient ion source, has been successfully applied in untargeted metabolomics studies in different scientific fields (Salter et al., 2011; Ifa et al., 2009; Steiner and Larson, 2009; Fernández et al., 2006; Chernetsova et al., 2010; Hajslova et al., 2011; Cajka et al., 2011; Dove et al., 2012; Jones and Fernández, 2013; Zang et al., 2017); to date, however, no studies have been reported to explore oceanic biological systems. In DART-MS, a stream of metastable atomic or molecular species generated within the heated discharge He or N2 gas is directed at the sample, and ions are suctioned into the mass spectrometer (Cody et al., 2005). Thermally desorbed analytes, typically having MW<1000, are ionized following atmospheric pressure chemical ionization (ACPI)-like pathways (Cody et al., 2005; Song et al., 2009a, b; McEwen and Larsen, 2009). Therefore, a major limitation is that it requires analytes to be volatile or semivolatile, which reduces the metabolome coverage. An important advantage of DART, when compared to electrospray ionization (ESI) for seawater analysis, is that it is less affected by high salt levels (Kaylor et al., 2014; Tang et al., 2004), thus avoiding the desalinization processes that may lead to sample alteration. Conversely, ESI sources allow for the coupling of MS to chromatographic systems that provide an additional parameter to improve confidence in the compound identification when compared to an authentic chemical standard.
In the present work, a transmission mode (TM)-DART-quadrupole time-of-flight (QTOF)-MS-based analytical method was developed to interrogate the seawater DOM composition in SML and underlying water (ULW) samples collected during a field campaign at the Cabo Verde islands during September–October 2017. An untargeted metabolomics approach, addressed here as seaomics, was implemented to successfully discriminate the SML from ULW samples based on a selected panel of 11 ionic species. Tentative identification of the discriminant panel provided insight into the family of compounds that may be involved in air–water transfer processes and photochemical reactions at the air–water interface of the ocean surface. In addition, secondary organic aerosol formation potency from the SML interfacial photochemical products was explored during the field campaign by using a lab-to-field approach. To our knowledge, this is the first study to apply an untargeted TM-DART-QTOF-MS-based seaomics analytical strategy coupled to multivariate statistical analysis to investigate the DOM seawater composition.
2.1 Chemicals
An LC–MS grade Acetonitrile was purchased from Fisher Chemical (North Carolina, USA). Ultrapure water with 18.2 MΩ cm resistivity (Thermo Scientific Barnstead MicroPure UF/UV ultrapure water system; Massachusetts, USA) was used to prepare standard solutions. Commercial seawater (S9883), glucose, xylose, fructose, galactosamine, mannitol, L-glycine, L-alanine, γ-aminobutyric acid (GABA), L-serine, L-proline, L-valine, L-threonine, L-isoleucine, L-leucine, L-asparagine, L-aspartic acid, L-glutamine, L-glutamic acid, L-methionine, L-histidine, L-phenylalanine, L-arginine, L-tryptophan, 2-amino-4,5-dimethoxybenzoic acid, 2-cyanoguanidine, flecainide acetate, lacosamide, enalapril maleate, 4-bromophenol, and mercaptosuccinic acid were purchased from Sigma-Aldrich (St. Louis, Missouri, USA). Decanoic acid, docosanoic acid, dodecanoic acid, eicosanoic acid, and octadecanoic acid were purchased from Larodan AB (Solna, Sweden). Potassium bromide (KBr) was purchased from Biopak (Ciudad Autónoma de Buenos Aires, Argentina), and phenol was purchased from Carlo Erba Reagents SA (Sabadell, Barcelona, Spain).
2.2 Sample collection at the Cabo Verde field campaign
Sea surface microlayer (SML) samples were manually collected by the traditional glass plate (GP) method (van Pinxteren et al., 2012) and with an automatic catamaran named MarParCat (CAT) by using the same sampling principle as GP. The MarParCat is an autonomous catamaran for sampling the SML on rotating glass plates. Larger quantities of SML samples can be collected with this method in a shorter time. Underlying water (ULW) samples were collected from 1.0 m sea subsurface during the same time window as the SML samples, using both strategies, i.e., manual sampling addressed as GP and MarParCat (Table S1 in the Supplement). SML and ULW samples that were collected at the same site are addressed as paired samples (Table S1). The samples analyzed in the present study (n=22) were collected between 18 September 2017 and 10 October 2017 and stored at −20 ∘C until processing. Information related to sampling conditions, sample salinity, pH, and temperature is provided in Table S1. Dissolved organic carbon (DOC) levels varied between 1.8 and 3.2 mg L−1 in the SML and between 0.9 and 2.8 mg L−1 in the ULW (van Pinxteren et al., 2019).
2.3 Aerosol particle formation experiments at the Cabo Verde islands
A subset of collected SML seawater samples were subjected to on-site experiments using a lab-to-field approach to test whether they were photochemically active (Ciuraru et al., 2015). Before each experiment, a 100 mL SML sample was conditioned to room temperature and divided into 12 aliquots. These were centrifuged at 3500 rpm and 4 ∘C for 25 min to exclude colloids and aggregates (particulate matter), using a 5702R centrifuge (Eppendorf, Hamburg, Germany). Subsequently, 2 mL of surface solution was collected from each centrifugal vessel to isolate closer representations of SML samples considering the dilution factor inherent to the collection process, i.e., SML diluted with the ULW contribution, which led to a total sample volume of 24 mL for subsequent experiments. Centrifugation was aimed at concentrating SML samples as a condition for aerosol formation.
Sample irradiation was conducted using a cylindrical quartz cell reactor (2 cm diameter, 10 cm length, and 30 mL volume), half-filled with 14 mL of SML solution, thereby recreating an air–water interface with a maximum area of 20 cm2. Experimental details of the reactor can be found elsewhere (Ciuraru et al., 2015). This quartz reactor was surrounded by UV lamps in a ventilated box, which maintained the system at a relatively constant room temperature. The interface was irradiated by means of 210 W actinic UV irradiation peaking at 350 nm (the spectrum is displayed in Fig. S1 in the Supplement, Supporting Text 1), which was supplied by seven low-pressure mercury UV lamps (Philips) and one extra UV pen ray (UVP, Philips).
This experimental approach allowed for the reproduction of the air–sea exchanges under quiescent conditions and for the investigation of particle formation that potentially arises from the reaction between photochemically emitted gaseous products and OH radicals. For this purpose, the quartz cell was continuously flushed with 600 sccm purified air, thus entraining the air–water interfacial-exchange gaseous products into a potential aerosol mass (PAM) oxidation flow reactor with a 254 nm light supply (hereafter referred to as OFR254). Particle formation via OH radical photochemistry in the OFR254 was monitored by using a scanning mobility particle sizer (SMPS, model 3976, TSI Incorporated, Minnesota, USA) and one extra ultrafine condensation particle counter (UCPC, model 3776, TSI Incorporated, Minnesota, USA; d50>2.5 nm). A description of the OFR254 operation and a scheme of the experimental setup are detailed in the Supplement (Figs. S2 and S3). Blank experiments were routinely conducted by using ultrapure water (18.2 MΩ cm resistivity).
