the Creative Commons Attribution 4.0 License.
the Creative Commons Attribution 4.0 License.
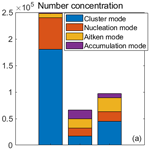
Variation of size-segregated particle number concentrations in wintertime Beijing
Ying Zhou
Yiliang Liu
Yueyun Fu
Juha Kangasluoma
Tommy Chan
Kaspar R. Daellenbach
Federico Bianchi
Tom V. Kokkonen
Yongchun Liu
Joni Kujansuu
Veli-Matti Kerminen
Tuukka Petäjä
Jingkun Jiang
Markku Kulmala
The spatial and temporal variability of the number size distribution of aerosol particles is an indicator of the dynamic behavior of Beijing's atmospheric pollution cocktail. This variation reflects the strength of different primary and secondary sources, such as traffic and new particle formation, as well as the main processes affecting the particle population. In this paper, we report size-segregated particle number concentrations observed at a newly developed Beijing station during the winter of 2018. Our measurements covered particle number size distributions over the diameter range of 1.5 nm–1 µm (cluster mode, nucleation mode, Aitken mode and accumulation mode), thus being descriptive of a major fraction of the processes taking place in the atmosphere of Beijing. Here we focus on explaining the concentration variations in the observed particle modes, by relating them to the potential aerosol sources and sinks, and on understanding the connections between these modes. We considered haze days and new particle formation event days separately. Our results show that during the new particle formation (NPF) event days increases in cluster mode particle number concentration were observed, whereas during the haze days high concentrations of accumulation mode particles were present. There was a tight connection between the cluster mode and nucleation mode on both NPF event and haze days. In addition, we correlated the particle number concentrations in different modes with concentrations of trace gases and other parameters measured at our station. Our results show that the particle number concentration in all the modes correlated with NOx, which reflects the contribution of traffic to the whole submicron size range. We also estimated the contribution of ion-induced nucleation in Beijing, and we found this contribution to be negligible.
- Article
(1068 KB) - Full-text XML
-
Supplement
(374 KB) - BibTeX
- EndNote
Atmospheric aerosols are the main ingredient of China's pollution cocktail (Kulmala, 2015). Aerosols have gained increasing attention due to their effects on human heath, climate and visibility (Lelieveld et al., 2015; IPCC, 2007). Currently, air quality standards for cities in China consider particle mass instead of number concentration (WHO, 2000), which may ignore the potential adverse effect of ultrafine particles on health (diameter less than 100 nm). It has been shown that ultrafine particles can penetrate deep into the respiratory tract, ending up in the blood circulation, which allows them to accumulate in the brain (Oberdörster et al., 2004). Indeed, studies have pointed out that ultrafine particles, which contribute to a negligible fraction of the mass concentration, dominate the total number concentration in urban areas (von Bismarck-Osten et al., 2013; Wehner et al., 2004; Wu et al., 2008). Due to their high concentrations, ultrafine particles' toxicological effects are enhanced by their large total surface area (Kreyling et al., 2004).
Apart from their health effects, the temporal and spatial variation of particle number concentrations of different sizes is a good indicator of the strength of their emission sources. Aerosols are emitted directly as primary particles, such as sea salt or dust particles; as a result of natural phenomena (Solomos et al., 2011); or they can be formed through new particle formation (Kulmala, 2003; Kulmala et al., 2004, 2013; Kerminen et al., 2018; Chu et al., 2019). Newly formed particles can grow up to diameters of 20–100 nm within a day (Kulmala et al., 2004), and they have been found to contribute to a major fraction of the global cloud condensation nuclei (CCN) population, thus indirectly affecting the climate (Kerminen et al., 2012). For all aforementioned reasons, and in order to form a collective and complete picture about atmospheric aerosol particles to understand their origin and potential impacts at a specific location, the whole size distribution of these particles needs to be studied.
Recently, due to urbanization and increased population, megacities have increased their contribution to atmospheric aerosol pollution massively (Baklanov et al., 2016). Interestingly, more people live inside eastern Asia (specifically, China and India) than outside this region (https://www.unfpa.org/swop, last access: 1 November 2019). Therefore, it is important to study the contributions of different sources to size-segregated number concentrations in order to inspire policy makers and the public on measures that need to be taken in order to reduce particulate pollution. Many studies in various cities in China have tackled this topic. For instance, 2 years of observations of particle number size distributions at a site in northern Beijing reported that traffic emissions were the major source of nucleation (3–20 nm) and Aitken (20–100 nm) mode particles in urban Beijing (Wang et al., 2013). On the other hand, research conducted in western downtown Nanjing reported that local new particle formation events were the main contributors to both nucleation (5–20 nm) mode and CCN particle populations (Dai et al., 2017). Measurements of nucleation mode particle concentrations in urban Hong Kong reported the dominant contribution of combustion sources to the nucleation mode (5.5–10 nm) (D. W. Wang et al., 2014), whereas observations in urban Guangzhou found that accumulation and secondary transformation of particles were the main reasons for high concentrations of accumulation mode particles (100–660 nm) (Yue et al., 2010). However, only a few studies in China have reported measurements of cluster mode (sub-3 nm) particles and related them to new particle formation events (Cai et al., 2017; Xiao et al., 2015; Yao et al., 2018; Yu et al., 2016).
The observation of sub-3 nm particles and ions has been made possible by recent major developments in instrumentation, such as the particle size magnifier (PSM) (Vanhanen et al., 2011), diethylene glycol-based scanning mobility particle sizer (DEG-SMPS) (Jiang et al., 2011) and neutral cluster and air ion spectrometers (NAISs) (Manninen et al., 2016; Mirme et al., 2007).
In complicated environments like Beijing, it is very hard to relate each particle mode to a specific source. Indeed, several sources could contribute to aerosol particles in the same size range. For instance, cluster mode particles mainly originate from secondary gas-to-particle transformation processes (Kulmala et al., 2013), although recently also traffic has been identified as a source for these particles (Rönkkö et al., 2017). While cluster mode particles can grow into the Aitken mode, other sources like traffic also contribute to this mode, making the source identification of the Aitken mode complicated (Pirjola et al., 2012). Various anthropogenic activities and biogenic processes contribute to accumulation mode particle sizes. Thus, correlating trace gases and aerosol concentrations of different sizes during different time periods help narrow down these aerosol sources.
In this study, we analyzed the number concentration of four submicron aerosol modes: cluster mode (sub-3 nm), nucleation mode (3–25 nm), Aitken mode (25–100 nm) and accumulation mode (100–1000 nm). Our aims were (i) to investigate the number concentration variations of size-segregated aerosol number concentrations for each mode, (ii) to explore the relationships between the different modes under different atmospheric conditions, (iii) to connect the number size distribution modes with multiple trace gases (NOx, SO2, CO and O3) and PM2.5 (particulate matter with aerodynamic diameter less than 2.5 µm); and (iv) to quantify the contribution of new particle formation (NPF) and haze formation to different particle modes in wintertime in Beijing. Our work increases understanding on the sources of the different sized particles in Beijing, China, and the work complements studies in other megacities.
2.1 Description of the Beijing station
Beijing, as the capital of China, accommodates more than 20 million people within 16 800 km2, and only 1400 km2 of urban areas, with expanding economic activity, construction and industry. Beijing, as one of the largest megacities in the world, is located in the North China Plain, and it is one of the most industrialized regions in China. Mountains surround Beijing from the west, north and northwest.
For our study, we analyzed data collected at the newly developed station which is part of the Aerosol and Haze Laboratory in Beijing. The urban station follows the concept of Station for Measuring Ecosystem and Atmospheric Relations (SMEAR) (Hari and Kulmala, 2005). Our station is located on the western campus of Beijing University of Chemical Technology (BUCT). It is constructed on the fifth floor of the teaching building on the campus. The sampling lines extend to the rooftop of the building around 20 m above the ground level, or they are going directly through windows for selected instruments. The station represents a typical area in urban Beijing that is subject to pollution sources, such as traffic, cooking and long-range transport of pollution. The campus is surrounded by highways and main roads from the east (3rd Ring Road), north (Zizhuyuan Road) and southeast (Zizhu Bridge). From the east, west and south, the campus is surrounded by residential and commercial areas.
Measurements at Beijing started on 16 January 2018 (Lu et al., 2019). Our measurements continued until present, except during the necessary instrument maintenance periods and unavoidable factors such as power cuts. The data included in this study were collected between 16 January and 15 March 2018, being representative of Beijing winter conditions.
2.2 Instrumentation
For a comprehensive measurement of particles, a full set of particle measuring instrumentation was operated. First, a nano-condensation nucleus counter system (nCNC), consisting of a particle size magnifier (PSM, model A10, Airmodus Oy, Finland) and a butanol condensation particle counter (CPC) (model A20, Airmodus Oy, Finland) measured the number concentration of small clusters or particles of 1.2–2.5 nm in mobility diameter (Vanhanen et al., 2011). To minimize the sampling losses, the PSM was sampling horizontally through a window to the north through a short stainless-steel sampling inlet extending ∼1.2 m outward from the building. The length of the sampling tube was 1.33 m and its inner diameter was 0.8 cm. To further improve the sampling efficiency, a core sampling tube (Kangasluoma et al., 2016) was utilized. The total flow rate was 7.5 L min−1, from which 5 L min−1 was used as a transport flow while the nCNC sample flow rate was 2.5 L min−1. In the operation of the PSM, the saturator flow rate scanned from 0.1 to 1.3 L min−1 and scanned back from 1.3 to 0.1 L min−1 within 240 s. We averaged the data over six scans to make it smoother, and therefore the time resolution of PSM data was 12 min. The data were inverted with a kernel function method (Chan et al., 2020). When comparing the particle number concentrations obtained with the expectation-maximization method, the cluster mode particle number concentration was, on average, 2 times higher on the NPF event days and 11 times higher on the haze days (Cai et al., 2018). Therefore, there is some uncertainty in the reported cluster mode particle concentrations.
