the Creative Commons Attribution 4.0 License.
the Creative Commons Attribution 4.0 License.
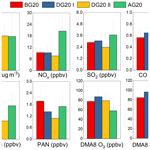
Exploring the inconsistent variations in atmospheric primary and secondary pollutants during the 2016 G20 summit in Hangzhou, China: implications from observations and models
Gen Zhang
Honghui Xu
Hongli Wang
Likun Xue
Jianjun He
Wanyun Xu
Rongguang Du
Chang Liu
Zeyuan Li
Wanting Jiang
Linlin Liang
Yan Yan
Xiaoyan Meng
Complex aerosol and photochemical pollution (ozone and peroxyacetyl nitrate, PAN) frequently occur in eastern China, and mitigation strategies to effectively alleviate both kinds of pollution are urgently needed. Although the effectiveness of powerful control measures implemented by the Chinese State Council has been comprehensively evaluated in terms of reducing atmospheric primary pollutants, the effectiveness in mitigating photochemical pollution is less assessed and therefore the underlying mechanisms are still poorly understood. The stringent emission controls implemented from 24 August to 6 September 2016 during the summit for the Group of Twenty (G20) provide us a unique opportunity to address this issue. Surface concentrations of atmospheric O3, PAN, and their precursors including volatile organic compounds (VOCs) and nitrogen dioxides (NOx), in addition to the other trace gases and particulate matter, were measured at the National Reference Climatological Station (NRCS) (30.22∘ N, 120.17∘ E, 41.7 m a.s.l) in urban Hangzhou. We found significant decreases in atmospheric PAN, NOx, total VOCs, PM2.5, and sulfur dioxide (SO2) under the unfavorable meteorological conditions during G20 (DG20) relative to the adjacent period before and after G20 (BG20 and AG20), indicating that the powerful control measures were effective in reducing the pollutant emissions in Hangzhou. Unlike with the other pollutants, daily maximum 8 h average (DMA8) O3 exhibited a slight increase and then decrease from BG20 to AG20, which was mainly attributed to the variation in the solar irradiation intensity and regional transport in addition to the contribution from the implementation of stringent control measures. Results from an observation-based chemical model (OBM) indicated that acetaldehyde and methylglyoxal (MGLY) were the most important second-generation precursors of PAN, accounting for 37.3 %–51.6 % and 22.8 %–29.5 % of the total production rates including the reactions of OVOCs, propagation of other radicals, and other minor sources. Moreover, we confirmed the production of PAN and O3 was sensitive to VOCs throughout the whole period, specifically dominated by aromatics in BG20 and DG20 but by alkenes in AG20. These findings suggested that reducing emissions of aromatics, alkenes, and alkanes would mitigate photochemical pollution including PAN and O3. Source appointment results attributed the reductions of VOC source and ozone formation potentials (OFPs) during G20 to the effective emission controls on traffic (vehicle exhaust) and industrial processes (solvent utilization and industrial manufacturing). However, fuel combustion and biogenic emissions both weakened such an effect with a sizable contribution to the VOC mixing ratios (18.8 % and 20.9 %) and OFPs (25.6 % and 17.8 %), especially during the latter part of G20 (G20 II) when anthropogenic VOCs were substantially reduced. This study highlights the effectiveness of stringent emission controls in relation to traffic and industrial sources, but a coordinated program related to controlling fuel combustion and biogenic emissions is also required to address secondary pollution.
- Article
(4188 KB) - Full-text XML
-
Supplement
(826 KB) - BibTeX
- EndNote
Complex atmospheric pollution including particulate matter and photochemical pollution (ozone, O3; peroxyacetyl nitrate, PAN) is a pervasive environmental issue in eastern China (Geng et al., 2007; Ding et al., 2013; Mo et al., 2015; Li et al., 2016; Zhang et al., 2018). Numerous mitigation strategies have been released by the Chinese government, such as the nationwide application of flue-gas desulfurization (FGD) devices in power plants after 2006 (Feng et al., 2014) and the “Atmospheric Pollution Prevention and Control Action Plan” in 2013 (Zhang et al., 2016). As expected, ambient concentrations of primary gas pollutants such as sulfur dioxide (SO2) (Koukouli et al., 2016) and nitrogen oxides () (de Foy et al., 2016) showed a good response to emission reductions. However, secondary atmospheric pollutants such as ozone and secondary aerosols frequently exceeded their respective Chinese Grade II standards over urban cities in China (Wang et al., 2014). Severe haze pollution, mainly comprised of PM2.5 (particles within the 2.5 µm diameter range), still occur in China during wintertime, although it started to decline during the 11th Five-Year Plan period (Huang et al., 2014; Cheng et al., 2016; Miao et al., 2018; Miao and Liu, 2019). Surface O3 has also exhibited a rapid increasing trend over China since 2000 (Verstraeten et al., 2015; Wang et al., 2017), with high levels (9.5–14.0 ppbv) of PAN often encountered during O3 pollution events (Shao et al., 2009; Liu et al., 2010; G. Zhang et al., 2012, 2014, 2015; Xue et al., 2014c). Due to the highly nonlinear response of O3 and PAN to primary pollutant emissions, in addition to intricate photochemical reactions, the mitigation of secondary photochemical pollution is even more challenging. In the troposphere, O3 and PAN are both formed in photochemical reactions of VOCs in the presence of NOx. However, PAN is exclusively formed by the oxidation of a small part of VOCs that can generate peroxy acetyl radical (CH3C(O)O2, PA) including oxygenated VOCs (OVOCs) such as acetaldehyde, acetone, methacrolein (MACR), methyl vinyl ketone (MVK), and methylglyoxal (MGLY) (Williams et al., 2000; LaFranchi et al., 2009), while O3 formation involves almost all VOCs. Therefore, PAN is considered to be a better indicator of photochemical smog than O3 (McFadyen and Cape, 2005). In addition, these OVOCs are mainly oxidation products (here referred to as secondary precursors of PAN) of a certain class of hydrocarbons (e.g., ethane, propene, isoprene, and some aromatics) through the oxidation of OH, NO3, and O3. The relative importance of individual precursors to the formation of PAN and O3 varies from place to place depending on the reactivity and composition of VOCs. Identification of the dominant precursors is the key to effective control of photochemical pollution, which, however, remains poorly characterized in China.
Recently, a series of temporary and stringent emission control measures were implemented in China during several mega-events including the 29th Summer Olympic Games (August 2008), the 21st Asia-Pacific Economic Cooperation (APEC) conference (November 2014), and the China Victory Day Parade (Victory Parade 2015) in Beijing (Verstraeten et al., 2015) and the surrounding areas (Xu et al., 2010; Y. J. Zhang et al., 2012; Gao et al., 2011; Li et al., 2017). During these events, the effectiveness of a series of emission control measures in reducing atmospheric primary pollutants, in particular the particulate matter, has been comprehensively evaluated, but less so for photochemical pollution.
In September 2016, the Group of Twenty (G20) summit was hosted in Hangzhou, the capital city of Zhejiang Province, which is located along the central Yangtze River Delta (YRD) in the eastern part of China. Similar to other major events held in Beijing, rigorous temporal control measures were set to reduce emissions of air pollutants in Hangzhou and the adjacent regions including Zhejiang, Shanghai, Jiangsu, and Anhui provinces from 24 August to 7 September including phase I (24–27 August) and phase II (28 August–6 September). These control measures included restrictions on the number of vehicles, limited production or complete shutdown of industrial enterprises, and temporary cessation of construction activities, and the target sources included vehicles, paint and solvent use, steel factories, chemical factories, and power plants. During phase I the government implemented strict emission control measures on industrial sources, power plants, and residential activities and phase II referred to the additional control measures on vehicles in the Hangzhou and the surrounding provinces (including Zhejiang, Jiangsu, Jiangxi, and Anhui).
