the Creative Commons Attribution 4.0 License.
the Creative Commons Attribution 4.0 License.
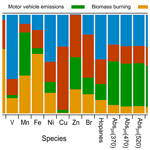
Optical source apportionment and radiative effect of light-absorbing carbonaceous aerosols in a tropical marine monsoon climate zone: the importance of ship emissions
Qiyuan Wang
Huikun Liu
Ping Wang
Wenting Dai
Ting Zhang
Youzhi Zhao
Jie Tian
Wenyan Zhang
Yongming Han
Junji Cao
Source-specific optical properties of light-absorbing carbonaceous (LAC) aerosols in the atmosphere are poorly understood because they are generated by various sources. In this study, a receptor model combining multi-wavelength absorption and chemical species was used to explore the source-specific optical properties of LAC aerosols in a tropical marine monsoon climate zone. The results showed that biomass burning and ship emissions were the dominant contributors to average aerosol light absorption. The source-specific absorption Ångström exponent (AAE) indicated that black carbon (BC) was the dominant LAC aerosol in ship and motor vehicle emissions. Moreover, brown carbon (BrC) was present in biomass-burning emissions. The source-specific mass absorption cross section (MAC) showed that BC from ship emissions had a stronger light-absorbing capacity compared to emissions from biomass burning and motor vehicles. The BrC MAC derived from biomass burning was also smaller than the BC MAC and was highly dependent on wavelength. Furthermore, radiative effect assessment indicated a comparable atmospheric forcing and heating capacity of LAC aerosols between biomass burning and ship emissions. This study provides insights into the optical properties of LAC aerosols from various sources. It also sheds more light on the radiative effects of LAC aerosols generated by ship emissions.
- Article
(2560 KB) - Full-text XML
-
Supplement
(6985 KB) - BibTeX
- EndNote
Carbonaceous aerosols are abundant in PM2.5 (particulate matter with an aerodynamic diameter ≤ 2.5 µm) (e.g., 20 %–50 % of PM2.5 mass; Putaud et al., 2010; Tao et al., 2017) and have extensively been explored due to their implications on global climate forcing (IPCC, 2013). Among the complex carbonaceous compounds are the light-absorbing carbonaceous (LAC) aerosols which are mainly associated with absorption of light. LAC aerosols consist of black carbon (BC) and brown carbon (BrC). BC is a short-lived climate forcer with a strong ability to absorb sunlight. Moreover, it is regarded as the second largest contributor of positive anthropogenic climate forcing after carbon dioxide (Bond et al., 2013). On the other hand, BrC refers to a class of light-absorbing organic compounds with enhanced light absorption at short wavelengths (e.g., near-ultraviolet region). Therefore, it is a potential contributor to atmospheric heating at both global and regional scales (Laskin et al., 2015).
The optical properties of LAC aerosols are closely related to their sources as well as atmospheric conditions and secondary processing. However, distinguishing source-specific light absorption by LAC from a mixture of aerosols in the atmosphere is still a challenge. It is possible to use multi-wavelength light absorption data to identify optical source apportionment based on the Beer–Lambert law (e.g., Aethalometer model and multi-wavelength absorption analyzer model; Sandradewi et al., 2008; Massabò et al., 2015), which can typically explain two different types of sources (e.g., fossil fuels versus biomass burning). Results from this method are highly dependent on the use of the source-specific absorption Ångström exponent (AAE). However, due to lack of source-specific AAE data, most studies use empirical values reported in previous literature (e.g., Healy et al., 2017; Küpper et al., 2018; Zheng et al., 2019). This may create inconsistencies in the reported results because the source-specific AAE varies with the type of fuels and their burning efficiencies (Tian et al., 2019).
In addition, optical source apportionment can be obtained using receptor models (e.g., positive matrix factorization (PMF) and Multilinear Engine (ME-2)). Several studies have utilized receptor models to identify sources, first based on the sole chemical species or mass spectra information. Thereafter, a multiple linear regression model is used to apportion the contribution of each source to the optical parameters of an aerosol (Qin et al., 2018; Tian et al., 2020). This method may be referred to as indirect optical source apportionment. In contrast, Forello et al. (2019) coupled chemical species with multi-wavelength absorption in ME-2 to directly perform optical source apportionment. Compared to the indirect approach, the additional optical data in receptor models can improve the performance of source apportionment because each source has its own optical features. Furthermore, it may eliminate potential uncertainties caused by multiple operations in the indirect approach. However, the application of direct optical source apportionment is scarce at the moment.
Alternatively, laboratory studies may effectively be used to explore the optical properties of LAC from a specific source (e.g., vehicle engine exhaust, coal combustion, and biomass burning) (Tian et al., 2019; Xie et al., 2017). However, the optical properties of LAC may significantly change due to the complex atmospheric processes that they undergo after emission into the atmosphere. Therefore, it is critical to identify LAC aerosols from different sources in the atmosphere using specific methods in order to obtain their optical properties. Furthermore, to the best of our knowledge, there is no study focusing on the optical properties of ship-exhaust-related LAC aerosols in the atmosphere. This presents a challenge to our understanding of the role of ship emissions in the climate considering that it is a significant part of discharge from the transport sector.
In this study, multi-wavelength aerosol light absorption and chemical species were measured in Sanya, a coastal city in China. This was done to investigate the optical properties of LAC aerosols from ship emissions and other sources. A dataset combining optical and chemically speciated data was used simultaneously in a receptor model to obtain the optical source apportionment. Afterwards, the source-specific optical properties of LAC aerosols were determined and characterized. Finally, the impact of radiative effect induced by LAC aerosols from different sources was evaluated. This study provides insights into the source-specific optical properties of LAC aerosols from various sources. Additionally, it reinforces knowledge on the radiative effects of LAC aerosols.
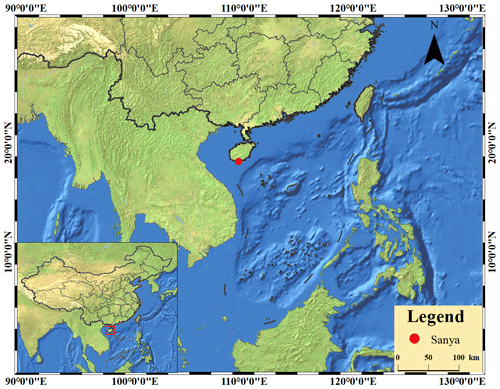
Figure 1Map showing the Sanya sampling site and surrounding areas. The map was drawn using ArcGIS software. The base map is the World Topographic Map from © ESRI (Environmental Systems Research Institute, Inc.) (http://www.arcgis.com/home/item.html?id=30e5fe3149c34df1ba922e6f5bbf808f, last access: February 2020).
2.1 Sampling site
The sampling site is located in Sanya, a small city (an area of 1921.5 km2 and a total population of 0.59 million as at 2017) in southernmost tip of the Hainan Island in southern China (Fig. 1). Comprehensive measurements were taken in spring from 12 April to 14 May 2017 on the rooftop of a teaching building (about 20 m above ground level) in Hainan Tropical Ocean University (18.30∘ N, 109.52∘ E). The sampling site is predominantly an educational and residential area with typical urban sources of emissions including vehicles and cooking appliances. Sanya lies within a tropical marine monsoon climate zone; therefore the weather was warm (temperature = 28 ± 3 ∘C) and wet (relative humidity (RH) = 81 ± 12 %) during the study.
2.2 Online and offline measurements
A model AE33 Aethalometer (Magee Scientific, Berkeley, CA, USA) was used to determine the light absorption coefficients of the aerosols at multiple wavelengths (Abs(λ); λ is wavelength) with a PM2.5 cyclone (SCC 1.829, BGI Inc. USA). Briefly, the collected particles were desiccated (RH = 22 ± 7 %) using a Nafion® dryer (MD-700-24S-3; Perma Pure, Inc., Lakewood, NJ, USA) before measurement with the AE33 Aethalometer. As shown in Fig. S1, the loss of Abs(λ) caused by the dryer was ignored. Afterwards, seven light-emitting diodes (λ= 370, 470, 520, 590, 660, 880, and 950 nm) in the AE33 Aethalometer were used to irradiate the filter deposition spot to obtain light attenuation as previously described (Drinovec et al., 2015). Since the AE33 Aethalometer records the BC mass concentrations, the Abs(λ) values at each wavelength were retrieved by getting the product of BC mass concentration ([BC]) and mass absorption cross section (MAC) used in the instrument (Abs(λ)= [BC] × MAC) (Drinovec et al., 2015). One of the advantages of the AE33 Aethalometer is that it resolves the filter loading effect using a dual-spot compensation technique. Further details regarding the principles of operation of the AE33 Aethalometer have been outlined by Drinovec et al. (2015).
