the Creative Commons Attribution 4.0 License.
the Creative Commons Attribution 4.0 License.
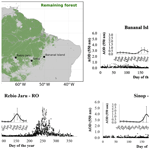
Characterization of the radiative impact of aerosols on CO2 and energy fluxes in the Amazon deforestation arch using artificial neural networks
Renato Kerches Braghiere
Marcia Akemi Yamasoe
Nilton Manuel Évora do Rosário
Humberto Ribeiro da Rocha
José de Souza Nogueira
Alessandro Carioca de Araújo
In vegetation canopies with complex architectures, diffuse solar radiation can enhance carbon assimilation through photosynthesis because isotropic light is able to reach deeper layers of the canopy. Although this effect has been studied in the past decade, the mechanisms and impacts of this enhancement over South America remain poorly understood. Over the Amazon deforestation arch large amounts of aerosols are released into the atmosphere due to biomass burning, which provides an ideal scenario for further investigation of this phenomenon in the presence of canopies with complex architecture. In this paper, the relation of aerosol optical depth and surface fluxes of mass and energy are evaluated over three study sites with artificial neural networks and radiative transfer modeling. Results indicate a significant effect of the aerosol on the flux of carbon dioxide between the vegetation and the atmosphere, as well as on energy exchange, including that surface fluxes are sensitive to second-order radiative impacts of aerosols on temperature, humidity, and friction velocity. CO2 exchanges increased in the presence of aerosol in up to 55 % in sites with complex canopy architecture. A decrease of approximately 12 % was observed for a site with shorter vegetation. Energy fluxes were negatively impacted by aerosols over all study sites.
- Article
(3512 KB) - Full-text XML
-
Supplement
(1023 KB) - BibTeX
- EndNote
This research was carried out in part at the Jet Propulsion Laboratory, California Institute of Technology, under a contract with the National Aeronautics and Space Administration. California Institute of Technology. Government sponsorship acknowledged. © 2020.
The composition of the atmosphere is determined by the emission and transport of gases and aerosols at different scales, chemical and microphysical processes, wet and dry deposition, or by the distribution of the land surface and oceanic ecosystems around the globe. These processes are represented by the biogeochemical cycle, and they involve interactions between different components of the Earth system. These interactions are generally nonlinear and might produce negative or positive radiative forcing signals in the climate system (Forster et al., 2007).
The state of the climate system is governed by the energy balance, which is defined as the amount of energy going into the climate system minus the amount of energy dissipated by it mostly through the emission of longwave radiation. In the long term, the amount of solar radiation absorbed by the Earth's atmosphere and surface is balanced out by the same amount of longwave radiation emitted by them. About half of the solar radiation is absorbed by the Earth's surface. This energy is transferred to the atmosphere by heating the air in contact with the surface, through evapotranspiration and longwave radiation emission, which can be absorbed by clouds and greenhouse gases. The atmosphere in turn emits longwave energy back to the surface as well as to space (Kiehl and Trenberth, 1997; Trenberth, 2009).
The Earth's climate system has undergone a number of changing processes considered “natural” throughout the history of the planet. The average temperature of the planet has always been directly linked to the amount of CO2 in the atmosphere. The uncertainties on the energy balance associated with greenhouse gases have been reduced over the past years; however, uncertainties associated with atmospheric aerosols remain substantial, despite the various advances achieved since the first studies relating aerosols and the climate system (Boucher et al., 2013). Aerosols are technically defined as solid or liquid particles suspended in the air (Seinfeld and Pandis, 2006), which can be emitted from natural or anthropogenic sources. They can be found in different shapes and chemical composition, which may vary according to their source of emission and/or processes they are subjected to once in the atmosphere. They can also be classified into primary, i.e., when they are directly emitted, or secondary, i.e., when they are formed in the atmosphere through a physicochemical process known as gas–particle conversion.
The typical diameter of aerosols ranges from a few nanometers to tens of micrometers (Seinfeld and Pandis, 2006), and it can impact their time spent in the atmosphere and their ability to interact with radiation.
Aerosols can travel long distances which increase the time they are exposed to radiation and the probability of interaction with clouds. In addition, the accumulation mode (0.1 µm < particle size < 2.5 µm) interacts most effectively with solar radiation, although the nuclei-mode aerosols (0.1 µm > particle size) are mostly found over the continents and coastal areas, directly related to human activities. Nuclei-mode aerosols account for about 10 % of the aerosols of the globe (Textor et al., 2006). Aerosols emitted by natural sources are the dominant type in the climate system. In climate models, aerosols are generally divided into five general categories: dust, marine spray, sulfur aerosols or sulfate, and carbon compounds, which are divided into two subcategories: particulate organic material and black carbon (Chin et al., 2002).
The direct radiative effect of aerosols can absorb and scatter radiation in the shortwave band and longwave band, which directly impacts the radiative balance of the climate system (Forster et al., 2007). The net impact of the direct radiative effect of aerosols decreases the available solar energy at the surface. In the last decades, there has been a 2.7 % reduction observed in the incident direct solar irradiance at the surface, a phenomenon known as “global dimming” (Stanhill and Cohen, 2001). Although the mechanisms associated with the direct radiative effect of aerosols are well described in the literature, uncertainties are still significant, especially with respect to the spatial and temporal distribution of the aerosols in the atmosphere (Forster et al., 2007; Fletcher et al., 2018). In addition, the radiative effects of aerosols can impact different compartments of the climate system, such as the biosphere, for example.
The biosphere interacts with the climate system through biogeophysical and biogeochemical processes, which can cause both positive and negative responses on the radiative balance, and some of these responses, especially at a local scale, can be quite significant (Wang et al., 2014; He et al., 2017; Persad and Caldeira, 2018).
The overall reduction in incident radiation at the surface has negatively impacted gross primary productivity (GPP) in light-limited ecosystems (Black et al., 2006; Unger et al., 2017; Ezhova et al., 2018). In addition, a decrease in total solar radiation is followed by an increase in its diffuse component, which in turn can increase plant productivity due to a higher production efficiency per unit of incident radiation in the canopy, an effect known as “the diffuse fertilization effect” (Kanniah et al., 2012). In ecosystems with densely vegetated canopies, the direct shortwave radiation reaches the upper part of the vegetation canopy, while most leaves at medium canopy height or at bottom canopy layers remain shaded. Carbon assimilation increases under atmospheric conditions with a moderate aerosol load and high fraction of diffuse radiation (Niyogi et al., 2004) and decreases as the concentration of aerosols increases to a level where the overall radiation is substantially reduced (Yamasoe et al., 2006).
The spatial distribution and nature of the shortwave radiation is critical for the productivity of terrestrial ecosystems and further studies on the impacts of light limitation on vegetation productivity are needed. In light-limited ecosystems such as rainforests and temperate forests, the radiative impact of aerosols on vegetation productivity must be evaluated in combination with other environmental factors, such as temperature and water (O'Sullivan et al., 2016). With fieldwork and numerical studies in the Australian savannah, Whitley et al. (2011) suggested that vegetation productivity was more influenced by light limitation than by water limitation. Actually, the response of photosynthetic capacity to an increase in the concentration of atmospheric aerosol has been extensively studied over almost the past 2 decades (Roderick et al., 2001; Gu et al., 2002; Niyogi et al., 2004; Yamasoe et al., 2006; Oliveira et al., 2007; Mercado et al., 2009; Doughty et al., 2010; Kanniah et al., 2010, 2012, 2013; Zhang et al., 2010; Li et al., 2014; Rap et al., 2015; Tang and Dubayah, 2017) through observations (Steiner et al., 2013) and modeling (Moreira et al., 2017) frameworks.
In this paper, however, we investigate the impact of atmospheric aerosols on the carbon balance over three sites in South America in the deforestation arch of the Amazon forest, making use of a novel approach and combining two different types of modeling techniques. First, we make use of artificial neural networks (ANNs), a machine learning algorithm that can approximate any nonlinear deterministic function (Gentine et al., 2018), to build a new modeling framework from scratch relating a number of state variables (radiation, temperature, humidity, as well as the aerosol optical depth) to energy and mass fluxes for three study sites representing a range of structurally different ecosystems in the Amazon; and second, we use a radiative transfer scheme driven with a unique aerosol optical model developed in do Rosário et al. (2009) and described in do Rosário et al. (2011). A recent study has performed a modeling evaluation of the radiative impact of aerosols over a temperate forest with complex structure in the USA (Lee et al., 2018), but the studies in the Amazon remain mostly observational.
Machine learning has significantly advanced in the last decades, being applied to a number of different research areas and more recently used in Earth system studies as a powerful tool to link physical process models with data-driven relationships (Schneider et al., 2017; Huntingford et al., 2019; Reichstein et al., 2019; Watson-Parris et al., 2019). There are a number of different machine learning techniques that have been applied to Earth system studies including random forests (Rodriguez-Galiano et al., 2012; Yang et al., 2016; McGovern et al., 2017), model tree ensembles (Jung et al., 2010; Yang et al., 2016), empirical orthogonal functions (Yang et al., 2019), principal components analysis (Gosh and Mujumdar, 2008), and ANNs (Krasnopolsky et al., 2005; Goyal et al., 2014; Gentine et al., 2018; Nguyen et al., 2018; Buckland et al., 2019; Wu et al., 2019).
We have chosen ANNs over other machine learning methods because once trained, they are computationally efficient, as most of the computational demand is required during the training phase. Perhaps this is one of the main reasons for their large applicability in several different studies, an advantage over more resource-demanding machine learning techniques. Although ANNs are highly data dependent, sometimes leading to problems of over-fitting and generalizations, ANNs are a good approach for problems with large datasets because they allow any number of input variables.
The main goal of this paper is to investigate, step by step, the effects of the atmospheric aerosol on the variability of CO2 and sensible (H) and latent (LE) heat fluxes in the Amazon region over the deforestation arch, based on the study of three specific experimental sites. The use of ANNs allows us to build up a statistical modeling framework relating meteorological (i.e., temperature, humidity, friction velocity) and radiative variables (i.e., incident photosynthetically active radiation (PAR) at the top of the canopy, the percentage of diffuse PAR) to mass and energy fluxes in the forest, in a first approach to address the radiative impacts on mass and energy fluxes. The follow-up analysis is based on the derivation of a second group of ANNs relating radiative variables to meteorological ones in order to identify the second-order effect of aerosols on surface fluxes. Finally, we make use of the radiative transfer (RT) theory with a process-based RT model to create a hypothetical scenario without aerosols and estimate the potential fluxes of mass and energy, not only considering the first-order impact of aerosols, but also the second-order impact by making use of two distinct groups of ANNs.
