the Creative Commons Attribution 4.0 License.
the Creative Commons Attribution 4.0 License.
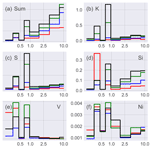
Investigating size-segregated sources of elemental composition of particulate matter in the South China Sea during the 2011 Vasco cruise
Miguel Ricardo A. Hilario
Melliza T. Cruz
Maria Obiminda L. Cambaliza
Jeffrey S. Reid
Peng Xian
James B. Simpas
Nofel D. Lagrosas
Sherdon Niño Y. Uy
Steve Cliff
Yongjing Zhao
The South China Sea (SCS) is a receptor of numerous natural and anthropogenic aerosol species from throughout greater Asia. A combination of several developing countries, archipelagic and peninsular terrain, a strong Asian monsoon climate, and a host of multi-scale meteorological phenomena make the SCS one of the most complex aerosol–meteorological systems in the world. However, aside from the well-known biomass burning emissions from Indonesia and Borneo, the current understanding of aerosol sources is limited, especially in remote marine environments. In September 2011, a 2-week research cruise was conducted near Palawan, Philippines, to sample the remote SCS environment. Size-segregated aerosol data were collected using a Davis Rotating Uniform size-cut Monitor (DRUM) sampler and analyzed for concentrations of 28 elements measured via X-ray fluorescence (XRF). Positive matrix factorization (PMF) was performed separately on the coarse, fine, and ultrafine size ranges to determine possible sources and their contributions to the total elemental particulate matter mass. The PMF analysis resolved six sources across the three size ranges: biomass burning, oil combustion, soil dust, a crustal–marine mixed source, sea spray, and fly ash. Additionally, size distribution plots, time series plots, back trajectories and satellite data were used in interpreting factors. The multi-technique source apportionment revealed the presence of biogenic sources such as soil dust, sea spray, and a crustal–marine mixed source. Anthropogenic sources were also identified: biomass burning, oil combustion, and fly ash. Mass size distributions showed elevated aerosol concentrations towards the end of the sampling period, which coincided with a shift of air mass back trajectories to southern Kalimantan. Covariance between coarse-mode soil dust and fine-mode biomass burning aerosols were observed. Agreement between the PMF and the linear regression analyses indicates that the PMF solution is robust. While biomass burning is indeed a key source of aerosol, this study shows the presence of other important sources in the SCS. Identifying these sources is not only key for characterizing the chemical profile of the SCS but, by improving our picture of aerosol sources in the region, also a step forward in developing our understanding of aerosol–meteorology feedbacks in this complex environment.
- Article
(9930 KB) -
Supplement
(1173 KB) - BibTeX
- EndNote
In the midst of several developing countries, the South China Sea (SCS) is a receptor for a multitude of natural and anthropogenic sources of aerosol. At the same time, the region exhibits some of the world's most complicated meteorology due to its archipelagic and peninsular terrain and strong Asian monsoon climate. Thus, the SCS hosts one of the world's most complex and sensitive composition and climate regimes (Balasubramanian et al., 2003; Yusuf and Francisco, 2009; Atwood et al., 2013a, b; Reid et al., 2012, 2013, 2015). It is known to be impacted not only by dust storms and industrial pollution from China (Wang et al., 2011; Atwood et al., 2013a) but also by biomass burning emissions from the Maritime Continent (Balasubramanian et al., 2003; Lin et al., 2007; Cohen et al., 2010a, b; Wang et al., 2011; Reid et al., 2013, 2015, 2016). The transport of such emissions is enabled by the long atmospheric residence times of fine particles (Cohen et al., 2010a), potentially creating regional and global concerns through their effects on radiative forcing (Nakajima et al., 2007; Boucher et al., 2013; Lin et al., 2014; Ge et al., 2014) and cloud properties (Sorooshian et al., 2009; Lee et al., 2012; Boucher et al., 2013; Ross et al., 2018).
Highlighting the unique combination of terrain and sea that feeds into the complexity of the meteorological environment of the region, Reid et al. (2012) and Xian et al. (2013) posed the long-range hypothesis that monsoonal flows and higher-frequency meteorological phenomena are a major factor in seasonal aerosol dispersion. Biomass burning plumes are known to cause severe haze episodes due to these monsoonal flows, raising concentrations of particulate matter (PM) to impact cloud physics and, in some cases, to dangerous air quality levels across large areas, particularly in association with positive phases of the El Niño–Southern Oscillation (ENSO) (Engling et al., 2014; Fujii et al., 2015). Likewise, biomass burning is a significant contributor to the region's cloud condensation nuclei (CCN) budget in all years, as are the region's significant anthropogenic emissions (Balasubramanian et al., 2003; Field et al., 2008; Reid et al., 2012, 2013, 2015, 2016; Atwood et al., 2017).
Partly due to the emphasis on dramatic biomass burning as the primary source of aerosol particles in the region, the contributions of other regional sources are not well understood or perhaps underappreciated. As the SCS is host to major population centers, industry, major ports, and coal and oil combustion are expected to be an important regional source of aerosol particles in the Maritime Continent (MC). Coarse-mode dust and biogenic particles may also play a role as ice nuclei (O'Sullivan et al., 2014), as biomass burning plumes are known to entrain such particles (Reid et al., 1994, 2005; Schlosser et al., 2017). As such, a network of interacting sources exists in the region surrounding the SCS, wherein aerosol particles mix during transport and complicate source apportionment. Understanding the nature of sources in the remote MC and their contributions is key to characterizing the aerosol environment in the SCS and its relationship with cloud behavior and precipitation patterns in the region; this is particularly true given the higher sensitivity of clouds to particle perturbations at lower concentrations. However, the source apportionment of aerosol particles is complicated by their complex chemistry and interactions with the marine environment (Atwood et al., 2013a, 2017).
As part of the Seven SouthEast Asian Studies program (7-SEAS), a research cruise (Reid et al., 2015) was conducted in late September 2011 on board the Philippine-flagged M/Y Vasco in the vicinity of the northern Palawan archipelago. The goal of this cruise was to observe the behavior of aerosol particles in the SCS and to test the transport hypothesis proposed in Reid et al. (2012) that the Philippines is a long-range receptor of aerosol species transported across the SCS during the Asian summer monsoon from Borneo, Sumatra, and the Malay Peninsula. In particular, the cruise aimed to observe that emissions from the Maritime Continent were reaching the southwest monsoon trough. The Palawan archipelago is a good receptor site for regional emissions due to its largely rural settlements and its location upwind relative to the rest of the Philippines. The sampling period coincided with the passage of one tropical storm and two tropical cyclones (TCs). Of particular importance is the passage of TC Nesat beginning on 26 September 2011, as TC inflow arms are known to cause abrupt changes in regional flows.
As part of the 2011 Vasco cruise, particulate matter was collected using a size-segregated Davis Rotating Uniform size-cut Monitor (DRUM) impactor analyzed for elemental composition. While Reid et al. (2015) noted the presence of smoke plumes in two episodes during the cruise, their initial analysis of the region's atmospheric chemistry also suggested the events were a mix of biomass burning and oil or shipping emissions, due to elevated levels of vanadium. Additionally, differences in elemental ratios, mass fractions, and back trajectory origins between the two events support the presence of other sources besides biomass burning. From the initial analysis of aerosol chemistry presented by Reid et al. (2015), this study aims to identify aerosol sources in the SCS, highlight the source variability present in the region, and further develop the current understanding of the effect of regional meteorological phenomena on aerosol dispersion. The paper shows that, although biomass burning is a major source of aerosols in the SCS, anthropogenic sources such as oil combustion also play an important role in the chemical profile of the region. As we report, soil transport was observed as well.
In this paper we expand on the original 2011 Vasco cruise analysis to quantitatively apportion sampled biomass burning and anthropogenic aerosol species. Positive matrix factorization (PMF) was performed on size-segregated, elemental PM to detect possible size-specific sources (Han et al., 2006; van Pinxteren et al., 2016). Indeed, the relationship between the aerodynamic diameter of a particle and its source has been well-established in the literature (Reid et al., 1994; Balasubramanian et al., 2003; Han et al., 2006; Lestari et al., 2009; Wimolwattanapun et al., 2011; Santoso et al., 2011; Karanasiou et al., 2009; Seneviratne et al., 2010; Atwood et al., 2013a; Lin et al., 2015; Cahill et al., 2016). Aerosol factors and characteristics were then used to spawn back trajectories to identify individual island emissions areas.
2.1 Overall cruise sampling and environment
A general overview of the 2011 cruise can be found in Reid et al. (2015), and a brief summary is provided here. Sampling was conducted around the Palawan archipelago, an island chain located at the southwestern edge of the Philippines in between the SCS and the Sulu Sea. Sampling was performed between Manila and the northern tip of Palawan Island on board the M/Y Vasco, which left Manila Bay on 17 September 2011 and returned on 30 September 2011 (Fig. 1). The majority of samples were collected around the areas of El Nido and Malampaya Sound (111.1∘ N, 119.3∘ E), where the vessel was stationed from 21 to 28 September. The largely rural population of Palawan made it an ideal receptor for regional rather than local emissions.
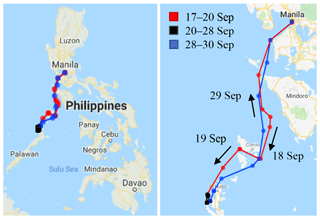
Figure 1Path taken by the M/Y Vasco on 17–20 September (red), 20–28 September (black), and 28–30 September (blue). The majority of sampling was done at the northern end of Palawan island. Image courtesy of Google Maps (map data ©2018 Google).
The cruise was conducted at the end of the Asian summer monsoon, which usually lasts from June through September (Loo et al., 2014; Chang et al., 2005). The Asian monsoon is caused by the annual progress of the sun and asymmetrical heating of air masses due to the complex terrain of Southeast Asia (Chang et al., 2005). The campaign coincided with the peak burning season in southern Kalimantan and southern Sumatra, which have been measured to be the highest emitters of biomass burning plumes in the MC (Reid et al., 2012). As the southwest monsoon is characterized by winds traveling southwest to northeast, Reid et al. (2015) proposed that the Philippines was an excellent receptor for regional emissions from the MC.
Although 2011 was a moderate La Niña year, it was noted that fire activity and precipitation levels resembled a neutral year (Reid et al., 2015). The cruise took place when the Madden-Julian Oscillation (MJO) was transitioning from the wet phase to the dry phase, which is expected to enhance burning activity and transport. With the passage of tropical cyclones (TCs), significant aerosol events were observed as propagating across the region.
Reid et al. (2015) described three tropical events that occurred during the cruise, specifically Tropical Storm (TS) Haitang, TC Nesat, and Super Typhoon Nalgae. The presence of inflow arms in the SCS has been suggested to affect the aerosol environment by bringing more MC air into the region (Reid et al., 2015). The passage of Nesat was observed to abruptly affect air mass trajectories, coinciding with an enhancement of several elements during the last 2 d of the cruise.
Figure 2 shows the evolution of the meteorological environment over the cruise period with comparisons between aerosol optical depth (AOD) derived from the Moderate Resolution Imaging Spectroradiometer (MODIS) on board the Terra and Aqua satellites, back trajectories from NOAA Hybrid Single Particle Lagrangian Integrated Trajectory Model (HYSPLIT), and 850 hPa smoke concentrations from the Navy Aerosol Analysis and Prediction System (NAAPS). Back trajectories were run for 72 h, ending at 00:00 coordinated universal time (UTC), i.e., 08:00 local time (LT), and constrained isobarically, 300 m above ground level (a.g.l.).
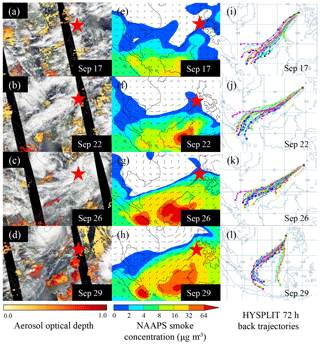
Figure 2Satellite images of the SCS region taken from (a–d) NASA Worldview with an overlaid AOD; (e–h) NAAPS smoke concentration plots (µg m−3; 850 hPa); and (i–l) HYSPLIT ensemble back trajectories during the cruise for 17, 22, 26, and 29 September (isobaric; 300 m a.g.l.; 72 h; ending at 00:00 UTC, i.e., 08:00 LT). The red star indicates location of the Vasco.