2.4 Sample preparation for DART-MS analysis
Samples were thawed at 4 ∘C for 5 h; neither desalination nor filtration was performed. Samples were split into 8 mL aliquots using 15 mL conical tubes and were subsequently frozen at −20 ∘C until lyophilization. Quality-control (QC) samples were prepared by mixing equal volumes of all of the samples including both collection methods before the sample lyophilization (QCALL) and after metabolite extraction and reconstitution in acetonitrile (QCMIX22). The chemical standard mixtures used for the analytical method development and as system suitability samples (SSSs) were prepared in ultrapure water for sugars, and amino acids, and in methanol–water mixtures for lipids and by combining all the standards from the three families of compounds (Table S2). The sample preparation blank was prepared with ultrapure water as follows: fresh ultrapure water was stored for 2 d at −20 ∘C in a new plastic bottle equivalent to those used for sample collection; it was subsequently thawed, split in 8 mL aliquots, and stored in 15 mL conical tubes at −20 ∘C until lyophilization. This protocol was also implemented to prepare the commercial seawater samples (CSW) that were used for analytical method development. Blanks, QCs, SSSs, and samples were lyophilized at 0.280 mbar for 48 h by using an Alpha 1-4 LSCbasic freeze dryer (Martin Christ, Göttingen, Germany). The SML samples, ULW samples, QCs, and SSSs were lyophilized with sample blanks in different batches to evaluate possible cross-contamination. Lyophilized samples were shipped from TROPOS (Germany) to CIBION-CONICET (Argentina), where they were stored at −80 ∘C until the TM-DART-QTOF-MS analysis occurred. Lyophilized residues were reconstituted in 1200 µL of acetonitrile, yielding a concentration factor of 6.67. Reconstituted samples were vortex mixed for 5 min for metabolite extraction and centrifuged for 10 min at 4861×g and 20 ∘C to favor the formation of a salt pellet. For each sample, 500 µL of supernatant was collected for further analysis.
2.5 DART-MS analysis
A DART® SVP ionization source (IonSense Inc., Massachusetts, USA) was coupled to a Xevo G2-S QToF mass spectrometer (Waters Corporation, Wilmslow, UK) by means of a VAPUR® interface flange (IonSense Inc., Massachusetts, USA). The DART source was operated with He as the discharge gas heated to 300 ∘C, and the data were acquired in the negative ionization mode. A transmission mode (TM)-DART geometry was implemented for sample analysis, by setting a distance of 2.5 cm in the rail holding the source. This allowed for the use of the minimum possible DART-to-sample distance to provide the greatest sensitivity (Zang et al., 2017; Jones and Fernández, 2013). Samples were deposited in a stainless-steel mesh that was subsequently placed in a linear rail-based sampler, which was digitally controlled to minimize variance in sample position. Figure S4 illustrates the experimental design for depositing samples in different spots of the mesh to avoid cross-contamination. A protocol for calibrating the mass spectrometer across the range of m∕z 50–850 by using the DART source operated in TM was developed by using a mixture of standards prepared in a water–methanol solution (1:1 v∕v) that would provide almost equidistant m∕z peaks. Signals of different adduct ions from 2-cyanoguanidine, enalapril maleate, mercaptosuccinic acid, 2-amino-4,5-dimethoxybenzoic acid, flecainide acetate, and lacosamide were used for the time-of-flight (TOF) calibration (Table S3). Drift correction was performed after data acquisition by using stearic acid present as an ambient contaminant. The [M-H]− adduct ion with m∕z 283.2643 was chosen as a lock mass to have a high degree of accuracy in the exact mass measurement. Data were acquired in the continuum mode in the range of m∕z 50–850, and the scan time was set to 1 s. A standard solution of enalapril 3.7 µM was used as an additional SSS and added to each mesh in spot no. 3 (Fig. S4) to evaluate mass accuracy of the [M-H]− ion at m∕z 375.1925. The resolving power and mass accuracy of the TM-DART-QTOF-MS system were 23 000 full width at half maximum and 0.2 mDa at m∕z 375.1925, respectively. A total of 12 spots per mesh were utilized for analysis. Each spot contained three droplets of 20 µL of the same sample, which was dried at room temperature before analysis. The mesh holder was moved at a speed of 0.2 mm s−1 for data acquisition. Mesh nos. 1–11 included a solvent (SV) blank (acetonitrile); a commercial seawater control; a sample preparation blank (using ultrapure water); a QCMIX22 (pooled QC sample from all reconstituted samples: 10 SML + 12 ULW); and technical triplicates of all SML and ULW samples (Fig. S4). As indicated in Fig. S4, mesh no. 12 included QCALL samples (pooled QC sample from all samples before lyophilization: 10 SML + 12 ULW samples). For TM-DART-QTOF-MS/MS experiments, the product ion mass spectra were acquired with collision cell voltages between 10 and 40 V, depending on the analyte. Ultrahigh-purity argon (≥99.999 %) was used as the collision gas. Data acquisition and processing were carried out by using MassLynx 4.1 (Waters Corporation, Milford, Massachusetts, USA). Data were acquired for each spot, and acquisition over each mesh was automatically performed through synchronization between the DART software (IonSense, Inc.) and MassLynx (Waters Corporation, Milford, Massachusetts, USA). System suitability procedures were performed to verify that the method and associated instrumentation were fully functioning before and during the analysis of experimental samples.