In this study, to evaluate the effectiveness of emission control measures on reducing pollutant concentrations, we compared the variations in atmospheric O3, PAN, particulate matter, VOCs, NOx, and other trace gases before, during, and after G20, also demonstrating the effect of meteorological conditions by using the WRF-Chem model. An observation-based chemical box model (OBM) was used to identify the predominant precursors and key chemical processes in PAN and O3 formation and to further assess the effect of reducing their respective precursors before, during, and after G20. Positive matrix factorization (PMF) was employed to appoint the corresponding sources of various VOCs and compare their variations and their respective ozone formation potentials (OFPs) before, during, and after G20.
2.1 Observations
In situ observations of atmospheric PAN, O3, and VOCs and a suite of associated chemical species and meteorological parameters, including NOx, CO, SO2, and fine particulate matter (PM2.5), were conducted at an urban site named the National Reference Climatological Station (NRCS) (30.22∘ N, 120.17∘ E, 41.7 m a.s.l) in the center of Hangzhou as shown in Fig. 1. PAN was measured by a modified gas chromatograph (Agilent 7890B, USA) equipped with an electron capture detector, which has been described in our previous studies in detail (G. Zhang et al., 2012; Zhang et al., 2014, 2015). Trace gases including O3, SO2, NOx, and CO were detected by a set of commercial trace gas analyzers (Thermo Environmental Instruments Inc., USA, i-series 49i, 43i, 42i, and 48i, respectively; Zhang et al., 2018). All trace gas analyzers were calibrated weekly and zero checked daily. Ambient VOCs were measured by using an online gas chromatographer (Syntech Spectras Instrument Co., Ltd., the Netherlands) coupled with dual detectors (photoionization detector, PID, and flame ionization detector, FID) for quantifying C2–C5 VOCs (GC955 series 811) and PID for detecting C6–C12 VOCs (GC955 series 611). Ambient PM2.5 samples were collected using co-located Thermo Scientific (formerly R&P) model 1405D samplers. Coarse PM and PM2.5, split by a virtual impactor, each accumulate on the system's exchangeable tapered element oscillating microbalance (TEOM) filters. By maintaining a flow rate of 1.67 L min−1 through the coarse sample flow channel and 3 L min−1 through the PM2.5 sample channel, and measuring the total mass accumulated on each of the TEOM filters, the device can calculate the mass concentration of both the PM2.5 and coarse PM sample streams in near-real time.
2.2 Models
2.2.1 WRF-Chem model
To quantify the separate effects of meteorological conditions (EMCs) and emission control measures (ECCs) on observed particulate concentrations, we performed simulations using the Weather Research and Forecasting model coupled to Chemistry (WRF-Chem). WRF-Chem V3.9 was used to simulate the variation in PM2.5 concentration from 6 August 2016 00:00 UTC to 16 September 2016 00:00 UTC. The Multi-resolution Emission Inventory for China at 0.25∘ in 2016, developed by Tsinghua University (http://www.meicmodel.org/, last access: 2 May 2020), was used as input for WRF-Chem. WRF-Chem was configured to have two nested domains, i.e., an outer domain with horizontal resolution of 25 km (140×100 grid points) covering east China and the surrounding areas and an inner domain with 5 km resolution (101×101 grid points) covering the Yangtze River Delta. Hangzhou is located in the center of the domain. Vertically, there were a total of 35 layers extending to the model top at 50 hPa, with 16 levels below 2 km. The National Centers for Environmental Prediction (NCEP) Final Operational Global Analysis (FNL) data available at every 6 h were used for meteorological driving fields. Analysis nudging was used for domain one. The RADM2 chemical mechanism and MADE/SORGAM aerosols were used in this study. Here we assumed no significant change in chemical processes (specifically the other reactive gases involved in the chemical reactions with these pollutants) from BG20 to AG20. Thereby, the net contribution (NCC) of emission controls and meteorological conditions primarily results in the difference between observed PM2.5 before and during G20, which is represented by the ratio of observed PM2.5 (BG20) minus observed PM2.5 (DG20 II) to observed PM2.5 (BG20). The effect of meteorological conditions (EMCs) was quantified by comparing the modeled PM2.5 without emission controls before and during G20 under their respective meteorological conditions (Eq. 1). Thereby, the effect of emission controls (ECCs) could be obtained through the difference between NCC and EMCs before and during G20 (Eq. 2) below.
In general, the modeled results of PM2.5 before and after G20 can reproduce the observation results (mean bias (MB) =2.46, root-mean-square error (RMSE) =15.5, R=0.63, p < 0.01), providing the basis of the following comparison.
2.2.2 Backward trajectory analysis
To determine the influence of regional transport on the pollutant concentrations, 24 h air mass back trajectories starting at 300 m from the NRCS site were calculated by using the National Oceanic and Atmospheric Administration (NOAA) HYSPLIT-4 model with a grid and the final meteorological database. The 6-hourly final archive data were obtained from the National Centers for Environmental Prediction's Global Data Assimilation System (GDAS) wind field reanalysis. GDAS uses a spectral medium-range forecast model. More details can be found at https://www.arl.noaa.gov/hysplit/hysplit/ (last access: 2 May 2020). The model was run 24 times per day. The method used in trajectory clustering was based on the GIS-based software TrajStat (Wang et al., 2004).
2.2.3 Observation-based chemical box model (OBM)
Here we used the OBM model to simulate in situ PAN and O3 production and their sensitivity to changes in PAN and O3 precursors, which has been successfully implied in our previous studies (Xue et al., 2014a, c, 2016; Li et al., 2018). In brief, the model was built on the latest version of the Master Chemical Mechanism (MCM v3.3), an explicit mechanism describing the degradation of 143 primarily emitted VOCs, resulting in 17 224 reactions involving 5833 molecular and free-radical species (Saunders et al., 2003). In addition to the existing reactions in MCM v3.3, the heterogeneous reactions of NO2, HO2, NO3, and N2O5 were also incorporated. In addition, we also optimized the model with some physical processes such as the variations in boundary layer height and solar zenith angle, dry deposition, and the dilution of air pollutants within the planetary boundary layer (Xue et al., 2014b). The photolysis frequencies appropriate for Hangzhou are parameterized using a two-stream isotropic-scattering model under clear-sky conditions. In this study, all of these reactions were tracked and grouped into a small number of formation pathways, such as acetaldehyde, acetone, MACR, MVK, MGLY, other OVOCs, reactions of O3 with isoprene and peroxymethacryloyl nitrate (MPAN), and propagation of other radicals to PA. The production rate of PA could be estimated as the sum of these reaction rates. The ozone production rates were calculated through the oxidation of NO by HO2 and RO2, and its destruction rates were mainly facilitated by O3 photolysis and reaction with NO, NO2, OH, HO2, and unsaturated VOCs. Moreover, we investigated the sensitivities of PAN and O3 formation to their respective precursor species by introducing a relative incremental reactivity (RIR) concept which is widely applied in the OBM investigation of PAN and ozone formation (Chameides et al., 1999; Xue et al., 2014c). In this calculation, we performed model calculations during the period of 20 August–10 September 2016, during which VOC measurements were available. The model was run based on the hourly average profiles of PAN, O3, CO, SO2, NO, NO2, C2–C10 non-methane hydrocarbons (NMHCs), air temperature and pressure, and relative humidity (RH) measured at the NRCS site. During the simulation, the model was pre-run for 3 d constrained to the data of 20–22 August so that it reached a steady state for the unmeasured species (e.g., MACR, MVK, HONO, radicals). A more detailed description of this model has been given in previous studies (Jenkin et al., 2003; Xue et al., 2014a, c).
