the Creative Commons Attribution 4.0 License.
the Creative Commons Attribution 4.0 License.
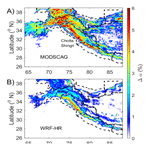
Impact of light-absorbing particles on snow albedo darkening and associated radiative forcing over high-mountain Asia: high-resolution WRF-Chem modeling and new satellite observations
Chandan Sarangi
Yun Qian
Karl Rittger
Kathryn J. Bormann
Ying Liu
Hailong Wang
Guangxing Lin
Thomas H. Painter
Light-absorbing particles (LAPs), mainly dust and black carbon, can significantly impact snowmelt and regional water availability over high-mountain Asia (HMA). In this study, for the first time, online aerosol–snow interactions are enabled and a fully coupled chemistry Weather Research and Forecasting (WRF-Chem) regional model is used to simulate LAP-induced radiative forcing on snow surfaces in HMA at relatively high spatial resolution (12 km, WRF-HR) compared with previous studies. Simulated macro- and microphysical properties of the snowpack and LAP-induced snow darkening are evaluated against new spatially and temporally complete datasets of snow-covered area, grain size, and impurity-induced albedo reduction over HMA. A WRF-Chem quasi-global simulation with the same configuration as WRF-HR but a coarser spatial resolution (1∘, WRF-CR) is also used to illustrate the impact of spatial resolution on simulations of snow properties and aerosol distribution over HMA. Due to a more realistic representation of terrain slopes over HMA, the higher-resolution model (WRF-HR) shows significantly better performance in simulating snow area cover, duration of snow cover, snow albedo and snow grain size over HMA, as well as an evidently better atmospheric aerosol loading and mean LAP concentration in snow. However, the differences in albedo reduction from model and satellite retrievals is large during winter due to associated overestimation in simulated snow fraction. It is noteworthy that Himalayan snow cover has high magnitudes of LAP-induced snow albedo reduction (4 %–8 %) in pre-monsoon seasons (both from WRF-HR and satellite estimates), which induces a snow-mediated radiative forcing of ∼30–50 W m−2. As a result, the Himalayas (specifically the western Himalayas) hold the most vulnerable glaciers and mountain snowpack to the LAP-induced snow darkening effect within HMA. In summary, coarse spatial resolution and absence of snow–aerosol interactions over the Himalayan cryosphere will result in significant underestimation of aerosol effects on snow melting and regional hydroclimate.
- Article
(7956 KB) -
Supplement
(2394 KB) - BibTeX
- EndNote
Light-absorbing aerosol particles (LAPs; airborne dust and black carbon (BC) specks), can impact regional water availability over Asia in three ways. Firstly, LAPs can directly interact with incoming solar radiation and induce thermodynamical modifications to synoptic-scale circulations (Hansen et al., 1997; Ramanathan et al., 2001; Bond et al., 2013; Lau et al., 2006; Bollasina et al., 2011; Li et al., 2016). Secondly, acting as cloud condensation nuclei, changes in concentrations of these particles can lead to microphysical modification of cloud systems and precipitations (Fan et al., 2016; Li et al., 2016; Qian et al., 2009; Sarangi et al., 2017). Finally, deposition of LAPs in the snowpack can also darken the snow, reduce its surface albedo, and accelerate snow warming and melting (Warren and Wiscombe, 1980; Qian et al., 2015, 2011, 2009; Lau et al., 2010; B. Xu et al., 2009; Hadley and Kirchstetter, 2012; Dang et al., 2017). Modeling studies have suggested that the LAP-induced snow darkening mechanism has warming and snow-melting efficacy even greater than that of greenhouse gases (GHGs) (Hansen and Nazarenko, 2004; Flanner et al., 2007; Qian et al., 2011; Skiles et al., 2012). To give perspective, the concentration of just 100 ng of BC in 1 g of snowpack will reduce the visible-wavelength albedo of a grain radius of 1000 µm by 10 % (Fig. 1b of Warren, 2013). A chain of positive feedback mechanisms results in such a large impact of LAPs (Qian et al., 2015). Initially, as snow starts to melt, the concentration of LAPs in snowpack increases because a portion of LAPs accumulate at the surface of the snowpack instead of getting washed away with meltwater (Conway et al., 1996; Flanner et al., 2007; Doherty et al., 2010). This increase in LAP concentration leads to enhanced warming of the snowpack and thereby increases the effective snow grain size, which further lowers snow albedo (Warren and Wiscombe, 1980; Hadley and Kirchstetter, 2012). Nonetheless, at higher concentrations, grain sizes can again be reduced due to the loss of mass from surface layers with the intense melting (Painter et al., 2013). As this process continues, sufficient snowmelt occurs to expose the darker underlying surface, leading to enhanced warming and snow ablation commonly known as “snow–albedo feedback” (Warren and Wiscombe, 1980; Hansen and Nazarenko, 2004; Flanner et al., 2007; Qian et al., 2015). In turn, this earlier loss of snow cover induces surface warming and perturbing regional circulations (Hansen and Nazarenko, 2004; Lau et al., 2010; Qian et al., 2011). This LAP-induced modification of snow–albedo feedback is identified as one of major forcing agents affecting climate change with a high level of uncertainty (IPCC, 2013).
High-mountain Asia (HMA) includes the Tibetan Plateau, central Asian mountains and the Himalayan cryosphere. It holds the largest glacial cover (∼9500 glaciers) outside the polar region (Dyurgerov, 2001). Observations revealed a historical decadal increase in the surface air temperature over HMA in range of 0.6–1.8 ∘C (Shrestha et al., 1999; Wang et al., 2008), and the warming is faster over higher elevations (>4000 m) in the last 3 decades (J. Xu et al., 2009; Ghatak et al., 2014). The Himalayan glacier area has cumulatively decreased by ∼16 % during the period from 1962 to 2004 (Kulkarni et al., 2010) and the pre-monsoon snow cover has been decreasing at a decadal rate of ∼0.8 million km2 for the last 50 years (Brown and Robinson, 2011). The average retreat rate on the north slope of Mount Everest is as high as 5.5–9.5 m yr−1 (Ren et al., 2006). The Himalayan cryosphere contributes to the stream flow in the Indus and Ganges river systems by ∼50 % and ∼10 %–30 %, respectively (Khan et al., 2017). Warming and glacier retreat over the Himalayan cryosphere have a great potential to impact the freshwater availability for about 700 million people, modify regional hydrology and disturb the agrarian economy of all South Asian countries (Bolch et al., 2012; Immerzeel et al., 2010; Kaser et al., 2010; Singh and Bengtsson, 2004; Barnett et al., 2005; Yao et al., 2007). Therefore, it is critical to disentangle the factors contributing to glacier retreat and snowmelt over HMA.
Regional warming due to increasing greenhouse gases (Ren and Karoly, 2006) has been reported as the primary cause of the high rate of warming and glacier retreat over HMA. However, in the last decade, advancement in remote sensing and availability of measurements from several field campaigns suggest that the contribution of LAP loading (in the atmosphere) to the warming and glacier melting over HMA is probably greater than previously believed (Ramanathan et al., 2007; Prasad et al., 2009; Menon et al., 2010). Continuous observations over the Nepal Climate Observatory Pyramid (NCO-P) facility located at 5079 m a.s.l. in the southern foothills of Mt. Everest revealed very high concentrations of black carbon (Marcq et al., 2010) and desert dust (Bonasoni et al., 2010), especially in pre-monsoon months from the Indo-Gangetic Plain. Atmospheric LAPs are scavenged to the snow/ice surface by dry and wet deposition and cause measurable snow darkening and melting (Gautam et al., 2013; Yasunari et al., 2010, 2013; Nair et al., 2013; Ménégoz et al., 2014; Ming et al., 2008; Flanner and Zender, 2005). Thus, LAPs deposited in snow and associated snow darkening have been suggested as a key factor in the early snowmelt and rapid glacier retreat over HMA (Yasunari et al., 2010; Ming et al., 2008; B. Xu et al., 2009; Flanner et al., 2007; Qian et al., 2011).