2.6 Seaomics data analysis
The Progenesis Bridge (Waters Corporation, Milford, Massachusetts, USA) application was used for data preprocessing. This software allowed for the defining of the lock mass for drift correction after acquisition and merged the original data into a Gaussian profile. Spectral features (m∕z values) were further extracted from the TM-DART-QTOF-MS data using Progenesis QI version 2.1 (Nonlinear Dynamics, Waters Corporation, Milford, Massachusetts, USA). An absolute ion intensity filter was applied in the peak-picking process for integration, thus defining a threshold for the aggregate run. Only SML and ULW samples were considered for peak picking. This process yielded 889 features (m∕z) that were detected within the samples. Subsequently, six features were removed due to high mass defects (potential salt clusters). For the correction of intermesh effects, a quality-control-based robust locally estimated scatterplot smoothing (LOESS) signal correction method (Dunn et al., 2011) was applied by using QCMIX22 samples. This strategy allowed correcting for the temporal signal fluctuation of each feature along the total acquisition time. Subsequently, features with relative standard deviation (RSD) >30 % in QCMIX22 were discarded, and only those with a 5-fold average intensity in samples compared to the blanks (i.e., sample preparation blanks and solvent blanks) were retained. Manual curation of features was also performed to eliminate redundancy (isotopic peaks from the same feature), to retain signals with a detected isotopic pattern, and to account for resolution limitations in the peak-picking process. Moreover, only those monoisotopic peaks with intensity >103 in the continuum spectra were retained. The final curated matrix consisted of 51 features (m∕z values) and was normalized by the total ion area. Abundance values from technical triplicates were averaged, except for the SML GP2 sample, for which only two replicates were considered. The matrices obtained before and after averaging the technical replicates (data set S1 in the Supplement) were utilized to build unsupervised and supervised multivariate statistical analysis models using MATLAB R2015a (MathWorks, Natick, Massachusetts, USA) with PLS_Toolbox version 8.1 (Eigenvector Research, Inc., Manson, Washington, USA). Principal component analysis (PCA) (Johnson and Wichern, 2007) and t-distributed stochastic neighbor embedding (t-SNE; Van Der Maaten and Hinton, 2008) techniques were used to track the data quality, reduce the data dimensionality, and identify potential outliers in the data set as well as to identify sample clusters and evaluate the analytical method reproducibility. Orthogonal projections to latent structures discriminant analysis (OPLS-DA; Trygg et al., 2007; Bylesjö et al., 2006; Trygg and Wold, 2002; Shrestha and Vertes, 2010), coupled with a genetic algorithm (GA) variable selection method, were applied to find a feature panel that maximized the classification accuracy for the binary comparison of the SML and ULW samples. The selected group of discriminant features had the lowest root mean square error of cross-validation (RMSECV) at the conclusion of the GA variable selection process. This process was performed five different times. The selected panel yielded the lowest RMSECV and exhibited the largest feature overlap with the other four panels. The parameters for the GA were as follows: population size – 64; variable window width – 1; percent of initial terms (variables) – 15; target minimum no. of variables – 5, target maximum no. of variables – 15; penalty slope – 0.03; maximum generations – 100; percent at convergence – 50; mutation rate – 0.005; crossover – double; regression choice – PLS; no. of latent variables – 5; cross-validation – contiguous; no. of splits – 10; no. of iterations – 10; and replicate runs – 10. The OPLS-DA model was cross-validated using venetian blinds with four data splits and one sample per blind to account for overfitting. The data were preprocessed by autoscaling prior to the PCA or OPLS-DA. The PCA was also performed to inspect the data before and after GA variable selection (i.e., on the curated spectral feature matrix and on the discriminant feature panel). Fold changes were calculated for paired samples for each discriminant feature by comparing the sample replicate average values for the SML and ULW samples. The Wilcoxon paired signed rank test was used to compare SML with ULW samples (p<0.05). Median fold changes were calculated for each discriminant feature (Table S4).
2.7 Metabolite identification procedure
Metabolite identification was attempted for the discriminant features resulting from the GA variable selection process. The elemental formulae were generated based on accurate masses and isotopic patterns and taking the stringent conditions for isotope ratios into account. For those cases in which there was an overlap between isotopic peaks of different features, the isotopic pattern was not considered for molecular formula generation. In addition, fragmentation patterns obtained from TM-DART-QTOF-MS/MS experiments were used for tentative identification.
3.1 TM-DART-QTOF-MS-based method optimization
Figure 1 illustrates the untargeted TM-DART-QTOF-MS seaomics analytical workflow implemented for the analysis of seawater samples collected during the Cabo Verde field campaign. A TM geometry was implemented to analyze samples in a flow-through fashion to increase the reproducibility with a lower risk of cross-contamination (Zhou et al., 2010a, b; Jones and Fernández, 2013; Perez et al., 2010; Zang et al., 2017; Jones et al., 2014). The analytical method development involved the following: (i) the optimization of the ion source stabilization time, which was accomplished in 60 s, and the synchronization between data acquisition and the linear-rail control; (ii) the selection of He over N2 to generate the plasma, based on higher sensitivity obtained with the former; (iii) the optimization of the He temperature set at 300 ∘C; (iv) the selection of acetonitrile for metabolite extraction; (v) the optimization of the solvent volume required for extraction to allow for maximum metabolite concentration, considering that the seawater metabolome is comprised of organic compounds with a wide range of physicochemical properties and levels, and to allow for enough sample volume for technical replicates, QCs, and tandem MS analyses; and (vi) the optimization of the sample volume deposited on the mesh to maximize signal-to-noise ratio (number of sample droplets and droplet volume). The selected OM extraction method with acetonitrile as an extracting solvent favored the analysis of lipophilic compounds. In addition, to enhance the detection of organic acids, the analytical method was optimized by operating the DART ion source in negative ionization mode, since it follows negative ionization APCI-like mechanisms including electron capture, dissociative electron capture, proton abstraction, and anion adduction (McEwen and Larsen, 2009; Cody and Dane, 2013; Gross, 2014).
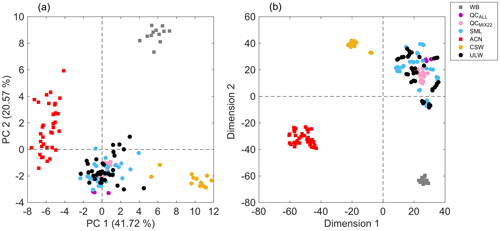
Figure 2(a) PCA score plot showing the first two principal components, and a (b) bidimensional t-SNE plot of seawater samples (circles) with solvent blanks (squares). The plot can be read by using the following: WB – sample preparation blanks using ultrapure water (gray); QCALL – pooled sample from all seawater samples before lyophilization (purple); QCMIX22 – pooled sample from all reconstituted seawater samples (pink); SML – sea surface microlayer water samples (light blue); ACN – acetonitrile (red); CSW – commercial seawater samples (gold); and ULW – underlying water samples (black). PCA and t-SNE models were built using the 51 extracted features and all replicates were included.
3.2 Seawater sample fingerprinting
The curated data matrix, comprised of 51 features, i.e., m∕z values, and all sample replicates (data set S1 in the Supplement), was used to build a PCA model that accumulated 62.29 % of the total variance in the first two principal components (PCs) (Fig. 2). The 2D score plot illustrated in Fig. 2a shows distinguishable separation between acetonitrile blanks, sample preparation blanks, commercial seawater samples, and seawater samples collected during the field campaign. Since the maximum data variance in a PCA model is in the direction of the base of the eigenvectors of the covariance matrix, the largest differences are given by seawater samples that are compared to blanks. However, seawater samples from the Cabo Verde islands were discriminated from commercial seawater samples. In addition, QCMIX22 replicates clustered together, which indicates reproducibility in the sample preparation method, high data quality, and adequate performance of the analytical platform. Moreover, overlapping of both types of QC samples (QCMIX22 and QCALL) suggested reproducibility in the sample extraction protocol. Solvent blanks from different mesh and different positions (spots) were clustered together, which suggests negligible cross-contamination in the analysis. Results provided by the t-SNE model (Fig. 2b), which is a nonlinear dimensionality reduction technique, were in agreement with those provided by the linear transformation-based technique of PCA and emphasized the reproducibility of the developed analytical method for seawater sample analysis. This was further evidenced by the visualization of sample replicate clusters in a t-SNE model that only included SML and ULW samples (Fig. S5).
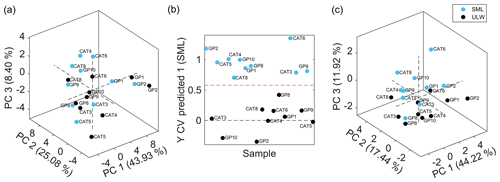
Figure 3(a) PCA score plot showing the first three principal components of sea surface microlayer samples (SML, light blue) and ultralow seawater samples (ULW, black). PCA was done based on 51 extracted features with averaged values from technical replicates. Accounted variance: PC 1, 43.93 %; PC 2, 25.08 %; and PC 3, 8.40 %. (b) Cross-validated (CV) prediction plot of orthogonal projections to latent structures discriminant analysis (OPLS-DA) model of SML samples (light blue) and ULW samples (black). The model consisted of 5 LVs with 82.19 % and 95.41 % total captured X-block and Y-block variances, respectively. The CV accuracy, sensitivity, and specificity were 100 %. (c) PCA score plot showing the first three principal components of SML samples (light blue) and ULW samples (black). PCA was done based on 11 discriminant features selected by the genetic algorithm. Variance accounted for PC 1, 44.22 %; PC 2, 17.44 %; and PC 3, 11.92 %.