2.2 Aerosol sampling and analysis
Size-resolved aerosol samples were collected during the cruise using a Davis Rotating Uniform size-cut Monitor (DRUM) continuously sampling cascade impactor. Samples were collected with a 10 µm inlet and eight size cuts at 5, 2.5, 1.15, 0.75, 0.56, 0.34, 0.26, and 0.10 µm at a 90 min time resolution from midday 17 September until midday 30 September local time. Particles were collected on Mylar strips coated with Apiezon grease. The eight drums were rotated at a consistent rate to create a temporal record of mass concentration (Raabe et al., 1988). X-ray fluorescence (XRF) was performed on the DRUM samples at the Advanced Light Source (ALS) of Lawrence Berkeley National Laboratory to measure mass concentrations of 28 elements, ranging from Na to Pb. In this study, data were filtered based on location notes from the cruise such that samples collected in the vicinity of Manila Bay were excluded from the analysis. Additionally, samples during an 8 h pump failure that occurred on 20 September were also excluded from the dataset. In the analysis, the stages were aggregated into three modes: coarse (1.15–10 µm), fine (0.34–1.15 µm), and ultrafine (0.10–0.34 µm) modes. A large difference in the concentrations of stage 6 (0.34–0.56 µm) compared to (the adjacent) stages 5 (0.56–0.75 µm) and 7 (0.26–0.34 µm) was observed. The sharp decrease in concentrations in stage 6 despite the high concentrations in stages 5 and 7 has been observed in other studies involving the DRUM sampler; this is likely due to DRUM sampling artifacts and does not reflect the true aerosol mass distribution (Atwood et al., 2013a). Nevertheless, the two size-resolved modes lend themselves to size-segregated analysis. In this study, we simply report the mass distributions as sampled by the DRUM.
In addition to the DRUM sampler, eight sets of PM2.5 filters were collected during the cruise and were chemically analyzed for information on species such as sulfate, nitrate, and organic carbon. The PM2.5 filters were described more fully in Reid et al. (2015). Mass reconstruction was performed on the PM2.5 filter data according to the methodology of Malm and Hand (2007). Results are shown in Fig. S1 and discussed briefly in Sect. 3.1.
2.3 Model and satellite data
NOAA HYSPLIT model back trajectories (Draxler et al., 1998, 1999; Rolph et al., 2017; Stein et al., 2015) were generated throughout the cruise period to investigate locations of aerosol emission. HYSPLIT back trajectories have been used in several studies to establish air mass source regions (Lin et al., 2007; Cohen et al., 2010a; Atwood et al., 2013a, 2017). Back trajectories were run for 72 h for heights of 500 and 300 m to investigate possible vertical inhomogeneity that has been noted in other SCS papers (Atwood et al., 2013a). Trajectory endpoints corresponded to cruise coordinates. Trajectories were constrained isobarically to limit vertical wind velocity since our area of interest is surface-level emission.
The NAAPS reanalysis product (Lynch et al., 2016), with driving meteorology from the Navy Global Environmental Model (NAVGEM), was used to provide overall aerosol and meteorological context to the analysis. This reanalysis utilizes a modified version of the NAAPS as its core and assimilates quality-controlled retrievals of aerosol optical depth (AOD) from MODIS on Terra and Aqua and the Multi-angle Imaging SpectroRadiometer (MISR) on Terra (Zhang et al., 2006; Hyer et al., 2011; Shi et al., 2014). NAAPS characterizes anthropogenic and biogenic fine aerosols (including sulfate, and primary and secondary organic aerosols), as well as dust, biomass burning smoke, and sea salt aerosols. Smoke from biomass burning is derived from near-real-time satellite-based thermal anomaly data to construct smoke source functions (Reid et al., 2009) with additional orbital corrections on MODIS-based emissions and regional tuning. The system has been successfully used to monitor biomass burning plumes and to study the relationship of the aerosol life cycle to weather systems over the MC (Reid et al., 2012, 2015, 2016; Atwood et al., 2013b; Xian et al., 2013).
Active fire hotspot data were downloaded from the Fire Information for Resource Management System (FIRMS) (https://firms.modaps.eosdis.nasa.gov/, last access: 19 July 2017). Active fire hotspots and aerosol optical depth (AOD) at a wavelength of 550 nm were tracked throughout the cruise via MODIS. MODIS detects thermal anomalies across a region to identify possible fire activity. MODIS-derived AOD was used to derive large-scale estimates of PM2.5 in some studies (e.g., Zheng et al., 2017). In the study, MODIS was used to track burning emissions that were found to be particularly prevalent in eastern Malaysia and Indonesia. The use of MODIS to track active fire hotspots has been used in other studies to understand seasonal trends in agricultural burning (Reid et al., 2012) and to identify and locate burning-related sources when used in conjunction with HYSPLIT back trajectories (Atwood et al., 2017).
The NASA Worldview site (https://worldview.earthdata.nasa.gov/, last accessed: 28 April 2018), an application operated by NASA's Goddard Space Flight Center Earth Science Data and Information System (ESDIS) project, was used to supplement the satellite data by providing true color images of the region and is particularly useful in demonstrating sudden changes of cloud environment or monsoon flow caused by tropical cyclones.
2.4 Positive matrix factorization
Positive matrix factorization (PMF) was used to study the covariance of elemental species. PMF is a multivariate factor analysis technique used in source apportionment that resolves a sample matrix X (i×j) of i samples and j species into matrices G (i×k), F (k×j), and E (i×j), i.e., the source contribution matrix, source profile matrix, and residual matrix, respectively, with the assumption of k factors as follows:
The goal of PMF is to determine the number of factors or sources k such that the solution will be physically interpretable. Developed by Paatero and Tapper (Paatero and Tapper, 1994), PMF is a well-established approach used in previous source apportionment studies (Polissar et al., 1998; Lee et al., 1999; Han et al., 2006; Chan et al., 2008; Karanasiou et al., 2009; Lestari et al., 2009; Santoso et al., 2011; Wimolwattanapun et al., 2011). PMF provides more physically realistic results compared to other factor analysis techniques due to nonnegative constraints in the model and better treatment of missing or below detection limit (BDL) values by increasing the associated uncertainty (Paterson et al., 1999).
PMF outputs source profiles (F) and source contributions (G). PMF source profiles were normalized to the percent of species sum, defined as the percent concentration of an element apportioned to a source. An outlier threshold distance α was used to reduce the effect of extremely large data points and was set at a value of 4.0 to be consistent with other PMF studies (Lee et al., 1999; Han et al., 2006).
Prior to analysis via PMF, the 28 elements measured via XRF were filtered based on their Pearson's R correlation with the total elemental PM mass per mode in order to improve the interpretability of PMF factors. A minimum Pearson's R value of 0.0 was used, which removed elements that were negatively correlated with the total elemental PM. From the 28 elements identified by XRF, 20 elements in the coarse mode, 22 elements in the fine mode, and 19 elements in the ultrafine mode were included in the PMF analysis. Comparing profiles with and without the correlation-based filtering, there was no significant change in factor interpretation. This indicates that the removed elements were unnecessary for improving the PMF results (Liao et al., 2019; Ma et al., 2019). Tables S1–S3 (Supplement) show the correlation coefficients of coarse-, fine-, and ultrafine-mode elements. The filtering of elements through correlation with total PM per mode was observed to improve the interpretability of the PMF outputs and remove the need for the matrix rotation parameter, Fpeak.
Data screening was performed based on the approach of Polissar et al. (1998) and Han et al. (2006) to ensure that no erroneous data points were included in the analysis. BDL values were replaced by half the detection limit and relative uncertainties were set to 100 % (Han et al., 2006). Signal-to-noise ratios were determined and elements with low ratios (less than 0.2) were excluded from the dataset (Paatero and Hopke, 2003). Measured elemental concentrations below the detection limit of XRF were replaced with half the detection limit and their relative uncertainties were set to 100 % as done in Han et al. (2006). Detection limit values and error values were based on values provided by the Lawrence Berkeley National Laboratory.
The current study employs a size-resolved PMF approach as a supplement to the other analysis methods. PMF is a powerful tool that quantifies the contributions of PM sources and is useful for forming an initial understanding of the possible sources from the data. However, PMF may neglect important events, particularly short-term ones, that can reveal insightful interactions between identified sources and is unable to dissociate covarying sources as it assumes orthogonality between factors (Van Pinxteren et al., 2016).
For this study, we included only the DRUM elemental data for PMF analysis. Speciated data from the PM2.5 filter was excluded due to the limited number of filters available (eight quartz and eight Teflon filters). The much higher temporal resolution (174 timestamps) from the DRUM sampler, in addition to its collection across eight size ranges, provided the necessary data resolution for PMF while offering the additional degree of freedom of size-resolved collection. Due to the limitations inherent in a 2-week research cruise, the collected dataset is not expected to provide a full quantitative inventory of sources but instead provides an opportunity to study short-term aerosols events to gain a better understanding of source variability in the SCS region.
3.1 Reconstructed mass and DRUM mass distributions
Mass reconstruction performed on the PM2.5 filters shows an increasing trend in aerosol loadings towards the end of the cruise (Fig. S1a). A large event beginning on 28 September is characterized by heightened contributions of particulate organic matter. A smaller aerosol event was also detected by the 23 and 25 September filters. The mass reconstruction shows that 53 % of the total PM2.5 gravimetric mass is accounted for by the reconstructed components, which includes organic carbon (Fig. S1b). The elemental contribution to the total PM2.5 mass was estimated as the summed contributions of the reconstructed sulfate, sea salt, and soil components according to formulas from Malm and Hand (2007) and Chow et al. (2015). Reconstructed elemental components derived from the DRUM sampler compose 21.2 % of the total PM2.5 mass. This is approximately twice the value calculated with filter-collected elemental concentrations (11.7 %). PM2.5 Teflon filters have been observed to show lower concentrations than rotating-drum impactors for several elements, attributed to insufficient background subtractions when computing for filter concentrations (Venecek et al., 2016). Other potential factors in this discrepancy include a complicated sampling environment that may result in filter losses during collection and the long filter collection times during the cruise.
Elemental mass size distributions show normalized species concentrations (dM∕dlog Dp) across all eight DRUM stages and can be used to validate the signal of a mode-specific tracer. In addition to isolating the signal of a tracer, changes in the mass distributions of key elements over time indicate periods when mode-specific sources are present. Figure 3 depicts the mass size distributions of the (a) summed elemental PM and the following key elements (b) potassium (K) as a tracer for biomass burning in the fine and ultrafine modes; (c) sulfur (S), a general indicator of combustion; (d) silicon (Si) for soil dust; (e) vanadium (V) and (f) nickel (Ni), which are often paired as tracers of oil combustion; (g) iron (Fe), another key tracer for dust; and (h) chlorine (Cl), a reasonable tracer for sea spray given the sampling location. Figure 3 is further divided into time periods, distinguished by color: 18–19 September (red), 19–24 September (blue), 24–27 September (green), and 27–30 September (black).
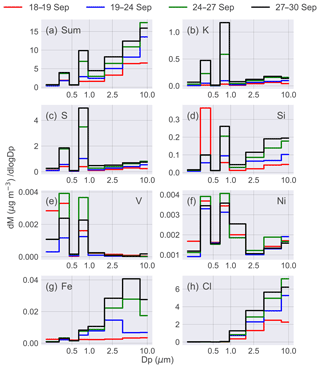
Figure 3Time evolution of mass size distributions over the cruise period: (a) sum of all measured elements, (b) potassium, (c) sulfur, (d) silicon, (e) vanadium, (f) nickel, (g) iron, and (h) chlorine. The time periods are colored as follows: 18–19 September (red), 19–24 September (blue), 24–27 September (green), and 27–30 September (black). Stage numbers are shown in (a).