A particle size distribution (PSD) system measured the particle number size distribution in the size range of 3–10 000 nm (Liu et al., 2016). It included a nano-scanning mobility particle sizer (nano-SMPS, 3–55 nm, mobility diameter), a long SMPS (25–650 nm, mobility diameter) and an aerodynamic particle sizer (APS, 0.55–10 µm, aerodynamic diameter). The PSD system sampled from the rooftop using a 3 m long sampling tube. A cyclone that removed particles larger than 10 µm was added in front of the sample line. The time resolution of the PSD system data was 5 min.
A neutral cluster and air ion spectrometer (NAIS, model 4-11, Airel, Estonia) measured number size distributions of particles (2.5–42 nm, mobility diameter) and ions (0.7–42 nm, mobility diameter) (Manninen et al., 2016; Mirme and Mirme, 2013). It switched between detecting either naturally charged ions or total particles (including the uncharged fraction) with unipolar charging. It measured 2 min in the neutral mode, 2 min in the ion mode and then offset for 30 s for every measurement cycle. The NAIS was sampling horizontally from the north window. The copper sampling tube with an outer diameter of 4 cm extended 1.6 m outside the window. To increase the sampling efficiency, the sampling flow rate was 54 L min−1.
The trace-gas monitors measured carbon monoxide (CO), sulfur dioxide (SO2), nitrogen oxides (NOx) and ozone (O3) concentrations with Thermo Environmental Instruments models 48i, 43i-TLE, 42i, and 49i, respectively. They all sampled through a common inlet through the roof of the building. The length of the sampling tube was approximately 3 m. The time resolution of CO, NOx, and O3 data were 5 min, whereas the time resolution of SO2 data was 1 h before 22 January 2018 and 5 min after that.
The PM2.5 data were obtained from the nearest national monitoring station, Wanliu station, around 3 km north of our station. The PM2.5 data from Wanliu station compared nicely with the PM2.5 data from three other adjacent national stations. The time resolution of the PM2.5 data was 1 h, and these data were recorded every hour. Detailed information is reported in Cao et al. (2014).
We measured the relative humidity (RH, %), visibility (km), wind speed (m s−1) and wind direction (∘) from a weather station on the roof of our station.
When data sets having different time resolutions were used, we chose the lowest time resolution as the common time resolution. Data with higher time resolutions were merged to the common time resolution by taking median numbers between two time points of the new time series.
2.3 NPF events and haze days classification
We classified days into “NPF event days” and “haze days”. The days that did not fit either of these two categories were marked as “other days”, and they were excluded from our future analysis unless otherwise specified. We observed 28 NPF event days and 24 haze days in total, between 16 January and 15 March 2018, which are the dates included in this study. Figure 1 describes the specific calendar of events with the aforementioned categories of days.
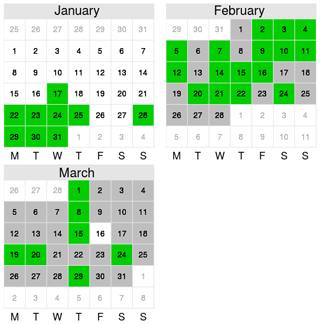
Figure 1Calendar of different types of days during our observations. NPF event days are marked in green and haze days are marked in gray, whereas missing or undefined days are marked in white. The data included in this study are between January 16 and March 15.
We identified the NPF event days following the method introduced in Dal Maso et al. (2005), which requires an appearance of a new mode below 25 nm and that the new mode shows signs of growth for several hours (Dal Maso et al., 2005; Kulmala et al., 2012). Haze events were identified as having a visibility less than 10 km and ambient relative humidity below 80 % (China Meteorological Administration). Individual days were classified as haze days when the haze event lasted for at least 12 consecutive hours. During our study periods, there was no overlap between the NPF events and haze days, as these two phenomena never occurred during the same time period. While the NPF events appeared right after sunrise and lasted for several hours, the haze events did not have any specific time of appearance but lasted from a few hours up to several days.
The particle number size distribution was divided into four modes according to their diameter: cluster mode (sub-3 nm), nucleation mode (3–25 nm), Aitken mode (25–100 nm), and accumulation mode (100–1000 nm). We calculated cluster mode particle number concentrations the using the PSM data, nucleation mode particle number concentration using the NAIS particle mode data, and Aitken and accumulation mode particle number concentrations using the PSD system data. The particle PSD and NAIS had an overlapping particle size distribution over the mobility diameter range of 3–42 nm. As shown in Fig. S1 in the Supplement, total particle number concentrations from the NAIS and PSD system correlated well with each other on both NPF event days (R2 was 0.92) and haze days (R2 was 0.90) in the overlapping size range. The slopes between the total particle number concentration from the PSD system and that from the NAIS were 0.90 and 0.85 on the NPF event days and haze days, respectively. The particle number size distribution in the overlapping size range of the NAIS and PSD system matched well on both NPF event days and haze days as shown in Fig. S2.
Moreover, since new particle formation events were only observed during daytime in Beijing, our analysis concentrated mostly on the time period 08:00 to 14:00 LT, local time, unless specified otherwise.
2.4 Parameter calculation
2.4.1 Calculation of the growth rate
The growth rates of cluster and nucleation mode particles were calculated from positive ion data and particle data from neutral cluster and air ion spectrometer (NAIS), respectively, by using the appearance time method introduced by Lehtipalo et al. (2014). In this method, the particle number concentration of particles of size dp is recorded as a function of time, and the appearance time of particles of size dp is determined as the time when their number concentration reaches 50 % of its maximum value during new particle formation (NPF) events.
The growth rates (GRs) were calculated according to
where t2 and t1 are the appearance times of particles with sizes of dp2 and dp1, respectively. Figure S3 shows an example of how this method was used.
2.4.2 Calculation of the coagulation sink
The coagulation sink (CoagS) was calculated according to Eq. (2), introduced by Kulmala et al. (2012):
where K(dp, d′p) is the coagulation coefficient of particles with sizes of dp and d′p, and is the particle number concentration with size of d′p.
2.4.3 Calculation of the formation rate
The formation rate of 1.5 nm particles (J1.5) was calculated using particle number concentrations measured with a PSM. The formation rate of 1.5 nm ions () was calculated using positive and negative ions data from the NAIS as well as PSM data. The upper limit used was 3 nm. The values of J1.5 and were calculated following the methods introduced by Kulmala et al. (2012) with Eqs. (3) and (4), respectively:
where CoagSdp is the coagulation sink in the size range of and GR is the growth rate.
The fourth and fifth terms on the right-hand side of Eq. (4) represent ion–ion recombination and charging of neutral particles by smaller ions, respectively; α is the ion–ion recombination coefficient and χ is the ion–aerosol attachment coefficient.
3.1 General character of particle modes and trace gases
3.1.1 Submicron particles and PM2.5
Particle number concentrations of different modes varied depending on the period, as shown in Fig. 2. We observed that the cluster and nucleation mode particle concentrations were the highest on the NPF event days. In fact, the cluster and nucleation mode particles dominated the total particle number concentration with an average contribution of 96 % (Fig. 3). On the haze days, the average contribution levels of the four modes were about equal. Aitken and accumulation mode particles contributed to 52 % of the total particle number concentration on the haze days, as compared to 4 % on the NPF event days.
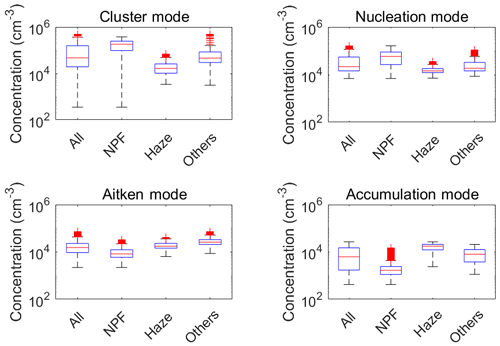
Figure 2Particle number concentrations in the cluster, nucleation, Aitken and accumulation modes on all the days, NPF event days, haze days and other days. The whiskers include 99.3 % of data of every group. Data out of 1.5 times the interquartile range are located outside of the whiskers and considered outliers. The boxes show the median (red line), 25th and 75th percentiles. Data marked with red plus symbols represent outliers.
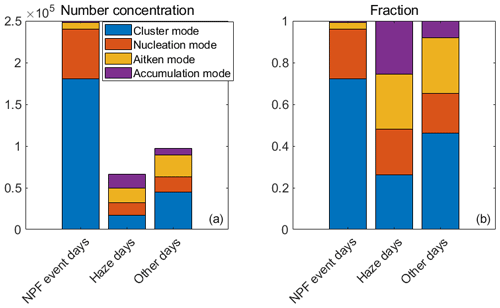
Figure 3The median size-segregated number concentrations (a) and the median fraction of each mode to the total particle number concentration (b) on the NPF event days, haze days and other days.
On the haze days, we observed a surprising concentration of cluster mode particles in spite of the high concentrations of Aitken and accumulation particles. Since large particles are expected to efficiently scavenge clusters and slow-growing particles by coagulation (Kerminen et al., 2001; Kulmala et al., 2017), this is indicative of either airborne cluster formation (Kulmala et al., 2007) or vehicular emissions of clusters and nucleation mode particles (e.g., Rönkkö et al., 2017) during haze. The ratio between nucleation mode and cluster mode particle median number concentration was close to unity (0.84), which might indicate their common source on haze days, in comparison to the smaller ratio of 0.3 during the NPF days. It is therefore likely that the primary particles dominated the nucleation mode on the haze days, while the growth of cluster mode particles into nucleation mode explains the nucleation mode particles on NPF days.
The median concentrations of Aitken and accumulation mode particles were 16 000 cm−3 and 17 500 cm−3, respectively, on the haze days and 8240 cm and 1670 cm−3, respectively, on the NPF event days. Overall, these concentrations were a factor of 2.1 and 10.5 times higher, respectively, on the haze days than on the NPF event days. The PM2.5 mass concentration was clearly higher on the haze days compared with the NPF event days (Fig. 4). The PM2.5 mass concentration in urban areas is dominated by accumulation mode particles, with a clearly smaller contribution by ultrafine (cluster, nucleation and Aitken mode) particles (Feng et al., 2010).