With this approach, this study evaluates (i) the impacts of aerosol optical depth at 550 nm on fluxes of heat, water, and CO2; (ii) the characteristics of aerosol present in each of the experimental sites; and (iii) the isolated impact of aerosols on mass and energy fluxes through statistical modeling with ANNs, in comparison to other environmental factors, such as temperature, moisture, and turbulence.
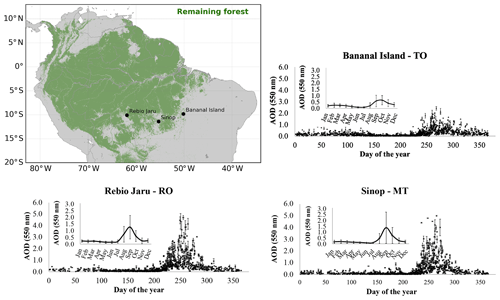
Figure 1Geographical representation of the northern region of Brazil. The darker green area represents remaining forest. The black dots indicate the location of the study sites: Rebio Jaru, RO (west), Sinop, MT (center), and Bananal Island, TO (east). Boxes show the temporal distribution of the aerosol optical depth at 550 nm over the three sites derived from MODIS from 2003 and 2008 and the monthly mean values for the period, with the standard deviation represented by vertical bars.
2.1 Sites
The deforestation arch consists of 248 municipalities in Brazil going from the state of Rondônia to the state of Maranhão, across the Brazilian savannah and Amazonian biomes (Araújo Junior et al., 1998), and it is about 3000 km long and up to 600 km wide. Between July and October 1987, the region of the deforestation arch lost approximately 50 000 km of forests in the states of Pará, Mato Grosso, Rondônia, and Acre and released more than 500×106 t of carbon into the atmosphere, 44×106 t of which were in the form of CO2 (Song et al., 2015). The three flux sites evaluated in this paper are in the Brazilian deforestation arch (Fig. 1).
2.1.1 Bananal Island
Bananal Island is the largest river island on the planet, with approximately 2×106 ha and measuring almost 360 km in the north–south direction and 80 km in the east–west. Bananal Island is a floodplain region. The flux tower of Bananal Island was installed in the northern part of the island, within the boundaries of Canton State Park, by the Laboratory of Climate and Biosphere of the Institute of Astronomy, Geophysics, and Atmospheric Sciences of the University of São Paulo. The average height of the canopy is approximately between 15 to 18 m. The instrumental platform was mounted in a 40 m high structure located approximately 2 km east of the Araguaia River, at the geographic coordinates 9∘49.27′ S, 50∘08.92′ W, at 120 m a.s.l. The data monitoring started on the 27 October 2003 and the project was based on the facilities of the Canguçú Research Centre of the Instituto Ecológica – UFT, approximately 20 km south of the micrometeorological tower (Borma et al., 2009).
2.1.2 Rebio Jaru
In the northeastern part of the state of Rondônia, Brazil, the Jaru Biological Reserve has approximately 60 000 ha. The entire basin of the Tarumã river is in the reserve and covers about 75 % of the unit. The land has a large number of small streams and springs keeping a moist vegetation throughout the year. The vegetation is mainly characterized as an open tropical forest with some palm trees. There are also a few areas with lianas and bamboo trees, as well as small spots of dense ombrophile forest, characteristic of the Amazon region (IBAMA, 2006). The average height of the canopy is approximately 35 m, but some trees can reach up to 45 m. The tower is located 1240 m from the river bank in a more preserved region (Gomes, 2011). Data from Rebio Jaru were collected from August to October 2007, at the geographic coordinates 10∘04.71′ S, 61∘56.01′ W, at 148 m a.s.l. Eddy covariance instruments were installed at 62 m in height (Bosveld and Beljaars, 2001).
2.1.3 Sinop
This study site is located in an area of logging with forest management in Fazenda Maracaí. The farm is located approximately 50 km to the northwest of the center of Sinop, Mato Grosso, Brazil, at coordinates 11∘24.70′ S, 55∘19.39′ W, at 423 m a.s.l. The Maracaí farm consists of a transitional tropical forest (between Brazilian savannah and open ombrophile forest) with a continuous canopy, composed of trees 25 to 28 m in height (Vourlitis et al., 2008). The vegetation consists of perennial trees that are characteristic of the transition forest in Mato Grosso. The diversity is high, and there is no predominance of a single tree species. There are approximately 80 species and 35 families of trees with diameter at breast height around 10 cm. The climatological seasonality for the ecotone of the transition forest is similar to that of the tropical and closed forest. The sonic anemometer was installed at 42 m height. The site is part of the LBA (Large Scale Biosphere-Atmosphere Experiment in Amazonia) integrated project and has been carrying out measurements of this nature since 1999.
2.2 Flux tower data
Surface fluxes of CO2, sensible heat, and latent heat were obtained through the eddy covariance technique applied to data collected with sonic anemometers and infrared gas analyzers installed in flux towers located at Bananal Island, Sinop, and the Jaru Biological Reserve (Rebio Jaru). Fluxes were obtained with a temporal resolution of 30 min. Measurements of meteorological variables, such as temperature, relative humidity, and wind speed were collected. Except for the tower in Sinop, the other towers presented radiative measurements such as incident solar radiation and incident and reflected PAR. For Sinop, these variables were calculated by a radiative transfer model, libRadtran, further described in Sect. 3.1.
Table 1Geographic coordinates, altitudes, flux tower height, and the sensors used in the three experimental sites.
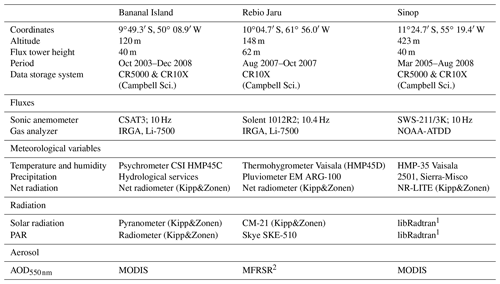
1 Values obtained from the radiative transfer model. 2 Multifilter Rotating Shadowband Radiometer.
Geographic coordinates, altitudes, flux tower heights, and the type of sensors used in each one of the three sites are presented in Table 1. A Multifilter Rotating Shadowband Radiometer data provided aerosol optical depth at 550 nm estimates per minute, and 30 min averaged values were calculated, available only for the Rebio Jaru site.
The study period of this work was limited to the dry season for the following reasons: (a) the maximum numbers of fire outbreaks occur in August, September, and October; (b) in terms of the temporal distribution of the aerosol optical depth, there is a significant increase in the months of August, September, and October; (c) the analysis of the intrinsic properties of the aerosols becomes important because it indicates the chemical composition and size of the particles. Only the months of August, September, and October were considered in this analysis in order to keep consistency of the type of aerosols being evaluated.
2.3 MODIS data
The aerosol optical depth (AOD) at 550 nm used in this study was obtained from the Moderate Resolution Imaging Spectroradiometer (MODIS) for Bananal Island and Sinop. In Rebio Jaru AOD values were obtained in situ with a shadow band radiometer (Multifilter Rotating Shadowband Radiometer, MFRSR) (Table 1). MODIS is an instrument on board the Terra and Aqua satellites. Terra's orbit around the globe is synchronized and runs from north to south across the Equator line in the morning (∼10:30 local time), while Aqua presents passages from south to north by the Equator in the afternoon (∼13:30 local time). MODIS observes the entire ocean surface of the Earth and almost the entire terrestrial surface in a period of 1 to 2 d and acquires data in 36 spectral bands. The aerosol product from MODIS is the aerosol optical depth measured over the oceans and a portion of the continents, globally. In addition, the aerosol size distribution is derived over the oceans, and the aerosol type over continents. Daily MODIS level-2 data are produced with a spatial resolution of 10×10 km2 over nadir. The level 2 product was chosen due to its estimation of the aerosol retrieval uncertainty to assist with error analyses as well as a best estimate of the aerosol optical depth data with quality assurance flags already applied. Although spatial resolution is coarser at level 2, lower product uncertainty improved our analysis.
Two groups of MODIS aerosol data products were collected: MOD04_L2, containing data from the Terra platform, and MYD04_L2, containing data from the Aqua platform. The MODIS aerosol product is used to build the climatological variance of aerosols, sources and sinks of specific types of aerosols (e.g., sulfate aerosols, biomass burning), the interaction of aerosols with clouds, and atmospheric corrections of surface reflectance obtained from remote sensing.
Pre-assumptions about the general structure of aerosol size distribution are necessary for the inversion of the MODIS data and the distribution is described as two log-normal curves: a unique mode, to characterize the particles of accumulation mode (aerosol radius < 0.5 µm) and a single coarse mode to describe dust and salt particles (aerosol radius > 1.0 µm). The aerosol parameters that are thus recovered are the relationship between the two modes (fine and coarse), spectral optical depth, and mean particle size. The quality control of these products is based on the comparison with terrestrial stations and climatology. We used instantaneous and monthly fields of the aerosol optical depth at 550 nm (AOD550nm), referring to collection 051 (Hubanks, 2012), level 2. The pixels over the flux towers were considered. Episodes contaminated by clouds were excluded from the analysis.
The MODIS aerosol data were acquired at the moment of each satellite overpass (Terra and Aqua) over the site of interest, and a single point was used to represent the 30 min interval. Uncertainties associated with each variable were estimated from the standard deviations obtained for the 30 min intervals, except for the MODIS AOD550nm measurements, in which the uncertainty of the AOD550nm in an area of 10×10 km2 was estimated following Levy et al. (2009) as
In Rebio Jaru, in situ AOD measurement was conducted in a relatively limited period of time, covering only part of the dry season of 2007, yet the high temporal resolution of the aerosol optical depth data (every minute) enabled a more robust analysis of the diurnal cycle. On the other hand, at Bananal Island and in Sinop, the time series of flux measurement was longer (from 2003 to 2008 and from 2005 to 2008, respectively). However, AOD data were limited to a maximum of two points a day from MODIS, but the analysis of these experimental sites presented possibilities of characterization of the interannual cycles.
In this section, we discuss the two models used in this study: a physical process-based atmospheric radiative transfer model – libRadtran (Mayer and Kylling, 2005) – and a statistically based model – the artificial neural networks. First, we describe the radiative transfer model; second, we present the machine learning technique (ANNs), and describe the specific methodology used in our experiments. Finally, we describe statistical metrics used to evaluate our results.
3.1 Radiative transfer model
The radiative transfer model libRadtran1.6-beta (Mayer and Kylling, 2005) was used to perform calculations with the incident solar radiation at the top of the atmosphere (TOA) specified for the Amazon region with a tropical atmosphere profile. Ozone concentrations were set to 300 DU based on values obtained by do Rosário et al. (2009), who found an average value of integrated ozone content in the atmospheric column of 257 DU. In this paper, surface albedos from the International Geosphere-Biosphere Programme (IGBP) classification (Loveland and Belward, 1997) were used.
