the Creative Commons Attribution 4.0 License.
the Creative Commons Attribution 4.0 License.
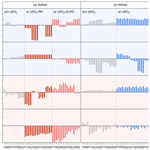
Model bias in simulating major chemical components of PM2.5 in China
Ruqian Miao
Yan Zheng
Xi Cheng
Paul I. Palmer
Manish Shrivastava
Jianping Guo
Qiang Zhang
Yuhan Liu
Zhaofeng Tan
Xuefei Ma
Shiyi Chen
Limin Zeng
Keding Lu
Yuanhang Zhang
High concentrations of PM2.5 (particulate matter with an aerodynamic diameter less than 2.5 µm) in China have caused severe visibility degradation. Accurate simulations of PM2.5 and its chemical components are essential for evaluating the effectiveness of pollution control strategies and the health and climate impacts of air pollution. In this study, we compared the GEOS-Chem model simulations with comprehensive datasets for organic aerosol (OA), sulfate, nitrate, and ammonium in China. Model results are evaluated spatially and temporally against observations. The new OA scheme with a simplified secondary organic aerosol (SOA) parameterization significantly improves the OA simulations in polluted urban areas, highlighting the important contributions of anthropogenic SOA from semivolatile and intermediate-volatility organic compounds. The model underestimates sulfate and overestimates nitrate for most of the sites throughout the year. More significant underestimation of sulfate occurs in winter, while the overestimation of nitrate is extremely large in summer. The model is unable to capture some of the main features in the diurnal pattern of the PM2.5 chemical components, suggesting inaccuracies in the presented processes. Potential model adjustments that may lead to a better representation of the boundary layer height, the precursor emissions, hydroxyl radical concentrations, the heterogeneous formation of sulfate and nitrate, and the wet deposition of nitric acid and nitrate have been tested in the sensitivity analysis. The results show that uncertainties in chemistry perhaps dominate the model biases. The proper implementation of heterogeneous sulfate formation and the good estimates of the concentrations of sulfur dioxide, hydroxyl radical, and aerosol liquid water are essential for the improvement of the sulfate simulation. The update of the heterogeneous uptake coefficient of nitrogen dioxide significantly reduces the modeled concentrations of nitrate. However, the large overestimation of nitrate concentrations remains in summer for all tested cases. The possible bias in the chemical production and the wet deposition of nitrate cannot fully explain the model overestimation of nitrate, suggesting issues related to the atmospheric removal of nitric acid and nitrate. A better understanding of the atmospheric nitrogen budget, in particular, the role of the photolysis of particulate nitrate, is needed for future model developments. Moreover, the results suggest that the remaining underestimation of OA in the model is associated with the underrepresented production of SOA.
- Article
(2212 KB) - Full-text XML
-
Supplement
(1158 KB) - BibTeX
- EndNote
In developing countries like China and India, the concentrations of PM2.5 (particulate matter with an aerodynamic diameter less than 2.5 µm) often exceed air-quality standards, leading to visibility reduction and negative health effects (Chan and Yao, 2008; Lelieveld et al., 2015). Chemical transport models (CTMs) are valuable tools to evaluate the PM2.5 pollution and its health and climate impacts. Studies have shown that the CTMs can reproduce the spatial and temporal variations of the surface PM2.5 concentrations in China. For example, the Weather Research and Forecasting and Community Multiscale Air Quality (WRF/CMAQ) model has reproduced the monthly averaged concentrations of PM2.5 at the air-quality sites in 60 Chinese cities (J. Hu et al., 2016). The MICS-Asia Phase III studies further show the normalized mean biases (NMBs) of less than 50 % for daily or monthly mean PM2.5 concentrations for various CTMs (Gao et al., 2018; Chen et al., 2019). However, when the simulations of PM2.5 components have compensating errors, the model still reproduces the PM2.5 mass and biases the evaluation of the effectiveness of the emission control strategies. Model evaluations in China have reached an agreement that the CTMs generally underestimate the concentrations of organic aerosol (OA) (Fu et al., 2012; Han et al., 2016) and sulfate (Wang et al., 2014; G. J. Zheng et al., 2015) but overestimate the concentrations of nitrate (Wang et al., 2013; Chen et al., 2019). The underestimation of OA and the overestimation of nitrate are also present in the studies for the United States and Europe, while the sulfate concentrations are reproduced in those regions (Heald et al., 2012; Drugé et al., 2019; Jiang et al., 2019).
Uncertainties exist in meteorological fields, emission inventories, and the physical and chemical processes, which contribute to the model biases in the PM2.5 simulations. For example, models are well recognized to reproduce temperature (T) and relative humidity (RH), but it is difficult for them to capture the near-surface wind fields (Guo et al., 2016a; Gao et al., 2018; J. Hu et al., 2016). Boundary layer structures greatly affect the PM2.5 concentrations (Z. Li et al., 2017; Su et al., 2018). Evaluations of the boundary layer (e.g., boundary layer height, BLH) in the CTMs are limited (Bei et al., 2017; Chen et al., 2016). For primary PM2.5 and the secondary precursors of PM2.5, the uncertainties of their emissions in Asia are large, ranging from tens to several hundreds of percent (M. Li et al., 2017b). The bottom-up and top-down estimates of the emissions of sulfur dioxide (SO2), nitrogen oxides (NOx), ammonia (NH3), volatile organic compounds (VOCs), and organic carbon (OC) show significant differences in magnitude and seasonal variability (Koukouli et al., 2018; Qu et al., 2019; L. Zhang et al., 2018; Cao et al., 2018; Fu et al., 2012).
For sulfate, the model underestimation has been attributed largely to the heterogeneous production. The proposed heterogeneous formation mechanisms include the SO2 oxidation by nitrogen dioxide (NO2) directly (Cheng et al., 2016; Wang et al., 2016) or indirectly (L. Li et al., 2018), by O2 via transition metal ion (TMI) catalysis (G. Li et al., 2017) or radical chain reactions (Hung and Hoffmann, 2015; Hung et al., 2018), and by hydrogen peroxide (Ye et al., 2018). Among them, TMI-catalyzed oxidation of SO2 perhaps dominates the sulfate formation during the haze periods, constrained by the observations of sulfate oxygen isotopes (Shao et al., 2019). The mechanisms are still under debate. The heterogeneous formation can however be simplified in models as a reactive uptake process to achieve a better agreement of sulfate concentrations (Wang et al., 2014; G. J. Zheng et al., 2015; J. Li et al., 2018). Similar to sulfate, the contribution of heterogeneous chemistry to the nitrate formation remains unclear. The uptake coefficients of dinitrogen pentoxide (N2O5), NO2, and nitrate radical (NO3•) are sensitive to experimental conditions and range by orders of magnitude (Bertram and Thornton, 2009; McDuffie et al., 2018; Brown and Stutz, 2012; Spataro and Ianniello, 2014). The parameterizations of nitrate heterogeneous production differ significantly among models (Holmes et al., 2019; Alexander et al., 2020; J. Li et al., 2018; Wang et al., 2012).
For OA, the complexity of its secondary formation and aging processes and the lack of emission inventories of intermediate-volatility (IVOCs) and semivolatile organic compounds (SVOCs) affect the model performance (Chen et al., 2017, and references therein). Substantial model–observation discrepancies are present in the comparisons of the mass concentration and the oxidation state of OA as well as the contributions of various formation pathways (Tsigaridis et al., 2014; Heald et al., 2011; Chen et al., 2015). Moreover, the oxidant levels affect the chemical processes (Lu et al., 2018). The model capability in simulating the concentrations of major oxidants like hydroxyl radical (OH•) and hydroperoxy radical (HO2•) is rarely evaluated. In addition, unusual biases of the meteorological fields and chemical processes may occur during the severe haze periods (daily mean PM2.5>75 µg m−3) (An et al., 2019). The models often significantly underestimate the PM2.5 concentrations during the haze events (Wang et al., 2014; G. J. Zheng et al., 2015). Various model biases from meteorology, emissions, and the physical and chemical processes interact with each other nonlinearly. It is therefore important to evaluate the model for all individual components of PM2.5.
On the other hand, observations may be biased, which is rarely considered when evaluating the model–observation discrepancies. For example, filter-based analysis of the PM2.5 components can contain positive or negative artifacts for semivolatile species, resulting from improper use of denuder and backup filters (Liu et al., 2014). Such artifacts are often large (>50 %) (Chow, 1995) and have been ignored in most model–observation comparisons (Wang et al., 2013; Qin et al., 2015). Online measurements by aerosol mass spectrometers have less uncertainty (∼30 %) compared to filter-based analysis (Canagaratna et al., 2007). Most of the measurements are however conducted for submicron particles (DeCarlo et al., 2006; Ng et al., 2011). The particulate mass in the supermicron domain needs to be considered in the model–observation comparisons (Elser et al., 2016).
In this study, we synthesized a comprehensive dataset of the concentrations of major PM2.5 components (i.e., sulfate, nitrate, ammonium, and OA) from 55 online measurements at urban sites and 22 at non-urban sites in China. The latest version of GEOS-Chem nested-grid model simulations was evaluated with this dataset as well as a long-term online dataset that consists of hourly measurements of the major PM2.5 components from 2011 to 2013 in Beijing. Potential factors that may contribute to the model–observation gaps were discussed. Sensitivity analyses were conducted for two case periods to evaluate the contributions of the individual potential factors to the model–observation gaps and the contributions of various combinations of these factors.
The campaign-average mass concentrations of sulfate, nitrate, ammonium, and OA as well as the sampling information are listed in Table S1 of the Supplement, including 77 surface online measurements from 2006 to 2016 in China. The dataset covers the regions of the North China Plain (NCP), Yangtze River Delta (YRD), Pearl River Delta (PRD), and Northwest China (NW). The measurements were made using an Aerodyne high-resolution time-of-flight aerosol mass spectrometer (HR-ToF-AMS), quadrupole aerosol mass spectrometer (Q-AMS), and aerosol chemical speciation monitor (ACSM), which are mostly for submicron particles (Y. J. Li et al., 2017). The long-term ACSM measurements of submicron particle composition at an urban site in the Institute of Atmospheric Physics, Beijing (IAP; 39∘58′28′′ N, 116∘22′16′′ E) from July 2011 to May 2013 are also used herein (Sun et al., 2015). The long-term data have a time resolution of 15 min and were averaged to an hour for comparison with the model results. All data were corrected by collection efficiency as stated in the original publications. Model results plausibly represent fine particles not submicron portion in polluted environments. The submicron-to-fine mass ratios are about 0.8 for sulfate, nitrate, ammonium, and OA in summer and winter in the NCP and may decrease to 0.5 during the severe haze episodes under high RH (Fig. S1 in the Supplement) (Zheng et al., 2020; Sun et al., 2020). We therefore divided the submicron observation data by 0.8 for the four species when comparing to the model results.
The meteorological parameters (e.g., T, RH, wind speed, and wind direction) and the concentrations of gaseous pollutants including ozone (O3), carbon monoxide (CO), SO2, and NO2 were measured at the Peking University Urban-Atmosphere Environment Monitoring Station (PKUERS; 39∘59′21′′ N, 116∘18′25′′ E) from July 2011 to May 2013. Both the IAP and PKUERS sites are in the same GEOS-Chem model grid. The monthly mean NH3 concentrations are taken from the 2007–2010 observations at the IAP site (Pan et al., 2012). The BLH in Beijing (39∘48′00′′ N, 116∘28′12′′ E) was derived from the radiosonde observations at 08:00, 14:00 (only in the summer), and 20:00 during July 2011 to May 2013 by using the bulk Richardson number method (Guo et al., 2016b, 2019). All the times of day given in the paper refer to Beijing time (UTC+8). The radiosonde-derived BLH is greater in spring and summer and lower in autumn and winter, which is consistent with the findings from the satellite observations and the ground-based ceilometer measurements (W. Zhang et al., 2016; Tang et al., 2016).
Moreover, the observed concentrations of OH• and HO2•, gaseous nitrous acid (HONO) and nitric acid (HNO3), and isoprene in Beijing are taken from literature, including the studies in south Beijing (Wangdu; 38∘39′36′′ N, 115∘12′00′′ E) from 7 June to 8 July 2014 and in north Beijing (Huairou; 40∘24′36′′ N, 116∘40′48′′ E) from 6 January to 5 March 2016 (Tan et al., 2017, 2018; Liu et al., 2019), and additional isoprene measurements at the PKUERS site during the summer of 2011 (Zhang et al., 2014). The observed concentrations of NO3• and aromatic compounds are taken from the measurements at the PKUERS site in September 2016 (Wang et al., 2017a) and in summer and winter of 2011–2012 (Wang et al., 2015), respectively.
The atmospheric chemical transport model GEOS-Chem 12.0.0 (https://doi.org/10.5281/zenodo.1343547) was run at nested grids with horizontal resolution over Asia and the adjacent area (11∘ S–55∘ N, 60–150∘ E) and 47 vertical levels between the surface and ∼0.01 hPa. Boundary conditions were provided by the global simulations at horizontal resolution. Both global and nested simulations were spun up for 1 month. MERRA2 reanalysis meteorological data from the NASA Global Modeling and Assimilation Office (GMAO) were used to drive the model. For comparisons with long-term observations at IAP, the model simulations were performed for the ACSM measurement periods. When comparing with the campaign-average data, the model simulations for the year 2012 were used. For other comparisons, the model simulations were run for the measurement periods.