To investigate the possibility of seawater sample clustering, a PCA model was built with the 51 extracted and curated features for averaged technical replicates of SML and ULW samples. Figure 3a shows the PCA score plot, including the first three principal components that accounted for 43.93 %, 25.08 %, and 8.40 % variance, respectively. No outliers were detected by this analysis, and no sample clustering was visualized in the score plot. Thus, sample discrimination was further attempted by means of OPLS-DA coupled to a GA variable selection method to find a reduced set of features that would allow for sample classification and class membership prediction. A panel of 11 features with the lowest RMSECV was selected through the GA process. Figure 3b shows the cross-validated prediction plot using the selected feature panel by means of a model that consisted of five latent variables that interpreted 82.19 % and 95.41 % variance from the X block (feature abundances) and Y block (class membership), respectively. This OPLS-DA model resulted in a 100 % cross-validated accuracy, sensitivity, and specificity; therefore, there was no sample misclassification. Sample classification was further evaluated by means of a nonsupervised method by using the 11 discriminant features to discard possible overfitting by the supervised multivariate model. Figure 3c shows a certain degree of sample separation into clusters in the PC3 dimension according to the seawater sample collection depth, i.e., SML or ULW.
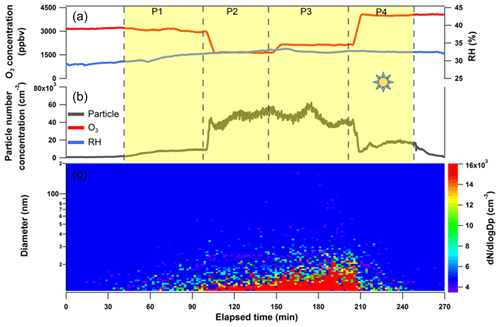
Figure 4Irradiation experiment for SML CAT 8 sample in a quartz cell and subsequent particle formation from the SML interfacial gaseous products via OH radical photochemistry in the OFR. The (a) O3 mixing ratio and humidity in the OFR; (b) particle concentration measured by CPC; and (c) particle size distribution profiles scanned by SMPS downstream of the OFR. The yellow shading represents the time period in which the quartz cell containing the concentrated SML sample was illuminated. P1 to P4 correspond to different operations to the OFR in varying oxidation degrees of the gaseous products from the quartz cell.
3.3 SOA formation potency from SML samples
A subset of SML samples (CAT 8, GP 10, CAT 6, CAT 3, and CAT 4) that were analyzed by the TM-DART-QTOF-MS seaomics strategy were also subject to on-site experiments during the field campaign by using a lab-to-field approach to test their SOA formation potency. The outcome of a typical SML irradiation experiment is illustrated for sample CAT 8 in Fig. 4. The different time periods (P) when the experimental parameters were modified along the experiment are indicated in the figure. In the absence of light (before P1), no particle formation was detected downstream of the preconditioned OFR (5.0 ppmv initial O3 and half-power UV light supply). However, when SML samples were exposed to actinic irradiation (periods P1–P4), particle formation was detected in the OFR254. Moreover, the particle number concentration exhibited trends that were dependent on OH exposure (OHexp; P2–P3). Gaseous products were probably generated from the photosensitized reactions at the SML interface and subsequently reacted with OH radicals in the OFR254, which led to particle formation.
Because of the difficulty associated with on-site measuring total OHR (OH radical reactivity) from the cell reactor or tracing OHexp in the OFR, we only tested the particle generation rates qualitatively with respect to various oxidation degrees, by changing the UV light intensity or O3 concentration in the OFR. Assuming that the photochemistry occurring at the SML interface was at a steady state, the air–water exchanged gaseous products were constantly entrained into the OFR, and the estimated particle generation rates/OHexp for each period followed the trend of . During P1, particle concentration gradually increased with SML illumination, and the final number concentration exceeded 8×103 cm−3. These particles exhibited a median diameter of several nanometers at the edge of the lower 10 nm size limit of the SMPS detection system; thus, measuring the particle size distribution was not possible. During P2, the UV light intensity was doubled in the OFR by turning all lamps on. A particle burst was detected by the UCPC, together with a shift towards larger particle sizes. The oxidation capacity in the OFR was further enhanced by supplying additional external O3 (initial mixing ratio of 7.0 ppmv). The total particle concentration decreased while larger particles were formed. During P4, one UV lamp in the OFR was turned off, and a sharp decrease in particle concentration was observed, but the final concentration was still higher than during P1 (Fig. 4). Particle formation was observed for CAT 8 and GP 10 SML samples. The results from the atmospheric simulation experiments conducted on SML samples were in agreement with previous laboratory studies that demonstrated air–sea interfacial-driven chemistry as a source of marine secondary aerosol (Roveretto et al., 2019; Ciuraru et al., 2015; Fu et al., 2015).
Table 1Identification of discriminant features based on accurate mass (a), isotopic pattern (b), and MS/MS experiments (c). Features with p<0.05 are highlighted in bold (Wilcoxon paired signed-rank test). Fold-change trends in binary comparisons are indicated with arrows: ↑ – increased levels and ↓ – decreased levels.
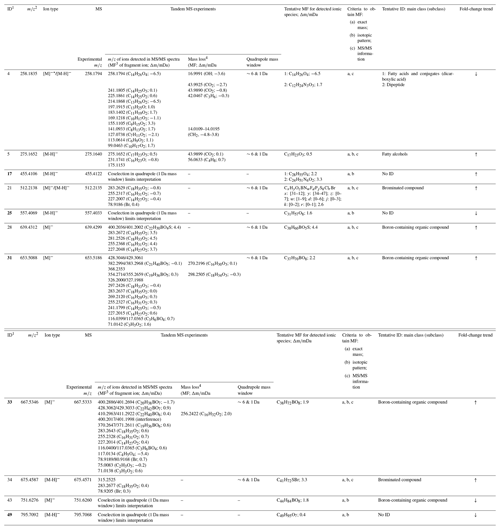
1 Feature code. 2 m∕z value obtained from Progenesis QI. 3 Molecular formula. 4 Possible neutral losses are indicated in parentheses.
3.4 Discriminant compound identification and role in aerosol particle formation
Compound identification was attempted for the 11 features of the discriminant panel. The coupling of the DART source to a high-resolution mass spectrometer allowed for the generation of elemental formulae for unknown compounds which, together with tandem MS capability, contributed to their identification. Figure S6 shows the high-resolution continuum mass spectra obtained for each of the discriminant features detected in all samples and obtained from the GA selection process. The analysis of fragment ions detected in tandem MS experiments, together with neutral loss analysis, provided information regarding the functional groups and contributed to filter molecular formulae obtained by accurate mass and isotopic pattern analysis. Table 1 describes the ionic species associated with the discriminant features and their corresponding molecular formulae, and it provides information about product ions and neutral and/or radical losses identified in TM-DART-QTOF-MS/MS experiments. In addition, the table includes the family of compounds identified with a certain confidence level. In general, discriminant features comprised saturated fatty acids, fatty alcohols, peptides, brominated compounds, and boron-containing organic compounds.