For the three sites, the surface type was set to a deciduous broadleaf forest. The day of the year provides information about the Earth–Sun distance, which affects the amount of radiation incident at the TOA, and it was also used as input to the code. The correction for molecular absorption, parameterized from the LOWTRAN model (Pierluissi and Peng, 1985) and adopted by the SBDART code (Ricchiazzi et al., 1998), was implemented. The resolution of the corrections for molecular absorption was 0.5 nm at wavelengths below 350 and 1.0 nm above. The DISORT method (DIScrete ORdinate Radiative Transfer Solvers) was used to solve the radiative transfer equations because of its versatility and broad use (Mayer et al., 2010). Four streams were used in the simulations performed in this paper. Previous studies have shown that differences in incident irradiance at the surface with at least four streams are minimal (Ma et al., 2011). The PAR waveband ranges between 400 and 700 nm and total solar radiation between 280 and 4000 nm.
The input data required to describe the intensive optical properties of the aerosols were obtained from models created with the Mie theory from do Rosário (2011), derived from the Aerosol Robotic Network (AERONET) products for three experimental sites: Alta Floresta, Abracos Hill, and Rio Branco. These models carry spectral information about the linear extinction coefficient (Q) in km−1, the single scattering albedo (ω0), and the asymmetry factor for the phase function, calculated from the Henyey–Greenstein method (Henyey and Greenstein, 1941). The spectral resolution of the aerosol model in the PAR spectral region was 25 nm, and over the entire solar spectrum it varied from approximately 10 nm at shorter wavelengths up to 700 nm at longer wavelengths.
Three optical models were developed for the biomass burning aerosol emitted over Amazon, which are different in scattering and absorption properties: a more scattering, an intermediate type, and a more absorbing aerosol type. Also, in this study, sensitivity analysis performed with data from the Biological Reserve of Jaru showed that the more scattering aerosol model is the one that best represented the biomass burning aerosols of that season, associated with higher values of AOD550nm. Therefore, in this study, the aerosol model used for all three study sites was the more scattering type.
The specific input parameters of the radiative transfer equations are solar zenith angle and the aerosol optical depth at 550 nm. The model provides the integrated value of the downward direct irradiance, the downward diffuse irradiance, and the upward diffuse irradiance, at the altitude determined by the user. All calculations were determined at the surface. From these values, it is possible to obtain the descending global irradiance (the sum of the contributions of the direct and diffuse irradiance incident at the surface) and the diffuse fraction, i.e., the ratio between the diffuse and incident global irradiance at the surface.
3.2 Artificial neural networks
ANNs have great potential because of their capacity to represent the complexity of the phenomena that are analyzed through them. Traditionally, physical simulation systems have great difficulty in reproducing complex responses of natural events because unknown processes are often unresolved or represented through simplified approaches called “parameterization schemes”, which can limit forecast predictive skill (Huntingford et al., 2019). ANNs can be considered a network of many relatively simple processors, called units or nodes, in which there is a small amount of local memory. In ANNs, these basic local memory units are commonly layered and communicate through channels or connections and operate only on their own media, that is, with their local data and their input parameters (Papale and Valentini, 2003). They can be seen as robust parallel processors with a natural ability to store knowledge gained from experimentation and make this learning available for future use. This means that, with this method, it is possible to create hypothetical scenarios to evaluate the behavior of the output variables, in relation to possible modifications in the input variables.
ANNs are trained from examples contained in the total set of input data. The “weights” are randomly provided at the beginning of the process and modified according to the rule used in the backpropagation method. In this paper, two methods of resolution of ANNs were used. (i) The multilayer perceptron (MLP) is a direct and unidirectional power supply model of artificial neural networks, which maps the input datasets to an appropriate set of output data. The MLP uses the backpropagation technique to train the networks. The most commonly used functions in this method are both sigmoid (Bayram et al., 2016). (ii) The radial basis function (RBF) uses functions of radial basis as a function of activation of the nodes of the neural networks. The RBF method typically has three layers: the input, the intermediate, with a nonlinear activation function, and the output, with a linear function. The input parameters are modeled as a vector composed of real numbers, and the outputs are scalar functions with vector input values (Bayram et al., 2016).
The MLP is a function f: RD→RL, where D is the size of the input vector x and L is the size of the output vector f(x), such that, in matrix notation,
with bias vectors b(1), b(2); weight matrices W(1), W(2), and activation functions G and s. W(1) is the weight matrix connecting the input vector to the hidden layer. Choices of s include tan h and the logistic sigmoid function with ).
The RBF is a function with respect to the origin or a certain point c:
RBF neural networks are good for novelty detection because each neuron is centered on a training example, and so inputs far away from all neurons constitute novel patterns; but it is not as good at extrapolation because it gives the same weight to every attribute as they are considered equally in the distance computation.
For all ANNs used in this paper, 200 neural networks were generated for each one of the different output variables, from all three study sites, and only the one with the smallest error between modeled and observed output was evaluated. From the total dataset, 70 % of the data was randomly chosen and used in the training process of the ANNs, 15 % in the validation process, and 15 % in the testing process. The set used in the training process has the function of exemplifying relations between inputs and outputs to the ANN. This data partitioning was selected to allow the vast majority (> 2∕3) of the data to be used for training, and the remaining data (less than one-third) were equally partitioned between validation and testing because both processes are thought to have the same importance in the ANN construction.
We have used the two most recommended back-propagation algorithms for optimization of ANNs (Rojek, 2017) – the Broyden–Fletcher–Goldfarb–Shanno (BFGS) and radial basis function training algorithm (RBFT) – and the sum of squares (SOSs) to choose between MPL and RBF by selecting the one which presented the smallest deviation to the training data.
The variables used to train the ANNs were incident photosynthetically active radiation at the top of the canopy (PARi) in watts per square meter, the partitioning of photosynthetically active radiation in its diffuse form (PARdif) in percentage, derived from the method of Reindl et al. (1990), the solar zenith angle (SZA) in degrees, the average air temperature in the canopy (T) in degrees Celsius, the vapor pressure deficit (VPD) in kilopascal, the friction velocity (u*) in meters per second, and the aerosol optical depth at 550 nm (AOD550nm). Therefore, seven variables were used as inputs of the ANN, and the flux of carbon dioxide (FCO2), in micromol of CO2 per square meter per second, was selected as output.
The best carbon flux ANN for all sites presented similarities such as all three being generated from the RBF training model, using a Gaussian function for the activation of the hidden units, and the identity function for the activation of the output. However, the ANNs presented a different number of hidden units. The network generated for Bananal Island presented 30 hidden units, the one generated for Sinop used 21, and that for Jaru 19. Basically, the larger the number of hidden units in a neural network, the more robust the model is; i.e., the neural network has a greater capacity to model more complex relationships. At the end, the number of hidden units provides an indication that the database of the three analyzed sites presented different complexities in the relationship between the variables introduced at the moment of the construction of the networks. However, the functions that best managed to describe these relations were the same and the difference between them is the error magnitude between output data used for training and the model output.
The performance of the ANN results was evaluated using the coefficient of determination (r2), which is the Pearson coefficient (r) squared, root mean square error (RMSE), and mean absolute error (MAE), defined as
where p is the total number of data points used for validation only, excluding training and testing, y is the output values of the generated ANNs, and t is the values derived from the flux tower data (FCO2, H, and LE) or meteorological variables (T, VPD, and u*).
RMSE is used to evaluate differences between the values predicted by a mathematical or physical model (y) and the observed values (t). RMSE is a good measure of the accuracy of a model, but only to compare calculations and observations of the same variable, since it is scale dependent. It is obtained by the following equation:
Finally, the use of the MAE does not take into account whether an error was overestimated or underestimated once it is characterized as the average of the errors committed by a model and can be obtained from the following equation:
4.1 Evaluation of ANNs for CO2, sensible heat, and latent heat fluxes
In the absence of clouds, aerosols were responsible for changing the amount of solar radiation available at the surface and the partition between direct and diffuse radiation. This, in turn, impacted surface temperature, and water vapor, among other changes able to affect photosynthesis.
Flux data were combined with meteorological and satellite data. It is important to emphasize that the flux data have a sample interval of 30 min, while the satellite data are a “snapshot” of the atmosphere when the passage occurs in a very short time interval, which registers a value referred to in a single minute but over a 10×10 km2 area.
From the integration of the database it was possible to make an analysis using ANNs. Some precautions were taken in order to avoid a misleading analysis of the ANNs. For example, values of u* smaller or equal to 0.2 m s−1 were excluded from the training, testing, and validation processes to avoid uncertain CO2 flux data collected in episodes with less turbulence and therefore a less mixed atmosphere. All data points for all variables above or below three standard deviations were excluded from the input datasets of the ANNs because they were considered to be outliers.
Seven variables were used as inputs of the ANNs: photosynthetically active radiation incident on surface (PARi), in watts per square meter; the partition of photosynthetically active radiation in diffuse form (PARdif), in percent, derived from the method of Reindl (1990); the solar zenith angle (SZA), in degrees; the mean air temperature in the canopy (T) in degrees Celsius; the vapor pressure deficit (VPD) in kilopascal; the friction velocity (u*), in meters per second; and the aerosol optical depth at 550 nm (AOD550nm). The flux of carbon dioxide (FCO2) (in µmol CO2 m−2 s−1) was selected as output.
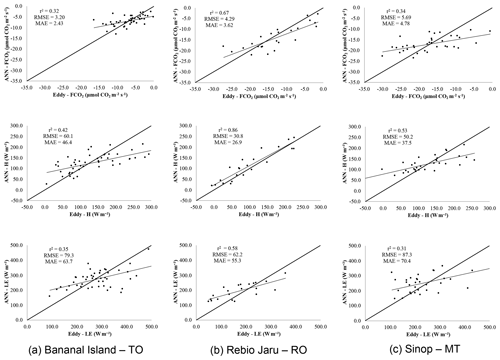
Figure 2FCO2 (µmol CO2 m−2 s−1), H (W m−2), and LE (W m−2) calculated from flux tower data through eddy covariance (x axis) versus modeled by ANNs (y axis), with r2, RMSE, and MAE. The 1:1 straight line (thick) and the linear fit (thin) are also represented for the three study sites.
Figure 2 shows the FCO2 dataset used for validation, obtained through the eddy covariance method on the x axis (eddy) and the ones calculated by the generated ANN, on the y axis, with the respective determination coefficient (r2), RMSE, and MAE, for all three sites.