The GEOS-Chem model simulates the ozone–NOx–hydrocarbon–aerosol chemistry (Park et al., 2003, 2004; Liao et al., 2007). Aerosol thermodynamic equilibrium is performed by ISORROPIA-II (Fountoukis and Nenes, 2007; Pye et al., 2009). The simulation of OA includes primary organic aerosol (POA) and secondary organic aerosol (SOA). The model assumes that 50 % of POA emitted from combustion sources is hydrophobic, and hydrophobic POA converts to hydrophilic POA with an e-folding time of 1.15 d. A ratio of 1.6 is applied to account for the non-carbon mass in POA (Turpin et al., 2000). SOA is simulated by the so-called “simple” SOA scheme (Hodzic and Jimenez, 2011; Kim et al., 2015). SOA precursor surrogates are estimated from the emissions of biogenic volatile organic compounds (i.e., isoprene and terpenes) and CO from the combustion of biomass, biofuel, and fossil fuel. The simple SOA scheme assumes that the irreversible conversion from precursors to particle-phase SOA takes a fixed timescale of 1 d and that 50 % of biogenic SOA precursors are emitted as particle-phase SOA. The SOA yields of isoprene and terpenes are set to be 3 % and 10 %, respectively. The SOA yield of biomass burning emissions is set to be 1.3 % of CO, and the yield for fossil-fuel combustion is set to be 6.9 %. These yields are derived from the observed ratios between SOA and CO in aged air masses from the studies in the United States (Hayes et al., 2015) and are able to reproduce the OA mass without detailed SOA chemistry in the southeast United States (Kim et al., 2015). Because of the lack of related measurements in China, we did not change these yields herein.
The wet deposition of soluble aerosols and gases includes convective updraft, rainout, and washout as described by Liu et al. (2001). SOA is treated as being highly soluble with a fixed Henry's law coefficient of 105 M atm−1 and a scavenging efficiency of 80 % (Chung and Seinfeld, 2002). The Henry's law coefficients may vary in magnitude, depending on the SOA types (Hodzic et al., 2014). However, Hodzic et al. (2016) show that the modeled SOA masses are not sensitive to the changes of Henry's law coefficients. Dry deposition is calculated by a standard resistance-in-series model for the aerodynamic boundary layer and canopy surface resistance (Wesely, 1989).
Heterogeneous uptake of SO2 into aerosol liquid water is not included in the standard simulations but in the sensitivity runs in Sect. 4.3. The parameterizations of the SO2 uptake coefficient () include depending on RH or on aerosol liquid water content (ALWC) (B. Zheng et al., 2015; J. Li et al., 2018). The heterogeneous uptake of N2O5 and NO2 is an important contributor to nitrate in northern China (Wang et al., 2017b; Wen et al., 2018). The uptake coefficients of and on the aerosol surface vary by several orders of magnitude, depending on temperature, particle-phase state, composition, ALWC, pH, and so on (Bertram and Thornton, 2009; Abbatt et al., 2012; McDuffie et al., 2018, and references therein). The standard model uses relatively high values of and (McDuffie et al., 2018; Davis et al., 2008). Lower values are tested in the sensitivity runs. For SOA, the heterogeneous formation varies by sources and aging processes (Donahue et al., 2012). Heterogeneous production of SOA is not tested in the model because of the lack of good parameterizations (Chen et al., 2017).
Global anthropogenic emissions in GEOS-Chem are provided by the Community Emissions Data System (CEDS) (Hoesly et al., 2018), including the monthly emissions of gaseous pollutants (SO2, NOx, NH3, CH4, CO, and VOCs) and carbonaceous aerosols (black carbon (BC) and OC). Anthropogenic emissions of CO, NOx, SO2, BC, OC, and VOCs in China are provided by the Multi-resolution Emission Inventory for China (MEIC v1.3; http://meicmodel.org, last access: 23 October 2020) for the years 2010, 2012, 2014, and 2016. The emissions in 2011, 2013, and 2015 are interpolated from the emissions of the 2 adjacent years. We use an improved inventory for agriculture emissions of NH3 in China (L. Zhang et al., 2018). This inventory shows stronger peak emissions in the summer than other inventories such as the Regional Emission in Asia (REAS2), PKU-NH3, and the Emission Database for Global Atmospheric Research (EDGAR), which agrees better with the top-down estimates (L. Zhang et al., 2018). The non-agricultural NH3 emissions in China are taken from the study done by Huang et al. (2012), which is based on the year 2006 and represents the low-end estimates as the emissions increased rapidly after 2006 (Kang et al., 2016; Meng et al., 2017). Table S2 lists the total anthropogenic emissions of primary PM2.5 and the gaseous precursors of PM2.5 in China for 2012. The MIX Asian emission inventories are used for the anthropogenic emissions in the rest of Asia (M. Li et al., 2017b), which has combined the South Korea inventory (CAPSS; Lee et al., 2011), the Indian inventory (ANL-India; Lu et al., 2011; Lu and Streets, 2012), and the REAS2 inventory (Kurokawa et al., 2013). Our simulations used sector-specific MEIC diurnal patterns for the anthropogenic emissions of CO, NOx, SO2, BC, OC, and VOCs from power, industry, residential, transportation, and agriculture sectors (Fig. S2) and the MEIC agriculture diurnal patterns for all anthropogenic emissions of NH3 in China. NOx emission from soils and lightning are included in the model (Hudman et al., 2012; Murray et al., 2012). The biogenic emissions are calculated from the Model of Emissions of Gases and Aerosols from Nature (MEGAN v2.1) (Guenther et al., 2012). The emissions from biomass burning are provided by the Global Fire Emission Database (GFED4) (Giglio et al., 2013).
4.1 Compensating errors from simulations of individual PM2.5 components
Figure 1 shows the scatterplots of the simulated and the observed campaign-average concentrations of secondary inorganic aerosol (SIA) and OA over China as well as the statistical values such as NMB, root mean square error (RMSE), and Pearson's correlation coefficient (R) for the model–observation comparisons. For most of the sites, the sulfate concentrations are underestimated (NMB = −0.39, R=0.45), while the nitrate concentrations are overestimated (NMB = 0.82, R=0.57) by the model. Such underestimation for sulfate and the overestimation for nitrate in CTMs is a known problem for China (Gao et al., 2018; Chen et al., 2019). The model performance for ammonium (NMB = 0.06, R=0.58) is better than the performances for sulfate and nitrate. For OA, previous model studies typically underestimate the OA mass concentrations by over 40 % (Fu et al., 2012; Zhao et al., 2016). The simple SOA scheme shows improved performance on OA for all seasons (NMB = −0.26, R=0.70).
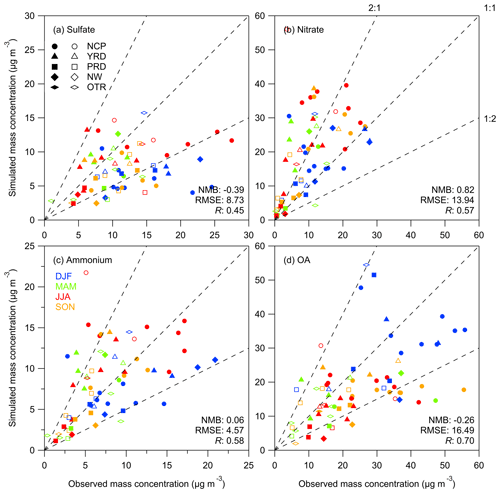
Figure 1Scatterplots of the simulated and observed campaign-average mass concentrations of (a) sulfate, (b) nitrate, (c) ammonium, and (d) OA in China. The solid and open symbols represent the urban and non-urban sites, respectively. Colors and shapes of the symbols represent seasons and regions, respectively. The observations were conducted during 2006 to 2016 for submicron particles, and the data were divided by a submicron-to-fine ratio of 0.8. The model simulations were run for the year 2012.
On a seasonal basis, the underestimation of sulfate occurs all year round, and the greatest underestimation occurs in winter (NMB = −0.54) (Fig. 1a and Table S3). The seasonality of the model bias is partially explained by the underestimation of SO2 emissions in winter (Wang et al., 2014; Koukouli et al., 2018). Similar to other models, our model fails to reproduce the high sulfate concentrations during the haze periods because of the underrepresented heterogeneous production (Wang et al., 2014; G. J. Zheng et al., 2015). Severe haze events occurred more often in winter in China, contributing to the seasonality of the model bias. By contrast, the nitrate concentrations are largely overestimated in all seasons, especially in spring, summer, and autumn (NMB = 0.79–1.28). Wang et al. (2013) showed the summertime overestimation for East Asia. Heald et al. (2012) showed the summertime, autumn time, and wintertime overestimation for the eastern United States. The model bias is smaller in winter (NMB = 0.41) when higher concentrations of nitrate are present (Fig. 1b). For ammonium, the model underestimates its concentrations in winter and spring but overestimates its concentrations in summer and autumn. Both the uncertainties of HNO3 and NH3 simulations may affect the modeled ammonium concentrations (Wen et al., 2018; Xu et al., 2019). Similar to sulfate, the underestimation of OA occurs all year round, and the model performs worst in autumn. Biases in the precursor emissions as well as the assumed nonseasonal conversion rate from precursors to particle-phase SOA are the possible reasons for the seasonality of the OA bias. The R value is much lower in summer (i.e., 0.28 compared with ≥0.49 in other seasons), showing more complexity of the biases in the OA simulations.
Tables S4 and S5 list the statistical values for the model–observation comparisons in different regions and urban versus non-urban sites, respectively. The model biases for sulfate, nitrate, and OA are consistently positive or negative among regions (Table S4), suggesting that the model biases are general problems in China. The underestimation of sulfate is over 40 % (NMB) in most regions except YRD, and the overestimation of nitrate is over 80 % (NMB) in most regions except NW. The OA simulations show much lower NMB (−10 %) and RMSE values in YRD and PRD than in NCP and NW. For ammonium, the model significantly overestimates its concentrations in YRD and underestimates its concentrations in NW. The former may be explained by the excessive formation of ammonium nitrate and ammonium sulfate through thermodynamic equilibrium under conditions of abundant NH3 in YRD in the model (L. Zhang et al., 2018). The latter is likely a result of combined factors including emissions, meteorology, and thermodynamic equilibrium. Moreover, the mean observed concentrations of sulfate, nitrate, ammonium, and OA at urban sites are 20 %–90 % greater than those at non-urban sites (Fig. 1 and Table S5). The model shows greater simulated concentrations of nitrate, ammonium, and OA at urban sites, which is consistent with the observations. The model–observation gaps for nitrate (NMB = 1.22) and ammonium (NMB = 0.33) are greater in non-urban areas, whereas the gaps for sulfate (NMB = −0.44) and OA (NMB = −0.31) are greater in urban areas. This result suggests perhaps different driving forces of the model biases for the SIA species.
The model simulations are further compared with the 2-year hourly observations in Beijing. Figure S3 shows the simulation-to-observation ratios for the SIA species and OA. The mean and median values of the simulation-to-observation ratios of the mass concentrations of non-refractory PM2.5 (NR-PM2.5) are generally within the measurement uncertainty of 30 %. Compensation of the underestimation of sulfate and OA by the overestimation of nitrate leads to the good performance on NR-PM2.5. The seasonal variations of the model biases for the SIA species and OA in Beijing are consistent with the findings in the nationwide comparisons (Table S3), except that the greatest underestimation of OA occurs in spring instead of autumn. Figure S4 shows the simulation-to-observation ratios when excluding the periods of NR-PM2.5 mass concentrations over 150 µg m−3. During these periods, the submicron-to-fine ratios may decrease from 0.8 (used herein) to 0.5. The model biases and their seasonal variations in Fig. S4 are similar to the previous results, suggesting insignificant impacts of severe haze periods on the statistic evaluations.
Figure 2 shows the diurnal patterns of the observed and the simulated concentrations of sulfate, nitrate, ammonium, and OA for four seasons in Beijing. Considerable differences exist. For instance, the observed sulfate shows a daytime concentration buildup (2–4 µg m−3) in spring and summer, suggesting a photochemical production (Sun et al., 2015). The wintertime diurnal pattern shows a steady but later enhancement (∼5 µg m−3) in the afternoon. The simulated profiles show fewer daytime concentration elevations (0–2 µg m−3), suggesting insufficient production, overestimated boundary layer dilution, or removal during the day in the model (Fig. 2a). By contrast, the observed diurnal variations of hourly mean nitrate and ammonium concentrations are less than sulfate (Fig. 2b–c). The concentrations of simulated nitrate that are 2–5 times greater at night suggest overpredicted nighttime production, underestimated boundary layer dilution, or underestimated atmospheric removal of nitrate. Nighttime production of nitrate by the heterogeneous uptake of N2O5 and NO2 is an important pathway of nitrate production in northern China (Wang et al., 2018; Alexander et al., 2020). On the other hand, the simulated profiles for nitrate and ammonium show large reductions of daytime concentrations, especially in summer, which are not shown in the observed profiles. The model largely overestimates daytime gaseous HNO3 concentrations in Beijing (Fig. S5). The total nitrate (particulate nitrate + gaseous HNO3) accumulated excessively in the model simulations, which needs further investigations.
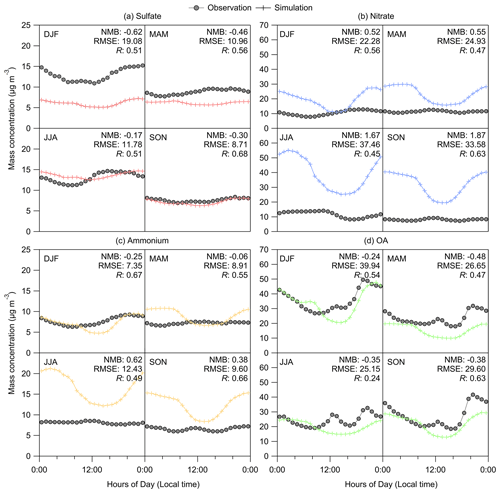
Figure 2Diurnal profiles of the simulated and observed hourly mean concentrations of (a) sulfate, (b) nitrate, (c) ammonium, and (d) OA at the IAP site in Beijing from July 2011 to May 2013. The observed concentrations were divided by a submicron-to-fine ratio of 0.8.
