the Creative Commons Attribution 4.0 License.
the Creative Commons Attribution 4.0 License.
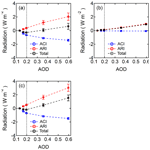
Impact of biomass burning aerosols on radiation, clouds, and precipitation over the Amazon: relative importance of aerosol–cloud and aerosol–radiation interactions
Lixia Liu
Siwen Wang
Chao Wei
Mira L. Pöhlker
Christopher Pöhlker
Paulo Artaxo
Manish Shrivastava
Meinrat O. Andreae
Ulrich Pöschl
Biomass burning (BB) aerosols can influence regional and global climate through interactions with radiation, clouds, and precipitation. Here, we investigate the impact of BB aerosols on the energy balance and hydrological cycle over the Amazon Basin during the dry season. We performed simulations with a fully coupled meteorology–chemistry model, the Weather Research and Forecasting model coupled with Chemistry (WRF-Chem), for a range of different BB emission scenarios to explore and characterize nonlinear effects and individual contributions from aerosol–radiation interactions (ARIs) and aerosol–cloud interactions (ACIs). The ARIs of BB aerosols tend to suppress low-level liquid clouds by local warming and increased evaporation and to facilitate the formation of high-level ice clouds by enhancing updrafts and condensation at high altitudes. In contrast, the ACIs of BB aerosol particles tend to enhance the formation and lifetime of low-level liquid clouds by providing more cloud condensation nuclei (CCN) and to suppress the formation of high-level ice clouds by reducing updrafts and condensable water vapor at high altitudes (>8 km).
For scenarios representing the lower and upper limits of BB emission estimates for recent years (2002–2016), we obtained total regional BB aerosol radiative forcings of −0.2 and 1.5 W m−2, respectively, showing that the influence of BB aerosols on the regional energy balance can range from modest cooling to strong warming. We find that ACIs dominate at low BB emission rates and low aerosol optical depth (AOD), leading to an increased cloud liquid water path (LWP) and negative radiative forcing, whereas ARIs dominate at high BB emission rates and high AOD, leading to a reduction of LWP and positive radiative forcing. In all scenarios, BB aerosols led to a decrease in the frequency of occurrence and rate of precipitation, caused primarily by ACI effects at low aerosol loading and by ARI effects at high aerosol loading. The dependence of precipitation reduction on BB aerosol loading is greater in a strong convective regime than under weakly convective conditions.
Overall, our results show that ACIs tend to saturate at high aerosol loading, whereas the strength of ARIs continues to increase and plays a more important role in highly polluted episodes and regions. This should hold not only for BB aerosols over the Amazon, but also for other light-absorbing aerosols such as fossil fuel combustion aerosols in industrialized and densely populated areas. The importance of ARIs at high aerosol loading highlights the need for accurately characterizing aerosol optical properties in the investigation of aerosol effects on clouds, precipitation, and climate.
- Article
(1767 KB) - Full-text XML
-
Supplement
(1238 KB) - BibTeX
- EndNote
Biomass burning as a main source of fine particles can influence weather and climate through complex feedbacks with radiation and clouds on regional and global scales (Ramanathan et al., 2001; Kaufman and Koren, 2006; Rosenfeld et al., 2008; Shrivastava et al., 2017; Ditas et al., 2018). Aerosols emitted from biomass burning contain black carbon (BC) and brown carbon, which enable them to scatter and absorb solar radiation directly, the so-called “direct radiative effect” (Charlson et al., 1992; Ackerman et al., 2000). Absorption and scattering of radiation can lead to spatial perturbation and redistribution of energy, therefore trigger subsequent changes in surface energy budget, ground–atmosphere flux exchange, atmospheric thermodynamic stability, and cloud evolution (Li, 1998; Feingold et al., 2005; Cheng et al., 2008a, b; Ding et al., 2013; Huang et al., 2016; Johnson et al., 2004), the so called “semi-direct effect” (Hansen et al., 1997; Ackerman et al., 2000). These processes, induced by the aerosol radiative effects, are referred to as aerosol–radiation interactions (ARIs; IPCC, 2013). By acting as cloud condensation nuclei (CCN) and ice nuclei (IN; Crutzen and Andreae, 1990; Roberts et al., 2001; Spracklen et al., 2011), BB aerosols influence the number concentration and size distribution of cloud droplets (Rosenfeld, 2000; Reutter et al., 2009) and thereby change the cloud albedo, i.e., the “first indirect radiative effect” (Albrecht, 1989; Kaufman and Fraser, 1997), and cloud lifetime, i.e., the “second indirect radiative effect” (Twomey, 1977; Jiang and Feingold, 2006). The latent heat release that accompanies these internal microphysical processes may modify atmospheric stability and affect convection strength and even subsequent cloud development (Rosenfeld et al., 2008). Such adjustments driven by aerosol microphysical effects are classified as aerosol–cloud interactions (ACIs; IPCC, 2013). Each class of interactions, and their interplay can affect the weather and climate system, leading to enhanced or buffered effects (Tao et al., 2007; Koren et al., 2008; Stevens and Feingold, 2009; Wang et al., 2013).
Mainly driven by deforestation and agricultural practices (Echalar et al., 1998; Reddington et al., 2015), biomass burning events prevail in the Amazon Basin (Setzer and Pereira, 1991) during the dry season, typically between July and October (Gan et al., 2004), injecting large amounts of aerosols into the atmosphere. Long-range transport of BB aerosols from southern Africa further increases aerosol loadings during this period (Holanda et al., 2020). Particle numbers during the peak of the burning season in the Amazon may increase 1 order of magnitude compared to the concentration levels during seasons without biomass fires (Martins et al., 1998; Andreae et al., 2002; Roberts et al., 2003; Martin et al., 2010). As most of the Amazon region is located in the equatorial and subequatorial area with the Intertropical Convergence Zone passing across it, the radiation budget and convection system there play important roles in the global energy balance, carbon storage, and transport of water vapor (Sengupta et al., 1990; Bony et al., 2006; Su et al., 2011) and aerosols (Freitas et al., 2005). During the dry season, precipitation amounts are relatively low, rendering the rainforest ecosystem more vulnerable to rainfall changes. Therefore, perturbations imposed by BB aerosols during the dry season are important for climate and ecology in Amazonia and even globally (Andreae et al., 2004).
Extensive investigations regarding the influence of BB aerosols on radiation and convection in this region by observation (Williams et al., 2002; Andreae et al., 2004; Lin et al., 2006; Gonçalves et al., 2015; Braga et al., 2017; Cecchini et al., 2017) and modeling studies (Feingold et al., 2005; Liu, 2005; Zhang et al., 2008; Wu et al., 2011; Ten Hoeve et al., 2012; Kolusu et al., 2015) have been conducted. BB aerosols were reported to cause a negative direct radiative forcing ranging from several to tens of watts per square meter (W m−2) at the top of atmosphere (TOA) over the Amazon area (Procopio et al., 2004; Zhang et al., 2008; Sena et al., 2013; Kolusu et al., 2015). Yet, their total radiative forcing varies in sign and magnitude between different modeling estimates (Ten Hoeve et al., 2012; Kolusu et al., 2015; Archer-Nicholls et al., 2016) because of uncertainties associated with the prescription of aerosol optical properties, cloud sensitivity to BB aerosols, model resolution (Archer-Nicholls et al., 2016), etc. The BB aerosols over the Amazon were observed to efficiently increase cloud droplet number and decrease cloud droplet radius (Andreae et al., 2004; Cecchini et al., 2017). However, satellite remote sensing measurements showed both suppression and enhancement of cloud fraction with the presence of BB aerosols in the Amazon (Kaufman and Fraser, 1997; Koren et al., 2004; Kaufman and Koren, 2006; Koren et al., 2008) and suggested a dependence of cloud response on aerosol concentrations (Koren et al., 2008; Jiang et al., 2018). Simulations by both cloud-resolving models and regional atmosphere–aerosol coupled models found enhanced cloud water burdens due to the microphysical effects of BB aerosols (Wu et al., 2011; Reutter et al., 2014; Chang et al., 2015). Their radiative effect was shown by large-eddy simulation to efficiently diminish liquid cloud amount by evaporating cloud droplets and suppressing vapor availability from land–atmosphere flux exchange (Feingold et al., 2005). Precipitation from convective clouds was also reported to be either inhibited or invigorated based on observations from in situ, aircraft, and satellite remote sensing measurements (Andreae et al., 2004; Lin et al., 2006; Gonçalves et al., 2015). Cloud-resolving modeling found nonlinear relationships between aerosol loading and precipitation through the microphysical effects of BB aerosols (Carslaw et al., 2013; Chang et al., 2015). Regional modeling studies showed that their radiative effect could cause an overall reduction in precipitation but may increase nighttime precipitation (Wu et al., 2011) and intensify the extreme high precipitation rates (Kolusu et al., 2015).
Interannual variability is a prominent characteristic of the biomass burning intensity in the Amazon (Kaufman and Fraser, 1997; Bevan et al., 2009; Pöhlker et al., 2019). However, most previous studies assessed the climate response to the perturbation from BB aerosols based on the emission scenario of 1 specific year (Zhang et al., 2008, 2009; Wu et al., 2011; Ten Hoeve et al., 2012; Kolusu et al., 2015; Archer-Nicholls et al., 2016). Given the possible nonlinear relationship between convection and aerosol concentration and the sensitivity of aerosol radiative forcing to aerosol loading, the necessity of a thorough assessment of radiation, clouds, and precipitation response to BB aerosols over an extensive range of emissions is underscored. Although Thornhill et al. (2018) estimated the difference in cloud response between high- and low-emission-intensity scenarios, this difference may not be adequate to serve as constraint for estimating BB aerosols' impact on background Amazon climate, since the perturbations due to BB aerosols may be nonlinear and have been proven to be strongly dependent on the reference emission setting (Wang, 2005; Martins et al., 2009). In this study, we performed WRF-Chem simulations over the Amazon Basin in September 2014 with a “clean” condition, defined by the absence of influence from biomass burning, and a set of emission scenarios resembling the realistic interannual emission variability in the dry season, to investigate the effects of BB aerosols on the Amazon radiation budget, clouds, and precipitation quantitatively and mechanistically. Comparison of the precipitation in central Amazonia in the year 2014 with that averaged over 18 years (1998–2016) indicates that the atmospheric conditions in this region in 2014 are climatically representative (Pöhlker et al., 2016). Therefore, the present study based on September 2014 may serve to represent the typical sensitivity behavior of the dry season climate to BB aerosol concentration variations. Climatically significant estimates of BB aerosols' radiative forcing, which may require statistics of over 30 years (Fiedler et al., 2017), are out of the scope of this study. As case study simulations imply that the initial convection response may influence secondary convection (Khain et al., 2005), monthly averaged effects of BB aerosols were assessed here to demonstrate an overall characteristic for the whole month. Individual processes of ARI and ACI were disentangled in our simulations, based on which the relative significance of the two pathways and their sensitivity to emission intensity were quantified. In this paper, the model description and experiment design are documented in Sect. 2. The overall impacts of BB aerosol emissions on radiation, meteorological conditions, clouds, and precipitation are shown in Sect. 3. Conclusions are in Sect. 4.
2.1 Model description
WRF-Chem is an online-coupled meteorology–chemistry model that integrates meteorology and chemistry with aerosol–radiation–cloud feedbacks (Grell et al., 2005). WRF-Chem version 3.9.1 was used in this study to investigate the impact of BB aerosols on the energy budget and hydrological cycle over the Amazon Basin.