3.1.2 Trace gases
In this work, we considered four trace gases (SO2, CO, NOx and O3) in our analysis (Fig. 5), as these compounds are most commonly used to evaluate air quality and pollution sources in China (Hao and Wang, 2005; Han et al., 2011). During our observation period, the median concentrations of SO2, CO and NOx on haze days were 5.1, 1400 and 27 ppb, respectively. While high, these concentrations are lower than the corresponding concentrations (18, 2200, 75 ppb, respectively) during the extremely severe haze episode that took place in Beijing in January 2013 (Y. S. Wang et al., 2014b). The median concentration of O3 was 10 ppb on the haze days during our observations, a little bit higher than the severe haze episode in 2013 (< 7 ppb; Y. S. Wang et al., 2014b).
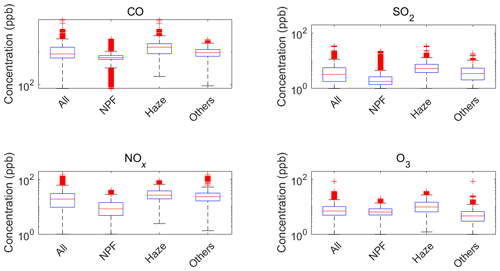
Figure 5Trace-gas mixing ratios of CO, SO2, NOx and O3 on all the days, NPF event days, haze days and other days. The boxes show the median (red line), 25th and 75th percentiles of the mixing ratios. Data marked with red plus symbols represent outliers as in Fig. 1.
The median levels of SO2, CO, NOx and O3 were 230 %, 50 %, 100 % and 50 % higher, respectively, on the haze days than on the NPF days. SO2, CO and NOx are usually considered tracers of primary pollution, so their lower levels on the NPF event days indicate that relatively clean conditions favor NPF events (Vahlsing and Smith, 2012; Tian et al., 2018).
3.2 Diurnal behavior
In order to draw a clear picture of the evolution of size-segregated particle number concentrations, we analyzed the diurnal concentration behavior of the different trace-gases (Fig. 6) and particle modes (Fig. 7).
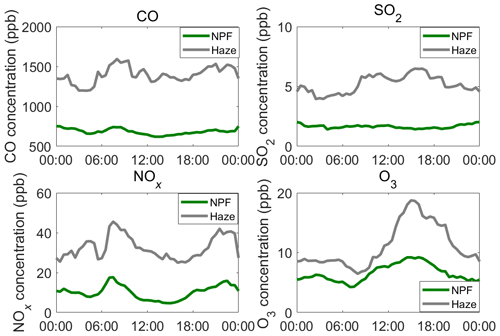
Figure 6Diurnal variation of trace-gas (CO, SO2, NOx and O3 separately) mixing ratios on the NPF event days (green lines) and haze days (gray lines) separately. The time resolution was 30 min for every data point. Every data point here represents the median of all data at the same time of the day.
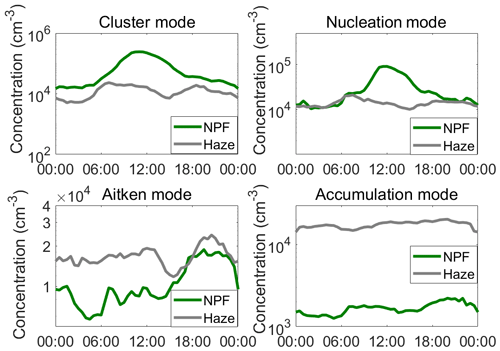
Figure 7Diurnal variation of particle number concentration of every mode (cluster, nucleation, Aitken and accumulation mode separately) on the NPF event days (green lines) and the haze days (gray lines). The time resolution was 30 min for every data point. Every data point here represents the median of all data at the same time of the day.
Since trace gases have more definitive sources than particles, we can get some insight into particle sources by comparing their diurnal patterns with those of particles in different modes. For instance, CO is usually emitted as the by-product of inefficient combustion of biomass or fossil fuels (Pétron et al., 2004; Lowry et al., 2016). We observed similar diurnal patterns for NOx and CO, with an increase during the morning rush hour followed by another peak at around 15:00 LT, suggesting similar sources. Due to lower human activities and traffic during nighttime, lower concentrations of NOx and CO were observed. Earlier observations in urban areas having high-NOx concentrations found that O3 was consumed by its reaction with NO, while NO2 works as precursor for O3 via photochemical reactions (Wang et al., 2017). In our observations, the diurnal pattern of O3 was opposite to that of NOx, which is consistent with O3 loss by large amounts of freshly emitted NO during rush hour and O3 production by photochemical reactions involving NO2 after rush hour in the morning.
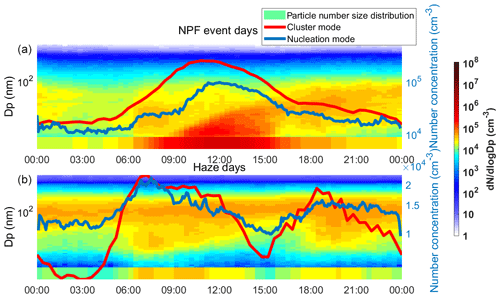
Figure 8Median diurnal patterns of the particle number size distribution over the size range of 1.5–1000 nm and number concentrations of cluster mode (red lines) and nucleation mode (blue lines) particles on the NPF event days (a) and haze days (b). The time resolution of every data point of particle number size distribution and cluster mode particle number concentration was 12 min. The time resolution of every data point of nucleation mode particle number concentration was 5 min.
In Fig. 8, we show the median diurnal pattern of particle number size distribution on the NPF event days and haze days separately. On the NPF event days, we observed cluster formation from diameters smaller than 3 nm. The growth of newly formed particles lasted for several hours, resulting in a consecutive increase in the particle number concentrations in all four modes. During traffic rush hour in the morning and evening, we observed an increase in particle number concentrations in the size range of cluster mode to around 100 nm.
On the haze days, we still observed an increase in particle number concentration in the size range of cluster mode to Aitken mode during rush hour. Traditionally, NPF events occur during the time window between sunrise and sunset by photochemical reactions (Kerminen et al., 2018; Dada et al., 2018). The binary or ternary nucleation between sulfuric acid and water, ammonia, or amines are usually thought of as sources of atmospheric cluster mode particles, especially in heavily polluted environments (Kulmala et al., 2013, 2014; Yao et al., 2018; Chu et al., 2019). The burst of cluster mode particle number concentration outside the traditional NPF time window, especially during the rush hour in the afternoon, suggests a very different source of cluster mode particles from traditional nucleation, e.g., nucleation from gases emitted by traffic (Rönkkö et al., 2017).
As shown in Fig. 7, on the NPF event days, the cluster mode particle number concentration started to increase at the time of sunrise and peaked around noon with a wide single peak, showing the typical behavior related to NPF events (Kulmala et al., 2012). Comparatively, on the haze days, the cluster mode particle number concentration showed a double peak pattern similar to the diurnal cycle of NOx (Fig. 6). This observation is consistent with our discussion above that traffic emission possibly contributed to cluster mode particles. By comparing cluster mode particle number concentrations between the haze days and NPF event days, we estimated that traffic-related cluster mode particles could contribute up to 40 %–50 % of the total cluster mode particle number concentration on the NPF event days.
Similar to the cluster mode, the nucleation mode had a single peak for the NPF event days. Nucleation mode particle number concentrations started to increase shortly after the corresponding increases in the cluster mode, which could be attributed to the growth of cluster mode particles into the nucleation mode. The observed peak of the nucleation mode particle number concentrations had a shoulder at around 07:00–09:00 LT concurrent with the morning peak of the NOx concentration, which indicates a contribution from traffic to the nucleation mode. It is important to note, however, that the height of this shoulder was only 20 % of the maximum nucleation mode particle number concentration. These results suggest that, compared with atmospheric NPF, traffic contributed much less to the nucleation mode particle number concentration.
During the haze days, the diurnal pattern of the nucleation mode particle number concentration was similar to that of NOx, showing no peak during the daytime for rush hour. This suggests that the nucleation mode particles were dominantly from traffic emissions on the haze days. Additionally, it is important to note that during the haze days, we observed different maximum concentrations for morning versus evening peaks, implying a higher contribution of traffic in the morning than in the afternoon. This result is in line with the diurnal cycle of NOx during the haze days.
On the NPF event days, Aitken mode particles are mainly attributed to two different sources that are hard to distinguish from each other: primary and secondary sources, such as combustion and growth of newly formed particles, respectively. In comparison to the cluster and nucleation modes that had pronounced diurnal cycles during the NPF event days, the Aitken mode particle number concentration had a pattern similar to NOx before 09:00 in the morning. This implies that traffic emissions were important sources to maintain Aitken mode particle concentrations in the morning hours. The Aitken mode particle number concentration increased during the afternoon hours, probably due to the growth of the nucleation mode particles via multicomponent condensation and possibly some other gas-to-particle conversion pathways. The concurrent decrease in the nucleation mode particle number concentration supports this view. The Aitken mode particle number concentration increase in the evening was concurrent with the increase in CO and NOx, which could be attributed to combustion sources (Roberts and Jones, 2004; Koponen et al., 2001).
On the haze days, the Aitken mode particle number concentration experienced little change before about 14:00 LT, contrary to both CO and NOx concentrations, indicating a small contribution by primary sources during that time of the day. It is important to mention that the growth of particles is not limited to the days when new particle formation occurs. In fact, on the haze days, the wind was typically more stagnant, reducing the vertical mixing of pollutants and their horizontal advection (Zheng et al., 2015). The increase in Aitken mode particle number concentration started at around 16:00 LT and peaked at around 20:00 LT similar to the NPF event days. This is concurrent with the increase in the NOx and CO concentrations, which might be attributed to traffic emissions.
The concentration of accumulation mode particles was an order of magnitude higher during the haze days compared with the NPF days, causing a higher condensation sink (on average 0.015 s−1 for the NPF event days and 0.10 s−1 for the haze days, as shown in Fig. S4), and thus introducing a reason why NPF did not take place on the haze days (Kulmala et al., 2017). The concentration, on the other hand, did not experience much diurnal variation. There was a slight increase in the accumulation mode particle number concentration during the morning rush hour starting at around 06:00 LT concurrent with the increase in the Aitken mode particle number concentration. The second slight increase started at around 16:00 LT, 2 h later than that of the Aitken mode, suggesting a secondary contribution to accumulation mode particles. On the NPF event days, the accumulation mode had a similar diurnal pattern as SO2, implying that SO2 participated in the formation of accumulation mode on the NPF event days.