For OA, the model is unable to reproduce the midday and evening peaks for all seasons (Fig. 2d). Previous positive matrix factorization (PMF) analysis of the OA mass spectra suggests that cooking emissions contribute to the midday peaks of the OA concentrations and that the evening peaks are driven by mixed primary emissions including cooking, traffic, and coal combustion (W. Hu et al., 2016; Sun et al., 2015). Cooking emissions are not included explicitly in the model, and the emissions of POA and SOA precursors from traffic and coal combustion are uncertain (Tao et al., 2018; Peng et al., 2019). We compared the modeled POA and SOA with PMF-derived POA and oxygenated OA (OOA) (Sun et al., 2018). The model reproduces the monthly mean concentrations of PMF-derived POA (Fig. 3a), suggesting that the MEIC POA inventory generally represents the particle-phase SVOCs emissions under ambient conditions. The model underestimation of OA is mainly from SOA as indicated by the underestimation of the monthly mean concentrations of PMF-derived OOA (i.e., 50 %–70 % of the observed OA mass) (Fig. 3b). Figures S6 and S7 show the model performance of the simple SOA scheme and the traditional scheme (so-called “semivolatile POA scheme” in GEOS-Chem) in simulating OA. The semivolatile POA scheme significantly underestimates both POA and SOA. This scheme treats 1.27 times of the POA inventory as the emissions of SVOCs, among which only 1.5 % of the carbon remains as POA (Pye and Seinfeld, 2010). There is also a lack of constraints on the SOA production from IVOCs and SVOCs. In addition, the modeled profiles show earlier morning peaks of OA, especially in winter, which are mainly caused by the POA emissions of the residential sector. The lack of this feature in the observed OA mass suggests perhaps an overestimation of this source.
4.2 Potential contributors to the model–observation discrepancies
We focus here on measurements in Beijing to discuss the potential contributors to the model bias. Table 1 lists the statistics including mean bias (MB), NMB, and RMSE of T, RH, wind speed, wind direction, and BLH between the MERRA2 outputs and the observations in Beijing. The MERRA2 reanalysis reproduces T (NMB < 2 %) and RH (NMB < 15 % except for winter) but is unable to reproduce the wind speed and direction. Large RMSE for surface wind directions is a common problem in meteorological reanalysis products as well as the WRF simulations. The overestimation of wind speed (1–2 times) is slightly greater than the bias reported in other studies and may cause some underestimation of PM2.5 (J. Hu et al., 2016; Wang et al., 2014). The MERRA2 slightly overestimates BLH at 14:00 compared with the radiosonde measurements in summer (NMB = 0.34). For 08:00 and 20:00, MERRA2 underestimates the radiosonde-derived BLH in autumn and winter. Bei et al. (2017) indicated that the uncertainties in temperature and wind field simulations lead to the frequent underestimation of the nighttime BLH in January 2014 in Beijing by the ensemble WRF meteorology. Such underestimation of BLH may lead to overestimated nighttime concentrations of PM2.5 in autumn and winter. The large RMSE values for the BLH comparisons at 08:00 and 20:00 suggest that the nighttime simulation of PM2.5 may have greater meteorological uncertainty than the daytime simulation (Fig. S8 and Table 1).
Table 1Comparisons of the observed and simulated meteorological parameters, including T, RH, wind speed, wind direction, and BLH, for the four seasons during the period of July 2011 to May 2013 at the PKUERS site. “OBS” and “SIM” represent the mean values of the observations and simulations, respectively.
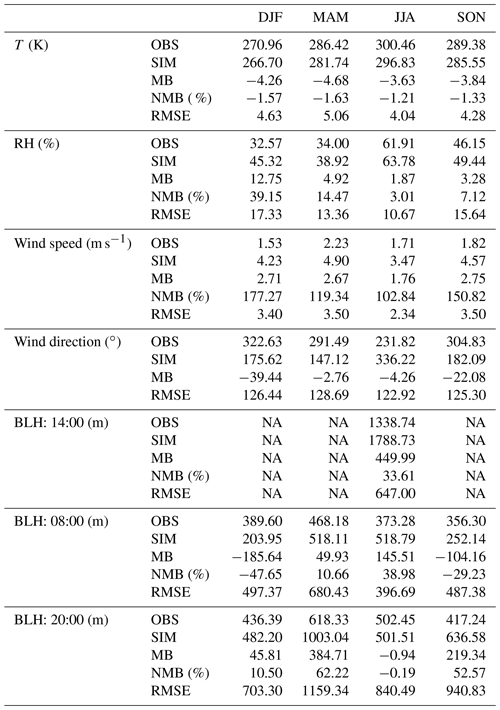
NA – not available.
The uncertainties related with the emission data including their temporal profiles are considered to be important sources of inaccuracies in modeled concentrations (M. Li et al., 2017a). The uncertainty of SO2 emissions affects surface sulfate concentrations. Our model underestimates SO2 concentrations in winter and overestimates its concentrations in summer in Beijing (Fig. 4a). Consistently, top-down estimates suggest lower SO2 emissions in summer and higher in winter in China compared with the MEIC inventory (Koukouli et al., 2018). Improving SO2 emissions may reduce the model bias for sulfate. Our model largely underestimates NO2 concentrations year round (Fig. 4b). The bottom-up NOx inventory has about 50 % uncertainty (M. Li et al., 2017b). Top-down estimates suggest however lower NO2 emissions in Beijing and its surrounding area than the MEIC inventory (Qu et al., 2017), which conflicts with the underestimation of NO2. The relatively coarse model grid is probably a reason for the low modeled NO2 concentrations at the site. Moreover, laboratory and field measurements show that the NO2 uptake coefficient () on the aerosol surface ranges from 10−8 to 10−4 (Spataro and Ianniello, 2014, and references therein; M. Li et al., 2019, and references therein). The default GEOS-Chem model uses a relatively high of 10−4, which may cause the underestimated NO2 concentrations as well as the overestimated concentrations of nitrate, daytime HNO3 (Fig. S5), and nighttime HONO (Fig. S9) (Alexander et al., 2020). The NO2 concentration increased over 70 % when the model turned off the NO2 uptake. For NH3, the model underestimates its monthly mean concentrations in Beijing (Fig. 4c). The non-agriculture NH3 emissions are based on the year 2006 and can be greater in 2012 because of the rapid economic growth (Kang et al., 2016; Meng et al., 2017). Several studies show that the non-agriculture emissions are the dominant NH3 sources during haze periods in Beijing when the transport of rural agricultural NH3 emission to urban is limited under stagnant weather conditions (Pan et al., 2016; Sun et al., 2017). The underestimation of NH3 affects the ammonium simulations when the thermodynamic equilibrium is sensitive to HNO3+NH3 (Nenes et al., 2020). For SOA precursors, Fig. 4d shows that the model underestimates surface CO concentrations in Beijing, which may contribute to the model underestimation of anthropogenic SOA. The modeled summertime isoprene concentrations in Beijing are lower than the observations by 20 %–90 %, affecting the simulations of biogenic SOA (Table S6). The model also underestimates the concentrations of aromatic VOCs, similar to previous studies (Liu et al., 2012). Such underestimation would not affect the SOA simulations herein because the simple SOA scheme no longer derives aromatic SOA from the concentrations of aromatic VOCs. Instead, the model treats aromatic SOA as a part of anthropogenic SOA, which is estimated on the basis of the parameterizations on CO.
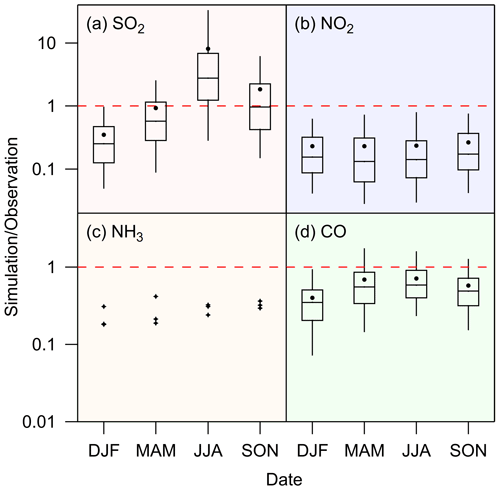
Figure 4The simulation-to-observation ratios of the concentrations of (a) SO2, (b) NO2, (c) NH3, and (d) CO in Beijing. The upper and lower edges of the boxes, the whiskers, the middle lines, and the solid dots in panels (a), (b), and (d) denote the 25th and 75th percentiles, the 5th and 95th percentiles, the median values, and the mean values of the simulation-to-observation ratios of the hourly mean concentrations of the corresponding species at the PKUERS site from July 2011 to May 2013, respectively. The solid dots in (c) represent the simulation-to-observation ratios of the monthly mean concentrations of NH3 at the IAP site from December 2007 to November 2010. The dashed red lines show the 1 : 1 simulation-to-observation ratio.
Oxidants are essential in chemical conversions. Figure 5a–b show the modeled and the observed concentrations of OH• and HO2• radicals in Beijing. The peak concentrations of OH• and HO2• radicals are underestimated by a factor of 1.5–2 and 2–4, respectively, explained by the missing source of daytime HONO (Fig. S9) (Liu et al., 2019; L. Zhang et al., 2016; J. Zhang et al., 2018). Such underestimation suggests insufficient atmospheric oxidation capacity in the model, meaning reduced formation of sulfate and nitrate. Figure 5c shows that the model overestimates the surface O3 concentrations in winter. Common problems have been reported in other studies in China and other northern hemispheric locations by various CTMs (J. Hu et al., 2016; Travis et al., 2016; Young et al., 2018; J. Li et al., 2019). Nevertheless, the overestimated O3 has little influence on the SOA simulation by the simple SOA scheme and has minor impacts on SIA because of the dominant contribution from the photochemical and heterogeneous pathways. Moreover, NO3• affects the formation of nitrate and SOA (Ng et al., 2017). Measurements of NO3• in Beijing show nighttime peak concentrations of less than 6 pptv in summer and below the detection limit of 2.4 pptv in winter (Wang et al., 2017a). The modeled concentrations are 3 times greater than the peak concentrations in summer (Fig. 5d), suggesting a possible overestimation of nighttime oxidation.
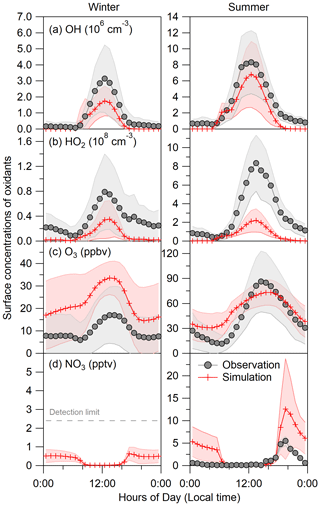
Figure 5Diurnal profiles of the hourly mean simulated and observed concentrations of (a) OH• and (b) HO2• at the Wangdu site from June to July 2014 and at the Huairou site from January to March 2016, (c) O3 at the PKUERS site from July 2011 to May 2013, and (d) NO3• at the PKUERS site in January and September 2016 in Beijing. The shaded areas indicate mean value ±1 standard deviation.
In addition, the heterogeneous production of sulfate and SOA is not included in the standard models, leading to certain underestimations. The model uses relatively high values of , which may lead to the overestimation of nitrate (McDuffie et al., 2018; Davis et al., 2008; Jaegle et al., 2018). The lack of nitryl chloride formation from the N2O5 uptake in the model may contribute to the overestimation of nitrate in northern China (Sarwar et al., 2014). Another bias is the high default value of as described previously. Biases may also relate to the atmospheric removal of the SIA species (Jaegle et al., 2018; Luo et al., 2019). For example, the GEOS-Chem model underestimates the wet deposition of nitrate in China by 15 %–23 %, especially in urban areas, which may affect both nitrate and ammonium in summer when the wet deposition fluxes are large (Zhao et al., 2017; Xu et al., 2018). The model possibly overestimates the surface resistance of HNO3 (Shah et al., 2018). The test with doubling the deposition velocity of HNO3 however suggests a minor impact of this factor on the nitrate simulations (Heald et al., 2012). Finally, the photolysis of particle-phase nitrate may be significant and affect the nitrate concentrations (Romer et al., 2018; Kasibhatla et al., 2018). In Beijing, the photolysis rate of particle-phase nitrate remains unclear, and the thick coating of PM2.5 may reduce the photolysis (Ye et al., 2017).
4.3 Relative importance of various factors to the model bias
From the factors described above, we chose the ones that are expected to significantly affect the PM2.5 simulations and can be constrained by ambient or laboratory measurements to conduct the sensitivity analysis. Their potential contributions to the model bias of PM2.5 components are evaluated for two case periods, 21–26 August and 21–27 December 2012, for summer and winter, respectively. The simulations for both weeks show the highest R value for the correlations with the observations during the seasons. The 2 weeks are chosen to address general model biases that are not specific to haze conditions. No severe haze episodes occurred during the 2 weeks.