The Carbon-Bond Mechanism version Z (CBMZ) gas-phase chemistry mechanism (Zaveri and Peters, 1999) and the Model for Simulating Aerosol Interactions and Chemistry (MOSAIC) aerosol module (Zaveri et al., 2008) were selected. The aerosol size distribution is described by eight discrete size bins defined by their lower and upper dry particle diameters ranging from 39 nm to 10 µm. Aerosols are assumed internally mixed in each bin to engage in microphysical processes. To participate in the radiative processes, each aerosol component is prescribed with a refractive index based on the values suggested in Barnard (2010). To avoid the overestimation of the particle absorption cross section when using the internal mixing of BC with other aerosol components (Bond and Bergstrom, 2006), the Maxwell–Garnett mixing rule assuming spheres of BC distributed randomly throughout a mixture of other aerosol components was applied in this study (Bond and Bergstrom, 2006). Note that the process of BC aging (Peng et al., 2016; Wang et al., 2018) has not been implemented in the model. In the future, it would be desirable to implement BC aging (Peng et al., 2016; Wang et al., 2018) in order to more accurately simulate the mixing state of BC-containing aerosols. With the mixed refractive indices, the aerosol extinction efficiency, single-scattering albedo, and asymmetry factor are computed using a Mie algorithm for each size bin and wavelength. The total optical properties are then obtained by integrating over all of the size bins and used as inputs to the RRTMG radiation transfer model for the shortwave (Fast et al., 2006) and longwave spectrum (Zhao et al., 2013). Aerosol–cloud interactions are accounted for in the model through three pathways: activation of aerosol particles to form cloud droplets as well as their resuspension from evaporating cloud droplets, aqueous chemistry, and wet deposition (Chapman et al., 2009). Aerosols are treated as “interstitial” or “cloud-borne” according to whether they are activated as CCN, and the calculation of the activation process follows the methodology of Abdul-Razzak (Abdul-Razzak and Ghan, 2002). The two-moment Lin microphysics scheme (Lin et al., 1983; Rutledge, 1984) was employed in this study, where prognostic cloud droplet number is treated based on activated aerosols following Ghan et al. (1997), and the autoconversion of cloud droplets to rain droplets is dependent on droplet number (Liu et al., 2005) so that aerosols are allowed to potentially influence the rain rate and liquid clouds (Ghan et al., 1997; Chapman et al., 2009). The aerosol-aware Lin microphysics scheme has been used previously in investigating aerosol impacts on synoptic cyclones (Ye et al., 2019), regional fog (Lee et al., 2016), and local convection systems (Wu et al., 2011). In order to validate the response of our model to increasing CCN, monthly mean domain-averaged cloud droplet radii and corresponding cloud-base CCN concentrations were calculated for simulations with different emission rates, shown in Fig. S1 in the Supplement. The sensitivity of cloud droplet radius to increasing CCN concentration is pronounced at lower CCN concentrations, while the response tends to saturate at higher CCN concentrations. The saturation of the response of droplet radius to aerosol concentration has also been observed by satellite (Breon et al., 2002). These observations suggested a saturation point at AOD of 0.3, which corresponds to the relatively higher aerosol concentration scenario (EMIS3) in our study. Cloud-borne aerosols and trace gases dissolved in cloud water interact through aqueous chemistry, which may modify aerosol composition and content. The aqueous-phase chemistry is based on the Carnegie Mellon University (CMU) bulk aqueous-phase chemical mechanism (Fahey and Pandis, 2001). Wet deposition of aerosols includes in- and below-cloud removal through being collected by rain, graupel, and snow (Chapman et al., 2009) and through being scavenged by precipitation washout (Easter et al., 2004), respectively. Other major schemes utilized, e.g., the RRTMG longwave and shortwave radiation scheme (Mlawer et al., 1997; Pincus et al., 2003), the Yonsei University (YSU) boundary layer scheme (Hong, 2010), the Rapid Update Cycle (RUC) land surface scheme (Smirnova et al., 1997, 2000), the Grell–Devenyi cumulus parameterization (Grell and Devenyi, 2002), and the Fast-J photolysis rate scheme (Wild et al., 2000), are described in Table 1.
In this study, three nested domains with horizontal resolutions of 75, 15, and 3 km were set up over South America, as shown in Fig. 1. Domain1 covers most of the South American continent, with the biomass burning source region included. Domain3 centers around the Amazon Tall Tower Observatory (ATTO) site to represent the typical climate and environment of the central Amazon Basin (Andreae et al., 2015) and uses cloud-resolving grid spacing with the Grell cumulus parameterization turned off (Table 1). Vertical layers of 29 levels extending from ground to 50 hPa were employed for all domains. The outer domains were two-way coupled with initial and boundary meteorological and chemical conditions from the 6 h National Centers for Environmental Prediction (NCEP) Final Analysis (FNL) data and Model for Ozone and Related Chemical Tracers, version 4 (MOZART-4), global chemical transport model output (Emmons et al., 2010), respectively. The Four Dimensional Data Assimilation (FDDA) of temperature, horizontal wind, and moisture was applied for the outer domains to reduce meteorological biases (Otte et al., 2012). The innermost domain was driven one way by initial and boundary inputs from the outer domain. No nudging was used in the innermost domain. The aerosol-induced perturbations were estimated with the meteorological fields simulated in domain3. Anthropogenic emissions were from the EDGAR-HTAPv2, a global gridded air pollution emission dataset with a resolution of (http://edgar.jrc.ec.europa.eu/htap_v2, last access: 6 October 2020; Janssens-Maenhout et al., 2015). The biogenic emissions were generated online by Model of Emissions of Gases and Aerosols from Nature (MEGAN; Guenther et al., 2006). The Fire Inventory from NCAR version 1.5 (FINNv1.5; Wiedinmyer et al., 2011), which provides global estimates of the trace gas and particle emissions from open fires updated daily with 1 km resolution, was used to provide the biomass burning emissions. The primary organic matter (POM) emission rate was converted from organic carbon (OC) emission based on an observed ratio of 1.5 between the mass of POM and OC (Reid et al., 2005). The conversion factor 1.5, broadly used in WRF-Chem simulations for biomass burning emission (Ge et al., 2014; Archer-Nicholls et al., 2015), represents the lower end of the range of POM∕OC ratios for fresh aerosol emissions from biomass burning (Yokelson et al., 2009; Takahama et al., 2011; Brito et al., 2014; Collier et al., 2016; Andreae, 2019). Plume ascent from fire emission sources is calculated by a plume rise parameterization (Grell et al., 2011; Freitas et al., 2007). The simulation spans from 24 August to 30 September 2014, when the Amazon Basin was undergoing its dry season with biomass burning prevalent. The simulation was conducted at 72 h time slots, with the last 48 h being used for analysis. In each recycle, the meteorological field was reinitialized, while the chemical field was restarted from the preceding run. The first 6 d of the simulation were used as spinup. Details on model configurations are listed in Table 1.
2.2 Design of numerical experiments
In order to quantitatively investigate the impact of BB aerosols on radiation, cloud, and precipitation, a set of BB aerosol emission scenarios generated by multiplying different aerosol emission factors (X) with the original BB aerosol emission scenario was applied to all domains. As subgrid convective parameterization can cause uncertainties to the impacts from BB aerosols due to the lack of aerosol–cloud interactions in the subgrid convective parameterization (Archer-Nicholls et al., 2016), the analysis of BB aerosol effects in Sect. 3 is based on the domain3 simulation where convections are explicitly resolved at 3 km resolution. Simulations of domain3, namely PC3_EMISX, were conducted using the BB aerosol emission scenario (EMISX) and chemical boundary conditions from the outer-domain simulation with the corresponding emission scenario. A control simulation CC3 was conducted without influence of biomass burning emissions. Then the total effects of BB aerosols can be evaluated from the difference between the PC3_EMISX and CC3 simulations.
As shown in Fig. 2, the biomass burning emissions during September undergo large annual variations, e.g., the emission in 2007 is 6 times as much as that in 2014. The variation pattern of PM10 emitted from BB in September is consistent with an interannual variation in MODIS-retrieved AOD over the Amazon (Sena et al., 2013). Based on the range of emission intensities from 2002 to 2016, we set three emission scenarios representing different emission strength: EMIS1 for emission in 2014; EMIS3 for an average intensity over all years; and EMIS6 for the emission intensity in 2007, which corresponds to the maximum emission intensity from 2002 to 2016. In addition, emission scenario EMIS0.5 was added to mimic the reduced BB emissions projected assuming the influence of enhanced government regulation policy (Streets, 2007). The domain3-averaged AOD in the simulations for the EMIS0.5, EMIS1, EMIS3, and EMIS6 emission scenarios is used to represent the aerosol concentration under the corresponding emission scenarios in the analysis in Sect. 3.
To assess the ACI and the ARI effect of BB aerosols separately and jointly, we calculated the ACI and ARI effect following the method used in Archer-Nicholls et al. (2016). Parallel simulations with PC3_EMISX and CC3 were performed in the absence of aerosol radiative feedbacks, namely PCNR3_EMISX and CCNR3, respectively (Table 2). The ACI effect of BB aerosols in each emission scenario can be assessed from the difference between PCNR3_EMISX and CCNR3, where aerosols were radiatively inactive and only the aerosol effect on cloud microphysics was included. Then the ARI effect of BB aerosols was obtained by deducting the ACI effect from the aerosol total effect (Archer-Nicholls et al., 2016). This way of calculating the ARI of BB aerosols enables assessments of the ARI solely from BB aerosols without the influence of aerosols from other origins (Ghan et al., 2012). Due to the nonlinear nature of the cloud system, which involves complicated microphysics–dynamics–thermodynamics feedbacks (Stevens and Feingold, 2009), the ARI effect calculated as the residual component of the aerosol total effect aside from the ACI part may be different from directly contrasting the simulations with and without the radiative effect from BB aerosols. To assess this uncertainty, we compared the ARI effect on clouds obtained here with its counterpart, i.e., the difference between PC3_EMIX and PCNR3_EMISX, which directly computes the effect associated with aerosol–radiation interactions from all aerosols, based on the EMIS6 scenario to minimize the influence of aerosols not from BB (Table S1). It shows that the uncertainty in the ARI quantification associated with the cloud nonlinear microphysics–dynamics–thermodynamics feedbacks is very small and would not have a significant influence on the ARI assessment in this study.
The WRF-Chem simulation with the EMIS1 scenario was evaluated for the meteorological conditions and the aerosol field using ground-based, radiosonde, and satellite remote sensing measurements (see Sections S1–S3 in the Supplement). The results show that the model simulation at 3 km resolution reasonably reproduces the metrological field in terms of surface conditions, vertical atmospheric structure, and regional precipitation. The total cloud fraction and liquid cloud amount are well captured by the model, while the simulated ice water amount shows lower magnitude than the observations. The model generates close agreement of the predicted aerosol properties with the observations, including the aerosol optical properties (AOD and single-scattering albedo) and the CCN concentrations at different supersaturation conditions. Details of the model evaluation are provided in the Supplement. The satisfactory performance of the model enables it to provide reliable assessments of the BB aerosol effects on the regional climate through aerosol–radiation–cloud interactions.
3.1 Impact on radiation
Figure 3 shows the diurnal cycle of the BB aerosol impact on the domain-averaged all-sky shortwave radiation based on the EMIS1 emission scenario. The ACI effect, in which BB aerosols act as CCN, causes negative radiative perturbations to shortwave radiation both at TOA (Fig. 3a) and the surface (Fig. 3b) during the daytime. This can be attributed to the increased cloud albedo as a result of larger cloud liquid water path (LWP) and smaller cloud droplet radius (Table 3) caused by the ACI (Twomey, 1977). The shortwave radiative forcing (RF) at TOA is estimated to be −0.7 W m−2 for the ACI effect.
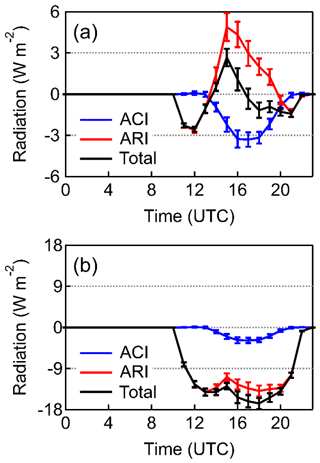
Figure 3Diurnal variation in changes in all-sky shortwave radiation at (a) TOA and (b) surface in the EMIS1 emission scenario. Error bars denote the standard error.
Table 3Summary of monthly mean perturbations caused by the ARI and ACI of BB aerosols in the EMIS1 and EMIS6 emission scenarios.
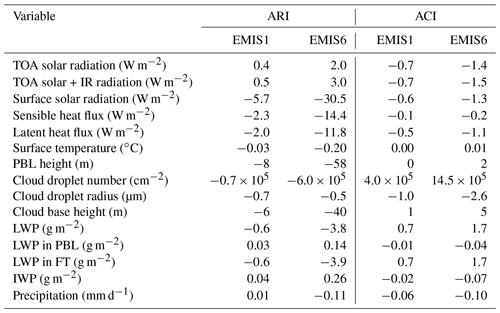
The radiation perturbations due to the ARI effect are more complicated as they involve the direct radiative effect of BB aerosols themselves and subsequent cloud adjustments. Figures 3 and S10 show a clear difference in radiative forcing with and without considering clouds (all-sky versus clear-sky conditions). In clear-sky cases, BB aerosols reduce the shortwave radiation reaching the ground by directly scattering and absorbing incident solar radiation, leading to a reduction of shortwave radiation at the surface of −6.7 W m−2 (Fig. S10b). The clear-sky shortwave RF by ARI at TOA is negative for most of the day except at local noon (15:00 to 17:00 UTC) when the planetary boundary layer (PBL) fully develops (Fig. S10a). This diurnal variation can be explained by the evolution of aerosol vertical distributions. The vertical location of absorbing aerosols is an important controlling factor for their absorptivity (Samset and Myhre, 2011). When aerosols are lifted higher by the vigorously grown PBL, the absorption of solar radiation by BB aerosols is amplified, resulting in more heating and positive forcing. On average, the clear-sky shortwave RF by ARI at TOA is about −0.7 W m−2 and corresponds to a cooling effect on the Earth–atmosphere systems, which is consistent in sign with observational and modeling results in this region (Sena et al., 2013; Archer-Nicholls et al., 2016; Thornhill et al., 2018). When taking clouds into consideration, the all-sky shortwave radiative perturbation by ARI is about −5.7 and 0.4 W m−2 at the surface and TOA (Table 3), respectively. Compared with the clear-sky results, the positive shifts of radiative perturbation by ARI in all-sky conditions at both the surface and TOA indicate less solar radiation reflected back to space. This can be accounted for by the decreased liquid cloud water content (Table 3) due to the BB aerosols' radiative effect, which results in more incident solar radiation (so-called semi-direct effect). Seen from the diurnal cycle of shortwave forcing by the ARI (Fig. 3a), the time period when the radiative forcing is positive becomes longer, although negative values still exist in the early morning and late afternoon when the cloud response is negligible (Fig. 6b). In previous studies, the positive radiative forcing associated with the reduction of cloud cover was shown to be very strong (Zhang et al., 2008), even to the point of being able to reverse the sign of the BB aerosols' direct radiative forcing over the Amazon (Koren et al., 2004; Archer-Nicholls et al., 2016).
