the Creative Commons Attribution 4.0 License.
the Creative Commons Attribution 4.0 License.
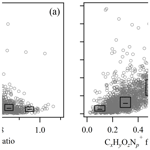
Contributions of biomass-burning, urban, and biogenic emissions to the concentrations and light-absorbing properties of particulate matter in central Amazonia during the dry season
Suzane S. de Sá
Luciana V. Rizzo
Brett B. Palm
Pedro Campuzano-Jost
Douglas A. Day
Lindsay D. Yee
Rebecca Wernis
Gabriel Isaacman-VanWertz
Joel Brito
Samara Carbone
Yingjun J. Liu
Arthur Sedlacek
Stephen Springston
Allen H. Goldstein
Henrique M. J. Barbosa
M. Lizabeth Alexander
Paulo Artaxo
Jose L. Jimenez
Scot T. Martin
Urbanization and deforestation have important impacts on atmospheric particulate matter (PM) over Amazonia. This study presents observations and analysis of PM1 concentration, composition, and optical properties in central Amazonia during the dry season, focusing on the anthropogenic impacts. The primary study site was located 70 km downwind of Manaus, a city of over 2 million people in Brazil, as part of the GoAmazon2014/5 experiment. A high-resolution time-of-flight aerosol mass spectrometer (AMS) provided data on PM1 composition, and aethalometer measurements were used to derive the absorption coefficient babs,BrC of brown carbon (BrC) at 370 nm. Non-refractory PM1 mass concentrations averaged 12.2 µg m−3 at the primary study site, dominated by organics (83 %), followed by sulfate (11 %). A decrease in babs,BrC was observed as the mass concentration of nitrogen-containing organic compounds decreased and the organic PM1 O:C ratio increased, suggesting atmospheric bleaching of the BrC components. The organic PM1 was separated into six different classes by positive-matrix factorization (PMF), and the mass absorption efficiency Eabs associated with each factor was estimated through multivariate linear regression of babs,BrC on the factor loadings. The largest Eabs values were associated with urban (2.04±0.14 m2 g−1) and biomass-burning (0.82±0.04 to 1.50±0.07 m2 g−1) sources. Together, these sources contributed at least 80 % of babs,BrC while accounting for 30 % to 40 % of the organic PM1 mass concentration. In addition, a comparison of organic PM1 composition between wet and dry seasons revealed that only part of the 9-fold increase in mass concentration between the seasons can be attributed to biomass burning. Biomass-burning factor loadings increased by 30-fold, elevating its relative contribution to organic PM1 from about 10 % in the wet season to 30 % in the dry season. However, most of the PM1 mass (>60 %) in both seasons was accounted for by biogenic secondary organic sources, which in turn showed an 8-fold seasonal increase in factor loadings. A combination of decreased wet deposition and increased emissions and oxidant concentrations, as well as a positive feedback on larger mass concentrations are thought to play a role in the observed increases. Furthermore, fuzzy c-means clustering identified three clusters, namely “baseline”, “event”, and “urban” to represent different pollution influences during the dry season. The baseline cluster, representing the dry season background, was associated with a mean mass concentration of 9±3 µg m−3. This concentration increased on average by 3 µg m−3 for both the urban and the event clusters. The event cluster, representing an increased influence of biomass burning and long-range transport of African volcanic emissions, was characterized by remarkably high sulfate concentrations. The urban cluster, representing the influence of Manaus emissions on top of the baseline, was characterized by an organic PM1 composition that differed from the other two clusters. The differences discussed suggest a shift in oxidation pathways as well as an accelerated oxidation cycle due to urban emissions, in agreement with findings for the wet season.
- Article
(11339 KB) - Full-text XML
-
Supplement
(3795 KB) - BibTeX
- EndNote
The Amazon basin has undergone significant urbanization and deforestation in the past decades (Davidson et al., 2012; Martin et al., 2017; van Marle et al., 2017). An understanding of how the composition of atmospheric particulate matter (PM) changes due to anthropogenic activities and how these changes affect PM optical properties is essential for quantifying the global anthropogenic radiative forcing (IPCC, 2013; Sena et al., 2013). Light absorption coefficients, babs, and their spectral dependence, commonly referred to as the Ångström absorption exponent, åabs, are needed for accurate interpretation of satellite-retrieved aerosol optical depth (AOD) for climate modeling. Estimates of the mass absorption efficiency Eabs for PM subcomponents are useful for models to estimate optical effects based on PM composition and mass concentrations (Laskin et al., 2015).
Organic material that can efficiently absorb radiation in the near-ultraviolet through the blue end of the visible spectrum, with decreasing absorption efficiency as wavelength increases, is termed “brown carbon” (BrC) (Pöschl, 2003; Andreae and Gelencsér, 2006; Laskin et al., 2015). By comparison, black carbon (BC) absorbs light efficiently throughout the visible spectrum. Although global climate models have typically treated organic PM as purely scattering, several studies have shown that brown carbon can contribute substantially to light absorption by PM, especially in regions affected by biomass-burning and urban emissions (Andreae and Gelencsér, 2006; Ramanathan et al., 2007; Bond et al., 2011; Bahadur et al., 2012; Ma and Thompson, 2012; Feng et al., 2013). In addition to primary emissions of BrC, secondary production of BrC can occur from the oxidation of non-methane volatile organic compounds (NMVOCs) present in biomass smoke (Saleh et al., 2014) and from atmospheric multiphase reactions involving a wide range of precursor NMVOCs (Nozière et al., 2007; De Haan et al., 2009; Nguyen et al., 2012; Lee et al., 2013; Lin et al., 2014; Powelson et al., 2014). The specific sources, chemical characteristics, and optical properties of BrC remain largely unconstrained.
Biomass-burning and urban pollution can affect the concentrations, composition, and properties of atmospheric PM. In Amazonia, urban pollution is significant downwind of large cities such as Manaus, Brazil (Kuhn et al., 2010; Martin et al., 2017; Cirino et al., 2018; de Sá et al., 2018). Martin et al. (2017) reported increased concentrations of particles, nitrogen oxides, carbon monoxide, and hydroxyl radicals for in-plume compared to out-of-plume conditions downwind of Manaus. Liu et al. (2016) and de Sá et al. (2017) demonstrated that the Manaus pollution plume shifted the oxidation pathway of isoprene, thereby significantly affecting gas- and particle-phase compositions. de Sá et al. (2018) determined that the submicron PM mass concentration increased by up to 3-fold for polluted compared to background conditions downwind of Manaus during the wet season.
Most biomass burning in Amazonia is related to human activities (Davidson et al., 2012; Artaxo et al., 2013; Aragão et al., 2014; van Marle et al., 2017). Among the main activities are the clearing of land and the burning of waste for several agricultural purposes as well as the burning of wood as fuel (Crutzen and Andreae, 1990; van Marle et al., 2017). Burning events are most frequent in the period of August through October, corresponding to the dry season (Setzer and Pereira, 1991; Artaxo et al., 2013; Martin et al., 2016). These activities can affect the biogeochemical cycles, atmospheric chemistry, precipitation, and climate throughout Amazonia (Crutzen and Andreae, 1990; Andreae et al., 2004; Lin et al., 2006). PM1 mass concentrations typically increase by an order of magnitude between the wet and dry seasons in the Amazon, which has been commonly attributed to the increased biomass-burning emissions (Artaxo et al., 1994, 2013; Holben et al., 1996; Martin et al., 2010b, and references therein). Related increases in babs by 1 order of magnitude have also been attributed to biomass burning (Rizzo et al., 2011, 2013; Artaxo et al., 2013). Although black carbon is usually the main light-absorbing component for atmospheric particles smaller than 1 µm (PM1), absorption by the organic BrC component of PM1 could also be significant (Rizzo et al., 2013; Wang et al., 2016; Saturno et al., 2017). Palm et al. (2018) showed that the formation potential of secondary organic PM1 increased by a factor of 1.7 in the dry season compared to the wet season, although biomass-burning gases were not dominant precursors in either season. An understanding of the types and optical properties of organic components that may affect PM1 light absorption in the Amazon and elsewhere is still emerging (Laskin et al., 2015).
The study herein investigates the contributions of biomass burning, urban emissions, and biogenic emissions to the composition and optical properties of organic PM1 in central Amazonia during the dry season. Positive-matrix factorization (PMF) of organic mass spectra measured by an aerosol mass spectrometer (AMS) was used to identify component classes of the organic PM1. A fuzzy c-means clustering analysis of pollution indicators was employed to identify different conditions at the measurement site, as influenced by biomass-burning and urban emissions. Connections are made between the optical properties of organic PM1, including babs,BrC and Eabs, and its component classes. Taken together, these three pieces of analysis allow for insights into the changes in particle concentration, composition, and optical properties associated with the influences of biomass-burning and urban pollution downwind of Manaus.
2.1 Research sites and measurements
The primary site of this study was called “T3”, located 70 km to the west of Manaus, Brazil, in central Amazonia (Martin et al., 2016; inset of Fig. 1a). The pollution plume from Manaus was primarily westerly in the dry season and was modeled to intercept the T3 site about 60 % of the time (Martin et al., 2017). Analyses of observational data sets have labeled pollution episodes for at least 15 to 30 % of the time (Thalman et al., 2017; Cirino et al., 2018). Auxiliary sites “T0a” and “T2” served as references for background and urban-polluted conditions, respectively, in relation to T3. The T0a site was located at the Amazon Tall Tower Observatory (Andreae et al., 2015), about 150 km to the northeast of Manaus, and the air masses were typically upwind of the urban region without the influence of Manaus pollution. The T2 site was located 8 km to the west of Manaus, directly downwind of the city, and air masses were therefore typically heavily polluted at this site. During the dry season, the three sites were also affected by both nearby and long-range-transported biomass-burning emissions. The study period from 15 August to 15 October 2014 corresponded to the second Intensive Operating Period (IOP2) of the GoAmazon2014/5 experiment (Martin et al., 2016).
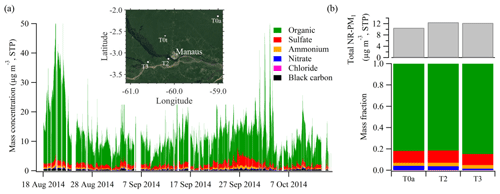
Figure 1PM1 composition during the dry season from 15 August to 15 October 2014, representing the second Intensive Operating Period (IOP2) of the GoAmazon2014/5 experiment. Results are shown for measurements at T3 in comparison to other sites. (a) PM1 mass concentrations of non-refractory AMS organic, sulfate, ammonium, nitrate, and chloride. Mass concentrations of SP2 refractory black carbon (rBC) are also plotted. rBC refers to the carbon content of graphite-like components that are strongly light absorbing (Pöschl, 2003). (b) Summed mass concentrations (top) and segregated mass fractions (bottom) of the non-refractory species at the T0a, T2, and T3 sites. The inset of panel (a) shows the locations of the relevant research sites for this study. A larger map is shown in Fig. 3. T0a is the Amazon Tall Tower Observatory (Andreae et al., 2015). T2 is a site 8 km downwind of Manaus, just across the Black River (“Rio Negro”) (Cirino et al., 2018). Measurements at T0a and T2 were made by an aerosol chemical speciation monitor (ACSM). Concentrations in both panels were adjusted to standard temperature (273.15 K) and pressure (105 Pa) (STP).
At the T3 site, mass concentrations of non-refractory PM1 components (organic, sulfate, ammonium, nitrate, and chloride) were measured by a high-resolution time-of-flight AMS (DeCarlo et al., 2006; Sueper, 2018). A detailed description of operation was provided in de Sá et al. (2017). In brief, the AMS was deployed inside a temperature-controlled research container, and ambient data were collected every other 4 min. Data analysis was performed using SQUIRREL (1.56D) and PIKA (1.14G) of the AMS software suite (DeCarlo et al., 2006). The mean composition-dependent collection efficiency was 0.51 (Sect. S1; Fig. S1 in the Supplement) (Middlebrook et al., 2012). Organic and inorganic nitrate concentrations were estimated from the AMS measurements based on the ratio of the signal intensity of to that of NO+ (Sect. S1, Fig. S2 in the Supplement) (Fry et al., 2009, 2013; Farmer et al., 2010). Sulfate measured by the AMS includes contributions from organosulfates (Farmer et al., 2010; Glasius et al., 2018). The oxygen-to-carbon (O:C) and hydrogen-to-carbon (H:C) ratios of the organic PM1 were calculated following the methods of Canagaratna et al. (2015).
Several other instruments complemented the AMS measurements. Gas- and particle-phase semi-volatile tracers were obtained by a semi-volatile thermal desorption aerosol gas chromatograph (SV-TAG) (Isaacman-VanWertz et al., 2016; Yee et al., 2018), and NMVOCs were obtained by a proton-transfer-reaction time-of-flight mass spectrometer (PTR-ToF-MS) (Jordan et al., 2009; Liu et al., 2016). In addition, measurements of NOy, O3, particle number, and CO concentrations were employed in the analyses (Martin et al., 2016). Refractory black carbon (rBC) concentrations were measured by a single particle soot photometer (SP2). Meteorological variables, including temperature, relative humidity, and solar irradiance were also measured. Particle absorption coefficients, babs(λ), were obtained by a seven-wavelength aethalometer (370, 430, 470, 520, 565, 700, and 880 nm; Magee Scientific, model AE-31) following the methods and corrections of Rizzo et al. (2011). Additional measurements of non-refractory particle composition and concentration from the T0a and T2 sites were made by an aerosol chemical speciation monitor (ACSM) at each site (Ng et al., 2011; Andreae et al., 2015; Martin et al., 2016).
Air-mass back-trajectories were estimated using Hybrid Single-Particle Lagrangian Integrated Trajectory (HYSPLIT4; Draxler and Hess, 1998). Data sets of the S-band radar of the System for Amazon Protection (SIPAM) in Manaus (Machado et al., 2014) provided precipitation data, which allowed to filter out trajectories that intercepted precipitation. The HYSPLIT4 simulations started at 100 m above T3 and were calculated up to 2 d back in time for every 12 min to match with the radar data. Input meteorological data to the simulations were obtained on a grid of 0.5∘ × 0.5∘ from the Global Data Assimilation System (GDAS). Additional information on the back-trajectory calculations and on the radar were described in de Sá et al. (2018).
2.2 Brown carbon light absorption
The analysis partitioned the total absorption babs(λ) measured by the aethalometer between BrC and BC contributions as follows:
The dependence on wavelength was expressed by the absorption Ångström exponent (åabs) as follows:
For the characterization of BrC absorption, the value of at 370 nm was sought. To calculate babs,BrC(370), an assumption has to be made about the spectral dependency of BC light absorption. In this study, åabs,BC was assumed to be wavelength independent, and å was calculated for each sample based on babs at the wavelengths 700 and 880 nm (Eq. 2), assuming absorption to be insignificant for BrC and dominated by BC in this spectral range. Calculations of babs,BrC(370) using alternative treatments to retrieve åabs,BC were also carried out. These treatments included the assumption that åabs,BC is equal to 1.0 and wavelength-independent (e.g., Yang et al., 2009), or the assumption that åabs,BC has a spectral dependency itself (Wang et al., 2016; Saturno et al., 2018a). The results from these different treatments correlated with one another (R2>0.9), and the babs,BrC estimate used in this study and detailed in the steps below represented a lower bound among the differing assumptions (Sect. S4).
For each point in time, babs,BrC(370) was estimated by the following steps: (1) and assuming that at red wavelengths (e.g., Andreae and Gelencsér, 2006; Wang et al., 2016); (2) å was calculated from Eq. (2) using babs,BC(700) and babs,BC(880); (3) babs,BC(370) was calculated from Eq. (2), using babs,BC(880) and å = å under the assumption that åabs,BC was independent of wavelength (e.g., Andreae and Gelencsér, 2006; Moosmüller et al., 2009); and finally (4) babs,BrC(370) was obtained by Eq. (1) using babs,BC(370) and babs(370). The value of babs,BrC at 430 nm was also obtained by the same process. Based on babs(370) and babs(430), åabs(370,430) was estimated. Hereafter, babs and babs,BrC refer to 370 nm, and åabs refers to the range of 370 to 430 nm.
The aethalometer, like other filter-based measurement schemes (e.g., PSAP, TAP, or MAAP), is prone to artifacts. These artifacts may originate from light scattering by the filter media itself, the influence of the filter media on the microphysical properties of the collected particle (e.g., potential change in hygroscopic particle size), and the impact of the multiple scattered photons on the measured optical extinction (e.g., enhanced particle absorption as discussed by Nakayama et al., 2010). While several correction schemes have been developed to address these artifacts, the individual schemes do not approach these problems in the same way, which may lead to different results among them (Weingartner et al., 2003; Schmid et al., 2006; Collaud Coen et al., 2010; Rizzo et al., 2011; Ammerlaan et al., 2017). For the present analysis, the correction scheme used was described by Rizzo et al. (2011). The potential impact of the different correction schemes on the analysis interpretation was not examined.
3.1 Contributions of biomass-burning and urban emissions to fine-mode PM
3.1.1 Comparison of PM concentration and composition across sites
A comparison between the T3 site and the upwind sites can provide a first-order estimate of the effects of Manaus urban pollution on PM1 concentration and composition (de Sá et al., 2018). During the dry season of 2014, organic compounds dominated the composition at T3, contributing 83±6 % (mean ± 1 standard deviation) of the non-refractory PM1 (NR-PM1), followed by sulfate (11±5 %) (Fig. 1a). Mean NR-PM1 mass concentrations and relative compositions at T3 and at T0a and T2 are represented in Fig. 1b for comparison. Organic material consistently constituted 80 % to 85 % of NR-PM1 across all three sites. By comparison, the contribution of organic material to NR-PM1 typically ranged from 70 % to 80 % during the wet season (de Sá et al., 2018).