2.2.4 Positive matrix factorization (PMF) model
Positive matrix factorization (PMF) is an effective source apportionment receptor model based on the fingerprints of the sources that does not require the source profiles prior to analysis and has no limitation on source numbers (Hopke, 2003; Pentti and Unto, 1994). The data used in PMF are of the form of an i×j matrix X, in which i is the sampling number and j is the number of species. Based on chemical mass balance of the pollutants, the following equation can be derived with
where p is the number of the sources (i.e., the number of factors), f is the profile of each source, g refers to the contribution of each factor to the total concentration, and e is the residual. Factor contributions and profiles are derived by minimizing the total scaled residual Q:
where u is the uncertainty of the sampling data. More details about principles have been found elsewhere (Cai et al., 2010; Zhang et al., 2013; Li et al., 2015, 2017). In this study, we used the EPA PMF 5.0 model to identify major VOC sources and their temporal variations. We discarded the species that were below the method detection limit (MDL) for more than 50 % of the time or showed a significantly smaller signal-to-noise ratio (S∕N). The uncertainties for each sample and species were calculated based on the following equation if the concentration is greater than the MDL provided:
Values below the detection limit were replaced by one-half of the MDL, and their overall uncertainties were set at five-sixths of the MDL values. In this analysis, different numbers of factors were tested. The robust mode was used to reduce the influence of extreme values on the PMF solution. More than 95 % of the residuals were between −3 and 3 for all compounds. The Q values in the robust mode were approximately equal to the degrees of freedom.
In order to comprehensively evaluate air quality during the G20 period, we compared the concentrations of pollutants during G20 with those in the adjacent time period in 2016. According to the control measure schemes, we classified the whole period into three episodes: 1 week before G20 (BG20) (16–23 August 2016), during G20 (DG20) (24 August–6 September) including phase I (24–27 August) and phase II (28 August–6 September), and 1 week after G20 (AG20) (7–15 September).
3.1 Evolution of meteorological condition
First, we looked into the day-to-day variations in meteorological parameters and atmospheric pollutants from BG20 to AG20 in Fig. S1 in the Supplement. In the period of BG20 and the beginning of DG20 I (16–25 August), a subtropical anticyclone dominated Hangzhou and the surrounding area, leading to a continuous 10 d with a daily mean temperature of 31.5 ∘C ranging from 29.9 to 32.5 ∘C and strong solar irradiation intensity (mean daily maximum value: 369.4 W m−2), favorable for the photochemical production of O3 and PAN. The highest O3 (113.4 ppbv) occurred at 13:00 CST on 25 August under the maximum air temperature of 35.2 ∘C. Meanwhile, the mean daily maximum height of the mixing boundary layer (MBL) during this period was up to ca. 1895 m, beneficial for the diffusion of atmospheric primary pollutants in the vertical direction. In addition, the prevailing wind was from the east (15.1 %) with a mean wind speed of 2.9 m s−1. Results from the backward trajectory simulations demonstrated that the air masses from the east originated from the East China Sea and Yellow Sea, bringing in clean marine air (Fig. S2). Thus, meteorological conditions before G20 were favorable for the dispersal of atmospheric pollutants. On 26 and 27 August, the weather pattern changed to a cold continental high with showery and windy days. The total precipitation and mean wind speed both reached their respective maximums of 14.6 mm and 3.7 m s−1 on 26 August. Accordingly, all species except CO significantly decreased by 12.3 % for SO2, 29.7 % for NOx, 6.7 % for PM2.5, 11.9 % for daily maximum 8 h average (DMA8) O3, and 56.1 % for PAN relative to BG20. With respect to the last half of DG20 I and the beginning of DG20 II (28–31 August), the prevailing wind experienced a shift from northwest to west and to the southwest. On 28 August, the prevailing wind was from the north with a average daily maximum wind speed of 3.9 m s−1 during G20, and the relative humidity rapidly decreased by 26.2 % relative to the previous day. As seen in Fig. S3, air masses arrived at Hangzhou from the north, passing through all of Jiangsu Province and northern parts of Zhejiang Province, two of the most developed provinces in China, with intense human activities. They carried higher PM2.5, SO2, NOx, and CO loadings than the other clusters (see Supplement Table S1). On 1 September, the prevailing wind was from the southwest with high wind speeds (3.3 m s−1). Results from back trajectories indicated that the southwesterly air masses originated from northern Jiangxi Province, were transported over western Zhejiang Province, and arrived at Hangzhou, with high concentration loadings of SO2, particulate matter, O3, and PAN. The increased relative humidity (56.5 %) relative to 49.5 % on 31 August was beneficial for the formation of particulate matter. During 2–4 September, the Hangzhou area witnessed stable meteorological conditions with weak wind (ws < 2.6 m s−1), continuously high air temperature (daily maximum average: 32.2 ∘C), and moderate relative humidity (ca. 60 %). Such conditions were favorable for the accumulation of particulate matter and the photochemical production of O3. It caused significant increases by 25.1 % for PM2.5, 16.7 % for PM10, and 10.7 % for O3 compared with BG20, in contrast to the large decreases by 56.4 % for SO2 and 27.9 % for NOx due to the implementation of emission control measures. Overall, the meteorological conditions during G20 II were not favorable for the dispersal of atmospheric primary pollutants but beneficial for producing O3. However, with the stringent control measures, the most distinct drops in pollutants concentrations were found on 5 September, with large reductions of 50.0 % for PM2.5, 18.3 % for DMA8 O3, 55.7 % for SO2, 41.3 % for NOx, and 65.6 % for PAN relative to BG20. Within AG20, 7 rainy days with mean daily total precipitation of 18.7 mm occurred as well as 6 d with low wind speed (ca. 2.0 m s−1) and 8 d with low MBL (< 1000 m). Such meteorological conditions were beneficial for scavenging the particulate matter and SO2 by wet deposition in addition to the accumulation of NOx. In addition, weak solar irradiation intensity was not favorable for the photochemical formation of O3 and PAN. On 7 September a moderate shower lasted from 2:00 to 11:00 CST with daily total precipitation of 9.5 mm, accompanied by low air temperature (21.5 ∘C) and wind speed (1.8 m s−1). Compared with the previous day, significant decreases in DMA8 O3 (22.6 %) were found as expected, while together with a small reduction in PM2.5 (2.7 %) and unexpected increases in NOx (41.1 %) and SO2 (175.1 %) were also found, indicating that emissions immediately bounced back after lifting the ban on emission controls.