The total shortwave RF at TOA by BB aerosols is a result of the competing ACI and ARI effects. Figure 4a shows the total shortwave RF caused by BB aerosols from emission scenarios with different aerosol emission intensities (represented as the domain-averaged AOD). The relative importance of the ACI and ARI effects on shortwave RF varies with the aerosol loading under the same atmospheric conditions. The total shortwave forcing is negative at lower aerosol loading, dominated by the ACI effect, but shifts to be positive at higher aerosol loading, driven by the ARI effect. This is expected because the addition of aerosols changes the cloud properties more severely at low background aerosol concentrations than at higher aerosol abundance where the microphysical effect tends to be saturated (Twomey, 1977; Roberts et al., 2003); and the ARI effect associated with aerosol extinction of radiation intensifies with increasing aerosol concentration (Koren et al., 2004).
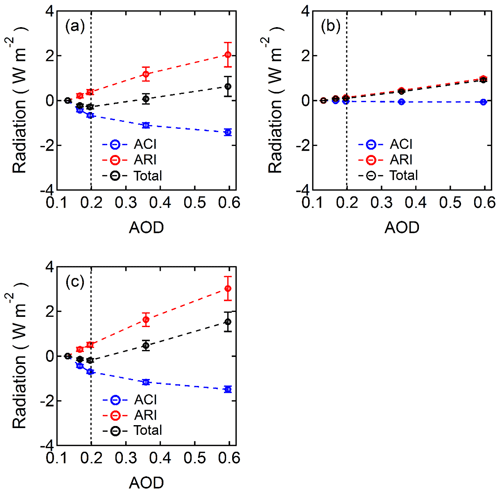
Figure 4Changes in (a) shortwave, (b) longwave, and (c) total radiation budgets at TOA with increasing BB emission intensity (indicated by domain-averaged AOD in each emission scenario). The vertical dotted line in each plot indicates the EMIS1 scenario. Error bars denote the standard error.
Such nonlinear ACI and ARI effects of BB aerosols are consistent with their effects on cloud water (Fig. 5a), implying the importance of cloud adjustments for affecting BB aerosol RF. At TOA, the monthly mean shortwave RF by BB aerosols (ACI + ARI) is −0.3 and 0.6 W m−2 for the EMIS1 and EMIS6 scenarios, respectively (Table 3). Similar in magnitude, a modeling study in the Amazon dry season using the HadGEM3-GA3 model showed a monthly mean shortwave RF of 1.35 W m−2 with AOD increasing from 0.19 to 0.67 (Thornhill et al., 2018). The longwave RF by BB aerosols is of comparable magnitude to the shortwave radiative forcing (Table S4). The ARI effect is the driving force for the positive longwave RF, as the outgoing infrared radiation can be directly trapped by black carbon contained within the BB particles (Ramachandran and Kedia, 2010). In addition, high clouds mainly comprised of ice are also efficient at blocking outgoing longwave radiation (Hartmann et al., 1992), yielding a positive longwave RF at TOA. Therefore, the ARI-induced larger amount of cloud ice content (Fig. 6b) can result in positive longwave RF as well. The positive longwave RF resulting from increased ice cloud is in agreement with the satellite observations of tropical deep convection, where a strong warming was caused by increased convective cloud anvils impacted by aerosols (Koren et al., 2010). However, it should be noted that, as the ice cloud response is a crucial factor for determining the longwave RF, the lack of parameterization of the aerosols' role as IN adds uncertainty to the simulated longwave RF by BB aerosols. The appreciable magnitude of longwave RF (Thornhill et al., 2018; Archer-Nicholls et al., 2016) underlines the necessity of further studies to constrain the BB aerosol effect on high clouds. The all-band RF (shortwave plus longwave) of BB aerosols changes sign with increasing emission intensity of BB aerosols, with values of −0.2 and 1.5 W m−2 for the EMIS1 and EMIS6 scenarios, respectively.
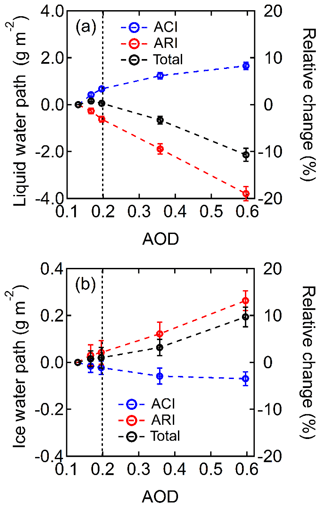
Figure 5Changes in (a) cloud LWP and (b) cloud IWP with increasing BB emission intensity (indicated by domain-averaged AOD in each emission scenario). The vertical dotted line in each plot indicates the EMIS1 scenario. Error bars denote the standard error.
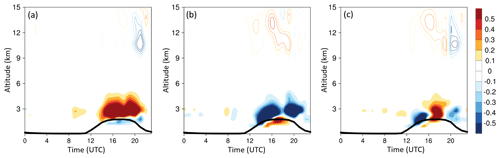
Figure 6Diurnal variation in the vertical distribution of the domain-averaged difference in cloud water (shaded; in mg kg−1) and cloud ice (contour lines; in 0.1 mg kg−1) caused by BB aerosols' (a) ACI, (b) ARI, and (c) total effect in the EMIS1 emission scenario. The thick black line represents the PBL height.
At the surface, a reduction in shortwave radiation is induced by the presence of BB aerosols, which intensifies with higher emission intensity. Compared with previous model estimates, a −15.9 W m−2 shortwave reduction estimated from a multiday biomass burning simulation in 2006 using WRF-Chem (Wu et al., 2011) is of similar magnitude to the −17.1 W m−2 in this study using the EMIS3 scenario, which is almost equivalent to the emission intensity of the year 2006. However, the magnitude of the estimates diverges in different models – e.g., −28.2 W m−2 was induced by an increase in AOD of about 0.4 using the GATOR-GCMOM model (Ten Hoeve et al., 2012), and W m−2 was calculated with an increase in AOD of about 0.5 using HadGEM3-GA3 (Thornhill et al., 2018), which may result from different parameterizations of the aerosol optical properties and treatments of cloud response. The decreased solar radiation at the surface is balanced mostly (over 90 %) by a reduced sensible and latent heat flux (Table 3) and marginally by the earth-emitted infrared radiation. Specifically, the ARI led to a decrease of −2.9 % (−17.6 %) and −2.0 % (−12.0 %) for sensible heat and latent heat, respectively, in the EMIS1 (EMIS6) scenario, which could impose an inhibiting effect on cloud formation (Yu et al., 2002; Feingold et al., 2005; Jiang and Feingold, 2006; Rosenfeld et al., 2008).
3.2 Impact on atmospheric stability
Figure 7 shows the diurnal and vertical distribution of the BB aerosol impact on the domain-averaged air temperature, relative humidity (RH), and vertical velocity for the EMIS1 scenario. Pronounced responses of air temperature and RH occur below 5 km, where aerosols are concentrated. Affected by the ARI, air temperature is reduced within the PBL but is increased at the top of the PBL (Fig. 7b). As aforementioned, BB aerosols reduce the incident solar radiation at the surface and therefore decrease the heat flux from the ground to the atmosphere. Consequently, the air temperature within the PBL drops, with a diurnal maximum reduction of over −0.05 ∘C near the surface. The solar radiation absorbed by the black carbon in BB aerosols heats the atmosphere (Bond and Bergstrom, 2006), producing an increase in air temperature by about 0.03 ∘C near the top of the PBL. This vertical distribution of temperature responses tends to stabilize the PBL and suppress the upward velocity (Fig. 7b). On the other hand, the increased air temperature at the top of the PBL destabilizes the air above and stimulates updrafts (Feingold et al., 2005; Koch and Del Genio, 2010). The intensified upward airflow delivers more water vapor to higher altitudes, leading to a pronounced RH increase at altitudes above 10 km. The ACI effect acts opposite to the ARI effect in changing the thermodynamic structure. The air cools at the top of the PBL, since more evaporation-induced cooling is generated with more but smaller cloud droplets (Table 3). In contrast, higher air temperatures within the PBL can be the result of less evaporative cooling from precipitating hydrometeors (Fig. 8a). Overall, the thermodynamic response to BB aerosols is dominated by the ARI effect. The diurnal mean change in surface air temperature is −0.2 ∘C in the EMIS6 scenario (Table 3), in agreement with other modeling results for the Amazon area (Kolusu et al., 2015; Thornhill et al., 2018).
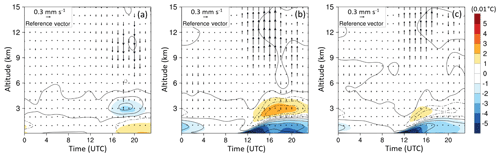
Figure 7Domain-averaged difference in air temperature (shaded, in intervals of 0.01 ∘C), relative humidity (contour lines, in intervals of 0.1 %), and updraft velocity (arrows) caused by BB aerosols' (a) ACI, (b) ARI, and (c) total effect in the EMIS1 emission scenario.
3.3 Impact on cloud
Figure 6 shows the diurnal and vertical distribution of domain-averaged changes in cloud water and cloud ice concentration caused by BB aerosols. By serving as CCN, BB aerosols create more cloud droplets and cause a reduction in the cloud droplet size (Table 3) due to competition for water vapor, which slows down the transfer rate from cloud to rain (Rosenfeld et al., 2008; Chang et al., 2015; Braga et al., 2017). Consequently, cloud water in the free troposphere is increased by the ACI effect throughout the day (Fig. 6a) at the expense of rainwater concentration, while the diminished cloud water within the PBL corresponds to the warmer air temperature (Fig. 7a) and suppressed moisture flux from the ground surface (Table 3).
The response of cloud water to ARI also varies with altitude. The increased RH within the PBL by the aerosol radiative effect (Fig. 7b) lowers the cloud base height (Table 3) and favors cloud persistence, resulting in higher cloud water content (Johnson et al., 2004). In contrast, the aerosol radiative heating near the top of the PBL (Fig. 7b) decreases the RH and therefore “burns off” the liquid clouds (Feingold et al., 2005; Huang et al., 2016). Such contrasting cloud water responses to the BB aerosol radiative effect between different layers were also found by a large-scale RegCM3 simulation covering South America (Zhang et al., 2008). The increased cloud water in the lower troposphere (0–2 km) was attributed to large-scale moisture convergence. Here, the simulation over a smaller region located in the central Amazon Basin shows that the local modification of the thermodynamic structure by BB aerosols is able to contribute to the effect as well.
Integrated over the atmosphere, the cloud LWP is enhanced by the ACI but reduced by the ARI effect (Ackerman et al., 2000; Johnson et al., 2004; Feingold et al., 2005). Therefore, the overall change in cloud water amount by BB aerosols results from the competition between the ACI and ARI effects. Figure 5 displays the dependence of the overall response of cloud water on the emission intensity (represented as AOD). Weaker emission scenarios yield higher cloud water, driven by the ACI effect, while stronger emissions lead to an opposite response of cloud water, dominated by the ARI effect. The simulated dependence of cloud water change on aerosol amount agrees with satellite measurements of the total cloud fraction over the Amazon region (Koren et al., 2004).