The tested factors for the sensitivity runs are listed in Table 2. Case 0 represents the standard model simulations. The nighttime BLH was multiplied by 3.6 based on the lowest median value of the MERRA2-to-observation ratios at 08:00 and 20:00 (Fig. S8) when the original BLH was lower than 500 m (i.e., the median of the observed BLH) in Case 1. The SO2 emissions in China were multiplied by 0.8 in summer and 1.5 in winter in Case 2 based on the minimum and maximum values of the ratios between the top-down estimates provided by Koukouli et al. (2018) and the MEIC inventory (Fig. S10), respectively. The non-agriculture NH3 emissions in China were scaled up by 1.4 as suggested by Kang et al. (2016) in Case 3. In Case 4, the reaction rate coefficients for the reactions that directly involve OH• oxidation and affect the formation and loss of PM2.5 such as the gaseous formation of sulfuric acid and HNO3 and the oxidation of HNO3 were multiplied by 1.5 in summer and 2 in winter to offset the influence of underestimated OH• concentrations. The multipliers of 1.5 and 2 were derived on the basis of the largest ratio of simulated to observed hourly mean OH• concentrations between 09:00 and 15:00. In terms of the heterogeneous formation of sulfate, we added two types of parameterizations for in Cases 5 and 6. One derives the uptake coefficient of SO2 from RH () (B. Zheng et al., 2015), and the other calculates the coefficient as a function of ALWC () (J. Li et al., 2018). The former is in the order of 10−5, and the latter is in the range of 10−6 to 10−4. For comparisons, the uptake coefficients are in G. Li et al. (2017) and 10−9 to 10−3 in Shao et al. (2019). In Case 7, we reduced the value of from the parameterization of Evans and Jacob (2005) to 10−3 to represent the lower end in the worldwide observations (McDuffie et al., 2018, and references therein). Similarly, the was modified from 10−4 to 10−6 in Case 8 according to the median value of recent laboratory results (Spataro and Ianniello, 2014, and references therein; M. Li et al., 2019, and references therein). The increase of wet deposition of nitrate is tested, for which we applied the seasonal variation to in-cloud condensation water for rainout parameterization and updated the washout parameterization of HNO3 based on the method introduced by Luo et al. (2019) in Case 9. Cases 10 to 50 are the runs with various combinations of the modifications in Cases 1 to 9 (Table S7) for the two case periods. We did not run tests for OA because of the lack of sufficient ambient and laboratory constraints. Scaling up the CO emissions in autumn and winter (Fig. 4d) would lead to significant overestimation of OA in non-urban areas.
Figure 6 shows the simulation-to-observation ratios of hourly mean mass concentrations of NR-PM2.5, sulfate, nitrate, and ammonium for Cases 0 to 9. The nocturnal BLH, the non-agriculture NH3 emissions, the OH• levels, and the wet deposition of nitrate have minor impacts on the model performance of these components. The updated SO2 emissions in Case 2 substantially improve the model simulation of sulfate in Beijing, although further improvements are needed in winter. Similar to previous findings, the heterogeneous uptake of SO2 in Cases 5 and 6 increases the simulated sulfate concentrations and leads to better model–observation comparisons in winter (B. Zheng et al., 2015; J. Li et al., 2018). However, both of the cases lead to the overestimation of sulfate concentrations in summer. The nitrate overestimation in summer leads to the overpredicted ALWC that promotes the excess heterogeneous sulfate formation. The variances of the simulation-to-observation ratios for both cases are greater than the standard simulation in Case 0, indicating the limitation of the heterogeneous parameterizations. A mechanistic approach other than using indirect indicators like RH and ALWC along with accurate SO2 emissions as discussed in Sect. 4.2 may be necessary to improve the seasonality of the sulfate simulation.
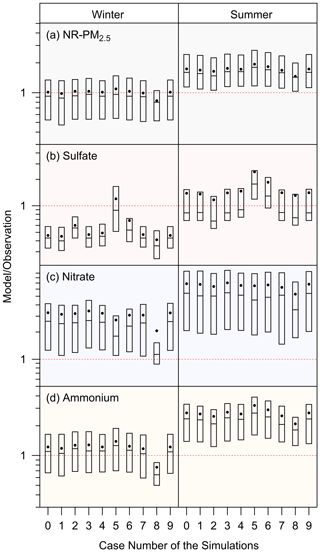
Figure 6Box-and-whisker plots of the simulation-to-observation ratios of hourly mean mass concentrations of (a) NR-PM2.5, (b) sulfate, (c) nitrate, and (d) ammonium for the standard simulation (i.e., Case 0) and Cases 1 to 9 during the selected wintertime and summertime periods. The upper and lower edges of the boxes, the middle lines, and the solid dots denote the 25th and 75th percentiles, the median values, and the mean values, respectively. The dashed red lines show the 1 : 1 simulation-to-observation ratio.
The reduced in Case 7 leads to a minor reduction of simulated nitrate concentrations, suggesting that the uncertainty of heterogeneous uptake of N2O5 is not the main cause of the overestimation of nitrate. The simulations with more reasonable in Case 8 are able to reproduce the observed nitrate concentrations in winter, indicating that the biased NO2 uptake is an important contributor to the overestimation of nitrate and nighttime HONO (Fig. S9). However, the reduced alone is insufficient to correct the nitrate concentrations in summer. In China, especially in northern China, the nitrate formation is likely more sensitive to HNO3 than to NH3 under the control of aerosol acidity and ALWC (Nenes et al., 2020). The model shows the excessive nitrate availability when the photochemical production might be underestimated because of the underestimated oxidant concentrations. The insufficient improvements by constraining the heterogeneous chemical production in Cases 7–8 suggests that the nitrate bias is perhaps related to the insufficient removal of nitrate or HNO3 in the model. The updated wet deposition of nitrate in Case 9 can reduce the summertime monthly mean concentrations by about 20 % (Fig. S11) but is still minor compared with the large overestimation. Insufficient dry deposition of HNO3 and nitrate and the photolysis losses of particulate nitrate to produce HONO and NOx (Ye et al., 2017) as well as the joint influence of multiple factors (discussed later) are possible explanations for the overestimation of nitrate. Nemitz et al. (2004) indicated greater dry deposition velocities of nitrate than the values used typically in models. However, the relative contributions of the dry deposition of nitrate and ammonium to the total deposition of nitrate + HNO3 and ammonium + NH3 are small (<10 %) (Zhao et al., 2017). We expect a minor influence of such uncertainties on the issue of nitrate overestimation. Heald et al. (2012) showed a minor impact of the uncertainty in the dry deposition of HNO3 on the nitrate simulations. The photolysis of particulate nitrate is therefore likely substantial, which has been ignored in the model simulations.
Sulfate and nitrate simulations interact with each other through thermodynamic equilibrium. As shown in Fig. 6, adding the heterogeneous formation of sulfate reduces the simulation-to-observation ratios of nitrate in winter (i.e., the median ratio from 2.6 to 1.8–2.3 in Cases 5–6) and the simulated weekly mean concentrations of nitrate by 16 %–36 % (Fig. S12a). The heterogeneous formation of sulfate may affect aerosol pH and ALWC that determine the sensitivity of nitrate formation to NH3 or to HNO3 (Nenes et al., 2020). On the other hand, the reduced leads to the reduction of the simulation-to-observation ratios of sulfate (i.e., about 0.1 reduction of the median ratios) and the weekly mean simulated sulfate concentrations by 12 %–20 % (Fig. S12b). The reduced decreases the HONO concentrations by 98 % and hence the OH• levels by 26 %–74 % in Beijing, which leads to lower concentrations of sulfate. In addition, the reduced decreases ALWC through reducing nitrate, which also slows down the heterogeneous sulfate formation.
Figure 7 shows the improvements of absolute NMB () and R values for the sulfate and nitrate simulations in Cases 5, 6, and 8 (with updated heterogeneous formation of sulfate and nitrate) and Cases 10 to 50 relative to Case 0. In winter, the parameterization of heterogeneous sulfate formation on RH in the cases with improves R but leads to greater , while the parameterization on ALWC in the cases with improves but slightly decreases R (Fig. 7a). By contrast, all the cases with or show worse R values in summer, and only the cases with generally improve . The results suggest that the parameterization on ALWC is better in terms of the overall model performance than the parameterization on RH. The decreased R in summer in the cases with is perhaps because the biased inorganic aerosol concentrations and the underrepresented organic contribution in the ALWC calculations lead to large uncertainty in the simulated ALWC and sulfate concentrations (Pye et al., 2009). For nitrate, the cases with updated show large improvements of in both seasons (Fig. 7b). The changes of R are small.
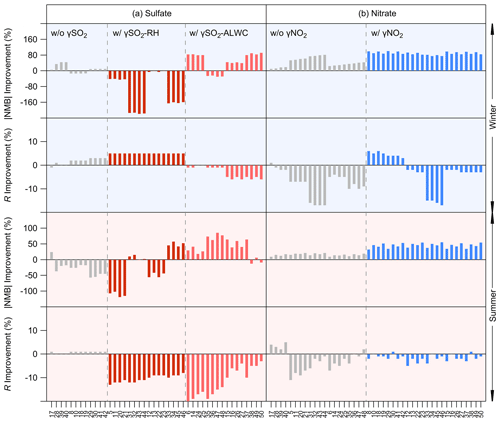
Figure 7The improvements of the R and the values for (a) sulfate and (b) nitrate in Cases 10–50 relative to Case 0 (standard) during the selected wintertime and summertime periods. For comparison, Cases 5 (with ), 6 (with ), and 8 (reduced ) are also plotted. Positive values in or R improvement mean improved simulations.
The combination of the heterogeneous factors with other factors in Cases 10–50 shows various changes in model improvements. For the cases with , updating SO2 emissions and reducing lead to the most significant further improvements in , especially in summer (Fig. 7a). Such interaction suggests that the parameterization of heterogeneous sulfate formation is sensitive to the precursor concentrations, the oxidation, and the nitrate-induced ALWC in China. For nitrate, the combinations of the case with heterogeneous sulfate formation can worsen R in winter (Fig. 7b), explained by the limitation of NH3 relative to high sulfate concentration that affects the nitrate partitioning. This impact is perhaps smaller in summer because of the greater NH3 emissions. The combination of the reduced with the improved leads to the most significant further reduction of in summer, and other factors lead to minor improvements.
Case 50 represents the combination of all factors (including not ). It shows an R value of 0.8/0.9 (winter/summer) and an value of 0.05/0.3 for sulfate, and an R value of 0.8/0.7 and an value of 0.3/2.1 for nitrate. By contrast, the standard simulation in Case 0 shows an R value of 0.9/0.9 and an value of 0.6/0.3 for sulfate, and an R value of 0.9/0.7 and an value of 2.0/4.7 for nitrate. For sulfate, the is largely improved in winter by the combination of all factors. In summer, the influence of all factors seems to be canceled out and therefore leads to an insignificant change in . For nitrate, the combination of all factors can greatly improve the in both seasons, although the overestimation of nitrate is still very large in summer.
We evaluated the GEOS-Chem model simulations with a nationwide dataset in China and a long-term hourly dataset in Beijing for sulfate, nitrate, ammonium, and OA. The underestimation of sulfate and the overestimation of nitrate concentrations for most of the sites suggest general problems in the model. The simple SOA scheme significantly improves the OA simulations in China, suggesting that the SOA formation from anthropogenic precursors is perhaps the main reason for the underestimation of OA in previous studies. The remaining underestimation of OA is plausibly associated with the insufficient SOA production in the model. The model–observation agreement shows significant seasonality. Sulfate is mostly underestimated in winter, and nitrate is significantly overestimated in all seasons. The model is unable to reproduce the diurnal patterns of nitrate and ammonium. Sensitivity analysis for factors related to meteorology, emission, chemistry, and wet deposition with laboratory constraints show that uncertainties in chemistry perhaps dominate the model bias. Among the individual factors, updated heterogeneous parameterizations for SO2 and NO2 significantly reduce the model–observation gaps of sulfate and nitrate (decreasing winter/summer by 0.48/0.09 and 1.98/1.51), respectively. Accurate sulfate simulations in China may be achieved by joint factors, for example, heterogeneous sulfate formation along with accurate SO2 emissions and well-reproduced oxidant and ALWC conditions. Good sulfate simulations improve the nitrate simulations by altering the sensitivity of nitrate formation to HNO3 or to HNO3 + NH3, especially in winter when NH3 might be limited. The combination of all factors biases sulfate by 30 % in summer and nitrate by 30 % in winter, which are within the measurement uncertainties. However, the all-factor simulations still overestimate nitrate by 210 % in summer (470 % in standard simulations), highlighting the model issues related to atmospheric removal of HNO3 and nitrate. The insufficient dry and wet deposition of HNO3 and nitrate likely play minor roles, suggesting that the photolysis of particulate nitrate might be substantial in polluted environments. The nitrate simulations require a better understanding of the atmospheric reactive nitrogen budget, especially the role of the photolysis of particle-phase nitrate. Simultaneous measurements of major reactive nitrogen species including NOx, N2O5, NO3•, HONO, HNO3, NH3, and particle-phase nitrogen in field campaigns can provide critical datasets for future model investigations.
Data presented in this paper are available upon request to the corresponding author.
The supplement related to this article is available online at: https://doi.org/10.5194/acp-20-12265-2020-supplement.
QC designed the study. RM performed the model simulations and conducted the data analysis. YS, JG, KL, YuZ, SC, LZ, YaZ, XC, YL, ZT, and XM provided the observation data. QZ provided the MEIC inventories and the diurnal profiles of emissions. QC and RM prepared the paper with contributions from PIP, MS, JG, and KL.
The authors declare that they have no conflict of interest.
This work was supported by the MOST National Key R&D Program of China (2017YFC0209802), the National Natural Science Foundation of China (91544107, 51861135102, 41875165), and the 111 Project of Urban Air Pollution and Health Effects (B20009). Paul I. Palmer was supported by NERC grant no. NE/N006879/1. Manish Shrivastava was supported by the U.S. DOE, Office of Science, Office of Biological and Environmental Research, through the Early Career Research Program. The Pacific Northwest National Laboratory is operated for DOE by the Battelle Memorial Institute under contract DE-AC06-76RL01830. This work was also supported by the State Key Joint Laboratory of Environment Simulation and Pollution Control (15Y02ESPCP and 16Y01ESPCP). The authors thank Weili Lin and Lin Zhang for technical support and helpful discussions.
This research has been supported by the MOST National Key Research and Development Program of China (grant no. 2017YFC0209802), the National Natural Science Foundation of China (grant nos. 51861135102, 91544107, and 41875165), the Natural Environment Research Council (grant no. NE/N006879/1), and the State Key Joint Laboratory of Environmental Simulation and Pollution Control (grant nos. 15Y02ESPCP and 16Y01ESPCP).
This paper was edited by Kostas Tsigaridis and reviewed by two anonymous referees.
Abbatt, J. P. D., Lee, A. K. Y., and Thornton, J. A.: Quantifying trace gas uptake to tropospheric aerosol: recent advances and remaining challenges, Chem. Soc. Rev., 41, 6555–6581, https://doi.org/10.1039/c2cs35052a, 2012.