The Rebio Jaru site presented the highest r2 (0.67) of the three evaluated sites, which represents a high correlation between the measured and modeled CO2 flux data. This site presented continuous AOD550nm data coverage throughout the day. At the other two sites, a single day had a maximum of two AOD550nm data points associated with the passages of the Terra and Aqua satellites. This result indicates that a continuous daytime coverage of AOD generated a more robust ANN influencing FCO2 with more predictability.
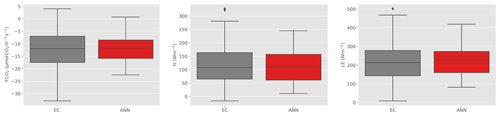
Figure 3Box and whisker plots with extreme data points added for the diurnal cycle of FCO2 (µmol CO2 m−2 s−1), H (W m−2), and LE (W m−2) calculated from flux tower data through eddy covariance (EC) (gray) and modeled by ANNs (red) for 6 d (23, 24, 25 and 29 August and 1 and 10 September) over the dry season of 2007 in Rebio Jaru. The height of the box portion is given by the interquartile range of the dataset and extends from the 25th to 75th percentile. The horizontal bar within the box denotes the median value. The ends of the whiskers are drawn to the10th and 90th percentile values. The extreme values are points at the maximum and minimum.
Figure 3 shows box and whisker plots for the diurnal cycle of FCO2 (µmol CO2 m−2 s−1), H (W m−2), and LE (W m−2) for flux tower data obtained through the eddy covariance (EC) method and modeled with ANNs for 6 d (23, 24, 25 and 29 August and 1 and 10 September) over the dry season of 2007 in Rebio Jaru. It is possible to see that on all evaluated days, the ANN results represent the measured data well. Although for the three fluxes (FCO2, H, and LE), the ANNs are not able to capture the extreme values of the distribution (the 10th and 90th percentiles), the median and both quartiles are well represented. During the evaluated period (August, September, and October 2007), the mean value of FCO2 was −12.2 µmol CO2 m−2 s−1, while the average FCO2 generated by the ANN for the same period was −12.4 µmol CO2 m−2 s−1, a relative difference of 1.9 %.
The Bananal Island site presented the lowest values of RMSE (3.20) and MAE (2.43). because of its long temporal series, from October 2003 to December 2008, with 320 input points, which was important to establish a more robust mapping of the intra-seasonal variability. Throughout the evaluated period, the mean observed FCO2 for Bananal Island was −7.0 µmol CO2 m−2 s−1, while the average FCO2 generated by the ANN was −7.1 µmol CO2 m−2 s−1, a relative difference of 1.3 %.
The Sinop site presented the largest discrepancies between the model and observed FCO2 with an r2 of 0.37, RMSE of 5.69 µmol CO2 m−2 s−1, and MAE of 4.78 µmol CO2 m−2 s−1. Because its database is of intermediate size compared to the other sites (237 points) and no PAR measurements were performed at Sinop, values were generated with the radiative transfer model libRadtran. The errors associated with the outputs of the radiative transfer model were included in the neural network training process. The relative difference between the mean observed and simulated FCO2 was 1.2 %.
In order to evaluate the radiative effects of aerosol optical depth on turbulent energy fluxes, the same type of analysis as performed for carbon dioxide flux was done for sensible heat flux (H) and latent heat flux (LE). The evaluated period was also the same: August, September, and October, the dry and burning season in the region. For all three experimental sites the ANNs were made from the same variables used in the networks related to FCO2.
All data points above or below three standard deviations were excluded from the input sets because they were considered to be outliers and because of the choice of ANNs.
As for FCO2, Rebio Jaru presented the highest r2 (0.87) for sensible heat flux results. However, the same behavior was not observed when evaluating latent heat flux, which presented a relatively lower r2 (0.58) in comparison. In general, the overall results of LE compared to H presented relatively smaller r2 values. The diurnal cycle of H is more well-behaved in relation to LE as it depends on the diurnal cycle of temperature. Latent heat flux, on the other hand, is greatly influenced by the advection of moisture coming from other sources not exclusively related to stomatal opening by the local vegetation. The presence of springs nearby could explain peaks in local maxima of latent heat flux. This can be seen in Fig. S3 in the Supplement, which shows the diurnal cycle (for some days of the experiment) of measured H and LE fluxes (black dots) and the respective curves calculated from ANNs (red lines).
During the evaluated period (August, September, and October 2007), the mean H observed was 117.4 Wm−2, while the average of H generated by ANN for the same period was 111.7 Wm−2, a relative difference of 4.9 %. For LE, the mean value of the observed data was 218.1 Wm−2 and the average value generated by ANN was 218.7 Wm−2, a relative difference of 0.3 %. Although the analyses performed between the validation data and those obtained through ANNs presented better results for H, the relative difference between the mean values of LE – calculated and observed – was lower.
It is important to emphasize that the analysis performed between the validation data and the modeled data generated by ANNs is a way of determining if the neural network is robust. This does not necessarily mean that a low r2 indicates that a given ANN has low predictability. The evaluation of the relative deviation is a way of measuring the accuracy of an ANN. Although it is possible to note in Fig. 2b that the ANN of H could better approximate the measured values, the relative error associated with ANN of LE was around 4.6 % lower.
Bananal Island presented intermediate r2 values (0.42 for H and 0.35 for LE), which indicates an average correlation between the observed and the modeled data. For relative deviations, the values were lower than 3 % for H data and lower than 1 % for LE.
For Sinop, the analyses of H presented an r2 of 0.53, considered from medium to high performance of the ANN, and a relative deviation of 0.5 %. For LE, the r2 was 0.27 and the relative error was 1.2 %.
4.2 Modeling CO2 fluxes with ANNs with constant temperature and moisture
From the generated ANNs, tests were performed to determine the behavior of FCO2 in different scenarios for all three evaluated sites. In the first test, the values of T, VPD, and u* were fixed in order to isolate aerosol radiative effect on the availability of photosynthetically active radiation at the surface and the partitioning of it in its direct and diffuse forms. This first test is hypothetical, since it is known that the fixed variables also suffer influences of AOD550nm. In the test, AOD550nm varied from 0.0 to 2.0 and the radiative transfer model libRadtran was used to estimate values of PARi and PARdif values for three different solar zenith angles (15, 30, and 45∘). The results related to Bananal Island can be seen in Fig. 4. The other sites showed similar behavior.
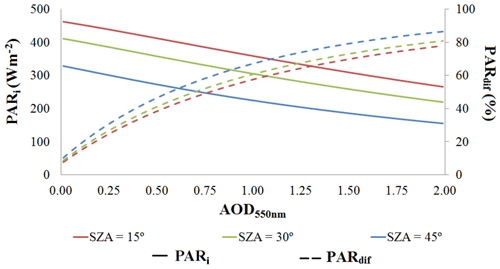
Figure 4PARi (Wm−2) (continuous lines) and PARdif ( %) (dashed lines) versus AOD550nm, for three different SZAs (15, 30, and 45∘) calculated by the radiative transfer model, libRadtran, for Bananal Island for a characteristic day of the dry season.
The values of T, VPD, and u* were fixed at the “optimal point” of FCO2, i.e., for which values of T, VPD, and u* FCO2 presented the largest absolute values. For the other sites, arbitrary values were fixed close to those found at Bananal Island. Table 2 shows the values of T, VPD, and u* fixed for each one of the evaluated sites. Figure 5 shows the variation in FCO2 in relation to AOD550nm for three different SZAs (15, 30, and 45∘) at the three evaluated sites. For Bananal Island and Rebio Jaru, the increase in AOD550nm generates an initial decrease in the absolute values of FCO2 for almost all SZAs, except for the solar zenith angle of 30∘ at Bananal Island, which appears to have no impact on CO2 flux until AOD550nm ∼0.25. However, from this value on, the FCO2 values show a decreasing trend.
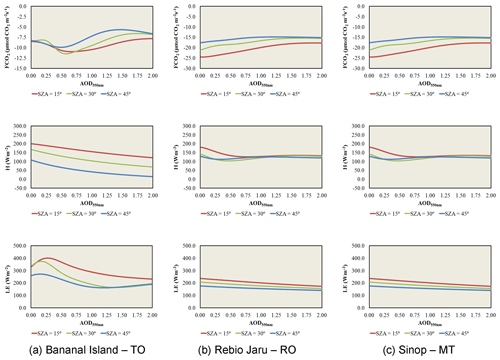
Figure 5Modeled FCO2 (µmol CO2 m−2 s−1), H (W m−2), and LE (W m−2) via ANNs versus AOD550nm for three SZAs (15, 30, and 45∘) for three study sites. Values of temperature, VPD, and u* were kept constant. PARi and PARdif were obtained through libRadtran.
Table 2Fixed values of temperature (T), vapor pressure deficit (VPD), and friction velocity (u*) for three sites evaluated in the first test.
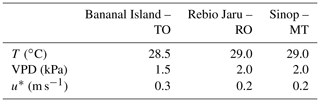
The FCO2 at Bananal Island presents a minimum point with the variation in AOD550nm, while in Rebio Jaru, FCO2 presents a more stable behavior. At Bananal Island, after the minimum point of the curve, FCO2 starts to increase with AOD550nm; i.e., there is an optimal range of AOD550nm, which is dependent on the SZA. For SZA , this interval is associated with AOD550nm between 0.59 and 0.93. For SZA , the interval is narrower, with AOD550nm ranging from 0.57 to 0.61. And for SZA , AOD550nm is between 0.45 and 0.55. For higher values of AOD550nm (above 1.75), the behavior of FCO2 also stabilizes but at different points, dependent on SZA.
In Rebio Jaru, on the other hand, this behavior associated with an “optimal range” of AOD550nm influence on the FCO2 curve is not observed. It is possible to identify a value of AOD550nm where the value of FCO2 stabilizes and does not present significant variations anymore. This value of AOD550nm, associated with the stabilization of the curves, also appears correlated with the solar zenith angle. For SZA , this value of AOD550nm is 0.98, for SZA it is 0.88, and for SZA it is 0.65. When analyzing the results for Sinop, this behavior of a decrease in the absolute value of FCO2 with the increase in AOD550nm is not verified. The addition of AOD550nm values from 0.0 to 2.0 generates increases in absolute values, that is, the CO2 changes between the local vegetation and the atmosphere decrease with a higher aerosol load. This behavior is observed up to a value of AOD550nm from where FCO2 value stabilizes, depending on the SZA. For SZA , this value of AOD550nm is 1.85, for SZA it is 1.70, and for SZA AOD550nm =1.25.