The cloud ice content is invigorated by BB aerosols, driven by the ARI effect (Fig. 6b). Through radiation absorption, BB aerosols heat the air, evaporate liquid cloud, and promote upward flux of vapor and moisture to higher altitudes (Fig. 7b), facilitating cloud ice formation there. Similar ice enhancement due to aerosol radiative heating was also seen in simulations of dust–radiation interaction (Dipu et al., 2013). This positive response of cloud ice and updraft velocity to ARI corresponds to the thermodynamic invigoration mechanism proposed in Wang et al. (2013), which suggested larger convective available potential energy (CAPE) above PBL could be induced by the absorbing aerosols in the lower troposphere. In contrast, the ACI tends to act in opposition to the ARI effect but in a minor magnitude, showing a moderate negative response (Fig. 6a). The ACI effect has been reported to invigorate deep convection when more abundant, smaller cloud drops are uplifted to boost the cloud microphysical processes at higher altitudes (Rosenfeld et al., 2008), which, however, is sensitive to the background environment (Khain et al., 2005; Fan et al., 2009). Hints of this effect are only seen during a narrow time span around 18:00 and 22:00 UTC, as indicated by increased cloud ice (Fig. 6a) and precipitating hydrometeors (Fig. 8a). However, the enhancement is insignificant in magnitude and overwhelmed by the negative responses that persist during the rest of the diurnal cycle, which may result from different cloud types and environmental conditions from those in Rosenfeld et al. (2008). Generally, the monthly mean domain-averaged results show a negative effect of the ACI on cloud ice water path (IWP; Fig. 5b). However, the role of ACI could be more complicated than what is found here, because the ACI effect may potentially modulate the impact of ARI on the cloud ice (Shi et al., 2014; Huang et al., 2019), e.g., by influencing latent heat release, since the ACI effect is turned on when the ARI effect is assessed. The overall increase in cloud ice is in agreement with the fine-resolution simulation results over the biomass burning area by the GATOR-GCMOM model (Ten Hoeve et al., 2012). Although the absolute response of ice concentration is smaller compared to the cloud water change, the relative change in ice concentration is remarkable (Fig. 5b; Lee et al., 2017). It should be noted that uncertainties associated with BB aerosol effects on cloud ice exist, because of the lack of IN parameterization (Fan et al., 2018). Field observations suggested that the BC in the BB aerosols could contribute substantially to ice nucleation (McCluskey et al., 2014), which may influence the estimate of the response of cloud ice to BB aerosols.
3.4 Impact on precipitation
To show the response of precipitation, the diurnal and vertical distribution of domain-averaged changes in precipitating hydrometeors (sum of rain, snow, and graupel) based on the EMIS1 scenario is presented in Fig. 8. The domain-averaged rainwater below the freezing level height of about 5 km shows a prominent negative response to ACI during most of the daytime (Fig. 8a). As discussed before, by acting as CCN, the BB aerosols reduce cloud droplet radius, slow down the conversion rate from cloud to rain, and therefore inhibit warm rain formation. On the other hand, consistent with the responses of cloud ice, precipitating hydrometeors are enhanced by the ACI effect in the local afternoon and early night due to the invigorated convection. Generally, an overall reduction in precipitation is induced by the ACI, similar to previous WRF-Chem simulations of BB aerosol microphysical effects in the Amazon (Wu et al., 2011). The results of the ARI effect show an overall positive impact on precipitating hydrometeor concentrations (Fig. 8b) and consequent surface precipitation (Fig. 9a) in the EMIS1 scenario. With the influence of the ARI effect, significant enhancement appears in the precipitating hydrometeors above the freezing level beginning in the early morning, indicating cold rain processes. Specifically, the graupel concentration, which is mainly responsible for the cold rain response (Fig. S13), is promoted as more supercooled cloud droplets efficiently feed the growth of graupel. The increased supercooled cloud water concentration could be a result of the enhanced updraft promoted by the ARI (Fig. 7b). By 17:00–18:00 UTC, the precipitation reaching the surface is increased correspondingly. The increase in precipitation by the local ARI effect was also found in previous simulations of light-absorbing aerosols, including black carbon (Lin et al., 2016) and mineral dust (Dipu et al., 2013; Shi et al., 2014; Huang et al., 2019). Influenced by the overall effect of both the ACI and ARI mechanisms, a reduction of precipitating hydrometeors is prominent in the morning and afternoon, while enhanced precipitating hydrometeor abundance occurs in a narrow time span from local noon to early afternoon. The variation in convection response throughout the convective evolution cycle implies a possible dependence of aerosol–radiation—cloud interactions on environmental stabilization, which is also shown by the observation that BB aerosols tend to increase precipitation under unstable conditions (Gonçalves et al., 2015).
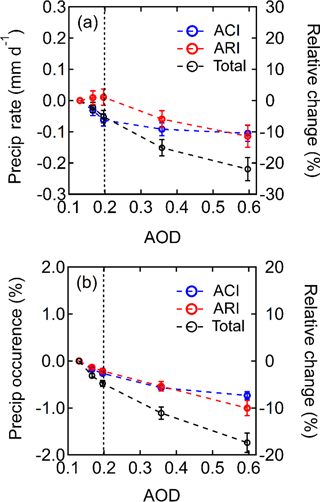
Figure 9Changes in domain-averaged (a) precipitation rate and (b) precipitation occurrence with increasing BB emission intensity (indicated by domain-averaged AOD in each emission scenario). The vertical dotted line in each plot indicates the EMIS1 scenario. Error bars denote the standard error.
The response of the precipitation rate to different emission intensities of BB aerosols (represented as AOD) is shown in Fig. 9a. The precipitation reduction by ACI is climatically significant in all emission scenarios, with a monthly mean change of −7 % and −11 % at EMIS1 and EMIS6, respectively. The precipitation rate is slightly increased by the ARI at low aerosol loading due to invigorated daytime precipitation as discussed above. However, at high emission intensity, the strong radiative dimming effect of BB aerosols dramatically reduces surface heating (Table 3), which damps the ARI-induced convection invigoration (Fig. S12b) and leads to an overall suppression of convection and a significant reduction of precipitation (Rosenfeld et al., 2008), as reflected by diminished liquid clouds (Fig. S14) and precipitating hydrometeors (Fig. S12b). This dimming effect is even more pronounced than the ACI effect in reducing precipitation for the EMIS6 scenario (Fig. 9). Taking the ACI and ARI effects together, the monthly mean precipitation rate is decreased by BB aerosols at all emission scenarios used in this study. A reduction of −5 % and −23 % is calculated for the EMIS1 and EMIS6 emission scenarios, respectively, aligning in magnitude with a precipitation change by −14.5 % for the switch of aerosol loading from the low-emission to the high-emission scenario in the Amazon found by Thornhill et al. (2018). To examine the precipitation responses at different precipitation intensities (Fig. 10), a threshold of daily maximum 3 h accumulated precipitation exceeding 3 mm, the upper boundary of the domain averaged amount (Fig. S6), is used to distinguish the intensive precipitation grids from the light precipitation ones. High convective strength indicated by larger CAPE (Fig. 10) corresponds to intensive precipitation, whereas relatively weaker convection is associated with the light precipitation regime. Intensive precipitation shows a significant nonlinear ARI response, whereas light precipitation tends to be reduced monotonically by the ARI. The precipitation reduction by ACI at low aerosol concentration is less prominent in heavy than in light rainfall, due possibly to the dynamic feedbacks in deep convection (Rosenfeld et al., 2008). By contrast, a stronger ACI effect at larger aerosol amounts is shown in heavy precipitation as a result of the larger potential for CCN activation in strong convection (Reutter et al., 2009). The dependence of precipitation change on aerosol concentration is greater for the intensive precipitation than the light precipitation regime, given that the precipitation responses in the EMIS1 and EMIS6 scenarios are −3 % and −27 %, respectively, for the intensive regime and −8 % and −17 %, respectively, for the light precipitation. This is consistent with the rainfall sensitivity to increasing aerosol concentration for strong and weak convection in Chang et al. (2015). The dominance role of ARI over ACI at high aerosol loadings is found at both regimes. The precipitation occurrence (calculated as the ratio of precipitating grid cells to the total domain grid cells over the simulation period), which is approximately 11 % in the clean case, is reduced noticeably by both the ACI and ARI effects (Fig. 9). The more extensive dry area coverage due to the presence of BB aerosols may act to aggravate the precipitation shortage for the Amazon forest in the dry season (Cox et al., 2008).
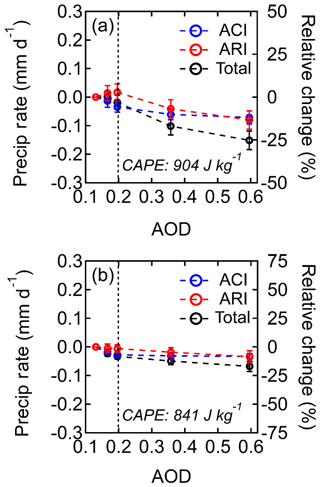
Figure 10Changes in domain-averaged precipitation rate with increasing BB emission intensity (indicated by domain-averaged AOD in each emission scenario) in (a) the intensive precipitation regime and (b) light precipitation regime. The vertical dotted line in each plot indicates the EMIS1 scenario. Error bars denote the standard error.
In this study, a comprehensive assessment of the impacts of BB aerosols on the regional radiation balance, cloud properties, and precipitation and their sensitivity to interannual variations in BB aerosol emissions was conducted using the fully coupled WRF-Chem model with a 3 km resolution domain in the central Amazon Basin for the dry season. Parallel numerical experiments were performed with different emission scenarios by scaling up and down the original emission rate of the year 2014. These experiments with varying emission scenarios, together with experiments switching off the aerosol–radiation interactions in the model, were performed to separate the effects of ARI and ACI, which enables us to quantify each effect individually and compare their relative significance.
The results show that the shortwave RF by BB aerosols is the outcome of a competition between positive RF by the ARI effect and negative RF by the ACI effect, which is driven largely by the cloud response. The positive shortwave RF associated with cloud reduction due to the semi-direct effect of the BB aerosols counteracts the negative direct shortwave RF and constitutes the dominant component of ARI-induced effective shortwave RF. Contrarily, the ACI-induced more numerous but smaller cloud droplets increase cloud albedo and thereby exert a negative indirect shortwave RF. The relative significance of the ACI and ARI effects varies with aerosol loading, with a dominant role of the former at low aerosol emission rate, while the latter dominates at high emission intensity. The positive longwave RF by BB aerosols is driven by the ARI effect, through both aerosol direct radiative forcing and subsequent adjustment of enhanced ice cloud. The all-band aerosol RF is −0.2 and 1.5 W m−2 for BB aerosols in the EMIS1 and EMIS6 scenarios, respectively. Surface shortwave radiation is reduced by BB aerosols, with an estimate of −17.1 W m−2 for a multiyear-averaged emission intensity (EMIS3), which is compensated for mostly by suppression of sensible and latent heat flux from ground to the atmosphere. The response of cloud LWP to BB aerosols is driven in opposite directions by the ARI and ACI effects. The surface cooling generated by radiation extinction together with the atmospheric heating from absorption of solar radiation stabilizes the atmosphere, inhibits convection development, and thereby decreases the cloud LWP. In contrast, higher cloud LWP is produced by the ACI effect through inhibited warm rain formation. The relative significance of the competing effects depends on the aerosol amount, consistent with the aerosol shortwave radiative forcing response, implying a crucial role of cloud adjustments in determining aerosol radiative forcing on the Earth–atmosphere system. Enhanced cloud IWP with the presence of BB aerosols is related to a stronger upward flux of water vapor induced by the ARI effect.
Lower precipitation occurrence is induced by both the ARI and ACI effects, which implies a larger fraction of dry areas in the Amazon Basin when affected by BB aerosols, threatening to exacerbate droughts during the dry season. The domain-averaged precipitation rate is diminished substantially by ACI consistently over all the emission scenarios used in this study, implying the importance of including ACI effects on the subgrid cumulus convection when applying large-scale simulations at coarse grid resolution (Archer-Nicholls et al., 2016). Strong suppression of warm rain formation is responsible for the precipitation reduction caused by the ACI, but in the lower-emission scenarios, an ACI-induced invigoration of deep convection occurs during a narrow time period, due to latent heat release from more abundant smaller droplets aloft (Rosenfeld et al., 2008). The precipitation response to the ARI effect is nonlinear due to the effects of mixed-phase precipitation. At low BB aerosol emission rates, enhanced mixed-phase precipitation is found as a result of higher graupel content with the enhanced supply of supercooled cloud droplets by the ARI, while the invigoration disappears in the high-emission scenarios with reduced presence of supercooled cloud droplets due to overwhelming suppression of convection by BB aerosols. Reduction in monthly mean precipitation rate by the overall effects of BB aerosols is found for all emission scenarios and intensifies with aerosol loading, which may imply a positive feedback between precipitation scavenging and aerosol concentration for intense BB events. A reduction of monthly mean precipitation rate by −5 % and −23 % is estimated for the EMIS1 and EMIS6 scenarios, respectively, suggesting a strong sensitivity of precipitation to aerosol concentration. The sensitivity of precipitation change to aerosol concentration is more prominent in the intensive precipitation regime than in the light precipitation case.