In addition, a photoacoustic extinctiometer (PAX, Droplet Measurement Technologies, Boulder, CO, USA) was used to directly measure aerosol light absorption at λ= 532 nm. It was set in parallel with the AE33 Aethalometer using the same PM2.5 cyclone and Nafion® dryer. Briefly, the PAX adopts an intracavity photoacoustic technique, with a modulated laser beam heating up the sampled particles in an acoustic chamber. The pressure wave generated from heating is then detected by a sensitive microphone. Moreover, aerosol light scattering can be measured using a wide-angle integrating reciprocal nephelometer in a scattering chamber. In this study, different concentration gradients of ammonium sulfate and freshly generated propane soot were used to calibrate light-scattering and absorption measurements, respectively. The calibration procedure was described in detail by Q. Wang et al. (2018a).
The PM2.5 quartz-fiber filters (8×10 inch) (QM/A; GE Healthcare, Chicago, IL, USA) were collected during the day (from 08:00 to 20:00) and at night (from 20:00 to 08:00 the next day) using a high-volume air sampler (Tisch Environmental, Inc., USA) with a flow rate of 1.13 m3 min−1. Before sampling, the blank quartz-fiber filters were heated in a muffle furnace at 805 ∘C for 3 h to remove possible impurities. After sampling, the quartz-fiber filters were saved in a freezer at about −20 ∘C to minimize evaporation of volatile material before chemical analyses. Finally, field blanks were collected and analyzed to eliminate potential background artifacts.
The collected quartz-fiber filters were used to analyze inorganic elements, carbonaceous matter, water-soluble ions, and organics. An energy-dispersive X-ray fluorescence (ED-XRF) spectrometer (Epsilon 5 ED-XRF, PANalytical B.V., Netherlands) was used to determine the titanium (Ti), vanadium (V), manganese (Mn), ferrum (Fe), nickel (Ni), copper (Cu), zinc (Zn) and bromine (Br) quantities. A detailed description of the principles of ED-XRF has been highlighted by Xu et al. (2012). Moreover, a thermal–optical carbon analyzer (Desert Research Institute model 2001, Atmoslytic Inc., Calabasas, CA, USA) was used to analyze organic carbon (OC) and elemental carbon (EC). A detailed analytical procedure has been described elsewhere (Chow et al., 2007). An ion chromatograph (IC, Dionex 600; Dionex Corporation, Sunnyvale, CA, USA) was also used to quantify the water-soluble cations (i.e., Na+, K+, Mg2+, Ca2+ and NH) and anions (i.e., Cl−, NO and SO) as described by Zhang et al. (2011). Finally, an in-injection port thermal desorption (TD) instrument coupled with an Agilent 7890/5975C gas chromatographer–mass spectrometer (GC–MS) (Agilent Technologies, Santa Clara, CA, USA) was used to determine the hopanes using a protocol described by J. Wang et al. (2016).
2.3 Segregation of BC and BrC absorption
The Abs(λ) consisted of light absorption from both LAC aerosols (BC and BrC) and mineral dust (Wang et al., 2013). Therefore, LAC absorption (AbsLAC(λ)) was calculated as follows:
where AbsBC(λ), AbsBrC(λ) and Absmineral(λ) were absorption of light by BC, BrC and mineral dust at λ= 370, 470, 520, 590, 660 or 880 nm, respectively (in units of per million meters). The Absmineral(λ) was retrieved from the optical source apportionment as discussed in Sect. 3.2. With an assumption of BC only absorbing at λ= 880 nm, the AbsBC(λ) at wavelengths of 370, 470, 520, 590 and 660 was extrapolated as follows:
where AAEBC represents BC AAE, which was assumed to be 1.1 based on a study by Lack and Langridge (2013). Combining Eqs. (1) and (2) gave the following equation:
From the perspective of emission and formation, the Abs(λ) could be divided into light absorption contributed by primary emissions (Abspri(λ)) and secondary formation (Abssec(λ)). Therefore, the Abs(λ) could be calculated as follows:
A BC-tracer method was utilized to separate Abssec(λ) from Abspri(λ), and Eq. (4) could further be developed as follows (Wang et al., 2019a):
where described the ratio of Abs(λ) to BC mass concentration in primary emissions (in units of square meters per gram) and [BC] denoted the mass concentration of BC in the atmosphere (in units of micrograms per cubic meter). This was retrieved from the relationship between Abs(880) measured by the AE33 Aethalometer and EC mass concentration. Finally, the ratio was determined using a minimum R-squared (MRS) method previously described by Wang et al. (2019a).
2.4 Estimation of optical parameters
AAE reflects spectral dependence of aerosol light absorption and can be used to distinguish the chemical composition of LAC aerosols. For example, LAC aerosol dominated by BC has an AAE close to 1.0 while the presence of BrC results in an AAE larger than 1.0 (Andreae and Gelencsér, 2006). As described previously, AAE could be retrieved using a power-law function as follows (Andreae and Gelencsér, 2006):
where C is a constant independent of wavelength.
Additionally, MAC could be used to reflect the light absorption capacity of aerosols. The MACs of BC and BrC at different wavelengths (MACBC(λ) and MACBrC(λ), respectively) were calculated with AbsBC(λ) and AbsBrC(λ) divided by the corresponding mass concentrations of BC and organic matter (OM), respectively:
where the mass concentration of OM was estimated by a factor of 1.8 times that of OC mass concentration (Turpin and Lim, 2001).
2.5 Receptor model source apportionment
The PMF version 5.0 (PMF5.0) from the US Environmental Protection Agency (Norris et al., 2014) was applied to determine the contribution of various sources to aerosol light absorption. The principle of PMF has been described elsewhere (Paatero and Tapper, 1994). Briefly, PMF decomposes the initial dataset into a factor contribution matrix Gik (i×k dimensions) and a factor profile matrix Fkj (k×j dimensions) and then iteratively minimizes the object function Q:
where Xij was the value of the jth species in the ith sample, Eij described the model residual and σij represented uncertainty, which was calculated as follows:
where MDL was the method detection limit and the error fraction was set to 10 % (Rai et al., 2020). The uncertainties of the PMF5.0 results were evaluated by the following analyses: bootstrap (BS), displacement (DISP) and bootstrap–displacement (BS–DISP). The BS analysis assesses the random errors in PMF solutions while DISP estimates rotational ambiguity. On the other hand, BS–DISP estimates both random errors and rotational ambiguity. A more detailed description of the three error estimation methods has been provided by Paatero et al. (2014) and Brown et al. (2015).
2.6 The Optical Properties of Aerosols and Clouds (OPAC) model
The OPAC model was used to retrieve the following parameters: aerosol optical depth (AOD), single-scattering albedo (SSA) and asymmetric parameter (AP). The parameters were important in estimating the radiative effect of aerosols. A detailed description of the OPAC software package was given by Hess et al. (1998). The refractive index of BC and other species used in the OPAC model is shown in Fig. S2. The measured mass concentrations of OC, EC and water-soluble ions as well as the estimated mineral dust loading (= [Fe] ∕ 0.035) during the day were used in the OPAC model to estimate the optical parameters. Moreover, the BC number concentration in the OPAC model was constrained by the measured EC mass concentration. Although several water-soluble ions and mineral dust were obtained, they did not contain all the water-soluble and insoluble material. Therefore, based on the measured data, the number concentrations of water-soluble and insoluble materials were tuned. This was done until the differences between the OPAC-derived light scattering, light absorption and SSA versus the corresponding PAX-measured values were within 5 % (Fig. S3). After the aerosol light extinction coefficient (sum of light scattering and absorption) was obtained, the AOD was estimated as follows (Hess et al., 1998):
where Hj,max and Hj,min are the upper and lower boundaries in layer j, σe,j is the surface aerosol light extinction coefficient in layer j, h is the layer height, represents the aerosol light extinction coefficient that was normalized to 1 particle cm−3, Nj is the number concentration in layer j, and Z was the scale height. Furthermore, the OPAC-derived AODs were tuned to match the satellite-derived AODs (https://giovanni.gsfc.nasa.gov/giovanni, last access: January 2020) by altering the scale height in OPAC until the difference between them was within 5 %. Owing to closure with AOD and anchoring of chemical composition, the assumptions in the OPAC model did not have a significant impact on the estimation of radiative effect in subsequent Sect. 2.7 (Satheesh and Srinivasan, 2006).