4.3 Modeling temperature, humidity, and wind with ANNs
In the first test, temperature, VPD, and u* were fixed as described in Table 2, but these variables are directly related to the available energy at the surface, and, therefore, these variables would also be impacted in the presence of aerosols. Artificial neural networks were built from the same database used to make the previous ANNs but varying its input and output values.
The first variable to be evaluated was the temperature. Three variables were used as input parameters in the training processes of the temperature ANN: PARi (Wm−2), PARdif (%), and SZA (∘). These variables were chosen as inputs for the neural network of temperature because radiation directly impact surface temperature. The available energy at the surface is proportional to PARi and PARdif, linked to the diurnal cycle.
The second evaluated variable was VPD. This variable is related to temperature, which is related to PARi, PARdif, and SZA. Thus, these four variables were used as input values in the construction of the VPD ANN.
Finally, u* is also affected by energy available at the surface but to a lower degree once it is directly dependent on wind speed. All other variables (except for the AOD550nm itself, in order to avoid overtraining) were used as input values in the construction of the u* ANN.
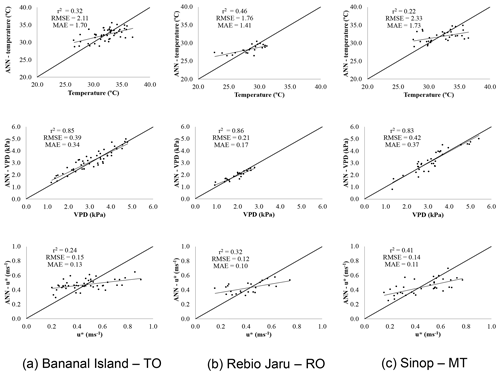
Figure 6Temperature (∘C), VPD (kPa), and u* (m s−1) obtained from ANNs generated with observed values for the three study sites, with respective r2, MAE, and RMSE. The 1:1 line (thick) and the best linear fit (thin) are also shown.
Figure 6 shows data used for the validation, i.e., without interference in the construction of the networks. The values of the x axis correspond to the observed values and those of the y axis to the values generated by the neural networks. The results for the neural networks of VPD, for all evaluated sites, were the most compatible with the values observed, and they presented an r2 greater than 0.8. This is mainly because VPD is closely related to temperature. The explanation of the variance of VPD through the variables used as inputs of the ANNs was mainly governed by temperature. At Bananal Island, temperature explained 50.5 % of the VPD variance; in Rebio Jaru and Sinop, it explained 65.6 % and 38.1 %, respectively. Temperature and friction velocity presented a moderate value of r2 (between 0.2 and 0.5) for all cases. However, in the overall sensitivity analysis, PARi was mostly responsible for the explanation of the variance of temperature.
4.4 Isolating the radiative impact of aerosols on CO2, sensible heat, and latent heat fluxes with ANN modeling
From the generated ANNs, a study was performed in order to identify what the relative differences would be in FCO2, H, and LE fluxes between real observed conditions and an atmosphere without aerosols, i.e., with AOD550nm =0.0 for the evaluated cases.
First, the radiative transfer model, libRadtran, was used to obtain PARi and PARdif values for an atmosphere with AOD550nm =0.0. For the calculations, the real values of SZA and day of the year were used. The results for the Rebio Jaru site can be visualized in Fig. 7. For the other study sites, the same behavior of the curves is observed.
It is possible to identify that the observed values of PARi are systematically lower than those obtained by the radiative transfer model, while the opposite is observed for PARdif. Aerosol decreases the incident shortwave global radiation at the surface by absorption and scattering processes, while it increases the percentage of diffuse radiation due to the scattering. From PARi and PARdif calculated for an aerosol-free atmosphere, temperature, VPD, and u* were calculated through the ANNs. The SZA values used as input in the libRadtran were the same as those used in the ANNs.
All variables obtained by both models (libRadtran and ANNs) – except for the values of AOD550nm, which were set to zero, and SZAs, which were kept the same as in the original database – were used in calculations of fluxes of CO2, H, and LE. Table 3 summarizes the results. The mean real values and standard deviations of each of the variables of the initial set of data, the mean model values, and the respective standard deviations are also shown for all variables. The relative difference between the means of the actual values and the simulated ones indicates whether the model presented values larger than the real ones (positive) or smaller (negative).
PARi and PARdif variables agreed over the three sites, when comparing the actual and simulated values. The average of the modeled PARi values, without aerosol, was 12.4 % higher than the average of the real values for the Bananal Island site, 29.8 % higher for Rebio Jaru, and 26.3 % higher for Sinop. On the other hand, the average of the model values of PARdif was 35.9 % lower than the average of the real values for Bananal Island, 52.0 % lower for Rebio Jaru, and 38.0 % lower for Sinop.
Temperature also showed agreement regarding the increase in the mean values modeled in relation to the mean real values. At Bananal Island, the increase was 11.3 %, in Rebio Jaru it was 7.4 %, and in Sinop the relative difference was 1.7 %. The relative difference between the actual and modeled mean values of VPD and u* did not show agreement for the three experimental sites. However, Bananal Island presented a significant increase in VPD (∼50 %) for an atmosphere with no aerosols.
As demonstrated by Doughty et al. (2010) for a tropical forest, there is a decrease of approximately 13 % in the FCO2 for every 1 ∘C increase in temperature, when it is above 28 ∘C. Due to the high correlation between VPD and T, the results of the model without aerosols agree with the authors.
For FCO2, Bananal Island and Rebio Jaru presented higher mean modeled values; i.e., a lower exchange of CO2 with the atmosphere is expected under the effect of episodes without aerosols. Sinop presented the opposite effect. This is mainly due to the differences in structural local characteristics of each site. The response of photosynthetic capacity to an increase in diffuse radiation may vary between different ecosystems and seems to be related to the different properties of canopies (Zhang et al., 2010; Kanniah et al., 2012; Tang and Dubayah, 2017). Niyogi (2004) showed that carbon assimilation grows with an increase in aerosol concentration in the case of forests and cultivated areas and decreases over pastures.
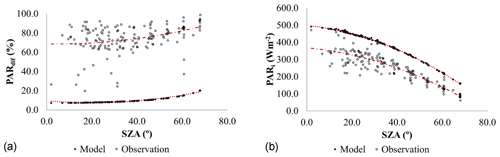
Figure 7PARdif (%) (a) and PARi (Wm−2) (b) versus SZA (∘), for Rebio Jaru. The observed values of PARi and PARdif – obtained by the method of Reindl (1990) – are represented by open circles, while the values calculated through libRadtran with AOD=0 are represented by the black dots. The curves fitted to the datasets are shown in red.
Table 3Mean values with standard deviations of the turbulent fluxes of CO2 (FCO2), sensible heat (H), and latent heat (LE), in µmol CO2 per square meter per second and watts per square meter, respectively. These values were used as input parameters for building ANNs (measured), mean values and standard deviations generated from the aerosol free scenarios (modeled), and relative differences (%) between the measured and the modeled values for all three study sites.
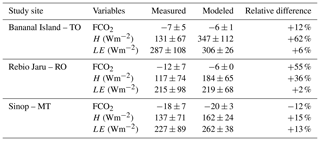
The surrounding areas of the flux tower in Sinop show several regions of pasture, which later became soy or maize plantations. For this reason, the less complex canopy structures in the region (with lower vegetation and more homogeneity in the vertical and horizontal distribution) have opposite responses to that proposed by the “diffuse fertilization effect” theory. The complexity of the canopies at Bananal Island and Rebio Jaru confirms the effect.
The same type of test was performed in order to evaluate the relative differences in H and LE under conditions of an observed aerosol concentration and a simulated atmosphere without aerosols, with AOD550nm =0.0. In this test, energy (H and LE) fluxes were obtained using variables calculated by the models (libRadtran and ANNs), except for the values of AOD550nm (0.0) and SZAs, which were obtained through the original data. Table 3 shows the average real values and the standard deviations of the fluxes, H and LE, for the observed database, the average model values, and the relative difference between the means of the real values and the simulated ones.
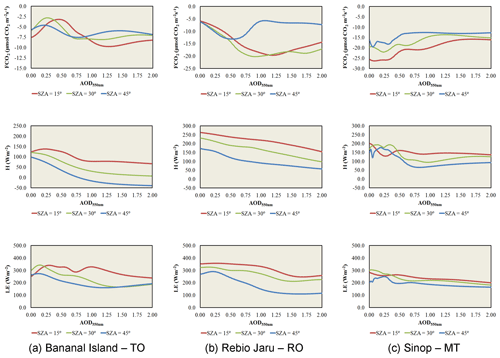
Figure 8Modeled FCO2 (µmol CO2 m−2 s−1), H (W m−2), and LE (W m−2) via ANNs versus AOD550nm for three SZAs (15, 30, and 45∘) for three study sites. Values of temperature, VPD, and u* were statistically modeled with ANNs built from meteorological data. PARi and PARdif were obtained through libRadtran.
For all three sites, the values of sensible and latent heat fluxes calculated through the ANNs for a scenario without aerosols were higher than the mean observed values. It was observed that, for all experimental sites, an aerosol-free atmosphere was associated with higher PARi and lower PARdif values, which means that in an aerosol-free atmosphere, there are conditions for more available energy at the surface, which may explain the increase in the mean values of sensible and latent heat fluxes. The higher availability of surface radiative energy was used for the increase in temperature. The analysis of energy fluxes indicates that in the presence of aerosols, there is a total reduction in both heat and water exchange between the surface and the atmosphere over the evaluated sites.
Over all experimental sites, sensible heat flux was shown to be more susceptible to changes in AOD550nm compared to latent heat flux. This fact is greatly observed at Bananal Island and Rebio Jaru, but less in Sinop. A possible explanation for this phenomenon can be obtained by evaluating the absorption spectrum of water. The spectral region most affected by the presence of biomass burning aerosols is the PAR, and evaluations were limited to this spectrum only. This spectral region interacts very little with water. Another possible explanation is due to the temperature rise in the canopy itself. The vegetation responds to temperature increase with a stomatal closure, which decreases evapotranspiration. Aerosols are more efficient in affecting the exchange of heat between the atmosphere and the vegetation than the exchange of moisture between the two.
In this study, we evaluated the impact of AOD550nm on land fluxes of CO2, sensible heat, and latent heat for three study sites located in the deforestation arch region of the Brazilian Amazon using a combination of ANNs and radiative transfer modeling.
Aerosols were responsible for changing the amount of solar radiation available at the surface and the partition between direct and diffuse radiation. The radiative impact of aerosols caused variations in the temperature and humidity as well as in FCO2. For the impact of aerosol on the flux of CO2, the modeled values with ANN for Rebio Jaru indicated a strong dependence on solar zenith angle. Temperature and VPD were important in explaining FCO2 variance for all study sites.