While previous studies have underlined the significance of LAP deposition in snow over HMA, the estimation of LAP-induced snow darkening and associated radiative forcing is still highly uncertain (Qian et al., 2015). Many of these studies used online global model simulations at coarse spatial resolutions of ∼50–150 km (Flanner and Zender, 2005; Ming et al., 2008; Qian et al., 2011; Kopacz et al., 2011; Zhang et al., 2015). Other studies employed offline simulation of the snow albedo effect using measured or modeled concentrations of deposited LAPs in surface snow or estimated from atmospheric loading and ice cores (Yasunari et al., 2013; Nair et al., 2013; Wang et al., 2015; He et al., 2014; Santra et al., 2019; Thind et al., 2019). The complex terrain of HMA, seasonal snowfall and near-surface air circulation are not well resolved by coarse global climate models (Kopacz et al., 2011; Ménégoz et al., 2014). Similarly, offline estimations are limited in scope because they are site specific and are based on simplified assumptions about deposition rates. Ideally, online high-resolution simulations allowing for LAP–snow interactions should facilitate a more realistic understanding of LAP deposition to snow and LAP-induced snow darkening effect in terms of both magnitude and spatial variability over HMA.
In this study, a modified version of the online chemistry coupled with Weather Research and Forecasting regional model (WRF-Chem v3.5.1), which is then fully coupled with the SNICAR (SNow, ICe, and Aerosol Radiative) model, is used to perform the first-ever high-resolution (12 km) simulation over the HMA region for the water year 2013–2014 (1 October 2013 to 30 September 2014). Satellite observations of snow properties like snow albedo, grain size and LAP-induced snow darkening from MODSCAG and MODDRFS retrievals are used for evaluation (Painter et al., 2009, 2012). The main objective of this study is to evaluate the skill of the high-resolution WRF-Chem model in simulating properties of snowpack, aerosol distribution, LAP in snow and LAP-induced snow darkening over HMA using spatially and temporally complete (STC) remotely sensed snow surface properties (SSPs) from MODIS (Dozier et al., 2008; Rittger et al., 2016). Our second objective is to demonstrate the benefit for aerosol and snow distributions in high-resolution runs by comparing to a coarsely gridded quasi-global model simulation over HMA. This quasi-global simulation is run with the same WRF-Chem configuration but at 1∘ spatial resolution. Finally, the spatiotemporal variation in simulated LAP deposition, snow albedo darkening and snow-mediated LAP radiative forcing (LAPRF) over HMA is discussed. The model details and datasets used are described in Sect. 2. Results and discussions are presented in Sect. 3 followed by conclusions in Sect. 4.
Below, we provide details on the aerosol module used in WRF-Chem, interactive coupling with aerosol and SNICAR via a land model, and the model setup for both 12 km and 1∘ resolution runs. The details for the remote-sensing observations that are used to evaluate the models are also provided.
2.1 Coupled WRF-Chem–CLM–SNICAR model description
The WRF-Chem simulation is performed at 12 km×12 km horizontal resolution (hereafter referred to as WRF-HR) with 210×150 grid cells (64–89∘ E, 23–40∘ N) (Fig. 1) and 35 vertical layers. The simulation was conducted from 20 September 2013 to 30 September 2014, to provide 1 year of results (following a 10 d model spin-up). ERA-Interim reanalysis data at 0.7∘ horizontal resolution and 6 h temporal intervals are used for meteorological initial and lateral boundary conditions. The simulation is re-initialized every fourth day to prevent the drift of model meteorology. Different model physics options chosen are the MYJ (Mellor–Yamada–Janjić) planetary boundary layer scheme, Morrison two-moment microphysics scheme, community land model (CLM), Kain–Fritsch cumulus scheme, and Rapid Radiative Transfer Model for GCMs (RRTMG) for longwave and shortwave radiation schemes.
The CBM-Z (carbon bond mechanism) photochemical mechanism (Zaveri and Peters, 1999) coupled with the eight-bin MOSAIC (Model for Simulating Aerosol Interactions and Chemistry) aerosol model (Zaveri et al., 2008) are used. This is the most sophisticated aerosol module available for the WRF-Chem model. The sectional approach with eight discrete size bins is used to represent the size distributions of all the major aerosol components (including sulfate, nitrate, ammonium, black carbon (BC), organic carbon (OC), sea salt, and mineral dust) in the model. The processes of nucleation, condensation, coagulation, aqueous-phase chemistry and water uptake by aerosols in each bin size are included in the MOSAIC module. Dry deposition of aerosol mass and number is simulated by including both diffusion and gravitational effects as per Binkowski and Shankar (1995). Wet removal of aerosols follows Easter et al. (2004) and Chapman et al. (2009) and includes grid-resolved impaction and interception processes for both in-cloud (rainout) and below-cloud (washout) aerosol removal. Processes involved in convective transport and wet removal of aerosols by cumulus clouds are described in Zhao et al. (2013a).
Anthropogenic emissions used in our study are at 0.5∘ × 0.5∘ horizontal resolution and are taken from the NASA INTEX-B mission Asian emission inventory for the year 2006 (Zhang et al., 2009). Biomass burning emissions at 0.5∘ × 0.5∘ horizontal resolution for the water year 2013–2014 are obtained from the Global Fire Emissions Database, Version 3 (GFEDv3) (Van Der Werf et al., 2010), which are vertically distributed in our simulation using the injection heights prescribed by Dentener et al. (2006) for the Aerosol Comparisons between Observations and Models (AEROCOM) project. Sea salt and dust emissions follow Zhao et al. (2014). Dust surface emission fluxes are calculated with the Georgia Institute of Technology-Goddard Global Ozone Chemistry Aerosol Radiation and Transport (GOCART) dust emission scheme (Ginoux et al., 2001), and emitted into the eight MOSAIC size bins with respective mass fractions of 10−6 %, 10−4 %, 0.02 %, 0.2 %, 1.5 %, 6 %, 26 % and 45 %.
Aerosol optical properties are computed as a function of wavelength for each model grid cell. The Optical Properties of Aerosols and Clouds (OPAC) dataset (Hess et al., 1998) is used for the shortwave (SW) and longwave (LW) refractive indices of aerosols and a complex refractive index of aerosols (assuming internal mixture) is calculated by volume averaging for each chemical constituent of aerosols for each bin. A spectrally invariant value of 1.53±0.003i is used for the SW complex refractive index of dust. Fast et al. (2006) and Barnard et al. (2010) provide detailed descriptions of the computation of aerosol optical properties such as extinction coefficient, single-scattering albedo (SSA) and asymmetry factor in WRF-Chem. Following Zhao et al. (2011, 2013b), aerosol radiative feedback is coupled with the Rapid Radiative Transfer Model (RRTMG) (Mlawer et al., 1997), and the direct radiative forcing of individual aerosol species in the atmosphere are diagnosed. Aerosol–cloud interactions are included in the model following Gustafson et al. (2007).