The NR-PM1 mass concentrations across the three sites differed slightly (Fig. 1b, top panel). The mean concentration at the T0a site upwind of Manaus was 10.5 µg m−3. The mean concentrations at the T2 site just downwind of Manaus and at the T3 site further downwind were 12.5 and 12.2 µg m−3, respectively, representing an increase of about 20 % relative to the upwind site. By comparison, increases of 200 % to 300 % relative to the upwind site were observed during the wet season (de Sá et al., 2018). In absolute mass concentration, however, the difference between upwind and downwind sites of 1 to 2 µg m−3 was similar between seasons, suggesting contributions from urban pollution of the same order of magnitude in both seasons. The larger percent increase for the wet season is explained by background concentrations of 1 µg m−3, which are an order of magnitude lower compared to the dry season.
The time series of organic mass concentrations across the three sites were well correlated across the 2 months when considering the timescale of a day (Fig. 2a; ). Similar behavior was observed for sulfate mass concentrations (Fig. 2b; ). The T0a and T3 sites were separated by 215 km. This result shows that sources and processes of PM1 production at a regional scale were important during the dry season. The data also show that for timescales of an hour the sites were less correlated ( for sulfate, and for organic mass concentrations). The large spikes in organic mass concentrations observed at T3 but generally smaller at T2 and absent at T0a could be explained by episodic fires along the Solimões River, especially during nighttime (Fig. 3).
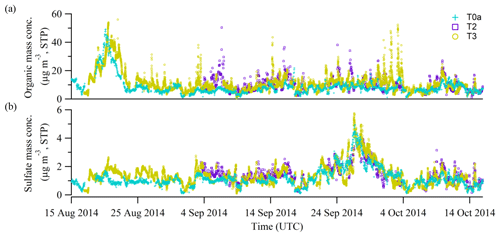
Figure 2Time series of (a) organic and (b) sulfate mass concentrations at the T0a, T2, and T3 sites. Concentrations were adjusted to standard temperature (273.15 K) and pressure (105 Pa).
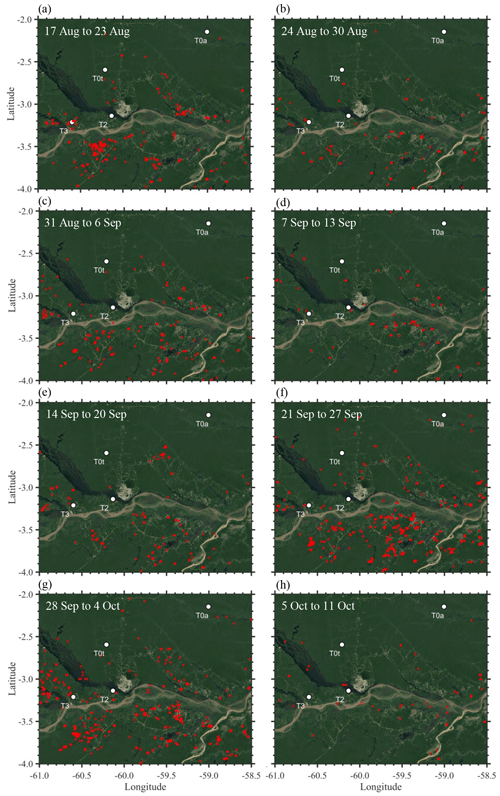
Figure 3Fire locations in the upwind region of the T3 site for each week of IOP2. Transport times from the fires to the T3 site represent up to 15 h at the scale of this figure and typical wind speeds. The plotted data were obtained from the fire database of the Brazilian National Institute of Spatial Research (INPE, 2018). Underlying image: Google Maps.
In addition to the widespread and frequent occurrence of fires in the Amazon basin during the dry season (Fig. 3), meteorological conditions may also favor a regional reach of events (Sect. S3). For example, high organic concentrations were observed during the period of 17 to 23 August. During that week, widespread biomass-burning activity in the basin (beyond the scale of Fig. 3), in conjunction with a lack of precipitation events, clear skies, and temperatures of 35 ∘C during daytime, allowed for intense photochemical activity and buildup of PM1. There appeared to be an offset in PM1 concentrations by 1 d between T0a and T3 during that time, which would be consistent with the transport across 215 km from T0a to T3 for typical easterlies averaging 3 m s−1 over the course of a day. In short, a combination of regional-scale biomass-burning activity and meteorological conditions greatly influenced the mass concentration of PM1 at the three sites.
The diel variability of organic and sulfate mass concentrations for the three sites is shown in Fig. 4. Organic mass concentrations were slightly higher at the T2 and T3 sites compared to the T0a site, as expected. The variability was larger at the T2 and T3 sites, especially at night. These two sites are closer to populated areas along the path of the Solimões River and thus are also closer to local biomass-burning sources. Activities include burning of crops and trash in houses and farms as well burning of wood in brick kilns (Martin et al., 2016; Cirino et al., 2018). Stagnant air and a shallow boundary layer during the night might explain how variable biomass-burning emissions lead to larger organic mass concentrations and variability at night compared to the day.
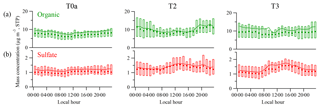
Figure 4Diel trends of (a) organic and (b) sulfate mass concentrations at the T0a, T2, and T3 sites. Lines represent means, solid markers show medians, and boxes span interquartile ranges. Local time is UTC minus 4 h. Concentrations were adjusted to standard temperature (273.15 K) and pressure (105 Pa).
The influence of anthropogenic emissions on daytime chemistry is apparent in the diel trends of the sulfate mass concentrations. Sulfate concentrations had low variability throughout the day at T0a, indicating a prevalence of diffuse regional sources that had variations dampened after many hours or days of transport. Possible sources include the atmospheric oxidation of biogenic emissions (DMS, H2S) from the upwind forest and ocean, as well as long-range transport of fossil fuel combustion emissions from cities in northeastern Brazil and of biomass burning and volcanic emissions from Africa (Andreae et al., 1990; Martin et al., 2010a; Saturno et al., 2018b). Biomass burning can be an important source of sulfate and its precursors (Andreae and Merlet, 2001; Fiedler et al., 2011). For the T2 and T3 sites, sulfate concentrations increased in the morning hours and peaked in the afternoon. The Manaus sulfate source consists of the burning of heavy fuel oil for electricity production, refinery operations, and more diffuse traffic sources, and these emissions reach the T3 site in the afternoon, when OH levels are also the highest (de Sá et al., 2017). In addition, biomass-burning emissions around T2 and T3 might also have contributed to the increase in sulfate concentrations during the afternoons.
3.1.2 Comparison of PM concentration and composition across clusters for the T3 site
A second approach to investigate the changes in concentrations and compositions of the PM with pollution influences employed a combination of PMF and fuzzy c-means (FCM) clustering. The PMF analysis was applied to the organic mass spectra to separate the organic PM1 into representative component classes (Sect. “Classification of organic PM1 by positive-matrix factorization”). The FCM clustering algorithm was applied to auxiliary measurements to identify times of urban and biomass-burning influences at the T3 site (Sect. “Cluster analysis”). The results of the FCM analysis were crossed with the findings of the PMF analysis for further insights into pollution-related variability of PM concentration and composition (Sect. “Comparison of PM1 composition among clusters”).
Classification of organic PM1 by positive-matrix factorization
The organic mass spectra recorded by the AMS at the T3 site were analyzed by PMF (Ulbrich et al., 2009). Details and diagnostics of the PMF analysis are presented in the Supplement (Sect. S1). Following the nomenclature used in de Sá et al. (2018), “mass spectrum” and “mass concentration” refer to the direct AMS measurements, while “factor profile” and “factor loading” are their counterpart mathematical products obtained from the PMF analysis. A six-factor solution was obtained, and the factor profiles, diel trends of the factor loadings, and the time series of the factor loadings and other related measurements are plotted in Fig. 5. The correlations of factor loadings with co-located measurements of gas- and particle-phase species are presented in Fig. 6.
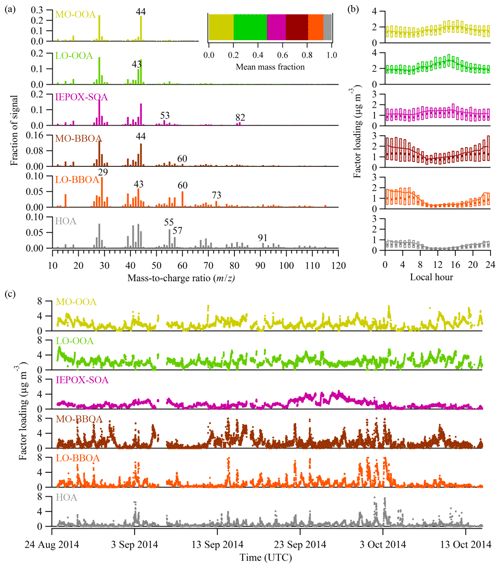
Figure 5PMF analysis of the time series of AMS organic mass spectra collected at the T3 site. (a) Mass spectral profile of each factor represented at unit mass resolution. The inset shows the mean fractional loading of each factor. The factor profiles are shown in more detail, colored by ion families, in Fig. S5. (b) Diel trends for the loadings of each PMF factor. Local time is UTC minus 4 h. Lines represent means, solid markers show medians, and boxes span interquartile ranges. (c) Time series of the factor loadings.
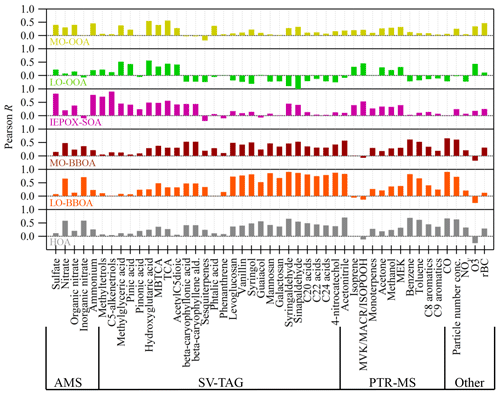
Figure 6Pearson R correlations between the loading of each PMF factor and concentrations of selected measurements at the T3 site. Abbreviations include tricarballylic acid (TCA), methyl-butyl-tricarboxylic acid (MBTCA), methyl vinyl ketone (MVK), methacrolein (MACR), isoprene hydroxyhydroperoxides (ISOPOOH), and rBC. SV-TAG measurements refer to particle-phase concentrations, except for sesquiterpenes which refer to total concentrations and mostly occurred in the gas phase. The C8 and C9 aromatics include the xylene and trimethylbenzene isomers, respectively. The C20, C22, and C24 acids include eicosanoic, docosanoic, and tetracosanoic acids, respectively.
The factors were interpreted considering the mass spectral characteristics of the factor profiles and the correlations between factor loading and mass concentrations of co-located measurements. Three resolved factors interpreted as secondary production and processing closely matched the counterpart profiles of the wet season (R≥0.99; Table 1) (de Sá et al., 2018). These three factors consisted of a more-oxidized oxygenated factor (“MO-OOA”), a less-oxidized oxygenated factor (“LO-OOA”), and an isoprene epoxydiols-derived factor (“IEPOX-SOA”). Temporal correlations with external tracers and oxidation characteristics were also similar to those of the wet season, corresponding to the first Intensive Operating Period (IOP1) (Fig. 6; Table 1; de Sá et al., 2018). Although a hydrocarbon-like factor (“HOA”) was analogous to its counterpart in IOP1 (R=0.94), it also had characteristics of an IOP1 anthropogenic-dominated factor (“ADOA”) tied to other urban sources including cooking. The HOA factor of IOP2 therefore represented a mix of the HOA and ADOA factors of IOP1, which could not be separated by PMF in IOP2 due to their lower relative contributions. The interpretation of the HOA, IEPOX-SOA, LO-OOA, and MO-OOA factors follows that of IOP1, as presented in de Sá et al. (2018). The following discussion focuses on the two biomass-burning factors of IOP2.
Table 1Characteristics of the PMF factor profiles. Listed are f44 and f60, corresponding to the organic signal fraction at m∕z 44 and 60, respectively, as well as the oxygen-to-carbon (O:C) and hydrogen-to-carbon (H:C) ratios. Values and uncertainties were calculated by running the PMF analysis in “bootstrap mode” (Ulbrich et al., 2009). The Pearson R correlations between the factor profiles of IOP2 and their counterparts in IOP1 are also listed (i.e., dry season compared to wet season). “n/a” means “not applicable”. Elemental ratios were calibrated by the “improved-ambient” method, which has an estimated uncertainty of 12 % for O:C and 4% for H:C (Canagaratna et al., 2015).
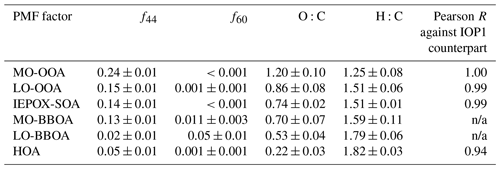
A less-oxidized factor (“LO-BBOA”) and a more-oxidized factor (“MO-BBOA”) were resolved for IOP2. For IOP1, a single “BBOA” factor was resolved, and it accounted for 9 % of the organic PM1 mass concentration. For IOP2, there were enough differences in mass spectral features and temporal contributions, as well as larger overall contributions of biomass burning, that the PMF analysis identified two different factors. The MO-BBOA and LO-BBOA factors, respectively, accounted for 18 % and 12 % of the mean organic PM1 mass concentration. Therefore, the relative contribution of biomass burning to organic PM1 during the dry season was at least a factor of 3 higher compared to the wet season (a more detailed discussion is presented at the end of this section).
The LO-BBOA and MO-BBOA factor profiles had a distinct peak at nominal m∕z 60 () (Fig. 5a). The fractional intensity f60 at m∕z 60 was larger for LO-BBOA (0.051) than for MO-BBOA (0.013). A peak at m∕z 73 () was also present in both profiles, although its intensity was 3–4 times smaller than that at m∕z 60. The peaks at m∕z 60 and 73 are attributed to fragments of levoglucosan and other anhydrous sugars that are produced by the pyrolysis of biomass (Schneider et al., 2006; Cubison et al., 2011). Accordingly, the loadings of both factors correlated with the concentrations of several biomass-burning tracers in the particle phase, including levoglucosan, vanillin, 4-nitrocatechol, syringol, mannosan, syringaldehyde, sinapaldehyde, and long-chain alkanoic acids (C20, C22, C24) and of tracers in the gas phase (acetonitrile) (Fig. 6). The loadings also correlated with less-specific tracers, including CO concentration and particle number concentration. The Pearson R correlations were typically higher for the LO-BBOA factor than for the MO-BBOA factor.
The LO-BBOA profile had the greatest ratio of signal intensity of the C2H3O+ ion (m∕z 43) to that of the ion (m∕z 44) compared to all other factors (Fig. 5a). In comparison, the MO-BBOA profile had a high intensity for the ion and a low intensity for the C2H3O+ ion. The MO-BBOA and LO-BBOA factors had O:C ratios of 0.70±0.07 and 0.53±0.04, respectively. In addition, the LO-BBOA factor loading had higher correlation with the estimated inorganic nitrate concentrations than with the total nitrate concentrations, whereas the MO-BBOA factor did not (Fig. 6; Sect. S1 in the Supplement describes the nitrate estimates). Taken together, these results point to a less-oxidized, higher-volatility character of the LO-BBOA factor and a more-oxidized, lower-volatility character of the MO-BBOA factor, both with biomass-burning characteristics (Jimenez et al., 2009; Cubison et al., 2011; Gilardoni et al., 2016; Zhou et al., 2017).
The extent of the biomass-burning influence and atmospheric oxidation on the composition of organic PM1 can be visualized in a scatter plot of f44 and f60 (Fig. 7a) (Cubison et al., 2011). A background f60 value of 0.3 % ± 0.06 % (vertical black dashed line) indicates a threshold for negligible or completely oxidized biomass-burning PM1. Points in the lower right of the f44–f60 representation usually characterize PM1 tied to recent biomass-burning emissions. For IOP1 (blue markers), all points lie on or close to the background value suggested by Cubison et al. (2011), indicating the absence of a strong influence from biomass burning. During the wet season, biomass burning was limited to local sources or to sources far enough away such as Africa that the PM1 was extensively oxidized by arrival in central Amazonia (de Sá et al., 2018). For IOP2 (red markers), the f60 values are greater for most observations, showing that for most times T3 was influenced to some extent by biomass burning (see Sect. “Comparison of PM1 composition among clusters”). This finding is in line with the widespread occurrence of fires during the dry season (Fig. 3). As suggested by the robust trend in Fig. 7a, the f44 value increases and the f60 value decreases from the bottom right to the upper left as the organic PM1 emitted by biomass burning is oxidized in the atmosphere. The f60 and f44 values of the LO-BBOA and MO-BBOA profiles, plotted as diamonds, lie on the linear trend.
The LO-BBOA factor of high f60∕f44 and low O:C thus appears associated with primary PM1 emitted by biomass burning. The MO-BBOA factor, characterized by low f60∕f44 and high O:C, may represent a combination of primary PM1 of higher oxygen content as well as secondary PM1 tied to biomass burning in its early stages of atmospheric processing (Cubison et al., 2011; Gilardoni et al., 2016). These secondary pathways could include (i) the heterogeneous oxidation of primary PM1, such as that represented by the LO-BBOA factor, and (ii) the oxidation of gas-phase biomass-burning emissions or of species evaporated from primary PM1, followed by the condensation of the gas-phase products onto the PM1.