3.3 Correlation between the particle modes, trace-gases and PM2.5 concentrations
Beijing's atmosphere is a very complicated environment (Kulmala, 2015). Aerosol particles in the atmosphere of Beijing are subject to aerosol dynamical processes, surface reactions, coagulation, deposition and transport, thus hindering direct connection with their sources based on physical size distributions only. However, by correlating each particle mode to various trace gases, we can get indications on the sources of particles. In this section, we use CO, SO2, NOx and O3 as tracers. By examining responses of size-segregated particle number concentrations to changes in trace-gas and PM2.5 concentrations (Table 1a and b), we can get further insights into the main sources of particles in each mode and into the dynamical processes experienced by these particles under different pollution levels. Of course, not all sources or dynamics can be captured using this approach. In addition, due to the complex physical and chemical processes experienced by the particles, the correlation analysis cannot quantify the strength of individual sources or dynamical processes.
Table 1(a) Correlation coefficients between size-segregated particle number concentrations and trace-gas mixing ratios and PM2.5 concentration on the NPF event days. (b) Correlation coefficients between size-segregated particle number concentrations and trace-gas mixing ratios and PM2.5 concentration on the haze days. The time window was 08:00–14:00 LT. High correlation coefficients () are marked in bold and italic.
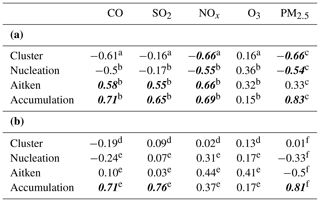
a Included 665 data points (the time resolution was 12 min), b Included 1620 data points (the time resolution was 5 min), c Included 151 data points (the time resolution was 1 h), d Included 620 data points (the time resolution was 12 min), e Included 1460 data points (the time resolution was 5 min), f Included 89 data points (the time resolution was 1 h).
3.3.1 Connection with SO2
SO2 is a key precursor for H2SO4 through photochemical reactions in Beijing, which is in turn a requirement for new particle formation in megacity environments (Wang et al., 2013; Yao et al., 2018). Although being a very important precursor of NPF, SO2 had lower concentrations on the NPF event days than on the haze days (Fig. 9). High concentrations of SO2 have been ascribed to regional pollution and an anthropogenic condensation sink even in semipristine environments (Dada et al., 2017). Earlier observations report that the main sources of SO2 are power plants, traffic and industry, so SO2 can be used as a tracer for regional pollution (Yang et al., 2018; Lu et al., 2010).
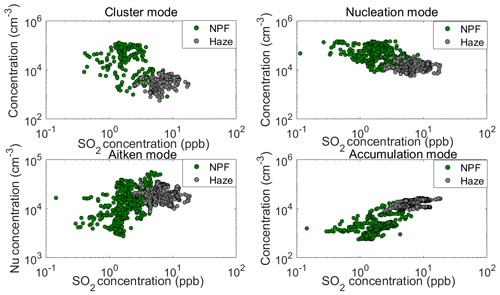
Figure 9Correlation between the SO2 concentration and particle number concentration in each mode. The time resolution of the data points was 1 h.
Generally, as shown in Fig. 9, the SO2 concentration correlated negatively with both cluster and nucleation mode particle number concentrations. Higher SO2 concentrations were encountered on more polluted days when NPF events were suppressed due to the high particle loadings, explaining the overall negative correlation. However, if we look at the NPF event days and haze days separately, we cannot see any clear correlation between the SO2 concentration and cluster mode or nucleation mode particle number concentration, as shown also in Table 1a and b. This result indicates that during our observations, NPF occurred in relatively clean conditions, but the strength of a NPF event was not sensitive to the regional pollution level as long as NPF was able to occur.
On the NPF event days, the SO2 concentration correlated positively with the concentrations of both Aitken and accumulation mode particles during the chosen NPF time window, whereas on the haze days no correlation between the SO2 concentration and Aitken mode particle number concentration could be observed. This suggests that regional and transported pollution contributed to Aitken and accumulation mode particles on the NPF event days, while on haze days the transported and regional pollution was only a prominent factor affecting accumulation mode particle number concentration. In addition, SO2 contributes to heterogeneous reactions on particle surfaces, explaining that a fraction of accumulation mode particles could have resulted from the growth of Aitken mode particles (Ravishankara, 1997).
3.3.2 Connection with NOx
NOx is usually considered the pollution tracer mainly from traffic (Beevers et al., 2012). As shown in Table 1a and Fig. 10, the NOx concentration correlated negatively with both cluster and nucleation mode particle number concentrations on the NPF event days. Compared with the correlation between SO2 and cluster and nucleation mode particle number concentrations, this result indicates that local traffic emissions affected cluster and nucleation mode particles more than regional pollution on the NPF event days.
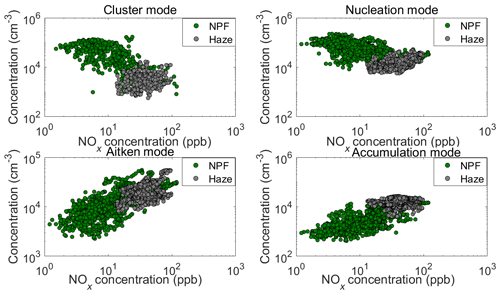
Figure 10Correlation between the NOx concentration and particle number concentration in each mode. The time resolution of the data points was 1 h.
On the haze days, we did not see any correlation between the cluster mode particle number concentration and NOx concentration (Table 1b), although according to our analysis above, traffic emissions can be the source of cluster mode particles during the haze days. One possible reason for this is that the relationship between cluster mode particle number concentration and NOx concentration was not linear. Earlier studies pointed out that the dilution ratio is the dominant factor affecting the number size distribution of nanoparticles generated from traffic gas emissions (Shi and Harrison, 1999; Shi et al., 2001). Temperature and humidity were also identified as factors affecting nanoparticle number size distribution nucleated from tailpipe emissions (Shi et al., 2001). Such factors would decrease the correlation between the cluster and nucleation mode particle number concentrations and NOx concentration.
The Aitken mode particle number concentration correlated positively with the NOx concentration on both NPF event days and haze days, suggesting that traffic emissions might be an important source of Aitken mode particles.
The accumulation mode particle number concentration correlated positively with the NOx concentration on the NPF event days, which is consistent with earlier studies showing that traffic emissions can contribute to accumulation mode particles in urban areas (Vu et al., 2015). On the haze days, the accumulation mode particle number concentration correlated less with NOx than with SO2, suggesting that regional and transported pollution was a more important contributor to accumulation mode particles than traffic emissions.
3.3.3 Connection with CO
CO has some similar sources as NOx, such as traffic. On the NPF event days, the CO concentration correlated with particle number concentrations in each mode in a very similar way as NOx did, suggesting that CO and NOx had common sources, such as traffic emissions, on the NPF event days. This result confirms our analysis above that traffic emissions could suppress NPF and growth on the NPF event days, in addition to which they might be important sources of the Aitken and accumulation mode particles.
On the haze days, CO transported from polluted areas dominated the total CO concentration. The CO concentration had a positive correlation with the accumulation mode particle number concentration, but no clear correlation with the particle number concentration of the three other modes. This result confirms our analysis above that on the haze days, local emissions dominated Aitken particle number concentrations, while regional and transported pollution affected accumulation mode particle number concentrations more than local emissions.
3.3.4 Connection with O3
Ozone is a secondary pollution trace gas, and its concentration represents the oxidative capacity of the atmosphere. Earlier observations found that high O3 concentrations favor NPF by enhancing photochemical reactions (Qi et al., 2015). However, we did not see any correlation between the O3 concentration and cluster mode particle number concentration, suggesting that O3 was not the limiting factor for cluster mode particle formation.
The O3 concentration correlated positively with both nucleation and Aitken mode particle number concentration on the NPF event days during the NPF time window, whereas on the haze days O3 concentration correlated only with the Aitken mode particle number concentration.
The above results suggest that O3 influences heterogeneous reactions and particle growth rather than the formation of new aerosol particles.
3.3.5 Connection with PM2.5
As shown in Fig. 11, the PM2.5 concentration correlated negatively with the cluster and nucleation mode particle number concentrations and positively with the accumulation mode particle number concentration. High PM2.5 concentrations tend to suppress NPF by increasing the sinks of vapors responsible for nucleation and growth of cluster and nucleation mode particles. The particles causing high PM2.5 concentrations also serve as sinks of cluster and nucleation mode particles by coagulation.
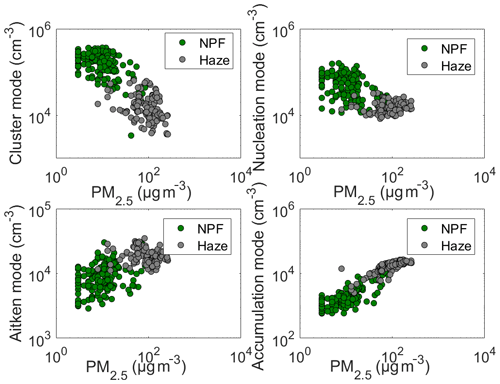
Figure 11Correlation between PM2.5 concentration and particle number concentration in each mode on the NPF event days (green dots) and the haze days (gray dots) separately. The time resolution of the data points was 1 h.
As shown in Table 1a and Fig. 13, the Aitken mode particle number concentration correlated positively with the PM2.5 concentration on the NPF event days. A possible reason for this could be the tight connection between the Aitken and accumulation mode particles on the NPF event days (Table 2a) and the observation that accumulation mode particles are usually the main contributor to PM2.5 in Beijing (Liu et al., 2013). On the haze days, the Aitken mode particle number concentration correlated negatively with the PM2.5 concentration (Table 1b). A possible reason for this is that pre-existing large particles acted as a sink for Aitken mode particles by coagulation as well as a sink for vapors responsible for the growth of smaller particles into the Aitken mode. In addition, while PM2.5 is dominated by regional and transported secondary aerosols, Aitken mode particles mainly originate from local emissions such as traffic and cooking in Beijing (Wu et al., 2007; Wang et al., 2013; Du et al., 2017; de Jesus et al., 2019).