The increasingly used Snow, Ice, and Aerosol Radiation (SNICAR) model simulates the snow properties and associated radiative heating rates of multilayer snowpacks (Flanner and Zender, 2005; Flanner et al., 2009, 2012, 2007). Fundamentally, it employs the snow albedo theory (parameterization) based on Warren and Wiscombe (1980) and the two-stream radiative approximation for multiple layers from Toon et al. (1989). SNICAR can also simulate aerosol radiative effect in snow for studying the LAP heating and snow aging (Flanner et al., 2007). Recently, laboratory and site measurements have been used to validate the SNICAR-simulated change of snow albedo for a given BC concentration in snow (Hadley and Kirchstetter, 2012; Brandt et al., 2011). For radiative transfer calculations, SNICAR defines layers matching with the five thermal layers in the Community Land Model (CLM) that vertically resolve the snow densification and meltwater transport (Oleson et al., 2010). Note that the number of snow layers and thickness of top snow surface layer are predicted in the CLM model. Fresh snowfall and melting continuously affect the model surface snow layer thickness (3 cm or less). Therefore, LAP concentrations within each snow layer depend on meltwater flushing, new snowfall and associated top layer evolution (Flanner et al., 2007, 2012; Oleson et al., 2010). In WRF-Chem–SNICAR coupled model, BC and dust deposition on snow is calculated in a prognostic approach through dry and wet deposition processes. BC in snow can be represented as externally and internally mixed with precipitation hydrometeors depending on the removal mechanism involved, but dust is considered to only mix externally with snow grains (following Flanner et al., 2012). SNICAR in WRF-Chem simulates four tracers of dust based on size (with diameters of 0.1–1, 1–2.5, 2.5–5 and 5–10 µm) and two tracers of BC (externally and internally mixed BC with 0.2 µm dry diameter) in snow. The MOSAIC aerosol model simulates dust in the atmosphere with eight size bins (0.039–0.078, 0.078–0.156, 0.156–0.312, 0.312–0.625, 0.625–1.25, 1.25–2.5, 2.5–5.0 and 5.0–10.0 µm in dry diameter). The first four bins are coupled with the smallest bin of dust particles in SNICAR. While the next two MOSAIC bins (fifth and sixth) map into the second bin of SNICAR, the seventh and eighth MOSAIC dust bins correspond to the third and fourth SNICAR dust bins (Zhao et al., 2014), respectively. Deposition of LAPs to snow in SNICAR is immediately mixed in the CLM surface snow layer (<3 cm). CLM adds excess water in the layer above to the layer beneath during melting. The scavenging of aerosols in snow by meltwater is assumed to be proportional to its mass mixing ratio of the meltwater multiplied by a scavenging factor. Scavenging factors for externally mixed BC and internally mixed BC are assumed to be 0.03 and 0.2, respectively, and 0.02, 0.02, 0.01 and 0.01 for the four dust bins (all externally mixed). Although these scavenging factors are comparable to observations (Doherty et al., 2013), the scavenging ratios can be highly heterogeneous and introduce high uncertainty into the estimation of LAP concentrations in snow (Flanner et al., 2012; Qian et al., 2014). More detailed description about the aerosol deposition and mixing processes, computation of optical properties of snow, and LAPs in WRF-Chem–CLM–SNICAR coupling can be found in Zhao et al. (2014) and Flanner et al. (2012).
Configured in the way similar to the WRF-HR, a coarse (1∘ × 1∘) gridded WRF-Chem simulation is also performed using a quasi-global model (hereafter referred as WRF-CR) with 360×130 grid cells (60∘ S–70∘ N, 180∘ W–180∘ E). Periodic boundary conditions are used in the zonal direction. REanalysis of the TROpospheric (RETRO) anthropogenic emissions for the year 2010 (ftp://ftp.retro.enes.org/pub/emissions/aggregated/anthro/0.5x0.5/, last access: 1 October 2017) are used for anthropogenic aerosol and precursor gas emissions in the coarsely gridded quasi-global WRF-Chem simulation except for Asia and the United States. INTEX-B anthropogenic emissions (Zhang et al., 2009) and the US National Emission Inventory are used for Asia and the US, respectively. Emissions of biomass burning aerosols, sea salt and dust are treated in the same way as described above for the WRF-HR simulation. More details about the quasi-global WRF-Chem simulation can be found in Zhao et al. (2013a), Hu et al. (2016). Chemical initial and boundary conditions to the WRF-HR simulation are provided by these quasi-global WRF-CR runs for the same time period to include long-range-transported chemical species.
2.2 Aerosol optical depth (AOD) dataset
The Aerosol Robotic Network (AERONET – https://aeronet.gsfc.nasa.gov, last access: 1 October 2017) is a global network of ground-based remote-sensing stations that provides quality-controlled measurements of AOD with uncertainties of ∼0.01 under clear-sky conditions over India (Holben et al., 1998; Dubovik et al., 2000). CIMEL sun scanning spectral radiometers are used to measure direct sun radiance at eight spectral channels (340, 380, 440, 500, 675, 870, 940 and 1020 nm) and measure spectral columnar AOD (Holben et al., 1998). AERONET provides measurements at ∼15 min temporal resolution from sunrise to sunset.
Skyradiometer Network (SKYNET) is another global network of ground-based spectral scanning radiometer (POM-01L, Prede, Japan) stations that provides quality-controlled measurements of AOD (Nakajima et al., 1996). With an automatic sun scanner and sensor, it measures sky irradiance in five wavelengths i.e., 400, 500, 675, 870 and 1020 nm. The measured monochromatic irradiance data are processed by using Skyrad.Pack version 4.2 software. Calibration of the sky radiometer is carried out on a monthly basis (http://atmos3.cr.chiba-u.jp/skynet/data.html, last access: 1 October 2017). Details of the instrumentation and software protocol can be found in Campanelli et al. (2007) and Ningombam et al. (2015). In this study, we have also used AOD measurements at 500 nm over Merak, a high-altitude SKYNET station in the Himalayas for the water year 2013–2014.
The Moderate Resolution Imaging Spectroradiometer (MODIS) instrument on board the NASA Aqua satellite provides global coverage of daily radiance observations (at 13:30 LT) in 36 spectral channels. Over northern India, Tripathi et al. (2005) have shown that MODIS observations correlate well with ground-based measurements. For the evaluation of model-simulated AOD, 1∘ gridded Level 3 AOD estimates (collection 6) at the 0.55 µm wavelength obtained from the MODIS instrument are used during the water year 2013–2014. However, the MODIS land aerosol algorithm uses a dark target approach (Levy et al., 2007), which is known to have large uncertainties over arid and mountainous surfaces (Levy et al., 2010).
2.3 Spatially and temporally complete MODSCAG and MODDRFS retrievals
Subpixel snow-covered area and snow grain size are retrieved from MODIS-observed surface reflectance data using the physically based MODIS Snow-Covered Area and Grain size algorithm (MODSCAG) (Painter et al., 2009). In each snow-covered pixel, MODSCAG attributes a fractional snow-covered area and grain size using spectral mixture analysis to determine the proportion of the pixel that is snow and not snow. MODSCAG more accurately identifies snow cover throughout the year than the widely used MODIS snow product: MOD10A1 (Rittger et al., 2013). The MODSCAG snow-mapping algorithm for fraction of snow-covered area has an uncertainty of ∼5 % (Rittger et al., 2013). The current study incorporates pixel level snow cover area and snow grain size from MODSCAG over the HMA region to evaluate snowpack simulation and LAP-induced albedo reduction. Further, the MODIS Dust Radiative Forcing in Snow (MODDRFS) model (Painter et al., 2012) is used to determine the LAP-induced albedo reduction over HMA. MODDRFS uses spectral reflectance differences between the measured snow spectral albedo and the modeled clean snow spectral albedo. The pixel-level clean snow spectrum corresponding to MODSCAG-retrieved snow grain sizes is calculated using discrete ordinate radiative transfer solutions for visible wavelengths and solar zenith angles. Coupled, these products provide the determination of snow albedo for the fractional snow cover with LAP inclusion.