The LO-BBOA and MO-BBOA factor loadings had greater magnitude and variability at night compared to during the day (Fig. 5b). Their summed loading, represented as “BBOAT”, accounted for 40 % and 13 % of the organic PM1 during night and day, respectively. Overall, they accounted for 30 % of the organic PM1. This result reflects the importance of fire activity during all times of day and during the entirety of IOP2 (Fig. 3). The surface concentrations were lower during the day because biomass-burning emissions are diluted with the development of the planetary boundary layer (PBL) and with the increased wind speeds as compared to the stagnant air and shallower PBL at night. The occurrence of significant dilution indicates that the emission sources were at least in part within a day of transport, meaning a distance on the order of a few hundred kilometers. The fractional contribution of the MO-BBOA factor to BBOAT shifted from 0.7 to 0.5 from day to night, while that of LO-BBOA correspondingly shifted from 0.3 to 0.5 (Fig. 7b). This result is consistent with an additional secondary contribution to the MO-BBOA loading during daytime, including from LO-BBOA oxidation and possibly tied to photochemical processing, on top of a primary source from biomass burning.
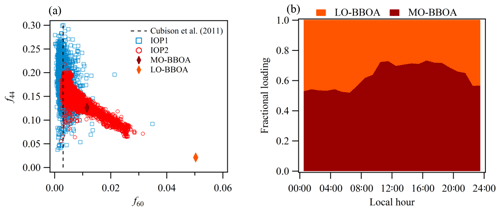
Figure 7Analysis of the organic PM1 sampled at the T3 site in relation to biomass burning. (a) Scatter plot of the AMS signal fraction at m∕z 44 (f44) against that at m∕z 60 (f60). Red circles represent measurements during the dry season (IOP2), and blue squares represent measurements at the same site during the wet season (IOP1) (de Sá et al., 2018). Diamonds represent the MO-BBOA and LO-BBOA factors of IOP2. The dashed line represents a reference for negligible influence by biomass burning based on several field studies (Cubison et al., 2011). (b) Diel trends of the fractional loadings of the MO-BBOA and LO-BBOA factors relative to their sum BBOAT. Local time is UTC minus 4 h.
Although the footprint of biomass burning is geographically more widespread throughout the basin compared to the urban footprint of nearby Manaus, fire incidence and large-scale emissions have historically concentrated in a region known as the arc of deforestation along the southern rim of the forest (Fuzzi et al., 2007; Artaxo et al., 2013). Several campaigns have focused on the effects of biomass burning during the dry season at locations that are highly affected by fires, usually in the states of Rondônia or Mato Grosso, within the arc of deforestation (SCAR-B, Kaufman et al., 1998; LBA-SMOCC, Fuzzi et al., 2007; LBA-EUSTACH, Andreae et al., 2002; TROFEE, Yokelson et al., 2007; SAMBBA, Morgan et al., 2013). At a ground site in Porto Velho, Rondônia, a PMF analysis of ACSM data showed that 70 % of the organic PM1 could be attributed to biomass burning (Brito et al., 2014). Compared to the present study, in which at least 30 % of the organic PM1 can be directly attributed to biomass burning, the contributions of fires to PM1 in the arc of deforestation region are considerably larger.
The combined contribution of 30 % by MO-BBOA and LO-BBOA at T3 represents a lower bound of biomass-burning influence because more-oxidized material from biomass burning could be accounted for by the MO-OOA factor. In the limiting assumption that all MO-OOA loadings originated from BBOA loadings, an upper limit of 50 % can be established for the mean contribution of biomass burning to organic PM1 concentrations at T3. Considering that all organic PM1 components have been observed to age into MO-OOA at similar rates (Jimenez et al., 2009), a more likely estimate of 38 % can be derived by assuming that all factors contribute to MO-OOA proportionally to their ambient concentrations.
An important implication of these results, together with those of the wet season, is that although PM1 concentrations increase on average by a factor of 8.5 between seasons, not all of the increase is due to biomass burning, which has been a common assumption in previous studies (Artaxo et al., 1994, 2002, 2013; Holben et al., 1996; Echalar et al., 1998; Maenhaut et al., 1999; Andreae et al., 2002; Mace et al., 2003; Martin et al., 2010b; Rizzo et al., 2013; Brito et al., 2014; Pöhlker et al., 2016). In absolute mass concentrations, the contribution from biomass burning increased from 0.12 µg m−3 in the wet season to 3.4 µg m−3 in the dry season, which represents a 30-fold increase. This result corresponds to a change in percentage contribution to organic PM1 from 9 % to 30 % (not counting with the mass presumably present in MO-OOA). Nevertheless, the contribution from secondary biogenic sources (and their anthropogenically affected processes), as represented by the LO-OOA and IEPOX-SOA factors, also increased by around 8-fold from 0.6 to 4.8 µg m−3. In absolute terms, this mass increase (of 4.2 µg m−3) is comparable to the one associated with biomass burning (3.3 µg m−3). Because the 8-fold mass increase of LO-OOA and IEPOX-SOA was similar to the 8.5-fold increase in total organic PM1, these factors show a similar mass percentage contribution of 42 % to organic PM1 for both seasons. The MO-OOA factor loadings increased by 6-fold from 0.4 to 2.3 µg m−3. Because this relative increase was smaller than that of the total organic PM1, the MO-OOA factor had a decrease from 30 % to 20 % of contribution to organic PM1. The contribution from urban sources, as represented by the HOA and ADOA factors, increased by 3-fold between seasons, from 0.24 to 0.76 µg m−3, representing a decrease in mass percentage contribution from 18 % to 7 %.
Therefore, reasons other than increased biomass burning in the dry season must have played a role in increasing organic PM1 concentrations. Importantly, the mass concentrations of sulfate and ammonium also increased by 6-fold between seasons (Fig. S10), indicating that atmospheric physical processes governing particle mass concentrations possibly played an important role. In this context, reduced wet deposition due to reduced convection in the dry season may be one important contributor to the organic PM1 increases (Machado et al., 2004; Nunes et al., 2016; Chakraborty et al., 2018). Another aspect is that biogenic NMVOC (BVOC) emissions are typically higher in the dry season (Yáñez-Serrano et al., 2015; Alves et al., 2016), which might partly explain the increases in LO-OOA, IEPOX-SOA, and MO-OOA factors. In addition, the directly measured biogenic (total) secondary organic PM1 formation potential of ambient air increased by a factor of 2.4 (1.7) between seasons (Palm et al., 2018). Increased organic mass available for partitioning may account for another factor of 2 (Palm et al., 2018). As a consequence of increased PM1 mass concentrations, the lifetime of semi-volatile gases may also be increased, since lifetime against dry deposition is much larger for particles than for gases (Knote et al., 2015). Increased oxidant levels during the dry season could also be a contributing factor (Rummel et al., 2007; Artaxo et al., 2013; Andreae et al., 2015; Yáñez-Serrano et al., 2015; Fuentes et al., 2016).
Cluster analysis
The time series of the afternoon concentrations of particle number, NOy, ozone, rBC, carbon monoxide, and sulfate were analyzed by fuzzy c-means clustering at the time resolution of the AMS measurements. The algorithm attributed degrees of cluster membership to each data point based on similarity in the sets of input concentrations (Sect. S2). The scope was restricted to data sets for which 10 h air-mass back-trajectories did not intersect precipitation. The scope also excluded data sets tied to the lowest 10 % of solar irradiance averaged over the previous 4 h at T3 (Sect. S2 in the Supplement). This approach aimed to capture fair-weather conditions and thereby minimize the role of otherwise confounding processes that influence mass concentrations, such as boundary layer dynamics and wet deposition.
Three clusters, labeled “baseline,” “event,” and “urban,” were identified based on a combination of minimization of the FCM objective function and an assessment of meaningfulness of the resolved set of clusters. Illustrative examples of the obtained degrees of membership (0 to 1) are plotted in Fig. 8a for several time windows. The concentrations of the input and additional species are plotted in Fig. 8b and c. The PMF results of Sect. “Classification of organic PM by positive-matrix factorization” are plotted for comparison in Fig. 8d. Air-mass back-trajectories are plotted in Fig. 9 for time windows predominantly associated with only one cluster.
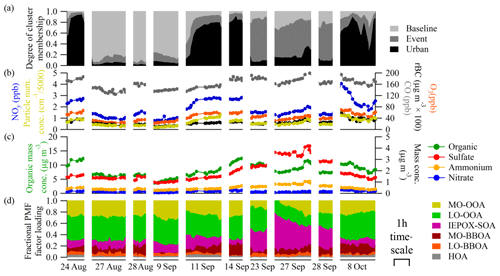
Figure 8Results of the cluster analysis by FCM clustering for afternoon periods (12:00 to 16:00 LT) presented by several case studies. The shown case studies represent 30 % of the FCM results. (a) Degree of membership in each of the three clusters. The sum of degrees of membership across all clusters is unity. (b) Pollution indicators: concentrations of NOy, O3, CO, rBC, and particle number count are plotted. (c) PM1 mass concentrations for organic, sulfate, nitrate, and ammonium species. (d) Fractional contribution of each factor to the PM1 organic mass concentration.
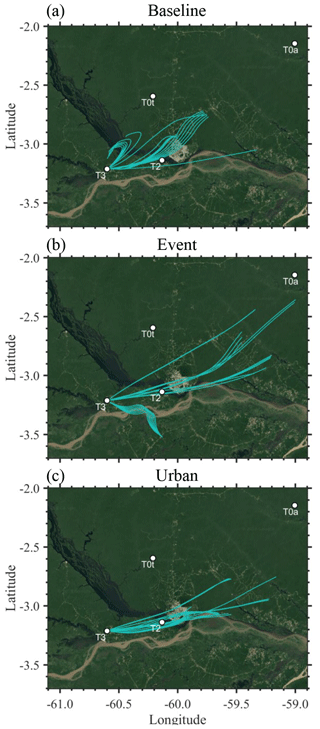
Figure 9Air-mass back-trajectories associated with the three clusters of the FCM analysis. Trajectories were calculated using HYSPLIT4 in steps of 12 min and are shown for 10 h (Draxler and Hess, 1998). A total of 20 trajectories are plotted for each cluster, corresponding to the times of highest degree of membership to that cluster.
All three clusters reflected, albeit to different degrees, some influence of biomass burning. For the wet season, de Sá et al. (2018) identified clusters representing background conditions, which were characterized by low concentrations of particle number, NOy, and O3. For the dry season, no similar cluster was identified. As shown in Fig. 3, there were fires in the region at all times (cf. Martin et al., 2017).
The baseline cluster had the lowest concentrations of pollutant indicators, representing influences of far-field biomass burning on top of natural (i.e., biogenic) emissions and atmospheric processing. The cluster centroid corresponded to 1.3 ppb NOy, 30 ppb ozone, and 2000 particles cm−3 (Table S1 in the Supplement). Results for 27 August, 28 August, and 9 September illustrate these lower concentrations compared to the other days (Fig. 8). The back-trajectories associated with the baseline cluster did not intersect the urban area of Manaus, especially the southern region of presumed higher emissions (Fig. 9a; de Sá et al., 2018).
The event cluster referred to conditions of increased influence from biomass burning and long-range transport of volcanic emissions from Africa. The cluster corresponded to a 10 d period from 22 September to 1 October in which biomass burning intensified in the surroundings of T3 as well as more broadly in the Amazon basin (Fig. 3f and g). Coincidentally, plumes carrying emissions from the Nyamuragira–Nyiragongo volcanoes in Africa were also observed to reach central Amazonia during that time period, as demonstrated by Saturno et al. (2018b). This cluster was characterized by higher concentrations of all species in relation to the baseline cluster (Table S1). In particular, the sulfate concentrations (2.3 µg m−3 at the centroid) were the highest among the three clusters. Results for 23, 27, and 28 September illustrate these findings for T3, with sulfate concentrations reaching 4 µg m−3 (Fig. 8). This trend in sulfate concentrations was consistent across all three sites (Fig. 2). The back-trajectories associated with the event cluster were variable, passing to the north, directly over, and to the south of Manaus, although always with an east component (Fig. 9b). The long-range transport and increased regional fire count during the event period thus appeared more important in defining this cluster than did the directions of the back-trajectories in a smaller scale, making Manaus emissions of secondary importance.
The urban cluster had the highest centroid concentrations of NOy (2.6 ppb), ozone (56.4 ppb), and particle number (4600 cm−3) among the three clusters (Table S1). It represented conditions for which both biomass-burning and urban emissions were relevant, and these emissions may have interacted before reaching the T3 site. The results for 24 August, 11 September, 14 September, and 8 October illustrate the high pollutant concentrations (Fig. 8). The back-trajectories associated with the urban cluster consistently passed over Manaus and, more specifically, over the southern region where human activities were more concentrated (Fig. 9c).
Comparison of PM1 composition among clusters
Species mass concentrations and PMF factor loadings associated with the cluster centroids were determined (Sect. S2). The resulting organic, sulfate, ammonium, nitrate, and chloride mass concentrations associated with each cluster are represented in Fig. 10a. The PMF factor loadings associated with each cluster are likewise represented in Fig. 10b.
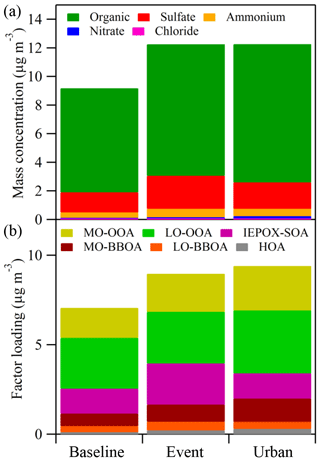
Figure 10PM1 characterization represented by the centroids of the FCM clusters. (a) Mass concentrations of AMS species. (b) PMF factor loadings. Results are for afternoon time periods. Table S1 lists the values presented in this figure.
The summed NR-PM1 mass concentrations for the centroids of the event and urban clusters were both 12.3 µg m−3. This concentration was 33 % higher than that representing the baseline cluster (9.2 µg m−3). This result thus agrees with that based on direct comparison of PM1 mass concentrations between the T3 and the T0a sites (Sect. 3.1.1). Therefore, the overall effect of Manaus pollution was to add 1 to 3 µg m−3 on top of the upwind concentrations. Increases in the organic mass concentration dominated the overall increase in PM1 mass concentration because organic species dominated the composition for all three clusters. The increases in organic mass concentration for the event and urban clusters relative to the baseline cluster were 26 % and 33 %, respectively (Fig. 10a).
Sulfate concentrations also increased relative to the baseline cluster, corresponding to 65 % for the event cluster and 31 % for the urban cluster. This result indicates that strong biomass-burning emissions reaching areas downwind of Manaus as well as long-range transport of volcanic emissions from as far away as Africa may increase sulfate concentrations in those areas beyond the sulfate values driven by the anthropogenic activities in the city. In other words, there were several other in-basin as well as out-of-basin sources of sulfate besides Manaus that could sustain relatively high sulfate concentrations (Chen et al., 2009; de Sá et al., 2017; Saturno et al., 2018b).
The relationship between clusters and PMF factors is represented in Fig. 10b. All three clusters were associated with an organic PM1 composition dominated by secondary production. The baseline cluster was largely dominated by the LO-OOA factor (40 %). By comparison, the event cluster had significant increases in the LO-BBOA, MO-BBOA, and IEPOX-SOA factor loadings. The increase in LO-BBOA and MO-BBOA loadings (40 %) can be associated with the increased contributions of primary and secondary particle components from biomass burning, respectively. The LO-BBOA factor had the highest loading (0.5 µg m−3) for the event cluster, consistent with the high incidence of fires during the period represented by this cluster. The increase of 65 % in IEPOX-SOA loading can be explained by the disproportionally higher increase of 65 % in the sulfate concentration (which favors higher IEPOX-SOA loadings), accompanied by the relatively moderate increase of 34 % in NOy concentration (which suppresses IEPOX-SOA loadings), leading to a net increase in IEPOX-SOA loadings (Table S1; de Sá et al., 2017).
The composition of the organic PM1 associated with the urban cluster differed from that of the two other clusters, as indicated by the factor contributions (Fig. 10). Compared to the baseline cluster, the loadings of all factors except IEPOX-SOA increased. An increase in HOA loading is consistent with emissions in the city, including from vehicles and power plants. An increase in the loadings associated with secondary processes, as represented by the MO-OOA, LO-OOA, and MO-BBOA factors, can be explained by the accelerated oxidation cycle in the plume. In brief, an increase in the concentrations of both precursors and oxidants provided by urban emissions accelerates the production of secondary PM1 and thereby increases the PM1 concentrations downwind of the city (Martin et al., 2017; de Sá et al., 2018).