For Bananal Island and Rebio Jaru, the increase in AOD550nm initially generates a decrease in the absolute values of FCO2 to AOD550nm ∼0.25. However, for higher values of AOD550nm, FCO2 decreased, which indicates higher photosynthetic rates. In Sinop this behavior was not observed.
We developed a group of ANNs relating meteorological and radiative variables for three sites in the Amazon region (Fig. 8). For Bananal Island, the behavior of decreasing FCO2 with the increase in AOD550nm remained. For Rebio Jaru, FCO2 decreases with AOD550nm up to approximately AOD550nm =0.50 and showed the opposite behavior after that. For Sinop, the behavior between both scenarios remained basically the same.
In order to obtain the total radiative impact of aerosols on FCO2, a third test consisted of a study to define the relative differences in FCO2 between conditions in the presence of aerosol and without aerosols (AOD550nm =0.0) in a combination of two distinct methodologies: ANNs and radiative transfer modeling. The average of the modeled PARi values without aerosol was higher than the average of the real values for all sites, while the average of modeled values of PARdif was lower, as expected. Temperature also increased in the modeled scenario without aerosols in relation to the one containing aerosols. The relative difference between the actual and modeled mean values of VPD and u* did not show agreement for the three experimental sites.
Bananal Island and Rebio Jaru presented higher values of modeled CO2 flux in the scenario without aerosol, while Sinop presented the opposite result. This was due to the canopy structural properties of Sinop and surroundings presenting several crop fields. For this reason, the less complex canopy architecture in the region acted to decrease the photosynthetic response of the vegetation to less total incident PAR and more radiation in its diffuse form. We conclude that in the absence of aerosols, CO2 flux from the atmosphere to the surface in Rebio Jaru could be reduced to more than half its current values in the presence of aerosols. This effect is only observable by taking secondary effects into account.
The same type of evaluation was performed for the fluxes of sensible heat and latent heat using ANNs. When evaluating the variables that best explain the variance of sensible heat flux for Bananal Island, VPD and T showed a strong influence of 27.4 % and 23.7 %, respectively. This fact indicates that energy fluxes of Bananal Island are influenced by temperature. In the case of Rebio Jaru, PARi explained about 17 % of the variance of H. For Sinop, there is no favorable variable explaining the variances of H and LE. However, friction velocity (u*) presented the highest index of sensitivity (15 %), which indicates that the variance of both energy fluxes was more influenced by turbulence itself.
In the first test, the behavior of the fluxes with AOD550nm showed similarities in all three sites. The average behavior of both H and LE decreased with an increase in aerosol optical depth. The increase in aerosol concentration decreases total incident radiative energy at the surface and thus generates a systematic decrease in H and LE. In the second test, the variation in other meteorological variables affected the fluxes and, although differences were present, the general behavior of energy fluxes with aerosol optical depth remained. Sensible heat flux and latent heat flux decreased with higher AOD550nm.
In the scenario with AOD550nm =0.0, the values of sensible and latent heat fluxes were higher than the observed values. This indicates that in an atmosphere without aerosols, more energy is available at the surface. Sensible heat flux was shown to be more susceptible to changes in AOD550nm compared to latent heat flux. This fact is reported in the Bananal Island and Rebio Jaru datasets, but less in Sinop. The radiative spectral region most affected by the presence of the biomass burning aerosols is the PAR, and only the aerosol optical depth at 550 nm was evaluated. The radiation at 550 nm interacts little with water vapor. Also, an increase in canopy temperature may lead to stomatal closure decreasing total evapotranspiration, which can impact latent heat flux negatively.
The two groups of ANNs developed in this study, i.e., characterizing the first- and second-order radiative impact of aerosols on mass and energy fluxes, can be used for flux data gap filling, as well as modeling and evaluating future climate. More evaluations of the impact of aerosols on energy fluxes throughout the Amazon basin are needed, and machine learning techniques are an efficient and accessible tool to develop these studies.
It is important to highlight some important caveats of the ANN methodology used to drawn our conclusions, including, but not limited to, general extrapolations; for example, a limited number of sites were used in this study; there is a high dependence on sampling together with other data biases, e.g., only the dry season used here; and there was the non-consideration of all confounding factors of the examined problem – e.g., a limited number of input variables (seven) were used to build the ANNs throughout these experiments. Yet, using restricted datasets and objective choices of features to study rather than an extensive and generic approach remains valid and important. Moreover, expert intervention associated with best practices is expected to diminish these weaknesses associated with machine learning techniques (Reichstein et al., 2019).
Future efforts must include ways to go around the main caveats of this work, including the following: (i) the addition of multi-source (more field sites), multi-scale (the whole Amazon basin represented at different resolutions), and complex spatiotemporal relations (intra- and inter-seasonal variability of flux data, as well as advection of aerosols from different sources); (ii) uncertainty estimation should be integrated into models; (iii) using physically based models to test the statistical ones, e.g., linking radiative transfer and ecophysiology models to evaluate the theoretical first-order impact of aerosols on light scattering and photosynthesis. Deep learning techniques such as recurrent neural networks (Schmidhuber, 2015) or long short-term memories (Hochreiter and Schmidhuber, 1997) may be able to contour some of these weaknesses (Reichstein et al., 2019).
Nevertheless, coupling machine learning techniques at local and regional levels with land surface models (Moreira et al., 2017) and Earth system models (Malavelle et al., 2019) can improve the representation of poorly known process-based mechanisms or add information about unknown relationships between meteorological variables and surface fluxes.
Flux and aerosol data used in this study are available at https://doi.org/10.6084/m9.figshare.8239691.v1 (Braghiere et al., 2019).
The supplement related to this article is available online at: https://doi.org/10.5194/acp-20-3439-2020-supplement.
RKB designed the study, conducted the analysis, and wrote the paper with help from all the other authors. MAY coordinated the intensive field campaign at Rebio Jaru in the dry season of 2007 and contributed to the writing of the paper. NMEdR developed the aerosols optical models. HRdR, JdSN, and ACdA coordinated the measurements and shared the flux data for Bananal Island, Sinop, and Rebio Jaru, respectively.
The authors declare that they have no conflict of interest.
This research was carried out in part at the Jet Propulsion Laboratory, California Institute of Technology, under a contract with the National Aeronautics and Space Administration. The authors would like to thank the staff from ICMBio at Rebio Jaru. Renata Aguiar, Federal University of Rondônia, Campus Ji Paraná, and the LBA local office for collecting and processing the flux data. Also, thanks to Frederico Trindade Teófilo and students of the LBA local office, as well as to Ruth Souza and other employees for the support during the field campaign. The authors thank three anonymous reviewers, whose comments improved the paper.
This work was partly funded by the Coordenação de Aperfeiçoamento de Pessoal de Nível Superior – Brasil (CAPES) – Finance Code 001, at the University of São Paulo, Brazil. Marcia Akemi Yamasoe acknowledges FAPESP (grant number 06-56550-5) and the National Council for Scientific and Technological Development – Brasil (CNPq) for grant number 313005/2018-4.
This paper was edited by Janne Rinne and reviewed by three anonymous referees.
Araújo Junior, G. J. L. D. de, Pereira, J. A. R., Silva, T. M. V. da, Mafra, H., Brass, J. A., Lockwood, R. N., Higgins, R. G., and Riggan, P. J.: Utilização do sensor AIRDAS (Airborne Infrared Disaster Assessment System) no monitoramento de desflorestamentos no norte do Estado do Mato Grosso – Brasil, in: Simpósio Brasileiro de Sensoriamento Remoto, 9 (SBSR), edited by: Krug, T., Rudorff, B. F. T., and de Freitas, U. M., 1433–1442, INPE, São José dos Campos, 1998.
Bayram, S., Ocal, M. E., Laptali Oral, E., and Atis, C. D.: Comparison of multi layer perceptron (MLP) and radial basis function (RBF) for construction cost estimation: the case of Turkey, J. Civ. Eng. Manag., 22, 480–490, https://doi.org/10.3846/13923730.2014.897988, 2016.
Black, K., Davis, P., Lynch, P., Jones, M., McGettigan, M., and Osborne, B.: Long-term trends in solar irradiance in Ireland and their potential effects on gross primary productivity, Agr. Forest Meteorol., 141, 118–132, https://doi.org/10.1016/j.agrformet.2006.09.005, 2006.
Borma, L. S., da Rocha, H. R., Cabral, O. M., von Randow, C., Collicchio, E., Kurzatkowski, D., Brugger, P. J., Freitas, H., Tannus, R., Oliveira, L., Rennó, C. D., and Artaxo, P.: Atmosphere and hydrological controls of the evapotranspiration over a floodplain forest in the Bananal Island region, Amazonia, J. Geophys. Res., 114, G01003, https://doi.org/10.1029/2007JG000641, 2009.
Bosveld, F. C. and Beljaars, A. C. M.: The impact of sampling rate on eddy-covariance flux estimates, Agr. Forest Meteorol., 109, 39–45, https://doi.org/10.1016/S0168-1923(01)00257-X, 2001.
Boucher, O., Randall, D., Artaxo, P., Bretherton, C., Feingold, G., Forster, P., Kerminen, V.-M. V.-M., Kondo, Y., Liao, H., Lohmann, U., Rasch, P., Satheesh, S. K., Sherwood, S., Stevens, B., Zhang, X. Y., and Zhan, X. Y.: Clouds and Aerosols, Clim. Chang. 2013 Phys. Sci. Basis. Contrib. Work. Gr. I to Fifth Assess. Rep. Intergov. Panel Clim. Chang., https://doi.org/10.1017/CBO9781107415324.016, 2013.
Buckland, C. E., Bailey, R. M., and Thomas, D. S. G.: Using artificial neural networks to predict future dryland responses to human and climate disturbances, Sci. Rep., 9, 3855, https://doi.org/10.1038/s41598-019-40429-5, 2019.
Chin, M., Ginoux, P., Kinne, S., Torres, O., Holben, B. N., Duncan, B. N., Martin, R. V., Logan, J. A., Higurashi, A., and Nakajima, T.: Tropospheric Aerosol Optical Thickness from the GOCART Model and Comparisons with Satellite and Sun Photometer Measurements, J. Atmos. Sci., 59, 461–483, https://doi.org/10.1175/1520-0469(2002)059<0461:TAOTFT>2.0.CO;2, 2002.
do Rosário, N. E., Yamasoe, M. A., and Longo, K. M.: Aerosol Optical Depth and Ångström Coefficient retrievals over the Amazon Forest during 2007 biomass burning season, 1100, 494–497, https://doi.org/10.1063/1.3117029, 2009.
do Rosário, N. E., Yamasoe, M. A., Brindley, H., Eck, T. F., and Schafer, J.: Downwelling solar irradiance in the biomass burning region of the southern Amazon: Dependence on aerosol intensive optical properties and role of water vapor, J. Geophys. Res.-Atmos., 116, D18304, https://doi.org/10.1029/2011JD015956, 2011.