Reflectance inputs to MODSCAG and MODDRFS are degraded by cloud cover, off-nadir views and data errors, but can be filtered in time and space to improve data quality and consistency. Our method for spatially cleaning and filling (Dozier et al., 2008; Rittger et al., 2016) combines noise filtering, snow/cloud interpolation and smoothing to improve the daily estimates of snow surface properties (SSPs). Using remotely sensed forest height maps (Simard et al., 2011) and MODSCAG vegetation fraction, we adjust the satellite-viewable snow cover to account for snow under tree canopy (Rittger et al., 2016). We weight the observations based on satellite viewing angle that varies from 0 to 65∘ with larger uncertainties in off-nadir views (Dozier et al., 2008). The result is a set of spatially and temporally complete (STC) SSPs. Use of these products in an energy balance model to estimate snow water equivalent based on reconstruction produced more accurate snow cover than the Snow Data Assimilation System (SNODAS) or an interpolation of observations from snow pillows (Bair et al., 2016). In this study we use STC versions of MODSCAG and MODDRFS when comparing our WRF model output. The incomplete remotely sensed dataset would be difficult to use given the gaps in data and uncertainties related to viewing angle (Dozier et al., 2008). Hereafter, the use of MODSCAG and MODDRFS terms will invariably refer to these STC-MODSCAG and STC-MODDRFS products.
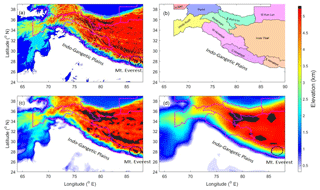
Figure 1Panel (a) illustrates the terrain elevation at 1 km resolution from the ETOPO1 dataset. Panel (b) shows glacier classification over the HMA region used in this study following the Randolph Glacier Inventory. Panels (c, d) illustrate terrain representation in the WRF-HR run (12 km) and WRF-CR (1∘) run, respectively. For reference, Mt. Everest (shown as black circle in c) is distinctly represented in (a) and (c), but not in (b).
2.4 Variation in terrain representation in WRF-HR and WRF-CR
Figure 1a illustrates the variations in terrain height over HMA at a resolution of 1 arc using the ETOPO1 global relief model, a publicly available global topographical dataset (Amante and Eakins, 2009). It clearly shows the enormous relief in terrain as we move from the Indo-Gangetic Plain (IGP) to the crest of the Himalayas and into the Tibetan Plateau (TP). The majority of HMA is above 4 km altitude with many Himalayan peaks at an altitude higher than 6 km. Figure 1b illustrates the mountain ranges and glaciers classified as per the Randolph Glacier Inventory in the Fifth Assessment Report of the Intergovernmental Panel on Climate Change (Pfeffer et al., 2014). Specifically, the Pamirs, Hindu Kush, Karakoram, Kunlun, and Himalayas hold the most number of glaciers in HMA. Figure 1c and d illustrate the representation of terrain elevation in WRF-HR and WRF-CR, respectively. Compared to Fig. 1a, locations of mountain peaks (altitude >5.5 km) are better represented in WRF-HR compared to WRF-CR, as is particularly evident over the Karakoram, Kunlun, and Himalayan ranges. Moreover, the steep rise in elevation between IGP and TP is also well represented by WRF-HR, whereas it is more gradual in WRF-CR.
2.5 Methodology
Simulations of the snow macro- and microphysical properties, aerosol loading, and LAP in snow concentration from WRF-HR, WRF-CR and observational estimates (datasets described above) over HMA are compared in Sect. 3.1 and 3.2. In Sect. 3.3, the WRF-HR-simulated LAP-induced snow albedo reduction values over HMA are compared with corresponding MODIS satellite-based STC-MODSCAG and STC-MODDRFS. Lastly, a discussion on the high-resolution model-simulated LAP-induced radiative forcing estimates over HMA is also presented in context with previous studies and other atmospheric forcing.
The simulated fractional snow-covered area (fSCA), duration of snow cover over a grid in terms of number of snow cover days (NSDs), snow albedo (α), and snow grain sizes (SGSs) and LAP-induced snow albedo darkening (Δα) for midday (10:00–14:00 LT) conditions from both the WRF models are compared with corresponding STC-MODSCAG and STC-MODDRFS retrievals over HMA. The number of snow cover days (NSDs: defined as days having fSCA values ≥0.01) during the water year 2013–2014 is determined over each grid from STC-MODSCAG and both model runs. They are compared with corresponding values from STC-MODSCAG retrievals, which are observed during Terra overpasses at 10:30 LT. We have used a window from 10:00 to 14:00 LT for representing midday averages of modeled variables to incorporate the variability due to differences in timing (between model and real scenario) of weather conditions like precipitation and clouds. In addition, the change in snow albedo during 10:00–14:00 LT is <0.01 (Bair et al., 2018), which is low compared to other uncertainties related to model physics and data retrieval. The WRF-CR-simulated variables and STC-MODSCAG and STC-MODDRFS retrievals are gridded to the resolution of WRF-HR (12 km) for ease of comparison. We have compared annual mean values as well as seasonal mean values for the winter (December–February) and pre-monsoon (April–June) seasons, separately. We have not considered the monsoon period in our analyses because the snow cover during the monsoon is negligible (except in glaciated regions at high altitudes) relative to other months (Fig. S1 in the Supplement). Simulated SGS values only from the topmost snow surface layer are compared to the MODSCAG SGS retrievals. To evaluate spatial heterogeneity in our model, the seasonal and annual distribution of these variables is calculated separately for each subregion (shown in Fig. 1) within HMA. In addition, to gain an understanding of the extent of temporal variability present in LAP-induced effects, we have also presented daily midday variation in LAP-induced snow darkening and LAP-induced radiative forcing at the surface over the Chhota Shigri glacier region (32.1–32.35∘ N, 77.4–77.7∘ E) located in the Chandra–Bhaga river basin of Lahaul valley, Pir Panjal range, in the western Himalayas. It is an accessible and representative site for glacier mass balance studies in the western Himalayas. Chhota Shigri glacier has a cumulative glaciological mass loss of −6.72 m w.e. between 2002 and 2014 (Azam et al., 2016).
The simulated aerosol optical depth (AOD) is compared with available in situ observations (described in Sect. 2.2). Here, quality-assured (Level 2) midday (10:00 to 14:00 LT) averages of AOD (550 nm) at seven AERONET stations (Lahore, Jaipur, Kanpur, Gandhi college, Kathmandu and CAS) and one SKYNET site within our study region are used to evaluate the simulated AOD values. Further, the simulated distribution of LAP concentration in snow at a few sites is compared with field measurements. Only a few field measurements of concentration of BC (LAPBC) and dust particles (LAPdust) in the snow surface or the surface layer are available over glaciated regions within our study domain. In this study, measurements of LAPBC over Muztagh Ata on the eastern slopes of the Pamirs (Xu et al., 2006), the Uttarakhand region of the western Himalayas (Svensson et al., 2018), East Rongbuk at 6.4 km altitude (Ming et al., 2012, 2008; B. Xu et al., 2009) and a composite of recent in situ measurements from various studies near the NCO-pyramid site in Nepal at 5–6 km altitude (Kaspari et al., 2014; Yasunari et al., 2013; Jacobi et al., 2015; Ginot et al., 2014) are used. We have used more than one study to have a range of annual mean LAP values over each location so in principle the observations are representative of different years and different snow depths over the same glacier. Moreover, simulated annual mean LAP concentration only from the topmost snow surface layer is compared to the observed surface snow LAP in snow concentration, which introduce differences in the snow sample depth used for the evaluation. However, to minimize the influence of snow sample depth variation, we have only used data in the literature which are observed as snow surface measurement or from snow pits having a thickness less than 15 cm. Similarly, the point measurements used for evaluating LAPdust are over Abramov glacier on the western slopes of the Pamirs (Schmale et al., 2017), Muztagh Ata on the eastern slopes of the Pamirs (Wake et al., 1994) , East Rongbuk (Ming et al., 2012) and near NCO-pyramid station (Ginot et al., 2014).