The similarity in IEPOX-SOA factor loading for the baseline and the urban clusters may be explained by the following aspects. First, the lifetime of IEPOX-derived PM in the boundary layer is thought to be around 2 weeks (Hu et al., 2016). Therefore, a substantial fraction of this component observed at T3 will be formed upwind of the Manaus plume. Second, favored conditions for IEPOX production and uptake are low NO concentrations (i.e., HO2-dominant pathway for the ISOPOO radical) and high sulfate concentrations (de Sá et al., 2017). Sulfate concentrations increased by 31 %, and NOy concentrations, used as an indicator for exposure of the air mass to NO concentrations, increased by 100 % for the urban compared to the baseline cluster. These two changes work against one another with respect to IEPOX production and uptake. For the wet season, de Sá et al. (2017) reported that the IEPOX-SOA factor loading was more sensitive to changes in NOy concentration for 1 ppb and less. By comparison, NOy concentrations in the dry season were consistently greater than this value. Due to this lower sensitivity, large increases in NOy may not be tied to large decreases in IEPOX-SOA factor loading in the dry season. In sum, the opposite roles of sulfate and NOy concentrations can explain the net zero change in IEPOX-SOA factor loadings between baseline and urban clusters. Because all of the loadings for other factors increased, the fractional loading of IEPOX-SOA decreased from 26 % to 15 %.
3.2 Contributions of biomass-burning and urban emissions to brown carbon
3.2.1 Brown carbon light absorption
The diel trends of babs, babs,BrC, , and åabs are shown in Fig. 11. Both babs and babs,BrC were larger and had greater variability at night compared to day. The variability of the fractional contribution of BrC to the total absorption, represented by , was smaller than the variability of its components babs and babs,BrC (i.e., Fig. 11c compared to Fig. 11a–b). The absorptive contributions of BC and BrC thus co-varied to some extent, suggesting a partial overlap in sources, which is consistent with previous studies (Collier et al., 2016; Jen et al., 2019). Furthermore, the fractional contribution increased from 0.2 in the day to 0.4 at night. The absorption Ångström exponent åabs followed a similar diel trend, on average ranging from 2 during the day to 3 during the night (Fig. 11d). Compared to the diel trends of the six PMF factor loadings, the diel trends of the absorption properties were most similar to those of the MO-BBOA, LO-BBOA, and HOA factors (Fig. 5b).
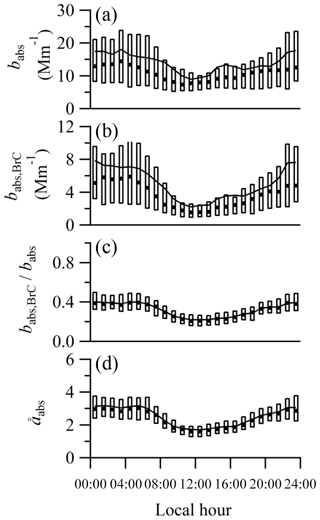
Figure 11Diel trends of PM1 optical properties. (a) Total absorption coefficient babs (370 nm). (b) Absorption coefficient babs,BrC of brown carbon (370 nm). (c) Fractional contribution of babs,BrC to babs. (d) Absorption Ångström exponent åabs from 370 to 430 nm. Local time is UTC minus 4 h.
Figure 12 illustrates connections between babs,BrC and the organic PM1 chemical composition. BrC light absorption decreases for increases in the O:C ratio (Fig. 12a). Conversely, light absorption increases for decreases in the concentration of nitrogen-containing species, as represented by the family (Fig. 12b). In addition, light absorption increases as the fractional contribution of the family to organic PM1 increases and that of the family decreases (Fig. S14). The diel trends of Fig. 11 and the O:C ratios of Fig. 12a support an association of BrC light absorption with HOA and LO-BBOA factor loadings. These factors had the lowest O:C values (Table 1), and they are associated with recent urban and biomass-burning emissions, which are typically important sources of brown carbon (Laskin et al., 2015, and references therein).
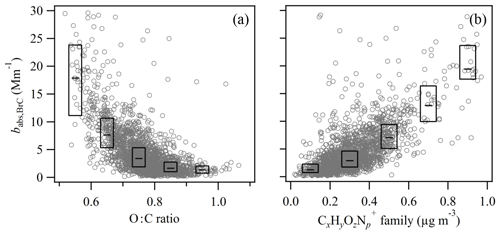
Figure 12Relationships between the BrC absorption coefficient and the organic PM1 composition. Scatter plots of babs,BrC against (a) the O:C ratio and (b) the mass concentration of the nitrogen-containing family. For the family, all ions contain at least one C atom and one N atom, meaning x>0, y≥0, z≥0, and p>0. Boxes indicate interquartile ranges, and horizontal lines within the boxes indicate medians. For panel (a), each bin width is 0.1, from 0.5 to 1.0, and for panel (b), each bin width is 0.2, from 0 to 1.0. In complement, Fig. S14 shows the relationships between the BrC absorption coefficient and the fractional contributions of the and families to organic PM1.
The decrease in babs,BrC as O:C increases suggests that the atmospheric processing of organic material bleaches the BrC components under the conditions of central Amazonia. This behavior has been observed in several laboratory studies: BrC species and thus their optical properties can be modified through atmospheric processing, which may involve reactions at the gas–particle interface, reactions in the aqueous phase of particle and cloud droplets, and photolysis driven by sunlight (Laskin et al., 2015; Zhao et al., 2015; Sumlin et al., 2017; Lee et al., 2014; Romonosky et al., 2015). In addition, Saleh et al. (2014) provided evidence that both primary and secondary material from biomass burning may absorb light, and that the secondary component may be less absorptive than the primary component in the visible spectral range. Lin et al. (2016) found that the absorbance at 300 nm by biomass-burning particles decayed with a half-life of approximately 16 h against photolysis under typical atmospheric conditions. Forrister et al. (2015) followed plumes from wildfires aboard an aircraft during the 2013 NASA SEAC4RS mission over the continental US and estimated a half-life of 9 to 15 h for the decay of BrC light absorption in the plumes.
An important contribution of nitrogen-containing organic molecules to babs,BrC is suggested by the relationship in Fig. 12b. The percent contribution of the family to each PMF factor profile is listed in Table 2 and is highest for the HOA and LO-BBOA factors. The correlations of factor loadings with the mass concentrations as well as with the babs,BrC values are highest for these two factors (R>0.8 and R>0.6, respectively) (Table 2). The correlations of the MO-BBOA factor loading with these two parameters are lower but still significant. By comparison, the corresponding correlations for the IEPOX-SOA, LO-OOA, and MO-OOA factor loadings are all lower than 0.5. These results further support that the HOA and LO-BBOA factors to a larger extent and the MO-BBOA factor to a lesser extent were tightly associated with nitrogen-containing, light-absorbing organic molecules.
Table 2Relationship of PMF factors to organonitrogen characteristics. Listed for each factor are the mean loading of the time series, the percent contribution of the family to the factor profile, and the mean mass concentration of the family (based on multiplication of columns 2 and 3), as well as the Pearson R correlation of factor loading against the mass concentration of , the mass concentration of organic nitrates, and babs,BrC. The family corresponds to the sum of all ions containing at least one C atom and one N atom, as measured by the AMS. Detailed family-colored spectra showing the nitrogen-containing ions for all PMF factors are presented in Fig. S6, and the most important ion fits are shown in Fig. S7. The AMS method characterizes organic nitrates through the NO+ and fragments, which remain distinct from the larger fragments of the family (Sect. S1 and discussion therein).
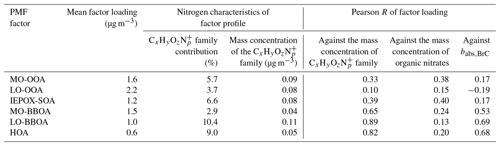
In contrast to the family, the correlations between PMF factor loadings and mass concentrations of organic nitrates are low (R<0.4, Table 2; Fig. S12). For the HOA, LO-OOA, and MO-OOA factors associated with BrC light absorption, the correlations are small (R<0.25). The implication could be that the family is closely tied to PM1 constituted by reduced nitrogen compounds and nitrogen–aromatic compounds. By comparison, organic nitrates are more strongly tied to photochemical production of secondary PM1 and represent more-oxidized forms of nitrogen, including in aliphatic molecules.
Several studies have suggested that nitrogen-containing organic molecules are important absorbers in organic PM (Sun et al., 2007; Lin et al., 2016). Claeys et al. (2012) characterized humic-like substances (HULIS) present in PM collected during the biomass-burning season in Amazonia and identified nitro-aromatic catechols and aromatic carboxylic acids among the main constituents. Nitrophenol derivatives have been identified as major BrC components in several other urban and rural locations worldwide (Kitanovski et al., 2012; Desyaterik et al., 2013; Kahnt et al., 2013; Mohr et al., 2013). Importantly, Lin et al. (2016) further verified that compounds that are usually interpreted as secondary, such as nitro-phenols and derivatives, can be produced in the heat-laden, NMVOC-rich, high-NOx conditions of the biomass-burning process, being subsequently emitted as primary material. Furthermore, Yee et al. (2013) observed the quick conversion of guaiacol and syringol to nitro-guaiacol and nitro-syringol, respectively, in the presence of HONO even without heat or photo-oxidation. It is possible that BrC from other combustion sources could have similar characteristics based on this reasoning, helping to explain the association found in this study between BrC absorption and the LO-BBOA and HOA factors. Regarding the further atmospheric processing of these nitrogen-containing organic compounds, laboratory studies have shown that hydroxy radical oxidation of nitro-aromatic species in aqueous solutions leads to fragmentation into smaller organic acids (e.g., oxalic, glycolic, malonic, and isocyanic) or, in general, reduces the size of the conjugated molecular systems, leading to a decrease in light absorption at visible wavelengths (Sumlin et al., 2017; Hems and Abbatt, 2018). These findings may help to explain the bleaching of BrC as the material becomes more oxidized. In the context of the PMF factors, these smaller later-generation products may then be associated with the MO-OOA factor or may partition to the gas phase depending on their volatility.
Scatter plots of åabs against markers of biomass burning are shown in Fig. 13. The Pearson R correlations against log 10(f60∕f44) and (BBOAT∕organic PM1) are 0.87 and 0.75, respectively. The f60∕f44 ratio is a tracer for the influence of fresh biomass burning, and an association of åabs and with this quantity was also reported for Boulder, Colorado, USA (Lack et al., 2013). These relationships could be useful parameterizations to estimate åabs when optical measurements are not available but AMS/ACSM measurements are, at least during times of biomass-burning influence in central Amazonia. Worldwide, observed values of åabs range from <2 to 11 for particles tied to biomass burning (Chakrabarty et al., 2010; Saleh et al., 2014). The value of åabs reached 6 for the highest observed values of (f60∕f44). It approached 1.0 in the limit of , which indicates little influence of proximate biomass burning (Fig. 13a; cf. upper left of Fig. 7a). Further observations elsewhere in the Amazon and on other regions are needed before the parameterizations suggested in Fig. 13 between åabs and markers of biomass burning can be generalized with confidence.
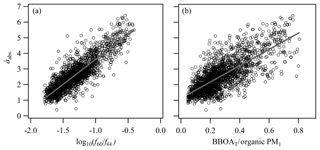
Figure 13Relationships between the absorption Ångström exponent and indicators of biomass burning. Scatter plots of åabs against (a) log 10(f60∕f44) of the AMS analysis (R=0.87), and (b) the ratio of the BBOAT loading to the organic PM1 mass concentration (R=0.75). BBOAT loading is the sum of the MO-BBOA and LO-BBOA factor loadings. The åabs value corresponds to 370 to 430 nm. In panel (a), the slope and intercept are 3.2±0.1 and 6.8±0.1, respectively. In panel (b), they are 5.2±0.1 and 1.1±0.1.
3.2.2 Contribution of organic PM components to BrC absorption
Herein, advantage is taken of the representation of the organic PM in its subcomponents provided by the PMF factors to estimate a mass absorption efficiency for each of them. The absorption coefficient is the sum of the absorption coefficient of the n parts of the organic PM (“Org”):
The treatment assumes the absence of cross-interactions among the parts and holds for a single wavelength. The absorption coefficient babs,i of part i is defined as follows:
where Eabs,i is the mass absorption efficiency and Ci is the mass concentration of part i. Based on Eqs. (3) and (4), the following model was constructed for babs,BrC by using the PMF factor loadings as a proxy for the mass concentrations of organic PM1 components:
where Gi correspond to loadings of factor i, and the unknowns are the mass absorption efficiencies Eabs,i associated with each PMF factor. An intercept B was added to account for the variability not explained by the PMF factors. Other studies have also made use of multivariate linear regression to retrieve mass absorption efficiencies (Hand and Malm, 2007; Washenfelder et al., 2015; Ealo et al., 2018).
Estimates of Eabs,i were obtained using a constrained linear least-squares algorithm applied to Eq. (5), where the inputs were the observed babs,BrC and the factor loadings for each point in time during IOP2. The input data are represented in the scatter plots of Gi against babs,BrC shown in Fig. 14a to f. A non-negative constraint on the model coefficients Eabs,i was included for physical meaning. The algorithm was applied in bootstrap with replacement of residuals for 104 runs, and convergence of the bootstrap results was checked by varying the number of samples. The resulting estimates of mean and standard error of Eabs for all PMF factors are listed in Table 3.
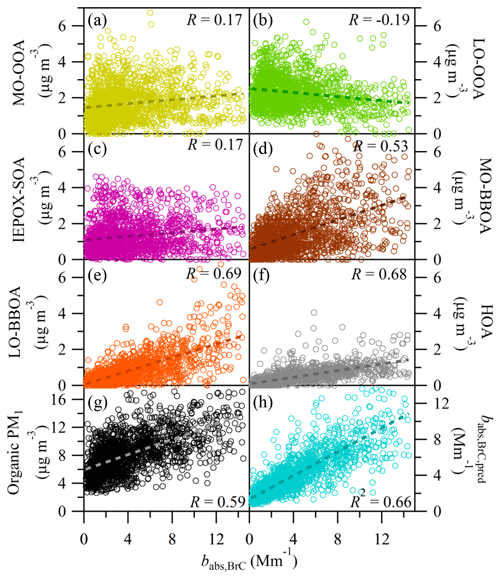
Figure 14Scatter plots against babs,BrC of (a)–(f) PMF factor loadings, (g) organic PM1 mass concentration, and (h) values predicted by a multivariate linear regression model using PMF factor loadings as parameters as described by Eq. (5).
Table 3Results of the constrained linear least-squares regression analysis for the BrC absorption coefficient (Eq. 5). (a) Mass absorption efficiency Eabs associated with each PMF factor. (b) Model intercept. The mean, standard error (SE), and 95 % confidence interval (CI) are listed in each panel. They were obtained through bootstrap of the regression analysis considering different samples (i.e., sets of points in time) for 104 runs. Unit of Mm−1 represents 10−6 m−1. The coefficient of determination R2 between predicted and observed babs,BrC was 0.66. The symbol “∗” indicates that the estimated value was statistically not higher than zero at the significance level of 5 %.
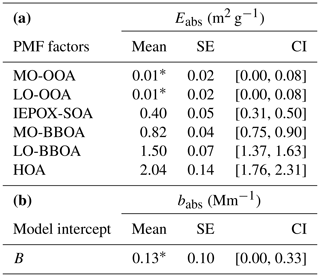
A scatter plot of the predicted against the observed babs,BrC is shown in Fig. 14h. The model captured 66 % of the variance in babs,BrC, and the PMF factor loadings can be considered good predictors of the BrC absorption under the study conditions. Physical factors not directly represented in this statistical model, such as the effects of mixing state, size distribution, and so on for BrC absorption, either have low variability under the study conditions or alternatively have co-variability also captured in the PMF factor loadings.
The highest values of Eabs at 370 nm were associated with the HOA and LO-BBOA factors (2.04±0.14 and 1.50±0.07 m2 g−1, respectively). These results support the interpretation presented in the previous section about the association of the HOA and LO-BBOA factors with light absorption. As a point of comparison, Ealo et al. (2018) conducted a study in the northwestern Mediterranean and found the highest mass absorption efficiencies, ranging from 0.9 to 1.7 m2 g−1 at 637 nm, for traffic and industrial sources. As another point of comparison, Eabs of 2 to 3 m2 g−1 at 300 nm was reported for HULIS extracts from PM2.5 filter samples collected under biomass-burning conditions during the Amazon dry season in Rondônia, Brazil (Hoffer et al., 2006). HULIS have been recognized as important components of BrC from biomass burning (Mukai and Ambe, 1986; Andreae and Gelencsér, 2006; Graber and Rudich, 2006). The Eabs value of the MO-BBOA factor was 0.82±0.04 m2 g−1. The result of Eabs,MO-BBOA<Eabs,LO-BBOA is consistent with an interpretation of photochemically driven oxidation and bleaching during the atmospheric transport of biomass-burning emissions.
The Eabs value of the IEPOX-SOA factor was 0.40±0.05 m2 g−1, and the Eabs values of the MO-OOA and LO-OOA factors (0.01±0.02 m2 g−1) were not statistically different from zero. Laboratory studies suggest that biogenic PM does not appreciably absorb light in the near-UV and visible range, although this result may change with atmospheric exposure to ammonia and amines, changes in particle acidity, and other factors (Nakayama et al., 2012; Liu et al., 2013; Flores et al., 2014; Lin et al., 2014; Laskin et al., 2015). Biogenic PM is typically characterized by carbonyls, carboxyls, and hydroxyls without substantial conjugation; this composition does not have the low-energy electronic transitions relevant for BrC light absorption (Laskin et al., 2015). By contrast, PM produced by the photo-oxidation of aromatic NMVOCs, such as toluene, m-xylene, naphthalene, and trimethylbenzene, tends to absorb significantly, and the light absorption is greater for PM produced under conditions of higher NOx concentrations because of the production of nitro-aromatic compounds (Zhong and Jang, 2011; Liu et al., 2012, 2015; Lee et al., 2014). This absorption, however, may decrease with atmospheric processing as previously discussed for the case of biomass-burning emissions, which is also reflected in the negligible value of Eabs for MO-OOA. In central Amazonia, the organic PM is dominated by biogenic forest precursors even in the pollution plume of Manaus, which helps to explain the negligible Eabs value for LO-OOA. It may also be that some aromatic PM is associated with the HOA factor, which has a high Eabs value.