An expected limitation of TM-DART-QTOF-MS analysis was associated with the spectral overlap; thus, in some cases the isotopic pattern was not considered for compound identification. However, two different quadrupole-mass windows of 6 and 1 Da were used in tandem MS experiments to mitigate this problem. The mass window of 6 Da allowed for the investigation of the complete isotopic profile, with a high sensitivity at the expense of lower selectivity than the narrower mass window. In contrast, the mass window of 1 Da provided more confidence in the identification of product ions with higher selectivity at the expense of lower sensitivity than the broader mass window. In cases of low precursor ion intensity or quadrupole coselection, the MS/MS spectra were not collected (Table 1).
Different types of species were generated for desorbed and ionized analytes (M) by the plasma-based source operated in negative mode, including [M-H]−, [M]−, and [M]−• ionic species. The generation of a radical anion, [M]−•, was suggested for feature no. 4 based on the product ions detected in tandem MS experiments and the generated molecular formulae. Based on the tentative identification of feature no. 4, additional experiments were performed with chemical standards including a dicarboxylic acid (succinic acid) and saturated fatty acids under the same experimental conditions as for the seawater sample analysis. Different ionic species were detected in these experiments, except for radical anions. However, literature evidence suggests that the production of radical anions based on electron-capture mechanisms occurs in He-based plasma sources (Cody and Dane, 2016; Bridoux and Machuron-Mandard, 2013; Jorabchi et al., 2013).
Based on the analysis of the isotopic patterns and tandem MS results, several features were identified as oxygenated boron-containing organic compounds. In these compounds, the boron atom is speculated to be functionalized with saturated fatty acids yielding tetra coordinated boron esters that would generate [M]− anions. Boron-containing compounds are known to be ubiquitous in vascular plants, marine algal species, and microorganisms (Dembitsky et al., 2002). Four out of five features identified as boron-containing organic compounds functionalized with saturated fatty acids as well as features identified as fatty alcohols were enriched in the SML samples when compared to ULW samples (Table S4).
Compounds having a bromine atom in their molecular formula were also tentatively identified in the discriminant panel and are suggested to be halogenated compounds rather than bromine adduct ions. This hypothesis is based on the results yielded by the comparative analysis of a saturated acetonitrile solution with KBr and 2 mM phenol, and the analysis of an acetonitrile solution of 4-bromophenol (Fig. S7) that was used as a model compound. The [M-H]− ion was detected in the analysis of 4-bromophenol, but the [M + Br]− adduct ion was not observed for the KBr saturated solution containing phenol. The two features (nos. 21 and 34) that were identified as halogenated compounds were enriched in the SML samples (Table S4). Possible sources of halogenated compounds in the SML samples are photochemical reactions occurring at the air–water interface (Roveretto et al., 2019; Donaldson and George, 2012). It is worth noting that organic compounds identified in the discriminant panel may have derived both from the secreted (exometabolome) and/or intracellular metabolites (endometabolome) of biological organisms, such as algal species and microorganisms present in seawater, since the samples were not filtered. In a real environment, therefore, some of these compounds may be present in lower levels than those detected in the present work, or they may not be available to participate in the sea surface secondary organic aerosol (SOA) chemistry.
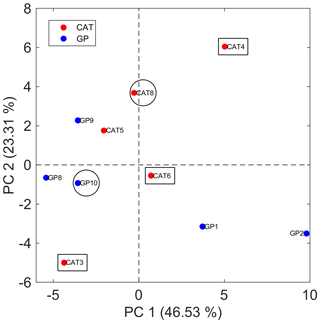
Figure 5Bidimensional PCA score plot for SML samples using the matrix with 51 features for averaged technical replicates. Samples that were evaluated for particle formation during the Cabo Verde field campaign are indicated with circles (led to SOA formation) and rectangles (did not lead to SOA formation).
Putative identification of the discriminant panel capable of differentiating SML from ULW samples provides further evidence to support SOA formation detected by the lab-to-field approach during the campaign. The PCA score plot illustrated in Fig. 5 shows that SML samples were not distinguished based on the collection method, i.e., GP or CAT, and points out those SML samples that were also evaluated for SOA formation during the field campaign. As previously discussed, two of these SML samples (CAT 8 and GP 10) yielded SOA formation (Fig. 4). Since CAT 8 and GP 10 were separated in the bidimensional score map from the group formed from CAT 3, CAT 4, and CAT 6, a further PCA model was built only with those samples (n=5) that were analyzed by both TM-DART-QTOF-MS and the lab-to-field approach (Fig. S8). Figure S8a shows that PC2 clearly separates samples according to SOA formation. Four out of seven features that mainly contribute to sample class separation with the largest absolute values in the loadings plot associated with PC2, and illustrated in Fig. S8B, were putatively identified as boron-containing organic compounds (Table S5). Despite the limitations associated with the low number of samples used to perform statistical analysis, the results suggest that SML samples that led to particle formation were enriched on boron-containing organic compounds and other unidentified molecules (Table S5).
An untargeted TM-DART-QTOF-MS-based analytical method coupled to multivariate statistical analysis allowed for the analysis of organic compounds present in the SML and ULW seawater samples collected during a field campaign at the Cabo Verde islands without the need for desalinization. This seaomics approach was successfully implemented to discriminate the SML from ULW samples. Tentative identification of the discriminant metabolite panel suggests that halogenated compounds, fatty alcohols, and oxygenated boron-containing organic compounds are available for air–water transfer processes and photochemical reactions at the air–water interface of the ocean. Combined results from TM-DART-QTOF-MS and on-site SOA formation testing experiments on SML samples suggest that organic compounds enriched at the air–water interface may be contributing to the differential SOA-forming ability of SML samples. This strategy, implemented for the first time in this collaborative study, provides new opportunities for improving the characterization of seawater OM content, and discovering compounds involved in aerosol formation processes.
The mass spectrometry data have been deposited in the MetaboLights public repository, with the data set identifier MTBLS1198 (https://www.ebi.ac.uk/metabolights/MTBLS1198, last access: 25 May 2020) (Zabalegui et al., 2020).
The supplement related to this article is available online at: https://doi.org/10.5194/acp-20-6243-2020-supplement.
MEM, MvP, HH, and CG designed the collaborative study. MvP and HH designed the sample collection methods. MM processed the samples until they were stored at −80 ∘C. MEM, MM, and NZ developed the TM-DART-MS-based seaomics strategy and analyzed the data. MEM, NZ, MM, AD, NH, and CG contributed to optimizing the TM-DART-MS-based analytical method. NZ and MM conducted TM-DART-MS and MS/MS experiments. MR, CL, and CG conducted on-site aerosol particle formation experiments. MEM, NZ, and CG wrote the paper. All authors revised the paper.
The authors declare that they have no conflict of interest.