3.2 Evolution of pollutant concentrations
Statistically, observed daytime concentrations of PM2.5, NOx, and SO2 in DG20 II both exhibited significant decreases relative to those in BG20 with the reduction ratios of 11.3 %, 17.0 %, and 18.0 %, respectively (Fig. 2). Furthermore, by using the WRF-Chem model, we quantified the contributions of the emission control measures (ECCs) of 63.5 %, 44.1 %, and 31.2 % to the reductions of PM2.5, SO2, and NO2 in DG20 II relative to BG20, respectively, but for the meteorological conditions it made negative contributions. This evidence indicated that powerful control measures have had an effect on reducing pollutant emissions in Hangzhou under unfavorable meteorological conditions. The large decreases in NOx and SO2 reflected the reduction of vehicle exhaust and coal consumption during G20 in Hangzhou and the surrounding areas. It is worth noting that CO showed gradual increases (ca. 20.7 %) from BG20 to DG20, which was mainly attributed to the weak control on fuel combustion. Specifically, residential usage and liquid natural gas and petroleum gas around the YRD regions during this period might account for such a unique pattern of CO. The other two types of fuel combustion including straw combustion and coal combustion were both excluded as discussed in Sect. 3.4. Under the same stringent control measures, the variation in O3 was not consistent with the primary pollutants. Observed DMA8 O3 increased by 12.4 % in DG20 I relative to BG20, which was attributed to regional transport from the northern provinces and the enhanced solar radiation intensity. Afterwards, DMA8 O3 decreased by 33.4 % from DG20 II to AG20 (Fig. 2), as did the peak values of mean daily O3 in DG20 II compared to BG20 and DG20 I (Fig. S3). This evidence suggests that additional vehicle controls implemented during DG20 II might have played an important role in reducing atmospheric O3 pollution in Hangzhou reflected by shaping such unique diurnal variation, which was also confirmed by the decreased OFP from vehicle exhaust below. Elevated O3 during DG20 rush hours (as shown in Figs. S1 and S2) was attributed to the reduced titration of fresh NO emission under the control measures on vehicle exhaust. Considering such effects, Ox (represented by the sum of O3+NO2) was used to determine the local photochemical formation. The variation in DMA8 Ox was similar to O3, with a distinct decreasing trend from DG20 II to AG20. For PAN, it showed a different pattern with O3. Daytime PAN exhibited a significant decrease (ca. 45.4 %) from BG20 to DG20 II, and then it sharply increased to similar magnitudes in AG20 with BG20. Thereby, it indicates the significant effectiveness of emission control measures in reducing local photochemical formation of O3 and PAN. The underlying formation mechanisms of PAN and O3 including their respective key precursors and chemical process are elucidated in Sect. 3.3.
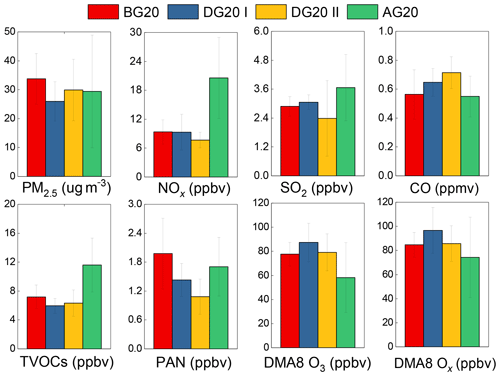
Figure 2The comparisons of daytime PM2.5, NOx, SO2, CO, the total VOCs, PAN, DMA8 O3, and DMA8 Ox. before, during, and after G20, denoted as BG20, DG20, and AG20, respectively. The error bars represent the standard deviations.
With respect to VOCs, the mixing ratios of total VOCs also showed a significant reduction of 20.0 % in DG20 compared with BG20, but they increased by 104.1 % in AG20 after control (Table S2). Alkanes were the most abundant VOC group (55.4 %) in all periods and were reduced by 19.8 % from BG20 to DG20. Conversely, alkenes increased by 20.0 % in DG20 compared to BG20, among which ethylene accounted for 63.9 %–78.0 % during the three periods, although other alkenes decreased to a minor extent. As expected, aromatics were reduced by 49.7 % in DG20 compared with BG20. Ambient mixing ratios of specific VOCs at the NRCS station are summarized in Table S3. Ethane, ethylene, benzene, and toluene are the four most abundant species during all the periods. Compared with BG20, except ethane, isopentane, and ethylene, the mixing ratios of all species decreased in DG20. Ethylene, as a representative tracer of fuel combustion, showed a continuous increase from BG20 to AG20, possibly indicating the ineffectiveness of control measures in this source.
3.3 Identification of the key precursors and chemical processes for PAN and O3
To identify the key precursors and chemical processes for PAN, we employed the observation-based model to investigate the daytime average contributions to PA radical production rates directly from individual pathways for these four episodes (Fig. 3). Acetaldehyde (e.g., oxidation of OH and NO3) and MGLY (e.g., photolysis and oxidation by OH and NO3) were the most important sources of PA in Hangzhou, accounting for 37.3 %–51.6 % and 22.8 %–29.5 % of the total production rates. This was in agreement with the findings obtained from the other typical urban areas such as Beijing (Xue et al., 2014c; Liu et al., 2010; Zhang et al., 2015), Tokyo (Kondo et al., 2008), Houston, Nashville (Roberts et al., 2001), and Sacramento (LaFranchi et al., 2009). Reactions of OVOCs and propagation of other radicals to PA (mainly including decomposition of some RO radicals and reactions of some higher acyl peroxy radicals with NO) were also significant sources, with average contributions of 7.1 %–9.1 % and 18.1 %–27.0 %, respectively. A minor contribution (∼1 % in total) originated from the other pathways of O3 + isoprene, O3 + MPAN, acetone, and MVK. Acetaldehyde and other OVOCs are mainly photooxidation products of hydrocarbons; thus it is necessary to further identify the first-generation precursors of PAN here. We tested the model sensitivity by introducing the concept of relative incremental reactivity (RIR), which is widely used in the OBM study of ozone formation (Chameides et al., 1999). Here RIR is defined as the ratio of decrease in PAN production rates to decrease in precursor concentrations (e.g., 20 % reduction is used in this study). A number of sensitivity model runs were performed to calculate the RIRs for NOx, alkane, alkene, and aromatic classes as well as the individual C2–C10 hydrocarbon species. As shown in Fig. 4a, production of PAN was sensitive to VOCs from BG20 to AG20. Meanwhile, the negative RIR values for NOx also indicated a VOC regime of PAN production around the G20 period in urban Hangzhou. In terms of biogenic VOCs (BVOCs), the positive RIR values for isoprene (0.18–0.38) from BG20 to AG20 implied that in situ formation of PAN at the NRCS was highly sensitive to isoprene. As for anthropogenic VOCs (AVOCs), alkenes and aromatics were the most important first-generation PAN precursors, with an RIR range of 0.24–0.37 and 0.26–0.52, respectively. Furthermore, we identified the other specific VOCs controlling PAN production, which were xylenes, trans/cis-2-butenes, trimethylbenzenes, toluene, and propene evidenced by their positive RIRs. Compared with their individual RIRs between the control and non-control periods, the in situ production of PAN was dominated by aromatics in BG20 and DG20 I but controlled by alkenes in AG20. In addition to secondary acetaldehyde formed by the oxidation of ethanol, most aromatics were mainly emitted by vehicle exhaust. The decreased RIRs of aromatics together with the decreased contribution ratios of acetaldehyde to the PA radical formation during G20 both indicated the effectiveness of control measures on vehicle exhaust in reducing atmospheric PAN concentration. Similar to PAN, the daytime average RIRs for major groups of O3 precursors during the episodes are shown in Fig. 4b. Overall, the in situ ozone formation was also controlled by VOCs from BG20 to AG20. AVOCs were dominated by alkenes and aromatics, along with their increasing and decreasing RIRs. With continued emission control, the RIR for AVOCs showed a gradual decrease from BG20 to DG20 but increased after G20. In contrast, BVOCs (mainly isoprene) exhibited gradual increases for all periods, especially during phase II in DG20 and AG20 when their RIRs were both higher than those for AVOCs. Thereby, the contribution of BVOCs to the photochemical production of O3 weakened the effect of stringent control measures on reducing surface O3. The RIRs for NOx were negative throughout the period of G20, also indicating a VOC-limited regime for the sensitivity of ozone formation. This suggests that reducing emissions of aromatics, alkenes, and alkanes would alleviate O3 formation, yet cutting NOx emissions may aggravate the local O3 problems.