Table 2(a) Correlation coefficients between particle number concentration of every mode on NPF event days. (b) Correlation coefficients between particle number concentration of every mode on haze days. The time window was 08:00–14:00 LT. High correlation coefficients () are marked with bold and italic.
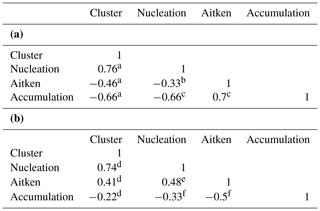
a Included 516 data points (the time resolution was 12 min). b Included 1251 data points (the time resolution was 5 min). c Included 1331 data points (the time resolution was 5 min). d Included 342 data points (the time resolution was 12 min). e Included 824 data points (the time resolution was 5 min). f Included 845 data points (the time resolution was 5 min).
3.4 Correlation between different particle modes
Table 2a and b as well as Fig. 12 shows the correlation between particle number concentrations in different modes. On the NPF event days, cluster and nucleation mode particle number concentrations correlated positively with each other due to their common dominant source, NPF. Both cluster and nucleation mode particle number concentrations correlated negatively with the Aitken and accumulation mode particle number concentrations because, as discussed earlier, high concentrations of large particles tend to suppress NPF and subsequent growth of newly formed particles.
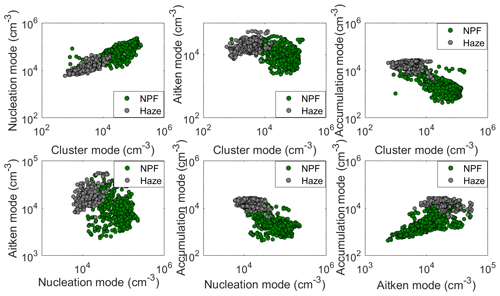
Figure 12Correlation between different particle modes on the NPF event days (green dots) and the haze days (gray dots). The time resolution of data in the plots of correlation between cluster mode and other modes was 12 min, and the time resolution of other data points was 5 min.
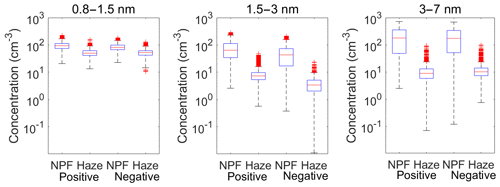
Figure 13Positive and negative ion number concentrations in the size bins of 0.8–1.5, 1.5–3 and 3–7 nm on the NPF event days and the haze days separately. The whiskers include 99.3 % of data of every group. Data out of 1.5 times the interquartile range are located outside of the whiskers and considered outliers. The lines in the boxes represent the median value, the lower part of the boxes represents 25 % of the number concentration, and the upper part of the boxes represents 75 % of the number concentration. Data marked with red plus symbols represent outliers.
On the NPF event days, Aitken and accumulation mode particle number concentrations correlated positively with each other, as well as with the SO2 and NOx concentration. This suggests that on the NPF event days, Aitken and accumulation mode particles both formed during regional transportation as secondary particles and were emitted by traffic as primary particles.
On the haze days, cluster and nucleation mode particle number concentrations correlated positively with each other and with the Aitken mode particle number concentration. This is suggestive of similar dominating sources for these particles, most likely traffic emissions. Similar to the NPF event days, cluster and nucleation mode particle number concentrations correlated negatively with the accumulation mode particle number concentration, even though this correlation was rather weak (Table 2b). As expected based on the discussion in Sect. 3.3.5, the Aitken mode particle number concentration had a negative correlation with the accumulation mode particle number concentration on the haze days.
3.5 Atmospheric ions and ion-induced nucleation in Beijing
In order to estimate the contribution of ions to the total cluster mode particle number concentration and the importance of ion-induced nucleation in Beijing, we studied ion number concentrations in the size range of 0.8–7 nm by dividing them into three subsize bins: constant pool (0.8–1.5 nm), charged clusters (1.5–3 nm) and larger ions (3–7 nm). As shown in Fig. 13, number concentrations of positive ions were higher than those negative ions in all the size bins on both NPF event days and haze days. We will only discuss positive ions here.
The median number concentration of positive ions in the constant pool on NPF event days was only 100 cm−3 in Beijing, much less than that in the boreal forest (600 cm−3; Mazon et al., 2016). Also, the median number concentration of positive charged clusters was 20 cm−3 on the NPF event days, and the ratio to the total cluster mode particle number concentration was 0.001 to 0.004 during the NPF time window (Fig. 14). This ratio is comparable to that observed in San Pietro Capofiume (0.004), in which the anthropogenic pollution level was also high but clearly lower than that observed in another megacity in China, Nanjing (0.02; Kontkanen et al., 2017). Considerably higher ratios were observed in clean environments, for example during winter in the boreal forest at Hyytiälä, Finland (0.7; Kontkanen et al., 2017). The median number concentration of larger ions (3–7 nm) on the NPF event days was 30 cm−3, a little bit higher than the charged cluster mode particle number concentration, indicating that not all of the larger ions originate from the growth of charged clusters, but rather from charging of neutral particles by smaller ions. On the haze days, charged ion number concentrations were much lower than those on the NPF days, which could be attributed to the higher condensation sink.
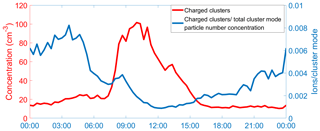
Figure 14Diurnal pattern of charged clusters (1.5–3 nm) number concentration (red line) and ratio of charged clusters to total cluster mode (1.5–3 nm) particle number concentration on the NPF event days (blue line). The time resolution of the used data was 12 min.
The diurnal pattern of the ratio of number concentration between charged and total cluster mode particles was the highest during the night with a maximum of 0.008, and it had a trough during daytime with a minimum of 0.001 on the NPF event days. Such a diurnal pattern is similar to earlier observations in Nanjing, San Pietro Capofiume and Hyytiälä (Kontkanen et al., 2017). This ratio reached its minimum around noon, because the total cluster mode particle number concentration reached its maximum around that time due to NPF. The ratio had a small peak at around 09:00 LT, similar to earlier observations in Centreville and Po Valley (Kontkanen et al., 2016, 2017). The possible reason is that charged clusters were activated earlier in the morning than neutral clusters. The ratio increased from the midnight until about 04:00 LT, similar to the number concentration of charged clusters.
As shown in Fig. 15, the diurnal median of the ratio between the formation rate of positive ions of 1.5 nm () and the total formation rate clusters of 1.5 nm (J1.5) varied from 0.0009 to 0.006. This result is comparable to observations in Shanghai, where the positive ion-induced nucleation contributed only 0.05 % to the total formation rate of 1.7 nm particles (J1.7) (Yao et al., 2018).
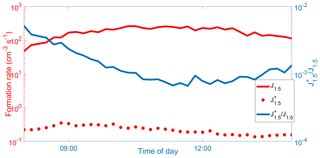
Figure 15Diurnal pattern of formation rate of positive charged clusters of 1.5 nm (red dots) and neutral clusters of 1.5 nm (red line) and the ratio between them (blue line) on the NPF event days during the NPF time window we chose. The time resolution of the used data was 12 min.
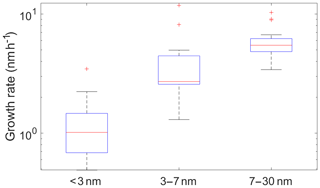
Figure 16Growth rates of cluster mode and nucleation mode particles generated from NPF events. The lines in the boxes represent the median value, the lower part of the boxes represents 25 % of the growth rates and the upper part of the boxes represents 75 % of the growth rates. Data marked with red plus symbols represent outliers.
3.6 Particle growth rates
The growth rates of particles generated from NPF events were examined in three size ranges: < 3, 3–7 and 7–25 nm (Fig. 16). The median growth rates of particles in these size ranges were 1.0, 2.7 and 5.5 nm h−1, respectively. The growth rate of cluster mode particles was comparable with that observed in Shanghai (1.5 n h−1; Yao et al., 2018). The notable increase in the particle growth rate with an increasing particle size is a very typical feature in the sub-20 nm size range (Kerminen et al., 2018), and it may also extend to larger particle sizes (Paasonen et al., 2018).
Our observations are in line with the reported range of nucleation mode particle growth rates of 0.1–11.2 nm h−1 in urban areas of Beijing (Wang et al., 2017b; Jayaratne et al., 2017). Such growth rates can explain the observed increases of Aitken mode particle number concentrations in the afternoon.
We measured particle number concentrations over a wide range of particle diameters (1.5–1000 nm) on both NPF event days and haze days in wintertime Beijing. To our knowledge, this was the first time when cluster mode particle number concentrations have been reported on haze days in Beijing.
The observed responses of particle number concentrations in different modes (cluster, nucleation, Aitken and accumulation mode) to changes in trace-gas and PM2.5 concentrations were quite heterogeneous, suggesting different sources and dynamics experienced by each mode. NPF was the dominant source of cluster and nucleation mode particles. Ion-induced nucleation did not play an important role during the NPF events. The growth rates of cluster and nucleation mode particles increased with an increasing particle size. Traffic emissions contributed to every mode and were the dominant source of cluster and nucleation mode particles on the haze days. The main sources of Aitken mode particles were local emissions, while transported and regional pollution as well as growth from the nucleation mode also contributed to the Aitken mode. The main source of accumulation mode particles was regional and transported pollution. PM2.5 affected the number concentration of sub-100 nm particles by competing for vapors responsible for particle growth and by acting as a sink for particles by coagulation. The main contributors to the PM2.5 mass concentration were accumulation mode particles on the haze days.
As demonstrated here and in many other studies (e.g., Brines et al., 2015), ultrafine particles (< 100 nm in diameter) tend to dominate the total aerosol particle number concentration in megacities like Beijing. More attention should therefore be put on ultrafine particles in urban environments. We found that both NPF and traffic emissions are important sources of ultrafine particles in Beijing. To improve our understanding of the potential effects of ultrafine particles on health and air quality, we need to do more research on their sources and physical and chemical properties. Laboratory and model analysis on dynamics of ultrafine particles would help us to understand the evolution of particle number size distributions. In addition, to identify and locate other possible sources, long-term observations on ultrafine particles down to the cluster mode as well as source apportionment analyses, such as cluster analysis and receptor model studies, are still needed. Ultrafine particles should also be taken into consideration when making policies to control air pollution. New regulations should be designed to control primary emission sources, such as traffic, or precursor emissions for secondary ultrafine particles involving NPF and subsequent particle growth.