The mass distribution of summed elemental PM (Fig. 3a) is informative, as it shows distinct peaks in the coarse and sub-micrometer ranges, pointing to a combustion or anthropogenic signal during the cruise. The total mass size distribution shows that, over time, a regime change occurred around 24 September, during which the general back trajectory origin shifts to the Maritime Continent. Comparing the magnitude of the summed mass distribution to those of the key species, it is clear that S contributed a significant part of the sub-micrometer mass. Elements associated with combustion showed peaks in stage 5 (0.56–0.75 µm) and stage 7 (0.26–0.34 µm). K, S, and Si have very similar changes in their mass size distributions over the cruise period that are suggestive of a common source (Fig. 3b–d). During the latter half of the cruise, a regime shift occurred, wherein back trajectory origins shifted to southern Kalimantan (Fig. 2). We observe coincident enhancements in K, S, and Si – indicative of a common source, likely biomass burning. These elements have strong peaks in stages 5 and 7 during the whole cruise, but particularly high values are observed during the last days of the sampling period (27–30 September). A general enhancement late in the cruise is likely related to the increase in the number of active fire hotspots reported by Reid et al. (2015), who attributed these hotspots primarily to Indonesian Kalimantan and southern Sumatra. As the cruise took place during the end of the Asian summer monsoon, 300 m a.g.l. winds were predominantly southwesterly. A shift in back trajectories at the end of the cruise to the western and southern coasts of Borneo is observable in Fig. 2l, suggesting the source of the late-cruise enhancement to be the MC, which hosts elevated aerosol background levels during this time of year from seasonal burning (Reid et al., 2013). The advection of this large aerosol event can be observed in the NAAPS smoke model over the region (Fig. 2g, h). The attribution of late-cruise aerosol enhancement to the MC is in agreement with Reid et al. (2015), who noted that the AOD maps and southwesterly flows towards the end of the cruise were suggestive of southwesterly transport from the MC to SCS.
Covariance of Si (Fig. 3d) with K and S suggests possible fine soil entrainment caught in burning updraft (Reid et al., 2015). The stage 5 and stage 7 peaks in S are similar to those observed for northern SCS in the springtime (Atwood et al., 2013a); however, we report enhanced values, attributed to the timing of the sampling period during the MC burning season.
Interestingly, Si shows a strong peak early in the cruise (18–19 September) unique to the ultrafine mode, which indicates this particular signal may not originate from soil dust but from fly ash (Xie et al., 2009). As the Vasco was traveling past the islands of Mindoro and Coron en route to Palawan, local sources are likely the cause of the ultrafine Si enhancement. This early cruise Si signal is further examined through later time series and regressions.
V shows a mass distribution characteristic of a combustion source with strong peaks in stage 5, stage 7, and stage 8 (0.10–0.26 µm) (Fig. 3e). Almost no contribution was observed for coarser stages 1 through 4 (0.75–10 µm), indicating that V did not originate from soil (Lin et al., 2015) and can be treated as a tracer for oil combustion. Ni shows a similar mass distribution (Fig. 3f) but had a larger spread over the eight stages than V, which may be due to contributions from other sources such as fly ash (Davison et al., 1974).
Fe and Cl, well-known tracers for soil dust and sea spray, respectively, showed coarse-mode distributions that taper off considerably in the sub-micrometer stages (Fig. 3g, h). Cl shows a purely coarse distribution, indicative of the influence of sea spray considering the sample location (Viana et al., 2008; Gugamsetty et al., 2012; Farao et al., 2014). Fe shows small peaks in stage 4 (0.75–1.15 µm), stage 5, and stage 7; however, these do not constitute a significant signal relative to its coarse-mode concentrations. As such, we treat Fe as our coarse-mode soil dust tracer. The mass distribution of Fe is observed to increase across stages 1 through 3 (2.5–10 µm) over the cruise period. The increase in coarse Fe coincides with the NAAPS-simulated transport of smoke (Fig. 2g, h) and mirrors the enhancements of K, S, Si (Fig. 4a, b), and Al (Fig. S2a, b). These patterns suggest that coarse soil dust accompanies smoke emissions, possibly through entrainment. The presence of soil dust is further corroborated by Fig. 3d, which shows the presence of Si in the coarse mode. The distinct coarse- and fine-mode peaks of Al and Si indicate separate soil dust sources. As fine-mode particles have longer residence times (Cohen et al., 2010a), the fine peaks may be an indicator of long-range transport of fine soil dust through the SCS.
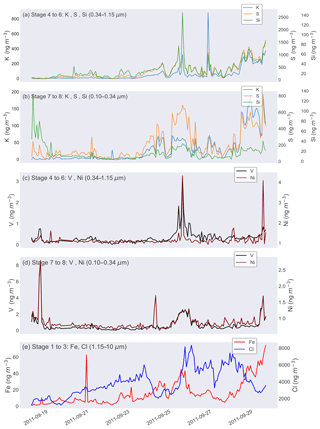
Figure 4Time series of (a) stage 4–6 K, S, and Si; (b) stage 7–8 K, S, and Si; (c) stage 4–6 V and Ni; (d) stage 7–8 V and Ni; and (e) stage 1–3 Fe and Cl.
Interpreting DRUM data reveals insights about the composition and interpretation of sources. Table 1 shows the ratios of elemental PM1.15 ∕ PM10 mass concentrations. As in Atwood et al. (2013a), the ratio slope was computed by taking the slope of the linear regression line between elemental PM1.15 and PM10 mass concentrations, accompanied by r2 values. Direct averages of per-timestamp ratios of PM1.15 and PM10 were also taken to compute for ratio averages, accompanied by the standard deviation of the ratios. Fe and Cl both had ratios of 0.06, which confirms the predominantly coarse nature of these species. As commonly used tracers of soil dust, Al and Si show moderate ratio slope values of 0.51 and 0.29, respectively, suggesting that Al resides in both coarse and fine (PM1.15) modes, while Si is predominantly coarse. As expected, elements commonly associated with anthropogenic species such as V, K, and S show high PM1.15 ∕ PM10 ratios (0.8 and above), which indicates that these elemental particles largely reside in the fine and ultrafine modes. The high ratios of V, K, and S provide evidence for the presence of anthropogenic emissions from sources such as oil combustion and biomass burning, while the low ratios of Fe and Cl support their treatment as tracers for soil dust and sea spray, respectively.
The time-resolved DRUM data are important for showing variations in species which may be representative of important aerosol events. Thus, observations on the time-resolved DRUM data can aid in our analysis. At the beginning of the cruise, between 18 and 19 September, V, Ni, and Si show enhancements in stages 5, 7, and 8. The stage 7 Si peak during this time is the maximum concentration over the entire cruise period, therefore this warrants further analysis through later time series and regressions. The period of 19–24 September shows a low point in the DRUM peaks of several elements, most notably combustion tracers K and V (Fig. 3b, e), while Cl (Fig. 3h) shows higher peaks in the coarse mode, which suggests a period of clean marine aerosol. This period was described by Reid et al. (2015) as the cleanest of the cruise. The NAAPS model shows nearly zero smoke concentration at the sampling site (Fig. 2f), while 72 h HYSPLIT back trajectories indicate that air masses originate from the central SCS (Fig. 2j). From 24 to 27 September, we observed the first major aerosol event, characterized by the stage 5 and 7 enhancements of several combustion elements: K, S, Si, V, and Ni (Fig. 3b–f). Fe, our coarse-mode soil dust tracer, shows enhancements in stages 1 to 3 (Fig. 3g), which points to combustion-related entrainment of soil dust in the coarse mode. The NAAPS model (Fig. 2g) depicts the intensification and spread of a smoke-related aerosol event that had been escalating in southern Kalimantan since 22 September, reaching the Vasco around 26 September. During this mid-cruise period, concentrations of biomass burning species K, S, Si, and Al are elevated and oil combustion tracers V and Ni show their maximum concentrations for the cruise in stages 5 and 7 (Fig. 4e, f). The last period, 28 to 30 September, depicts the highest concentrations of elements associated with biomass burning (Fig. 4a, b; Fig. S2a, b). As seen in the NAAPS smoke model (Fig. 2h) and HYSPLIT model (Fig. 2l), the westward movement of TC Nesat across the region alters back trajectories so that they travel around Borneo island, reaching southern Kalimantan, which hosted a high active fire hotspot density during this time (Reid et al., 2015), thus bringing polluted air masses toward the sampling site. Stage 5 and 7 peaks of K and S are quite notable as no other stages show significant enhancements in response to this event. Fe and Si show similar changes but instead for the coarser stages 1 to 3 (Fig. 3d, g), indicating a covariance of soil dust and biomass burning tracers. The temporal trends from the DRUM data serve as an entry point into the time series analysis. By identifying key DRUM stages and time periods per element based on their mass size distributions, we can then examine these stages to observe aerosol events over the cruise period.
3.2 Time series of selected elements
The first few days of the cruise showed an 18 September event in oil combustion tracers V and Ni in the ultrafine mode (Fig. 4d), with a coincident but lower-magnitude response in the fine mode (Fig. 4c). Ultrafine-mode V and Ni show their maxima for the cruise period during this time, expanded further in Sect. 5. High concentrations of ultrafine Si were sampled during this time from the beginning of the cruise until 19 September when it dropped to stable background levels. This early cruise enhancement was also seen in its mass distribution plot (Fig. 3d). As the Vasco was traveling among islands, the Si signal may be due to local sources en route to the El Nido sampling site.
Reid et al. (2015) noted periods of clean regime after departing Manila Bay through midday 22 September, observable in the consistently low concentrations of various elements (Fig. 4). Chlorine shows a gradual increase in concentration from 20 September until 24 September. Chlorine, although it ages into HCl, is assumed to be fresh due to the sampling location and can therefore be used as an indicator of sea spray. Interestingly, coarse-mode Cl (Fig. 4e) showed peak concentration times during low points in the concentrations of anthropogenic aerosol species (Fig. 4a–d), marking periods of clean marine aerosol on 22–24 September and 26–28 September. Wet deposition processes are likely responsible for the suppressed anthropogenic aerosol concentrations as precipitation was prevalent during these periods (Reid et al., 2015). Conversely, peaks in the concentrations of anthropogenic aerosol occurred during dry periods of the cruise when precipitation was low: 24–26 September and 28–30 September. During the periods of clean marine aerosol, back trajectories shift away from source regions and traverse open sea (Fig. 2j, k), which also hosts a lower shipping route density compared to coastal regions (Fig. S3, Supplement). The first half of the cruise also saw the lowest concentrations from species associated with biomass burning, specifically sub-micrometer K, S, Si, (Fig. 4a, b), and Al (Fig. S2a, b, Supplement). These species track each other quite well throughout the cruise period, indicating a common source.
The event between 24 and 26 September is observable on the time series of several key elements. The plume was the first of two distinct plume events reported by Reid et al. (2015), with the later plume occurring on 29 September. The enhancement of all elements in Fig. 4 suggests a mix of biomass burning, oil combustion, and soil dust influences within the 24–26 September plume. Fine-mode V and Ni show their maximum concentrations for the cruise during this event (Fig. 4c). Although these two plumes appeared as one uniform progression across the SCS region on the NAAPS smoke model (Fig. 2h), the time series showed the presence of two distinct events (Fig. 4), which is corroborated by observations from Reid et al. (2015). During this period, aerosol concentrations dropped sharply before recovering due to the passage of squall lines, observed in the time series for K, S, Si, Fe, and Cl (Fig. 4a, b, e). As concluded in Reid et al. (2015), frequent short-term events, such as cold pools and squall lines, must be accounted for in modeling studies in order to properly capture aerosol–convection interaction.
The period between plumes (26–28 September) is characterized by an overall drop in the aerosol concentration of species associated with anthropogenic sources (K, S, V, and Ni; Fig. 4a–d). As Cl concentrations show peak values during this period (Fig. 4d), this indicates a period of pure marine aerosol sampling similar to the 22–24 September clean period. Coinciding with the passage of TC Nesat through the SCS, the observed drop in aerosol concentration is attributed to a possible restriction of shipping traffic in response to the TC and scavenging of aerosols by precipitation along the TC inflow arm (Fig. 2c) (Reid et al., 2015).