Alexander, B., Sherwen, T., Holmes, C. D., Fisher, J. A., Chen, Q., Evans, M. J., and Kasibhatla, P.: Global inorganic nitrate production mechanisms: comparison of a global model with nitrate isotope observations, Atmos. Chem. Phys., 20, 3859–3877, https://doi.org/10.5194/acp-20-3859-2020, 2020.
An, Z., Huang, R. J., Zhang, R., Tie, X., Li, G., Cao, J., Zhou, W., Shi, Z., Han, Y., Gu, Z., and Ji, Y.: Severe haze in northern China: A synergy of anthropogenic emissions and atmospheric processes, P. Natl. Acad. Sci. USA, 201900125, https://doi.org/10.1073/pnas.1900125116, 2019.
Bei, N., Wu, J., Elser, M., Feng, T., Cao, J., El-Haddad, I., Li, X., Huang, R., Li, Z., Long, X., Xing, L., Zhao, S., Tie, X., Prévôt, A. S. H., and Li, G.: Impacts of meteorological uncertainties on the haze formation in Beijing–Tianjin–Hebei (BTH) during wintertime: a case study, Atmos. Chem. Phys., 17, 14579–14591, https://doi.org/10.5194/acp-17-14579-2017, 2017.
Bertram, T. H. and Thornton, J. A.: Toward a general parameterization of N2O5 reactivity on aqueous particles: the competing effects of particle liquid water, nitrate and chloride, Atmos. Chem. Phys., 9, 8351–8363, https://doi.org/10.5194/acp-9-8351-2009, 2009.
Brown, S. S. and Stutz, J.: Nighttime radical observations and chemistry, Chem. Soc. Rev., 41, 6405–6447, https://doi.org/10.1039/c2cs35181a, 2012.
Canagaratna, M. R., Jayne, J. T., Jimenez, J. L., Allan, J. D., Alfarra, M. R., Zhang, Q., Onasch, T. B., Drewnick, F., Coe, H., Middlebrook, A., Delia, A., Williams, L. R., Trimborn, A. M., Northway, M. J., DeCarlo, P. F., Kolb, C. E., Davidovits, P., and Worsnop, D. R.: Chemical and microphysical characterization of ambient aerosols with the aerodyne aerosol mass spectrometer, Mass Spectrom. Rev., 26, 185–222, https://doi.org/10.1002/mas.20115, 2007.
Cao, H., Fu, T.-M., Zhang, L., Henze, D. K., Miller, C. C., Lerot, C., Abad, G. G., De Smedt, I., Zhang, Q., van Roozendael, M., Hendrick, F., Chance, K., Li, J., Zheng, J., and Zhao, Y.: Adjoint inversion of Chinese non-methane volatile organic compound emissions using space-based observations of formaldehyde and glyoxal, Atmos. Chem. Phys., 18, 15017–15046, https://doi.org/10.5194/acp-18-15017-2018, 2018.
Chan, C. K. and Yao, X.: Air pollution in mega cities in China, Atmos. Environ., 42, 1–42, https://doi.org/10.1016/j.atmosenv.2007.09.003, 2008.
Chen, D., Liu, Z., Fast, J., and Ban, J.: Simulations of sulfate–nitrate–ammonium (SNA) aerosols during the extreme haze events over northern China in October 2014, Atmos. Chem. Phys., 16, 10707–10724, https://doi.org/10.5194/acp-16-10707-2016, 2016.
Chen, L., Gao, Y., Zhang, M., Fu, J. S., Zhu, J., Liao, H., Li, J., Huang, K., Ge, B., Wang, X., Lam, Y. F., Lin, C.-Y., Itahashi, S., Nagashima, T., Kajino, M., Yamaji, K., Wang, Z., and Kurokawa, J.: MICS-Asia III: multi-model comparison and evaluation of aerosol over East Asia, Atmos. Chem. Phys., 19, 11911–11937, https://doi.org/10.5194/acp-19-11911-2019, 2019.
Chen, Q., Heald, C. L., Jimenez, J. L., Canagaratna, M. R., Zhang, Q., He, L. Y., Huang, X. F., Campuzano-Jost, P., Palm, B. B., Poulain, L., Kuwata, M., Martin, S. T., Abbatt, J. P. D., Lee, A. K. Y., and Liggio, J.: Elemental composition of organic aerosol: The gap between ambient and laboratory measurements, Geophys. Res. Lett., 42, 4182–4189, https://doi.org/10.1002/2015GL063693, 2015.
Chen, Q., Fu, T.-M., Hu, J., Ying, Q., and Zhang, L.: Modelling secondary organic aerosols in China, Natl. Sci. Rev., 4, 806–809, https://doi.org/10.1093/nsr/nwx143, 2017.
Cheng, Y., Zheng, G., Wei, C., Mu, Q., Zheng, B., Wang, Z., Gao, M., Zhang, Q., He, K., Carmichael, G., Pöschl, U., and Su, H.: Reactive nitrogen chemistry in aerosol water as a source of sulfate during haze events in China, Sci. Adv., 2, e1601530, https://doi.org/10.1126/sciadv.1601530, 2016.
Chow, J. C.: Measurement Methods to Determine Compliance with Ambient Air Quality Standards for Suspended Particles, J. Air Waste Manage., 45, 320–382, https://doi.org/10.1080/10473289.1995.10467369, 1995.
Chung, S. H. and Seinfeld, J. H.: Global distribution and climate forcing of carbonaceous aerosols, J. Geophys. Res.-Atmos., 107, AAC 14-1–AAC 14-33, https://doi.org/10.1029/2001JD001397, 2002.
Davis, J. M., Bhave, P. V., and Foley, K. M.: Parameterization of N2O5 reaction probabilities on the surface of particles containing ammonium, sulfate, and nitrate, Atmos. Chem. Phys., 8, 5295–5311, https://doi.org/10.5194/acp-8-5295-2008, 2008.
DeCarlo, P. F., Kimmel, J. R., Trimborn, A., Northway, M. J., Jayne, J. T., Aiken, A. C., Gonin, M., Fuhrer, K., Horvath, T., Docherty, K. S., Worsnop, D. R., and Jimenez, J. L.: Field-Deployable, High-Resolution, Time-of-Flight Aerosol Mass Spectrometer, Anal. Chem., 78, 8281–8289, https://doi.org/10.1021/ac061249n, 2006.
Donahue, N. M., Kroll, J. H., Pandis, S. N., and Robinson, A. L.: A two-dimensional volatility basis set – Part 2: Diagnostics of organic-aerosol evolution, Atmos. Chem. Phys., 12, 615–634, https://doi.org/10.5194/acp-12-615-2012, 2012.
Drugé, T., Nabat, P., Mallet, M., and Somot, S.: Model simulation of ammonium and nitrate aerosols distribution in the Euro-Mediterranean region and their radiative and climatic effects over 1979–2016, Atmos. Chem. Phys., 19, 3707–3731, https://doi.org/10.5194/acp-19-3707-2019, 2019.
Elser, M., Huang, R.-J., Wolf, R., Slowik, J. G., Wang, Q., Canonaco, F., Li, G., Bozzetti, C., Daellenbach, K. R., Huang, Y., Zhang, R., Li, Z., Cao, J., Baltensperger, U., El-Haddad, I., and Prévôt, A. S. H.: New insights into PM2.5 chemical composition and sources in two major cities in China during extreme haze events using aerosol mass spectrometry, Atmos. Chem. Phys., 16, 3207–3225, https://doi.org/10.5194/acp-16-3207-2016, 2016.
Evans, M. J. and Jacob, D. J.: Impact of new laboratory studies of N2O5 hydrolysis on global model budgets of tropospheric nitrogen oxides, ozone, and OH, Geophys. Res. Lett., 32, L09813, https://doi.org/10.1029/2005GL022469, 2005.
Fountoukis, C. and Nenes, A.: ISORROPIA II: a computationally efficient thermodynamic equilibrium model for K+-Ca2+-Mg2+--Na+---Cl−-H2O aerosols, Atmos. Chem. Phys., 7, 4639–4659, https://doi.org/10.5194/acp-7-4639-2007, 2007.
Fu, T.-M., Cao, J. J., Zhang, X. Y., Lee, S. C., Zhang, Q., Han, Y. M., Qu, W. J., Han, Z., Zhang, R., Wang, Y. X., Chen, D., and Henze, D. K.: Carbonaceous aerosols in China: top-down constraints on primary sources and estimation of secondary contribution, Atmos. Chem. Phys., 12, 2725–2746, https://doi.org/10.5194/acp-12-2725-2012, 2012.
Gao, M., Han, Z., Liu, Z., Li, M., Xin, J., Tao, Z., Li, J., Kang, J.-E., Huang, K., Dong, X., Zhuang, B., Li, S., Ge, B., Wu, Q., Cheng, Y., Wang, Y., Lee, H.-J., Kim, C.-H., Fu, J. S., Wang, T., Chin, M., Woo, J.-H., Zhang, Q., Wang, Z., and Carmichael, G. R.: Air quality and climate change, Topic 3 of the Model Inter-Comparison Study for Asia Phase III (MICS-Asia III) – Part 1: Overview and model evaluation, Atmos. Chem. Phys., 18, 4859–4884, https://doi.org/10.5194/acp-18-4859-2018, 2018.
Giglio, L., Randerson, J. T., and van der Werf, G. R.: Analysis of daily, monthly, and annual burned area using the fourth-generation global fire emissions database (GFED4), J. Geophys. Res.-Biogeo., 118, 317–328, https://doi.org/10.1002/jgrg.20042, 2013.
Guenther, A. B., Jiang, X., Heald, C. L., Sakulyanontvittaya, T., Duhl, T., Emmons, L. K., and Wang, X.: The Model of Emissions of Gases and Aerosols from Nature version 2.1 (MEGAN2.1): an extended and updated framework for modeling biogenic emissions, Geosci. Model Dev., 5, 1471–1492, https://doi.org/10.5194/gmd-5-1471-2012, 2012.
Guo, J., He, J., Liu, H., Miao, Y., Liu, H., and Zhai, P.: Impact of various emission control schemes on air quality using WRF-Chem during APEC China 2014, Atmos. Environ., 140, 311–319, https://doi.org/10.1016/j.atmosenv.2016.05.046, 2016a.
Guo, J., Miao, Y., Zhang, Y., Liu, H., Li, Z., Zhang, W., He, J., Lou, M., Yan, Y., Bian, L., and Zhai, P.: The climatology of planetary boundary layer height in China derived from radiosonde and reanalysis data, Atmos. Chem. Phys., 16, 13309–13319, https://doi.org/10.5194/acp-16-13309-2016, 2016b.
Guo, J., Li, Y., Cohen, J. B., Li, J., Chen, D., Xu, H., Liu, L., Yin, J., Hu, K., and Zhai, P.: Shift in the Temporal Trend of Boundary Layer Height in China Using Long-Term (1979–2016) Radiosonde Data, Geophys. Res. Lett., 46, 6080–6089, https://doi.org/10.1029/2019GL082666, 2019.
Han, Z., Xie, Z., Wang, G., Zhang, R., and Tao, J.: Modeling organic aerosols over east China using a volatility basis-set approach with aging mechanism in a regional air quality model, Atmos. Environ., 124, 186–198, https://doi.org/10.1016/j.atmosenv.2015.05.045, 2016.
Hayes, P. L., Carlton, A. G., Baker, K. R., Ahmadov, R., Washenfelder, R. A., Alvarez, S., Rappenglück, B., Gilman, J. B., Kuster, W. C., de Gouw, J. A., Zotter, P., Prévôt, A. S. H., Szidat, S., Kleindienst, T. E., Offenberg, J. H., Ma, P. K., and Jimenez, J. L.: Modeling the formation and aging of secondary organic aerosols in Los Angeles during CalNex 2010, Atmos. Chem. Phys., 15, 5773–5801, https://doi.org/10.5194/acp-15-5773-2015, 2015.
Heald, C. L., Coe, H., Jimenez, J. L., Weber, R. J., Bahreini, R., Middlebrook, A. M., Russell, L. M., Jolleys, M., Fu, T.-M., Allan, J. D., Bower, K. N., Capes, G., Crosier, J., Morgan, W. T., Robinson, N. H., Williams, P. I., Cubison, M. J., DeCarlo, P. F., and Dunlea, E. J.: Exploring the vertical profile of atmospheric organic aerosol: comparing 17 aircraft field campaigns with a global model, Atmos. Chem. Phys., 11, 12673–12696, https://doi.org/10.5194/acp-11-12673-2011, 2011.
Heald, C. L., Collett Jr., J. L., Lee, T., Benedict, K. B., Schwandner, F. M., Li, Y., Clarisse, L., Hurtmans, D. R., Van Damme, M., Clerbaux, C., Coheur, P.-F., Philip, S., Martin, R. V., and Pye, H. O. T.: Atmospheric ammonia and particulate inorganic nitrogen over the United States, Atmos. Chem. Phys., 12, 10295–10312, https://doi.org/10.5194/acp-12-10295-2012, 2012.
Hodzic, A. and Jimenez, J. L.: Modeling anthropogenically controlled secondary organic aerosols in a megacity: a simplified framework for global and climate models, Geosci. Model Dev., 4, 901–917, https://doi.org/10.5194/gmd-4-901-2011, 2011.
Hodzic, A., Aumont, B., Knote, C., Lee-Taylor, J., Madronich, S., and Tyndall, G.: Volatility dependence of Henry's law constants of condensable organics: Application to estimate depositional loss of secondary organic aerosols, Geophys. Res. Lett., 41, 4795–4804, https://doi.org/10.1002/2014gl060649, 2014.
Hodzic, A., Kasibhatla, P. S., Jo, D. S., Cappa, C. D., Jimenez, J. L., Madronich, S., and Park, R. J.: Rethinking the global secondary organic aerosol (SOA) budget: stronger production, faster removal, shorter lifetime, Atmos. Chem. Phys., 16, 7917–7941, https://doi.org/10.5194/acp-16-7917-2016, 2016.