The high-sensitivity and nonlinear relationship between regional radiation, liquid water content, precipitation, and BB aerosol abundance highlights the importance of comprehensive assessments of BB aerosol effects in the Amazon with multiannual aerosol emission scenarios. The variation in the ACI and ARI effects with increasing aerosol emission revealed a saturating tendency for the ACI, in contrast to a continuously increasing effect of the ARI at high aerosol loadings. This may shed light on the climatic importance of the ARI in highly polluted regions and during episodes with severe combustion aerosol emissions such as intensive wildfires, industrialization-related fossil fuel combustion, and agricultural crop waste burning. The key role of the ARI also highlights the importance of accurate representation of aerosols and their optical properties in models when addressing their climate effects.
It should be noted that this study only focuses on the local effects of BB aerosols for a typical region in the Amazon Basin. The large-scale response in the atmospheric field (Lee et al., 2014) caused by horizontally inhomogeneous responses to unevenly distributed aerosols is out of the scope of this study. The role of aerosols acting as IN has not been included in the WRF-Chem model used here. Parameterization of this mechanism is needed to better quantify aerosol effects on climate. In addition, further investigations on the formation mechanisms and light absorption associated with SOA are needed to better parameterize the physical and optical properties of organic aerosols in the model (Shrivastava et al., 2017, 2019), in order to better recognize the role of BB aerosols in the climate system. Furthermore, the sensitivity of the climate response to the concentration of BB aerosols may be influenced by the meteorological conditions, and as this study is based on September 2014, continuing model investigations based on varying and longer periods are needed to characterize the influence of variations in meteorology and to provide climatic assessments.
The model results presented in this work is available at https://doi.org/10.17617/3.4m (Liu, 2020).
The supplement related to this article is available online at: https://doi.org/10.5194/acp-20-13283-2020-supplement.
YC and HS designed and led the study. LL performed the model simulation and analyzed the data. LL, HS, and YC interpreted the results. SW and CW supported model simulation and data visualization. MOA, MS, and UP discussed the results. MP, CP, and PA contributed data for model validation. LL wrote the paper with input from all coauthors.
The authors declare that they have no conflict of interest.
We acknowledge the MODIS, TRMM, AERONET, and IGRA teams for the data used in this study. Furthermore, we would like to thank the ATTO project and all the people involved in it for support of the observations for model validation.
This research was supported by the Max Planck Society (MPG) through the Minerva Program. The ATTO project as well as the construction and operation of the ATTO site have also been supported by the German Federal Ministry of Education and Research (BMBF contracts 01LB1001A and 01LK1602B), the Brazilian Ministério da Ciência, Tecnologia e Inovação (MCTI/FINEP contract 01.11.01248.00), the Amazon State University (UEA), FAPEAM, LBA/INPA, and SDS/CEUC/RDS-Uatumã. Manish Shrivastava was supported by the U.S. Department of Energy (DOE), Office of Science, Office of Biological and Environmental Research, through the Atmospheric System Research (ASR) and Early Career Research programs.
The article processing charges for this open-access
publication were covered by the Max Planck Society.
This paper was edited by Hailong Wang and reviewed by two anonymous referees.
Abdul-Razzak, H. and Ghan, S. J.: A parameterization of aerosol activation - 3. Sectional representation, J. Geophys. Res.-Atmos., 107, 4026, https://doi.org/10.1029/2001jd000483, 2002.
Ackerman, A. S., Toon, O. B., Stevens, D. E., Heymsfield, A. J., Ramanathan, V. V., and Welton, E. J.: Reduction of tropical cloudiness by soot, Science, 288, 1042–1047, 2000.
Albrecht, B. A.: Aerosols, cloud microphysics, and fractional cloudiness, Science, 245, 1227–1230, https://doi.org/10.1126/science.245.4923.1227, 1989.
Andreae, M. O., Artaxo, P., Brandao, C., Carswell, F. E., Ciccioli, P., da Costa, A. L., Culf, A. D., Esteves, J. L., Gash, J. H. C., Grace, J., Kabat, P., Lelieveld, J., Malhi, Y., Manzi, A. O., Meixner, F. X., Nobre, A. D., Nobre, C., Ruivo, M. D. L. P., Silva-Dias, M. A., Stefani, P., Valentini, R., von Jouanne, J., and Waterloo, M. J.: Biogeochemical cycling of carbon, water, energy, trace gases, and aerosols in Amazonia: The LBA-EUSTACH experiments, J. Geophys. Res.-Atmos., 107, 8066, https://doi.org/10.1029/2001jd000524, 2002.
Andreae, M. O., Rosenfeld, D., Artaxo, P., Costa, A. A., Frank, G. P., Longo, K. M., and Silva-Dias, M. A.: Smoking rain clouds over the Amazon, Science, 303, 1337–1342, https://doi.org/10.1126/science.1092779, 2004.
Andreae, M. O., Acevedo, O. C., Araùjo, A., Artaxo, P., Barbosa, C. G. G., Barbosa, H. M. J., Brito, J., Carbone, S., Chi, X., Cintra, B. B. L., da Silva, N. F., Dias, N. L., Dias-Júnior, C. Q., Ditas, F., Ditz, R., Godoi, A. F. L., Godoi, R. H. M., Heimann, M., Hoffmann, T., Kesselmeier, J., Könemann, T., Krüger, M. L., Lavric, J. V., Manzi, A. O., Lopes, A. P., Martins, D. L., Mikhailov, E. F., Moran-Zuloaga, D., Nelson, B. W., Nölscher, A. C., Santos Nogueira, D., Piedade, M. T. F., Pöhlker, C., Pöschl, U., Quesada, C. A., Rizzo, L. V., Ro, C.-U., Ruckteschler, N., Sá, L. D. A., de Oliveira Sá, M., Sales, C. B., dos Santos, R. M. N., Saturno, J., Schöngart, J., Sörgel, M., de Souza, C. M., de Souza, R. A. F., Su, H., Targhetta, N., Tóta, J., Trebs, I., Trumbore, S., van Eijck, A., Walter, D., Wang, Z., Weber, B., Williams, J., Winderlich, J., Wittmann, F., Wolff, S., and Yáñez-Serrano, A. M.: The Amazon Tall Tower Observatory (ATTO): overview of pilot measurements on ecosystem ecology, meteorology, trace gases, and aerosols, Atmos. Chem. Phys., 15, 10723–10776, https://doi.org/10.5194/acp-15-10723-2015, 2015.
Andreae, M. O.: Emission of trace gases and aerosols from biomass burning – an updated assessment, Atmos. Chem. Phys., 19, 8523–8546, https://doi.org/10.5194/acp-19-8523-2019, 2019.
Archer-Nicholls, S., Lowe, D., Darbyshire, E., Morgan, W. T., Bela, M. M., Pereira, G., Trembath, J., Kaiser, J. W., Longo, K. M., Freitas, S. R., Coe, H., and McFiggans, G.: Characterising Brazilian biomass burning emissions using WRF-Chem with MOSAIC sectional aerosol, Geosci. Model Dev., 8, 549–577, https://doi.org/10.5194/gmd-8-549-2015, 2015.
Archer-Nicholls, S., Lowe, D., Schultz, D. M., and McFiggans, G.: Aerosol–radiation–cloud interactions in a regional coupled model: the effects of convective parameterisation and resolution, Atmos. Chem. Phys., 16, 5573–5594, https://doi.org/10.5194/acp-16-5573-2016, 2016.6.
Barnard, J. C., Fast, J. D., Paredes-Miranda, G., Arnott, W. P., and Laskin, A.: Technical Note: Evaluation of the WRF-Chem ”Aerosol Chemical to Aerosol Optical Properties” Module using data from the MILAGRO campaign, Atmos. Chem. Phys., 10, 7325–7340, https://doi.org/10.5194/acp-10-7325-2010, 2010.
Bevan, S. L., North, P. R. J., Grey, W. M. F., Los, S. O., and Plummer, S. E.: Impact of atmospheric aerosol from biomass burning on Amazon dry-season drought, J. Geophys. Res.-Atmos., 114, D09204, https://doi.org/10.1029/2008jd011112, 2009.
Bond, T. C., and Bergstrom, R. W.: Light Absorption by Carbonaceous Particles: An Investigative Review, Aerosol Sci. Tech. 40, 27–67, https://doi.org/10.1080/02786820500421521, 2006.
Bony, S., Colman, R., Kattsov, V. M., Allan, R. P., Bretherton, C. S., Dufresne, J. L., Hall, A., Hallegatte, S., Holland, M. M., Ingram, W., Randall, D. A., Soden, B. J., Tselioudis, G., and Webb, M. J.: How well do we understand and evaluate climate change feedback processes?, J. Climate, 19, 3445–3482, https://doi.org/10.1175/Jcli3819.1, 2006.
Braga, R. C., Rosenfeld, D., Weigel, R., Jurkat, T., Andreae, M. O., Wendisch, M., Pöschl, U., Voigt, C., Mahnke, C., Borrmann, S., Albrecht, R. I., Molleker, S., Vila, D. A., Machado, L. A. T., and Grulich, L.: Further evidence for CCN aerosol concentrations determining the height of warm rain and ice initiation in convective clouds over the Amazon basin, Atmos. Chem. Phys., 17, 14433–14456, https://doi.org/10.5194/acp-17-14433-2017, 2017.
Breon, F. M., Tanre, D., and Generoso, S.: Aerosol effect on cloud droplet size monitored from satellite, Science, 295, 834–838, https://doi.org/10.1126/science.1066434, 2002.
Brito, J., Rizzo, L. V., Morgan, W. T., Coe, H., Johnson, B., Haywood, J., Longo, K., Freitas, S., Andreae, M. O., and Artaxo, P.: Ground-based aerosol characterization during the South American Biomass Burning Analysis (SAMBBA) field experiment, Atmos. Chem. Phys., 14, 12069–12083, https://doi.org/10.5194/acp-14-12069-2014, 2014.
Carslaw, K. S., Lee, L. A., Reddington, C. L., Pringle, K. J., Rap, A., Forster, P. M., Mann, G. W., Spracklen, D. V., Woodhouse, M. T., Regayre, L. A., and Pierce, J. R.: Large contribution of natural aerosols to uncertainty in indirect forcing, Nature, 503, 67–71, https://doi.org/10.1038/nature12674, 2013.
Cecchini, M. A., Machado, L. A. T., Andreae, M. O., Martin, S. T., Albrecht, R. I., Artaxo, P., Barbosa, H. M. J., Borrmann, S., Fütterer, D., Jurkat, T., Mahnke, C., Minikin, A., Molleker, S., Pöhlker, M. L., Pöschl, U., Rosenfeld, D., Voigt, C., Weinzierl, B., and Wendisch, M.: Sensitivities of Amazonian clouds to aerosols and updraft speed, Atmos. Chem. Phys., 17, 10037–10050, https://doi.org/10.5194/acp-17-10037-2017, 2017.
Chang, D., Cheng, Y., Reutter, P., Trentmann, J., Burrows, S. M., Spichtinger, P., Nordmann, S., Andreae, M. O., Pöschl, U., and Su, H.: Comprehensive mapping and characteristic regimes of aerosol effects on the formation and evolution of pyro-convective clouds, Atmos. Chem. Phys., 15, 10325–10348, https://doi.org/10.5194/acp-15-10325-2015, 2015.
Chapman, E. G., Gustafson Jr., W. I., Easter, R. C., Barnard, J. C., Ghan, S. J., Pekour, M. S., and Fast, J. D.: Coupling aerosol-cloud-radiative processes in the WRF-Chem model: Investigating the radiative impact of elevated point sources, Atmos. Chem. Phys., 9, 945–964, https://doi.org/10.5194/acp-9-945-2009, 2009.
Charlson, R. J., Schwartz, S. E., Hales, J. M., Cess, R. D., Coakley, J. A., Jr., Hansen, J. E., and Hofmann, D. J.: Climate forcing by anthropogenic aerosols, Science, 255, 423–430, https://doi.org/10.1126/science.255.5043.423, 1992.
Cheng, Y. F., Wiedensohler, A., Eichler, H., Su, H., Gnauk, T., Brueggemann, E., Herrmann, H., Heintzenberg, J., Slanina, J., Tuch, T., Hu, M., and Zhang, Y. H.: Aerosol optical properties and related chemical apportionment at Xinken in Pearl River Delta of China, Atmos. Environ., 42, 6351–6372, https://doi.org/10.1016/j.atmosenv.2008.02.034, 2008a.
Cheng, Y. F., Wiedensohler, A., Eichler, H., Heintzenberg, J., Tesche, M., Ansmann, A., Wendisch, M., Su, H., Althausen, D., Herrmann, H., Gnauk, T., Bruggemann, E., Hu, M., and Zhang, Y. H.: Relative humidity dependence of aerosol optical properties and direct radiative forcing in the surface boundary layer at Xinken in Pearl River Delta of China: An observation based numerical study, Atmos. Environ., 42, 6373–6397, https://doi.org/10.1016/j.atmosenv.2008.04.009, 2008b.