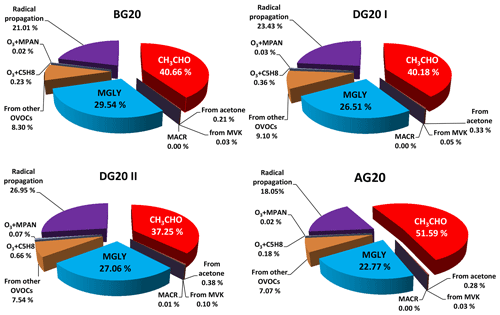
Figure 3Contributions of individual pathways to PA radical formation during the episodes of BG20, DG20 I, DG20 II, and AG20.
3.4 Identification of VOC sources and quantification of their respective ozone formation potential
To distinguish the various sources of VOCs, we compared the PMF profiles with the reference profiles from the literature as listed below. Seven sources were identified as follows: (1) gasoline evaporation, (2) solvent utilization, (3) industrial manufacturing, (4) industrial chemical feedstock, (5) vehicle exhaust, (6) fuel combustion, and (7) biogenic emission. Figure 5 exhibited the modeled source profiles together with the relative contributions of each source to individual species. The first source is characterized by a significant amount (78.5 %) of isopentane, which is a typical tracer for gasoline evaporation (Liu et al., 2008). Therefore, this source was identified as gasoline evaporation. The second source was rich in n-pentane and aromatics. Many aromatics such as benzene, toluene, ethylbenzene, and xylene (BTEX) are the dominant components of organic paints and were regarded as chemical tracers of solvent utilization (Watson et al., 2001). Significant amounts of ethylbenzene, xylenes, and n-pentane are present in the second source, accounting for 19.2 %, 58.8 %, and 98.8 %, respectively. Thus, the second source was identified as solvent utilization. The third source was characterized by high loading of cyclohexane (54.7 %) and BTEX (15.1 %–46.2 %). These compounds are confirmed to be typical species in industrial manufacturing in China (Liu et al., 2008). Thus, this source was representative of industrial manufacturing. The fourth source identified as industrial chemical feedstock (shown in Fig. 5) was characterized by very little contribution of alkanes and aromatics and large amounts of 3-ethyltoluene (29.4 %), 3-methylheptane (51.0 %), and n-hexane (47.1 %), which are typical proxies for industrial chemical feedstock (Liu et al., 2008; Mo et al., 2015). The fifth source was characterized by abundant 2-methylpentane (61.7 %) and BTEX, which is a typical tracer for vehicle exhaust (Liu et al., 2008; Li et al., 2015). In addition, 2,2,4-trimethylpentane is a fuel additive used to gain higher octane ratings (McCarthy et al., 2013) with high abundance of 21.4 % in this source, and thus it is identified as vehicle exhaust. The sixth source profile shown in Fig. 5 was in relation to 48.9 % of the total measured ethylene mixing ratios, major species of which were emitted from the fuel combustion process (Li et al., 2015). It was also characterized by significant amounts of ethane, propane, n-butane, propene, and benzene. Ethane and propane are the tracers of natural gas and liquid petroleum gas (LPG) usage, respectively, and the source profiles of resident fuel combustion in China contained alkenes (Wang et al., 2013). Coal combustion can release a large amount of BTEX into the atmosphere and styrene is a typical indictor of industrial manufacturing in China (Liu et al., 2008; Li et al., 2015). Thus, this source was believed to be fuel combustion related to industrial processes and residual usage. The seventh source was distinguished by a significant amount of isoprene, a representative indicator of biogenic emissions. About 93.1 % of the total isoprene mixing ratios are apportioned to this factor (Guenther et al., 1995). There were very small quantities of the other species such as aromatics (0 %–1.8 %) in this factor. Therefore, it was excluded from biomass burning but mainly identified as biogenic emissions. Figure 6 shows the variation in the seven sources during the four periods. Clearly, anthropogenic sources such as solvent utilization, industrial manufacturing, vehicle exhaust, fuel combustion, and industrial chemical feedstock were the predominant sources of the total VOCs before and after G20, as high as 52.4 %–81.7 %. Furthermore, anthropogenic emission showed significant reductions during the G20 response to the stringent emission control. In BG20, solvent utilization was the predominant contributor to VOC mixing ratios, contributing 1.88 ppbv, followed by vehicle exhaust (1.77 ppbv, 21.6 %), industrial manufacturing (1.55 ppbv, 19.0 %), biogenic emission (1.16 ppbv, 14.1 %), gasoline evaporation (0.83 ppbv, 10.1 %), and fuel combustion (0.35 ppbv, 4.3 %). The industry-related emissions (industrial manufacturing, chemical feedstock, and solvent utilization) together accounted for 50.0 % of the total VOC mixing ratios. The vehicle-related emission sources (vehicle exhaust and gasoline evaporation) accounted for 31.7 % of the total VOC mixing ratios. This indicated that traffic and industry sources were the major VOC sources before the control period. Compared with BG20, the contribution of solvent utilization was reduced to the largest extent, with a magnitude of 1.43 ppbv, followed by industrial manufacturing (0.69 ppbv) and vehicle exhaust (0.38 ppbv), during the first emission control period (DG20 I). According to the control strategy during G20, the control measures of source emission were mainly on the industry and power plants in DG20 I, and thus they were responsible for the large reduction of industry-related emissions including solvent utilization (76.0 %), industrial manufacturing (44.0 %), and vehicle exhaust (21.0 %). With the acceleration of emission control (DG20 II), the contribution of vehicle-related emissions was reduced as expected in vehicle exhaust (66.1 %) and gasoline evaporation (61.8 %) relative to DG20 I, while a significant increase was also found in fuel combustion with the increment of 0.7 ppbv (152.6 %). After G20, the contributions of vehicle-related emissions and industry-related emissions both showed rebounds due to lifting a ban on industry, power plant, and transport in and around Zhejiang Province. It should be mentioned that biogenic emissions also played a role in the VOC mixing ratios, contributing from 0.81 to 1.29 ppbv. About 20.9 % of the total VOC mixing ratios could be ascribed to biogenic emissions, acting as the second major source, during the G20 II period. This indicated that biogenic VOCs might make more of a contribution to the VOC mixing ratios, especially when anthropogenic VOCs were substantially reduced following the process of control measures.
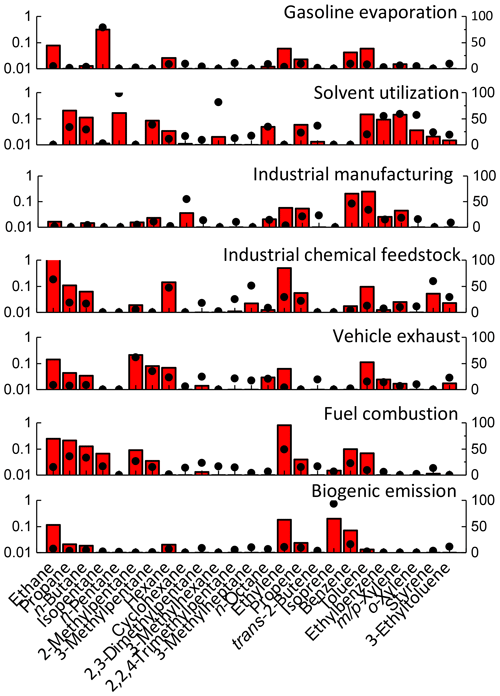
Figure 5Seven source profiles and their respective contribution resolved from PMF model. The bars are the profiles (ppbv, left axis), and the dots are the percentage contribution (%, right axis) from individual factors.