2.7 Estimations of radiative effect and heating rate
The LAC direct radiative effect (DRE) was estimated by the Santa Barbara DISORT (Discrete Ordinate Radiative Transfer) Atmospheric Radiative Transfer (SBDART) model in the shortwave spectral region of 0.25–4.0 µm. A detailed description of the SBDART model was given by Ricchiazzi et al. (1998). The AOD, SSA and AP are essential input parameters in the SBDART model and were obtained from the OPAC model (see Sect. 2.6). In addition to these, several other input parameters were included, namely the surface albedo, solar zenith angle and profiles of atmospheric parameters. The surface albedo was derived from the Moderate Resolution Imaging Spectroradiometer (https://modis-atmos.gsfc.nasa.gov/ALBEDO/index.html, last access: January 2020). On the other hand, the solar zenith angle was calculated with a specific time and location (i.e., latitude and longitude) using a small code from the SBDART model. Furthermore, six standard atmospheric vertical profiles (i.e., tropical, midlatitude summer, subarctic summer, midlatitude winter, subarctic winter and US62) were embedded in the SBDART model. They provided vertical distributions of temperature, pressure, water vapor and ozone density (Ricchiazzi et al., 1998). In this study, the midlatitude summer was selected to represent the situation of Sanya based on its classification as a midlatitude region. Obregón et al. (2015) demonstrated that the SBDART model could provide a reliable estimation of radiative effect. Moreover, aerosol DRE was defined as the difference in the radiation flux (F) either at the Earth's surface or at the top of the atmosphere, respectively with and without the aerosol in the atmosphere:
where ↓ and ↑ represent the downward and upward fluxes, respectively. Atmospheric DRE was then estimated by the difference between the DRE at the top of the atmosphere and the Earth's surface.
Further, the atmospheric heating rate (, in units of kelvin per day) caused by LAC aerosols was estimated using the first law of thermodynamics and hydrostatic equilibrium as follows (Liou, 2002):
where is the lapse rate and g stands for acceleration due to gravity while Cp describes the specific heat capacity of air at a constant pressure (1006 J kg−1 K−1). Additionally, ΔF is the atmospheric DRE contributed by LAC aerosols, and ΔP represents the atmospheric pressure difference (300 hPa).
Table 1Summary of light absorption at different wavelengths (Abs(λ), λ= 370, 470, 520, 590, 660 and 880 nm) and absorption Ångström exponent (AAE) of different emission sources.
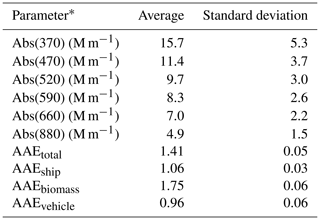
* AAEtotal represents the AAE caused by total light-absorbing aerosols while AAEship, AAEbiomass and AAEvehicle are AAE from ship emissions, biomass burning and motor vehicle emissions, respectively.
3.1 Overview of Abs(λ)
The AE33 absorption was first corrected using PAX measurement, and a strong correlation (r=0.96, p< 0.01) between them was found (Fig. S4). A slope of 2.3 was regarded as the correction factor and was comparable to the values of 2.0–2.6 reported by previous studies using a similar method (Qin et al., 2018; Tasoglou et al., 2017; Wang et al., 2019b). This difference may mainly be related to the matrix scattering and lensing effects. The time series of corrected Abs(λ) is shown in Fig. 2, and a statistical summary of the data is presented in Table 1. The average Abs(λ) values were 15.7 ± 5.3, 11.4 ± 3.7, 9.7 ± 3.0, 8.3 ± 2.6, 7.0 ± 2.2 and 4.9 ± 1.5 M m−1 at 370, 470, 520, 590, 660 and 880 nm, respectively, throughout the study. However, it is noteworthy that such single-wavelength calibrations may overestimate Abs(λ) at long wavelengths (i.e., λ= 590, 660 and 880 nm) and underestimate it at short wavelengths (i.e., λ= 370 and 470 nm) owing to the correction factor's dependence on wavelength (Kim et al., 2019). Compared to previous work, the Abs(λ) values in this study were lower than those obtained from urban areas in China and Europe (J. Wang et al., 2018; Liakakou et al., 2020). However, they were comparable to some rural and remote areas where anthropogenic activities were not intensive (Zanatta et al., 2016; Zhu et al., 2017). This suggests the possibility of a relatively small LAC burden in the atmosphere at Sanya during the study.
Additionally, AbsBC(λ) contributed more than 77 % to Abs(λ), whereas the contribution of AbsBrC(λ) was less than 17 % (Fig. 3). This was consistent with previous studies showing that BC was stronger at absorbing light compared to BrC at the near-ultraviolet to near-infrared wavelengths in the atmosphere (Massabò et al., 2015; Liakakou et al., 2020). However, laboratory studies reported that AbsBrC(λ) could exceed AbsBC(λ) at short wavelengths in fresh smoke from biomass burning, especially in the smoldering phase (Tian et al., 2019; Chow et al., 2018). Furthermore, the fraction of AbsBC(λ) increased with an increase in wavelength, although the fraction of AbsBrC(λ) showed an inverse trend with a dramatic drop from 17 % at 370 nm to 3 % at 660 nm as shown in Fig. 3. This suggests a stronger light-absorbing capacity by BrC at short wavelengths compared to the long ones. With regard to the relationship between Abs(λ) and carbonaceous composition, the AbsBC(λ) correlated well with EC mass concentration (r= 0.93, p< 0.01, Fig. S5). However, a weak but significant correlation was observed between AbsBrC(λ) and OC mass concentration (r= 0.27–0.42, p< 0.05, Fig. S6). The results further confirmed that BC was the dominant light-absorbing material in LAC aerosols while OC contained more non-light-absorbing carbon components compared to the light-absorbing ones.
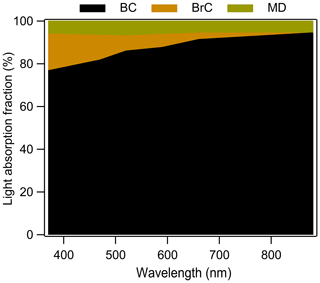
Figure 3Light absorption fractions of BC, BrC and MD in the total light-absorbing aerosols. BC: black carbon; BrC: brown carbon; MD: mineral dust.
3.2 Source apportionment of Abs(λ)
To quantify the contributions of various sources to Abs(λ), chemical species and Abspri(λ) were simultaneously used as input parameters in the PMF5.0 model. Online Abspri(λ) data were integrated to match each filter sampling time. The selected chemical species included carbonaceous matter (i.e., OC and EC), water-soluble cations (i.e., Na+, K+ and Ca2+), elements (i.e., Ti, V, Mn, Fe, Ni, Cu, Zn and Br) and hopanes. The mass concentrations of the chemicals are summarized in Table 2. Based on Eq. (5), Abssec(λ) accounted for less than 5 % of Abs(λ) (Table S1), suggesting a negligible impact of secondary formation on the light absorption capacity of aerosols during the study. Therefore, the uncertainty caused by using only Abspri(λ) in the model could be put to rest in the absence of an effective way to identify the source of secondary BrC.