The last days of the cruise were particularly eventful, as the largest aerosol event of the cruise period was visible on the NAAPS model in the form of smoke (Fig. 2h), accompanied by the spread of high AOD values throughout the SCS (Fig. 2d). Although the large areas of cloud cover created by TC Nesat hinder the detection of AOD on 26 September, the region was free enough of cloud cover by 29 September that significant AOD values were observed to visibly stretch from southern Kalimantan towards the Vasco sampling site (Fig. 2d). In general, the NAAPS smoke transport model agrees with the spatial distribution of high AOD. Here, NAAPS modeling of smoke transport is useful in demonstrating the event's northward advection and the severity of smoke concentration on Borneo on 26 September (Fig. 2h). Time series plots of elements associated with biomass burning (K, S, Si; Fig. 4a, b) and coarse-mode soil dust (Fe; Fig. 4d) show significant enhancements during this time that were also observed on their mass distributions (Fig. 3). HYSPLIT back trajectories show that air masses originate from southern Kalimantan during this period, as opposed to Peninsular Malaysia during the first half of the period (Fig. 2j, l). The shift in air mass trajectories is attributed to the passage of TC Nesat through the region as inflow arms from TCs have been observed to accelerate air mass advection across the SCS, bringing more MC air into the region (Reid et al., 2012, 2015). The observed transport of emissions from Borneo indicates that TC-enhanced long-range transport is a significant factor in SCS aerosol dispersion.
4.1 Source apportionment via positive matrix factorization
To verify groupings of key elements and aid in source identification, size-resolved PMF was performed. As described in Sect. 2, the eight-stage DRUM data were combined into coarse (1.15–10 µm), fine (0.34–1.15 µm), and ultrafine (0.10–0.34 µm) modes and the species included in the PMF analysis were then filtered based on their correlation to the aggregated PM concentration per mode. The PMF analysis resolved six sources across the three size ranges: biomass burning, oil combustion, soil dust, a crustal–marine mixed source, sea spray, and fly ash (Table 2). Due to the similarities in composition and temporal trends of the crustal–marine mixed source in the coarse mode and the sea spray factor in the fine mode, they are depicted together in Figs. 5–7 for simplicity.
One strength of PMF is its quantification of a source's contribution. Figure 5 shows the percent contribution of each source relative to the total elemental PM mass. As expected, natural sources such as the crustal–marine mixed source and soil dust mainly contribute to the coarse mode, while combustion-related sources such as biomass burning and oil combustion contribute to the fine and ultrafine modes. The identification of sea spray in the fine mode is likely due to the existence of Cl in stage 4 of the DRUM sampler (Fig. 3h). The existence of these sources in their expected modes is an indicator of the successful implementation of PMF. The following sections describe the observed characteristics of sources determined by PMF.
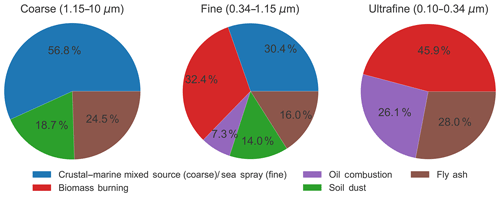
Figure 5Contributions of factors to the total elemental PM mass. The crustal–marine mixed source (coarse mode) and sea spray (fine mode) share the same color to reflect their similar chemical compositions.
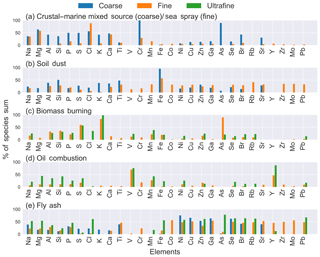
Figure 6PMF source profiles across different size ranges displayed by percent of species sum for (a) crustal–marine mixed source, (b) soil dust, (c) biomass burning, (d) oil combustion, and (e) fly ash. Coarse: stage 1–3 (1.15–10 µm; blue); fine: stage 4–6 (0.34–1.15 µm; orange); ultrafine: stage 7–8 (0.10–0.34 µm; green).
4.1.1 Crustal–marine mixed source
The crustal–marine mixed source was resolved in the coarse mode and is characterized by high apportionments for Mg, Cl, P, Al, Si, S, and Ca (Fig. 6a). This source explains nearly half of the variation in crustal elements such as Al, Si, and Ca. Na and Cl show the highest contribution to the factor mass, which indicates marine influence (Fig. S4, Supplement). These elements are indicative of a mix of marine and crustal emissions (Han et al., 2006; Wang et al., 2014), thus its identification as a crustal–marine mixed source. The mixed nature of the source points to the covariance of local crustal emissions from islands of the Maritime Continent and those nearby with sea spray. Cl has been treated as the tracer for this factor due to its high factor sum apportionment (Fig. S4, Supplement) and is considered marine in origin under the assumption that the sampled Cl originated from freshly produced sea spray (Atwood et al., 2013a). This is likely the case for the cruise, as sampling was done over sea water. The factor showed quite high mass contributions to the coarse mode (56.8 %) indicating its dominant influence on coarse elemental PM (Fig. 5a). Although both this factor and the coarse-mode soil dust factor are related to crustal emissions, the crustal–marine mixed source is distinct from the coarse-mode soil dust factor in terms of its temporal trend, most apparent during the 28–30 September aerosol event (Fig. 7a, b).
4.1.2 Sea spray
This factor was resolved in the fine mode and shows high apportionments for Na, Mg, Cl, and Ca. The identification of the factor as sea spray is evidenced by the nearly 100 % source apportionment of Cl. This factor showed fine (30.4 %) modes, attributed to the sampling location over water. As noted above, the appearance of this factor in the PMF analysis is due to the persistence of Cl in the 0.75–1.15 µm of the DRUM sampler (Fig. 3h). The covariance of the sea spray factor in the fine mode with the crustal–marine mixed source in the coarse mode points to the influence of marine emissions to some extent in both the fine and coarse modes, as suggested by a moderate correlation coefficient (0.67) between PM10 and PM2.5 Cl (Table 1).
4.1.3 Soil dust
This factor was characterized by the presence of Fe, Al, Si, K, Ca, Ti, and Zn in the coarse mode and Fe, Cr, Mn, and Y in the fine mode (Fig. 6b; Table 2). Several of these elements are associated with soil dust (Artaxo and Maenhaut, 1990; Artaxo et al., 1998; Lestari et al., 2009; Wimolwattanapun et al., 2011; Gugamsetty et al., 2012). Soil dust may originate from the nearby island of Palawan but can also potentially come from Borneo. The PMF model was able to distinguish between the crustal–marine mixed source and soil dust factors. As crustal–marine mixed emissions are assumed to be freshly sampled during the cruise and the temporal trends of the two sources are distinct (Fig. 7a, b), this suggests the possibility of a long-range transport mechanism for coarse-mode soil dust. The time series of coarse soil dust (Fig. 7b) tracks the fine biomass burning factor well (Fig. 7c), indicative of coarse soil dust particles entrained in biomass burning plumes. Fe serves as our tracer for soil dust due to its high apportionment in both soil dust modes. This factor showed mass contributions of 18.7 % and 14.0 % in the coarse and fine modes, respectively, which indicates the predominantly coarse-mode contribution of the factor (Fig. 5a, b).
4.1.4 Biomass burning
This factor was characterized by high levels of K and S and moderate levels of Al, As, and Si that were found to be associated with biomass burning in previous studies (Artaxo et al., 1998; Han et al., 2006; Lestari et al., 2009; Atwood et al., 2013a; Alam et al., 2014) (Fig. 6c; Table 2). This factor showed the highest percent contributions to the PM mass: 32.4 % and 45.9 % in the fine and ultrafine modes, respectively. The sources of the 26 September and 28–30 September events (Fig. 7c) will be investigated in Sect. 5. The presence of crustal elements Fe, Si, and Al in the source profile and the covariance of the coarse soil dust factor (Fig. 7b) with this factor (Fig. 7c) indicate possible soil dust entrainment during burning updraft (Reid et al., 2015; Schlosser et al., 2017).
4.1.5 Oil combustion
This factor was characterized by high levels of V (Fig. 7d; Table 2), a well-documented tracer for oil combustion (Hedberg et al., 2005; Mazzei et al., 2008; Becagli et al., 2012). As shown in Fig. 5, the oil combustion factor only appeared in the fine and ultrafine sizes, contributing 7.3 % and 26.1 %, respectively, to the total elemental PM mass. The increasing contribution towards finer stages corroborates the identification of the factor as an anthropogenic source. The presence of oil combustion is expected as the SCS hosts high shipping volume, particularly in parts of the Borneo coast (Fig. S3, Supplement).
4.1.6 Fly ash
This factor was observed in all size modes, characterized by high levels of trace metals Ni, Ga, Zn, Se, Br, Rb, and Pb across modes, with slight differences in composition per mode (Fig. 6e) and a source contribution without distinct events (Fig. 7e). The dominance of Ni, Zn, Se, and Br are indicative of fly ash (Davison et al., 1974; Markowski et al., 1985; Deonarine et al., 2015). Moderate apportionments of crustal elements Na, Mg, Al, Si, P, and Ti are also observed, suggestive of entrained soil. The source contribution time series shows a background-type signal. The factor contributed 24.5 %, 16.0 %, and 28.0 % to the total elemental PM mass for the coarse, fine, and ultrafine modes, respectively (Fig. 5). Long-range transport of fly ash from coal-fired power plants in Indonesia or Peninsular Malaysia may be responsible for the appearance of the factor, as no local coal-fired power plants could be found upwind of the sampling site in 2011.
The PMF analysis resolved the presence of six sources across the ultrafine, fine, and coarse modes, which aids in directing further analysis by identifying key species in the source profiles. Pearson correlation heat maps (Fig. S5–S7, Supplement) and matrices with numerical values (Tables S1–S3, Supplement) were constructed to examine the relationships between species. The first column of the correlation outputs (Fig. S5–S7, Tables S1–S3, Supplement) shows the correlation coefficient of the element when compared to the summed elemental PM for that mode. Similar groupings of elements were observed when compared to the PMF source profiles, indicating the robustness of the analysis. In the coarse mode (Fig. S5, Table S1, Supplement), we observe high correlations between Na, Mg, Cl, P, S, K, Ca, Br, and Sr, which are associated with sea spray and crustal sources (Han et al., 2006; Wang et al., 2014). Fe, Ti, Mn, Si, and Zn show moderate to high correlations in the coarse mode, indicative of dust (Karanasiou et al., 2009; Wimolwattanapun et al., 2011; Lin et al., 2015; Landis et al., 2017). In the fine mode, moderate to high correlations between Al, Si, P, S, K, and Br are observed (Fig. S6, Table S2, Supplement). Several of these biomass burning elements show similarly strong correlations in the ultrafine mode (Fig. S7, Table S3, Supplement). V and Ni show a high correlation coefficient (0.91) in the ultrafine mode, indicative of oil combustion.
The excellent correspondence between the observed groupings of elements based on correlation (Tables S2–S4, Supplement) and the sources resolved by PMF (Table 2) adds confidence to the identification of key sources during the cruise. However, as PMF is an unsupervised technique, it may not sufficiently disaggregate significant, consecutive aerosol events. Visually, two distinct ultrafine events occur between 18 and 19 September in Si (Fig. 4b) and V and Ni (Fig. 4d), which are merged by PMF in its oil combustion factor (Fig. 7d). The disproportionate enhancement of ultrafine-mode Si over V and Ni suggests a source other than oil combustion. Thus, to further expand on the relationships between elements, we turn to regression analysis.
4.2 Regressions of selected elements
An ultrafine Si event between 18 and 19 September was shown in the mass size distribution (Fig. 3d) and the time series (Fig. 4b) of ultrafine Si. Fly ash was the hypothesized source of the ultrafine Si signal; however, although the PMF analysis suggested the presence of fly ash, ultrafine Si was not significantly apportioned to the fly ash factor (Fig. 6e). Additionally, none of the factor contributions from PMF showed a similar trend between 18 and 19 September to that of ultrafine Si. This suggests that PMF may have mishandled the early Si enhancement (Fig. 4b) by merging it with an enhancement in V and Ni that occurred soon after (Fig. 4d). Regressions show that between 18 and 19 September Si had distinct ratio slopes and moderate correlations with P (r2=0.76), S (r2=0.73), and Al (r2=0.61) (Fig. 8; Table S4, Supplement) but poor correlations with fly ash tracers (As, Se, and Pb; r2<0.12). The high correlations of Si with P, Al, and S suggest a distinct source of Si between 18 and 19 September versus the rest of the cruise; but the low correlations with fly ash tracers rule out fly ash as a possible source. As the Vasco was traveling near islands, the source of the ultrafine Si enhancement is likely a local source en route to Palawan. The sudden enhancement may be related to a rapid nucleation event, as even sub-micrometer dust can be an important source of CCN in marine and coastal environments (Twohy et al., 2009).