This article is part of the special issue “Marine organic matter: from biological production in the ocean to organic aerosol particles and marine clouds (ACP/OS inter-journal SI)”. It is not associated with a conference.
María Eugenia Monge is a research staff member from CONICET (Consejo Nacional de Investigaciones Científicas y Técnicas, Argentina). Nadja Triesch and Sebastian Zeppenfeld from the TROPOS Atmospheric Chemistry Department (ACD) are acknowledged for their support during the SML collection and sample preparation. Coretta Bauer from the UFZ Helmholtz Centre for Environmental Research is acknowledged for assisting with sample lyophilization.
This research has been supported by the Marie Skłodowska-Curie Actions (MSCA) Research and Innovation Staff Exchange (RISE), and Horizon 2020 (H2020-MSCA-RISE-2015; grant no. 690958), which finances the European “MARSU” network. (MARSU represents the “MARine atmospheric Science Unravelled: analytical and mass spectrometric techniques development and application”.) Funding was also provided by the Argentine National Mass Spectrometry System (SNEM), CONICET, Ministerio de Ciencia, Tecnología e Innovación (MINCyT; grant no. project E-AC12), and the Leibniz Association Senatsausschuss Wettbewerb (SAW) project MarParCloud (grant no. SAW-2016-TROPOS-2).
This paper was edited by Paul Zieger and reviewed by three anonymous referees.
Bayliss, N. S. and Bucat, R. B.: Photolysis of Aqueous Nitrate Solutions, Aust. J. Chem., 28, 1865–1878, 1975.
Bridoux, M. C. and Machuron-Mandard, X.: Capabilities and limitations of direct analysis in real time orbitrap mass spectrometry and tandem mass spectrometry for the analysis of synthetic and natural polymers, Rapid Commun. Mass Spectrom., 27, 2057–2070, https://doi.org/10.1002/rcm.6664, 2013.
Brüggemann, M., Hayeck, N., and George, C.: Interfacial photochemistry at the ocean surface is a global source of organic vapors and aerosols, Nat. Commun., 9, 2101, https://doi.org/10.1038/s41467-018-04528-7, 2018.
Bylesjö, M., Rantalainen, M., Cloarec, O., Nicholson, J. K., Holmes, E., and Trygg, J.: OPLS discriminant analysis: combining the strengths of PLS-DA and SIMCA classification, J. Chemom., 20, 341–351, https://doi.org/10.1002/cem.1006, 2006.
Cajka, T., Riddellova, K., Tomaniova, M., and Hajslova, J.: Ambient mass spectrometry employing a DART ion source for metabolomic fingerprinting/profiling: a powerful tool for beer origin recognition, Metabolomics, 7, 500–508, https://doi.org/10.1007/s11306-010-0266-z, 2011.
Chernetsova, E. S., Bochkov, P. O., Ovcharov, M. V., Zhokhov, S. S., and Abramovich, R. A.: DART mass spectrometry: a fast screening of solid pharmaceuticals for the presence of an active ingredient, as an alternative for IR spectroscopy, Drug Test. Anal., 2, 292–294, https://doi.org/10.1002/dta.136, 2010.
Ciuraru, R., Fine, L., van Pinxteren, M., D'Anna, B., Herrmann, H., and George, C.: Photosensitized production of functionalized and unsaturated organic compounds at the air-sea interface, Sci. Rep.-UK, 5, 12741, https://doi.org/10.1038/srep12741, 2015.
Clendinen, C. S., Monge, M. E., and Fernandez, F. M.: Ambient mass spectrometry in metabolomics, Analyst, 142, 3101–3117, https://doi.org/10.1039/c7an00700k, 2017.
Cody, R. B. and Dane, A. J.: Soft Ionization of Saturated Hydrocarbons, Alcohols and Nonpolar Compounds by Negative-Ion Direct Analysis in Real-Time Mass Spectrometry, J. Am. Soc. Mass Spectr., 24, 329–334, https://doi.org/10.1007/s13361-012-0569-6, 2013.
Cody, R. B. and Dane, A. J.: Dopant-assisted direct analysis in real time mass spectrometry with argon gas, Rapid Commun. Mass Spectrom., 30, 1181–1189, https://doi.org/10.1002/rcm.7552, 2016.
Cody, R. B., Laramee, J. A., and Durst, H. D.: Versatile new ion source for the analysis of materials in open air under ambient conditions, Anal. Chem., 77, 2297–2302, https://doi.org/10.1021/ac050162j, 2005.
Dembitsky, V. M., Smoum, R., Al-Quntar, A. A., Ali, H. A., Pergament, I., and Srebnik, M.: Natural occurrence of boron-containing compounds in plants, algae and microorganisms, Plant Sci., 163, 931–942, https://doi.org/10.1016/S0168-9452(02)00174-7, 2002.
Donaldson, D. J. and George, C.: Sea-Surface Chemistry and Its Impact on the Marine Boundary Layer, Environ. Sci. Technol., 46, 10385–10389, https://doi.org/10.1021/es301651m, 2012.
Dove, A. D. M., Leisen, J., Zhou, M., Byrne, J. J., Lim-Hing, K., Webb, H. D., Gelbaum, L., Viant, M. R., Kubanek, J., and Fernández, F. M.: Biomarkers of Whale Shark Health: A Metabolomic Approach, PloS one, 7, e49379, https://doi.org/10.1371/journal.pone.0049379, 2012.
Dunn, W. B., Broadhurst, D., Begley, P., Zelena, E., Francis-McIntyre, S., Anderson, N., Brown, M., Knowles, J. D., Halsall, A., Haselden, J. N., Nicholls, A. W., Wilson, I. D., Kell, D. B., and Goodacre, R.: Procedures for large-scale metabolic profiling of serum and plasma using gas chromatography and liquid chromatography coupled to mass spectrometry, Nat. Protoc., 6, 1060–1083, https://doi.org/10.1038/nprot.2011.335, 2011.
Facchini, M. C., Rinaldi, M., Decesari, S., Carbone, C., Finessi, E., Mircea, M., Fuzzi, S., Ceburnis, D., Flanagan, R., Nilsson, E. D., de Leeuw, G., Martino, M., Woeltjen, J., and O' Dowd, C. D.: Primary submicron marine aerosol dominated by insoluble organic colloids and aggregates, Geophys. Res. Lett., 35, L17814, https://doi.org/10.1029/2008GL034210, 2008.
Fernández, F. M., Cody, R. B., Green, M. D., Hampton, C. Y., McGready, R., Sengaloundeth, S., White, N. J., and Newton, P. N.: Characterization of Solid Counterfeit Drug Samples by Desorption Electrospray Ionization and Direct-analysis-in-real-time Coupled to Time-of-flight Mass Spectrometry, Chem. Med. Chem., 1, 702–705, https://doi.org/10.1002/cmdc.200600041, 2006.
Fiehn, O., Kopka, J., Dormann, P., Altmann, T., Trethewey, R. N., and Willmitzer, L.: Metabolite profiling for plant functional genomics, Nat. Biotechnol., 18, 1157–1161, https://doi.org/10.1038/81137, 2000.