Hoesly, R. M., Smith, S. J., Feng, L., Klimont, Z., Janssens-Maenhout, G., Pitkanen, T., Seibert, J. J., Vu, L., Andres, R. J., Bolt, R. M., Bond, T. C., Dawidowski, L., Kholod, N., Kurokawa, J.-I., Li, M., Liu, L., Lu, Z., Moura, M. C. P., O'Rourke, P. R., and Zhang, Q.: Historical (1750–2014) anthropogenic emissions of reactive gases and aerosols from the Community Emissions Data System (CEDS), Geosci. Model Dev., 11, 369–408, https://doi.org/10.5194/gmd-11-369-2018, 2018.
Holmes, C. D., Bertram, T. H., Confer, K. L., Graham, K. A., Ronan, A. C., Wirks, C. K., and Shah, V.: The Role of Clouds in the Tropospheric NOx Cycle: A New Modeling Approach for Cloud Chemistry and Its Global Implications, Geophys. Res. Lett., 46, 4980–4990, https://doi.org/10.1029/2019GL081990, 2019.
Hu, J., Chen, J., Ying, Q., and Zhang, H.: One-year simulation of ozone and particulate matter in China using WRF/CMAQ modeling system, Atmos. Chem. Phys., 16, 10333–10350, https://doi.org/10.5194/acp-16-10333-2016, 2016.
Hu, W., Hu, M., Hu, W., Jimenez, J. L., Yuan, B., Chen, W., Wang, M., Wu, Y. S., Chen, C., Wang, Z., Peng, J., Zeng, L., and Shao, M.: Chemical composition, sources, and aging process of submicron aerosols in Beijing: Contrast between summer and winter, J. Geophys. Res.-Atmos., 121, 1955–1977, https://doi.org/10.1002/2015JD024020, 2016.
Huang, X., Song, Y., Li, M., Li, J., Huo, Q., Cai, X., Zhu, T., Hu, M., and Zhang, H.: A high-resolution ammonia emission inventory in China, Global Biogeochem. Cy., 26, GB1030, https://doi.org/10.1029/2011GB004161, 2012.
Hudman, R. C., Moore, N. E., Mebust, A. K., Martin, R. V., Russell, A. R., Valin, L. C., and Cohen, R. C.: Steps towards a mechanistic model of global soil nitric oxide emissions: implementation and space based-constraints, Atmos. Chem. Phys., 12, 7779–7795, https://doi.org/10.5194/acp-12-7779-2012, 2012.
Hung, H.-M. and Hoffmann, M. R.: Oxidation of Gas-Phase SO2 on the Surfaces of Acidic Microdroplets: Implications for Sulfate and Sulfate Radical Anion Formation in the Atmospheric Liquid Phase, Environ. Sci. Technol., 49, 13768–13776, https://doi.org/10.1021/acs.est.5b01658, 2015.
Hung, H.-M., Hsu, M.-N., and Hoffmann, M. R.: Quantification of SO2 Oxidation on Interfacial Surfaces of Acidic Micro-Droplets: Implication for Ambient Sulfate Formation, Environ. Sci. Technol., 52, 9079–9086, https://doi.org/10.1021/acs.est.8b01391, 2018.
Jaegle, L., Shah, V., Thornton, J. A., Lopez-Hilfiker, F. D., Lee, B. H., McDuffie, E. E., Fibiger, D., Brown, S. S., Veres, P., Sparks, T. L., Ebben, C. J., Wooldridge, P. J., Kenagy, H. S., Cohen, R. C., Weinheimer, A. J., Campos, T. L., Montzka, D. D., Digangi, J. P., Wolfe, G. M., Hanisco, T., Schroder, J. C., Campuzano-Jost, P., Day, D. A., Jimenez, J. L., Sullivan, A. P., Guo, H., and Weber, R. J.: Nitrogen Oxides Emissions, Chemistry, Deposition, and Export Over the Northeast United States During the WINTER Aircraft Campaign, J. Geophys. Res.-Atmos., 123, 12368–12393, https://doi.org/10.1029/2018JD029133, 2018.
Jiang, J., Aksoyoglu, S., El-Haddad, I., Ciarelli, G., Denier van der Gon, H. A. C., Canonaco, F., Gilardoni, S., Paglione, M., Minguillón, M. C., Favez, O., Zhang, Y., Marchand, N., Hao, L., Virtanen, A., Florou, K., O'Dowd, C., Ovadnevaite, J., Baltensperger, U., and Prévôt, A. S. H.: Sources of organic aerosols in Europe: a modeling study using CAMx with modified volatility basis set scheme, Atmos. Chem. Phys., 19, 15247–15270, https://doi.org/10.5194/acp-19-15247-2019, 2019.
Kang, Y., Liu, M., Song, Y., Huang, X., Yao, H., Cai, X., Zhang, H., Kang, L., Liu, X., Yan, X., He, H., Zhang, Q., Shao, M., and Zhu, T.: High-resolution ammonia emissions inventories in China from 1980 to 2012, Atmos. Chem. Phys., 16, 2043–2058, https://doi.org/10.5194/acp-16-2043-2016, 2016.
Kasibhatla, P., Sherwen, T., Evans, M. J., Carpenter, L. J., Reed, C., Alexander, B., Chen, Q., Sulprizio, M. P., Lee, J. D., Read, K. A., Bloss, W., Crilley, L. R., Keene, W. C., Pszenny, A. A. P., and Hodzic, A.: Global impact of nitrate photolysis in sea-salt aerosol on NOx, OH, and O3 in the marine boundary layer, Atmos. Chem. Phys., 18, 11185–11203, https://doi.org/10.5194/acp-18-11185-2018, 2018.
Kim, P. S., Jacob, D. J., Fisher, J. A., Travis, K., Yu, K., Zhu, L., Yantosca, R. M., Sulprizio, M. P., Jimenez, J. L., Campuzano-Jost, P., Froyd, K. D., Liao, J., Hair, J. W., Fenn, M. A., Butler, C. F., Wagner, N. L., Gordon, T. D., Welti, A., Wennberg, P. O., Crounse, J. D., St. Clair, J. M., Teng, A. P., Millet, D. B., Schwarz, J. P., Markovic, M. Z., and Perring, A. E.: Sources, seasonality, and trends of southeast US aerosol: an integrated analysis of surface, aircraft, and satellite observations with the GEOS-Chem chemical transport model, Atmos. Chem. Phys., 15, 10411–10433, https://doi.org/10.5194/acp-15-10411-2015, 2015.
Koukouli, M. E., Theys, N., Ding, J., Zyrichidou, I., Mijling, B., Balis, D., and van der A, R. J.: Updated SO2 emission estimates over China using OMI/Aura observations, Atmos. Meas. Tech., 11, 1817–1832, https://doi.org/10.5194/amt-11-1817-2018, 2018.
Kurokawa, J., Ohara, T., Morikawa, T., Hanayama, S., Janssens-Maenhout, G., Fukui, T., Kawashima, K., and Akimoto, H.: Emissions of air pollutants and greenhouse gases over Asian regions during 2000–2008: Regional Emission inventory in ASia (REAS) version 2, Atmos. Chem. Phys., 13, 11019–11058, https://doi.org/10.5194/acp-13-11019-2013, 2013.
Lee, D.-G., Lee, Y.-M., Jang, K.-W., Yoo, C., Kang, K.-H., Lee, J.-H., Jung, S.-W., Park, J.-M., Lee, S.-B., Han, J.-S., Hong, J.-H., and Lee, S.-J.: Korean National Emissions Inventory System and 2007 Air Pollutant Emissions, Asian J. Atmos. Environ., 5, 278–291, https://doi.org/10.5572/ajae.2011.5.4.278, 2011.
Lelieveld, J., Evans, J. S., Fnais, M., Giannadaki, D., and Pozzer, A.: The contribution of outdoor air pollution sources to premature mortality on a global scale, Nature, 525, 367–371, https://doi.org/10.1038/nature15371, 2015.
Li, G., Bei, N., Cao, J., Huang, R., Wu, J., Feng, T., Wang, Y., Liu, S., Zhang, Q., Tie, X., and Molina, L. T.: A possible pathway for rapid growth of sulfate during haze days in China, Atmos. Chem. Phys., 17, 3301–3316, https://doi.org/10.5194/acp-17-3301-2017, 2017.
Li, J., Chen, X., Wang, Z., Du, H., Yang, W., Sun, Y., Hu, B., Li, J., Wang, W., Wang, T., Fu, P., and Huang, H.: Radiative and heterogeneous chemical effects of aerosols on ozone and inorganic aerosols over East Asia, Sci. Total Environ., 622, 1327–1342, https://doi.org/10.1016/j.scitotenv.2017.12.041, 2018.
Li, J., Nagashima, T., Kong, L., Ge, B., Yamaji, K., Fu, J. S., Wang, X., Fan, Q., Itahashi, S., Lee, H.-J., Kim, C.-H., Lin, C.-Y., Zhang, M., Tao, Z., Kajino, M., Liao, H., Li, M., Woo, J.-H., Kurokawa, J., Wang, Z., Wu, Q., Akimoto, H., Carmichael, G. R., and Wang, Z.: Model evaluation and intercomparison of surface-level ozone and relevant species in East Asia in the context of MICS-Asia Phase III – Part 1: Overview, Atmos. Chem. Phys., 19, 12993–13015, https://doi.org/10.5194/acp-19-12993-2019, 2019.
Li, L., Hoffmann, M. R., and Colussi, A. J.: Role of Nitrogen Dioxide in the Production of Sulfate during Chinese Haze-Aerosol Episodes, Environ. Sci. Technol., 52, 2686–2693, https://doi.org/10.1021/acs.est.7b05222, 2018.
Li, M., Liu, H., Geng, G. N., Hong, C. P., Liu, F., Song, Y., Tong, D., Zheng, B., Cui, H. Y., Man, H. Y., Zhang, Q., and He, K. B.: Anthropogenic emission inventories in China: a review, Natl. Sci. Rev., 4, 834–866, https://doi.org/10.1093/nsr/nwx150, 2017a.
Li, M., Zhang, Q., Kurokawa, J.-I., Woo, J.-H., He, K., Lu, Z., Ohara, T., Song, Y., Streets, D. G., Carmichael, G. R., Cheng, Y., Hong, C., Huo, H., Jiang, X., Kang, S., Liu, F., Su, H., and Zheng, B.: MIX: a mosaic Asian anthropogenic emission inventory under the international collaboration framework of the MICS-Asia and HTAP, Atmos. Chem. Phys., 17, 935–963, https://doi.org/10.5194/acp-17-935-2017, 2017b.
Li, M., Su, H., Li, G., Ma, N., Pöschl, U., and Cheng, Y.: Relative importance of gas uptake on aerosol and ground surfaces characterized by equivalent uptake coefficients, Atmos. Chem. Phys., 19, 10981–11011, https://doi.org/10.5194/acp-19-10981-2019, 2019.
Li, Y. J., Sun, Y., Zhang, Q., Li, X., Li, M., Zhou, Z., and Chan, C. K.: Real-time chemical characterization of atmospheric particulate matter in China: A review, Atmos. Environ., 158, 270–304, https://doi.org/10.1016/j.atmosenv.2017.02.027, 2017.
Li, Z., Guo, J., Ding, A., Liao, H., Liu, J. J., Sun, Y., Wang, T., Xue, H., Zhang, H., and Zhu, B.: Aerosol and boundary-layer interactions and impact on air quality, Natl. Sci. Rev., 4, 810–833, https://doi.org/10.1093/nsr/nwx117, 2017.
Liao, H., Henze, D. K., Seinfeld, J. H., Wu, S., and Mickley, L. J.: Biogenic secondary organic aerosol over the United States: Comparison of climatological simulations with observations, J. Geophys. Res.-Atmos., 112, D06201, https://doi.org/10.1029/2006JD007813, 2007.
Liu, C.-N., Lin, S.-F., Awasthi, A., Tsai, C.-J., Wu, Y.-C., and Chen, C.-F.: Sampling and conditioning artifacts of PM2.5 in filter-based samplers, Atmos. Environ., 85, 48–53, https://doi.org/10.1016/j.atmosenv.2013.11.075, 2014.
Liu, H., Jacob, D. J., Bey, I., and Yantosca, R. M.: Constraints from 210Pb and 7Be on wet deposition and transport in a global three-dimensional chemical tracer model driven by assimilated meteorological fields, J. Geophys. Res.-Atmos., 106, 12109–12128, https://doi.org/10.1029/2000JD900839, 2001.
Liu, Y., Lu, K., Li, X., Dong, H., Tan, Z., Wang, H., Zou, Q., Wu, Y., Zeng, L., Hu, M., Min, K.-E., Kecorius, S., Wiedensohler, A., and Zhang, Y.: A Comprehensive Model Test of the HONO Sources Constrained to Field Measurements at Rural North China Plain, Environ. Sci. Technol., 53, 3517–3525, https://doi.org/10.1021/acs.est.8b06367, 2019.
Liu, Z., Wang, Y., Vrekoussis, M., Richter, A., Wittrock, F., Burrows, J. P., Shao, M., Chang, C.-C., Liu, S.-C., Wang, H., and Chen, C.: Exploring the missing source of glyoxal (CHOCHO) over China, Geophys. Res. Lett., 39, L10812, https://doi.org/10.1029/2012GL051645, 2012.
Lu, K., Guo, S., Tan, Z., Wang, H., Shang, D., Liu, Y., Li, X., Wu, Z., Hu, M., and Zhang, Y.: Exploring atmospheric free-radical chemistry in China: the self-cleansing capacity and the formation of secondary air pollution, Natl. Sci. Rev., 6, 579–594, https://doi.org/10.1093/nsr/nwy073, 2018.