Particle number concentrations are available by contacting Ying Zhou (yingzhouahl@163.com) or Lubna Dada (lubna.dada@helsinki.fi).
The supplement related to this article is available online at: https://doi.org/10.5194/acp-20-1201-2020-supplement.
YZ, YiL, YF and JuK contributed to data collection. YZ, TC and LD contributed to data inversion. YZ and LD contributed to analyzing the data. CY, BC, KRD, FB, TK, YoL and JoK contributed to maintaining the station. YZ, LD, JuK and VMK wrote the paper. TP, LW, JJ and MK provided helpful scientific discussions. All co-authors reviewed the article.
The authors declare that they have no conflict of interest.
This study received funding from Beijing University of Chemical Technology. This research has received funding from the National Natural Science Foundation of China (41877306). Kaspar R. Daellenbach acknowledges support by the Swiss National Science postdoc mobility grant P2EZP2_181599. Lin Wang acknowledges support by the National Key R&D Program of China (2017YFC0209505) and the National Natural Science Foundation of China.
This work has been supported by the European Commission (project ACTRIS2), European Research Council (ATM-GTP, grant no. 742206), the Academy of Finland Centre of Excellence in Atmospheric Sciences (grant no. 272041), and the Ministry of Science and Technology of the People's Republic of China (grant no. 2019YFC0214701).
This paper was edited by Astrid Kiendler-Scharr and reviewed by two anonymous referees.
Baklanov, A., Molina, L. T., and Gauss, M.: Megacities, air quality and climate, Atmos. Environ., 126, 235–249, https://doi.org/10.1016/j.atmosenv.2015.11.059, 2016.
Beevers, S. D., Westmoreland, E., de Jong, M. C., Williams, M. L., and Carslaw, D. C.: Trends in NOx and NO2 emissions from road traffic in Great Britain, Atmos. Environ., 54, 107–116, https://doi.org/10.1016/j.atmosenv.2012.02.028, 2012.
Brines, M., Dall'Osto, M., Beddows, D. C. S., Harrison, R. M., Gómez-Moreno, F., Núñez, L., Artíñano, B., Costabile, F., Gobbi, G. P., Salimi, F., Morawska, L., Sioutas, C., and Querol, X.: Traffic and nucleation events as main sources of ultrafine particles in high-insolation developed world cities, Atmos. Chem. Phys., 15, 5929–5945, https://doi.org/10.5194/acp-15-5929-2015, 2015.
Cai, R., Yang, D., Fu, Y., Wang, X., Li, X., Ma, Y., Hao, J., Zheng, J., and Jiang, J.: Aerosol surface area concentration: a governing factor in new particle formation in Beijing, Atmos. Chem. Phys., 17, 12327–12340, https://doi.org/10.5194/acp-17-12327-2017, 2017.
Cai, R., Yang, D., Ahonen, L. R., Shi, L., Korhonen, F., Ma, Y., Hao, J., Petäjä, T., Zheng, J., Kangasluoma, J., and Jiang, J.: Data inversion methods to determine sub-3 nm aerosol size distributions using the particle size magnifier, Atmos. Meas. Tech., 11, 4477–4491, https://doi.org/10.5194/amt-11-4477-2018, 2018.
Cao, C., Jiang, W. J., Wang, B. Y., Fang, J. H., Lang, J. D., Tian, G., Jiang, J. K., and Zhu, T. F.: Inhalable Microorganisms in Beijing's PM2.5 and PM10 Pollutants during a Severe Smog Event, Environ. Sci. Technol., 48, 1499–1507, https://doi.org/10.1021/es4048472, 2014.
Chan, T., Cai, R., Ahonen, L. R., Liu, Y., Zhou, Y., Vanhanen, J., Dada, L., Chao, Y., Liu, Y., Wang, L., Kulmala, M., and Kangasluoma, J.: Assessment of particle size magnifier inversion methods to obtain particle size distribution from atmospheric measurements, Atmos. Meas. Tech. Discuss., https://doi.org/10.5194/amt-2019-465, in review, 2020.
Chu, B. W., Kerminen, V. M., Bianchi, F., Yan, C., Petäjä, T., and Kulmala, M.: Atmospheric new particle formation in China, Atmos. Chem. Phys., 19, 115–138, https://doi.org/10.5194/acp-19-115-2019, 2019.
Dada, L., Paasonen, P., Nieminen, T., Mazon, S. B., Kontkanen, J., Peräkylä, O., Lehtipalo, K., Hussein, T., Petäjä, T., Kerminen, V. M., Bäck, J., and Kulmala, M.: Long-term analysis of clear-sky new particle formation events and nonevents in Hyytiälä, Atmos. Chem. Phys., 17, 6227–6241, https://doi.org/10.5194/acp-17-6227-2017, 2017.
Dada, L., Chellapermal, R., Buenrostro Mazon, S., Paasonen, P., Lampilahti, J., Manninen, H. E., Junninen, H., Petäjä, T., Kerminen, V.-M., and Kulmala, M.: Refined classification and characterization of atmospheric new-particle formation events using air ions, Atmos. Chem. Phys., 18, 17883–17893, https://doi.org/10.5194/acp-18-17883-2018, 2018.
Dai, L., Wang, H. L., Zhou, L. Y., An, J. L., Tang, L. L., Lu, C. S., Yan, W. L., Liu, R. Y., Kong, S. F., Chen, M. D., Lee, S. H., and Yu, H.: Regional and local new particle formation events observed in the Yangtze River Delta region, China, J. Geophys. Res.-Atmos., 122, 2389–2402, https://doi.org/10.1002/2016JD026030, 2017.
Dal Maso, M., Kulmala, M., Riipinen, I., Wagner, R., Hussein, T., Aalto, P. P., and Lehtinen, K. E. J.: Formation and growth of fresh atmospheric aerosols: eight years of aerosol size distribution data from SMEAR II, Hyytiala, Finland, Boreal Environ. Res., 10, 323–336, 2005.
de Jesus, A. L., Rahman, M. M., Mazaheri, M., Thompson, H., Knibbs, L. D., Jeong, C., Evans, G., Nei, W., Ding, A., Qiao, L., Li, L., Portin, H., Niemi, J. V., Timonen, H., Luoma, K., Petäjä, T., Kulmala, M., Kowalski, M., Peters, A., Cyrys, J., Ferrero, L., Manigrasso, M., Avino, P., Buonano, G., Reche, C., Querol, X., Beddows, D., Harrison, R. M., Sowlat, M. H., Sioutas, C., and Morawska, L.: Ultrafine particles and PM2.5 in the air of cities around the world: Are they representative of each other?, Environ. Int., 129, 118–135, https://doi.org/10.1016/j.envint.2019.05.021, 2019.
Du, W., Zhao, J., Wang, Y., Zhang, Y., Wang, Q., Xu, W., Chen, C., Han, T., Zhang, F., Li, Z., Fu, P., Li, J., Wang, Z., and Sun, Y.: Simultaneous measurements of particle number size distributions at ground level and 260 m on a meteorological tower in urban Beijing, China, Atmos. Chem. Phys., 17, 6797–6811, https://doi.org/10.5194/acp-17-6797-2017, 2017.
Feng, X., Dang, Z., Huang, W., Shao, L., and Li, W.: Microscopic morphology and size distribution of particles in PM2.5 of Guangzhou City, J. Atmos. Chem., 64, 37–51, https://doi.org/10.1007/s10874-010-9169-7, 2010.
Han, S. Q., Bian, H., Feng, Y. C., Liu, A. X., Li, X. J., Zeng, F., and Zhang, X. L.: Analysis of the Relationship between O3, NO and NO2 in Tianjin, China, Aerosol Air Qual. Res., 11, 128–139, https://doi.org/10.4209/aaqr.2010.07.0055, 2011.
Hao, J. M. and Wang, L. T.: Improving urban air quality in China: Beijing case study, J. Air Waste Manage., 55, 1298–1305, https://doi.org/10.1080/10473289.2005.10464726, 2005.
Hari, P. and Kulmala, M.: Station for measuring ecosystem-atmosphere relations (SMEAR II), Boreal Environ. Res., 10, 315–322, 2005.
IPCC: Summary for policymakers, Climate change 2007, 93–129, 2007.
Jayaratne, R., Pushpawela, B., He, C., Li, H., Gao, J., Chai, F., and Morawska, L.: Observations of particles at their formation sizes in Beijing, China, Atmos. Chem. Phys., 17, 8825–8835, https://doi.org/10.5194/acp-17-8825-2017, 2017.
Jiang, J. K., Zhao, J., Chen, M. D., Eisele, F. L., Scheckman, J., Williams, B. J., Kuang, C. A., and McMurry, P. H.: First Measurements of Neutral Atmospheric Cluster and 1–2 nm Particle Number Size Distributions During Nucleation Events, Aerosol Sci. Technol., 45, ii–v, https://dio.org/10.1080/02786826.2010.546817, 2011.
Kangasluoma, J., Franchin, A., Duplissy, J., Ahonen, L., Korhonen, F., Attoui, M., Mikkilä, J., Lehtipalo, K., Vanhanen, J., Kulmala, M., and Petäjä, T.: Operation of the Airmodus A11 nano Condensation Nucleus Counter at various inlet pressures and various operation temperatures, and design of a new inlet system, Atmos. Meas. Tech., 9, 2977–2988, https://doi.org/10.5194/amt-9-2977-2016, 2016.
Kerminen, V. M., Pirjola, L., and Kulmala, M.: How significantly does coagulational scavenging limit atmospheric particle production?, J. Geophys. Res.-Atmos., 106, 24119–24125, https://doi.org/10.1029/2001jd000322, 2001.