Fu, H., Ciuraru, R., Dupart, Y., Passananti, M., Tinel, L., Rossignol, S., Perrier, S., Donaldson, D. J., Chen, J., and George, C.: Photosensitized Production of Atmospherically Reactive Organic Compounds at the Air/Aqueous Interface, J. Am. Chem. Soc., 137, 8348–8351, https://doi.org/10.1021/jacs.5b04051, 2015.
Garabetian, F., Romano, J. C., Paul, R., and Sigoillot, J. C.: Organic matter composition and pollutant enhancement of sea surface microlayer material inside and outside of sliks, Mar. Environ. Res., 35, 323–329, https://doi.org/10.1016/0141-1136(93)90100-E, 1993.
Gershy, R. M.: Characterization of seawater organic matter carried by bubble-generated aerosols, Limnol. Oceanogr., 28, 309–319, https://doi.org/10.4319/lo.1983.28.2.0309, 1983.
Gross, J. H.: Direct analysis in real time-a critical review on DART-MS, Anal. Bioanal. Chem., 406, 63–80, https://doi.org/10.1007/s00216-013-7316-0, 2014.
Guitart, C., Garcia-Flor, N., Dachs, J., Bayona, J. M., and Albaiges, J.: Evaluation of sampling devices for the determination of polycyclic aromatic hydrocarbons in surface microlayer coastal waters, Mar. Pollut. Bull., 48, 961–968, https://doi.org/10.1016/j.marpolbul.2003.12.002, 2004.
Hajslova, J., Cajka, T., and Vaclavik, L.: Challenging applications offered by direct analysis in real time (DART) in food-quality and safety analysis, TrAC, Trends Anal. Chem., 30, 204–218, https://doi.org/10.1016/j.trac.2010.11.001, 2011.
Hardy, J. T.: The sea-surface microlayer: biology, chemistry and anthropogenic enrichment, Prog. Oceanogr., 11, 307–328, https://doi.org/10.1016/0079-6611(82)90001-5, 1982.
Harris, G. A., Galhena, A. S., and Fernandez, F. M.: Ambient Sampling/Ionization Mass Spectrometry: Applications and Current Trends, Anal. Chem., 83, 4508–4538, https://doi.org/10.1021/ac200918u, 2011.
Hunter, K. A. and Liss, P. S.: The Input of Organic Material to Oceans – Air-Sea Interactions and Organic Chemical Composition of Sea-Surface, Mar. Chem., 5, 361–379, https://doi.org/10.1016/0304-4203(77)90029-9, 1977.
Ifa, D. R., Jackson, A. U., Paglia, G., and Cooks, R. G.: Forensic applications of ambient ionization mass spectrometry, Anal. Bioanal. Chem., 394, 1995–2008, https://doi.org/10.1007/s00216-009-2659-2, 2009.
Johnson, R. A. and Wichern, D. W.: Applied Multivariate Statistical Analysis, (Sixth edition), New Jersey, ISBN 0-13-187715-1, Pearson Education, Inc., 2007.
Jones, C. M. and Fernández, F. M.: Transmission mode direct analysis in real time mass spectrometry for fast untargeted metabolic fingerprinting, Rapid Commun. Mass Spectrom., 27, 1311–1318, https://doi.org/10.1002/rcm.6566, 2013.
Jones, C. M., Monge, M. E., and Fernandez, F. M.: Metabolite profiling by direct analysis in real-time mass spectrometry, in: Mass Spectrometry in Metabolomics, edited by: Raftery, D., Methods in Molecular Biology (Methods and Protocols), Humana Press, New York, NY, 1198, https://doi.org/10.1007/978-1-4939-1258-2_18, 2014.
Jorabchi, K., Hanold, K., and Syage, J.: Ambient analysis by thermal desorption atmospheric pressure photoionization, Anal. Bioanal. Chem., 405, 7011–7018, https://doi.org/10.1007/s00216-012-6536-z, 2013.
Kaylor, A., Dwivedi, P., Pittman, J. J., Monge, M. E., Cheng, G., Li, S., and Fernandez, F. M.: Plasma-spray ionization (PLASI): a multimodal atmospheric pressure ion source for liquid stream analysis, J. Am. Soc. Mass Spectrom., 25, 1788–1793, https://doi.org/10.1007/s13361-014-0948-2, 2014.
Kovac, N., Bajt, O., Faganeli, J., Sket, B., and Orel, B.: Study of macroaggregate composition using FT-IR and H-1-NMR spectroscopy, Marine Chem., 78, 205–215, 2002.
Liss, P. S.: The chemistry of near-surface seawater, in: Dynamic processes in the chemistry of the upper ocean, edited by: Chesselet, R., Plenum, New York, 41–51, 1986.
Liss, P. S. and Duce, R. A. (Eds.): The Sea Surface and Global Change, J. Marine Biol. Assoc. UK, 77, 918, https://doi.org/10.1017/S0025315400036407, 1997.
McEwen, C. N. and Larsen, B. S.: Ionization Mechanisms Related to Negative Ion APPI, APCI, and DART, J. Am. Soc. Mass Spectr., 20, 1518–1521, https://doi.org/10.1016/j.jasms.2009.04.010, 2009.
Monge, M. E. and Fernández, F. M.: An Introduction to Ambient Ionization Mass Spectrometry, in: Ambient Ionization Mass Spectrometry, edited by: Domin, M. A. and Cody, R. B., The Royal Society of Chemistry, RSC Publishing, Cambridge, https://doi.org/10.1039/9781782628026-00001, 2014.
Monge, M. E., Harris, G. A., Dwivedi, P., and Fernandez, F. M.: Mass spectrometry: recent advances in direct open air surface sampling/ionization, Chem. Rev., 113, 2269–2308, https://doi.org/10.1021/cr300309q, 2013.
Nicholson, J. K. and Lindon, J. C.: Systems biology: Metabonomics, Nature, 455, 1054–1056, https://doi.org/10.1038/4551054a, 2008.
Perez, J. J., Harris, G. A., Chipuk, J. E., Brodbelt, J. S., Green, M. D., Hampton, C. Y., and Fernandez, F. M.: Transmission-mode direct analysis in real time and desorption electrospray ionization mass spectrometry of insecticide-treated bednets for malaria control, Analyst, 135, 712–719, https://doi.org/10.1039/b924533b, 2010.
Reeser, D. I., George, C., and Donaldson, D. J.: Photooxidation of Halides by Chlorophyll at the Air-Salt Water Interface, J. Phys. Chem. A, 113, 8591–8595, https://doi.org/10.1021/jp903657j, 2009a.
Reeser, D. I., Jammoul, A., Clifford, D., Brigante, M., D'Anna, B., George, C., and Donaldson, D. J.: Photoenhanced Reaction of Ozone with Chlorophyll at the Seawater Surface, J. Phys. Chem. C, 113, 2071–2077, https://doi.org/10.1021/jp805167d, 2009b.
Rossignol, S., Tinel, L., Bianco, A., Passananti, M., Brigante, M., Donaldson, D. J., and George, C.: Atmospheric photochemistry at a fatty acid-coated air-water interface, Science, 353, 699, https://doi.org/10.1126/science.aaf3617, 2016.