Lu, Z. and Streets, D. G.: Increase in NOx Emissions from Indian Thermal Power Plants during 1996–2010: Unit-Based Inventories and Multisatellite Observations, Environ. Sci. Technol., 46, 7463–7470, https://doi.org/10.1021/es300831w, 2012.
Lu, Z., Zhang, Q., and Streets, D. G.: Sulfur dioxide and primary carbonaceous aerosol emissions in China and India, 1996–2010, Atmos. Chem. Phys., 11, 9839–9864, https://doi.org/10.5194/acp-11-9839-2011, 2011.
Luo, G., Yu, F., and Schwab, J.: Revised treatment of wet scavenging processes dramatically improves GEOS-Chem 12.0.0 simulations of surface nitric acid, nitrate, and ammonium over the United States, Geosci. Model Dev., 12, 3439–3447, https://doi.org/10.5194/gmd-12-3439-2019, 2019.
McDuffie, E. E., Fibiger, D. L., Dube, W. P., Lopez-Hilfiker, F., Lee, B. H., Thornton, J. A., Shah, V., Jaegle, L., Guo, H., Weber, R. J., Reeves, J. M., Weinheimer, A. J., Schroder, J. C., Campuzano-Jost, P., Jimenez, J. L., Dibb, J. E., Veres, P., Ebben, C., Sparks, T. L., Wooldridge, P. J., Cohen, R. C., Hornbrook, R. S., Apel, E. C., Campos, T., Hall, S. R., Ullmann, K., and Brown, S. S.: Heterogeneous N2O5 Uptake During Winter: Aircraft Measurements During the 2015 WINTER Campaign and Critical Evaluation of Current Parameterizations, J. Geophys. Res.-Atmos., 123, 4345–4372, https://doi.org/10.1002/2018JD028336, 2018.
Meng, W., Zhong, Q., Yun, X., Zhu, X., Huang, T., Shen, H., Chen, Y., Chen, H., Zhou, F., Liu, J., Wang, X., Zeng, E. Y., and Tao, S.: Improvement of a Global High-Resolution Ammonia Emission Inventory for Combustion and Industrial Sources with New Data from the Residential and Transportation Sectors, Environ. Sci. Technol., 51, 2821–2829, https://doi.org/10.1021/acs.est.6b03694, 2017.
Murray, L. T., Jacob, D. J., Logan, J. A., Hudman, R. C., and Koshak, W. J.: Optimized regional and interannual variability of lightning in a global chemical transport model constrained by LIS/OTD satellite data, J. Geophys. Res.-Atmos., 117, D20307, https://doi.org/10.1029/2012JD017934, 2012.
Nemitz, E., Sutton, M. A., Wyers, G. P., Otjes, R. P., Mennen, M. G., van Putten, E. M., and Gallagher, M. W.: Gas-particle interactions above a Dutch heathland: II. Concentrations and surface exchange fluxes of atmospheric particles, Atmos. Chem. Phys., 4, 1007–1024, https://doi.org/10.5194/acp-4-1007-2004, 2004.
Nenes, A., Pandis, S. N., Weber, R. J., and Russell, A.: Aerosol pH and liquid water content determine when particulate matter is sensitive to ammonia and nitrate availability, Atmos. Chem. Phys., 20, 3249–3258, https://doi.org/10.5194/acp-20-3249-2020, 2020.
Ng, N. L., Herndon, S. C., Trimborn, A., Canagaratna, M. R., Croteau, P. L., Onasch, T. B., Sueper, D., Worsnop, D. R., Zhang, Q., Sun, Y. L., and Jayne, J. T.: An Aerosol Chemical Speciation Monitor (ACSM) for Routine Monitoring of the Composition and Mass Concentrations of Ambient Aerosol, Aerosol Sci. Tech., 45, 780–794, https://doi.org/10.1080/02786826.2011.560211, 2011.
Ng, N. L., Brown, S. S., Archibald, A. T., Atlas, E., Cohen, R. C., Crowley, J. N., Day, D. A., Donahue, N. M., Fry, J. L., Fuchs, H., Griffin, R. J., Guzman, M. I., Herrmann, H., Hodzic, A., Iinuma, Y., Jimenez, J. L., Kiendler-Scharr, A., Lee, B. H., Luecken, D. J., Mao, J., McLaren, R., Mutzel, A., Osthoff, H. D., Ouyang, B., Picquet-Varrault, B., Platt, U., Pye, H. O. T., Rudich, Y., Schwantes, R. H., Shiraiwa, M., Stutz, J., Thornton, J. A., Tilgner, A., Williams, B. J., and Zaveri, R. A.: Nitrate radicals and biogenic volatile organic compounds: oxidation, mechanisms, and organic aerosol, Atmos. Chem. Phys., 17, 2103–2162, https://doi.org/10.5194/acp-17-2103-2017, 2017.
Pan, Y., Tian, S., Liu, D., Fang, Y., Zhu, X., Zhang, Q., Zheng, B., Michalski, G., and Wang, Y.: Fossil Fuel Combustion-Related Emissions Dominate Atmospheric Ammonia Sources during Severe Haze Episodes: Evidence from 15N-Stable Isotope in Size-Resolved Aerosol Ammonium, Environ. Sci. Technol., 50, 8049–8056, https://doi.org/10.1021/acs.est.6b00634, 2016.
Pan, Y. P., Wang, Y. S., Tang, G. Q., and Wu, D.: Wet and dry deposition of atmospheric nitrogen at ten sites in Northern China, Atmos. Chem. Phys., 12, 6515–6535, https://doi.org/10.5194/acp-12-6515-2012, 2012.
Park, R. J., Jacob, D. J., Chin, M., and Martin, R. V.: Sources of carbonaceous aerosols over the United States and implications for natural visibility, J. Geophys. Res.-Atmos., 108, 4355, https://doi.org/10.1029/2002JD003190, 2003.
Park, R. J., Jacob, D. J., Field, B. D., Yantosca, R. M., and Chin, M.: Natural and transboundary pollution influences on sulfate-nitrate-ammonium aerosols in the United States: Implications for policy, J. Geophys. Res.-Atmos., 109, D15204, https://doi.org/10.1029/2003JD004473, 2004.
Peng, L., Zhang, Q., Yao, Z., Mauzerall, D. L., Kang, S., Du, Z., Zheng, Y., Xue, T., and He, K.: Underreported coal in statistics: A survey-based solid fuel consumption and emission inventory for the rural residential sector in China, Appl. Energ., 235, 1169–1182, https://doi.org/10.1016/j.apenergy.2018.11.043, 2019.
Pye, H. O. T. and Seinfeld, J. H.: A global perspective on aerosol from low-volatility organic compounds, Atmos. Chem. Phys., 10, 4377–4401, https://doi.org/10.5194/acp-10-4377-2010, 2010.
Pye, H. O. T., Liao, H., Wu, S., Mickley, L. J., Jacob, D. J., Henze, D. K., and Seinfeld, J. H.: Effect of changes in climate and emissions on future sulfate-nitrate-ammonium aerosol levels in the United States, J. Geophys. Res.-Atmos., 114, D01205, https://doi.org/10.1029/2008JD010701, 2009.
Qin, M., Wang, X., Hu, Y., Huang, X., He, L., Zhong, L., Song, Y., Hu, M., and Zhang, Y.: Formation of particulate sulfate and nitrate over the Pearl River Delta in the fall: Diagnostic analysis using the Community Multiscale Air Quality model, Atmos. Environ., 112, 81–89, https://doi.org/10.1016/j.atmosenv.2015.04.027, 2015.
Qu, Z., Henze, D. K., Capps, S. L., Wang, Y., Xu, X., Wang, J., and Keller, M.: Monthly top-down NOx emissions for China (2005–2012): A hybrid inversion method and trend analysis, J. Geophys. Res.-Atmos., 122, 4600–4625, https://doi.org/10.1002/2016JD025852, 2017.
Qu, Z., Henze, D. K., Theys, N., Wang, J., and Wang, W.: Hybrid Mass Balance/4D-Var Joint Inversion of NOx and SO2 Emissions in East Asia, J. Geophys. Res.-Atmos., 124, 8203–8224, https://doi.org/10.1029/2018JD030240, 2019.
Romer, P. S., Wooldridge, P. J., Crounse, J. D., Kim, M. J., Wennberg, P. O., Dibb, J. E., Scheuer, E., Blake, D. R., Meinardi, S., Brosius, A. L., Thames, A. B., Miller, D. O., Brune, W. H., Hall, S. R., Ryerson, T. B., and Cohen, R. C.: Constraints on Aerosol Nitrate Photolysis as a Potential Source of HONO and NOx, Environ. Sci. Technol., 52, 13738–13746, https://doi.org/10.1021/acs.est.8b03861, 2018.
Sarwar, G., Simon, H., Xing, J., and Mathur, R.: Importance of tropospheric ClNO2 chemistry across the Northern Hemisphere, Geophys. Res. Lett., 41, 4050–4058, https://doi.org/10.1002/2014GL059962, 2014.
Shah, V., Jaegle, L., Thornton, J. A., Lopez-Hilfiker, F. D., Lee, B. H., Schroder, J. C., Campuzano-Jost, P., Jimenez, J. L., Guo, H., Sullivan, A. P., Weber, R. J., Green, J. R., Fiddler, M. N., Bililign, S., Campos, T. L., Stell, M., Weinheimer, A. J., Montzka, D. D., and Brown, S. S.: Chemical feedbacks weaken the wintertime response of particulate sulfate and nitrate to emissions reductions over the eastern United States, P. Natl. Acad. Sci. USA, 115, 8110–8115, https://doi.org/10.1073/pnas.1803295115, 2018.
Shao, J., Chen, Q., Wang, Y., Lu, X., He, P., Sun, Y., Shah, V., Martin, R. V., Philip, S., Song, S., Zhao, Y., Xie, Z., Zhang, L., and Alexander, B.: Heterogeneous sulfate aerosol formation mechanisms during wintertime Chinese haze events: air quality model assessment using observations of sulfate oxygen isotopes in Beijing, Atmos. Chem. Phys., 19, 6107–6123, https://doi.org/10.5194/acp-19-6107-2019, 2019.
Spataro, F. and Ianniello, A.: Sources of atmospheric nitrous acid: state of the science, current research needs, and future prospects, J. Air Waste Manage., 64, 1232–1250, https://doi.org/10.1080/10962247.2014.952846, 2014.
Su, T., Li, Z., and Kahn, R.: Relationships between the planetary boundary layer height and surface pollutants derived from lidar observations over China: regional pattern and influencing factors, Atmos. Chem. Phys., 18, 15921–15935, https://doi.org/10.5194/acp-18-15921-2018, 2018.
Sun, K., Tao, L., Miller, D. J., Pan, D., Golston, L. M., Zondlo, M. A., Griffin, R. J., Wallace, H. W., Leong, Y. J., Yang, M. M., Zhang, Y., Mauzerall, D. L., and Zhu, T.: Vehicle Emissions as an Important Urban Ammonia Source in the United States and China, Environ. Sci. Technol., 51, 2472–2481, https://doi.org/10.1021/acs.est.6b02805, 2017.
Sun, Y., Xu, W., Zhang, Q., Jiang, Q., Canonaco, F., Prévôt, A. S. H., Fu, P., Li, J., Jayne, J., Worsnop, D. R., and Wang, Z.: Source apportionment of organic aerosol from 2-year highly time-resolved measurements by an aerosol chemical speciation monitor in Beijing, China, Atmos. Chem. Phys., 18, 8469–8489, https://doi.org/10.5194/acp-18-8469-2018, 2018.
Sun, Y., He, Y., Kuang, Y., Xu, W., Song, S., Ma, N., Tao, J., Cheng, P., Wu, C., Su, H., Cheng, Y., Xie, C., Chen, C., Lei, L., Qiu, Y., Fu, P., Croteau, P., and Worsnop, D. R.: Chemical Differences Between PM1 and PM2.5 in Highly Polluted Environment and Implications in Air Pollution Studies, Geophys. Res. Lett., 47, e2019GL086288, https://doi.org/10.1029/2019GL086288, 2020.
Sun, Y. L., Wang, Z. F., Du, W., Zhang, Q., Wang, Q. Q., Fu, P. Q., Pan, X. L., Li, J., Jayne, J., and Worsnop, D. R.: Long-term real-time measurements of aerosol particle composition in Beijing, China: seasonal variations, meteorological effects, and source analysis, Atmos. Chem. Phys., 15, 10149–10165, https://doi.org/10.5194/acp-15-10149-2015, 2015.
Tan, Z., Fuchs, H., Lu, K., Hofzumahaus, A., Bohn, B., Broch, S., Dong, H., Gomm, S., Häseler, R., He, L., Holland, F., Li, X., Liu, Y., Lu, S., Rohrer, F., Shao, M., Wang, B., Wang, M., Wu, Y., Zeng, L., Zhang, Y., Wahner, A., and Zhang, Y.: Radical chemistry at a rural site (Wangdu) in the North China Plain: observation and model calculations of OH, HO2 and RO2 radicals, Atmos. Chem. Phys., 17, 663–690, https://doi.org/10.5194/acp-17-663-2017, 2017.
Tan, Z., Rohrer, F., Lu, K., Ma, X., Bohn, B., Broch, S., Dong, H., Fuchs, H., Gkatzelis, G. I., Hofzumahaus, A., Holland, F., Li, X., Liu, Y., Liu, Y., Novelli, A., Shao, M., Wang, H., Wu, Y., Zeng, L., Hu, M., Kiendler-Scharr, A., Wahner, A., and Zhang, Y.: Wintertime photochemistry in Beijing: observations of ROx radical concentrations in the North China Plain during the BEST-ONE campaign, Atmos. Chem. Phys., 18, 12391–12411, https://doi.org/10.5194/acp-18-12391-2018, 2018.
Tang, G., Zhang, J., Zhu, X., Song, T., Münkel, C., Hu, B., Schäfer, K., Liu, Z., Zhang, J., Wang, L., Xin, J., Suppan, P., and Wang, Y.: Mixing layer height and its implications for air pollution over Beijing, China, Atmos. Chem. Phys., 16, 2459–2475, https://doi.org/10.5194/acp-16-2459-2016, 2016.