A comparison of the relative contributions of PMF factor loadings to organic PM1 mass concentration and to light absorption is presented in Fig. 15 (a and b, respectively). The contribution of each class of organic compounds to total absorption by organic PM1 was estimated for each point in time by multiplication of the Eabs value and the loading of each PMF factor during IOP2. The means and standard deviations of the resulting percentage contributions are listed in Table 4. Biomass-burning and urban emissions, as represented by the BBOA and HOA factors, appeared to contribute 80 % of babs,BrC while accounting for at least 30 % of the organic PM1 mass concentration. The IEPOX-SOA factor was associated with the balance of babs,BrC while representing 16 % of the organic PM1 mass concentration. Studies with further information on black carbon size distribution, particle mixing state, and the effect of RH on particle absorption are warranted to refine the estimates of Eabs for the components of organic PM1 and therefore their contributions to BrC light absorption. A similar attribution analysis to the one in Fig. 15b was carried out for the baseline, event, and urban clusters separately and is discussed in the Supplement (Fig. S15).
Table 4Contribution of PM1 components as represented by the PMF factors to organic mass concentrations and BrC light absorption. The contribution of the model intercept to BrC light absorption is also included. Values listed are resulting means and standard deviations of the contributions calculated throughout IOP2. Small differences between the values in the second column and the values represented in the inset of Fig. 5a are due to differences in data coverage by the aethalometer and AMS.
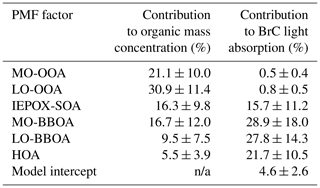
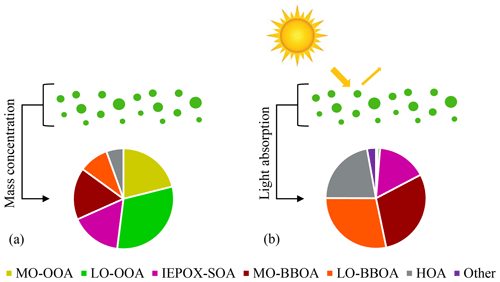
Figure 15Comparative relationship of the relative contributions of PMF factor loadings to (a) organic PM1 mass concentration and (b) organic PM1 light absorption. Results represent means for the full data sets of IOP2. The means and standard deviations are listed in Table 4. Results are for 370 nm. “Other” refers to the model intercept B (Eq. 5).
The BrC light absorption can have direct and indirect effects on radiative forcing, which ought to be further investigated for the Amazon region. The inclusion of BrC absorption in models may result in a positive direct radiative forcing (DRF) in regions of high BrC concentrations, in contrast to models that assume organic PM as a purely scattering component (Ramanathan and Carmichael, 2008; Myhre et al., 2013). Recent models have estimated the global BrC contribution to DRF to be in the range of 0.1 to 0.25 W m−2, corresponding to 10 % to 25 % of the DRF by BC (Feng et al., 2013). In addition, BrC in cloud water can absorb light and thereby facilitate water evaporation and cloud dispersion (Hansen et al., 1997). This effect may compensate the cooling that aerosol particles offer by serving as seeds for cloud droplet formation and may also provide a positive feedback as increased fire activity may provoke more fire-prone conditions by suppressing precipitation (Nepstad et al., 1999; Bevan et al., 2009; Gonçalves et al., 2015; Laskin et al., 2015). Another implication is that light absorption by BrC in the ultraviolet may significantly decrease photolysis rates, thereby affecting the concentrations of precursors and oxidants such as ozone and OH radicals in the atmosphere (Li et al., 2011; Jiang et al., 2012; Laskin et al., 2015).
The influence of urban and biomass-burning emissions on the otherwise natural concentrations, composition, and optical properties of organic PM1 in central Amazonia were investigated during the dry season. Positive-matrix factorization was used to classify the organic PM into subcomponents. The MO-OOA, LO-OOA, and IEPOX-SOA together accounted for about 62 % of the organic PM. The MO-BBOA and LO-BBOA factors together accounted for 31 %, and HOA for the remaining 7 %. An important conclusion is that the 8.5-fold increase in organic PM1 concentrations between the wet and dry seasons is not all due to biomass burning but also to a concurrent increase of biogenic secondary organic PM1 of 8-fold and smaller increases in urban PM1. Reasons that possibly played a role in such increases for the dry season are increased BVOC emissions, increased formation potential of biogenic secondary organic PM1, reduced wet and dry deposition and PBL ventilation of PM1 particles, and increased partitioning due to larger organic PM1 mass concentrations in the dry season.
The FCM clustering analysis identified the baseline, event, and urban clusters. Relative to the baseline cluster (9.2 µg m−3), both the event and the urban cluster had an increase of 3 µg m−3. For the event cluster, the increased sulfate concentrations together with only moderate increases in NOy, resulted in remarkable increases of almost 1 µg m−3 (65 %) in IEPOX-SOA factor loadings relative to the baseline cluster. Regarding the urban cluster, increases in the factor loadings of MO-BBOA (40 % to 90 %) and LO-OOA (20 % to 25 %) were observed in comparison to the other two clusters. At the same time, the IEPOX-SOA contribution was either the same or lower (by 40 %) in absolute loadings and always lower in relative contribution to organic PM (15 % of organic PM compared to 20 %–30 % for the other clusters). These changes in the makeup of organic PM were consistent with the changes observed for the wet season (de Sá et al., 2017, 2018). They were attributed partly to (i) a shift in oxidation pathways from HO2- to NO-dominant, and partly to (ii) an accelerated oxidation cycle that increases the mass concentration of secondary organic PM.
Optical properties of the PM1 were investigated, focusing on the organic component. The BrC absorption coefficient (babs,BrC) had an inverse relationship with the O:C ratio and a positive relationship with the family, indicating that BrC light in this region was associated with less-oxidized and N-containing organic compounds. The LO-BBOA and HOA factors had the lowest O:C ratios and highest relative contribution of family ions, suggesting that these factors represent BrC components. In addition, a tight relationship between åabs and log 10(f60∕f44) was found, corroborating the importance of BBOA factors for absorption properties of organic PM, and possibly providing a parameterization for åabs in the region. Further analysis determined the Eabs associated with each of the PMF factors. The results implied that the MO-OOA and LO-OOA factors were associated with non-absorbing components. The MO-BBOA (Eabs=0.8 m2 g−1), LO-BBOA (1.5 m2 g−1), and HOA (2.0 m2 g−1) factors were associated with 80 % of the light absorption by organic PM in the region. The remaining absorption (<10 %) was attributed to IEPOX-SOA (Eabs=0.4 m2 g−1).
Given the importance of biomass burning and the increasing importance of urban pollution in the Amazon forest, light absorption by atmospheric particulate matter could become more prevalent in this region in the future. Further field, laboratory, and modeling studies are warranted to (i) more finely map the importance of both urban and biomass-burning emissions at different locations in the Amazon region, (ii) characterize BrC components at the molecular level for structure–absorption relationships, and (iii) quantify the effects of BrC absorption on radiative forcing in the regional and global scales for current and future scenarios of increased human impacts.
The data sets used in this publication are available at the ARM Climate Research Facility database for the GoAmazon2014/5 experiment (https://www.arm.gov/research/campaigns/amf2014goamazon, last access: 1 August 2018).
The supplement related to this article is available online at: https://doi.org/10.5194/acp-19-7973-2019-supplement.
SSdS, LVR, and STM defined the scientific questions and scope of this study. STM, JLJ, MLA, AHG, and PA designed, planned, and supervised the broader GoAmazon2014/5 field experiment. SSdS, BBP, PCJ, and DAD carried out the AMS measurements and data processing. AS collected and quality checked the aethalometer data. LVR performed the BrC calculations based on the aethalometer data. LDY, RW, GYV, JB, SC, YJL, SS, and HMJB performed auxiliary data collection/processing and simulations. SSdS carried out the scientific analysis involving PMF and FCM. SSdS prepared the paper with contributions from all co-authors.
The authors declare that they have no conflict of interest.
This article is part of the special issue “Observations and Modeling of the Green Ocean Amazon (GoAmazon2014/5) (ACP/AMT/GI/GMD inter-journal SI)”. It is not associated with a conference.
Institutional support was provided by the central office of the Large-Scale Biosphere–Atmosphere Experiment in Amazonia (LBA), the National Institute of Amazonian Research (INPA), and Amazonas State University (UEA). Suzane S. de Sá acknowledges support by the Faculty for the Future Fellowship of the Schlumberger Foundation. Brett B. Palm acknowledges a US EPA STAR graduate fellowship (FP-91761701-0). This paper has not been reviewed by EPA and no endorsement should be inferred. Data access from the Sistema de Proteção da Amazônia (SIPAM) is gratefully acknowledged. The research was conducted under scientific license 001030/2012-4 of the Brazilian National Council for Scientific and Technological Development (CNPq).
This research has been supported by the Atmospheric Radiation Measurement (ARM) Climate Research Facility, a user facility of the United States Department of Energy (DOE, DE-SC0006680), Office of Science, sponsored by the Office of Biological and Environmental Research, and support from the Atmospheric System Research (ASR, DE-SC0011115, DE-SC0011105) program of that office. Additional funding was provided by the Amazonas State Research Foundation (FAPEAM 062.00568/2014 and FAPEAM 134/2016), the São Paulo State Research Foundation (grant nos. FAPESP 2013/05014-0, FAPESP 2017/17047-0, FAPESP 2013/50510-5, and FAPESP 2013/10156-8), the US National Science Foundation (grant nos. 1106400 and 1332998), and the Brazilian Scientific Mobility Program (CsF/CAPES). Brett B. Palm, Pedro Campuzano-Jost, Douglas A. Day, and Jose L. Jimenez were supported by DOE (BER/ASR (grant no. DE-SC0016559) and NSF (grant no. AGS-1822664)).
This paper was edited by Thomas Karl and reviewed by two anonymous referees.
Alves, E. G., Jardine, K., Tota, J., Jardine, A., Yãnez-Serrano, A. M., Karl, T., Tavares, J., Nelson, B., Gu, D., Stavrakou, T., Martin, S., Artaxo, P., Manzi, A., and Guenther, A.: Seasonality of isoprenoid emissions from a primary rainforest in central Amazonia, Atmos. Chem. Phys., 16, 3903–3925, https://doi.org/10.5194/acp-16-3903-2016, 2016.
Ammerlaan, B. A. J., Holzinger, R., Jedynska, A. D., and Henzing, J. S.: Aerosol light absorption measurements with a carbon analyser – Calibration and precision estimates, Atmos. Environ., 164, 1–7, https://doi.org/10.1016/j.atmosenv.2017.05.031, 2017.
Andreae, M. O. and Gelencsér, A.: Black carbon or brown carbon? The nature of light-absorbing carbonaceous aerosols, Atmos. Chem. Phys., 6, 3131–3148, https://doi.org/10.5194/acp-6-3131-2006, 2006.
Andreae, M. O. and Merlet, P.: Emission of trace gases and aerosols from biomass burning, Global Biogeochem. Cy., 15, 955–966, https://doi.org/10.1029/2000GB001382, 2001.
Andreae, M. O., Berresheim, H., Bingemer, H., Jacob, D. J., Lewis, B. L., Li, S. M., and Talbot, R. W.: The atmospheric sulfur cycle over the Amazon Basin: 2. Wet season, J. Geophys. Res.-Atmos., 95, 16813–16824, https://doi.org/10.1029/JD095iD10p16813, 1990.
Andreae, M. O., Artaxo, P., Brandão, C., Carswell, F. E., Ciccioli, P., da Costa, A. L., Culf, A. D., Esteves, J. L., Gash, J. H. C., Grace, J., Kabat, P., Lelieveld, J., Malhi, Y., Manzi, A. O., Meixner, F. X., Nobre, A. D., Nobre, C., Ruivo, M. d. L. P., Silva-Dias, M. A., Stefani, P., Valentini, R., von Jouanne, J., and Waterloo, M. J.: Biogeochemical cycling of carbon, water, energy, trace gases, and aerosols in Amazonia: The LBA-EUSTACH experiments, J. Geophys. Res.-Atmos., 107, LBA 33-31–LBA 33-25, https://doi.org/10.1029/2001JD000524, 2002.
Andreae, M. O., Rosenfeld, D., Artaxo, P., Costa, A. A., Frank, G. P., Longo, K. M., and Silva-Dias, M. A. F.: Smoking rain clouds over the Amazon, Science, 303, 1337–1342, https://doi.org/10.1126/science.1092779, 2004.
Andreae, M. O., Acevedo, O. C., Araùjo, A., Artaxo, P., Barbosa, C. G. G., Barbosa, H. M. J., Brito, J., Carbone, S., Chi, X., Cintra, B. B. L., da Silva, N. F., Dias, N. L., Dias-Júnior, C. Q., Ditas, F., Ditz, R., Godoi, A. F. L., Godoi, R. H. M., Heimann, M., Hoffmann, T., Kesselmeier, J., Könemann, T., Krüger, M. L., Lavric, J. V., Manzi, A. O., Lopes, A. P., Martins, D. L., Mikhailov, E. F., Moran-Zuloaga, D., Nelson, B. W., Nölscher, A. C., Santos Nogueira, D., Piedade, M. T. F., Pöhlker, C., Pöschl, U., Quesada, C. A., Rizzo, L. V., Ro, C.-U., Ruckteschler, N., Sá, L. D. A., de Oliveira Sá, M., Sales, C. B., dos Santos, R. M. N., Saturno, J., Schöngart, J., Sörgel, M., de Souza, C. M., de Souza, R. A. F., Su, H., Targhetta, N., Tóta, J., Trebs, I., Trumbore, S., van Eijck, A., Walter, D., Wang, Z., Weber, B., Williams, J., Winderlich, J., Wittmann, F., Wolff, S., and Yáñez-Serrano, A. M.: The Amazon Tall Tower Observatory (ATTO): overview of pilot measurements on ecosystem ecology, meteorology, trace gases, and aerosols, Atmos. Chem. Phys., 15, 10723–10776, https://doi.org/10.5194/acp-15-10723-2015, 2015.
Aragão, L. E. O. C., Poulter, B., Barlow, J. B., Anderson, L. O., Malhi, Y., Saatchi, S., Phillips, O. L., and Gloor, E.: Environmental change and the carbon balance of Amazonian forests, Biol. Rev., 89, 913–931, https://doi.org/10.1111/brv.12088, 2014.
Artaxo, P., Gerab, F., Yamasoe, M. A., and Martins, J. V.: Fine mode aerosol composition at three long-term atmospheric monitoring sites in the Amazon Basin, J. Geophys. Res.-Atmos., 99, 22857–22868, https://doi.org/10.1029/94JD01023, 1994.
Artaxo, P., Martins, J. V., Yamasoe, M. A., Procópio, A. S., Pauliquevis, T. M., Andreae, M. O., Guyon, P., Gatti, L. V., and Leal, A. M. C.: Physical and chemical properties of aerosols in the wet and dry seasons in Rondônia, Amazonia, J. Geophys. Res.-Atmos., 107, LBA 49-41–LBA 49-14, https://doi.org/10.1029/2001JD000666, 2002.
Artaxo, P., Rizzo, L. V., Brito, J. F., Barbosa, H. M. J., Arana, A., Sena, E. T., Cirino, G. G., Bastos, W., Martin, S. T., and Andreae, M. O.: Atmospheric aerosols in Amazonia and land use change: from natural biogenic to biomass burning conditions, Faraday Discuss., 165, 203–235, https://doi.org/10.1039/C3FD00052D, 2013.
Bahadur, R., Praveen, P. S., Xu, Y., and Ramanathan, V.: Solar absorption by elemental and brown carbon determined from spectral observations, P. Natl. Acad. Sci. USA, 109, 17366–17371, https://doi.org/10.1073/pnas.1205910109, 2012.
Bevan, S., L., North, P. R. J., Grey, W. M. F., Los Sietse, O., and Plummer, S. E.: Impact of atmospheric aerosol from biomass burning on Amazon dry-season drought, J. Geophys. Res.-Atmos., 114, D09204, https://doi.org/10.1029/2008JD011112, 2009.
Bond, T. C., Zarzycki, C., Flanner, M. G., and Koch, D. M.: Quantifying immediate radiative forcing by black carbon and organic matter with the Specific Forcing Pulse, Atmos. Chem. Phys., 11, 1505–1525, https://doi.org/10.5194/acp-11-1505-2011, 2011.
Brito, J., Rizzo, L. V., Morgan, W. T., Coe, H., Johnson, B., Haywood, J., Longo, K., Freitas, S., Andreae, M. O., and Artaxo, P.: Ground-based aerosol characterization during the South American Biomass Burning Analysis (SAMBBA) field experiment, Atmos. Chem. Phys., 14, 12069–12083, https://doi.org/10.5194/acp-14-12069-2014, 2014.
Canagaratna, M. R., Jimenez, J. L., Kroll, J. H., Chen, Q., Kessler, S. H., Massoli, P., Hildebrandt Ruiz, L., Fortner, E., Williams, L. R., Wilson, K. R., Surratt, J. D., Donahue, N. M., Jayne, J. T., and Worsnop, D. R.: Elemental ratio measurements of organic compounds using aerosol mass spectrometry: characterization, improved calibration, and implications, Atmos. Chem. Phys., 15, 253–272, https://doi.org/10.5194/acp-15-253-2015, 2015.