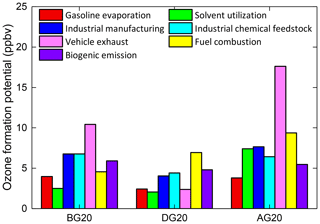
Figure 7Ozone formation potential (ppbv) of each source before, during, and after the control period during 2016 G20 in China.
Moreover, we quantified their respective ozone formation potential (OFP) before, during, and after G20 by using the latest maximum incremental reactivity (MIR) and the appointed concentration profiles above (see Fig. 7). Overall, the total OFP in DG20 was significantly reduced by the implement of stringent control measures compared with BG20 and AG20. Specifically, the OFPs of solvent utilization, industrial manufacturing, and vehicle exhaust both showed significant decreases (17.3 %–77.2 %) compared with BG20, while fuel combustion significantly increased by 52.2 % with the OFP of 6.9 ppbv, accounting for 25.6 % of the total during G20. Thus, it is clear that the high OFP of fuel combustion contributed by ethylene was also responsible for the enhanced concentration of O3 during G20. Such a high OFP from fuel combustion was also elucidated in APEC in Beijing (Li et al., 2015). To classify the specific fuel type, we first examined the fire spots derived from the NCAR Fire Inventory version 1.5 (FINNV1.5) in eastern China before, during, and after the period of 2016 G20 (see Fig. S4). Straw combustion was excluded according to the decrease in the number of fire spots in the same time period from BG20 to AG20. As mentioned above, industrial processes with coal combustion were strictly limited throughout the whole G20 period. To ensure clean energy was used in 2016 G20, local governments accelerated a supply of liquid natural gas during the 13th Five-Year Plan period in Hangzhou. In 2016, the consumption amounts of natural gas and liquid petroleum gas both increased up to 4.55×109 kg (54.4 %) and 5.09×108 kg (13.4 %) compared with those in 2015, respectively (Statistics Bureau of Zhejiang Province, 2016, 2017). Thus, liquid natural gas and petroleum gas were identified as the major fuel used residentially during G20. After G20, all anthropogenic sources showed significant increments of OFP, among which the fastest growth of a source was vehicle exhaust (17.6 ppbv, 638.4 %), followed by fuel combustion (9.4 ppbv, 35.1 %), industrial manufacturing (7.7 ppbv, 89.2 %), and solvent utilization (7.4 ppbv, 258.1 %).
In this study, ground-based concentrations of atmospheric trace gases and particulate matter, together with meteorological parameters, were measured at a NRCS site in urban Hangzhou before, during, and after G20. We found significant decreases in atmospheric VOCs, PM2.5, NOx, and SO2 in DG20 relative to BG20 and AG20, under unfavorable meteorological conditions (e.g., stable weather pattern and regional transport). This evidence indicated that the powerful control measures had an effect on their emissions in Hangzhou. Conversely, observed DMA8 O3 increased from BG20 to DG20 I, which was attributed to regional transport from the northern provinces and enhanced solar radiation intensity. Then it decreased from DG20 II to AG20. The decreases in the peak concentration of daily O3 and the OFP estimated from various VOC sources both suggested the effectiveness of stringent control measures in reducing atmospheric O3 concentrations. Unlike O3, PAN exhibited a gradual decrease from BG20 to DG20. With the OBM model, we found acetaldehyde and methylglyoxal (MGLY) to be the most important second-generation precursors of PAN, accounting for 37.3 %–51.6 % and 22.8 %–29.5 % of the total production rates. Furthermore, we confirmed that the production of PAN was sensitive to anthropogenic and biogenic VOCs (isoprene) throughout the whole period, specifically aromatics in BG20 and DG20 I but also alkenes in AG20. Similarly, the sensitivity of ozone formation was also under a VOC-limited regime throughout the G20 period. These findings suggest that reducing emissions of alkanes, alkenes, and aromatics would mitigate photochemical smog, including PAN and O3 formation. Furthermore, traffic (vehicle exhaust and gasoline evaporation) and industrial sources (solvent utilization, industrial manufacturing, and chemical feedstock) were found to be the major VOC sources before G20, accounting for ca. 50.0 % and 31.7 % of the total, respectively, with ozone formation potential (OFP) of 14.4 and 16.1 ppbv. Large decreases were found in the sources and OFPs of solvent utilization (74.1 % and 17.3 %), followed by vehicle exhaust (57.4 % and 77.2 %) and industrial manufacturing (56.0 % and 40.3 %) response to the stringent control measures during G20. We recommend paying attention to controlling fuel combustion and biogenic emissions, especially when anthropogenic VOCs have been substantially reduced following control measures.
The data in the figures in both the main text and the Supplement are available upon request to the corresponding author (Gen Zhang, zhanggen@cma.gov.cn).
The supplement related to this article is available online at: https://doi.org/10.5194/acp-20-5391-2020-supplement.
GZ and HX designed the research. HW, BQ, RD, and XM performed research. GZ, LX, JH, WX, CL, LL, ZL, KG, YY, and WJ analyzed data. GZ, HX, and LX wrote the paper.
The authors declare that they have no conflict of interest.
The authors appreciate the editor and four anonymous reviewers for their valuable comments. The authors are grateful to Xiaobin Xu for the help in discussions.
This research has been supported by the National Key Research and Development Program of China (grant no. 2016YFC0202300), the National Natural Science Foundation of China (grant nos. 41775127 and 41505108), Basic Research Fund of CAMS (2020Z002), the State Environmental Protection Key Laboratory of the Cause and Prevention of Urban Air Pollution Complex (grant no. Y201701), and the Zhejiang Provincial National Science Foundation (grant no. LY19D050002).
This paper was edited by Rolf Müller and reviewed by four anonymous referees.
Cai, C., Geng, F., Tie, X., Yu, Q., and An, J.: Characteristics and source apportionment of VOCs measured in Shanghai, China, Atmos. Environ., 44, 5005–5014, 2010.
Chameides, W. L., Xingsheng, L., Xiaoyan, T., Xiuji, Z., Luo, C., Kiang, C. S., St. John, J., Saylor, R. D., Liu, S. C., Lam, K. S., Wang, T., and Giorgi, F.: Is ozone pollution affecting crop yields in China?, Geophys. Res. Lett., 26, 867–870, 1999.
Cheng, Y. F., Zheng, G. J., Wei, C., Mu, Q., Zheng, B., Wang, Z. B., Gao, M., Zhang, Q., He, K. B., Carmichael, G., Pöschl, U., and Su, H.: Reactive nitrogen chemistry in aerosol water as a source of sulfate during haze events in China, Sci. Adv., 2, e1601530, https://doi.org/10.1126/sciadv.1601530, 2016.
de Foy, B., Lu, Z. F., and Streets, D. G.: Satellite NO2 retrievals suggest China has exceeded its NOx reduction goals from the twelfth Five-Year Plan, Sci. Rep.-UK, 6, 35912, https://doi.org/10.1038/srep35912, 2016.