Roveretto, M., Li, M., Hayeck, N., Brüggemann, M., Emmelin, C., Perrier, S., and George, C.: Real-Time Detection of Gas-Phase Organohalogens from Aqueous Photochemistry Using Orbitrap Mass Spectrometry, ACS Earth Space Chem., 3, 329–334, https://doi.org/10.1021/acsearthspacechem.8b00209, 2019.
Saccenti, E., Hoefsloot, H. C. J., Smilde, A. K., Westerhuis, J. A., and Hendriks, M. M. W. B.: Reflections on univariate and multivariate analysis of metabolomics data, Metabolomics, 10, 361–374, https://doi.org/10.1007/s11306-013-0598-6, 2014.
Salter, T. L., Green, F. M., Gilmore, I. S., Seah, M. P., and Stokes, P.: A comparison of SIMS and DESI and their complementarities, Surface and Interface Analysis, 43, 294–297, https://doi.org/10.1002/sia.3412, 2011.
Schneider, J. K. and Gagosian, R. B.: Particle size distribution of lipids in aerosols off the coast of Peru, J. Geophys. Res.-Atmos., 90, 7889–7898, https://doi.org/10.1029/JD090iD05p07889, 1985.
Shrestha, B. and Vertes, A.: Direct Analysis of Single Cells by Mass Spectrometry at Atmospheric Pressure, J. Vis. Exp., 43, e2144, https://doi.org/10.3791/2144, 2010.
Song, L., Dykstra, A. B., Yao, H., and Bartmess, J. E.: Ionization mechanism of negative ion-direct analysis in real time: A comparative study with negative ion-atmospheric pressure photoionization, J. Am. Soc. Mass. Spectrom., 20, 42–50, https://doi.org/10.1016/j.jasms.2008.09.016, 2009a.
Song, L., Gibson, S. C., Bhandari, D., Cook, K. D., and Bartmess, J. E.: Ionization mechanism of positive-ion direct analysis in real time: a transient microenvironment concept, Anal. Chem., 81, 10080–10088, https://doi.org/10.1021/ac901122b, 2009b.
Steiner, R. R. and Larson, R. L.: Validation of the Direct Analysis in Real Time Source for Use in Forensic Drug Screening, J. Forens. Sci., 54, 617–622, https://doi.org/10.1111/j.1556-4029.2009.01006.x, 2009.
Tang, K., Page, J. S., and Smith, R. D.: Charge competition and the linear dynamic range of detection in electrospray ionization mass spectrometry, J. Am. Soc. Mass Spectr., 15, 1416–1423, https://doi.org/10.1016/j.jasms.2004.04.034, 2004.
Trygg, J. and Wold, S.: Orthogonal projections to latent structures (O-PLS), J. Chemom., 16, 119–128, https://doi.org/10.1002/cem.695, 2002.
Trygg, J., Holmes, E., and Lundstedt, T.: Chemometrics in metabonomics, J. Proteome Res., 6, 469–479, https://doi.org/10.1021/pr060594q, 2007.
Van Der Maaten, L. and Hinton, G.: Visualizing data using t-SNE, J. Mach. Learn. Res., 9, 2579–2625, 2008.
van Pinxteren, M., Müller, C., Iinuma, Y., Stolle, C., and Herrmann, H.: Chemical Characterization of Dissolved Organic Compounds from Coastal Sea Surface Microlayers (Baltic Sea, Germany), Environ. Sci. Technol., 46, 10455–10462, https://doi.org/10.1021/es204492b, 2012.
van Pinxteren, M., Fomba, K. W., Triesch, N., Stolle, C., Wurl, O., Bahlmann, E., Gong, X., Voigtländer, J., Wex, H., Robinson, T.-B., Barthel, S., Zeppenfeld, S., Hoffmann, E. H., Roveretto, M., Li, C., Grosselin, B., Daële, V., Senf, F., van Pinxteren, D., Manzi, M., Zabalegui, N., Frka, S., Gašparović, B., Pereira, R., Li, T., Wen, L., Li, J., Zhu, C., Chen, H., Chen, J., Fiedler, B., von Tümpling, W., Read, K. A., Punjabi, S., C. Lewis, A. C., Hopkins, J. R., Carpenter, L. J., Peeken, I., Rixen, T., Schulz-Bull, D., Monge, M. E., Mellouki, A., George, C., Stratmann, F., and Herrmann, H.: Marine organic matter in the remote environment of the Cape Verde Islands – An introduction and overview to the MarParCloud campaign, Atmos. Chem. Phys. Discuss., https://doi.org/10.5194/acp-2019-997, in review, 2019.
Viant, M. R., Ebbels, T. M. D., Beger, R. D., Ekman, D. R., Epps, D. J. T., Kamp, H., Leonards, P. E. G., Loizou, G. D., MacRae, J. I., van Ravenzwaay, B., Rocca-Serra, P., Salek, R. M., Walk, T., and Weber, R. J. M.: Use cases, best practice and reporting standards for metabolomics in regulatory toxicology, Nat. Commun., 10, 3041, https://doi.org/10.1038/s41467-019-10900-y, 2019.
Weckwerth, W. and Morgenthal, K.: Metabolomics: from pattern recognition to biological interpretation, Drug Discovery Today, 10, 1551–1558, https://doi.org/10.1016/S1359-6446(05)03609-3, 2005.
Williams, P. M., Carlucci, A. F., Henrichs, S. M., Van Fleet, E. S., Horrigan, S. G., Reid, F. M. H., and Robertson, K. J.: Chemical and microbiological studies of sea-surface films in the southern Gulf of California and off the west coast of Baja California, Mar. Chem., 19, 17–98, https://doi.org/10.1016/0304-4203(86)90033-2, 1986.
Zabalegui, N., Manzi, M., Depoorter, A., Hayeck, N., Roveretto, M., Li, C., van Pinxteren, M., Herrmann, H., George, C., and Monge, M. E.: MTBLS1198: Seawater Analysis by Ambient Mass Spectrometry-Based Seaomics, available at: https://www.ebi.ac.uk/metabolights/MTBLS1198 (last access: 25 May 2020), MetaboLights, 2020.
Zang, X., Pérez, J. J., Jones, C. M., Monge, M. E., McCarty, N. A., Stecenko, A. A., and Fernández, F. M.: Comparison of Ambient and Atmospheric Pressure Ion Sources for Cystic Fibrosis Exhaled Breath Condensate Ion Mobility-Mass Spectrometry Metabolomics, J. Am. Soc. Mass Spectr., 28, 1489–1496, https://doi.org/10.1007/s13361-017-1660-9, 2017.
Zhou, M., Guan, W., Walker, L. D., Mezencev, R., Benigno, B. B., Gray, A., Fernández, F. M., and McDonald, J. F.: Rapid Mass Spectrometric Metabolic Profiling of Blood Sera Detects Ovarian Cancer with High Accuracy, Cancer Epidemiol. Biomarkers Prev., 19, 2262–2271, https://doi.org/10.1158/1055-9965.epi-10-0126, 2010a.
Zhou, M., McDonald, J. F., and Fernandez, F. M.: Optimization of a direct analysis in real time/time-of-flight mass spectrometry method for rapid serum metabolomic fingerprinting, J. Am. Soc. Mass Spectrom., 21, 68–75, https://doi.org/10.1016/j.jasms.2009.09.004, 2010b.