3.1 Snow physical, microphysical and optical properties
The largest values of region-averaged annual mean fSCA within HMA are observed in both the satellite retrievals and the model runs over the Karakoram region (mean=0.45) followed by the Pamirs, Himalayas and Hindu Kush in the HMA region (Fig. 2a and b). In comparison, the fSCA values over Kunlun and TP are lower (<0.3), but pockets of very high fSCA (∼0.7) are visible over the West Kunlun ranges (Fig. 2a). The annual mean fSCA values and the fine spatial variability are well simulated by WRF-HR (Fig. 2b) over the entire HMA region. Of exception are simulations over the Karakoram, where WRF-HR overestimates annual mean fSCA; however, the distribution of annual mean fSCA from WRF-HR and STC-MODSCAG agree in all the subregions (Fig. 2d). This observation is also largely valid for pre-monsoon months (Fig. 2f). However, significant overestimation in distribution of fSCA (by >0.2) during winter is present over the Pamirs, Karakoram, western Himalayas, TP and Kunlun regions (Fig. 2e). STC-MODSCAG retrievals illustrate that the Pamir (NSD=230 d) and Karakoram (NSD=270 d) ranges remain snow covered for 7–9 months of the year (Fig. 3a). Similarly, the grids in Hindu Kush (NSD=194 d), the western Himalayas (NSD=189 d) and the central Himalayas (NSD=191 d) are snow covered for ∼6–7 months. Mountains in the eastern Himalayas (NSD=142 d) remain snow covered for only 4–5 months of the year. The distribution of annual NSD values simulated by WRF-HR in each subregion is close to STC-MODSCAG values (Fig. 3d). Also, the spatial distribution and magnitude of simulated NSD by WRF-HR is similar to that from STC-MODSCAG for different seasons, separately (Fig. S2). Thus, overestimation of annual mean fSCA in WRF-HR during winter is not due to mere averaging error associated with underestimation in simulated NSD during winter (Fig. S2).
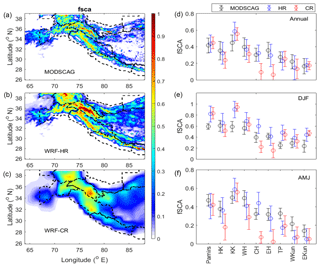
Figure 2Spatial distribution of annual mean snow cover fraction (fSCA) during midday (10:00–14:00 LT) for the water year 2013–2014 from (a) STC-MODSCAG retrievals, (b) simulated values from WRF-HR and (c) WRF-CR simulations. Panels (d)–(f) illustrate the distribution of midday mean fSCA over each subregion identified by glacier classification following the Randolph Glacier Inventory (x axis). The circle and vertical legs represent mean±standard deviation (SD) over each region for the (d) entire year, (e) winter (December–February) and (f) pre-monsoon (April–June) season, separately. Here, the Hindu Kush, Karakoram, western Himalayan, central Himalayan, eastern Himalayan, Tibetan Plateau, West Kunlun and East Kunlun regions are abbreviated as HK, KK, WH, CH, EH, TP, WKun and EKun, respectively.
We also calculated the number of days of snow cover with low fSCA (<0.5; Fig. 3b) and high fSCA (>0.5; Fig. 3c) values, separately. The grids in Kunlun, the northern slope of Karakoram, the eastern slope of the Pamirs and the TP region are dominated by snow cover of relatively low fSCA for most of their snow cover duration (Fig. 3b). But grids in Hindu Kush, the Himalayas and the southern slopes of Karakoram are generally covered with high fSCA values throughout the year (Fig. 3c). The distribution of simulated NSD values over each subregion for low and high fSCA scenarios is shown in Fig. 3e and f, respectively. WRF-HR can simulate the NSD over grids with high fSCA well (Fig. 3f) but significantly underestimates NSD over grids with low snow cover (Fig. 3e). Note that the regions dominated by low annual fSCA in this water year are actually the same regions where WRF-HR-simulated fSCA values are being overestimated in winter (Fig. 2e). Thus, simulation of fewer days with low fSCA (and vice versa) in WRF-HR might also be contributing partially to the overestimation of winter fSCA simulated in WRF-HR compared to STC-MODSCAG. Further, WRF-HR-simulated annual surface rainfall in winter is overestimated over the Karakoram, Himalayan and Hindu Kush ranges (Fig. S3). This indicates that overestimation of surface precipitation in WRF-HR may also contribute to the overestimation of fSCA over HMA in winter. Interestingly, WRF-CR-simulated NSD values for the low fSCA case are also in better agreement with STC-MODSCAG values (Fig. 3e). Winter mean distribution of WRF-CR-simulated fSCA over Kunlun, the western Himalayas and the TP region better match STC-MODSCAG values than the corresponding WRF-HR-simulated fSCA values (Fig. 2f). It is noteworthy that these subregions (which are dominated by low fSCA grids) receive snowfall from western disturbances during winter months. The cloud cover associated with the western disturbances over these subregions is extensive in winter, which also introduces uncertainty in MODSCAG retrievals and STC processing and contributes to the differences between WRF-HR and MODSCAG in fSCA.
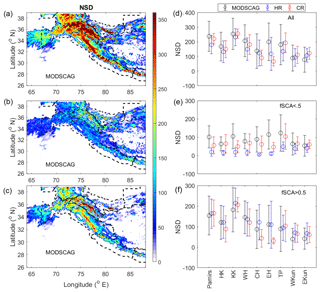
Figure 3Spatial distribution of snow duration in terms of NSD from (a) STC-MODSCAG retrievals. Panels (b) and (c) are similar to (a), but show the number of days when fSCA is below and above 0.5 over each grid, respectively. Panel (d) illustrates the distribution of NSD over each subregion identified by glacier classification following the Randolph Glacier Inventory. The circle and vertical legs represent mean±SD over each region for the entire year. Here, the Hindu Kush, Karakoram, western Himalayan, central Himalayan, eastern Himalayan, Tibetan Plateau, West Kunlun and East Kunlun regions are abbreviated as HK, KK, WH, CH, EH, TP, WKun and EKun, respectively. Panels (e) and (f) are similar to (d), but for NSD corresponding to fSCA values below and above 0.5, respectively.
Comparison between performance of WRF-CR and WRF-HR for fSCA clearly shows significant improvements in the WRF-HR simulations over the Hindu Kush and Himalayan ranges (Fig. 2d). For instance, the simulated annual mean fSCA in WRF-CR around Mt. Everest (shown as the black circle in Fig. 1) is less than 0.1 (Fig. 2c). This is contrary to the high fSCA values observed at Mt. Everest (0.7 in Fig. 2a) and simulated by WRF-HR (0.7 in Fig. 2b). Moreover, the improvement is present in both winter and pre-monsoon months, indicating its independence from meteorological variations (Fig. 2e and f). Analysis of NSD values indicates that the snow cover duration in WRF-HR also improved significantly over these slopes (Fig. S4) irrespective of the season. Note that WRF-CR underestimates the snow duration over Hindu Kush and the Himalayas by ∼2–6 months (Fig. S4) and the spatial location of grids with very high annual mean fSCA values (mountain ranges) improved in WRF-HR compared to the STC-MODSCAG data (Fig. 2a–c). The observed improvement in fSCA and NSD simulation over the slopes of the Himalayas and Hindu Kush can be attributed to better terrain representation in WRF-HR.