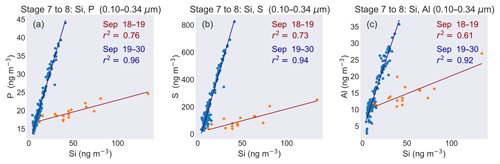
Figure 8Linear regressions of ultrafine Si and its most highly correlated elements, (a) P, (b) S, and (c) Al, divided into the cruise periods before 19 September (red) and after 19 September (blue).
As S is an indicator of general combustion (Atwood et al., 2013a), it is important to elucidate its relationship with tracers of other combustion sources. Multiple linear regression was performed on S on the fine and ultrafine modes (Fig. S8, Supplement). It was found that K and V were excellent predictors of S for most of the cruise but the model required the addition of Al to capture the variance in S between 24 and 26 September, suggesting an additional source during this period separate from biomass burning or oil combustion. A detailed description of the multiple linear regression analysis can be found in the Supplement. Further examining the relationships of S to these combustion sources, fine- and ultrafine-mode linear regressions of K and V, colored by the concentration of S per given time, were constructed to show the relationships between the three species (Fig. 9a, b). S covaries more with K than V, as seen with the clearer color gradient along the K axis, suggesting the origin of S during the cruise to be more dominantly from biomass burning rather than oil combustion.
The ratio between V and Ni is often used as an indicator of the type of oil combustion source (Hedberg et al., 2005; Nigam et al., 2006; Mazzei et al., 2008; Becagli et al., 2012; Lin et al., 2015). Linear regression plots of V and Ni have a slope of 3.64 in the ultrafine mode (Fig. 9c). Nigam et al. (2006) measured a V ∕ Ni ratio of 3.5–4 when sampling shipping emissions directly from the exhausts of various ship engines, which suggests shipping to be the main source of ultrafine-mode oil combustion during the cruise.
As soil composition varies geographically, soil dust ratios are excellent indicators of a plume's origin (Prospero et al., 1999; Song et al., 2006; Witt et al., 2006). Figure 8d shows linear regressions of soil dust elements in the coarse and fine modes. Al and Si, well-known indicators of dust (Viana et al., 2008; Tian et al., 2016; Landis et al., 2017), show moderate correlations with each other in the coarse and fine modes but slightly differ in ratio slopes between the fine (Al ∕ Si ∼ 1.3; r2=0.94) and coarse (Al ∕ Si ∼ 0.93; r2=0.78) modes (Fig. 9d). This is indicative of varying sources of fine- and coarse-mode soil, with coarse-mode soil dust enriched in Si; however, this could also be a matrix effect from the XRF analysis. As the Vasco remained near Palawan island, local dust could be the source of coarse-mode Si enrichment; however, soil dust from Borneo is also a possibility.
The regression analysis showed an early cruise enhancement in ultrafine Si that was merged by PMF with a V and Ni enhancement that occurred soon after, highlighting the importance of the regression analysis in addition to PMF to investigate the temporal characteristics of sources via elemental tracers. We suggest a local source en route to the main sampling area to be the cause of the enhancement, but fly ash is unlikely to be the source due to low correlations with its tracers As, Pb, and Se. The analysis also showed the strong associations of S with biomass burning and oil combustion; however, S was shown to covary more significantly with the former. Oil combustion was determined to originate from shipping, as indicated by a V ∕ Ni ratio within the range of that measured by a previous shipping emission study. Finally, we infer multiple sources of soil dust between the coarse and fine modes due to distinct Si–Al ratios between modes; however, we are unable to determine the exact sources due to lack of information regarding local and regional soil dust ratios.
5.1 18–19 September: ultrafine V and Ni enhancement from Sandakan, Sabah
As described in Sect. 3, ultrafine-mode V and Ni show a maximum around 18 September (Fig. 4d). As the Vasco was traveling near local islands, the event may originate from a local source; however, back trajectories propose an oil combustion source on Borneo. Back trajectories were generated every hour between 14:00 and 18:00 UTC (corresponding to 22:00 and 02:00 LT) on 18 September and show a westward shift along the eastern coast of Borneo (Fig. 10a). The coast of Borneo is largely forest (Fig. 10b) but hosts the city of Sandakan, one of Sabah's major ports (Fig. 10c, d). In addition to shipping traffic (Fig. 10d), Sandakan contains oil depots that are a major source of industry in the area. During the westward shift of the back trajectories, air masses pass through Sandakan at around 16:00 UTC, approximately the time of the sampled spike in V. The shipping activity and oil depots present in this area may be responsible for the spike in oil combustion tracers, indicating the complexity of aerosol transport in the region, as small cities like Sandakan may be a source of significant spikes in aerosol.
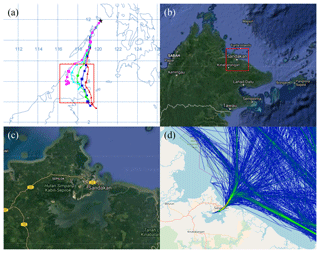
Figure 10Determination of the 18 September event using (a) HYSPLIT back trajectories, (b, c) a Google Maps view of the northeastern coast of Borneo (map data ©2018 Google), and (d) the density of shipping traffic from Sandakan, Sabah (source: MarineTraffic). Red squares indicate the location of the succeeding plot.
5.2 20–24 September: clean marine period
The first half of the cruise showed the lowest concentrations of elements associated with biomass burning K, S, Si, and Al. Back trajectories during this early period originate from the northern part of Borneo and do not penetrate deeply into the MC until late into the cruise (Fig. 2l). During this period, HYSPLIT back trajectories show that air mass pathways shift away from the Borneo coast towards open sea (Fig. 2j). In addition to the shift away from biomass burning sites, back trajectories between 22 and 24 September pass through areas of open sea that host lower levels of shipping traffic (Fig. S3, Supplement).
5.3 24–26 September: large mixed aerosol event from northwestern Borneo
Around 26 September, increases in fine-mode V and Ni occurred when air masses passed through the northwestern coast of Borneo, suggesting the presence of ports or oil depots, similar to the aforementioned spike on 18 September from Sandakan. Back trajectories generated every 6 h starting from 24 September 15:00 UTC until 26 September 09:00 UTC show little change over this period (not shown) and intersect with the shipping route hub located along northwestern Borneo, which would explain the V and Ni spikes (Figs. 2k, S1, Supplement). The enrichments of biomass burning and combustion tracers K and S in the sampled air mass span a wider period from 24 September to 26 September. This may be due to burning activity along the coast of Borneo, which hosts several MODIS-detected active fire hotspots. Late-night land breeze from the island may have advected polluted air masses towards the coast.
5.4 28–30 September: large biomass burning event from southern Kalimantan
Enhancements of these elements after 28 September coincide with a regional increase in AOD (Fig. 2d) and are captured by the NAAPS model in the form of a large smoke event advected northeast (Fig. 2h). Linear regressions show this large aerosol event at the end of the cruise as a distinct group of points with enhanced concentrations of K and S (Fig. S9, Supplement), suggesting an increase in biomass burning activity during this time. Reid et al. (2015) observed a sharp increase in the number of active fire hotspots, particularly in Sumatra and southern Kalimantan. As discussed above and depicted in Fig. 2, TC Nesat played a major in role in synoptic wind patterns during the cruise, causing a shift in back trajectories after 28 September to the southwest coast of Borneo. Thus, the enhancements of sub-micrometer K, S, Si, and Al likely originate from biomass burning in the MC.
This study describes the size-resolved aerosol elemental composition of particles collected by a DRUM rotating impactor during the 17 to 30 September 2011 M/Y Vasco cruise in the vicinity of Palawan island in the Philippines. This region was chosen due to its location as a receptor for MC aerosol sources, such as biomass burning, oil combustion, and soil dust. Meteorological conditions during the cruise were conducive to southwesterly long-range transport for seasonal burning aerosol, which was observed in the concentration time series of tracers and satellite-derived AOD. Size-resolved aerosol composition in the coarse (1.15–10 µm), fine (0.34–1.15 µm), and ultrafine (0.10–0.34 µm) modes were used as key tracers to ascertain source contributions. Despite the meteorological complexity of the SCS, we can gain insights into aerosol sources by focusing on key elemental species. The time series of key elements showed distinct events on 18–19, 24–26, and 28–30 September, with clean aerosol periods between events. These aerosol events served as case studies of sources in the region. While biomass burning is indeed a key source of aerosol, other sources such as oil combustion, crustal–marine mixed source, fly ash, and soil dust contribute to the chemical profile of the SCS during the southwest monsoon. Understanding these sources is key to characterizing aerosol composition and transport in the SCS and, by extension, developing our understanding of aerosol–cloud behavior in the region. As back trajectory analysis and aerosol chemistry showed the presence of multiple key sources, the general conclusions of the study are as follows.
-
Mass distributions of key elements showed the evolution of aerosol chemistry throughout the cruise and interesting covariances between modes. Stage 5 (0.56–0.75 µm) and stage 7 (0.26–0.34 µm) showed enhanced peaks in several elements associated with combustion. Throughout the cruise, mass distributions of V and Ni track each other well, both temporally and across DRUM stages, indicative of oil combustion. Mass distributions of V and Ni show higher values in the ultrafine mode between 18 and 19 September, indicative of an early oil combustion-enriched air mass which was identified to possibly originate from Sandakan, Sabah, Borneo. Mass distributions of K, Al, and S show large enhancements in the fine and ultrafine modes after 27 September, corroborated by a reported large aerosol event from Reid et al. (2015). The strong peaks of these biomass burning tracers, in combination with the rapid spread of high AOD and NAAPS-modeled smoke concentration across the region, provide evidence for intensive emissions from the MC. Coarse-mode soil dust elements such as Fe and Si showed similarly timed enhancements, attributed to soil particle entrainment during burning.
-
Short-term meteorological events such as TC Nesat played a key role in long-range transport as they propagated through the region, expediting the northeastward advection of aerosol emissions, an effect observed in previous studies (Atwood et al., 2013a; Reid et al., 2012, 2015). The sudden variations in aerosol concentration after 24 September can be connected to the movement of TC Nesat through the region. Prior to these events, aerosol concentrations remained at generally low levels, as NAAPS shows smoke was largely constrained to the Southern Hemisphere. The passage of TC Nesat advected air masses more northward, allowing them to penetrate deep enough into the Northern Hemisphere to be sampled by the Vasco. The TC's passage coincided with a shift in air mass origin from Peninsular Malaysia prior to 24 September to areas known for intense burning activity, most notably southern Kalimantan, by the end of the cruise. This corresponded to a mixed aerosol event from 24 to 26 September attributed to Brunei, Borneo, and a significant increase in biomass burning tracer concentrations from 28 to 30 September attributed to southern Kalimantan. Between these aerosol events, a clean marine event from 26 until 28 September was characterized by high concentrations of Cl and low levels of elements associated with anthropogenic sources. Back trajectories showed that air masses traveled through the open, central SCS, which suggests a good signal of sea spray was sampled. As the ship route brought the Vasco near islands, local crustal emissions covaried with sea spray aerosol, which resulted in the crustal–marine mixed source during the PMF analysis.