Ding, A. J., Fu, C. B., Yang, X. Q., Sun, J. N., Zheng, L. F., Xie, Y. N., Herrmann, E., Nie, W., Petäjä, T., Kerminen, V.-M., and Kulmala, M.: Ozone and fine particle in the western Yangtze River Delta: an overview of 1 yr data at the SORPES station, Atmos. Chem. Phys., 13, 5813–5830, https://doi.org/10.5194/acp-13-5813-2013, 2013.
Feng, C., Gao, X., Tang, Y., and Zhang, Y.: Comparative life cycle environmental assessment of flue gas desulphurization technologies in China, J. Clean. Prod., 68, 81–92, 2014.
Gao, Y., Liu, X., Zhao, C., and Zhang, M.: Emission controls versus meteorological conditions in determining aerosol concentrations in Beijing during the 2008 Olympic Games, Atmos. Chem. Phys., 11, 12437–12451, https://doi.org/10.5194/acp-11-12437-2011, 2011.
Geng, F. H, Zhao, C. S, Tang, X., Lu, G. L, and Tie, X. X: Analysis of ozone and VOCs measured in Shanghai: A case study, Atmos. Environ., 41, 989–1001, 2007.
Guenther, A., Hewitt, C. N., Erickson, D., Fall, R., Geron, C., Graedel, T., Harley, P., Klinger, L., Manuel, L., Mckay, W. A., Pierce, T., Scholes, B., Steinbrecher, R., Tallamraju, R., Taylor, J., and Zimmerman, P.: A global model of natural volatile organic compound emissions, J. Geophys. Res.-Atmos., 100, 8873–8892, 1995.
Hopke, P. K.: Recent developments in receptor modeling, J. Chemometr., 17, 255–265, 2003.
Huang, R. J., Zhang, Y. L., Bozzetti, C., Ho, K. F., Cao, J. J., Han, Y. M., Daellenbach, K. R., Slowik, J. G., Platt, S. M., Canonaco, F., Zotter, P., Wolf, R., Pieber, S. M., Bruns, E. A., Crippa, M., Ciarelli, G., Piazzalunga, A., Schwikowski, M., Abbaszade, G., Schnelle-Kreis, J., Zimmermann, R., An, Z. S., Szidat, S., Baltensperger, U., El Haddad, I., and Prevot, A. S. H.: High secondary aerosol contribution to particulate pollution during haze events in China, Nature, 514, 218–222, 2014.
Jenkin, M. E., Saunders, S. M., Wagner, V., and Pilling, M. J.: Protocol for the development of the Master Chemical Mechanism, MCM v3 (Part B): tropospheric degradation of aromatic volatile organic compounds, Atmos. Chem. Phys., 3, 181–193, https://doi.org/10.5194/acp-3-181-2003, 2003.
Kondo, Y., Morino, Y., Fukuda, M., Kanaya, Y., Miyazaki, Y., Takegawa, N., Tanimoto, H., McKenzie, R., Johnston, P., Blake, D. R., Murayama, T., and Koike, M.: Formation and transport of oxidized reactive nitrogen, ozone, and secondary organic aerosol in Tokyo, J. Geophys. Res.-Atmos., 113, D21310, https://doi.org/10.1029/2008JD010134, 2008.
Koukouli, M. E., Balis, D. S., van der A, R. J., Theys, N., Hedelt, P., Richter, A., Krotkov, N., Li, C., and Taylor, M.: Anthropogenic sulphur dioxide load over China as observed from different satellite sensors, Atmos. Environ., 145, 45–59, 2016.
LaFranchi, B. W., Wolfe, G. M., Thornton, J. A., Harrold, S. A., Browne, E. C., Min, K. E., Wooldridge, P. J., Gilman, J. B., Kuster, W. C., Goldan, P. D., de Gouw, J. A., McKay, M., Goldstein, A. H., Ren, X., Mao, J., and Cohen, R. C.: Closing the peroxy acetyl nitrate budget: observations of acyl peroxy nitrates (PAN, PPN, and MPAN) during BEARPEX 2007, Atmos. Chem. Phys., 9, 7623–7641, https://doi.org/10.5194/acp-9-7623-2009, 2009.
Li, J., Xie, S. D., Zeng, L. M., Li, L. Y., Li, Y. Q., and Wu, R. R.: Characterization of ambient volatile organic compounds and their sources in Beijing, before, during, and after Asia-Pacific Economic Cooperation China 2014, Atmos. Chem. Phys., 15, 7945–7959, https://doi.org/10.5194/acp-15-7945-2015, 2015.
Li, K., Li, J., Wang, W., Tong, S., Liggio, J., and Ge, M.: Evaluating the effectiveness of joint emission control policies on the reduction of ambient VOCs: Implications from observation during the 2014 APEC summit in suburban Beijing, Atmos. Environ., 164, 117–127, 2017.
Li, L., An, J. Y., Shi, Y. Y., Zhou, M., Yan, R. S., Huang, C., Wang, H. L., Lou, S. R., Wang, Q., Lu, Q., and Wu, J.: Source apportionment of surface ozone in the Yangtze River Delta, China in the summer of 2013, Atmos. Environ., 144, 194–207, 2016.
Li, Z., Xue, L., Yang, X., Zha, Q., Tham, Y. J., Yan, C., Louie, P. K. K., Luk, C. W. Y., Wang, T., and Wang, W.: Oxidizing capacity of the rural atmosphere in Hong Kong, Southern China, Sci. Total Environ., 612, 1114–1122, 2018.
Liu, Y., Shao, M., Fu, L., Lu, S., Zeng, L., and Tang, D.: Source profiles of volatile organic compounds (VOCs) measured in China: Part I, Atmos. Environ., 42, 6247–6260, 2008.
Liu, Z., Wang, Y. H., Gu, D. S., Zhao, C., Huey, L. G., Stickel, R., Liao, J., Shao, M., Zhu, T., Zeng, L. M., Liu, S. C., Chang, C. C., Amoroso, A., and Costabile, F.: Evidence of reactive aromatics as a major source of peroxy acetyl nitrate over China, Environ. Sci. Technol., 44, 7017–7022, 2010.
McCarthy, M. C., Aklilu, Y.-A., Brown, S. G., and Lyder, D. A.: Source apportionment of volatile organic compounds measured in Edmonton, Alberta, Atmos. Environ., 81, 504–516, 2013.
McFadyen, G. G. and Cape, J. N.: Peroxyacetyl nitrate in eastern Scotland, Sci. Total Environ., 337, 213–222, 2005.
Miao, Y. C., and Liu, S. H.: Linkages between aerosol pollution and planetary boundary layer structure in China, Sci. Total Environ., 650, 288–296, 2019.
Miao, Y. C., Liu, S. H., Guo, J. P., Huang, S. X., Yan, Y., and Lou, M. Y.: Unraveling the relationships between boundary layer height and PM2.5 pollution in China based on four-year radiosonde measurements, Environ. Pollut., 243, 1186–1195, 2018.
Mo, Z., Shao, M., Lu, S., Qu, H., Zhou, M., Sun, J., and Gou, B.: Process-specific emission characteristics of volatile organic compounds (VOCs) from petrochemical facilities in the Yangtze River Delta, China, Sci. Total Environ., 533, 422–431, 2015.
Pentti, P. and Unto, T.: Positive matrix factorization: a non-negative factor model with optimal utilization of error estimates of data values, Environmetrics, 5, 111–126, 1994.