Next, the simulated microphysical properties of the snowpack are evaluated against the remote-sensing retrievals. Spatial patterns in annual mean SGS from STC-MODSCAG are similar to that seen in fSCA with the highest values over the Karakoram and Himalayan ranges (Fig. 4a) corresponding to the highest elevations and likely the coldest temperatures hindering snow grain growth. This spatial distribution of annual mean SGS values is well simulated in WRF-HR runs (Fig. 4b). However, the annual mean values over each subregion are largely overestimated by 30–50 µm (Fig. 4d) relative to STC-MODSCAG. The seasonal distribution of region-segregated SGS values from WRF-HR also compares well with that from STC-MODSCAG retrievals (Fig. 4e). Simulated annual mean SGSs from WRF-CR (Fig. 4c) lack the fine spatial variability seen in STC-MODSCAG and WRF-HR. Moreover, the SGS estimates are largely underestimated (by up to 100 µm) by WRF-CR, specifically over grids in the central and eastern Himalayan, TP and Kunlun ranges (Fig. 4d). The large underestimation of SGS from WRF-CR and overestimation of SGS from WRF-HR is present for both pre-monsoon and winter months (not shown). The overestimation of SGS from WRF-HR values corroborates well with the finding that the simulated fSCA distribution from WRF-HR is largely skewed towards higher values (Fig. 3). Similarly, the unrealistically low mean values of SGS from WRF-CR over the Himalayan, TP and Kunlun ranges are consistent with the underestimation of fSCA and NSD values over these regions (Figs. 2 and 3). Meanwhile, SGS retrievals from STC-MODSCAG are based on observed surface reflectance; the modeled SGS is calculated from simulated snow mass in the top model layer in the grid. Hence, improvement in simulation of fSCA and NSD in high-resolution WRF-HR runs also caused the SGS values from WRF-HR to be closer to STC-MODSCAG retrievals than SGS from WRF-CR runs. It is worth noting that the presence of cloud cover influences STC-MODSCAG retrievals of SGS towards smaller grain sizes if clouds are misidentified as snow. This systematic error could also contribute to the SGS differences between WRF-HR and STC-MODSCAG estimates.
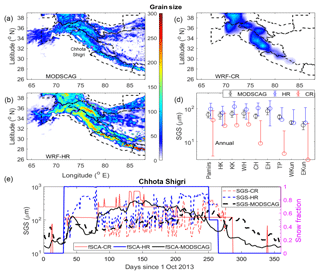
Figure 4Spatial distribution of annual mean snow grain size (SGS) during midday (10:00–14:00 LT) for the water year 2013–2014 from (a) STC-MODSCAG retrievals and simulated values from (b) WRF-HR and (c) WRF-CR runs are shown. Panel (d) illustrates the distribution of midday mean fSCA over each subregion identified by glacier classification following the Randolph Glacier Inventory. The circle and vertical legs represent mean±SD over each region for the entire year. Here, the Hindu Kush, Karakoram, western Himalayan, central Himalayan, eastern Himalayan, Tibetan Plateau, West Kunlun and East Kunlun regions are abbreviated as HK, KK, WH, CH, EH, TP, WKun and EKun, respectively. Panel (e) shows time series of daily midday SGS (hashed lines) and fSCA (solid lines) from MODSCAG (black), WRF-HR (blue) and WRF-CR (red) over a grid located near the Chhota Shigri glacier (marked by magenta diamond in Fig. 3a) of the western Himalayan region.
Quite anomalous to other grids in HMA, the WRF-CR-simulated SGS values over some grids near the Chhota Shigri glacier (marked by the magenta circle in Fig. 4a) of the western Himalayan subregion are closer to STC-MODSCAG observations than that simulated by WRF-HR runs. As a sanity check, daily variation in SGS (hashed lines in Fig. 4e) and fSCA (solid lines in Fig. 4e) from STC-MODSCAG (black), WRF-HR (blue) and WRF-CR (red) over this glacier are compared. Fractional snow cover from STC-MODSCAG gradually increases from 0.2 in November 2013 to 0.8 in February 2014 and subsequently decreases back to 0.1 by September 2014 at the glacier location. Corresponding SGS values from STC-MODSCAG closely followed the seasonal trend in fSCA varying around the values of 80–200 µm in winter. In comparison, simulated fSCA from WRF-HR values drastically increased to 1 at the beginning of November 2013 (from no snow cover before that), remained fully snow covered till mid-June 2014 and then suddenly became snow free after June. Compared to satellite estimates, fSCA values from WRF-HR are greater in magnitude throughout the duration of snow cover, indicating more snow mass simulated by WRF-HR. Associated SGS values (80–800 µm) simulated by WRF-HR are also greater than STC-MODSCAG estimates throughout the snow duration over the grid. However, the fSCA variation from WRF-CR over this grid is very close to the variation seen by STC-MODSCAG, and the associated SGS values (50–400 µm) from WRF-CR are also closer to the estimated STC-MODSCAG values, supporting our argument that biases in simulation of fSCA also affect the simulated annual mean SGS values.
The annual mean snow albedo (α) values and the distribution over each subregion from satellite estimates (by combing grain sizes from STC-MODSCAG and decrease in albedo from STC-MODDRFS; see Bair et al., 2016) and simulated by both models are presented in Fig. 5. The highest annual mean α values (∼0.65–0.75) are observed over mountain peaks in the Karakoram, Pamir and western Himalayan regions (Fig. 5a). The location and magnitude of annual mean α over these grids are closely reproduced in WRF-HR with an underestimation of <10 % (Fig. 5b). WRF-CR-simulated annual mean α values over these grids have a considerably larger underestimate of ∼50 % (Fig. 5c). Similar statistics are prevalent over all the subregions of HMA (Fig. 5d). Specifically, the distribution of α values from WRF-HR nearly matches the observed distribution, but the distribution of albedos from the coarser model, WRF-CR, are generally 0.2–0.3 lower when compared to the observations. The improvement of α estimation in WRF-HR runs compared to WRF-CR runs can be attributed to the relatively better simulation of fSCA (Fig. 2), NSD (Fig. 3) and SGS (Fig. 4) in WRF-HR. Simulated annual mean α values are primarily the composite albedo values of snow-covered grids. In WRF-Chem–SNICAR simulations, the composite albedo of a snow-covered grid box is computed as the weighted average of representative area fractions of sub-grid snow cover and snow-free regions. Thus, relatively lower values of simulated fSCA and NSD in WRF-CR runs compared to WRF-HR runs can contribute substantially to the relatively lower annual and seasonal mean α values simulated by WRF-CR. Note, the opposite nature in biases simulated by WRF-HR runs in mean albedo and SGS values. This is intuitive as smaller snow particles cover greater surface area and therefore reflect more solar radiation from the surface. A similar pattern in distribution of snow albedo from WRF-HR and WRF-CR is also found over the subregions for pre-monsoon and winter months, separately (Fig. 5e and f), indicating robust improvement in WRF-HR-simulated albedo values throughout the year. The differences in simulated α in WRF-HR with the observations increased during pre-monsoon months and were lower during winter. Here, it is worth mentioning that we are comparing instantaneous estimates obtained from Terra overpass during 10:00 LT with midday (10:00–14:00 LT) mean model values. The inherent diurnal variations in α values under clear-sky conditions in the pre-monsoon season (Bair et al., 2018) might contribute partially to the observed enhancement in differences during the pre-monsoon season. At the same time, the mean α values from STC-MODSCAG (representative of only snow-covered regions) are biases towards higher values than the corresponding simulated α values (composite albedo of the pixel) predominantly over the snow grids with annual mean fSCA values much smaller than 1.