-
Six sources across the three size modes were resolved by the PMF analysis: biomass burning, oil combustion, soil dust, crustal–marine mixed source, sea spray, and fly ash. A threshold Pearson R coefficient of 0.0 was used to filter species included in the PMF analysis to improve the interpretability of the PMF solution. Results show that natural sources, the crustal–marine mixed source and soil dust factors, were observed in only the coarse and fine modes, while anthropogenic sources, i.e., biomass burning, oil combustion, and fly ash, were resolved purely in the fine and ultrafine modes. A strong correspondence between key elements seen on the PMF source profiles and groupings of these elements on the correlation matrices adds confidence to the PMF solution. The biomass burning PMF factor showed the highest percent contributions to total elemental PM mass in the fine and ultrafine modes: 32.4 % in the fine mode and 45.9 % in the ultrafine mode. It is interesting to note that the relative contribution of the oil combustion factor increased significantly towards finer modes, 7.3 % in the fine mode but 26.1 % in the ultrafine mode, corroborating its anthropogenic identification. In terms of aerosol events, PMF source contributions were able to capture the most events seen in the raw elemental concentrations. Differences in the temporal variations between PMF-resolved sources suggest these sources are distinct. However, PMF did not differentiate between an early ultrafine Si spike from a distinct, subsequent spike in V, which demonstrates that PMF may merge events, leading to a loss in resolution as observed in other studies (Van Pinxteren et al., 2016). This, however, can be ameliorated with an in-depth, supervised analysis of the data as has been done in this study.
-
As stated above, spikes in oil combustion tracers V and Ni were observed on 18 September in the fine and ultrafine modes. HYSPLIT back trajectories suggest the origin of the air mass as Sandakan, an industrial area and port city of Sabah known for its oil depots and shipping activity located along the northeastern coast of Borneo. The spike in oil combustion suggests that a small city can cause drastic increases in tracer concentration depending on air mass trajectories. The strong presence of ultrafine mode Si from 18 to 19 September was also observed, but the time series of Si is distinct from the time series of V and Ni, suggestive of a source distinct from oil combustion.
-
The 24 to 26 September event coincided with the arrival of TC Nesat east of Luzon (northeast of the Vasco's location). Enhancements of multiple key tracers for biomass burning, oil combustion, and soil dust were observed, indicative of aerosols mixing within an air mass during transport. Biomass burning tracers K, S, Si, and Al show enhancements over a wider period (24–26 September) than those of oil combustion tracers V and Ni, which spiked at the end of the period. Furthermore, aerosol–convection interactions were observed as sharp dips in the concentrations of biomass burning and soil dust tracers around 25 September before recovery. Interestingly, this dip was not observed for oil combustion tracers V and Ni. This cold pool event was reported in detail by Reid et al. (2015), and this study further elaborated on its impact on PM of different elemental compositions. This case demonstrates the effect of short-term or high-frequency phenomena on aerosol transport in the MC. HYSPLIT back trajectories show that air masses begin to travel from the southwest MC in response to TC Nesat's inflow arm. Air masses during the 24–26 September event pass through Brunei, a shipping hub located along the northeastern coast of Borneo, which explains the increase in oil combustion tracers V and Ni. The coast was also observed to host a number of active fire hotspots. Land breeze may lead to the entrainment of burning plumes into the traveling air mass, which would explain the enrichment.
-
The 28–30 September aerosol event showed an enrichment in K and S that coincided with a shift in back trajectory origin to southern Kalimantan, which hosts a high fire hotspot density. MC burning may be characterized by an elevated K ∕ S ratio and strong fine and ultrafine mode peaks in the mass distributions of S and K. The 28–30 September event also coincided with the enhancement of soil dust elements in the coarse mode, indicative of soil particle entrainment during burning activity (Reid et al., 2015).
The study identified source locations of aerosol and characterized the plumes during the Vasco 2011 cruise; however, unanswered questions remain, such as the origin of the strong ultrafine Si signal detected early in the cruise (18–19 September), which may be connected to a rapid local nucleation event. The source location of the PMF-resolved fly ash factor also remains unidentified due to its complicated source contribution time series and unclear elemental profile. Investigation into cloud nuclei (CN) properties during the cruise may be done to further validate the intensity and timing of plumes. In addition to the findings of this study on the elemental PM, future research on other species collected during the 2011 and 2012 Vasco campaigns such as trace gases may complement and deepen our current understanding of the aerosol environment in the SCS through additional degrees of freedom, specifically utilizing the lifetimes of trace gases and inferring the potential for secondary aerosol formation during transport.
The Vasco ship data are available through correspondence with Jeffrey S. Reid (jeffrey.reid@nrlmry.navy.mil). MODIS AOD images were obtained from the NASA Worldview application: https://worldview.earthdata.nasa.gov/?v=74.90262917418548,-13.851043766027335,166.53020142703977,27.52453182940219&t=2011-09-17-T01:18:44Z&l=MODIS_Terra_Aerosol,MODIS_Aqua_Aerosol,Reference_Labels(hidden),Reference_Features(hidden),Coastlines,VIIRS_SNPP_CorrectedReflectance_TrueColor(hidden),MODIS_Aqua_CorrectedReflectance_TrueColor(hidden),MODIS_Terra_CorrectedReflectance_TrueColor (last access: 26 January 2020). HYSPLIT data are accessible through the NOAA READY website (https://www.ready.noaa.gov/hypub-bin/trajasrc.pl, last access: 26 January 2020, NOAA Air Resources Laboratory, 2020). NAAPS aerosol reanalysis data can be accessed at the US GODAE server: https://nrlgodae1.nrlmry.navy.mil/ftp/outgoing/nrl/NAAPS-REANALYSIS/2011/201109/ (last access: 26 January 2020, Office of Naval Research, 2020).
The supplement related to this article is available online at: https://doi.org/10.5194/acp-20-1255-2020-supplement.
MRAH performed the analysis and prepared the manuscript. MTC supervised the analysis, especially for the PMF section. MOLC supervised the analysis and provided input for the manuscript. JSR collected the data on board the Vasco, supervised the analysis, and provided input for the manuscript. PX provided the NAAPS smoke model outputs for Fig. 2 and provided input for the manuscript. JBS, NDL, and SNYU collected the data on board the Vasco. SC and YJZ performed the XRF analysis on the data.
The authors declare that they have no conflict of interest.
We acknowledge the use of imagery from the NASA Worldview application (https://worldview.earthdata.nasa.gov/, last access: 28 April 2018), part of the NASA Earth Observing System Data and Information System (EOSDIS). The authors gratefully acknowledge the NOAA Air Resources Laboratory (ARL) for the provision of the HYSPLIT transport and dispersion model and/or READY website (https://www.ready.noaa.gov/hypub-bin/trajasrc.pl, last access: 26 January 2020) used in this publication.
This paper was edited by Tuukka Petäjä and reviewed by two anonymous referees.
Alam, K., Mukhtar, A., Shahid, I., Blaschke, T., Majid, H., Rahman, S., Khan, R., and Rahman, N.: Source apportionment and characterization of particulate matter (PM10) in urban environment of Lahore, Aerosol Air Qual. Res., 14, 1851–1861, https://doi.org/10.4209/aaqr.2014.01.0005, 2014.
Artaxo, P. and Maenhaut, W.: Aerosol Characteristics and Sources for the Amazon Basin During the Wet Season, J. Geophys. Res., 95, 16971–16985, 1990.
Artaxo, P., Fernandas, E. T., Martins, J. V., Yamasoe, M. A., Maenhaut, W., Longo, K. M., Castanho, A., and Hobbs, P. V.: Large-scale aerosol source apportionment in Amazonia, J. Geophys. Res.-Atmos., 103, 31837–31847, https://doi.org/10.1029/98jd02346, 1998.
Atwood, S. A., Reid, J. S., Kreidenweis, S. M., Cliff, S. S., Zhao, Y., Lin, N.-H., Tsay, S.-C., Chu, Y.-C., and Westphal, D. L.: Size resolved measurements of springtime aerosol particles over the northern South China Sea, Atmos. Environ., 78, 134–143, https://doi.org/10.1016/j.atmosenv.2012.11.024, 2013a.
Atwood, S. A., Salinas, S. V., Chew, B. N., Reid, J. S., Balasubramanian, R., Yu, L. E., and Kreidenweis, S. M.: Analysis of source regions for smoke events in Singapore for the 2009 El Nino burning season, Atmos. Environ., 78, 219–230, https://doi.org/10.1016/j.atmosenv.2013.04.047, 2013b.
Atwood, S. A., Reid, J. S., Kreidenweis, S. M., Blake, D. R., Jonsson, H. H., Lagrosas, N. D., Xian, P., Reid, E. A., Sessions, W. R., and Simpas, J. B.: Size-resolved aerosol and cloud condensation nuclei (CCN) properties in the remote marine South China Sea – Part 1: Observations and source classification, Atmos. Chem. Phys., 17, 1105–1123, https://doi.org/10.5194/acp-17-1105-2017, 2017.
Balasubramanian, R., Qian, W.-B., Decesari, S., Facchini, M. C., and Fuzzi, S.: Comprehensive characterization of PM2.5 aerosols in Singapore, J. Geophys. Res., 108, 4523, https://doi.org/10.1029/2002jd002517, 2003.
Becagli, S., Sferlazzo, D. M., Pace, G., di Sarra, A., Bommarito, C., Calzolai, G., Ghedini, C., Lucarelli, F., Meloni, D., Monteleone, F., Severi, M., Traversi, R., and Udisti, R.: Evidence for heavy fuel oil combustion aerosols from chemical analyses at the island of Lampedusa: a possible large role of ships emissions in the Mediterranean, Atmos. Chem. Phys., 12, 3479–3492, https://doi.org/10.5194/acp-12-3479-2012, 2012.
Boucher, O., Randall, D. Artaxo, P., Bretherton, C., Feingold, G., Forster, P., Kerminen, V.-M., Kondo, Y., Liao, H., Lohmann, U., Rasch, P., Satheesh, S. K., Sherwood, S., Stevens, B., and Zhang, X. Y.: Clouds and Aerosols, in: Climate Change 2013: The Physical Science Basis, Contribution of Working Group I to the Fifth Assessment Report of the Intergovernmental Panel on Climate Change, edited by: Stocker, T. F., Qin, D., Plattner, G.-K., Tignor, M., Allen, S. K., Boschung, J., Nauels, A., Xia, Y., Bex, V., and Midgley, P. M., Cambridge University Press, Cambridge, UK, New York, NY, USA, 2013.
Cahill, T. A., Barnes, D. E., Lawton, J. A., Miller, R., Spada, N., C, R. D. W. and Kimbrough, S.: Transition metals in coarse, fine, very fine and ultra-fine particles from an interstate highway transect near Detroit, Adv. Stud. Behav., 37, 371–401, https://doi.org/10.1016/S0065-3454(07)37008-3, 2007.
Cahill, T. A., Barnes, D. E., Lawton, J. A., Miller, R., Spada, N., Willis, R. D., and Kimbrough, S.: Transition metals in coarse, fine, very fine and ultra-fine particles from an interstate highway transect near Detroit, Atmos. Environ., 145, 158–175, https://doi.org/10.1016/j.atmosenv.2016.09.023, 2016.
Chan, Y. C., Christensen, E., Golding, G., King, G., Gore, W., Cohen, D. D., Stelcer, E., Simpson, R., Denison, L., and Wong, N.: Source Apportionment of Ambient Volatile Organic Compounds in Major Cities in Australia By Positive Matrix Factorisation, Clean Air and Environmental Quality, 42, 22–29, 2008.
Chang, C. P., Zhuo, W., John, M., and Ching-Hwang, L.: Annual Cycle of Southeast Asia – Maritime Continent Rainfall and the Asymmetric, J. Climate, 18, 287–301, https://doi.org/10.1175/JCLI-3257.1, 2005.
Chow, J. C., Lowenthal, D. H., Chen, L.-W. A., Wang, X., and Watson, J. G.: Mass reconstruction methods for PM2.5: a review, Air Qual. Atmos. Health, 8, 243–263, https://doi.org/10.1007/s11869-015-0338-3, 2015.
Cohen, D. D., Crawford, J., Stelcer, E., and Bac, V. T.: Long range transport of fine particle windblown soils and coal fired power station emissions into Hanoi between 2001 to 2008, Atmos. Environ., 44, 3761–3769, https://doi.org/10.1016/j.atmosenv.2010.06.047, 2010a.
Cohen, D. D., Crawford, J., Stelcer, E., and Bac, V. T.: Characterisation and source apportionment of fine particulate sources at Hanoi from 2001 to 2008, Atmos. Environ., 44, 320–328, https://doi.org/10.1016/j.atmosenv.2009.10.037, 2010b.
Davison, R. L., Natusch, D. F. S., and Wallace, J. R.: Trace Elements in Fly Ash, Environ. Sci. Technol., 8, 1107–1113, https://doi.org/10.1021/es60098a003, 1974.