Collier, S., Zhou, S., Onasch, T. B., Jaffe, D. A., Kleinman, L., Sedlacek, A. J., Briggs, N. L., Hee, J., Fortner, E., Shilling, J. E., Worsnop, D., Yokelson, R. J., Parworth, C., Ge, X., Xu, J., Butterfield, Z., Chand, D., Dubey, M. K., Pekour, M. S., Springston, S., and Zhang, Q.: Regional Influence of Aerosol Emissions from Wildfires Driven by Combustion Efficiency: Insights from the BBOP Campaign, Environ. Sci. Technol., 50, 8613–8622, doi:10.1021/acs.est.6b01617, 2016.
Cox, P. M., Harris, P. P., Huntingford, C., Betts, R. A., Collins, M., Jones, C. D., Jupp, T. E., Marengo, J. A., and Nobre, C. A.: Increasing risk of Amazonian drought due to decreasing aerosol pollution, Nature, 453, 212–217, https://doi.org/10.1038/nature06960, 2008.
Crutzen, P. J., and Andreae, M. O.: Biomass burning in the tropics: impact on atmospheric chemistry and biogeochemical cycles, Science, 250, 1669–1678, https://doi.org/10.1126/science.250.4988.1669, 1990.
Ding, A. J., Fu, C. B., Yang, X. Q., Sun, J. N., Petäjä, T., Kerminen, V.-M., Wang, T., Xie, Y., Herrmann, E., Zheng, L. F., Nie, W., Liu, Q., Wei, X. L., and Kulmala, M.: Intense atmospheric pollution modifies weather: a case of mixed biomass burning with fossil fuel combustion pollution in eastern China, Atmos. Chem. Phys., 13, 10545–10554, https://doi.org/10.5194/acp-13-10545-2013, 2013.
Dipu, S., Prabha, T. V., Pandithurai, G., Dudhia, J., Pfister, G., Rajesh, K., and Goswami, B. N.: Impact of elevated aerosol layer on the cloud macrophysical properties prior to monsoon onset, Atmos Environ, 70, 454-467, 10.1016/j.atmosenv.2012.12.036, 2013.
Ditas, J., Ma, N., Zhang, Y., Assmann, D., Neumaier, M., Riede, H., Karu, E., Williams, J., Scharffe, D., Wang, Q., Saturno, J., Schwarz, J. P., Katich, J. M., McMeeking, G. R., Zahn, A., Hermann, M., Brenninkmeijer, C. A. M., Andreae, M. O., Pöschl, U., Su, H., and Cheng, Y.: Strong impact of wildfires on the abundance and aging of black carbon in the lowermost stratosphere, P. Natl. Acad. Sci. USA, 115, E11595–E11603, https://doi.org/10.1073/pnas.1806868115, 2018.
Easter, R. C., Ghan, S. J., Zhang, Y., Saylor, R. D., Chapman, E. G., Laulainen, N. S., Abdul-Razzak, H., Leung, L. R., Bian, X. D., and Zaveri, R. A.: MIRAGE: Model description and evaluation of aerosols and trace gases, J. Geophys. Res.-Atmos., 109, D20210, https://doi.org/10.1029/2004jd004571, 2004.
Echalar, F., Artaxo, P., Martins, J. V., Yamasoe, M., Gerab, F., Maenhaut, W., and Holben, B.: Long-term monitoring of atmospheric aerosols in the Amazon Basin: Source identification and apportionment, J. Geophys. Res.-Atmos., 103, 31849–31864, https://doi.org/10.1029/98jd01749, 1998.
Emmons, L. K., Walters, S., Hess, P. G., Lamarque, J.-F., Pfister, G. G., Fillmore, D., Granier, C., Guenther, A., Kinnison, D., Laepple, T., Orlando, J., Tie, X., Tyndall, G., Wiedinmyer, C., Baughcum, S. L., and Kloster, S.: Description and evaluation of the Model for Ozone and Related chemical Tracers, version 4 (MOZART-4), Geosci. Model Dev., 3, 43–67, https://doi.org/10.5194/gmd-3-43-2010, 2010.
Fahey, K. M. and Pandis, S. N.: Optimizing model performance: variable size resolution in cloud chemistry modeling, Atmos. Environ., 35, 4471–4478, https://doi.org/10.1016/S1352-2310(01)00224-2, 2001.
Fan, J., Yuan, T., Comstock, J. M., Ghan, S., Khain, A., Leung, L. R., Li, Z., Martins, V. J., and Ovchinnikov, M.: Dominant role by vertical wind shear in regulating aerosol effects on deep convective clouds, J. Geophys. Res., 114, D22206, https://doi.org/10.1029/2009jd012352, 2009.
Fan, J., Rosenfeld, D., Zhang, Y., Giangrande, S. E., Li, Z., Machado, L. A. T., Martin, S. T., Yang, Y., Wang, J., Artaxo, P., Barbosa, H. M. J., Braga, R. C., Comstock, J. M., Feng, Z., Gao, W., Gomes, H. B., Mei, F., Pöhlker, C., Pöhlker, M. L., Pöschl, U., and de Souza, R. A. F.: Substantial convection and precipitation enhancements by ultrafine aerosol particles, Science, 359, 411–418, https://doi.org/10.1126/science.aan8461, 2018.
Fast, J. D., Gustafson, W. I., Easter, R. C., Zaveri, R. A., Barnard, J. C., Chapman, E. G., Grell, G. A., and Peckham, S. E.: Evolution of ozone, particulates, and aerosol direct radiative forcing in the vicinity of Houston using a fully coupled meteorology-chemistry-aerosol model, J. Geophys. Res., 111, D21305, https://doi.org/10.1029/2005jd006721, 2006.
Feingold, G., Jiang, H. L., and Harrington, J. Y.: On smoke suppression of clouds in Amazonia, Geophys. Res. Lett., 32, L02804, https://doi.org/10.1029/2004gl021369, 2005.
Fiedler, S., Stevens, B., and Mauritsen, T.: On the sensitivity of anthropogenic aerosol forcing to model-internal variability and parameterizing a Twomey effect, J. Adv. Model Earth. Sy., 9, 1325–1341, https://doi.org/10.1002/2017ms000932, 2017.
Freitas, S. R., Longo, K. M., Diasb, M. A. F. S., Diasb, P. L. S., Chatfield, R., Prins, E., Artaxo, P., Grell, G. A., and Recuero, F. S.: Monitoring the transport of biomass burning emissions in South America, Environ. Fluid Mech., 5, 135–167, https://doi.org/10.1007/s10652-005-0243-7, 2005.
Freitas, S. R., Longo, K. M., Chatfield, R., Latham, D., Silva Dias, M. A. F., Andreae, M. O., Prins, E., Santos, J. C., Gielow, R., and Carvalho Jr., J. A.: Including the sub-grid scale plume rise of vegetation fires in low resolution atmospheric transport models, Atmos. Chem. Phys., 7, 3385–3398, https://doi.org/10.5194/acp-7-3385-2007, 2007.
Gan, M. A., Kousky, V. E., and Ropelewski, C. F.: The South America monsoon circulation and its relationship to rainfall over west-central Brazil, J. Climate, 17, 47–66, https://doi.org/10.1175/1520-0442(2004)017<0047:Tsamca>2.0.Co;2, 2004.
Ge, C., Wang, J., and Reid, J. S.: Mesoscale modeling of smoke transport over the Southeast Asian Maritime Continent: coupling of smoke direct radiative effect below and above the low-level clouds, Atmos. Chem. Phys., 14, 159–174, https://doi.org/10.5194/acp-14-159-2014, 2014.
Ghan, S. J., Leung, L. R., Easter, R. C., and AbdulRazzak, K.: Prediction of cloud droplet number in a general circulation model, J. Geophys. Res.-Atmos., 102, 21777–21794, https://doi.org/10.1029/97jd01810, 1997.
Ghan, S. J., Liu, X., Easter, R. C., Zaveri, R., Rasch, P. J., Yoon, J. H., and Eaton, B.: Toward a Minimal Representation of Aerosols in Climate Models: Comparative Decomposition of Aerosol Direct, Semidirect, and Indirect Radiative Forcing, J Climate, 25, 6461–6476, 10.1175/Jcli-D-11-00650.1, 2012.
Gonçalves, W. A., Machado, L. A. T., and Kirstetter, P.-E.: Influence of biomass aerosol on precipitation over the Central Amazon: an observational study, Atmos. Chem. Phys., 15, 6789–6800, https://doi.org/10.5194/acp-15-6789-2015, 2015.
Grell, G., Freitas, S. R., Stuefer, M., and Fast, J.: Inclusion of biomass burning in WRF-Chem: impact of wildfires on weather forecasts, Atmos. Chem. Phys., 11, 5289–5303, https://doi.org/10.5194/acp-11-5289-2011, 2011.
Grell, G. A. and Devenyi, D.: A generalized approach to parameterizing convection combining ensemble and data assimilation techniques, Geophys. Res. Lett., 29, 8-1–38-4, https://doi.org/10.1029/2002gl015311, 2002.
Grell, G. A., Peckham, S. E., Schmitz, R., McKeen, S. A., Frost, G., Skamarock, W. C., and Eder, B.: Fully coupled ”online” chemistry within the WRF model, Atmos. Environ., 39, 6957–6975, https://doi.org/10.1016/j.atmosenv.2005.04.027, 2005.
Guenther, A., Karl, T., Harley, P., Wiedinmyer, C., Palmer, P. I., and Geron, C.: Estimates of global terrestrial isoprene emissions using MEGAN (Model of Emissions of Gases and Aerosols from Nature), Atmos. Chem. Phys., 6, 3181–3210, https://doi.org/10.5194/acp-6-3181-2006, 2006.
Hansen, J., Sato, M., and Ruedy, R.: Radiative forcing and climate response, J. Geophys. Res.-Atmos., 102, 6831–6864, https://doi.org/10.1029/96jd03436, 1997.
Hartmann, D. L., Ockert-Bell, M. E., and Michelsen, M. L.: The Effect of Cloud Type on Earth's Energy Balance: Global Analysis, J. Climate, 5, 1281–1304, https://doi.org/10.1175/1520-0442(1992)005<1281:teocto>2.0.co;2, 1992.
Holanda, B. A., Pöhlker, M. L., Walter, D., Saturno, J., Sörgel, M., Ditas, J., Ditas, F., Schulz, C., Franco, M. A., Wang, Q., Donth, T., Artaxo, P., Barbosa, H. M. J., Borrmann, S., Braga, R., Brito, J., Cheng, Y., Dollner, M., Kaiser, J. W., Klimach, T., Knote, C., Krüger, O. O., Fütterer, D., Lavrič, J. V., Ma, N., Machado, L. A. T., Ming, J., Morais, F. G., Paulsen, H., Sauer, D., Schlager, H., Schneider, J., Su, H., Weinzierl, B., Walser, A., Wendisch, M., Ziereis, H., Zöger, M., Pöschl, U., Andreae, M. O., and Pöhlker, C.: Influx of African biomass burning aerosol during the Amazonian dry season through layered transatlantic transport of black carbon-rich smoke, Atmos. Chem. Phys., 20, 4757–4785, https://doi.org/10.5194/acp-20-4757-2020, 2020.
Hong, S. Y.: A new stable boundary-layer mixing scheme and its impact on the simulated East Asian summer monsoon, Q. J. Roy. Meteor. Soc., 136, 1481–1496, https://doi.org/10.1002/qj.665, 2010.
Huang, X., Ding, A., Liu, L., Liu, Q., Ding, K., Niu, X., Nie, W., Xu, Z., Chi, X., Wang, M., Sun, J., Guo, W., and Fu, C.: Effects of aerosol–radiation interaction on precipitation during biomass-burning season in East China, Atmos. Chem. Phys., 16, 10063–10082, https://doi.org/10.5194/acp-16-10063-2016, 2016.
Huang, C.-C., Chen, S.-H., Lin, Y.-C., Earl, K., Matsui, T., Lee, H.-H., Tsai, I. C., Chen, J.-P., and Cheng, C.-T.: Impacts of Dust–Radiation versus Dust–Cloud Interactions on the Development of a Modeled Mesoscale Convective System over North Africa, Mon. Weather Rev., 147, 3301–3326, https://doi.org/10.1175/mwr-d-18-0459.1, 2019.
IPCC: Climate Change 2013: The Physical Science Basis. Contribution of Working Group I to the Fifth Assessment Report of the Intergovernmental Panel on Climate Change, edited by: Stocker, T. F., D. Qin, G.-K. Plattner, M. Tignor, S. K. Allen, J. Boschung, A. Nauels, Y. Xia, V. Bex and P. M. Midgley, Cambridge University Press, Cambridge, United Kingdom and New York, NY, USA, 1535 pp., https://doi.org/10.1017/CBO9781107415324, 2013.
Janssens-Maenhout, G., Crippa, M., Guizzardi, D., Dentener, F., Muntean, M., Pouliot, G., Keating, T., Zhang, Q., Kurokawa, J., Wankmüller, R., Denier van der Gon, H., Kuenen, J. J. P., Klimont, Z., Frost, G., Darras, S., Koffi, B., and Li, M.: HTAP_v2.2: a mosaic of regional and global emission grid maps for 2008 and 2010 to study hemispheric transport of air pollution, Atmos. Chem. Phys., 15, 11411–11432, https://doi.org/10.5194/acp-15-11411-2015, 2015.