Kerminen, V. M., Paramonov, M., Anttila, T., Riipinen, I., Fountoukis, C., Korhonen, H., Asmi, E., Laakso, L., Lihavainen, H., Swietlicki, E., Svenningsson, B., Asmi, A., Pandis, S. N., Kulmala, M., and Petäjä, T.: Cloud condensation nuclei production associated with atmospheric nucleation: a synthesis based on existing literature and new results, Atmos. Chem. Phys., 12, 12037–12059, https://doi.org/10.5194/acp-12-12037-2012, 2012.
Kerminen, V. M., Chen, X. M., Vakkari, V., Petäjä, T., Kulmala, M., and Bianchi, F.: Atmospheric new particle formation and growth: review of field observations, Environ. Res. Lett., 13, 103003, https://doi.org/10.1088/1748-9326/aadf3c, 2018.
Kontkanen, J., Järvinen, E., Manninen, H. E., Lehtipalo, K., Kangasluoma, J., Decesari, S., Gobbi, G. P., Laaksonen, A., Petäjä, T., and Kulmala, M.: High concentrations of sub-3 nm clusters and frequent new particle formation observed in the Po Valley, Italy, during the PEGASOS 2012 campaign, Atmos. Chem. Phys., 16, 1919–1935, https://doi.org/10.5194/acp-16-1919-2016, 2016.
Kontkanen, J., Lehtipalo, K., Ahonen, L., Kangasluoma, J., Manninen, H. E., Hakala, J., Rose, C., Sellegri, K., Xiao, S., Wang, L., Qi, X., Nie, W., Ding, A., Yu, H., Lee, S., Kerminen, V.-M., Petäjä, T., and Kulmala, M.: Measurements of sub-3? nm particles using a particle size magnifier in different environments: from clean mountain top to polluted megacities, Atmos. Chem. Phys., 17, 2163–2187, https://doi.org/10.5194/acp-17-2163-2017, 2017.
Koponen, I. K., Asmi, A., Keronen, P., Puhto, K., and Kulmala, M.: Indoor air measurement campaign in Helsinki, Finland 1999 – the effect of outdoor air pollution on indoor air, Atmos. Environ., 35, 1465–1477, https://doi.org/10.1016/s1352-2310(00)00338-1, 2001.
Kreyling, W. G., Semmler, M., and Möller, W.: Dosimetry and toxicology of ultrafine particles, J. Aerosol Med., 17, 140–152, https://doi.org/10.1089/0894268041457147, 2004.
Kulmala, M.: How particles nucleate and grow, Science, 302, 1000–1001, https://doi.org/10.1126/science.1090848, 2003.
Kulmala, M.: Atmospheric chemistry: China's choking cocktail, Nature, 526, 497–499, https://doi.org/10.1038/526497a, 2015.
Kulmala, M., Vehkamäki, H., Petäjä, T., Dal Maso, M., Lauri, A., Kerminen, V. M., Birmili, W., and McMurry, P. H.: Formation and growth rates of ultrafine atmospheric particles: a review of observations, J. Aerosol Sci., 35, 143–176, https://doi.org/10.1016/j.jaerosci.2003.10.003, 2004.
Kulmala, M., Riipinen, I., Sipilä, M., Manninen, H. E., Petäjä, T., Junninen, H., Dal Maso, M., Mordas, G., Mirme, A., Vana, M., Hirsikko, A., Laakso, L., Harrison, R. M., Hanson, I., Leung, C., Lehtinen, K. E. J., and Kerminen, V. M.: Toward direct measurement of atmospheric nucleation, Science, 318, 89–92, https://doi.org/10.1126/science.1144124, 2007.
Kulmala, M., Petäjä, T., Nieminen, T., Sipilä, M., Manninen, H. E., Lehtipalo, K., Dal Maso, M., Aalto, P. P., Junninen, H., Paasonen, P., Riipinen, I., Lehtinen, K. E. J., Laaksonen, A., and Kerminen, V. M.: Measurement of the nucleation of atmospheric aerosol particles, Nat. Protoc., 7, 1651–1667, https://doi.org/10.1038/nprot.2012.091, 2012.
Kulmala, M., Kontkanen, J., Junninen, H., Lehtipalo, K., Manninen, H. E., Nieminen, T., Petäjä, T., Sipilä, M., Schobesberger, S., Rantala, P., Franchin, A., Jokinen, T., Järvinen, E., Äijälä, M., Kangasluoma, J., Hakala, J., Aalto, P. P., Paasonen, P., Mikkilä, J., Vanhanen, J., Aalto, J., Hakola, H., Makkonen, U., Ruuskanen, T., Mauldin, R. L., Duplissy, J., Vehkamäki, H., Bäck, J., Kortelainen, A., Riipinen, I., Kurtén, T., Johnston, M. V., Smith, J. N., Ehn, M., Mentel, T. F., Lehtinen, K. E. J., Laaksonen, A., Kerminen, V. M., and Worsnop, D. R.: Direct Observations of Atmospheric Aerosol Nucleation, Science, 339, 943–946, https://doi.org/10.1126/science.1227385, 2013.
Kulmala, M., Petaja, T., Ehn, M., Thornton, J., Sipila, M., Worsnop, D. R., and Kerminen, V. M.: Chemistry of Atmospheric Nucleation: On the Recent Advances on Precursor Characterization and Atmospheric Cluster Composition in Connection with Atmospheric New Particle Formation, Annu. Rev. Phys. Chem., 65, 21–37, 2014.
Kulmala, M., Kerminen, V. M., Petäjä, T., Ding, A. J., and Wang, L.: Atmospheric gas-to-particle conversion: why NPF events are observed in megacities?, Faraday Discuss., 200, 271–288, https://doi.org/10.1039/C6FD00257A, 2017.
Lehtipalo, K., Leppä, J., Kontkanen, J., Kangasluoma, J., Wimmer, D., Franchin, A., Schobesberger, S., Junninen, H., Petäjä, T., Sipilä, M., Mikkilä, J., Vanhanen, J., Worsnop, D. R., and Kulmala, M.: methods for determining particle size distribution and growth rates between 1 and 3 nm using the Particle Size Magnifier, Boreal Environ. Res., 19, 215–236, 2014.
Lelieveld, J., Evans, J. S., Fnais, M., Giannadaki, D., and Pozzer, A.: The contribution of outdoor air pollution sources to premature mortality on a global scale, Nature, 525, 367–371, https://doi.org/10.1038/nature15371, 2015.
Liu, X. G., Li, J., Qu, Y., Han, T., Hou, L., Gu, J., Chen, C., Yang, Y., Liu, X., Yang, T., Zhang, Y., Tian, H., and Hu, M.: Formation and evolution mechanism of regional haze: a case study in the megacity Beijing, China, Atmos. Chem. Phys., 13, 4501–4514, https://doi.org/10.5194/acp-13-4501-2013, 2013.
Liu, J. Q., Jiang, J. K., Zhang, Q., Deng, J. G., and Hao, J. M.: A spectrometer for measuring particle size distributions in the range of 3 nm to 10 µm, Front. Env. Sci. Eng., 10, 63–72, https://doi.org/10.1007/s11783-014-0754-x,2016.
Lowry, D., Lanoiselle, M. E., Fisher, R. E., Martin, M., Fowler, C. M. R., France, J. L., Hernandez-Paniagua, I. Y., Novelli, P. C., Sriskantharajah, S., O'Brien, P., Rata, N. D., Holmes, C. W., Fleming, Z. L., Clemitshaw, K. C., Zazzeri, G., Pommier, M., McLinden, C. A., and Nisbet, E. G.: Marked long-term decline in ambient CO mixing ratio in SE England, 1997–2014: evidence of policy success in improving air quality, Sci. Rep.-UK, 6, 25661, https://doi.org/10.1038/srep25661, 2016.
Lu, Y., Yan, C., Fu, Y., Chen, Y., Liu, Y., Yang, G., Wang, Y., Bianchi, F., Chu, B., Zhou, Y., Yin, R., Baalbaki, R., Garmash, O., Deng, C., Wang, W., Liu, Y., Petäjä, T., Kerminen, V.-M., Jiang, J., Kulmala, M., and Wang, L.: A proxy for atmospheric daytime gaseous sulfuric acid concentration in urban Beijing, Atmos. Chem. Phys., 19, 1971–1983, https://doi.org/10.5194/acp-19-1971-2019, 2019.
Lu, Z., Streets, D. G., Zhang, Q., Wang, S., Carmichael, G. R., Cheng, Y. F., Wei, C., Chin, M., Diehl, T., and Tan, Q.: Sulfur dioxide emissions in China and sulfur trends in East Asia since 2000, Atmos. Chem. Phys., 10, 6311–6331, https://doi.org/10.5194/acp-10-6311-2010, 2010.
Manninen, H. E., Mirme, S., Mirme, A., Petäjä, T., and Kulmala, M.: How to reliably detect molecular clusters and nucleation mode particles with Neutral cluster and Air Ion Spectrometer (NAIS), Atmos. Meas. Tech., 9, 3577–3605, https://doi.org/10.5194/amt-9-3577-2016, 2016.
Mazon, S. B., Kontkanen, J., Manninen, H. E., Nieminen, T., Kerminen, V.-M., and Kulmala, M.: A long-term comparison of nighttime cluster events and daytime ion formation in a boreal forest, Boeral Environ. Res., 21, 242–261, 2016.
Mirme, A., Tamm, E., Mordas, G., Vana, M., Uin, J., Mirme, S., Bernotas, T., Laakso, L., Hirsikko, A., and Kulmala, M.: A wide-range multi-channel air ion spectrometer, Boreal Environ. Res., 12, 247–264, 2007.
Mirme, S. and Mirme, A.: The mathematical principles and design of the NAIS – a spectrometer for the measurement of cluster ion and nanometer aerosol size distributions, Atmos. Meas. Tech., 6, 1061–1071, https://doi.org/10.5194/amt-6-1061-2013, 2013.
Oberdörster, G., Sharp, Z., Atudorei, V., Elder, A., Gelein, R., Kreyling, W., and Cox, C.: Translocation of inhaled ultrafine particles to the brain, Inhal. Toxicol., 16, 437–445, https://doi.org/10.1080/08958370490439597, 2004.
Paasonen, P., Peltola, M., Kontkanen, J., Junninen, H., Kerminen, V.-M., and Kulmala, M.: Comprehensive analysis of particle growth rates from nucleation mode to cloud condensation nuclei in boreal forest, Atmos. Chem. Phys., 18, 12085–12103, https://doi.org/10.5194/acp-18-12085-2018, 2018.