Deonarine, A., Kolker, A., and Doughten, M.: Trace Elements in Coal Ash, U.S. Geological Survey Fact Sheet 2015–3037, 6 pp., https://doi.org/10.3133/fs20153037, 2015.
Draxler, R. R. and Hess, G. D.: An overview of the HYSPLIT_4 modelling system for trajectories, Aust. Meteorol. Mag., 47, 295–308, 1998.
Draxler, R. R.: HYSPLIT4 user's guide. NOAA Tech. Memo. ERL ARL-230, NOAA Air Resources Laboratory, Silver Spring, MD, 1999.
Engling, G., He, J., Betha, R., and Balasubramanian, R.: Assessing the regional impact of indonesian biomass burning emissions based on organic molecular tracers and chemical mass balance modeling, Atmos. Chem. Phys., 14, 8043–8054, https://doi.org/10.5194/acp-14-8043-2014, 2014.
Farao, C., Canepari, S., Perrino, C., and Harrison, R. M.: Sources of PM in an industrial area: Comparison between receptor model results and semiempirical calculations of source contributions, Aerosol Air Qual. Res., 14, 1558–1572, https://doi.org/10.4209/aaqr.2013.08.0281, 2014.
Field, R. D. and Shen, S. S. P.: Predictability of carbon emissions from biomass burning in Indonesia from 1997 to 2006, J. Geophys. Res.-Biogeo., 113, 1–17, https://doi.org/10.1029/2008JG000694, 2008.
Fujii, Y., Tohno, S., Amil, N., Latif, M. T., Oda, M., Matsumoto, J., and Mizohata, A.: Annual variations of carbonaceous PM2.5 in Malaysia: influence by Indonesian peatland fires, Atmos. Chem. Phys., 15, 13319–13329, https://doi.org/10.5194/acp-15-13319-2015, 2015.
Ge, C., Wang, J., and Reid, J. S.: Mesoscale modeling of smoke transport over the Southeast Asian Maritime Continent: coupling of smoke direct radiative effect below and above the low-level clouds, Atmos. Chem. Phys., 14, 159–174, https://doi.org/10.5194/acp-14-159-2014, 2014.
Gugamsetty, B., Wei, H., Liu, C. N., Awasthi, A., Tsai, C. J., Roam, G. D., Wu, Y. C., and Chen, C. F.: Source Characterization and Apportionment of PM10, PM2.5 and PM0.1 by Using Positive Matrix Factorization, Aerosol Air Qual. Res., 12, 476–491, https://doi.org/10.4209/aaqr.2012.04.0084, 2012.
Han, J. S., Moon, K. J., Lee, S. J., Kim, Y. J., Ryu, S. Y., Cliff, S. S., and Yi, S. M.: Size-resolved source apportionment of ambient particles by positive matrix factorization at Gosan background site in East Asia, Atmos. Chem. Phys., 6, 211–223, https://doi.org/10.5194/acp-6-211-2006, 2006.
Hedberg, E., Gidhagen, L., and Johansson, C.: Source contributions to PM10 and arsenic concentrations in Central Chile using positive matrix factorization, Atmos. Environ., 39, 549–561, https://doi.org/10.1016/j.atmosenv.2004.11.001, 2005.
Hyer, E. J., Reid, J. S., and Zhang, J.: An over-land aerosol optical depth data set for data assimilation by filtering, correction, and aggregation of MODIS Collection 5 optical depth retrievals, Atmos. Meas. Tech., 4, 379–408, https://doi.org/10.5194/amt-4-379-2011, 2011.
Karanasiou, A. A., Siskos, P. A., and Eleftheriadis, K.: Assessment of source apportionment by Positive Matrix Factorization analysis on fine and coarse urban aerosol size fractions, Atmos. Environ., 43, 3385–3395, https://doi.org/10.1016/j.atmosenv.2009.03.051, 2009.
Landis, M. S., Pancras, J. P., Graney, J. R., White, E. M., Edgerton, E. S., Legge, A., and Percy, K. E.: Source apportionment of ambient fine and coarse particulate matter at the Fort McKay community site, in the Athabasca Oil Sands Region, Alberta, Canada, Sci. Total Environ., 584–585, 105–117, https://doi.org/10.1016/j.scitotenv.2017.01.110, 2017.
Lee, E., Chan, C. K., and Paatero, P.: Application of positive matrix factorization in source apportionment of particulate pollutants in Hong Kong, Atmos. Environ., 33, 3201–3212, https://doi.org/10.1016/S1352-2310(99)00113-2, 1999.
Lee, S.-S., Feingold, G., and Chuang, P. Y.: Effect of Aerosol on Cloud–Environment Interactions in Trade Cumulus, J. Atmos. Sci., 69, 3607–3632, https://doi.org/10.1175/jas-d-12-026.1, 2012.
Lestari, P. and Mauliadi, Y. D.: Source apportionment of particulate matter at urban mixed site in Indonesia using PMF, Atmos. Environ., 43, 1760–1770, https://doi.org/10.1016/j.atmosenv.2008.12.044, 2009.
Liao, H.-T., Chang, J.-C., Tsai, T.-T., Tsai, S.-W., Chou, C. C.-K., and Wu, C.-F.: Vertical distribution of source apportioned PM2.5 using particulate-bound elements and polycyclic aromatic hydrocarbons in an urban area, J. Expo Sci. Environ. Epidemiol., 1–11, https://doi.org/10.1038/s41370-019-0153-2, 2019.
Lin, C.-Y., Wang, Z., Chen, W.-N., Chang, S.-Y., Chou, C. C. K., Sugimoto, N., and Zhao, X.: Long-range transport of Asian dust and air pollutants to Taiwan: observed evidence and model simulation, Atmos. Chem. Phys., 7, 423–434, https://doi.org/10.5194/acp-7-423-2007, 2007.
Lin, G., Penner, J. E., Flanner, M. G., Sillman, S., Xu, L. and Zhou, C.: Radiative forcing of organic aerosol in the atmosphere and on snow: Effects of SOA and brown carbon, J. Geophys. Res.-Atmos., 119, 7453–7476, https://doi.org/10.1002/2013JD021186, 2014.
Lin, Y.-C., Tsai, C.-J., Wu, Y.-C., Zhang, R., Chi, K.-H., Huang, Y.-T., Lin, S.-H., and Hsu, S.-C.: Characteristics of trace metals in traffic-derived particles in Hsuehshan Tunnel, Taiwan: size distribution, potential source, and fingerprinting metal ratio, Atmos. Chem. Phys., 15, 4117–4130, https://doi.org/10.5194/acp-15-4117-2015, 2015.
Loo, Y. Y., Billa, L., and Singh, A.: Effect of climate change on seasonal monsoon in Asia and its impact on the variability of monsoon rainfall in Southeast Asia, Geosci. Front., 6, 817–823, https://doi.org/10.1016/j.gsf.2014.02.009, 2015.
Lynch, P., Reid, J. S., Westphal, D. L., Zhang, J., Hogan, T. F., Hyer, E. J., Curtis, C. A., Hegg, D. A., Shi, Y., Campbell, J. R., Rubin, J. I., Sessions, W. R., Turk, F. J., and Walker, A. L.: An 11-year global gridded aerosol optical thickness reanalysis (v1.0) for atmospheric and climate sciences, Geosci. Model Dev., 9, 1489–1522, https://doi.org/10.5194/gmd-9-1489-2016, 2016.
Ma, L., Dadashazar, H., Braun, R. A., MacDonald, A. B., Aghdam, M. A., Maudlin, L. C., and Sorooshian, A.: Size-resolved characteristics of water-soluble particulate elements in a coastal area: Source identification, influence of wildfires, and diurnal variability, Atmos. Environ., 206, 72–84, https://doi.org/10.1016/j.atmosenv.2019.02.045, 2019.
Malm, W. C. and Hand, J. L.: An examination of the physical and optical properties of aerosols collected in the IMPROVE program, Atmos. Environ., 41, 3407–3427, https://doi.org/10.1016/j.atmosenv.2006.12.012, 2007.
Mazzei, F., D'Alessandro, A., Lucarelli, F., Nava, S., Prati, P., Valli, G., and Vecchi, R.: Characterization of particulate matter sources in an urban environment, Sci. Total Environ., 401, 81–89, https://doi.org/10.1016/j.scitotenv.2008.03.008, 2008.
Markowski, G. R. and Filby, R.: Trace Element Concentration as a Function of Particle Size in Fly Ash from a Pulverized Coal Utility Boiler, Environ. Sci. Technol., 19, 796–804, https://doi.org/10.1021/es00139a005, 1985.
Nakajima, T., Yoon, S. C., Ramanathan, V., Shi, G. Y., Takemura, T., Higurashi, A., Takamura, T., Aoki, K., Sohn, B. J., Kim, S. W., Tsuruta, H., Sugimoto, N., Shimizu, A., Tanimoto, H., Sawa, Y., Lin, N. H., Lee, C. Te, Goto, D., and Schutgens, N.: Overview of the Atmospheric Brown Cloud East Asian Regional Experiment 2005 and a study of the aerosol direct radiative forcing in east Asia, J. Geophys. Res.-Atmos., 112, 1–23, https://doi.org/10.1029/2007JD009009, 2007.
Nigam, A., Welch, W., Wayne Miller, J., and Cocher III, D. R.: Effect of fuel sulphur content and control technology on PM emission from ship's auxiliary engine. Proceeding international aerosol conference, St. Paul, USA, 10–15 September 2006, 1531–1532, 2006.
NOAA Air Resources Laboratory: Air Resources Laboratory – HYSPLIT – Hybrid Single Particle Lagrangian Integrated Trajectory model, Real-time Environmental Applications and Display sYstem, available at: https://www.ready.noaa.gov/HYSPLIT.php, last access: 26 January 2020.
Office of Naval Research: Index of /ftp/outgoing/nrl/NAAPS-REANALYSIS/2011/201109, Global Ocean Data Assimilation Experiment, available at: https://nrlgodae1.nrlmry.navy.mil/ftp/outgoing/nrl/NAAPS-REANALYSIS/2011/201109/, last access: 26 January 2020.
O'Sullivan, D., Murray, B. J., Malkin, T. L., Whale, T. F., Umo, N. S., Atkinson, J. D., Price, H. C., Baustian, K. J., Browse, J., and Webb, M. E.: Ice nucleation by fertile soil dusts: relative importance of mineral and biogenic components, Atmos. Chem. Phys., 14, 1853–1867, https://doi.org/10.5194/acp-14-1853-2014, 2014.
Paatero, P. and Tapper, U.: Positive matrix factorization: A non-negative factor model with optimal utilization of error estimates of data values, Environmetrics, 5, 111–126, https://doi.org/10.1002/env.3170050203, 1994.
Paatero, P. and Hopke, P. K.: Discarding or downweighting high-noise variables in factor analytic models, Anal. Chim. Acta, 490, 277–289, https://doi.org/10.1016/S0003-2670(02)01643-4, 2003.
Paterson, K. G., Sagady, J. L., Hooper, D. L., Bertman, S. B., Carroll, M. A., and Shepson, P. B.: Analysis of air quality data using positive matrix factorization, Environ. Sci. Technol., 33, 635–641, https://doi.org/10.1021/es980605j, 1999.
Polissar, A. V., Hopke, P. K., Paatero, P., Malm, W. C., and Sisler, J. F.: Atmospheric aerosol over Alaska 2. Elemental composition and sources, J. Geophys. Res.-Atmos., 103, 19045–19057, https://doi.org/10.1029/98JD01212, 1998.
Prospero, J. M.: Long-range transport of mineral dust in the global atmosphere: Impact of African dust on the environment of the southeastern United States, P. Natl. Acad. Sci. USA, 96, 3396–3403, https://doi.org/10.1073/pnas.96.7.3396, 1999.
Raabe, O. G., Braaten, D. A., Axelbaum, R. L., Teague, S. V., and Cahill, T. A.: Calibration studies of the drum impactor, J. Aerosol Sci., 19, 183–195, https://doi.org/10.1016/0021-8502(88)90222-4, 1988.