Chakrabarty, R. K., Moosmüller, H., Chen, L.-W. A., Lewis, K., Arnott, W. P., Mazzoleni, C., Dubey, M. K., Wold, C. E., Hao, W. M., and Kreidenweis, S. M.: Brown carbon in tar balls from smoldering biomass combustion, Atmos. Chem. Phys., 10, 6363–6370, https://doi.org/10.5194/acp-10-6363-2010, 2010.
Chakraborty, S., Schiro, K. A., Fu, R., and Neelin, J. D.: On the role of aerosols, humidity, and vertical wind shear in the transition of shallow-to-deep convection at the Green Ocean Amazon 2014/5 site, Atmos. Chem. Phys., 18, 11135–11148, https://doi.org/10.5194/acp-18-11135-2018, 2018.
Chen, Q., Farmer, D. K., Schneider, J., Zorn, S. R., Heald, C. L., Karl, T. G., Guenther, A., Allan, J. D., Robinson, N., Coe, H., Kimmel, J. R., Pauliquevis, T., Borrmann, S., Pöschl, U., Andreae, M. O., Artaxo, P., Jimenez, J. L., and Martin, S. T.: Mass spectral characterization of submicron biogenic organic particles in the Amazon Basin, Geophys. Res. Lett., 36, L20806, https://doi.org/10.1029/2009GL039880, 2009.
Cirino, G. G., Brito, J., Barbosa, H. M. J., Rizzo, L. V., Tunved, P., de Sá, S. S., Jimenez, J. L., Palm, B. B., Carbone, S., Lavric, J., Souza, R. A. F., Wolff, S., Walter, D., Tota, J., Oliveira, M. B. L., Martin, S. T., and Artaxo, P.: Observations of Manaus urban plume evolution and interaction with biogenic emissions in GoAmazon 2014/5, Atmos. Environ., 191, 513–524, https://doi.org/10.1016/j.atmosenv.2018.08.031, 2018.
Claeys, M., Vermeylen, R., Yasmeen, F., Gómez-González, Y., Chi, X., Maenhaut, W., Mészáros, T., and Salma, I.: Chemical characterisation of humic-like substances from urban, rural and tropical biomass burning environments using liquid chromatography with UV/vis photodiode array detection and electrospray ionisation mass spectrometry, Environ. Chem., 9, 3, 273–284, https://doi.org/10.1071/EN11163, 2012.
Collaud Coen, M., Weingartner, E., Apituley, A., Ceburnis, D., Fierz-Schmidhauser, R., Flentje, H., Henzing, J. S., Jennings, S. G., Moerman, M., Petzold, A., Schmid, O., and Baltensperger, U.: Minimizing light absorption measurement artifacts of the Aethalometer: evaluation of five correction algorithms, Atmos. Meas. Tech., 3, 457–474, https://doi.org/10.5194/amt-3-457-2010, 2010.
Collier, S., Zhou, S., Onasch, T. B., Jaffe, D. A., Kleinman, L., Sedlacek, A. J., Briggs, N. L., Hee, J., Fortner, E., Shilling, J. E., Worsnop, D., Yokelson, R. J., Parworth, C., Ge, X., Xu, J., Butterfield, Z., Chand, D., Dubey, M. K., Pekour, M. S., Springston, S., and Zhang, Q.: Regional influence of aerosol emissions from wildfires driven by combustion efficiency: insights from the BBOP campaign, Environ. Sci. Technol., 50, 8613–8622, https://doi.org/10.1021/acs.est.6b01617, 2016.
Crutzen, P. J. and Andreae, M. O.: Biomass burning in the tropics: impact on atmospheric chemistry and biogeochemical cycles, Science, 250, 1669–1678, https://doi.org/10.1126/science.250.4988.1669, 1990.
Cubison, M. J., Ortega, A. M., Hayes, P. L., Farmer, D. K., Day, D., Lechner, M. J., Brune, W. H., Apel, E., Diskin, G. S., Fisher, J. A., Fuelberg, H. E., Hecobian, A., Knapp, D. J., Mikoviny, T., Riemer, D., Sachse, G. W., Sessions, W., Weber, R. J., Weinheimer, A. J., Wisthaler, A., and Jimenez, J. L.: Effects of aging on organic aerosol from open biomass burning smoke in aircraft and laboratory studies, Atmos. Chem. Phys., 11, 12049–12064, https://doi.org/10.5194/acp-11-12049-2011, 2011.
Davidson, E. A., de Araújo, A. C., Artaxo, P., Balch, J. K., Brown, I. F., Bustamante, M. M. C., Coe, M. T., DeFries, R. S., Keller, M., and Longo, M.: The Amazon basin in transition, Nature, 481, 321–328, https://doi.org/10.1038/nature10717, 2012.
DeCarlo, P. F., Kimmel, J. R., Trimborn, A., Northway, M. J., Jayne, J. T., Aiken, A. C., Gonin, M., Fuhrer, K., Horvath, T., Docherty, K. S., Worsnop, D. R., and Jimenez, J. L.: Field-deployable, high-resolution, time-of-flight aerosol mass spectrometer, Anal. Chem., 78, 8281–8289, https://doi.org/10.1021/ac061249n, 2006.
De Haan, D. O., Corrigan, A. L., Tolbert, M. A., Jimenez, J. L., Wood, S. E., and Turley, J. J.: Secondary organic aerosol formation by self-reactions of methylglyoxal and glyoxal in evaporating droplets, Environ. Sci. Technol., 43, 8184–8190, https://doi.org/10.1021/es902152t, 2009.
de Sá, S. S., Palm, B. B., Campuzano-Jost, P., Day, D. A., Newburn, M. K., Hu, W., Isaacman-VanWertz, G., Yee, L. D., Thalman, R., Brito, J., Carbone, S., Artaxo, P., Goldstein, A. H., Manzi, A. O., Souza, R. A. F., Mei, F., Shilling, J. E., Springston, S. R., Wang, J., Surratt, J. D., Alexander, M. L., Jimenez, J. L., and Martin, S. T.: Influence of urban pollution on the production of organic particulate matter from isoprene epoxydiols in central Amazonia, Atmos. Chem. Phys., 17, 6611–6629, https://doi.org/10.5194/acp-17-6611-2017, 2017.
de Sá, S. S., Palm, B. B., Campuzano-Jost, P., Day, D. A., Hu, W., Isaacman-VanWertz, G., Yee, L. D., Brito, J., Carbone, S., Ribeiro, I. O., Cirino, G. G., Liu, Y., Thalman, R., Sedlacek, A., Funk, A., Schumacher, C., Shilling, J. E., Schneider, J., Artaxo, P., Goldstein, A. H., Souza, R. A. F., Wang, J., McKinney, K. A., Barbosa, H., Alexander, M. L., Jimenez, J. L., and Martin, S. T.: Urban influence on the concentration and composition of submicron particulate matter in central Amazonia, Atmos. Chem. Phys., 18, 12185–12206, https://doi.org/10.5194/acp-18-12185-2018, 2018.
Desyaterik, Y., Sun, Y., Shen, X., Lee, T., Wang, X., Wang, T., and Collett, J. L.: Speciation of “brown” carbon in cloud water impacted by agricultural biomass burning in eastern China, J. Geophys. Res.-Atmos., 118, 7389–7399, https://doi.org/10.1002/jgrd.50561, 2013.
Draxler, R. and Hess, G.: An overview of the HYSPLIT_4 modeling system for trajectories, dispersion, and deposition, Aust. Meteorol. Mag., 47, 295–308, https://doi.org/10.5194/acp-13-8607-2013, 1998.
Ealo, M., Alastuey, A., Pérez, N., Ripoll, A., Querol, X., and Pandolfi, M.: Impact of aerosol particle sources on optical properties in urban, regional and remote areas in the north-western Mediterranean, Atmos. Chem. Phys., 18, 1149–1169, https://doi.org/10.5194/acp-18-1149-2018, 2018.
Echalar, F., Artaxo, P., Martins, J. V., Yamasoe, M., Gerab, F., Maenhaut, W., and Holben, B.: Long-term monitoring of atmospheric aerosols in the Amazon Basin: Source identification and apportionment, J. Geophys. Res.-Atmos., 103, 31849–31864, https://doi.org/10.1029/98JD01749, 1998.
Farmer, D. K., Matsunaga, A., Docherty, K. S., Surratt, J. D., Seinfeld, J. H., Ziemann, P. J., and Jimenez, J. L.: Response of an aerosol mass spectrometer to organonitrates and organosulfates and implications for atmospheric chemistry, P. Natl. Acad. Sci. USA, 107, 6670–6675, https://doi.org/10.1073/pnas.0912340107, 2010.
Feng, Y., Ramanathan, V., and Kotamarthi, V. R.: Brown carbon: a significant atmospheric absorber of solar radiation?, Atmos. Chem. Phys., 13, 8607–8621, https://doi.org/10.5194/acp-13-8607-2013, 2013.
Fiedler, V., Arnold, F., Ludmann, S., Minikin, A., Hamburger, T., Pirjola, L., Dörnbrack, A., and Schlager, H.: African biomass burning plumes over the Atlantic: aircraft based measurements and implications for H2SO4 and HNO3 mediated smoke particle activation, Atmos. Chem. Phys., 11, 3211–3225, https://doi.org/10.5194/acp-11-3211-2011, 2011.
Flores, J. M., Washenfelder, R., Adler, G., Lee, H., Segev, L., Laskin, J., Laskin, A., Nizkorodov, S., Brown, S., and Rudich, Y.: Complex refractive indices in the near-ultraviolet spectral region of biogenic secondary organic aerosol aged with ammonia, Phys. Chem. Chem. Phys., 16, 10629–10642, https://doi.org/10.1039/C4CP01009D, 2014.
Forrister, H., Liu, J., Scheuer, E., Dibb, J., Ziemba, L., Thornhill, K. L., Anderson, B., Diskin, G., Perring, A. E., Schwarz, J. P., Campuzano-Jost, P., Day, D. A., Palm, B. B., Jimenez, J. L., Nenes, A., and Weber, R. J.: Evolution of brown carbon in wildfire plumes, Geophys. Res. Lett., 42, 4623–4630, https://doi.org/10.1002/2015GL063897, 2015.
Fry, J. L., Kiendler-Scharr, A., Rollins, A. W., Wooldridge, P. J., Brown, S. S., Fuchs, H., Dubé, W., Mensah, A., dal Maso, M., Tillmann, R., Dorn, H.-P., Brauers, T., and Cohen, R. C.: Organic nitrate and secondary organic aerosol yield from NO3 oxidation of β-pinene evaluated using a gas-phase kinetics/aerosol partitioning model, Atmos. Chem. Phys., 9, 1431–1449, https://doi.org/10.5194/acp-9-1431-2009, 2009.
Fry, J. L., Draper, D. C., Zarzana, K. J., Campuzano-Jost, P., Day, D. A., Jimenez, J. L., Brown, S. S., Cohen, R. C., Kaser, L., Hansel, A., Cappellin, L., Karl, T., Hodzic Roux, A., Turnipseed, A., Cantrell, C., Lefer, B. L., and Grossberg, N.: Observations of gas- and aerosol-phase organic nitrates at BEACHON-RoMBAS 2011, Atmos. Chem. Phys., 13, 8585–8605, https://doi.org/10.5194/acp-13-8585-2013, 2013.
Fuentes, J. D., Chamecki, M., Santos, R. M. N. d., Randow, C. V., Stoy, P. C., Katul, G., Fitzjarrald, D., Manzi, A., Gerken, T., Trowbridge, A., Freire, L. S., Ruiz-Plancarte, J., Maia, J. M. F., Tóta, J., Dias, N., Fisch, G., Schumacher, C., Acevedo, O., Mercer, J. R., and Yañez-Serrano, A. M.: Linking meteorology, turbulence, and air chemistry in the Amazon rain forest, B. Am. Meteorol. Soc., 97, 2329–2342, https://doi.org/10.1175/bams-d-15-00152.1, 2016.
Fuzzi, S., Decesari, S., Facchini, M. C., Cavalli, F., Emblico, L., Mircea, M., Andreae, M. O., Trebs, I., Hoffer, A. s., Guyon, P., Artaxo, P., Rizzo, L. V., Lara, L. L., Pauliquevis, T., Maenhaut, W., Raes, N., Chi, X., Mayol-Bracero, O. L., Soto-García, L. L., Claeys, M., Kourtchev, I., Rissler, J., Swietlicki, E., Tagliavini, E., Schkolnik, G., Falkovich, A. H., Rudich, Y., Fisch, G., and Gatti, L. V.: Overview of the inorganic and organic composition of size-segregated aerosol in Rondonia, Brazil, from the biomass-burning period to the onset of the wet season, J. Geophys. Res.-Atmos., 112, D01201, https://doi.org/10.1029/2005JD006741, 2007.
Gilardoni, S., Massoli, P., Paglione, M., Giulianelli, L., Carbone, C., Rinaldi, M., Decesari, S., Sandrini, S., Costabile, F., Gobbi, G. P., Pietrogrande, M. C., Visentin, M., Scotto, F., Fuzzi, S., and Facchini, M. C.: Direct observation of aqueous secondary organic aerosol from biomass-burning emissions, P. Natl. Acad. Sci. USA, 113, 10013–10018, https://doi.org/10.1073/pnas.1602212113, 2016.
Glasius, M., Bering, M. S., Yee, L. D., de Sá, S. S., Isaacman-VanWertz, G., Wernis, R. A., Barbosa, H. M. J., Alexander, M. L., Palm, B. B., Hu, W., Campuzano-Jost, P., Day, D. A., Jimenez, J. L., Shrivastava, M., Martin, S. T., and Goldstein, A. H.: Organosulfates in aerosols downwind of an urban region in central Amazon, Environ. Sci.-Proc. Imp., 20, 1546–1558, https://doi.org/10.1039/C8EM00413G, 2018.
Gonçalves, W. A., Machado, L. A. T., and Kirstetter, P.-E.: Influence of biomass aerosol on precipitation over the Central Amazon: an observational study, Atmos. Chem. Phys., 15, 6789–6800, https://doi.org/10.5194/acp-15-6789-2015, 2015.
Graber, E. R. and Rudich, Y.: Atmospheric HULIS: How humic-like are they? A comprehensive and critical review, Atmos. Chem. Phys., 6, 729–753, https://doi.org/10.5194/acp-6-729-2006, 2006.
Hand, J. L. and Malm, W. C.: Review of aerosol mass scattering efficiencies from ground-based measurements since 1990, J. Geophys. Res.-Atmos., 112, D16203, https://doi.org/10.1029/2007JD008484, 2007.
Hansen, J., Sato, M., and Ruedy, R.: Radiative forcing and climate response, J. Geophys. Res.-Atmos., 102, 6831–6864, https://doi.org/10.1029/96JD03436, 1997.
Hems, R. F. and Abbatt, J. P. D.: Aqueous phase photo-oxidation of brown carbon nitrophenols: reaction kinetics, mechanism, and evolution of light absorption, ACS Earth Space Chem., 2, 225–234, https://doi.org/10.1021/acsearthspacechem.7b00123, 2018.
Hoffer, A., Gelencsér, A., Guyon, P., Kiss, G., Schmid, O., Frank, G. P., Artaxo, P., and Andreae, M. O.: Optical properties of humic-like substances (HULIS) in biomass-burning aerosols, Atmos. Chem. Phys., 6, 3563–3570, https://doi.org/10.5194/acp-6-3563-2006, 2006.
Holben, B. N., Setzer, A., Eck, T. F., Pereira, A., and Slutsker, I.: Effect of dry-season biomass burning on Amazon basin aerosol concentrations and optical properties, 1992–1994, J. Geophys. Res.-Atmos., 101, 19465–19481, https://doi.org/10.1029/96JD01114, 1996.
Hu, W., Palm, B. B., Day, D. A., Campuzano-Jost, P., Krechmer, J. E., Peng, Z., de Sá, S. S., Martin, S. T., Alexander, M. L., Baumann, K., Hacker, L., Kiendler-Scharr, A., Koss, A. R., de Gouw, J. A., Goldstein, A. H., Seco, R., Sjostedt, S. J., Park, J.-H., Guenther, A. B., Kim, S., Canonaco, F., Prévôt, A. S. H., Brune, W. H., and Jimenez, J. L.: Volatility and lifetime against OH heterogeneous reaction of ambient isoprene-epoxydiols-derived secondary organic aerosol (IEPOX-SOA), Atmos. Chem. Phys., 16, 11563–11580, https://doi.org/10.5194/acp-16-11563-2016, 2016.
INPE: Instituto Nacional de Pesquisas Espaciais: Banco de dados de queimadas, available at: http://www.inpe.br/queimadas/bdqueimadas/, last access: 1 February 2018.
IPCC: Summary for policymakers, in: Climate Change 2013 – The Physical Science Basis. Contribution of Working Group I to the Fifth Assessment Report of the Intergovernmental Panel on Climate Change, edited by: Stocker, T. F., Qin, D., Plattner, G.-K., Tignor, M., Allen, S. K., Boschung, J., Nauels, A., Xia, Y., Bex, V., and Midgley, P. M., Cambridge University Press, Cambridge, UK, and New York, NY, USA, Cambridge, 2013.