Doughty, C. E., Flanner, M. G., and Goulden, M. L.: Effect of smoke on subcanopy shaded light, canopy temperature, and carbon dioxide uptake in an Amazon rainforest, Global Biogeochem. Cy., 24, https://doi.org/10.1029/2009GB003670, 2010.
Ezhova, E., Ylivinkka, I., Kuusk, J., Komsaare, K., Vana, M., Krasnova, A., Noe, S., Arshinov, M., Belan, B., Park, S.-B., Lavrič, J. V., Heimann, M., Petäjä, T., Vesala, T., Mammarella, I., Kolari, P., Bäck, J., Rannik, Ü., Kerminen, V.-M., and Kulmala, M.: Direct effect of aerosols on solar radiation and gross primary production in boreal and hemiboreal forests, Atmos. Chem. Phys., 18, 17863–17881, https://doi.org/10.5194/acp-18-17863-2018, 2018.
Fletcher, C. G., Kravitz, B., and Badawy, B.: Quantifying uncertainty from aerosol and atmospheric parameters and their impact on climate sensitivity, Atmos. Chem. Phys., 18, 17529–17543, https://doi.org/10.5194/acp-18-17529-2018, 2018.
Forster, P., Ramaswamy, V., Artaxo, P., Berntsen, T., Betts, R., Fahey, D. W., Haywood, J., Lean, J., Lowe, D. C., Myhre, G., Nganga, J., Prinn, R., Raga, G., Schulz, M., and Van Dorland, R.: Changes in Atmospheric Constituents and in Radiative Forcing, in Climate Change 2007: The Physical Science Basis. Contribution of Working Group I to the Fourth Assessment Report of the Intergovernmental Panel on Climate Change, 2007.
Gentine, P., Pritchard, M., Rasp, S., Reinaudi, G., and Yacalis, G.: Could Machine Learning Break the Convection Parameterization Deadlock?, Geophys. Res. Lett., 45, 5742–5751, https://doi.org/10.1029/2018GL078202, 2018.
Gomes, J. B.: Conversão de florestas tropicais em sistemas pecuários na Amazônia: quais são as implicações no microclima da região?, Universidade Federal de Rondônia, 2011.
Ghosh, S. and Mujumdar, P. P.: Statistical downscaling of GCM simulations to streamflow using relevance vector machine, Adv. Water Resour., 31, 132–146, https://doi.org/10.1016/j.advwatres.2007.07.005, 2008.
Goyal, M. K., Bharti, B., Quilty, J., Adamowski, J., and Pandey, A.: Modeling of daily pan evaporation in sub tropical climates using ANN, LS-SVR, Fuzzy Logic, and ANFIS, Expert Syst. Appl., 41, 5267–5276, https://doi.org/10.1016/j.eswa.2014.02.047, 2014.
Gu, L., Baldocchi, D., Verma, S. B., Black, T. A., Vesala, T., Falge, E. M., and Dowty, P. R.: Advantages of diffuse radiation for terrestrial ecosystem productivity, J. Geophys. Res.-Atmos., 107, ACL 2-1–ACL 2-23, https://doi.org/10.1029/2001JD001242, 2002.
He, J., Glotfelty, T., Yahya, K., Alapaty, K., and Yu, S.: Does temperature nudging overwhelm aerosol radiative effects in regional integrated climate models?, Atmos. Environ., 154, 42–52, https://doi.org/10.1016/j.atmosenv.2017.01.040, 2017.
Henyey, L. C. and Greenstein, J. L.: Diffuse radiation in the Galaxy, Astrophys. J., 93, 70, https://doi.org/10.1086/144246, 1941.
Hochreiter, S. and Schmidhuber, J.: Long Short-Term Memory, Neural Comput., 9, 1735–1780, https://doi.org/10.1162/neco.1997.9.8.1735, 1997.
Hubanks, P. A.: MODIS Atmosphere QA Plan for Collection 005, 67, available at: https://atmosphere-imager.gsfc.nasa.gov/sites/default/files/ModAtmo/QA_Plan_C61_Master_2017_03_15.pdf, last access: 16 March 2020, 2017.
Huntingford, C., Jeffers, E. S., Bonsall, M. B., Christensen, H. M., Lees, T., and Yang, H.: Machine learning and artificial intelligence to aid climate change research and preparedness, Environ. Res. Lett., 14, 124007, https://doi.org/10.1088/1748-9326/ab4e55, 2019.
IBAMA: Plano de prevenção e combate aos incêndios florestais da Reserva Biologógica do Jaru (2006–2007), available at: http://piwik.ibama.gov.br/phocadownload/prevfogo/planos_operativos/plano_operativo_reserva_biolgica_do_jaru.pdf, last access: 16 March 2020, 2006.
Jung, M., Reichstein, M., Ciais, P., Seneviratne, S. I., Sheffield, J., Goulden, M. L., Bonan, G., Cescatti, A., Chen, J., de Jeu, R., Dolman, A. J., Eugster, W., Gerten, D., Gianelle, D., Gobron, N., Heinke, J., Kimball, J., Law, B. E., Montagnani, L., Mu, Q., Mueller, B., Oleson, K., Papale, D., Richardson, A. D., Roupsard, O., Running, S., Tomelleri, E., Viovy, N., Weber, U., Williams, C., Wood, E., Zaehle, S., and Zhang, K.: Recent decline in the global land evapotranspiration trend due to limited moisture supply, Nature, 467, 951–954, https://doi.org/10.1038/nature09396, 2010.
Kanniah, K. D., Beringer, J., Tapper, N. J., and Long, C. N.: Aerosols and their influence on radiation partitioning and savanna productivity in northern Australia, Theor. Appl. Climatol., 100, 423–438, https://doi.org/10.1007/s00704-009-0192-z, 2010.
Kanniah, K. D., Beringer, J., North, P., and Hutley, L.: Control of atmospheric particles on diffuse radiation and terrestrial plant productivity, Prog. Phys. Geogr. Earth Environ., 36, 209–237, https://doi.org/10.1177/0309133311434244, 2012.
Kanniah, K. D., Beringer, J., and Hutley, L.: Exploring the link between clouds, radiation, and canopy productivity of tropical savannas, Agr. Forest Meteorol., 182–183, 304–313, https://doi.org/10.1016/j.agrformet.2013.06.010, 2013.
Kiehl, J. T. and Trenberth, K. E.: Earth's Annual Global Mean Energy Budget, B. Am. Meteorol. Soc., 78, 197–208, https://doi.org/10.1175/1520-0477(1997)078<0197:EAGMEB>2.0.CO;2, 1997.
Krasnopolsky, V. M., Fox-Rabinovitz, M. S., and Chalikov, D. V.: New Approach to Calculation of Atmospheric Model Physics: Accurate and Fast Neural Network Emulation of Longwave Radiation in a Climate Model, Mon. Weather Rev., 133, 1370–1383, https://doi.org/10.1175/MWR2923.1, 2005.
Lee, M. S., Hollinger, D. Y., Keenan, T. F., Ouimette, A. P., Ollinger, S. V., and Richardson, A. D.: Model-based analysis of the impact of diffuse radiation on CO2 exchange in a temperate deciduous forest, Agric. For. Meteorol., 249, 377–389, https://doi.org/10.1016/j.agrformet.2017.11.016, 2018.
Levy, R. C., Leptoukh, G. G., Kahn, R., Zubko, V., Gopalan, A., and Remer, L. A.: A critical look at deriving monthly aerosol optical depth from satellite data, IEEE T. Geosci. Remote, 47, 2942–2956, https://doi.org/10.1109/TGRS.2009.2013842, 2009.
Li, T., Heuvelink, E., Dueck, T. A., Janse, J., Gort, G., and Marcelis, L. F. M.: Enhancement of crop photosynthesis by diffuse light: quantifying the contributing factors, Ann. Bot., 114, 145–156, https://doi.org/10.1093/aob/mcu071, 2014.
Loveland, T. R. and Belward, A. S.: The IGBP-DIS global 1km land cover data set, DISCover: First results, Int. J. Remote Sens., 18, 3289–3295, https://doi.org/10.1080/014311697217099, 1997.
Malavelle, F. F., Haywood, J. M., Mercado, L. M., Folberth, G. A., Bellouin, N., Sitch, S., and Artaxo, P.: Studying the impact of biomass burning aerosol radiative and climate effects on the Amazon rainforest productivity with an Earth system model, Atmos. Chem. Phys., 19, 1301–1326, https://doi.org/10.5194/acp-19-1301-2019, 2019.
Mayer, B. and Kylling, A.: Technical note: The libRadtran software package for radiative transfer calculations – description and examples of use, Atmos. Chem. Phys., 5, 1855–1877, https://doi.org/10.5194/acp-5-1855-2005, 2005.
Mayer, B., Hoch, S. W., and Whiteman, C. D.: Validating the MYSTIC three-dimensional radiative transfer model with observations from the complex topography of Arizona's Meteor Crater, Atmos. Chem. Phys., 10, 8685–8696, https://doi.org/10.5194/acp-10-8685-2010, 2010.
McGovern, A., Elmore, K. L., Gagne, D. J., Haupt, S. E., Karstens, C. D., Lagerquist, R., Smith, T., and Williams, J. K.: Using Artificial Intelligence to Improve Real-Time Decision-Making for High-Impact Weather, B. Am. Meteorol. Soc., 98, 2073–2090, https://doi.org/10.1175/BAMS-D-16-0123.1, 2017.
Mercado, L. M., Bellouin, N., Sitch, S., Boucher, O., Huntingford, C., Wild, M., and Cox, P. M.: Impact of changes in diffuse radiation on the global land carbon sink, Nature, 458, 1014–1017, https://doi.org/10.1038/nature07949, 2009.
Moreira, D. S., Longo, K. M., Freitas, S. R., Yamasoe, M. A., Mercado, L. M., Rosário, N. E., Gloor, E., Viana, R. S. M., Miller, J. B., Gatti, L. V., Wiedemann, K. T., Domingues, L. K. G., and Correia, C. C. S.: Modeling the radiative effects of biomass burning aerosols on carbon fluxes in the Amazon region, Atmos. Chem. Phys., 17, 14785–14810, https://doi.org/10.5194/acp-17-14785-2017, 2017.