Reid, J. S., Flocchini, R. G., Cahill, T. A., Ruth, R. S., and Salgado, D. P.: Local meteorological, transport, and source aerosol characteristics of late autumn Owens Lake (dry) dust storms, Atmos. Environ., 28, 1699–1706, https://doi.org/10.1016/1352-2310(94)90315-8, 1994.
Reid, J. S., Hyer, E. J., Prins, E. M., Westphal, D. L., Zhang, J., Wang, J., Christopher, S. A., Curtis, C. A., Schmidt, C. C., Eleuterio, D. P., Richardson, K. A., and Hoffman, J. P.: Global monitoring and forecasting of biomass-burning smoke: Description of and lessons from the fire Locating and Modeling of Burning Emissions (FLAMBE) program, IEEE J. Sel. Top. Appl. Earth Obs. Remote Sens., 2, 144–162, https://doi.org/10.1109/JSTARS.2009.2027443, 2009.
Reid, J. S., Xian, P., Hyer, E. J., Flatau, M. K., Ramirez, E. M., Turk, F. J., Sampson, C. R., Zhang, C., Fukada, E. M., and Maloney, E. D.: Multi-scale meteorological conceptual analysis of observed active fire hotspot activity and smoke optical depth in the Maritime Continent, Atmos. Chem. Phys., 12, 2117–2147, https://doi.org/10.5194/acp-12-2117-2012, 2012.
Reid, J., Hyer, E., Johnson, R., Holben, B. N., Yokelson, R., Zhang, J., Campbell, J., Christopher, S., DiGirolamo, L., Giglio, L., Holz, R., Kearney, C., Miettinen, J., Reid, E., Turk, F. J., Wang, J., Xian, P., Zhao, G., Balasubramanian, R., Chew, B. N., Janjai, S., Lagrosas, N., Lestari, P., Lin, N.-H., Mahmud, M., Nguyen, A. X., Norris, B., Oanth, N. T. K., Oo, M., Salinas, S. V., Welton, E. J., and Liew, S. C.: Observing and understanding the Southeast Asian aerosol system by remote sensing: An initial review and analysis for the Seven Southeast Asian Studies (7SEAS) program, Atmos. Res., 122, 403–468, https://doi.org/10.1016/j.atmosres.2012.06.005, 2013.
Reid, J. S., Lagrosas, N. D., Jonsson, H. H., Reid, E. A., Sessions, W. R., Simpas, J. B., Uy, S. N., Boyd, T. J., Atwood, S. A., Blake, D. R., Campbell, J. R., Cliff, S. S., Holben, B. N., Holz, R. E., Hyer, E. J., Lynch, P., Meinardi, S., Posselt, D. J., Richardson, K. A., Salinas, S. V., Smirnov, A., Wang, Q., Yu, L., and Zhang, J.: Observations of the temporal variability in aerosol properties and their relationships to meteorology in the summer monsoonal South China Sea/East Sea: the scale-dependent role of monsoonal flows, the Madden–Julian Oscillation, tropical cyclones, squall lines and cold pools, Atmos. Chem. Phys., 15, 1745–1768, https://doi.org/10.5194/acp-15-1745-2015, 2015.
Reid, J. S., Xian, P., Holben, B. N., Hyer, E. J., Reid, E. A., Salinas, S. V., Zhang, J., Campbell, J. R., Chew, B. N., Holz, R. E., Kuciauskas, A. P., Lagrosas, N., Posselt, D. J., Sampson, C. R., Walker, A. L., Welton, E. J., and Zhang, C.: Aerosol meteorology of the Maritime Continent for the 2012 7SEAS southwest monsoon intensive study – Part 1: regional-scale phenomena, Atmos. Chem. Phys., 16, 14041–14056, https://doi.org/10.5194/acp-16-14041-2016, 2016.
Ross, A. D., Holz, R. E., Quinn, G., Reid, J. S., Xian, P., Turk, F. J., and Posselt, D. J.: Exploring the first aerosol indirect effect over Southeast Asia using a 10-year collocated MODIS, CALIOP, and model dataset, Atmos. Chem. Phys., 18, 12747–12764, https://doi.org/10.5194/acp-18-12747-2018, 2018.
Rolph, G., Stein, A., and Stunder, B.: Real-time Environmental Applications and Display sYstem: READY, Environ. Model. Softw., 95, 210–228, https://doi.org/10.1016/j.envsoft.2017.06, 2017.
Santoso, M., Lestiani, D. D., Mukhtar, R., Hamonangan, E., Syafrul, H., Markwitz, A., and Hopke, P. K.: Preliminary study of the sources of ambient air pollution in Serpong, Indonesia, Atmos. Pollut. Res., 2, 190–196, https://doi.org/10.5094/apr.2011.024, 2011.
Schlosser, J. S., Braun, R. A., Bradley, T., Dadashazar, H., MacDonald, A. B., Aldhaif, A. A., Aghdam, M. A., Mardi, A. H., Xian, P., and Sorooshian, A.: Analysis of aerosol composition data for western United States wildfires between 2005 and 2015: Dust emissions, chloride depletion, and most enhanced aerosol constituents, J. Geophys. Res.-Atmos., 122, 8951–8966, https://doi.org/10.1002/2017JD026547, 2017.
Seneviratne, M. C. S., Waduge, V. A., Hadagiripathira, L., Sanjeewani, S., Attanayake, T., Jayaratne, N., and Hopke, P. K.: Characterization and source apportionment of particulate pollution in Colombo, Sri Lanka, Atmos. Pollut. Res., 2, 207–212, https://doi.org/10.5094/apr.2011.026, 2011.
Shi, Y., Zhang, J., Reid, J. S., Liu, B., and Hyer, E. J.: Critical evaluation of cloud contamination in the MISR aerosol products using MODIS cloud mask products, Atmos. Meas. Tech., 7, 1791–1801, https://doi.org/10.5194/amt-7-1791-2014, 2014.
Song, Y., Xie, S., Zhang, Y., Zeng, L., Salmon, L. G., and Zheng, M.: Source apportionment of PM2.5 in Beijing using principal component analysis/absolute principal component scores and UNMIX, Sci. Total Environ., 372, 278–286, https://doi.org/10.1016/j.scitotenv.2006.08.041, 2006.
Sorooshian, A., Feingold, G., Lebsock, M. D., Jiang, H., and Stephens, G. L.: On the precipitation susceptibility of clouds to aerosol perturbations, Geophys. Res. Lett., 36, L13803, https://doi.org/10.1029/2009GL038993, 2009.
Stein, A. F., Draxler, R. R, Rolph, G. D., Stunder, B. J. B., Cohen, M. D., and Ngan, F.: NOAA's HYSPLIT atmospheric transport and dispersion modeling system, B. Am. Meteor. Soc., 96, 2059–2077, https://doi.org/10.1175/BAMS-D-14-00110.1, 2015.
Tian, S. L., Pan, Y. P., and Wang, Y. S.: Size-resolved source apportionment of particulate matter in urban Beijing during haze and non-haze episodes, Atmos. Chem. Phys., 16, 1–19, https://doi.org/10.5194/acp-16-1-2016, 2016.
Twohy, C. H., Kreidenweis, S. M., Eidhammer, T., Browell, E. V., Heymsfield, A. J., Bansemer, A. R., Anderson, B. E., Chen, G., Ismail, S., DeMott, P. J., and Van Den Heever, S. C.: Saharan dust particles nucleate droplets in eastern Atlantic clouds, Geophys. Res. Lett., 36, L01807, https://doi.org/10.1029/2008GL035846, 2009.
Van Pinxteren, D., Fomba, K. W., Spindler, G., Müller, K., Poulain, L., Iinuma, Y., Löschau, G., Hausmann, A., and Herrmann, H.: Regional air quality in Leipzig, Germany: Detailed source apportionment of size-resolved aerosol particles and comparison with the year 2000, Faraday Discuss., 189, 291–315, https://doi.org/10.1039/c5fd00228a, 2016.
Venecek, M. A., Zhao, Y., Mojica, J., McDade, C. E., Green, P. G., Kleeman, M. J., and Wexler, A. S.: Characterization of the 8-stage Rotating Drum Impactor under low concentration conditions, J. Aerosol Science, 100, 140–154, https://doi.org/10.1016/j.jaerosci.2016.07.007, 2016.
Viana, M., Kuhlbusch, T. A. J., Querol, X., Alastuey, A., Harrison, R. M., Hopke, P. K., Winiwarter, W., Vallius, M., Szidat, S., Prévôt, A. S. H., Hueglin, C., Bloemen, H., Wåhlin, P., Vecchi, R., Miranda, A. I., Kasper-Giebl, A., Maenhaut, W., and Hitzenberger, R.: Source apportionment of particulate matter in Europe: A review of methods and results, J. Aerosol Sci., 39, 827–849, https://doi.org/10.1016/j.jaerosci.2008.05.007, 2008.
Wang, S.-H., Tsay, S.-C., Lin, N.-H., Hsu, N. C., Bell, S. W., Li, C., Ji, Q., Jeong, M.-J., Hansell, R. A., Welton, E. J., Holben, B. N., Sheu, G.-R., Chu, Y.-C., Chang, S.-C., Liu, J.-J., and Chiang, W.-L.: First detailed observations of long-range transported dust over the northern South China Sea, Atmos. Environ., 45, 4804–4808, https://doi.org/10.1016/j.atmosenv.2011.04.077, 2011.
Wang, Z., Sorooshian, A., Prabhakar, G., Coggon, M. M., and Jonsson, H. H.: Impact of emissions from shipping, land, and the ocean on stratocumulus cloud water elemental composition during the 2011 E-PEACE field campaign,
Wimolwattanapun, W., Hopke, P. K. and Pongkiatkul, P.: Source apportionment and potential source locations of PM2.5 and PM2.5−10 at residential sites in metropolitan Bangkok, Atmos. Pollut. Res., 2, 172–181, https://doi.org/10.5094/apr.2011.022, 2011.
Witt, M., Baker, A. R., and Jickells, T. D.: Atmospheric trace metals over the Atlantic and South Indian Oceans: Investigation of metal concentrations and lead isotope ratios in coastal and remote marine aerosols, Atmos. Environ., 40, 5435–5451, https://doi.org/10.1016/j.atmosenv.2006.04.041, 2006.
Xian, P., Reid, J. S., Atwood, S. A., Johnson, R. S., Hyer, E. J., Westphal, D. L., and Sessions, W.: Smoke aerosol transport patterns over the Maritime Continent, Atmos. Res., 122, 469–485, https://doi.org/10.1016/j.atmosres.2012.05.006, 2013.
Xie, R. K., Seip, H. M., Liu, L., and Zhang, D. S.: Characterization of individual airborne particles in Taiyuan City, China, Air Qual. Atmos. Heal., 2, 123–131, https://doi.org/10.1007/s11869-009-0039-x, 2009.
Yusuf, A. A. and Francisco, H.: Climate change vulnerability mapping for Southeast Asia, Economy and Environment Program for Southeast Asia (EEPSEA) report, available at: http://www.eepsea.net/ (last access: July 2014), 32 pp., 2009.
Zhang, J. and Reid, J. S.: MODIS Aerosol Product Analysis for Data Assimilation: Assessment of Level 2 Aerosol Optical Thickness Retrievals, J. Geophys. Res.-Atmos., 111, 22207, https://doi.org/10.1029/2005JD006898, 2006.
Zheng, C., Zhao, C., Zhu, Y., Wang, Y., Shi, X., Wu, X., Chen, T., Wu, F., and Qiu, Y.: Analysis of influential factors for the relationship between PM2.5 and AOD in Beijing, Atmos. Chem. Phys., 17, 13473–13489, https://doi.org/10.5194/acp-17-13473-2017, 2017.
- Abstract
- Introduction
- Sampling and methods
- Results I: mass distributions and time series of selected elements
- Results II: positive matrix factorization and regressions
- Results III: back trajectory analysis
- Summary and conclusions
- Data availability
- Author contributions
- Competing interests
- Acknowledgements
- Review statement
- References
- Supplement
- Abstract
- Introduction
- Sampling and methods
- Results I: mass distributions and time series of selected elements
- Results II: positive matrix factorization and regressions
- Results III: back trajectory analysis
- Summary and conclusions
- Data availability
- Author contributions
- Competing interests
- Acknowledgements
- Review statement
- References
- Supplement