Moreover, two to seven factors were selected to initiate the PMF5.0 run. Due to the additional factors, the Q∕Qexp ratio decreased with the increased number of factors as shown in Fig. S7. The decrease in Q∕Qexp was large when the factor number changed from 2 to 3 and 3 to 4 but stabilized as the factor number grew larger than 4, indicating that four factors may be the optimal solution. After multiple runs of the PMF5.0 model, four factor sources including ship emissions, motor vehicle emissions, biomass burning and fugitive dust were finally identified (Fig. 4a). Additionally, the modeled Abspri(λ) at different wavelengths showed strong correlations with the measured Abs(λ) (r= 0.82–0.89, p< 0.01, Fig. S8). The slopes of 0.92–0.98 were consistent with the absorption fractions of Abspri(λ) estimated by the BC-tracer method combined with the MRS approach (Table S1). The scaled residuals for each species varied between −3 and +3.
The uncertainty of each factor profile was further evaluated using BS, DISP and BS–DISP. The BS results showed that the reproducibility of each source factor was larger than 80 % (Table S2), indicating good stability. Therefore, this suggested that the four source factors were appropriate. No swaps occurred in DISP, indicating the stability of the selected solution. Furthermore, all BS–DISP runs were successful. Overall, these results pointed to the efficiency of the PMF5.0 model in performing optical source apportionment.
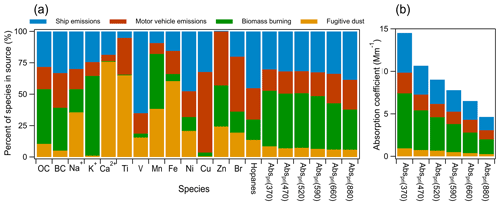
Figure 4(a) Contributions of the four sources to each species from the positive matrix factorization model and (b) the light absorption of primary aerosols from each source at different wavelengths (Abspri(λ), λ= 370, 470, 520, 590, 660 and 880 nm) during the study.
The first source factor was characterized by large proportions of V, Ni and hopanes as well as moderate amounts of OC, EC, Na+, K+, Cu and Abspri(λ) as shown in Fig. 4a. V and Ni were associated with oil fuel combustion (Moreno et al., 2010) and their ratio (V ∕ Ni) can be used to further identify ship engine emissions, which has a typical range of 2.5–4.0 (Cesari et al., 2014). The estimated V ∕ Ni ratio was 3.4 in this source factor, consistent with the previously established range of ship engine emissions. Since hydrocarbons are the major components of ship engine oil, hopanes, OC and EC can be produced as byproducts of the combustion process. Therefore, this source factor was assigned to ship emissions. The second source factor was associated with large amounts of Cu, Zn and Br as well as moderate proportions of hopanes, EC, Ti and Abspri(λ). Previous studies confirmed that hopanes, Br and EC were typically present in vehicle exhaust particles (Huang et al., 1994; Sheesley et al., 2009). Additionally, Zn and Cu were associated with lubricant and metal brake wear (Lin et al., 2015). Therefore, this source factor was allocated to motor vehicle emissions. The third source factor was dominated by high proportions of K+, OC, EC and Abspri(λ), which was an obvious feature of biomass burning (Forello et al., 2019). Finally, the fourth source factor was characterized by large amounts of several crustal materials such as Ca2+, Ti, Fe and Mn and was identified as fugitive dust.
Notably, biomass burning occupied the largest proportion of light absorption (Absbiomass(λ)) at 32 %–44 % of Abspri(λ) as shown in Fig. 4a. Sanya is a coastal city with heavy maritime traffic (e.g., the cargo handling capacity was larger than 5.8 million metric tons in 2017 at Sanya port, http://tjj.sanya.gov.cn/tjjsite/2019nnj/tjnj.shtml, last access: March 2020, in Chinese); therefore absorption of ship emissions (Absship(λ)) also had a significant contribution to Abspri(λ) (30 %–39 %). The contribution of motor vehicle emissions (Absvehicle(λ)=17 %–24 % of Abspri(λ)) was much lower than that of biomass burning and ship emissions. Moreover, the absorption of fugitive dust (Absdust(λ)) occupied less than 10 % of Abspri(λ), consistent with previous reports where it was identified as a minor contributor in the atmosphere (Yang et al., 2009; Zhao et al., 2019). This small absorptive fraction may be attributed to the low proportion of light-absorbing iron oxides in the atmosphere. Furthermore, the Abspri(λ) values of different sources all decreased with increased wavelength (Fig. 4b) although their relative contributions displayed distinct trends (Fig. 4a). The fraction of Absship(λ) and Absvehicle(λ) increased with an increase in wavelength while a reverse trend was observed in the Absbiomass(λ) fraction. This discrepancy can be explained by the large amount of BrC present in biomass-burning emissions which can result in more light absorption at short wavelengths relative to the long ones.
3.3 Source-dependent optical properties of LAC aerosols
According to a power-law function (Fig. 5), the average LAC AAE (1.41, Table 1) was greater than unity during the study, indicating the presence of both BC and BrC in the atmosphere. In addition, the estimated AAE of motor vehicle emissions (AAEvehicle) was 0.96. This was close to the previously reported range of 0.9–1.1 obtained from ambient observations using the radiocarbon method or vehicle-exhaust-related source experiments (Sandradewi et al., 2008; Zotter et al., 2017; Chow et al., 2018). This narrow range of AAEvehicle obtained by various studies suggests that the spectral dependence of vehicle-exhaust-related LAC absorption was less affected by atmospheric processes. Furthermore, the AAE of ship emissions (AAEship=1.06) was similar to that of AAEvehicle. These low spectral dependences of light absorption indicate that BC was the dominant compound in LAC aerosols from ship and motor vehicle emissions. Compared to marine engine emissions, the AAEship obtained in this study was consistent with the values derived from marine gas oil and diesel fuel emissions (1.0 ± 0.1) but was lower than heavy fuel oil exhaust (1.7±0.2) (Corbin et al., 2018). This indicates that Sanya may be influenced more by ships using distillate rather than heavy fuel oil.
The AAE of biomass burning (AAEbiomass=1.75) was larger than that from ship and motor vehicle emissions. This implied the presence of BrC in LAC aerosols derived from biomass burning in addition to BC. The observation corroborated with previous studies which showed that BrC was mainly derived from biomass burning rather than fossil fuels in the atmosphere (Laskin et al., 2015). Additionally, chamber studies showed that the AAEs of fresh smoke from biomass burning varied largely (e.g., 1.64–3.25), depending on the type of biomass and their burning efficiencies (Tian et al., 2019). The AAEbiomass from this study was close to those values (1.7–1.9) from the atmosphere constrained by the radiocarbon method (Sandradewi et al., 2008; Zotter et al., 2017). Given that the approach used in this study could retrieve the source-specific AAEs in the atmosphere, it can also improve the performance of those optical source apportionment models based solely on optical data.
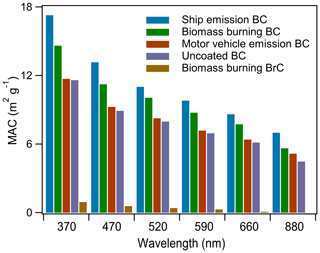
Figure 6The source-specific mass absorption cross section (MAC) of black carbon (BC) and brown carbon (BrC) at different wavelengths. The MACs of uncoated BC particles at each wavelength are extrapolated from 7.5 m2 g−1 at 550 nm (Bond and Bergstrom, 2006) by assuming an BC absorption Ångström exponent of 1.1.
Owing to the dominance of BC in ship and motor vehicle emissions, only MACBC(λ) was estimated for these two sources. The results of optical source apportionment revealed that the estimated MACBC(λ) values of motor vehicle emissions (MACBC,vehicle(λ)) were close to the values of uncoated BC particles at different wavelengths (Fig. 6). This indicated that vehicle-exhaust-related BC particles were mainly associated with local emissions and underwent minor atmospheric aging processes. In contrast, the MACBC(λ) of ship emissions (MACBC,ship(λ)) was 1.4–1.6 times larger than that of the uncoated ones (Fig. 6). This implied that ship-exhaust-related BC particles were prone to substantial aging during transit from the ocean. Freshly emitted BC particles from fossil fuels tend to mix externally with other substances and become internally mixed ones after aging (Xing et al., 2020). It was therefore unexpected for the obtained MACBC,ship(λ) to have a similar value as that of marine engine emissions reported by Corbin et al. (2018) (7.8 m2 g−1 at 780 nm, extrapolated to the same wavelengths in this study by assuming an AAEBC=1.1). Consequently, more work is needed to understand the large MACBC(λ) values from marine engine emissions.