Isaacman-VanWertz, G., Yee, L. D., Kreisberg, N. M., Wernis, R., Moss, J. A., Hering, S. V., de Sá, S. S., Martin, S. T., Alexander, M. L., Palm, B. B., Hu, W., Campuzano-Jost, P., Day, D. A., Jimenez, J. L., Riva, M., Surratt, J. D., Viegas, J., Manzi, A., Edgerton, E., Baumann, K., Souza, R., Artaxo, P., and Goldstein, A. H.: Ambient gas-particle partitioning of tracers for biogenic oxidation, Environ. Sci. Technol., 50, 9952–9962, https://doi.org/10.1021/acs.est.6b01674, 2016.
Jen, C. N., Hatch, L. E., Selimovic, V., Yokelson, R. J., Weber, R., Fernandez, A. E., Kreisberg, N. M., Barsanti, K. C., and Goldstein, A. H.: Speciated and total emission factors of particulate organics from burning western US wildland fuels and their dependence on combustion efficiency, Atmos. Chem. Phys., 19, 1013–1026, https://doi.org/10.5194/acp-19-1013-2019, 2019.
Jiang, X., Wiedinmyer, C., and Carlton, A. G.: Aerosols from fires: an examination of the effects on ozone photochemistry in the western United States, Environ. Sci. Technol., 46, 11878–11886, https://doi.org/10.1021/es301541k, 2012.
Jimenez, J. L., Canagaratna, M. R., Donahue, N. M., Prevot, A. S. H., Zhang, Q., Kroll, J. H., DeCarlo, P. F., Allan, J. D., Coe, H., Ng, N. L., Aiken, A. C., Docherty, K. S., Ulbrich, I. M., Grieshop, A. P., Robinson, A. L., Duplissy, J., Smith, J. D., Wilson, K. R., Lanz, V. A., Hueglin, C., Sun, Y. L., Tian, J., Laaksonen, A., Raatikainen, T., Rautiainen, J., Vaattovaara, P., Ehn, M., Kulmala, M., Tomlinson, J. M., Collins, D. R., Cubison, M. J., Dunlea, J., Huffman, J. A., Onasch, T. B., Alfarra, M. R., Williams, P. I., Bower, K., Kondo, Y., Schneider, J., Drewnick, F., Borrmann, S., Weimer, S., Demerjian, K., Salcedo, D., Cottrell, L., Griffin, R., Takami, A., Miyoshi, T., Hatakeyama, S., Shimono, A., Sun, J. Y., Zhang, Y. M., Dzepina, K., Kimmel, J. R., Sueper, D., Jayne, J. T., Herndon, S. C., Trimborn, A. M., Williams, L. R., Wood, E. C., Middlebrook, A. M., Kolb, C. E., Baltensperger, U., and Worsnop, D. R.: Evolution of organic aerosols in the atmosphere, Science, 326, 1525–1529, https://doi.org/10.1126/science.1180353, 2009.
Jordan, A., Haidacher, S., Hanel, G., Hartungen, E., Märk, L., Seehauser, H., Schottkowsky, R., Sulzer, P., and Märk, T. D.: A high resolution and high sensitivity proton-transfer-reaction time-of-flight mass spectrometer (PTR-TOF-MS), Int. J. Mass Spectrom., 286, 122–128, https://doi.org/10.1016/j.ijms.2009.07.005, 2009.
Kahnt, A., Behrouzi, S., Vermeylen, R., Safi Shalamzari, M., Vercauteren, J., Roekens, E., Claeys, M., and Maenhaut, W.: One-year study of nitro-organic compounds and their relation to wood burning in PM10 aerosol from a rural site in Belgium, Atmos. Environ., 81, 561–568, https://doi.org/10.1016/j.atmosenv.2013.09.041, 2013.
Kaufman, Y. J., Hobbs, P. V., Kirchhoff, V. W. J. H., Artaxo, P., Remer, L. A., Holben, B. N., King, M. D., Ward, D. E., Prins, E. M., Longo, K. M., Mattos, L. F., Nobre, C. A., Spinhirne, J. D., Ji, Q., Thompson, A. M., Gleason, J. F., Christopher, S. A., and Tsay, S. C.: Smoke, Clouds, and Radiation-Brazil (SCAR-B) experiment, J. Geophys. Res.-Atmos., 103, 31783–31808, https://doi.org/10.1029/98JD02281, 1998.
Kitanovski, Z., Grgić, I., Vermeylen, R., Claeys, M., and Maenhaut, W.: Liquid chromatography tandem mass spectrometry method for characterization of monoaromatic nitro-compounds in atmospheric particulate matter, J. Chromatogr. A, 1268, 35–43, https://doi.org/10.1016/j.chroma.2012.10.021, 2012.
Knote, C., Hodzic, A., and Jimenez, J. L.: The effect of dry and wet deposition of condensable vapors on secondary organic aerosols concentrations over the continental US, Atmos. Chem. Phys., 15, 1–18, https://doi.org/10.5194/acp-15-1-2015, 2015.
Kuhn, U., Ganzeveld, L., Thielmann, A., Dindorf, T., Schebeske, G., Welling, M., Sciare, J., Roberts, G., Meixner, F. X., Kesselmeier, J., Lelieveld, J., Kolle, O., Ciccioli, P., Lloyd, J., Trentmann, J., Artaxo, P., and Andreae, M. O.: Impact of Manaus City on the Amazon Green Ocean atmosphere: ozone production, precursor sensitivity and aerosol load, Atmos. Chem. Phys., 10, 9251–9282, https://doi.org/10.5194/acp-10-9251-2010, 2010.
Lack, D. A., Bahreini, R., Langridge, J. M., Gilman, J. B., and Middlebrook, A. M.: Brown carbon absorption linked to organic mass tracers in biomass burning particles, Atmos. Chem. Phys., 13, 2415–2422, https://doi.org/10.5194/acp-13-2415-2013, 2013.
Laskin, A., Laskin, J., and Nizkorodov, S. A.: Chemistry of atmospheric brown carbon, Chem. Rev., 115, 4335–4382, https://doi.org/10.1021/cr5006167, 2015.
Lee, A. K. Y., Zhao, R., Li, R., Liggio, J., Li, S.-M., and Abbatt, J. P. D.: Formation of light absorbing organo-nitrogen species from evaporation of droplets containing glyoxal and ammonium sulfate, Environ. Sci. Technol., 47, 12819–12826, https://doi.org/10.1021/es402687w, 2013.
Lee, H. J., Aiona, P. K., Laskin, A., Laskin, J., and Nizkorodov, S. A.: Effect of solar radiation on the optical properties and molecular composition of laboratory proxies of atmospheric brown carbon, Environ. Sci. Technol., 48, 10217–10226, https://doi.org/10.1021/es502515r, 2014.
Li, G., Bei, N., Tie, X., and Molina, L. T.: Aerosol effects on the photochemistry in Mexico City during MCMA-2006/MILAGRO campaign, Atmos. Chem. Phys., 11, 5169–5182, https://doi.org/10.5194/acp-11-5169-2011, 2011.
Lin, J. C., Matsui, T., Pielke, R. A., and Kummerow, C.: Effects of biomass-burning-derived aerosols on precipitation and clouds in the Amazon Basin: a satellite-based empirical study, J. Geophys. Res.-Atmos., 111, D19204, https://doi.org/10.1029/2005JD006884, 2006.
Lin, P., Aiona, P. K., Li, Y., Shiraiwa, M., Laskin, J., Nizkorodov, S. A., and Laskin, A.: Molecular characterization of brown carbon in biomass burning aerosol particles, Environ. Sci. Technol., 50, 11815–11824, https://doi.org/10.1021/acs.est.6b03024, 2016.
Lin, Y.-H., Budisulistiorini, S. H., Chu, K., Siejack, R. A., Zhang, H., Riva, M., Zhang, Z., Gold, A., Kautzman, K. E., and Surratt, J. D.: Light-absorbing oligomer formation in secondary organic aerosol from reactive uptake of isoprene epoxydiols, Environ. Sci. Technol., 48, 12012–12021, https://doi.org/10.1021/es503142b, 2014.
Liu, P., Zhang, Y., and Martin, S. T.: Complex refractive indices of thin films of secondary organic materials by spectroscopic ellipsometry from 220 to 1200 nm, Environ. Sci. Technol., 47, 13594–13601, https://doi.org/10.1021/es403411e, 2013.
Liu, P. F., Abdelmalki, N., Hung, H.-M., Wang, Y., Brune, W. H., and Martin, S. T.: Ultraviolet and visible complex refractive indices of secondary organic material produced by photooxidation of the aromatic compounds toluene and m-xylene, Atmos. Chem. Phys., 15, 1435–1446, https://doi.org/10.5194/acp-15-1435-2015, 2015.
Liu, S., Shilling, J. E., Song, C., Hiranuma, N., Zaveri, R. A., and Russell, L. M.: Hydrolysis of organonitrate functional groups in aerosol particles, Aerosol Sci. Tech., 46, 1359–1369, https://doi.org/10.1080/02786826.2012.716175, 2012.
Liu, Y., Brito, J., Dorris, M. R., Rivera-Rios, J. C., Seco, R., Bates, K. H., Artaxo, P., Duvoisin, S., Keutsch, F. N., Kim, S., Goldstein, A. H., Guenther, A. B., Manzi, A. O., Souza, R. A. F., Springston, S. R., Watson, T. B., McKinney, K. A., and Martin, S. T.: Isoprene photochemistry over the Amazon rain forest, P. Natl. Acad. Sci. USA, 113, 6125–6130, https://doi.org/10.1073/pnas.1524136113, 2016.
Ma, L. and Thompson, J. E.: Optical properties of dispersed aerosols in the near ultraviolet (355 nm): measurement approach and initial data, Anal. Chem., 84, 5611–5617, https://doi.org/10.1021/ac3005814, 2012.
Mace, K. A., Artaxo, P., and Duce, R. A.: Water-soluble organic nitrogen in Amazon Basin aerosols during the dry (biomass burning) and wet seasons, J. Geophys. Res.-Atmos., 108, 4512, https://doi.org/10.1029/2003JD003557, 2003.
Machado, L. A. T., Laurent, H., Dessay, N., and Miranda, I.: Seasonal and diurnal variability of convection over the Amazonia: a comparison of different vegetation types and large scale forcing, Theor. App. Climatol., 78, 61–77, https://doi.org/10.1007/s00704-004-0044-9, 2004.
Machado, L. A. T., Dias, M. A. F. S., Morales, C., Fisch, G., Vila, D., Albrecht, R., Goodman, S. J., Calheiros, A. J. P., Biscaro, T., Kummerow, C., Cohen, J., Fitzjarrald, D., Nascimento, E. L., Sakamoto, M. S., Cunningham, C., Chaboureau, J.-P., Petersen, W. A., Adams, D. K., Baldini, L., Angelis, C. F., Sapucci, L. F., Salio, P., Barbosa, H. M. J., Landulfo, E., Souza, R. A. F., Blakeslee, R. J., Bailey, J., Freitas, S., Lima, W. F. A., and Tokay, A.: The Chuva Project: how does convection vary across Brazil?, B. Am. Meteorol. Soc., 95, 1365–1380, https://doi.org/10.1175/bams-d-13-00084.1, 2014.
Maenhaut, W., Fernández-Jiménez, M. T., and Artaxo, P.: Long-term study of atmospheric aerosols in Cuiabá, Brazil: multielemental composition, sources and source apportionment, J. Aerosol Sci., 30, S259–S260, https://doi.org/10.1016/S0021-8502(99)80141-4, 1999.
Martin, S. T., Andreae, M. O., Artaxo, P., Baumgardner, D., Chen, Q., Goldstein, A. H., Guenther, A., Heald, C. L., Mayol-Bracero, O. L., McMurry, P. H., Pauliquevis, T., Pöschl, U., Prather, K. A., Roberts, G. C., Saleska, S. R., Dias, M. A. S., Spracklen, D. V., Swietlicki, E., and Trebs, I.: Sources and properties of Amazonian aerosol particles, Rev. Geophys., 48, RG2002, https://doi.org/10.1029/2008RG000280, 2010a.
Martin, S. T., Andreae, M. O., Althausen, D., Artaxo, P., Baars, H., Borrmann, S., Chen, Q., Farmer, D. K., Guenther, A., Gunthe, S. S., Jimenez, J. L., Karl, T., Longo, K., Manzi, A., Müller, T., Pauliquevis, T., Petters, M. D., Prenni, A. J., Pöschl, U., Rizzo, L. V., Schneider, J., Smith, J. N., Swietlicki, E., Tota, J., Wang, J., Wiedensohler, A., and Zorn, S. R.: An overview of the Amazonian Aerosol Characterization Experiment 2008 (AMAZE-08), Atmos. Chem. Phys., 10, 11415–11438, https://doi.org/10.5194/acp-10-11415-2010, 2010b.
Martin, S. T., Artaxo, P., Machado, L. A. T., Manzi, A. O., Souza, R. A. F., Schumacher, C., Wang, J., Andreae, M. O., Barbosa, H. M. J., Fan, J., Fisch, G., Goldstein, A. H., Guenther, A., Jimenez, J. L., Pöschl, U., Silva Dias, M. A., Smith, J. N., and Wendisch, M.: Introduction: Observations and Modeling of the Green Ocean Amazon (GoAmazon2014/5), Atmos. Chem. Phys., 16, 4785–4797, https://doi.org/10.5194/acp-16-4785-2016, 2016.
Martin, S. T., Artaxo, P., Machado, L., Manzi, A. O., Souza, R. A. F., Schumacher, C., Wang, J., Biscaro, T., Brito, J., Calheiros, A., Jardine, K., Medeiros, A., Portela, B., Sá, S. S. d., Adachi, K., Aiken, A. C., Albrecht, R., Alexander, L., Andreae, M. O., Barbosa, H. M. J., Buseck, P., Chand, D., Comstock, J. M., Day, D. A., Dubey, M., Fan, J., Fast, J., Fisch, G., Fortner, E., Giangrande, S., Gilles, M., Goldstein, A. H., Guenther, A., Hubbe, J., Jensen, M., Jimenez, J. L., Keutsch, F. N., Kim, S., Kuang, C., Laskin, A., McKinney, K., Mei, F., Miller, M., Nascimento, R., Pauliquevis, T., Pekour, M., Peres, J., Petäjä, T., Pöhlker, C., Pöschl, U., Rizzo, L., Schmid, B., Shilling, J. E., Dias, M. A. S., Smith, J. N., Tomlinson, J. M., Tóta, J., and Wendisch, M.: The Green Ocean Amazon Experiment (GoAmazon2014/5) observes pollution affecting gases, aerosols, clouds, and rainfall over the rain forest, B. Am. Meteorol. Soc., 98, 981–997, https://doi.org/10.1175/bams-d-15-00221.1, 2017.
Middlebrook, A. M., Bahreini, R., Jimenez, J. L., and Canagaratna, M. R.: Evaluation of composition-dependent collection efficiencies for the aerodyne aerosol mass spectrometer using field data, Aerosol Sci. Tech., 46, 258–271, https://doi.org/10.1080/02786826.2011.620041, 2012.
Mohr, C., Lopez-Hilfiker, F. D., Zotter, P., Prévôt, A. S. H., Xu, L., Ng, N. L., Herndon, S. C., Williams, L. R., Franklin, J. P., Zahniser, M. S., Worsnop, D. R., Knighton, W. B., Aiken, A. C., Gorkowski, K. J., Dubey, M. K., Allan, J. D., and Thornton, J. A.: Contribution of nitrated phenols to wood burning brown carbon light absorption in Detling, United Kingdom during Winter time, Environ. Sci. Technol., 47, 6316–6324, https://doi.org/10.1021/es400683v, 2013.
Moosmüller, H., Chakrabarty, R. K., and Arnott, W. P.: Aerosol light absorption and its measurement: A review, J. Quant. Spectrosc. Ra., 110, 844–878, https://doi.org/10.1016/j.jqsrt.2009.02.035, 2009.
Morgan, W. T., Allan, J. D., Flynn, M., Darbyshire, E., Hodgson, A., Johnson, B. T., Haywood, J. M., Freitas, S., Longo, K., Artaxo, P., and Coe, H.: Overview of the South American biomass burning analysis (SAMBBA) field experiment, AIP Conf. Proc., 1527, 587–590, https://doi.org/10.1063/1.4803339, 2013.
Mukai, H. and Ambe, Y.: Characterization of a humic acid-like brown substance in airborne particulate matter and tentative identification of its origin, Atmos. Environ., 20, 813–819, https://doi.org/10.1016/0004-6981(86)90265-9, 1986.
Myhre, G., Samset, B. H., Schulz, M., Balkanski, Y., Bauer, S., Berntsen, T. K., Bian, H., Bellouin, N., Chin, M., Diehl, T., Easter, R. C., Feichter, J., Ghan, S. J., Hauglustaine, D., Iversen, T., Kinne, S., Kirkevåg, A., Lamarque, J.-F., Lin, G., Liu, X., Lund, M. T., Luo, G., Ma, X., van Noije, T., Penner, J. E., Rasch, P. J., Ruiz, A., Seland, Ø., Skeie, R. B., Stier, P., Takemura, T., Tsigaridis, K., Wang, P., Wang, Z., Xu, L., Yu, H., Yu, F., Yoon, J.-H., Zhang, K., Zhang, H., and Zhou, C.: Radiative forcing of the direct aerosol effect from AeroCom Phase II simulations, Atmos. Chem. Phys., 13, 1853–1877, https://doi.org/10.5194/acp-13-1853-2013, 2013.