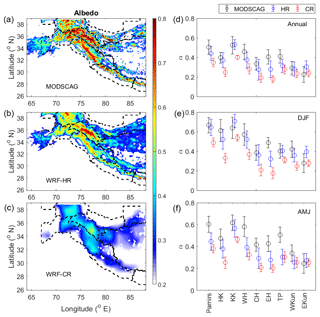
Figure 5Spatial distribution of annual mean snow albedo (α) during midday (10:00–14:00 LT) for the water year 2013–2014 from (a) STC-MODSCAG retrievals and simulated values from (b) WRF-HR and (c) WRF-CR runs is shown. Panels (d)–(f) illustrate the distribution of midday mean fSCA over each subregion identified by glacier classification following the Randolph Glacier Inventory. The circle and vertical legs represent mean±SD over each region for the (d) entire year, (e) winter (December–February) and (f) pre-monsoon (April–June) season, separately. Here, the Hindu Kush, Karakoram, western Himalayan, central Himalayan, eastern Himalayan, Tibetan Plateau, West Kunlun and East Kunlun regions are abbreviated as HK, KK, WH, CH, EH, TP, WKun and EKun, respectively.
3.2 Aerosol distribution and LAP in snow
We used available in situ and ground sun photometer measurements from seven different sites across our study domain (location shown in Fig. 6) to evaluate the simulated aerosol optical depth (AOD). The annual mean midday AOD at each site is shown in Fig. 6a. Three sites (i.e., Merak, CAS and Kathmandu shown in Fig. 6b–d) are located on the Himalayan slopes and the other four sites (Lahore, Jaipur, Kanpur and Gandhi College shown in Fig. 6e–h) are located in the Indo-Gangetic Plains. In situ measurements clearly illustrate a sharp decrease (4–5-fold) in mean AOD as we traverse higher up the Himalayan slope. The annual mean AOD for Lahore and Kanpur sites are 0.41 and 0.52, respectively, while the AOD over high-elevation sites, i.e., Merak and CAS, is 0.07 and 0.05, respectively. Also, MODIS-observed AOD values prominently show the reduction in annual mean AOD from the Indo-Gangetic Plains (MODIS-AOD ∼0.4–0.7) to the Tibet region (MODIS-AOD ∼0.1–0.2) (Fig. S5). Over the four sites in the Indo-Gangetic Plains, AOD simulated by both WRF-HR and WRF-CR runs is well correlated with observations (r=0.5–0.6, Fig. 6e–h). The biases in modeled AOD are also similar (in the range of 0.2–0.4) in the case of both WRF-HR and WRF-CR runs (Fig. 6e–h). Thus, no significant improvement in AOD values are achieved over the plain region with fine resolution. However, distinct and large improvement in simulated AOD is seen over the high-elevation sites due to the increase in spatial resolution. Note that AOD values from WRF-CR are not strongly correlated with observations at these sites (Fig. 6b–d) and also have very high positive biases in AOD values (even higher than annual mean values at the Merak and CAS stations). In contrast, the correlation between observations and WRF-HR is reasonably good (r=0.5–0.8 at these sites) using fine spatial resolution in WRF-HR. The positive biases in AOD from WRF-HR at the Merak and CAS sites are lower than corresponding WRF-CR values by an order of magnitude. Presence of lower biases in AOD from WRF-HR over high-elevation sites indicates that the observed sharp decrease in AOD values across the Himalayan slope is better captured by the higher-resolution WRF run (WRF-HR) than in the coarser run. Greater annual mean AOD value is simulated by WRF-CR over the entire HMA region compared to WRF-HR (Fig. S5) supporting an overestimation of AOD from WRF-CR at higher elevation in addition to the few sites. The presence of high biases (0.3–0.4) over Kathmandu valley even in WRF-HR runs indicates that model resolution even finer than 12 km is likely needed to better resolve the AOD distribution in complex terrain around valleys in Himalayan slope regions. Moreover, Jayarathne et al. (2018) show that many local emissions are not accounted for in coarse emissions, which causes underestimation in simulated regional AOD values in these valleys. Temporal variability in monthly mean AOD (relatively higher AOD in pre-monsoon months) is simulated reasonably well by both the model versions (Fig. S5).
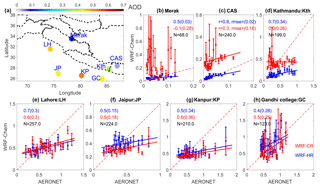
Figure 6Comparison of midday (averaged over 10:00–14:00 LT) aerosol optical depth (AOD) measured by a ground-based sun photometer at seven sites within the study domain with corresponding simulated AOD values from both WRF-HR and WRF-CR. The annual mean AOD values over each site are shown by color in (a). The other panels illustrate the comparison over each station shown by dots in (a). The N mentioned in the legend in each panel is the total number of days when collocated data between model and measurement are available over that site. These sample points are divided into 50 equal bins of ascending AERONET-AOD values (two percentiles each) and averaged. The standard deviation in each bin is shown by the vertical bars. The correlation coefficient values (r) are also mentioned in the legend followed in brackets by the relative error values (∑RMSE/mean obs).
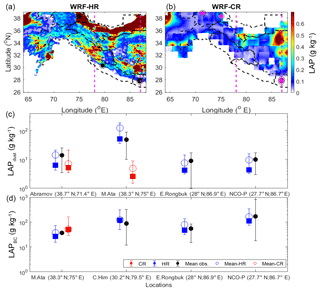
Figure 7Spatial distribution of annual mean LAP concentration in the snow top layer for the water year 2013–2014 simulated by (a) WRF-HR and (b) WRF-CR. The black dots in (a) denote the locations where observations of BC in snow are available. Similarly, the magenta dots in (b) denote the locations where observations of dust in snow are available. Panels (c)–(d) illustrate comparison of simulated LAPBC (top) and LAPdust (bottom), respectively, in the topmost snow layer with observed values over the marked locations in the Himalayan cryosphere. Simulated annual mean (circle) and distribution of midday mean LAP values (box plot) are shown. The top (bottom) edge of the box plot represents the 75th (25th) percentile of the distribution. The WRF-HR and WRF-CR values are represented by blue and red, respectively. The pink lines in (a) are the cross sections shown in Fig. 8.
Significant differences in simulated AOD over high elevations of Himalayan slopes and TP indicate that considerable differences might also be present in LAP concentrations in snow between the two WRF simulations. Annual mean LAP concentrations in the top snow layer from WRF-HR and WRF-CR simulations are compared in Fig. 7a–b. The comparison shows that LAP concentration in WRF-HR is significantly higher than WRF-CR-simulated values. Quantitatively, the WRF-HR-simulated annual mean LAP concentrations over the Pamir (0.5 g kg−1), Karakoram (0.45 g kg−1), Hindu Kush (0.2 g kg−1), western Himalayan (0.3 g kg−1), central Himalayan (0.2 g kg−1) and eastern Himalayan (0.08 g kg−1) ranges are 3–5 times higher than the same from WRF-CR runs. In contrast, WRF-HR-simulated LAP over the TP (0.21 g kg−1) and Kunlun ranges (0.8 g kg−1) is similar to the mean magnitude simulated by WRF-CR runs. As a sanity check, we evaluate the simulated LAP concentrations against those previously reported in the literature. Figure 7c and d illustrate the evaluation of mean annual LAP concentration from WRF-HR and WRF-CR associated with BC (LAPBC) and dust (LAPdust), respectively, against the reported data (shown as filled black circles). The locations of reported LAPBC (black filled dots) and LAPdust (magenta filled dots) are shown in Fig. 7c and d, respectively. First, the difference in the magnitude of LAPdust and LAPBC over HMA is striking. The LAPdust is more than 1000 times greater than LAPBC in both observations and the models. Second, LAPBC and LAPdust values from WRF-HR are much closer to reported values compared to WRF-CR values. The differences in mean of reported LAPBC and LAPdust distribution to that simulated by WRF-HR at various sites are in range of 5–30 µg kg−1 and 5–20 mg kg−1, respectively. WRF-CR simulates the concentrations of LAPBC and LAPdust over the Pamirs well (∼10 mg kg−1), but significantly underestimates the LAPBC and LAPdust (by an order of magnitude) over the Himalayan ranges. Thus, the WRF-HR better simulates aerosol and LAP concentration than the WRF-CR over the HMA region.