Tao, S., Ru, M. Y., Du, W., Zhu, X., Zhong, Q. R., Li, B. G., Shen, G. F., Pan, X. L., Meng, W. J., Chen, Y. L., Shen, H. Z., Lin, N., Su, S., Zhuo, S. J., Huang, T. B., Xu, Y., Yun, X., Liu, J. F., Wang, X. L., Liu, W. X., Cheng, H. F., and Zhu, D. Q.: Quantifying the rural residential energy transition in China from 1992 to 2012 through a representative national survey, Nat. Energy, 3, 567–573, https://doi.org/10.1038/s41560-018-0158-4, 2018.
Travis, K. R., Jacob, D. J., Fisher, J. A., Kim, P. S., Marais, E. A., Zhu, L., Yu, K., Miller, C. C., Yantosca, R. M., Sulprizio, M. P., Thompson, A. M., Wennberg, P. O., Crounse, J. D., St. Clair, J. M., Cohen, R. C., Laughner, J. L., Dibb, J. E., Hall, S. R., Ullmann, K., Wolfe, G. M., Pollack, I. B., Peischl, J., Neuman, J. A., and Zhou, X.: Why do models overestimate surface ozone in the Southeast United States?, Atmos. Chem. Phys., 16, 13561–13577, https://doi.org/10.5194/acp-16-13561-2016, 2016.
Tsigaridis, K., Daskalakis, N., Kanakidou, M., Adams, P. J., Artaxo, P., Bahadur, R., Balkanski, Y., Bauer, S. E., Bellouin, N., Benedetti, A., Bergman, T., Berntsen, T. K., Beukes, J. P., Bian, H., Carslaw, K. S., Chin, M., Curci, G., Diehl, T., Easter, R. C., Ghan, S. J., Gong, S. L., Hodzic, A., Hoyle, C. R., Iversen, T., Jathar, S., Jimenez, J. L., Kaiser, J. W., Kirkevåg, A., Koch, D., Kokkola, H., Lee, Y. H., Lin, G., Liu, X., Luo, G., Ma, X., Mann, G. W., Mihalopoulos, N., Morcrette, J.-J., Müller, J.-F., Myhre, G., Myriokefalitakis, S., Ng, N. L., O'Donnell, D., Penner, J. E., Pozzoli, L., Pringle, K. J., Russell, L. M., Schulz, M., Sciare, J., Seland, Ø., Shindell, D. T., Sillman, S., Skeie, R. B., Spracklen, D., Stavrakou, T., Steenrod, S. D., Takemura, T., Tiitta, P., Tilmes, S., Tost, H., van Noije, T., van Zyl, P. G., von Salzen, K., Yu, F., Wang, Z., Wang, Z., Zaveri, R. A., Zhang, H., Zhang, K., Zhang, Q., and Zhang, X.: The AeroCom evaluation and intercomparison of organic aerosol in global models, Atmos. Chem. Phys., 14, 10845–10895, https://doi.org/10.5194/acp-14-10845-2014, 2014.
Turpin, B. J., Saxena, P., and Andrews, E.: Measuring and simulating particulate organics in the atmosphere: problems and prospects, Atmos. Environ., 34, 2983–3013, https://doi.org/10.1016/s1352-2310(99)00501-4, 2000.
Wang, G., Zhang, R., Gomez, M. E., Yang, L., Levy Zamora, M., Hu, M., Lin, Y., Peng, J., Guo, S., Meng, J., Li, J., Cheng, C., Hu, T., Ren, Y., Wang, Y., Gao, J., Cao, J., An, Z., Zhou, W., Li, G., Wang, J., Tian, P., Marrero-Ortiz, W., Secrest, J., Du, Z., Zheng, J., Shang, D., Zeng, L., Shao, M., Wang, W., Huang, Y., Wang, Y., Zhu, Y., Li, Y., Hu, J., Pan, B., Cai, L., Cheng, Y., Ji, Y., Zhang, F., Rosenfeld, D., Liss, P. S., Duce, R. A., Kolb, C. E., and Molina, M. J.: Persistent sulfate formation from London Fog to Chinese haze, P. Natl. Acad. Sci. USA, 113, 13630–13635, https://doi.org/10.1073/pnas.1616540113, 2016.
Wang, H., Chen, J., and Lu, K.: Development of a portable cavity-enhanced absorption spectrometer for the measurement of ambient NO3 and N2O5: experimental setup, lab characterizations, and field applications in a polluted urban environment, Atmos. Meas. Tech., 10, 1465–1479, https://doi.org/10.5194/amt-10-1465-2017, 2017a.
Wang, H., Lu, K., Chen, X., Zhu, Q., Chen, Q., Guo, S., Jiang, M., Li, X., Shang, D., Tan, Z., Wu, Y., Wu, Z., Zou, Q., Zheng, Y., Zeng, L., Zhu, T., Hu, M., and Zhang, Y.: High N2O5 Concentrations Observed in Urban Beijing: Implications of a Large Nitrate Formation Pathway, Environ. Sci. Tech. Let., 4, 416–420, https://doi.org/10.1021/acs.estlett.7b00341, 2017b.
Wang, H., Lu, K., Chen, X., Zhu, Q., Wu, Z., Wu, Y., and Sun, K.: Fast particulate nitrate formation via N2O5 uptake aloft in winter in Beijing, Atmos. Chem. Phys., 18, 10483–10495, https://doi.org/10.5194/acp-18-10483-2018, 2018.
Wang, K., Zhang, Y., Nenes, A., and Fountoukis, C.: Implementation of dust emission and chemistry into the Community Multiscale Air Quality modeling system and initial application to an Asian dust storm episode, Atmos. Chem. Phys., 12, 10209–10237, https://doi.org/10.5194/acp-12-10209-2012, 2012.
Wang, M., Shao, M., Chen, W., Lu, S., Liu, Y., Yuan, B., Zhang, Q., Zhang, Q., Chang, C.-C., Wang, B., Zeng, L., Hu, M., Yang, Y., and Li, Y.: Trends of non-methane hydrocarbons (NMHC) emissions in Beijing during 2002–2013, Atmos. Chem. Phys., 15, 1489–1502, https://doi.org/10.5194/acp-15-1489-2015, 2015.
Wang, Y., Zhang, Q. Q., He, K., Zhang, Q., and Chai, L.: Sulfate-nitrate-ammonium aerosols over China: response to 2000–2015 emission changes of sulfur dioxide, nitrogen oxides, and ammonia, Atmos. Chem. Phys., 13, 2635–2652, https://doi.org/10.5194/acp-13-2635-2013, 2013.
Wang, Y., Zhang, Q., Jiang, J., Zhou, W., Wang, B., He, K., Duan, F., Zhang, Q., Philip, S., and Xie, Y.: Enhanced sulfate formation during China's severe winter haze episode in January 2013 missing from current models, J. Geophys. Res.-Atmos., 119, 10425–10440, https://doi.org/10.1002/2013JD021426, 2014.
Wen, L., Xue, L., Wang, X., Xu, C., Chen, T., Yang, L., Wang, T., Zhang, Q., and Wang, W.: Summertime fine particulate nitrate pollution in the North China Plain: increasing trends, formation mechanisms and implications for control policy, Atmos. Chem. Phys., 18, 11261–11275, https://doi.org/10.5194/acp-18-11261-2018, 2018.
Wesely, M. L.: Parameterization of Surface Resistances to Gaseous Dry Deposition in Regional-Scale Numerical-Models, Atmos. Environ., 23, 1293–1304, https://doi.org/10.1016/0004-6981(89)90153-4, 1989.
Xu, W., Liu, L., Cheng, M., Zhao, Y., Zhang, L., Pan, Y., Zhang, X., Gu, B., Li, Y., Zhang, X., Shen, J., Lu, L., Luo, X., Zhao, Y., Feng, Z., Collett Jr., J. L., Zhang, F., and Liu, X.: Spatial–temporal patterns of inorganic nitrogen air concentrations and deposition in eastern China, Atmos. Chem. Phys., 18, 10931–10954, https://doi.org/10.5194/acp-18-10931-2018, 2018.
Xu, Z., Liu, M., Zhang, M., Song, Y., Wang, S., Zhang, L., Xu, T., Wang, T., Yan, C., Zhou, T., Sun, Y., Pan, Y., Hu, M., Zheng, M., and Zhu, T.: High efficiency of livestock ammonia emission controls in alleviating particulate nitrate during a severe winter haze episode in northern China, Atmos. Chem. Phys., 19, 5605–5613, https://doi.org/10.5194/acp-19-5605-2019, 2019.
Ye, C., Zhang, N., Gao, H., and Zhou, X.: Photolysis of Particulate Nitrate as a Source of HONO and NOx, Environ. Sci. Technol., 51, 6849–6856, https://doi.org/10.1021/acs.est.7b00387, 2017.
Ye, C., Liu, P., Ma, Z., Xue, C., Zhang, C., Zhang, Y., Liu, J., Liu, C., Sun, X., and Mu, Y.: High H2O2 Concentrations Observed during Haze Periods during the Winter in Beijing: Importance of H2O2 Oxidation in Sulfate Formation, Environ. Sci. Tech. Let., 5, 757–763, https://doi.org/10.1021/acs.estlett.8b00579, 2018.
Young, P. J., Naik, V., Fiore, A. M., Gaudel, A., Guo, J., Lin, M. Y., Neu, J. L., Parrish, D. D., Rieder, H. E., Schnell, J. L., Tilmes, S., Wild, O., Zhang, L., Ziemke, J., Brandt, J., Delcloo, A., Doherty, R. M., Geels, C., Hegglin, M. I., Hu, L., Im, U., Kumar, R., Luhar, A., Murray, L., Plummer, D., Rodriguez, J., Saiz-Lopez, A., Schultz, M. G., Woodhouse, M. T., and Zeng, G.: Tropospheric Ozone Assessment Report: Assessment of global-scale model performance for global and regional ozone distributions, variability, and trends, Elementa-Sci. Anthrop., 6, 49, https://doi.org/10.1525/elementa.265, 2018.
Zhang, J., An, J., Qu, Y., Liu, X., and Chen, Y.: Impacts of potential HONO sources on the concentrations of oxidants and secondary organic aerosols in the Beijing-Tianjin-Hebei region of China, Sci. Total Environ., 647, 836–852, https://doi.org/10.1016/j.scitotenv.2018.08.030, 2018.
Zhang, L., Wang, T., Zhang, Q., Zheng, J., Xu, Z., and Lv, M.: Potential sources of nitrous acid (HONO) and their impacts on ozone: A WRF-Chem study in a polluted subtropical region, J. Geophys. Res.-Atmos., 121, 3645–3662, https://doi.org/10.1002/2015JD024468, 2016.
Zhang, L., Chen, Y., Zhao, Y., Henze, D. K., Zhu, L., Song, Y., Paulot, F., Liu, X., Pan, Y., Lin, Y., and Huang, B.: Agricultural ammonia emissions in China: reconciling bottom-up and top-down estimates, Atmos. Chem. Phys., 18, 339–355, https://doi.org/10.5194/acp-18-339-2018, 2018.
Zhang, Q., Yuan, B., Shao, M., Wang, X., Lu, S., Lu, K., Wang, M., Chen, L., Chang, C.-C., and Liu, S. C.: Variations of ground-level O3 and its precursors in Beijing in summertime between 2005 and 2011, Atmos. Chem. Phys., 14, 6089–6101, https://doi.org/10.5194/acp-14-6089-2014, 2014.
Zhang, W., Guo, J., Miao, Y., Liu, H., Zhang, Y., Li, Z., and Zhai, P.: Planetary boundary layer height from CALIOP compared to radiosonde over China, Atmos. Chem. Phys., 16, 9951–9963, https://doi.org/10.5194/acp-16-9951-2016, 2016.
Zhao, B., Wang, S., Donahue, N. M., Jathar, S. H., Huang, X., Wu, W., Hao, J., and Robinson, A. L.: Quantifying the effect of organic aerosol aging and intermediate-volatility emissions on regional-scale aerosol pollution in China, Sci. Rep., 6, 28815, https://doi.org/10.1038/srep28815, 2016.
Zhao, Y., Zhang, L., Chen, Y., Liu, X., Xu, W., Pan, Y., and Duan, L.: Atmospheric nitrogen deposition to China: A model analysis on nitrogen budget and critical load exceedance, Atmos. Environ., 153, 32–40, https://doi.org/10.1016/j.atmosenv.2017.01.018, 2017.
Zheng, B., Zhang, Q., Zhang, Y., He, K. B., Wang, K., Zheng, G. J., Duan, F. K., Ma, Y. L., and Kimoto, T.: Heterogeneous chemistry: a mechanism missing in current models to explain secondary inorganic aerosol formation during the January 2013 haze episode in North China, Atmos. Chem. Phys., 15, 2031–2049, https://doi.org/10.5194/acp-15-2031-2015, 2015.
Zheng, G. J., Duan, F. K., Su, H., Ma, Y. L., Cheng, Y., Zheng, B., Zhang, Q., Huang, T., Kimoto, T., Chang, D., Pöschl, U., Cheng, Y. F., and He, K. B.: Exploring the severe winter haze in Beijing: the impact of synoptic weather, regional transport and heterogeneous reactions, Atmos. Chem. Phys., 15, 2969–2983, https://doi.org/10.5194/acp-15-2969-2015, 2015.
Zheng, Y., Cheng, X., Liao, K., Li, Y., Li, Y. J., Huang, R.-J., Hu, W., Liu, Y., Zhu, T., Chen, S., Zeng, L., Worsnop, D. R., and Chen, Q.: Characterization of anthropogenic organic aerosols by TOF-ACSM with the new capture vaporizer, Atmos. Meas. Tech., 13, 2457–2472, https://doi.org/10.5194/amt-13-2457-2020, 2020.