Nguyen, P., Ombadi, M., Sorooshian, S., Hsu, K., AghaKouchak, A., Braithwaite, D., Ashouri, H., and Thorstensen, A. R.: The PERSIANN family of global satellite precipitation data: a review and evaluation of products, Hydrol. Earth Syst. Sci., 22, 5801–5816, https://doi.org/10.5194/hess-22-5801-2018, 2018.
Niyogi, D., Chang, H. I., Saxena, V. K., Holt, T., Alapaty, K., Booker, F., Chen, F., Davis, K. J., Holben, B., Matsui, T., Meyers, T., Oechel, W. C., Pielke, R. A., Wells, R., Wilson, K., and Xue, Y.: Direct observations of the effects of aerosol loading on net ecosystem CO2 exchanges over different landscapes, Geophys. Res. Lett., 31, L20506, https://doi.org/10.1029/2004GL020915, 2004.
Oliveira, P. H. F., Artaxo, P., Pires, C., De Lucca, S., Procópio, A., Holben, B., Schafer, J., Cardoso, L. F., Wofsy, S. C., and Rocha, H. R.: The effects of biomass burning aerosols and clouds on the CO2 flux in Amazonia, Tellus B, 59, 338–349, 2007.
O'Sullivan, M., Rap, A., Reddington, C. L., Spracklen, D. V., Gloor, M., and Buermann, W.: Small global effect on terrestrial net primary production due to increased fossil fuel aerosol emissions from East Asia since the turn of the century, Geophys. Res. Lett., 43, 8060–8067, https://doi.org/10.1002/2016GL068965, 2016.
Papale, D. and Valentini, R.: A new assessment of European forests carbon exchanges by eddy fluxes and artificial neural network spatialization, Glob. Change Biol., 9, 525–535, https://doi.org/10.1046/j.1365-2486.2003.00609.x, 2003.
Persad, G. G. and Caldeira, K.: Divergent global-scale temperature effects from identical aerosols emitted in different regions, Nat. Commun., 9, 3289, https://doi.org/10.1038/s41467-018-05838-6, 2018.
Pierluissi, J. H. and Peng, G.-S.: New Molecular Transmission Band Models For LOWTRAN, Opt. Eng., 24, https://doi.org/10.1117/12.7973523, 1985.
Rap, A., Spracklen, D. V., Mercado, L., Reddington, C. L., Haywood, J. M., Ellis, R. J., Phillips, O. L., Artaxo, P., Bonal, D., Restrepo Coupe, N., and Butt, N.: Fires increase Amazon forest productivity through increases in diffuse radiation, Geophys. Res. Lett., 42, 4654–4662, https://doi.org/10.1002/2015GL063719, 2015.
Reichstein, M., Camps-Valls, G., Stevens, B., Jung, M., Denzler, J., Carvalhais, N., and Prabhat: Deep learning and process understanding for data-driven Earth system science, Nature, 566, 195–204, https://doi.org/10.1038/s41586-019-0912-1, 2019.
Reindl, D. T., Beckman, W. A., and Duffie, J. A.: Evaluation of hourly tilted surface radiation models, Sol. Energy, 45, 9–17, https://doi.org/10.1016/0038-092X(90)90061-G, 1990.
Ricchiazzi, P., Yang, S., Gautier, C., and Sowle, D.: SBDART: A Research and Teaching Software Tool for Plane-Parallel Radiative Transfer in the Earth's Atmosphere, B. Am. Meteorol. Soc., 79, 2101–2114, https://doi.org/10.1175/1520-0477(1998)079<2101:SARATS>2.0.CO;2, 1998.
Roderick, M. L., Farquhar, G. D., Berry, S. L., and Noble, I. R.: On the direct effect of clouds and atmospheric particles on the productivity and structure of vegetation, Oecologia, 129, 21–30, https://doi.org/10.1007/s004420100760, 2001.
Rodriguez-Galiano, V. F., Ghimire, B., Rogan, J., Chica-Olmo, M., and Rigol-Sanchez, J. P.: An assessment of the effectiveness of a random forest classifier for land-cover classification, ISPRS J. Photogramm. Remote Sens., 67, 93–104, https://doi.org/10.1016/j.isprsjprs.2011.11.002, 2012.
Rojek, I.: Technological process planning by the use of neural networks, Artif. Intell. Eng. Des. Anal. Manuf., 31, 1–15, https://doi.org/10.1017/S0890060416000147, 2017.
Schmidhuber, J.: Deep learning in neural networks: An overview, Neural Networks, 61, 85–117, https://doi.org/10.1016/j.neunet.2014.09.003, 2015.
Schneider, T., Lan, S., Stuart, A., and Teixeira, J.: Earth System Modeling 2.0: A Blueprint for Models That Learn From Observations and Targeted High-Resolution Simulations, Geophys. Res. Lett., 44, 12396–12417, https://doi.org/10.1002/2017GL076101, 2017.
Seinfeld, J. H. and Pandis, S. N.: Atmospheric Chemistry and Physics: From Air Pollution to Climate Change, New Jersey, John Wiley & Sons, inc., 1225 p., Senna, 2006.
Song, X.-P., Huang, C., Saatchi, S. S., Hansen, M. C., and Townshend, J. R.: Annual Carbon Emissions from Deforestation in the Amazon Basin between 2000 and 2010, edited by B. Hérault, PLoS One, 10, e0126754, https://doi.org/10.1371/journal.pone.0126754, 2015.
Stanhill, G. and Cohen, S.: Global dimming: a review of the evidence for a widespread and significant reduction in global radiation with discussion of its probable causes and possible agricultural consequences, Agr. Forest Meteorol., 107, 255–278, https://doi.org/10.1016/S0168-1923(00)00241-0, 2001.
Steiner, A. L., Mermelstein, D., Cheng, S. J., Twine, T. E., and Oliphant, A.: Observed Impact of Atmospheric Aerosols on the Surface Energy Budget, Earth Interact., 17, 1–22, https://doi.org/10.1175/2013EI000523.1, 2013.
Tang, H. and Dubayah, R.: Light-driven growth in Amazon evergreen forests explained by seasonal variations of vertical canopy structure, P. Natl. Acad. Sci. USA, 114, 2640–2644, https://doi.org/10.1073/pnas.1616943114, 2017.
Textor, C., Schulz, M., Guibert, S., Kinne, S., Balkanski, Y., Bauer, S., Berntsen, T., Berglen, T., Boucher, O., Chin, M., Dentener, F., Diehl, T., Easter, R., Feichter, H., Fillmore, D., Ghan, S., Ginoux, P., Gong, S., Grini, A., Hendricks, J., Horowitz, L., Huang, P., Isaksen, I., Iversen, I., Kloster, S., Koch, D., Kirkevåg, A., Kristjansson, J. E., Krol, M., Lauer, A., Lamarque, J. F., Liu, X., Montanaro, V., Myhre, G., Penner, J., Pitari, G., Reddy, S., Seland, Ø., Stier, P., Takemura, T., and Tie, X.: Analysis and quantification of the diversities of aerosol life cycles within AeroCom, Atmos. Chem. Phys., 6, 1777–1813, https://doi.org/10.5194/acp-6-1777-2006, 2006.
Trenberth, K. E., Fasullo, J. T., and Kiehl, J.: Earth's Global Energy Budget, B. Am. Meteorol. Soc., 90, 311–324, https://doi.org/10.1175/2008BAMS2634.1, 2009.
Unger, N., Yue, X., and Harper, K. L.: Aerosol climate change effects on land ecosystem services, Faraday Discuss., 200, 121–142, https://doi.org/10.1039/C7FD00033B, 2017.
Vourlitis, G. L., De Souza Nogueira, J., De Almeida Lobo, F., Sendall, K. M., De Paulo, S. R., Antunes Dias, C. A., Pinto, O. B., and De Andrade, N. L. R.: Energy balance and canopy conductance of a tropical semi-deciduous forest of the southern Amazon Basin, Water Resour. Res., 44, https://doi.org/10.1029/2006WR005526, 2008.
Wang, Y., Wang, M., Zhang, R., Ghan, S. J., Lin, Y., Hu, J., Pan, B., Levy, M., Jiang, J. H., and Molina, M. J.: Assessing the effects of anthropogenic aerosols on Pacific storm track using a multiscale global climate model, P. Natl. Acad. Sci. USA, 111, 6894–6899, https://doi.org/10.1073/pnas.1403364111, 2014.
Watson-Parris, D., Sutherland, S., Christensen, M., Caterini, A., Sejdinovic, D., and Stier, P.: Detecting anthropogenic cloud perturbations with deep learning, available at: http://arxiv.org/abs/1911.13061 (last access: 12 March 2020), 2019.
Whitley, R. J., Macinnis-Ng, C. M. O., Hutley, L. B., Beringer, J., Zeppel, M., Williams, M., Taylor, D., and Eamus, D.: Is productivity of mesic savannas light limited or water limited? Results of a simulation study, Glob. Change Biol., 17, 3130–3149, https://doi.org/10.1111/j.1365-2486.2011.02425.x, 2011.
Yamasoe, M. A., von Randow, C., Manzi, A. O., Schafer, J. S., Eck, T. F., and Holben, B. N.: Effect of smoke and clouds on the transmissivity of photosynthetically active radiation inside the canopy, Atmos. Chem. Phys., 6, 1645–1656, https://doi.org/10.5194/acp-6-1645-2006, 2006.
Yang, H., Piao, S., Huntingford, C., Peng, S., Ciais, P., Chen, A., Zhou, G., Wang, X., Gao, M., and Zscheischler, J.: Strong but Intermittent Spatial Covariations in Tropical Land Temperature, Geophys. Res. Lett., 46, 356–364, https://doi.org/10.1029/2018GL080463, 2019.
Yang, Y., Guan, H., Batelaan, O., McVicar, T. R., Long, D., Piao, S., Liang, W., Liu, B., Jin, Z., and Simmons, C. T.: Contrasting responses of water use efficiency to drought across global terrestrial ecosystems, Sci. Rep., 6, 23284, https://doi.org/10.1038/srep23284, 2016.
Wu, C., Chen, Y., Peng, C., Li, Z., and Hong, X.: Modeling and estimating aboveground biomass of Dacrydium pierrei in China using machine learning with climate change, J. Environ. Manage., 234, 167–179, https://doi.org/10.1016/j.jenvman.2018.12.090, 2019.
Zhang, M., Yu, G.-R., Zhang, L.-M., Sun, X.-M., Wen, X.-F., Han, S.-J., and Yan, J.-H.: Impact of cloudiness on net ecosystem exchange of carbon dioxide in different types of forest ecosystems in China, Biogeosciences, 7, 711–722, https://doi.org/10.5194/bg-7-711-2010, 2010.