Roberts, J. M., Stroud, C. A., Jobson, B. T., Trainer, M., Hereid, D., Williams, E., Fehsenfeld, F., Brune, W., Martinez, M., and Harder, H.: Application of a sequential reaction model to PANs and aldehyde measurements in two urban areas, Geophys. Res. Lett., 28, 4583–4586, 2001.
Saunders, S. M., Jenkin, M. E., Derwent, R. G., and Pilling, M. J.: Protocol for the development of the Master Chemical Mechanism, MCM v3 (Part A): tropospheric degradation of non-aromatic volatile organic compounds, Atmos. Chem. Phys., 3, 161–180, https://doi.org/10.5194/acp-3-161-2003, 2003.
Shao, M., Lu, S. H., Liu, Y., Xie, X., Chang, C. C., Huang, S., and Chen, Z. M.: Volatile organic compounds measured in summer in Beijing and their role in ground-level ozone formation, J. Geophys. Res.-Atmos., 114, D00G06, https://doi.org/10.1029/2008JD010863, 2009.
Statistics Bureau of Zhejiang Province: Zhejiang Statistical Yearbook, China Statistical Publishing House, Beijing, 646 pp., 2016.
Statistics Bureau of Zhejiang Province: Zhejiang Statistical Yearbook, China Statistical Publishing House, Beijing, 663 pp., 2017.
Verstraeten, W. W., Neu, J. L., Williams, J. E., Bowman, K. W., Worden, J. R., and Boersma, K. F.: Rapid increases in tropospheric ozone production and export from China, Nat. Geosci, 8, 690–695, 2015.
Wang, Q., Geng, C., Lu, S., Chen, W., and Shao, M.: Emission factors of gaseous carbonaceous species from residential combustion of coal and crop residue briquettes, Front. Environ. Sci. Eng., 7, 66–76, 2013.
Wang, T., Xue, L. K., Brimblecombe, P., Lam, Y. F., Li, L., and Zhang, L.: Ozone pollution in China: A review of concentrations, meteorological influences, chemical precursors, and effects, Sci. Total. Environ., 575, 1582–1596, 2017.
Wang, Y. Q., Zhang, X. Y., Arimoto, R., Cao, J. J., and Shen, Z. X.: The transport pathways and sources of PM10 pollution in Beijing during spring 2001, 2002 and 2003, Geophys. Res. Lett., 31, L14110, https://doi.org/10.1029/2004GL019732, 2004.
Wang, Y. G., Ying, Q., Hu, J. L., and Zhang, H. L.: Spatial and temporal variations of six criteria air pollutants in 31 provincial capital cities in China during 2013–2014, Environ. Int., 73, 413–422, 2014.
Watson, J. G., Chow, J. C., and Fujita, E. M.: Review of volatile organic compound source apportionment by chemical mass balance, Atmos. Environ., 35, 1567–1584, 2001.
Williams, J., Roberts, J. M., Bertman, S. B., Stroud, C. A., Fehsenfeld, F. C., Baumann, K., Buhr, M. P., Knapp, K., Murphy, P. C., Nowick, M., and Williams, E. J.: A method for the airborne measurement of PAN, PPN, and MPAN, J. Geophys. Res.-Atmos., 105, 28943–28960, 2000.
Xu, Z., Liu, J. F., Zhang, Y. J., Liang, P., and Mu, Y. J.: Ambient levels of atmospheric carbonyls in Beijing during the 2008 Olympic Games, J. Environ. Sci., 22, 1348–1356, 2010.
Xue, L., Gu, R., Wang, T., Wang, X., Saunders, S., Blake, D., Louie, P. K. K., Luk, C. W. Y., Simpson, I., Xu, Z., Wang, Z., Gao, Y., Lee, S., Mellouki, A., and Wang, W.: Oxidative capacity and radical chemistry in the polluted atmosphere of Hong Kong and Pearl River Delta region: analysis of a severe photochemical smog episode, Atmos. Chem. Phys., 16, 9891–9903, https://doi.org/10.5194/acp-16-9891-2016, 2016.
Xue, L. K., Wang, T., Louie, P. K. K., Luk, C. W. Y., Blake, D. R., and Xu, Z.: Increasing external effects negate local efforts to control ozone air pollution: a case study of Hong Kong and implications for other Chinese cities, Environ. Sci. Techol., 48, 10769–10775, 2014a.
Xue, L. K., Wang, T., Gao, J., Ding, A. J., Zhou, X. H., Blake, D. R., Wang, X. F., Saunders, S. M., Fan, S. J., Zuo, H. C., Zhang, Q. Z., and Wang, W. X.: Ground-level ozone in four Chinese cities: precursors, regional transport and heterogeneous processes, Atmos. Chem. Phys., 14, 13175–13188, https://doi.org/10.5194/acp-14-13175-2014, 2014b.
Xue, L. K., Wang, T., Wang, X. F., Blake, D. R., Gao, J., Nie, W., Gao, R., Gao, X. M., Xu, Z., Ding, A. J., Huang, Y., Lee, S. C., Chen, Y. Z., Wang, S. L., Chai, F. H., Zhang, Q. Z., and Wang, W. X.: On the use of an explicit chemical mechanism to dissect peroxy acetyl nitrate formation, Environ. Pollut., 195, 39–47, 2014c.
Zhang, G., Mu, Y. J., Liu, J. F., and Mellouki, A.: Direct and simultaneous determination of trace-level carbon tetrachloride, peroxyacetyl nitrate, and peroxypropionyl nitrate using gas chromatography-electron capture detection, J. Chromatogr. A, 1266, 110–115, 2012.
Zhang, G., Mu, Y. J., Liu, J. F., Zhang, C. L., Zhang, Y. Y., Zhang, Y. J., and Zhang, H. X.: Seasonal and diurnal variations of atmospheric peroxyacetyl nitrate, peroxypropionyl nitrate, and carbon tetrachloride in Beijing, J. Environ. Sci., 26, 65–74, 2014.
Zhang, G., Mu, Y. J., Zhou, L. X., Zhang, C. L., Zhang, Y. Y., Liu, J. F., Fang, S. X., and Yao, B.: Summertime distributions of peroxyacetyl nitrate (PAN) and peroxypropionyl nitrate (PPN) in Beijing: Understanding the sources and major sink of PAN, Atmos. Environ., 103, 289–296, 2015.
Zhang, G., Xu, H., Qi, B., Du, R., Gui, K., Wang, H., Jiang, W., Liang, L., and Xu, W.: Characterization of atmospheric trace gases and particulate matter in Hangzhou, China, Atmos. Chem. Phys., 18, 1705–1728, https://doi.org/10.5194/acp-18-1705-2018, 2018.
Zhang, H., Wang, S., Hao, J., Wang, X., Wang, S., Chai, F., and Li, M.: Air pollution and control action in Beijing, J. Clean. Prod., 112, 1519–1527, 2016.
Zhang, R., Jing, J., Tao, J., Hsu, S.-C., Wang, G., Cao, J., Lee, C. S. L., Zhu, L., Chen, Z., Zhao, Y., and Shen, Z.: Chemical characterization and source apportionment of PM2.5 in Beijing: seasonal perspective, Atmos. Chem. Phys., 13, 7053–7074, https://doi.org/10.5194/acp-13-7053-2013, 2013.
Zhang, Y. J., Mu, Y. J., Liang, P., Xu, Z., Liu, J. F., Zhang, H. X., Wang, X. K., Gao, J., Wang, S. L., Chai, F. H., and Mellouki, A.: Atmospheric BTEX and carbonyls during summer seasons of 2008-2010 in Beijing, Atmos. Environ., 59, 186–191, 2012.