Jiang, H. L. and Feingold, G.: Effect of aerosol on warm convective clouds: Aerosol-cloud-surface flux feedbacks in a new coupled large eddy model, J. Geophys. Res.-Atmos., 111, D01202, https://doi.org/10.1029/2005jd006138, 2006.
Jiang, J. H., Su, H., Huang, L., Wang, Y., Massie, S., Zhao, B., Omar, A., and Wang, Z.: Contrasting effects on deep convective clouds by different types of aerosols, Nat. Commun., 9, 3874, https://doi.org/10.1038/s41467-018-06280-4, 2018.
Johnson, B. T., Shine, K. P., and Forster, P. M.: The semi-direct aerosol effect: Impact of absorbing aerosols on marine stratocumulus, Q. J. Roy. Meteor. Soc., 130, 1407–1422, https://doi.org/10.1256/qj.03.61, 2004.
Kaufman, Y. J. and Fraser, R. S.: The effect of smoke particles on clouds and climate forcing, Science, 277, 1636–1639, https://doi.org/10.1126/science.277.5332.1636, 1997.
Kaufman, Y. J. and Koren, I.: Smoke and pollution aerosol effect on cloud cover, Science, 313, 655–658, https://doi.org/10.1126/science.1126232, 2006.
Khain, A., Rosenfeld, D., and Pokrovsky, A.: Aerosol impact on the dynamics and microphysics of deep convective clouds, Q. J. Roy. Meteor. Soc., 131, 2639–2663, https://doi.org/10.1256/qj.04.62, 2005.
Koch, D. and Del Genio, A. D.: Black carbon semi-direct effects on cloud cover: review and synthesis, Atmos. Chem. Phys., 10, 7685–7696, https://doi.org/10.5194/acp-10-7685-2010, 2010.
Kolusu, S. R., Marsham, J. H., Mulcahy, J., Johnson, B., Dunning, C., Bush, M., and Spracklen, D. V.: Impacts of Amazonia biomass burning aerosols assessed from short-range weather forecasts, Atmos. Chem. Phys., 15, 12251–12266, https://doi.org/10.5194/acp-15-12251-2015, 2015.
Koren, I., Kaufman, Y. J., Remer, L. A., and Martins, J. V.: Measurement of the effect of Amazon smoke on inhibition of cloud formation, Science, 303, 1342–1345, https://doi.org/10.1126/science.1089424, 2004.
Koren, I., Martins, J. V., Remer, L. A., and Afargan, H.: Smoke invigoration versus inhibition of clouds over the Amazon, Science, 321, 946–949, https://doi.org/10.1126/science.1159185, 2008.
Koren, I., Remer, L. A., Altaratz, O., Martins, J. V., and Davidi, A.: Aerosol-induced changes of convective cloud anvils produce strong climate warming, Atmos. Chem. Phys., 10, 5001–5010, https://doi.org/10.5194/acp-10-5001-2010, 2010.
Lee, H.-H., Chen, S.-H., Kleeman, M. J., Zhang, H., DeNero, S. P., and Joe, D. K.: Implementation of warm-cloud processes in a source-oriented WRF/Chem model to study the effect of aerosol mixing state on fog formation in the Central Valley of California, Atmos. Chem. Phys., 16, 8353–8374, https://doi.org/10.5194/acp-16-8353-2016, 2016.
Lee, S. S., Kim, B. G., Lee, C., Yum, S. S., and Posselt, D.: Effect of aerosol pollution on clouds and its dependence on precipitation intensity, Clim. Dynam., 42, 557–577, https://doi.org/10.1007/s00382-013-1898-2, 2014.
Lee, S. S., Li, Z., Mok, J., Ahn, M.-H., Kim, B.-G., Choi, Y.-S., Jung, C.-H., and Yoo, H. L.: Interactions between aerosol absorption, thermodynamics, dynamics, and microphysics and their impacts on a multiple-cloud system, Clim. Dynam., 49, 3905–3921, https://doi.org/10.1007/s00382-017-3552-x, 2017.
Li, Z. Q.: Influence of absorbing aerosols on the inference of solar surface radiation budget and cloud absorption, J. Climate, 11, 5–17, https://doi.org/10.1175/1520-0442(1998)011<0005:Ioaaot>2.0.Co;2, 1998.
Lin, J. C., Matsui, T., Pielke, R. A., and Kummerow, C.: Effects of biomass-burning-derived aerosols on precipitation and clouds in the Amazon Basin: a satellite-based empirical study, J. Geophys. Res.-Atmos., 111, D19204, https://doi.org/10.1029/2005jd006884, 2006.
Lin, Y., Wang, Y., Pan, B. W., Hu, J. X., Liu, Y. G., and Zhang, R. Y.: Distinct Impacts of Aerosols on an Evolving Continental Cloud Complex during the RACORO Field Campaign, J. Atmos. Sci., 73, 3681–3700, https://doi.org/10.1175/Jas-D-15-0361.1, 2016.
Lin, Y. L., Farley, R. D., and Orville, H. D.: Bulk Parameterization of the Snow Field in a Cloud Model, J. Clim. Appl. Meteorol., 22, 1065–1092, https://doi.org/10.1175/1520-0450(1983)022<1065:Bpotsf>2.0.Co;2, 1983.
Liu, L.: Model results related to Liu et al 2020, Max Planck Society, https://dx.doi.org/10.17617/3.4m, 2020.
Liu, Y. G., Daum, P. H., and McGraw, R. L.: Size truncation effect, threshold behavior, and a new type of autoconversion parameterization, Geophys. Res. Lett., 32, L11811, https://doi.org/10.1029/2005gl022636, 2005.
Martin, S. T., Andreae, M. O., Artaxo, P., Baumgardner, D., Chen, Q., Goldstein, A. H., Guenther, A., Heald, C. L., Mayol-Bracero, O. L., McMurry, P. H., Pauliquevis, T., Pöschl, U., Prather, K. A., Roberts, G. C., Saleska, S. R., Dias, M. A. S., Spracklen, D. V., Swietlicki, E., and Trebs, I.: Sources and Properties of Amazonian Aerosol Particles, Rev. Geophys., 48, RG2002, https://doi.org/10.1029/2008rg000280, 2010.
Martins, J. A., Dias, M. A. F. S., and Goncalves, F. L. T.: Impact of biomass burning aerosols on precipitation in the Amazon: A modeling case study, J. Geophys. Res.-Atmos., 114, D02207, https://doi.org/10.1029/2007jd009587, 2009.
Martins, J. V., Artaxo, P., Liousse, C., Reid, J. S., Hobbs, P. V., and Kaufman, Y. J.: Effects of black carbon content, particle size, and mixing on light absorption by aerosols from biomass burning in Brazil, J. Geophys. Res.-Atmos., 103, 32041–32050, https://doi.org/10.1029/98jd02593, 1998.
McCluskey, C. S., DeMott, P. J., Prenni, A. J., Levin, E. J. T., McMeeking, G. R., Sullivan, A. P., Hill, T. C. J., Nakao, S., Carrico, C. M., and Kreidenweis, S. M.: Characteristics of atmospheric ice nucleating particles associated with biomass burning in the US: Prescribed burns and wildfires, J. Geophys. Res.-Atmos., 119, https://doi.org/10.1002/2014jd021980, 2014.
Mlawer, E. J., Taubman, S. J., Brown, P. D., Iacono, M. J., and Clough, S. A.: Radiative transfer for inhomogeneous atmospheres: RRTM, a validated correlated-k model for the longwave, J. Geophys. Res.-Atmos., 102, 16663–16682, https://doi.org/10.1029/97jd00237, 1997.
Otte, T. L., Nolte, C. G., Otte, M. J., and Bowden, J. H.: Does Nudging Squelch the Extremes in Regional Climate Modeling?, J. Climate, 25, 7046–7066, https://doi.org/10.1175/Jcli-D-12-00048.1, 2012.
Peng, J., Hu, M., Guo, S., Du, Z., Zheng, J., Shang, D., Levy Zamora, M., Zeng, L., Shao, M., Wu, Y. S., Zheng, J., Wang, Y., Glen, C. R., Collins, D. R., Molina, M. J., and Zhang, R.: Markedly enhanced absorption and direct radiative forcing of black carbon under polluted urban environments, P. Natl. Acad. Sci. USA, 113, 4266–4271, https://doi.org/10.1073/pnas.1602310113, 2016
Pincus, R., Barker, H. W., and Morcrette, J. J.: A fast, flexible, approximate technique for computing radiative transfer in inhomogeneous cloud fields, J. Geophys. Res.-Atmos., 108, 4376, https://doi.org/10.1029/2002jd003322, 2003.
Pöhlker, C., Walter, D., Paulsen, H., Könemann, T., Rodríguez-Caballero, E., Moran-Zuloaga, D., Brito, J., Carbone, S., Degrendele, C., Després, V. R., Ditas, F., Holanda, B. A., Kaiser, J. W., Lammel, G., Lavrič, J. V., Ming, J., Pickersgill, D., Pöhlker, M. L., Praß, M., Löbs, N., Saturno, J., Sörgel, M., Wang, Q., Weber, B., Wolff, S., Artaxo, P., Pöschl, U., and Andreae, M. O.: Land cover and its transformation in the backward trajectory footprint region of the Amazon Tall Tower Observatory, Atmos. Chem. Phys., 19, 8425–8470, https://doi.org/10.5194/acp-19-8425-2019, 2019.
Pöhlker, M. L., Pöhlker, C., Ditas, F., Klimach, T., Hrabe de Angelis, I., Araújo, A., Brito, J., Carbone, S., Cheng, Y., Chi, X., Ditz, R., Gunthe, S. S., Kesselmeier, J., Könemann, T., Lavrič, J. V., Martin, S. T., Mikhailov, E., Moran-Zuloaga, D., Rose, D., Saturno, J., Su, H., Thalman, R., Walter, D., Wang, J., Wolff, S., Barbosa, H. M. J., Artaxo, P., Andreae, M. O., and Pöschl, U.: Long-term observations of cloud condensation nuclei in the Amazon rain forest – Part 1: Aerosol size distribution, hygroscopicity, and new model parametrizations for CCN prediction, Atmos. Chem. Phys., 16, 15709–15740, https://doi.org/10.5194/acp-16-15709-2016, 2016.
Procopio, A. S., Artaxo, P., Kaufman, Y. J., Remer, L. A., Schafer, J. S., and Holben, B. N.: Multiyear analysis of amazonian biomass burning smoke radiative forcing of climate, Geophys. Res. Lett., 31, L03108, https://doi.org/10.1029/2003gl018646, 2004.
Ramachandran, S., and Kedia, S.: Black carbon aerosols over an urban region: Radiative forcing and climate impact, J. Geophys. Res., 115, D10202, https://doi.org/10.1029/2009jd013560, 2010.
Ramanathan, V., Crutzen, P. J., Kiehl, J. T., and Rosenfeld, D.: Aerosols, climate, and the hydrological cycle, Science, 294, 2119–2124, https://doi.org/10.1126/science.1064034, 2001.
Reddington, C. L., Butt, E. W., Ridley, D. A., Artaxo, P., Morgan, W. T., Coe, H., and Spracklen, D. V.: Air quality and human health improvements from reductions in deforestation-related fire in Brazil, Nat. Geosci., 8, 768–771, https://doi.org/10.1038/Ngeo2535, 2015.
Reid, J. S., Koppmann, R., Eck, T. F., and Eleuterio, D. P.: A review of biomass burning emissions part II: intensive physical properties of biomass burning particles, Atmos. Chem. Phys., 5, 799–825, https://doi.org/10.5194/acp-5-799-2005, 2005.
Reutter, P., Su, H., Trentmann, J., Simmel, M., Rose, D., Gunthe, S. S., Wernli, H., Andreae, M. O., and Pöschl, U.: Aerosol- and updraft-limited regimes of cloud droplet formation: influence of particle number, size and hygroscopicity on the activation of cloud condensation nuclei (CCN), Atmos. Chem. Phys., 9, 7067–7080, https://doi.org/10.5194/acp-9-7067-2009, 2009.
Reutter, P., Trentmann, J., Seifert, A., Neis, P., Su, H., Chang, D., Herzog, M., Wernli, H., Andreae, M. O., and Pöschl, U.: 3-D model simulations of dynamical and microphysical interactions in pyroconvective clouds under idealized conditions, Atmos. Chem. Phys., 14, 7573–7583, https://doi.org/10.5194/acp-14-7573-2014, 2014.
Roberts, G. C., Nenes, A., Seinfeld, J. H., and Andreae, M. O.: Impact of biomass burning on cloud properties in the Amazon Basin, J. Geophys. Res.-Atmos., 108, 4062, https://doi.org/10.1029/2001jd000985, 2003.