Since LAC aerosols derived from biomass burning were comprised of both BC and BrC, the MACBC(λ) and MACBrC(λ) were retrieved based on the results of optical source apportionment. The findings revealed that the MACBC(λ) of biomass burning (MACBC,biomass(λ)) was larger than MACBC,vehicle(λ) as shown in Fig. 6. This was consistent with previous studies showing a stronger capacity to absorb light by BC from biomass burning compared to that from motor vehicle emissions (Qiu et al., 2014; Q. Wang et al., 2018b). Moreover, the MACBC,biomass(λ) was smaller than MACBC,ship(λ), suggesting a stronger ability to absorb light by BC particles from ship exhaust. A broader implication of this observation is that more focus should be put on BC particles related to ship emissions due to their impact on climate given the increase in shipping activities globally.
The MACBrC(λ) of biomass burning (MACBrC,biomass(λ)) was highly dependent on wavelength, with 0.9 m2 g−1 at λ= 370 nm but dropping close to zero (0.02 m2 g−1) at λ= 660 nm (Fig. 6). Additionally, the MACBrC,biomass(λ) was several times to 2 orders of magnitude lower than MACBC(λ) from different sources, suggesting that BC had a stronger ability to absorb light compared to BrC. Notably, the MACBrC(λ) obtained in this study was within the range reported by previous investigations, although with differences among studies (Wang et al., 2019b; Cho et al., 2019). The differences in MACBrC(λ) may partly be related to biomass types and their burning efficiencies as well as the aging processes of BrC in the atmosphere. In addition, the use of different BrC substitutes (e.g., OM, organic aerosol or water-soluble organic carbon) may have impacted the calculation of MACBrC(λ). Compared to previous laboratory studies, the MACBrC,biomass(λ) obtained here was smaller than that of fresh smoke from biomass burning (Zhong and Jang, 2014; Pandey et al., 2016). Given that photobleaching is an effective way of turning BrC into a transparent organic substance (Laskin et al., 2015), the smaller atmospheric MACBrC,biomass(λ) observed in this study may be attributed to the elimination of organic chromophores induced by the bright sunlight at Sanya.
The MAC links the mass of a LAC aerosol to its ability to absorb light and is an important parameter in climate models to evaluate global or regional radiative effects of LAC aerosols. An equal MAC from different sources is often assumed in climate models (Bond et al., 2013) because identification of source-specific MACs in the atmosphere is still a challenge. However, this assumption can lead to uncertainties due to distinct MACs from various sources (e.g., MACBC,ship(λ)> MACBC,biomass(λ)> MACBC,vehicle(λ) in this study). The chemical-composition-based optical source apportionment approach may provide a potential solution to this challenge. Nonetheless, more source-specific MACs in different areas and seasons are needed in future studies to gauge the accuracy of climate models. Moreover, this approach may minimize the uncertainties of BC source apportionment using the “Aethalometer model” (Sandradewi et al., 2008; Zotter et al., 2017) due to the assumption of equal AAE and MAC from different sources.
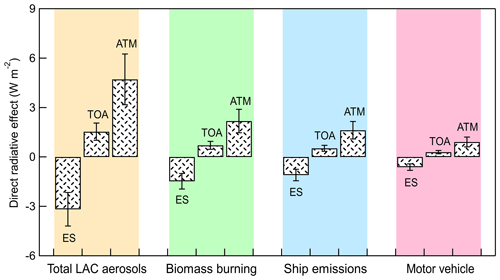
Figure 7Direct radiative effect (DRE) of light-absorbing carbonaceous (LAC) aerosols from biomass burning, ship emissions, and motor vehicle emissions. The error bar represents 1 standard deviation. ES, TOA, ATM represent the DRE at the Earth's surface, the top of the atmosphere, and in the atmosphere, respectively.
3.4 Impacts of LAC aerosols on radiative effect
Figure 7 shows the source-specific LAC DRE during the study. The LAC DRE varied from −5.5 to −1.6 W m−2 at the Earth's surface with an average cooling effect of −3.2 ± 1.0 W m−2. In contrast, the LAC aerosols produced a warm effect of +1.5 ± 0.5 W m−2 at the top of the atmosphere with a range of +0.8 to +2.8 W m−2, suggesting a net energy gain. The presence of LAC aerosols enhanced aerosol DRE at the top of the atmosphere by 62 % compared to the results of light scattering by aerosols only. Moreover, the difference between LAC DRE at the top of the atmosphere and the Earth's surface gave the atmospheric DRE (a net atmospheric absorption) of +4.7 ± 1.5 W m−2 and could generate a heating rate of 0.13 ± 0.04 K d−1.
With regard to LAC absorption sources, biomass burning was the largest contributor to LAC DRE at −1.5 ± 0.5 and +0.7 ± 0.2 W m−2 on the surface of the Earth and the top of the atmosphere, respectively. In addition, BrC from biomass burning had a lower contribution to LAC DRE compared to BC from the same source. However, the presence of BrC reinforced the LAC DRE of biomass burning by 21 % as opposed to BC only, suggesting a substantial radiative effect from BrC aerosol. Additionally, the LAC DRE values were −1.1 ± 0.4 and +0.5 ± 0.2 W m−2 for ship emissions and −0.6 ± 0.2 and +0.3 ± 0.1 W m−2 for motor vehicle emissions on the Earth's surface and the top of the atmosphere, respectively. The LAC DRE contributed by ship and motor vehicle emissions was mainly caused by BC aerosol. Although a larger BC atmospheric DRE was observed for biomass burning, ship emissions showed an equivalent capacity of radiative effect (0.5 (W m−2) (µg m) from per-unit BC mass concentration, generating atmospheric DRE. In contrast, motor vehicle emissions had a smaller value of 0.3 (W m−2) (µg m. Furthermore, the atmospheric heating rate of LAC aerosols was similar for biomass burning (0.06 ± 0.02 K d−1) and ship emissions (0.05 ± 0.01 K d−1) but larger than that produced by motor vehicle emissions (0.03 ± 0.01 K d−1). This further highlighted the importance of LAC aerosols from ship exhaust in atmospheric heating.
In this study, the optical properties and radiative effect of LAC aerosols in Sanya, a Chinese tropical marine monsoon climate zone, were explored. The study found that light absorption caused by primary emissions was the main contributor to LAC absorption while secondary processes played a minor role. Moreover, BC aerosol (> 77 %) contributed more to AbsLAC(λ) compared to BrC (< 17 %). Through a combination of chemical species and multi-wavelength absorption in a positive matrix factorization model, it was shown that biomass burning had the highest contribution to Abspri(λ) (32 %–44 %) followed by ship (30 %–39 %) and motor vehicle emissions (17 %–24 %). Fugitive dust had the lowest contribution (< 10 %). Moreover, source-specific AAE showed a similarity between ship and motor vehicle emissions (1.06 versus 0.96). The low spectral dependence of light absorption indicated that LAC aerosols were dominated by BC in ship and motor vehicle emissions. In contrast, a large AAE of 1.75 was found in biomass burning, indicating the presence of both BC and BrC. Additionally, source-specific MAC showed that BC particles from ship emissions had the strongest light-absorbing capacity, followed by biomass burning and motor vehicle emissions. Compared to BC MAC, the BrC MAC of biomass burning was smaller with a value of 0.9 m2 g−1 at λ= 370 nm but dropped to 0.02 m2 g−1 at λ= 660 nm. The radiative transfer model also showed that the atmospheric DRE caused by LAC aerosols was +4.7 ± 1.5 W m−2 during the study and corresponded to a heating rate of 0.13 ± 0.04 K d−1. The presence of BrC reinforced the LAC DRE of biomass burning by 21 % compared to BC only. Finally, ship emissions showed an equivalent capacity to produce radiative effect (0.5 (W m−2) (µg m) from per-unit BC mass concentration, generating atmospheric DRE. In contrast, motor vehicle emissions had a smaller value of 0.3 (W m−2) (µg m.
All data described in this study are available upon request from the corresponding authors.