Pétron, G., Granier, C., Khattatov, B., Yudin, V., Lamarque, J. F., Emmons, L., Gille, J., and Edwards, D. P.: Monthly CO surface sources inventory based on the 2000–2001 MOPITT satellite data, Geophys. Res. Lett., 31, L21107, https://doi.org/10.1029/2004gl020560, 2004.
Pirjola, L., Lähde, T., Niemi, J. V., Kousa, A., Rönkkö, T., Karjalainen, P., Keskinen, J., Frey, A., and Hillamo, R.: Spatial and temporal characterization of traffic emissions in urban microenvironments with a mobile laboratory, Atmos. Environ., 63, 156–167, https://doi.org/10.1016/j.atmosenv.2012.09.022, 2012.
Qi, X. M., Ding, A. J., Nie, W., Petäjä, T., Kerminen, V.-M., Herrmann, E., Xie, Y. N., Zheng, L. F., Manninen, H., Aalto, P., Sun, J. N., Xu, Z. N., Chi, X. G., Huang, X., Boy, M., Virkkula, A., Yang, X.-Q., Fu, C. B., and Kulmala, M.: Aerosol size distribution and new particle formation in the western Yangtze River Delta of China: 2 years of measurements at the SORPES station, Atmos. Chem. Phys., 15, 12445–12464, https://doi.org/10.5194/acp-15-12445-2015, 2015.
Ravishankara, A. R.: Heterogeneous and Multiphase Chemistry in the Troposphere, Science, 276, 1058–1065, https://doi.org/10.1126/science.276.5315.1058,1997.
Roberts, D. L. and Jones, A.: Climate sensitivity to black carbon aerosol from fossil fuel combustion, J. Geophys. Res., 109, D16202, https://doi.org/10.1029/2004jd004676, 2004.
Rönkkö, T., Kuuluvainen, H., Karjalainen, P., Keskinen, J., Hillamo, R., Niemi, J. V., Pirjola, L., Timonen, H. J., Saarikoski, S., Saukko, E., Järvinen, A., Silvennoinen, H., Rostedt, A., Olin, M., Yli-Ojanperä, J., Nousiainene, P., Kousa, A., and Dal Maso, M.: Traffic is a major source of atmospheric nanocluster aerosol, P. Natl. Acad. Sci. USA, 114, 7549–7554, https://doi.org/10.1073/pnas.1700830114, 2017.
Shi, J. P. and Harrison, R. M.: Investigation of Ultrafine Particle Formation during Diesel Exhaust Dilution, Environ. Sci. Technol., 33, 3730–3736, 1999.
Shi, J. P., Evans, D. E., Khan, A. A., and Harrison, R. M.: Sources and concentration of nanoparticles (< 10 nm diameter) in the urban atmosphere, Atmos. Environ., 35, 1193–1202, 2001.
Solomos, S., Kallos, G., Kushta, J., Astitha, M., Tremback, C., Nenes, A., and Levin, Z.: An integrated modeling study on the effects of mineral dust and sea salt particles on clouds and precipitation, Atmos. Chem. Phys., 11, 873–892, https://doi.org/10.5194/acp-11-873-2011, 2011.
Tian, X., Xie, P. H., Xu, J., Li, A., Wang, Y., Qin, M., and Hu, Z. K.: Long-term observations of tropospheric NO2, SO2 and HCHO by MAX-DOAS in Yangtze River Delta area, China, J. Environ. Sci.-China, 71, 207–221, https://doi.org/10.1016/j.jes.2018.03.006, 2018.
Vahlsing, C. and Smith, K. R.: Global review of national ambient air quality standards for PM10 and SO2 (24 h), Air Qual. Atmos. Hlth., 5, 393–399, https://doi.org/10.1007/s11869-010-0131-2, 2012.
Vanhanen, J., Mikkilä, J., Lehtipalo, K., Sipilä, M., Manninen, H. E., Siivola, E., Petäjä, T., and Kulmala, M.: Particle Size Magnifier for Nano-CN Detection, Aerosol Sci. Technol., 45, 533–542, https://doi.org/10.1080/02786826.2010.547889, 2011.
von Bismarck-Osten, C., Birmili, W., Ketzel, M., Massling, A., Petäjä, T., and Weber, S.: Characterization of parameters influencing the spatio-temporal variability of urban particle number size distributions in four European cities, Atmos. Environ., 77, 415–429, https://doi.org/10.1016/j.atmosenv.2013.05.029, 2013.
Vu, T. V., Delgado-Saborit, J. M., and Harrison, R. M.: Review: Particle number size distributions from seven major sources and implications for source apportionment studies, Atmos. Environ., 122, 114–132, https://doi.org/10.1016/j.atmosenv.2015.09.027, 2015.
Wang, D. W., Guo, H., Cheung, K., and Gan, F. X.: Observation of nucleation mode particle burst and new particle formation events at an urban site in Hong Kong, Atmos. Environ., 99, 196–205, https://doi.org/10.1016/j.atmosenv.2014.09.074, 2014a.
Wang, T., Xue, L. K., Brimblecombe, P., Lam, Y. F., Li, L., and Zhang, L.: Ozone pollution in China: A review of concentrations, meteorological influences, chemical precursors, and effects, Sci. Total Environ., 575, 1582–1596, https://doi.org/10.1016/j.scitotenv.2016.10.081, 2017.
Wang, Y. S., Yao, L., Wang, L. L., Liu, Z. R., Ji, D. S., Tang, G. Q., Zhang, J. K., Sun, Y., Hu, B., and Xin, J. Y.: Mechanism for the formation of the January 2013 heavy haze pollution episode over central and eastern China, Sci. China Earth Sci., 57, 14–25, https://doi.org/10.1007/s11430-013-4773-4, 2014b.
Wang, Z. B., Hu, M., Wu, Z. J., Yue, D. L., He, L. Y., Huang, X. F., Liu, X. G., and Wiedensohler, A.: Long-term measurements of particle number size distributions and the relationships with air mass history and source apportionment in the summer of Beijing, Atmos. Chem. Phys., 13, 10159–10170, https://doi.org/10.5194/acp-13-10159-2013, 2013.
Wehner, B., Wiedensohler, A., Tuch, T. M., Wu, Z. J., Hu, M., Slanina, J., and Kiang, C. S.: Variability of the aerosol number size distribution in Beijing, China: New particle formation, dust storms, and high continental background, Geophys. Res. Lett., 31, L22108, https://doi.org/10.1029/2004GL021596, 2004.
WHO: Air quality guidelines for Europe, 2nd Edn., Copenhagen, World Health Organization Regional Office for Europe, WHO Regional Publications, European Series No. 91, 2000.
Wu, Z. J., Hu, M., Liu, S., Wehner, B., Bauer, S., Ssling, A. M., Wiedensohler, A., Petaja, T., Dal Maso, M., and Kulmala, M.: New particle formation in Beijing, China: Statistical analysis of a 1-year data set, J. Geophys. Res.-Atmos., 112, D09209, https://doi.org/10.1029/2006JD007406, 2007.
Wu, Z. J., Hu, M., Lin, P., Liu, S., Wehner, B., and Wiedensohler, A.: Particle number size distribution in the urban atmosphere of Beijing, China, Atmos. Environ., 42, 7967–7980, https://doi.org/10.1016/j.atmosenv.2008.06.022, 2008.
Xiao, S., Wang, M. Y., Yao, L., Kulmala, M., Zhou, B., Yang, X., Chen, J. M., Wang, D. F., Fu, Q. Y., Worsnop, D. R., and Wang, L.: Strong atmospheric new particle formation in winter in urban Shanghai, China, Atmos. Chem. Phys., 15, 1769–1781, https://doi.org/10.5194/acp-15-1769-2015, 2015.
Yang, M., Ma, T. M., and Sun, C. W.: Evaluating the impact of urban traffic investment on SO2 emissions in China cities, Energ. Policy, 113, 20–27, https://doi.org/10.1016/j.enpol.2017.10.039, 2018.
Yao, L., Garmash, O., Bianchi, F., Zheng, J., Yan, C., Kontkanen, J., Junninen, H., Mazon, S. B., Ehn, M., Paasonen, P., Sipilä, M., Wang, M. Y., Wang, X. K., Xiao, S., Chen, H. F., Lu, Y. Q., Zhang, B. W., Wang, D. F., Fu, Q. Y., Geng, F. H., Li, L., Wang, H. L., Qiao, L. P., Yang, X., Chen, J. M., Kerminen, V. M., Petäjä, T., Worsnop, D. R., Kulmala, M., and Wang, L.: Atmospheric new particle formation from sulfuric acid and amines in a Chinese megacity, Science, 361, 278–281, https://doi.org/10.1126/science.aao4839, 2018.
Yu, H., Zhou, L. Y., Dai, L., Shen, W. C., Dai, W., Zheng, J., Ma, Y., and Chen, M. D.: Nucleation and growth of sub-3 nm particles in the polluted urban atmosphere of a megacity in China, Atmos. Chem. Phys., 16, 2641–2657, https://doi.org/10.5194/acp-16-2641-2016, 2016.
Yue, D. L., Hu, M., Wu, Z. J., Guo, S., Wen, M. T., Nowak, A., Wehner, B., Wiedensohler, A., Takegawa, N., Kondo, Y., Wang, X. S., Li, Y. P., Zeng, L. M., and Zhang, Y. H.: Variation of particle number size distributions and chemical compositions at the urban and downwind regional sites in the Pearl River Delta during summertime pollution episodes, Atmos. Chem. Phys., 10, 9431–9439, https://doi.org/10.5194/acp-10-9431-2010, 2010.
Zheng, G. J., Duan, F. K., Su, H., Ma, Y. L., Cheng, Y., Zheng, B., Zhang, Q., Huang, T., Kimoto, T., Chang, D., Pöschl, U., Cheng, Y. F., and He, K. B.: Exploring the severe winter haze in Beijing: the impact of synoptic weather, regional transport and heterogeneous reactions, Atmos. Chem. Phys., 15, 2969–2983, https://doi.org/10.5194/acp-15-2969-2015, 2015.