Roberts, M. C., Andreae, M. O., Zhou, J. C., and Artaxo, P.: Cloud condensation nuclei in the Amazon Basin: ”Marine” conditions over a continent?, Geophys. Res. Lett., 28, 2807–2810, https://doi.org/10.1029/2000gl012585, 2001.
Rosenfeld, D.: Suppression of rain and snow by urban and industrial air pollution, Science, 287, 1793–1796, 2000.
Rosenfeld, D., Lohmann, U., Raga, G. B., O'Dowd, C. D., Kulmala, M., Fuzzi, S., Reissell, A., and Andreae, M. O.: Flood or drought: How do aerosols affect precipitation?, Science, 321, 1309–1313, https://doi.org/10.1126/science.1160606, 2008.
Rutledge, S. A. H., P. V: The Mesoscale and Microscale Structure and Organization of Clouds and Precipitation in Midlatitude Cyclones. XII: A Diagnostic Modeling Study of Precipitation Development in Narrow Cold-Frontal Rainbands, J. Atmos. Sci., 20, 2949–2972, 1984.
Samset, B. H. and Myhre, G.: Vertical dependence of black carbon, sulphate and biomass burning aerosol radiative forcing, Geophys. Res. Lett., 38, L24802, https://doi.org/10.1029/2011gl049697, 2011.
Sena, E. T., Artaxo, P., and Correia, A. L.: Spatial variability of the direct radiative forcing of biomass burning aerosols and the effects of land use change in Amazonia, Atmos. Chem. Phys., 13, 1261–1275, https://doi.org/10.5194/acp-13-1261-2013, 2013.
Sengupta, S. K., Welch, R. M., Navar, M. S., Berendes, T. A., and Chen, D. W.: Cumulus Cloud Field Morphology and Spatial Patterns Derived from High Spatial-Resolution Landsat Imagery, J. Appl. Meteorol., 29, 1245–1267, https://doi.org/10.1175/1520-0450(1990)029<1245:Ccfmas>2.0.Co;2, 1990.
Setzer, A. W. and Pereira, M. C.: Amazonia Biomass Burnings in 1987 and an Estimate of Their Tropospheric Emissions, Ambio., 20, 19–22, 1991.
Shi, J. J., Matsui, T., Tao, W. K., Tan, Q., Peters-Lidard, C., Chin, M., Pickering, K., Guy, N., Lang, S., and Kemp, E. M.: Implementation of an aerosol-cloud-microphysics-radiation coupling into the NASA unified WRF: Simulation results for the 6–7 August 2006 AMMA special observing period, Q. J. Roy. Meteor. Soc., 140, 2158–2175, https://doi.org/10.1002/qj.2286, 2014.
Shrivastava, M., Cappa, C. D., Fan, J. W., Goldstein, A. H., Guenther, A. B., Jimenez, J. L., Kuang, C., Laskin, A., Martin, S. T., Ng, N. L., Petaja, T., Pierce, J. R., Rasch, P. J., Roldin, P., Seinfeld, J. H., Shilling, J., Smith, J. N., Thornton, J. A., Volkamer, R., Wang, J., Worsnop, D. R., Zaveri, R. A., Zelenyuk, A., and Zhang, Q.: Recent advances in understanding secondary organic aerosol: Implications for global climate forcing, Rev. Geophys., 55, 509–559, https://doi.org/10.1002/2016rg000540, 2017.
Shrivastava, M., Andreae, M. O., Artaxo, P., Barbosa, H. M. J., Berg, L. K., Brito, J., Ching, J., Easter, R. C., Fan, J., Fast, J. D., Feng, Z., Fuentes, J. D., Glasius, M., Goldstein, A. H., Alves, E. G., Gomes, H., Gu, D., Guenther, A., Jathar, S. H., Kim, S., Liu, Y., Lou, S., Martin, S. T., McNeill, V. F., Medeiros, A., de Sa, S. S., Shilling, J. E., Springston, S. R., Souza, R. A. F., Thornton, J. A., Isaacman-VanWertz, G., Yee, L. D., Ynoue, R., Zaveri, R. A., Zelenyuk, A., and Zhao, C.: Urban pollution greatly enhances formation of natural aerosols over the Amazon rainforest, Nat. Commun., 10, 1046, https://doi.org/10.1038/s41467-019-08909-4, 2019.
Smirnova, T. G., Brown, J. M., and Benjamin, S. G.: Performance of different soil model configurations in simulating ground surface temperature and surface fluxes, Mon. Weather Rev., 125, 1870–1884, https://doi.org/10.1175/1520-0493(1997)125<1870:Podsmc>2.0.Co;2, 1997.
Smirnova, T. G., Brown, J. M., Benjamin, S. G., and Kim, D.: Parameterization of cold-season processes in the MAPS land-surface scheme, J. Geophys. Res.-Atmos., 105, 4077–4086, https://doi.org/10.1029/1999jd901047, 2000.
Spracklen, D. V., Carslaw, K. S., Pöschl, U., Rap, A., and Forster, P. M.: Global cloud condensation nuclei influenced by carbonaceous combustion aerosol, Atmos. Chem. Phys., 11, 9067–9087, https://doi.org/10.5194/acp-11-9067-2011, 2011.
Stevens, B. and Feingold, G.: Untangling aerosol effects on clouds and precipitation in a buffered system, Nature, 461, 607–613, https://doi.org/10.1038/nature08281, 2009.
Streets, D. G.: Dissecting future aerosol emissions: Warming tendencies and mitigation opportunities, Climatic Change, 81, 313–330, https://doi.org/10.1007/s10584-006-9112-8, 2007.
Su, H., Jiang, J. H., Lu, X. H., Penner, J. E., Read, W. G., Massie, S., Schoeberl, M. R., Colarco, P., Livesey, N. J., and Santee, M. L.: Observed Increase of TTL Temperature and Water Vapor in Polluted Clouds over Asia, J. Climate, 24, 2728–2736, https://doi.org/10.1175/2010jcli3749.1, 2011.
Takahama, S., Schwartz, R. E., Russell, L. M., Macdonald, A. M., Sharma, S., and Leaitch, W. R.: Organic functional groups in aerosol particles from burning and non-burning forest emissions at a high-elevation mountain site, Atmos. Chem. Phys., 11, 6367–6386, https://doi.org/10.5194/acp-11-6367-2011, 2011.
Tao, W. K., Li, X. W., Khain, A., Matsui, T., Lang, S., and Simpson, J.: Role of atmospheric aerosol concentration on deep convective precipitation: Cloud-resolving model simulations, J. Geophys. Res.-Atmos., 112, D24s18, https://doi.org/10.1029/2007jd008728, 2007.
Ten Hoeve, J. E., Jacobson, M. Z., and Remer, L. A.: Comparing results from a physical model with satellite and in situ observations to determine whether biomass burning aerosols over the Amazon brighten or burn off clouds, J. Geophys. Res.-Atmos., 117, D08203, https://doi.org/10.1029/2011jd016856, 2012.
Thornhill, G. D., Ryder, C. L., Highwood, E. J., Shaffrey, L. C., and Johnson, B. T.: The effect of South American biomass burning aerosol emissions on the regional climate, Atmos. Chem. Phys., 18, 5321–5342, https://doi.org/10.5194/acp-18-5321-2018, 2018.
Twomey, S.: Influence of Pollution on Shortwave Albedo of Clouds, J. Atmos. Sci., 34, 1149–1152, https://doi.org/10.1175/1520-0469(1977)034<1149:Tiopot>2.0.Co;2, 1977.
Wang, C.: A modeling study of the response of tropical deep convection to the increase of cloud condensation nuclei concentration: 1. Dynamics and microphysics, Journal of Geophysical Research, 110, D21211, 10.1029/2004jd005720, 2005.
Wang, Y., Khalizov, A., Levy, M., and Zhang, R. Y.: New Directions: Light absorbing aerosols and their atmospheric impacts, Atmos. Environ., 81, 713–715, https://doi.org/10.1016/j.atmosenv.2013.09.034, 2013.
Wang, Y., Ma, P. L., Peng, J. F., Zhang, R. Y., Jiang, J. H., Easter, R. C., and Yung, Y. L.: Constraining Aging Processes of Black Carbon in the Community Atmosphere Model Using Environmental Chamber Measurements, J. Adv. Model Earth Sy., 10, 2514–2526, https://doi.org/10.1029/2018ms001387, 2018
Wiedinmyer, C., Akagi, S. K., Yokelson, R. J., Emmons, L. K., Al-Saadi, J. A., Orlando, J. J., and Soja, A. J.: The Fire INventory from NCAR (FINN): a high resolution global model to estimate the emissions from open burning, Geosci. Model Dev., 4, 625–641, https://doi.org/10.5194/gmd-4-625-2011, 2011.
Wild, O., Zhu, X., and Prather, M. J.: Fast-j: Accurate simulation of in- and below-cloud photolysis in tropospheric chemical models, J. Atmos. Chem., 37, 245–282, https://doi.org/10.1023/A:1006415919030, 2000.
Williams, E., Rosenfeld, D., Madden, N., Gerlach, J., Gears, N., Atkinson, L., Dunnemann, N., Frostrom, G., Antonio, M., Biazon, B., Camargo, R., Franca, H., Gomes, A., Lima, M., Machado, R., Manhaes, S., Nachtigall, L., Piva, H., Quintiliano, W., Machado, L., Artaxo, P., Roberts, G., Renno, N., Blakeslee, R., Bailey, J., Boccippio, D., Betts, A., Wolff, D., Roy, B., Halverson, J., Rickenbach, T., Fuentes, J., and Avelino, E.: Contrasting convective regimes over the Amazon: Implications for cloud electrification, J. Geophys. Res.-Atmos., 107, 8082, https://doi.org/10.1029/2001jd000380, 2002.
Wu, L., Su, H., and Jiang, J. H.: Regional simulations of deep convection and biomass burning over South America: 2. Biomass burning aerosol effects on clouds and precipitation, J. Geophys. Res., 116, D17209, https://doi.org/10.1029/2011jd016106, 2011.
Ye, X. Y., Cao, Q. M., Jiang, B. L., and Lin, W. S.: Numerical Simulation of the Effect of Cloud Condensation Nuclei Concentration on the Microphysical Processes in Typhoon Usagi, Adv. Meteorol., 2019, 8293062, https://doi.org/10.1155/2019/8293062, 2019.
Yokelson, R. J., Crounse, J. D., DeCarlo, P. F., Karl, T., Urbanski, S., Atlas, E., Campos, T., Shinozuka, Y., Kapustin, V., Clarke, A. D., Weinheimer, A., Knapp, D. J., Montzka, D. D., Holloway, J., Weibring, P., Flocke, F., Zheng, W., Toohey, D., Wennberg, P. O., Wiedinmyer, C., Mauldin, L., Fried, A., Richter, D., Walega, J., Jimenez, J. L., Adachi, K., Buseck, P. R., Hall, S. R., and Shetter, R.: Emissions from biomass burning in the Yucatan, Atmos. Chem. Phys., 9, 5785–5812, https://doi.org/10.5194/acp-9-5785-2009, 2009.
Yu, H. B., Liu, S. C., and Dickinson, R. E.: Radiative effects of aerosols on the evolution of the atmospheric boundary layer, J. Geophys. Res.-Atmos., 107, 4142, https://doi.org/10.1029/2001jd000754, 2002.
Zaveri, R. A. and Peters, L. K.: A new lumped structure photochemical mechanism for large-scale applications, J. Geophys. Res.-Atmos., 104, 30387–30415, https://doi.org/10.1029/1999jd900876, 1999.
Zaveri, R. A., Easter, R. C., Fast, J. D., and Peters, L. K.: Model for Simulating Aerosol Interactions and Chemistry (MOSAIC), J. Geophys. Res.-Atmos., 113, D13204, https://doi.org/10.1029/2007jd008782, 2008.
Zhang, Y., Fu, R., Yu, H. B., Dickinson, R. E., Juarez, R. N., Chin, M., and Wang, H.: A regional climate model study of how biomass burning aerosol impacts land-atmosphere interactions over the Amazon, J. Geophys. Res.-Atmos., 113, D14s15, https://doi.org/10.1029/2007jd009449, 2008.
Zhang, Y., Fu, R., Yu, H. B., Qian, Y., Dickinson, R., Dias, M. A. F. S., Dias, P. L. D., and Fernandes, K.: Impact of biomass burning aerosol on the monsoon circulation transition over Amazonia, Geophys. Res. Lett., 36, L10814, https://doi.org/10.1029/2009gl037180, 2009.
Zhao, C., Leung, L. R., Easter, R., Hand, J., and Avise, J.: Characterization of speciated aerosol direct radiative forcing over California, J. Geophys. Res.-Atmos., 118, 2372–2388, https://doi.org/10.1029/2012jd018364, 2013.