The supplement related to this article is available online at: https://doi.org/10.5194/acp-20-15537-2020-supplement.
QW, JC and YH designed the campaign. PW and YZ provided the observation site and assisted with field sampling and measurements. WD and TZ conducted the chemical analyses. HL ran the PMF5.0 and SBDART model. JT performed the cluster analysis of air-mass trajectories. WZ provided the ArcGIS map. QW conducted the data analysis and wrote the article with input from all co-authors.
The authors declare that they have no conflict of interest.
The authors are grateful to the editor and two anonymous referees for their helpful comments.
This research has been supported by the Strategic Priority Research Program of Chinese Academy of Sciences (grant no. XDB40000000), the Youth Innovation Promotion Association of the Chinese Academy of Sciences (grant no. 2019402), the Hainan Natural Science Foundation High-level Talent Project (grant no. 2019RC243) and the Science and Technology Cooperation Project of Sanya (grant nos. 2018YD14 and 2012YD38).
This paper was edited by James Allan and reviewed by two anonymous referees.
Andreae, M. O. and Gelencsér, A.: Black carbon or brown carbon? The nature of light-absorbing carbonaceous aerosols, Atmos. Chem. Phys., 6, 3131–3148, https://doi.org/10.5194/acp-6-3131-2006, 2006.
Bond, T. C., Doherty, S. J., Fahey, D. W., Forster, P. M., Berntsen, T., DeAngelo, B. J., Flanner, M. G., Ghan, S., Kärcher, B., Koch, D., Kinne, S., Kondo, Y., Quinn, P. K., Sarofim, M. C., Schultz, M. G., Schulz, M., Venkataraman, C., Zhang, H., Zhang, S., Bellouin, N., Guttikunda, S. K., Hopke, P. K., Jacobson, M. Z., Kaiser, J. W., Klimont, Z., Lohmann, U., Schwarz, J. P., Shindell, D., Storelvmo, T., Warren, S. G., and Zender, C. S.: Bounding the role of black carbon in the climate system: A scientific assessment, J. Geophys. Res.-Atmos., 118, 5380–5552, https://doi.org/10.1002/jgrd.50171, 2013.
Brown, S. G., Eberly, S., Paatero, P., and Norris, G. A.: Methods for estimating uncertainty in PMF solutions: Examples with ambient air and water quality data and guidance on reporting PMF results, Sci. Total Environ., 518–519, 626–635, https://doi.org/10.1016/j.scitotenv.2015.01.022, 2015.
Cesari, D., Genga, A., Ielpo, P., Siciliano, M., Mascolo, G., Grasso, F. M., and Contini, D.: Source apportionment of PM2.5 in the harbour–industrial area of Brindisi (Italy): Identification and estimation of the contribution of in-port ship emissions, Sci. Total Environ., 497–498, 392–400, https://doi.org/10.1016/j.scitotenv.2014.08.007, 2014.
Cho, C., Kim, S.-W., Lee, M., Lim, S., Fang, W., Gustafsson, Ö., Andersson, A., Park, R. J., and Sheridan, P. J.: Observation-based estimates of the mass absorption cross-section of black and brown carbon and their contribution to aerosol light absorption in East Asia, Atmos. Environ., 212, 65–74, https://doi.org/10.1016/j.atmosenv.2019.05.024, 2019.
Chow, J. C., Watson, J. G., Chen, L. W. A., Chang, M. C. O., Robinson, N. F., Trimble, D., and Kohl, S.: The IMPROVE_A temperature protocol for thermal/optical carbon analysis: Maintaining consistency with a long-term database, J. Air Waste Manage. Assoc., 57, 1014–1023, https://doi.org/10.3155/1047-3289.57.9.1014, 2007.
Chow, J. C., Watson, J. G., Green, M. C., Wang, X., Chen, L. W. A., Trimble, D. L., Cropper, P. M., Kohl, S. D., and Gronstal, S. B.: Separation of brown carbon from black carbon for IMPROVE and Chemical Speciation Network PM2.5 samples, J. Air Waste Manage. Assoc., 68, 494–510, https://doi.org/10.1080/10962247.2018.1426653, 2018.
Corbin, J. C., Pieber, S. M., Czech, H., Zanatta, M., Jakobi, G., Massabò, D., Orasche, J., El Haddad, I., Mensah, A. A., Stengel, B., Drinovec, L., Mocnik, G., Zimmermann, R., Prévôt, A. S. H., and Gysel, M.: Brown and black carbon emitted by a marine engine operated on heavy fuel oil and distillate fuels: Optical properties, size distributions, and emission factors, J. Geophys. Res.-Atmos., 123, 6175–6195, https://doi.org/10.1029/2017JD027818, 2018.
Drinovec, L., Močnik, G., Zotter, P., Prévôt, A. S. H., Ruckstuhl, C., Coz, E., Rupakheti, M., Sciare, J., Müller, T., Wiedensohler, A., and Hansen, A. D. A.: The “dual-spot” Aethalometer: an improved measurement of aerosol black carbon with real-time loading compensation, Atmos. Meas. Tech., 8, 1965–1979, https://doi.org/10.5194/amt-8-1965-2015, 2015.
Forello, A. C., Bernardoni, V., Calzolai, G., Lucarelli, F., Massabò, D., Nava, S., Pileci, R. E., Prati, P., Valentini, S., Valli, G., and Vecchi, R.: Exploiting multi-wavelength aerosol absorption coefficients in a multi-time resolution source apportionment study to retrieve source-dependent absorption parameters, Atmos. Chem. Phys., 19, 11235–11252, https://doi.org/10.5194/acp-19-11235-2019, 2019.
Healy, R. M., Sofowote, U., Su, Y., Debosz, J., Noble, M., Jeong, C. H., Wang, J. M., Hilker, N., Evans, G. J., Doerksen, G., Jones, K., and Munoz, A.: Ambient measurements and source apportionment of fossil fuel and biomass burning black carbon in Ontario, Atmos. Environ., 161, 34–47, https://doi.org/10.1016/j.atmosenv.2017.04.034, 2017.
Hess, M., Koepke, P., and Schult, I.: Optical Properties of Aerosols and Clouds: The Software Package OPAC, B. Am. Meteorol. Soc., 79, 831–844, https://doi.org/10.1175/1520-0477(1998)079<0831:OPOAAC>2.0.CO;2, 1998.
Huang, X., Olmez, I., Aras, N. K., and Gordon, G. E.: Emissions of trace elements from motor vehicles: Potential marker elements and source composition profile, Atmos. Environ., 28, 1385–1391, https://doi.org/10.1016/1352-2310(94)90201-1, 1994.
IPCC: Climate Change 2013: The Physical Science Basis, Cambridge University Press, Cambridge, UK, New York, NY, USA, 2013.
Kim, J.-H., Kim, S.-W., Ogren, J. A., Sheridan, P. J., Yoon, S.-C., Sharma, S., and Lin, N.-H.: Multiple scattering correction factor estimation for aethalometer aerosol absorption coefficient measurement, Aerosol Sci. Technol., 53, 160–171, https://doi.org/10.1080/02786826.2018.1555368, 2019.
Küpper, M., Quass, U., John, A. C., Kaminski, H., Leinert, S., Breuer, L., Gladtke, D., Weber, S., and Kuhlbusch, T. A. J.: Contributions of carbonaceous particles from fossil emissions and biomass burning to PM10 in the Ruhr area, Germany, Atmos. Environ., 189, 174–186, https://doi.org/10.1016/j.atmosenv.2018.06.039, 2018.
Lack, D. A. and Langridge, J. M.: On the attribution of black and brown carbon light absorption using the Ångström exponent, Atmos. Chem. Phys., 13, 10535–10543, https://doi.org/10.5194/acp-13-10535-2013, 2013.
Laskin, A., Laskin, J., and Nizkorodov, S. A.: Chemistry of atmospheric brown carbon, Chem. Rev., 115, 4335–4382, https://doi.org/10.1021/cr5006167, 2015.
Liakakou, E., Kaskaoutis, D. G., Grivas, G., Stavroulas, I., Tsagkaraki, M., Paraskevopoulou, D., Bougiatioti, A., Dumka, U. C., Gerasopoulos, E., and Mihalopoulos, N.: Long-term brown carbon spectral characteristics in a Mediterranean city (Athens), Sci. Total Environ., 708, 135019, https://doi.org/10.1016/j.scitotenv.2019.135019, 2020.