Nakayama, T., Kondo, Y., Moteki, N., Sahu, L. K., Kinase, T., Kita, K., and Matsumi, Y.: Size-dependent correction factors for absorption measurements using filter-based photometers: PSAP and COSMOS, J. Aerosol Sci., 41, 333–343, https://doi.org/10.1016/j.jaerosci.2010.01.004, 2010.
Nakayama, T., Sato, K., Matsumi, Y., Imamura, T., Yamazaki, A., and Uchiyama, A.: Wavelength dependence of refractive index of secondary organic aerosols generated during the ozonolysis and photooxidation of α-pinene, SOLA, 8, 119–123, https://doi.org/10.2151/sola.2012-030, 2012.
Nepstad, D. C., Verssimo, A., Alencar, A., Nobre, C., Lima, E., Lefebvre, P., Schlesinger, P., Potter, C., Moutinho, P., Mendoza, E., Cochrane, M., and Brooks, V.: Large-scale impoverishment of Amazonian forests by logging and fire, Nature, 398, 505–508, https://doi.org/10.1038/19066, 1999.
Ng, N. L., Herndon, S. C., Trimborn, A., Canagaratna, M. R., Croteau, P. L., Onasch, T. B., Sueper, D., Worsnop, D. R., Zhang, Q., Sun, Y. L., and Jayne, J. T.: An Aerosol Chemical Speciation Monitor (ACSM) for routine monitoring of the composition and mass concentrations of ambient aerosol, Aerosol Sci. Tech., 45, 780–794, https://doi.org/10.1080/02786826.2011.560211, 2011.
Nguyen, T. B., Lee, P. B., Updyke, K. M., Bones, D. L., Laskin, J., Laskin, A., and Nizkorodov, S. A.: Formation of nitrogen- and sulfur-containing light-absorbing compounds accelerated by evaporation of water from secondary organic aerosols, J. Geophys. Res.-Atmos., 117, D01207, https://doi.org/10.1029/2011JD016944, 2012.
Nozière, B., Dziedzic, P., and Córdova, A.: Formation of secondary light-absorbing “fulvic-like” oligomers: A common process in aqueous and ionic atmospheric particles?, Geophys. Res. Lett., 34, L21812, https://doi.org/10.1029/2007GL031300, 2007.
Nunes, A. M. P., Silva Dias, M. A. F., Anselmo, E. M., and Morales, C. A.: Severe Convection Features in the Amazon Basin: A TRMM-Based 15-Year Evaluation, Front. Earth Sci., 4, 37, https://doi.org/10.3389/feart.2016.00037, 2016.
Palm, B. B., de Sá, S. S., Day, D. A., Campuzano-Jost, P., Hu, W., Seco, R., Sjostedt, S. J., Park, J.-H., Guenther, A. B., Kim, S., Brito, J., Wurm, F., Artaxo, P., Thalman, R., Wang, J., Yee, L. D., Wernis, R., Isaacman-VanWertz, G., Goldstein, A. H., Liu, Y., Springston, S. R., Souza, R., Newburn, M. K., Alexander, M. L., Martin, S. T., and Jimenez, J. L.: Secondary organic aerosol formation from ambient air in an oxidation flow reactor in central Amazonia, Atmos. Chem. Phys., 18, 467–493, https://doi.org/10.5194/acp-18-467-2018, 2018.
Pöhlker, M. L., Pöhlker, C., Ditas, F., Klimach, T., Hrabe de Angelis, I., Araújo, A., Brito, J., Carbone, S., Cheng, Y., Chi, X., Ditz, R., Gunthe, S. S., Kesselmeier, J., Könemann, T., Lavriç, J. V., Martin, S. T., Mikhailov, E., Moran-Zuloaga, D., Rose, D., Saturno, J., Su, H., Thalman, R., Walter, D., Wang, J., Wolff, S., Barbosa, H. M. J., Artaxo, P., Andreae, M. O., and Pöschl, U.: Long-term observations of cloud condensation nuclei in the Amazon rain forest – Part 1: Aerosol size distribution, hygroscopicity, and new model parametrizations for CCN prediction, Atmos. Chem. Phys., 16, 15709–15740, https://doi.org/10.5194/acp-16-15709-2016, 2016.
Pöschl, U.: Aerosol particle analysis: challenges and progress, Anal. Bioanal. Chem., 375, 30–32, https://doi.org/10.1007/s00216-002-1611-5, 2003.
Powelson, M. H., Espelien, B. M., Hawkins, L. N., Galloway, M. M., and De Haan, D. O.: Brown carbon formation by aqueous-phase carbonyl compound reactions with amines and ammonium sulfate, Environ. Sci. Technol., 48, 985–993, https://doi.org/10.1021/es4038325, 2014.
Ramanathan, V. and Carmichael, G.: Global and regional climate changes due to black carbon, Nat. Geosci., 1, 221, https://doi.org/10.1038/ngeo156, 2008.
Ramanathan, V., Li, F., Ramana, M. V., Praveen, P. S., Kim, D., Corrigan, C. E., Nguyen, H., Stone, E. A., Schauer, J. J., Carmichael, G. R., Adhikary, B., and Yoon, S. C.: Atmospheric brown clouds: Hemispherical and regional variations in long-range transport, absorption, and radiative forcing, J. Geophys. Res.-Atmos., 112, D22S21, https://doi.org/10.1029/2006JD008124, 2007.
Rizzo, L. V., Correia, A. L., Artaxo, P., Procópio, A. S., and Andreae, M. O.: Spectral dependence of aerosol light absorption over the Amazon Basin, Atmos. Chem. Phys., 11, 8899–8912, https://doi.org/10.5194/acp-11-8899-2011, 2011.
Rizzo, L. V., Artaxo, P., Müller, T., Wiedensohler, A., Paixão, M., Cirino, G. G., Arana, A., Swietlicki, E., Roldin, P., Fors, E. O., Wiedemann, K. T., Leal, L. S. M., and Kulmala, M.: Long term measurements of aerosol optical properties at a primary forest site in Amazonia, Atmos. Chem. Phys., 13, 2391–2413, https://doi.org/10.5194/acp-13-2391-2013, 2013.
Romonosky, D. E., Laskin, A., Laskin, J., and Nizkorodov, S. A.: High-resolution mass spectrometry and molecular characterization of aqueous photochemistry products of common types of secondary organic aerosols, J. Phys. Chem. A, 119, 2594–2606, https://doi.org/10.1021/jp509476r, 2015.
Rummel, U., Ammann, C., Kirkman, G. A., Moura, M. A. L., Foken, T., Andreae, M. O., and Meixner, F. X.: Seasonal variation of ozone deposition to a tropical rain forest in southwest Amazonia, Atmos. Chem. Phys., 7, 5415–5435, https://doi.org/10.5194/acp-7-5415-2007, 2007.
Saleh, R., Robinson, E. S., Tkacik, D. S., Ahern, A. T., Liu, S., Aiken, A. C., Sullivan, R. C., Presto, A. A., Dubey, M. K., Yokelson, R. J., Donahue, N. M., and Robinson, A. L.: Brownness of organics in aerosols from biomass burning linked to their black carbon content, Nat. Geosci., 7, 647, https://doi.org/10.1038/ngeo2220, 2014.
Saturno, J., Pöhlker, C., Massabò, D., Brito, J., Carbone, S., Cheng, Y., Chi, X., Ditas, F., Hrabě de Angelis, I., Morán-Zuloaga, D., Pöhlker, M. L., Rizzo, L. V., Walter, D., Wang, Q., Artaxo, P., Prati, P., and Andreae, M. O.: Comparison of different Aethalometer correction schemes and a reference multi-wavelength absorption technique for ambient aerosol data, Atmos. Meas. Tech., 10, 2837–2850, https://doi.org/10.5194/amt-10-2837-2017, 2017.
Saturno, J., Holanda, B. A., Pöhlker, C., Ditas, F., Wang, Q., Moran-Zuloaga, D., Brito, J., Carbone, S., Cheng, Y., Chi, X., Ditas, J., Hoffmann, T., Hrabe de Angelis, I., Könemann, T., Lavrič, J. V., Ma, N., Ming, J., Paulsen, H., Pöhlker, M. L., Rizzo, L. V., Schlag, P., Su, H., Walter, D., Wolff, S., Zhang, Y., Artaxo, P., Pöschl, U., and Andreae, M. O.: Black and brown carbon over central Amazonia: long-term aerosol measurements at the ATTO site, Atmos. Chem. Phys., 18, 12817–12843, https://doi.org/10.5194/acp-18-12817-2018, 2018a.
Saturno, J., Ditas, F., Penning de Vries, M., Holanda, B. A., Pöhlker, M. L., Carbone, S., Walter, D., Bobrowski, N., Brito, J., Chi, X., Gutmann, A., Hrabe de Angelis, I., Machado, L. A. T., Moran-Zuloaga, D., Rüdiger, J., Schneider, J., Schulz, C., Wang, Q., Wendisch, M., Artaxo, P., Wagner, T., Pöschl, U., Andreae, M. O., and Pöhlker, C.: African volcanic emissions influencing atmospheric aerosols over the Amazon rain forest, Atmos. Chem. Phys., 18, 10391–10405, https://doi.org/10.5194/acp-18-10391-2018, 2018b.
Schmid, O., Artaxo, P., Arnott, W. P., Chand, D., Gatti, L. V., Frank, G. P., Hoffer, A., Schnaiter, M., and Andreae, M. O.: Spectral light absorption by ambient aerosols influenced by biomass burning in the Amazon Basin. I: Comparison and field calibration of absorption measurement techniques, Atmos. Chem. Phys., 6, 3443–3462, https://doi.org/10.5194/acp-6-3443-2006, 2006.
Schneider, J., Weimer, S., Drewnick, F., Borrmann, S., Helas, G., Gwaze, P., Schmid, O., Andreae, M. O., and Kirchner, U.: Mass spectrometric analysis and aerodynamic properties of various types of combustion-related aerosol particles, Int. J. Mass Spectrom., 258, 37–49, https://doi.org/10.1016/j.ijms.2006.07.008, 2006.
Sena, E. T., Artaxo, P., and Correia, A. L.: Spatial variability of the direct radiative forcing of biomass burning aerosols and the effects of land use change in Amazonia, Atmos. Chem. Phys., 13, 1261–1275, https://doi.org/10.5194/acp-13-1261-2013, 2013.
Setzer, A. W. and Pereira, M. C.: Amazonia biomass burnings in 1987 and an estimate of their tropospheric emissions, Ambio, 20, 19–22, 1991.
Sueper, D.: ToF-AMS Data Analysis Software Webpage, available at: http://cires1.colorado.edu/jimenez-group/wiki/index.php/ToF-AMS_Analysis_Software, last access: August 2018.
Sumlin, B. J., Pandey, A., Walker, M. J., Pattison, R. S., Williams, B. J., and Chakrabarty, R. K.: Atmospheric Photooxidation Diminishes Light Absorption by Primary Brown Carbon Aerosol from Biomass Burning, Environ. Sci. Tech. Let., 4, 540–545, https://doi.org/10.1021/acs.estlett.7b00393, 2017.
Sun, H., Biedermann, L., and Bond, T. C.: Color of brown carbon: A model for ultraviolet and visible light absorption by organic carbon aerosol, Geophys. Res. Lett., 34, L17813, https://doi.org/10.1029/2007GL029797, 2007.
Thalman, R., de Sá, S. S., Palm, B. B., Barbosa, H. M. J., Pöhlker, M. L., Alexander, M. L., Brito, J., Carbone, S., Castillo, P., Day, D. A., Kuang, C., Manzi, A., Ng, N. L., Sedlacek III, A. J., Souza, R., Springston, S., Watson, T., Pöhlker, C., Pöschl, U., Andreae, M. O., Artaxo, P., Jimenez, J. L., Martin, S. T., and Wang, J.: CCN activity and organic hygroscopicity of aerosols downwind of an urban region in central Amazonia: seasonal and diel variations and impact of anthropogenic emissions, Atmos. Chem. Phys., 17, 11779–11801, https://doi.org/10.5194/acp-17-11779-2017, 2017.
Ulbrich, I. M., Canagaratna, M. R., Zhang, Q., Worsnop, D. R., and Jimenez, J. L.: Interpretation of organic components from Positive Matrix Factorization of aerosol mass spectrometric data, Atmos. Chem. Phys., 9, 2891–2918, https://doi.org/10.5194/acp-9-2891-2009, 2009.
van Marle, M. J. E., Field, R. D., Werf, G. R., Estrada de Wagt, I. A., Houghton, R. A., Rizzo, L. V., Artaxo, P., and Tsigaridis, K.: Fire and deforestation dynamics in Amazonia (1973–2014), Global Biogeochem. Cy., 31, 24–38, https://doi.org/10.1002/2016GB005445, 2017.
Wang, X., Heald, C. L., Sedlacek, A. J., de Sá, S. S., Martin, S. T., Alexander, M. L., Watson, T. B., Aiken, A. C., Springston, S. R., and Artaxo, P.: Deriving brown carbon from multiwavelength absorption measurements: method and application to AERONET and Aethalometer observations, Atmos. Chem. Phys., 16, 12733–12752, https://doi.org/10.5194/acp-16-12733-2016, 2016.
Washenfelder, R. A., Attwood, A. R., Brock, C. A., Guo, H., Xu, L., Weber, R. J., Ng, N., Allen, H. M., Ayres, B. R., Karsten, B., Cohen, R. C., Draper, D. C., Duffey, K. C., Edgerton, E., Fry, J. L., Hu, W., Jimenez, J. L., Palm, B. B., Romer, P., and Brown, S.: Biomass burning dominates brown carbon absorption in the rural southeastern United States, Geophys. Res. Lett., 42, 653–664, https://doi.org/10.1002/2014GL062444, 2015.
Weingartner, E., Saathoff, H., Schnaiter, M., Streit, N., Bitnar, B., and Baltensperger, U.: Absorption of light by soot particles: determination of the absorption coefficient by means of aethalometers, J. Aerosol Sci., 34, 1445–1463, https://doi.org/10.1016/S0021-8502(03)00359-8, 2003.
Yáñez-Serrano, A. M., Nölscher, A. C., Williams, J., Wolff, S., Alves, E., Martins, G. A., Bourtsoukidis, E., Brito, J., Jardine, K., Artaxo, P., and Kesselmeier, J.: Diel and seasonal changes of biogenic volatile organic compounds within and above an Amazonian rainforest, Atmos. Chem. Phys., 15, 3359–3378, https://doi.org/10.5194/acp-15-3359-2015, 2015.
Yang, M., Howell, S. G., Zhuang, J., and Huebert, B. J.: Attribution of aerosol light absorption to black carbon, brown carbon, and dust in China – interpretations of atmospheric measurements during EAST-AIRE, Atmos. Chem. Phys., 9, 2035–2050, https://doi.org/10.5194/acp-9-2035-2009, 2009.
Yee, L. D., Kautzman, K. E., Loza, C. L., Schilling, K. A., Coggon, M. M., Chhabra, P. S., Chan, M. N., Chan, A. W. H., Hersey, S. P., Crounse, J. D., Wennberg, P. O., Flagan, R. C., and Seinfeld, J. H.: Secondary organic aerosol formation from biomass burning intermediates: phenol and methoxyphenols, Atmos. Chem. Phys., 13, 8019–8043, https://doi.org/10.5194/acp-13-8019-2013, 2013.
Yee, L. D., Isaacman-VanWertz, G., Wernis, R. A., Meng, M., Rivera, V., Kreisberg, N. M., Hering, S. V., Bering, M. S., Glasius, M., Upshur, M. A., Gray Bé, A., Thomson, R. J., Geiger, F. M., Offenberg, J. H., Lewandowski, M., Kourtchev, I., Kalberer, M., de Sá, S., Martin, S. T., Alexander, M. L., Palm, B. B., Hu, W., Campuzano-Jost, P., Day, D. A., Jimenez, J. L., Liu, Y., McKinney, K. A., Artaxo, P., Viegas, J., Manzi, A., Oliveira, M. B., de Souza, R., Machado, L. A. T., Longo, K., and Goldstein, A. H.: Observations of sesquiterpenes and their oxidation products in central Amazonia during the wet and dry seasons, Atmos. Chem. Phys., 18, 10433–10457, https://doi.org/10.5194/acp-18-10433-2018, 2018.
Yokelson, R. J., Karl, T., Artaxo, P., Blake, D. R., Christian, T. J., Griffith, D. W. T., Guenther, A., and Hao, W. M.: The Tropical Forest and Fire Emissions Experiment: overview and airborne fire emission factor measurements, Atmos. Chem. Phys., 7, 5175–5196, https://doi.org/10.5194/acp-7-5175-2007, 2007.
Zhao, R., Lee, A. K. Y., Huang, L., Li, X., Yang, F., and Abbatt, J. P. D.: Photochemical processing of aqueous atmospheric brown carbon, Atmos. Chem. Phys., 15, 6087–6100, https://doi.org/10.5194/acp-15-6087-2015, 2015.
Zhong, M. and Jang, M.: Light absorption coefficient measurement of SOA using a UV–Visible spectrometer connected with an integrating sphere, Atmos. Environ., 45, 4263–4271, https://doi.org/10.1016/j.atmosenv.2011.04.082, 2011.
Zhou, S., Collier, S., Jaffe, D. A., Briggs, N. L., Hee, J., Sedlacek III, A. J., Kleinman, L., Onasch, T. B., and Zhang, Q.: Regional influence of wildfires on aerosol chemistry in the western US and insights into atmospheric aging of biomass burning organic aerosol, Atmos. Chem. Phys., 17, 2477–2493, https://doi.org/10.5194/acp-17-2477-2017, 2017.