Lin, Y.-C., Tsai, C.-J., Wu, Y.-C., Zhang, R., Chi, K.-H., Huang, Y.-T., Lin, S.-H., and Hsu, S.-C.: Characteristics of trace metals in traffic-derived particles in Hsuehshan Tunnel, Taiwan: size distribution, potential source, and fingerprinting metal ratio, Atmos. Chem. Phys., 15, 4117–4130, https://doi.org/10.5194/acp-15-4117-2015, 2015.
Liou, K. N.: An introduction to atmospheric radiation, 2nd edn., Academic press, Elsevier Science, New York, 583 pp., 2002.
Massabò, D., Caponi, L., Bernardoni, V., Bove, M. C., Brotto, P., Calzolai, G., Cassola, F., Chiari, M., Fedi, M. E., Fermo, P., Giannoni, M., Lucarelli, F., Nava, S., Piazzalunga, A., Valli, G., Vecchi, R., and Prati, P.: Multi-wavelength optical determination of black and brown carbon in atmospheric aerosols, Atmos. Environ., 108, 1–12, https://doi.org/10.1016/j.atmosenv.2015.02.058, 2015.
Moreno, T., Querol, X., Alastuey, A., de la Rosa, J., Sánchez de la Campa, A. M., Minguillón, M., Pandolfi, M., González-Castanedo, Y., Monfort, E., and Gibbons, W.: Variations in vanadium, nickel and lanthanoid element concentrations in urban air, Sci. Total Environ., 408, 4569–4579, https://doi.org/10.1016/j.scitotenv.2010.06.016, 2010.
Norris, G., Duvall, R., Brown, S., and Bai, S.: EPA Positive Matrix Factorization (PMF) 5.0 Fundamentals and User Guide, available at: https://www.epa.gov/sites/production/files/2015-02/documents/pmf_5.0_user_guide.pdf (last access: September 2019), 2014.
Obregón, M. A., Serrano, A., Costa, M. J., and Silva, A. M.: Validation of libRadtran and SBDART models under different aerosol conditions, IOP Conf. Ser. Earth Environ. Sci., 28, 12010, https://doi.org/10.1088/1755-1315/28/1/012010, 2015.
Paatero, P. and Tapper, U.: Positive matrix factorization: A non-negative factor model with optimal utilization of error estimates of data values, Environmetrics, 5, 111–126, https://doi.org/10.1002/env.3170050203, 1994.
Paatero, P., Eberly, S., Brown, S. G., and Norris, G. A.: Methods for estimating uncertainty in factor analytic solutions, Atmos. Meas. Tech., 7, 781–797, https://doi.org/10.5194/amt-7-781-2014, 2014.
Pandey, A., Pervez, S., and Chakrabarty, R. K.: Filter-based measurements of UV–vis mass absorption cross sections of organic carbon aerosol from residential biomass combustion: Preliminary findings and sources of uncertainty, J. Quant. Spectrosc. Ra., 182, 296–304, https://doi.org/10.1016/j.jqsrt.2016.06.023, 2016.
Putaud, J. P., Van Dingenen, R., Alastuey, A., Bauer, H., Birmili, W., Cyrys, J., Flentje, H., Fuzzi, S., Gehrig, R., Hansson, H. C., Harrison, R. M., Herrmann, H., Hitzenberger, R., Hüglin, C., Jones, A. M., Kasper-Giebl, A., Kiss, G., Kousa, A., Kuhlbusch, T. A. J., Löschau, G., Maenhaut, W., Molnar, A., Moreno, T., Pekkanen, J., Perrino, C., Pitz, M., Puxbaum, H., Querol, X., Rodriguez, S., Salma, I., Schwarz, J., Smolik, J., Schneider, J., Spindler, G., ten Brink, H., Tursic, J., Viana, M., Wiedensohler, A., and Raes, F.: A European aerosol phenomenology–3: Physical and chemical characteristics of particulate matter from 60 rural, urban, and kerbside sites across Europe, Atmos. Environ., 44, 1308–1320, https://doi.org/10.1016/j.atmosenv.2009.12.011, 2010.
Qin, Y. M., Tan, H. B., Li, Y. J., Li, Z. J., Schurman, M. I., Liu, L., Wu, C., and Chan, C. K.: Chemical characteristics of brown carbon in atmospheric particles at a suburban site near Guangzhou, China, Atmos. Chem. Phys., 18, 16409–16418, https://doi.org/10.5194/acp-18-16409-2018, 2018.
Qiu, C., Khalizov, A. F., Hogan, B., Petersen, E. L., and Zhang, R.: High sensitivity of diesel soot morphological and optical properties to combustion temperature in a shock tube, Environ. Sci. Technol., 48, 6444–6452, https://doi.org/10.1021/es405589d, 2014.
Rai, P., Furger, M., Slowik, J. G., Canonaco, F., Fröhlich, R., Hüglin, C., Minguillón, M. C., Petterson, K., Baltensperger, U., and Prévôt, A. S. H.: Source apportionment of highly time-resolved elements during a firework episode from a rural freeway site in Switzerland, Atmos. Chem. Phys., 20, 1657–1674, https://doi.org/10.5194/acp-20-1657-2020, 2020.
Ricchiazzi, P., Yang, S., Gautier, C., and Sowle, D.: SBDART: A Research and Teaching Software Tool for Plane-Parallel Radiative Transfer in the Earth's Atmosphere, B. Am. Meteorol. Soc., 79, 2101–2114, https://doi.org/10.1175/1520-0477(1998)079<2101:SARATS>2.0.CO;2, 1998.
Sandradewi, J., Prévôt, A. S. H., Szidat, S., Perron, N., Alfarra, M. R., Lanz, V. A., Weingartner, E., and Baltensperger, U.: Using aerosol light absorption measurements for the quantitative determination of wood burning and traffic emission contributions to particulate matter, Environ. Sci. Technol., 42, 3316–3323, https://doi.org/10.1021/es702253m, 2008.
Satheesh, S. K. and Srinivasan, J.: A method to estimate aerosol radiative forcing from spectral optical depths, J. Atmos. Sci., 63, 1082–1092, https://doi.org/10.1175/JAS3663.1, 2006.
Sheesley, R. J., Schauer, J. J., Garshick, E., Laden, F., Smith, T. J., Blicharz, A. P., and Deminter, J. T.: Tracking personal exposure to particulate diesel exhaust in a diesel freight terminal using organic tracer analysis, J. Exposure Sci. Environ. Epidemiol., 19, 172–186, https://doi.org/10.1038/jes.2008.11, 2009.
Tao, J., Zhang, L., Cao, J., and Zhang, R.: A review of current knowledge concerning PM2.5 chemical composition, aerosol optical properties and their relationships across China, Atmos. Chem. Phys., 17, 9485–9518, https://doi.org/10.5194/acp-17-9485-2017, 2017.
Tasoglou, A., Saliba, G., Subramanian, R., and Pandis, S. N.: Absorption of chemically aged biomass burning carbonaceous aerosol, J. Aerosol Sci., 113, 141–152, https://doi.org/10.1016/j.jaerosci.2017.07.011, 2017.
Tian, J., Wang, Q., Ni, H., Wang, M., Zhou, Y., Han, Y., Shen, Z., Pongpiachan, S., Zhang, N., Zhao, Z., Zhang, Q., Zhang, Y., Long, X., and Cao, J.: Emission characteristics of primary brown carbon absorption from biomass and coal burning: Development of an optical emission inventory for China, J. Geophys. Res.-Atmos., 124, 1879–1893, https://doi.org/10.1029/2018JD029352, 2019.
Tian, J., Wang, Q., Han, Y., Ye, J., Wang, P., Pongpiachan, S., Ni, H., Zhou, Y., Wang, M., Zhao, Y., and Cao, J.: Contributions of aerosol composition and sources to particulate optical properties in a southern coastal city of China, Atmos. Res., 235, 104744, https://doi.org/10.1016/j.atmosres.2019.104744, 2020.
Turpin, B. J. and Lim, H.-J.: Species contributions to PM2.5 mass concentrations: Revisiting common assumptions for estimating organic mass, Aerosol Sci. Technol., 35, 602–610, https://doi.org/10.1080/02786820119445, 2001.
Wang, J., Ho, S. S. H., Ma, S., Cao, J., Dai, W., Liu, S., Shen, Z., Huang, R., Wang, G., and Han, Y.: Characterization of PM2.5 in Guangzhou, China: uses of organic markers for supporting source apportionment, Sci. Total Environ., 550, 961–971, https://doi.org/10.1016/j.scitotenv.2016.01.138, 2016.
Wang, J., Nie, W., Cheng, Y., Shen, Y., Chi, X., Wang, J., Huang, X., Xie, Y., Sun, P., Xu, Z., Qi, X., Su, H., and Ding, A.: Light absorption of brown carbon in eastern China based on 3-year multi-wavelength aerosol optical property observations and an improved absorption Ångström exponent segregation method, Atmos. Chem. Phys., 18, 9061–9074, https://doi.org/10.5194/acp-18-9061-2018, 2018.
Wang, L., Li, Z., Tian, Q., Ma, Y., Zhang, F., Zhang, Y., Li, D., Li, K., and Li, L.: Estimate of aerosol absorbing components of black carbon, brown carbon, and dust from ground-based remote sensing data of sun-sky radiometers, J. Geophys. Res.-Atmos., 118, 6534–6543, https://doi.org/10.1002/jgrd.50356, 2013.
Wang, Q., Cao, J., Han, Y., Tian, J., Zhu, C., Zhang, Y., Zhang, N., Shen, Z., Ni, H., Zhao, S., and Wu, J.: Sources and physicochemical characteristics of black carbon aerosol from the southeastern Tibetan Plateau: internal mixing enhances light absorption, Atmos. Chem. Phys., 18, 4639–4656, https://doi.org/10.5194/acp-18-4639-2018, 2018a.
Wang, Q., Cao, J., Han, Y., Tian, J., Zhang, Y., Pongpiachan, S., Zhang, Y., Li, L., Niu, X., Shen, Z., Zhao, Z., Tipmanee, D., Bunsomboonsakul, S., Chen, Y., and Sun, J.: Enhanced light absorption due to the mixing state of black carbon in fresh biomass burning emissions, Atmos. Environ., 180, 184–191, https://doi.org/10.1016/j.atmosenv.2018.02.049, 2018b.
Wang, Q., Han, Y., Ye, J., Liu, S., Pongpiachan, S., Zhang, N., Han, Y., Tian, J., Wu, C., Long, X., Zhang, Q., Zhang, W., Zhao, Z., and Cao, J.: High contribution of secondary brown carbon to aerosol light absorption in the southeastern margin of Tibetan Plateau, Geophys. Res. Lett., 46, 4962–4970, https://doi.org/10.1029/2019GL082731, 2019a.
Wang, Q., Ye, J., Wang, Y., Zhang, T., Ran, W., Wu, Y., Tian, J., Li, L., Zhou, Y., Hang Ho, S. S., Dang, B., Zhang, Q., Zhang, R., Chen, Y., Zhu, C., and Cao, J.: Wintertime optical properties of primary and secondary brown carbon at a regional site in the North China Plain, Environ. Sci. Technol., 53, 12389–12397, 10.1021/acs.est.9b03406, 2019b.
Xie, M., Hays, M. D., and Holder, A. L.: Light-absorbing organic carbon from prescribed and laboratory biomass burning and gasoline vehicle emissions, Sci. Rep., 7, 7318, https://doi.org/10.1038/s41598-017-06981-8, 2017.
Xing, J., Shao, L., Zhang, W., Peng, J., Wang, W., Shuai, S., Hu, M., and Zhang, D.: Morphology and size of the particles emitted from a gasoline-direct-injection-engine vehicle and their ageing in an environmental chamber, Atmos. Chem. Phys., 20, 2781–2794, https://doi.org/10.5194/acp-20-2781-2020, 2020.
Xu, H. M., Cao, J. J., Ho, K. F., Ding, H., Han, Y. M., Wang, G. H., Chow, J. C., Watson, J. G., Khol, S. D., Qiang, J., and Li, W. T.: Lead concentrations in fine particulate matter after the phasing out of leaded gasoline in Xi'an, China, Atmos. Environ., 46, 217–224, https://doi.org/10.1016/j.atmosenv.2011.09.078, 2012.
Yang, M., Howell, S. G., Zhuang, J., and Huebert, B. J.: Attribution of aerosol light absorption to black carbon, brown carbon, and dust in China – interpretations of atmospheric measurements during EAST-AIRE, Atmos. Chem. Phys., 9, 2035–2050, https://doi.org/10.5194/acp-9-2035-2009, 2009.
Zanatta, M., Gysel, M., Bukowiecki, N., Müller, T., Weingartner, E., Areskoug, H., Fiebig, M., Yttri, K. E., Mihalopoulos, N., Kouvarakis, G., Beddows, D., Harrison, R. M., Cavalli, F., Putaud, J. P., Spindler, G., Wiedensohler, A., Alastuey, A., Pandolfi, M., Sellegri, K., Swietlicki, E., Jaffrezo, J. L., Baltensperger, U., and Laj, P.: A European aerosol phenomenology-5: Climatology of black carbon optical properties at 9 regional background sites across Europe, Atmos. Environ., 145, 346–364, https://doi.org/10.1016/j.atmosenv.2016.09.035, 2016.
Zhang, T., Cao, J. J., Tie, X. X., Shen, Z. X., Liu, S. X., Ding, H., Han, Y. M., Wang, G. H., Ho, K. F., Qiang, J., and Li, W. T.: Water-soluble ions in atmospheric aerosols measured in Xi'an, China: Seasonal variations and sources, Atmos. Res., 102, 110–119, https://doi.org/10.1016/j.atmosres.2011.06.014, 2011.
Zhao, Z., Cao, J., Chow, J. C., Watson, J. G., Chen, A. L. W., Wang, X., Wang, Q., Tian, J., Shen, Z., Zhu, C., Liu, S., Tao, J., Ye, Z., Zhang, T., Zhou, J., and Tian, R.: Multi-wavelength light absorption of black and brown carbon at a high-altitude site on the Southeastern margin of the Tibetan Plateau, China, Atmos. Environ., 212, 54–64, https://doi.org/10.1016/j.atmosenv.2019.05.035, 2019.
Zheng, H., Kong, S., Wu, F., Cheng, Y., Niu, Z., Zheng, S., Yang, G., Yao, L., Yan, Q., Wu, J., Zheng, M., Chen, N., Xu, K., Yan, Y., Liu, D., Zhao, D., Zhao, T., Bai, Y., Li, S., and Qi, S.: Intra-regional transport of black carbon between the south edge of the North China Plain and central China during winter haze episodes, Atmos. Chem. Phys., 19, 4499–4516, https://doi.org/10.5194/acp-19-4499-2019, 2019.
Zhong, M. and Jang, M.: Dynamic light absorption of biomass-burning organic carbon photochemically aged under natural sunlight, Atmos. Chem. Phys., 14, 1517–1525, https://doi.org/10.5194/acp-14-1517-2014, 2014.
Zhu, C.-S., Cao, J.-J., Hu, T.-F., Shen, Z.-X., Tie, X.-X., Huang, H., Wang, Q.-Y., Huang, R.-J., Zhao, Z.-Z., Močnik, G., and Hansen, A. D. A.: Spectral dependence of aerosol light absorption at an urban and a remote site over the Tibetan Plateau, Sci. Total Environ., 590–591, 14–21, https://doi.org/10.1016/j.scitotenv.2017.03.057, 2017.
Zotter, P., Herich, H., Gysel, M., El-Haddad, I., Zhang, Y., Močnik, G., Hüglin, C., Baltensperger, U., Szidat, S., and Prévôt, A. S. H.: Evaluation of the absorption Ångström exponents for traffic and wood burning in the Aethalometer-based source apportionment using radiocarbon measurements of ambient aerosol, Atmos. Chem. Phys., 17, 4229–4249, https://doi.org/10.5194/acp-17-4229-2017, 2017.