It is interesting to note that finer spatial resolution resulted in lower AOD but greater LAP values in snow over some places in HMA. For more insight, the vertical distribution of aerosol concentration in altitude–latitude space (Fig. 8) across two latitudinal cross sections (magenta colored lines in Fig. 7a) is analyzed. Figure 8 illustrates the differences in simulated vertical distribution of mean aerosol number concentration along 78∘ E (row 1) and 87∘ E (row 2) for WRF-HR (left column) and WRF-CR (right column) runs, respectively. Corresponding terrain elevation (black solid line) and snow depth (magenta bars) are also overlaid in these plots. The latitude–altitude plots clearly illustrate that improved representation of the terrain in WRF-HR shows the sharp change in elevation over Himalayan foothills and causes a steeper natural barrier to the transport of aerosols uphill from the IGP to HMA region than in the WFR-CR model. Also, high spatial resolution enhances snowfall in WRF-HR over the HMA region relative to the WRF-CR model. While the former change increased annual dry deposition flux, more snowfall caused greater wet deposition annually in WRF-HR compared to WRF-CR (Fig. S6). The combination of these effects increases the deposition of aerosols and therefore LAP on the southern slopes of the Himalayas in the WRF-HR run. This explains the coexistence of higher LAP concentration/deposition and lower AOD across HMA in WRF-HR, compared to corresponding WRF-CR results.
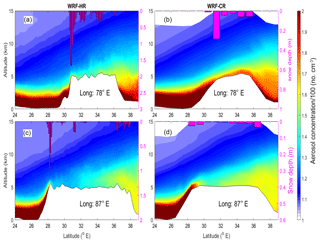
Figure 8Longitudinally averaged annual mean aerosol number concentration plotted in altitude–latitude space for two longitudinal traverses across the study domain, i.e., 78∘ N (a, b) and 87∘ N (c, d) for both WRF-HR ((a, c)) and WRF-CR ((b, d)). The corresponding terrain elevation is shown as a solid black line. Corresponding to each latitude, the longitudinally averaged annual mean snow depth is also presented as magenta color bars (using y axis on the right).
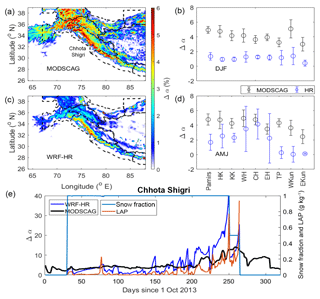
Figure 9Spatial distribution of annual mean LAP-induced snow albedo darkening (Δα) during midday (10:00–14:00 LT) for the water year 2013–2014 from (a) MODSCAG retrievals and (b) simulated values from WRF-HR is shown. Panels (c) and (d) illustrate the distribution of midday mean Δα over each subregion identified by glacier classification following the Randolph Glacier Inventory. The circle and vertical legs represent mean±SD over each region for the entire year. Here, the Hindu Kush, Karakoram, western Himalayan, central Himalayan, eastern Himalayan, Tibetan Plateau, West Kunlun and East Kunlun regions are abbreviated as HK, KK, WH, CH, EH, TP, WKun and EKun, respectively. Panel (e) shows time series of daily midday Δα from STC-MODSCAG (black) and WRF-HR (blue) over the grids located near the Chhota Shigri glacier (marked in a) of the western Himalayan region. Fractional snow cover and LAP concentrations from WRF-HR over the same grids are also included.
3.3 LAP-induced snow darkening and radiative forcing
STC-MODDRFS retrievals illustrate that locations in Hindu Kush and the western Himalayas have the highest annual mean LAP-induced reduction in snow albedo (Δα in %) followed by the Karakoram, central Himalayan and Pamir regions (Fig. 9a). WRF-HR simulated the spatial variations in annual mean Δα reasonably well (Fig. 9b), but the magnitudes are underestimated by ∼20 %–40 % throughout the domain. Note that the biases in annual mean values are lowest over grids in the Himalayan ranges (where the underestimation is within 20 %). Season-wise and region-wise distribution plots show that the WRF-HR biases are higher in winter months than the pre-monsoon months (Fig. 9c, d). Meanwhile, WRF-HR-simulated Δα values in the winter span between 1 % and 3 %; the corresponding STC-MODDRFS estimates of Δα are larger with values ranging between 3 % and 6 % (thus no overlap with model values) over all the subregions. In pre-monsoon months, the distribution of modeled WRF-HR Δα values over the Karakoram and Himalayan ranges is similar in magnitude, explaining the lower biases in annual mean values over the Himalayas. This spatiotemporal variability in differences between STC-MODDRFS retrievals and simulated Δα values is consistent with the variability in biases of fractional snow cover seen in WRF-HR (Fig. 2e, f). For a closer look, the difference in daily midday mean Δα values from STC-MODDRFS (black) and WRF-HR (blue) is compared (in Fig. 9e) over the grids of Chhota Shigri glacier (similar to Fig. 4e). Corresponding, midday mean fSCA (light blue) and LAP (orange) from WRF-HR are also plotted. Δα values from STC-MODDRFS are about 5 % during winter months but increase in pre-monsoon months until mid-June (peak value is 18 %). Albedo reduction is closely associated with the temporal progression in midday LAP concentration in snow over this region at a daily scale. In agreement, midday Δα values from WRF-HR are lower in winter months and higher in pre-monsoon months. Except occasional peaks, with magnitudes of 3 %–4 %, Δα values from WRF-HR largely remained below 3 % till late February. A steep increase in Δα values from WRF-HR is seen in March (monthly mean ∼4 %), April (9 %), May (13 %) and June (18 %).
As already discussed, the simulated fSCA values in WRF-HR are greater than observed fSCA from STC-MODSCAG for most of the winter season (Fig. 4e). Specifically, the underestimation in WRF-HR-simulated Δα values (Fig. 9c, d) in winter over Karakoram, Hindu Kush and the Himalayas is in agreement with corresponding overestimation of WRF-HR-simulated fSCA values over these regions (Fig. 2). STC-MODDRFS-estimated Δα is based on surface reflectance, while Δα calculated by model involves the surface layer depth. The surface snow layer in SNICAR–CLM continuously evolves as fresh snowfall is added or with snow melting, so the LAP concentrations in the surface layer depend on new snowfall, meltwater flushing and layer combination/division (Flanner et al., 2007, 2012; Oleson et al., 2010). Therefore, more precipitation and more snow coverage in winter can be a primary factor causing the underestimation of annual mean LAP concentration and LAP-induced snow darkening. Secondly, the associated overestimation in simulated SGS during winter (Fig. 4) can also contribute to the lower Δα values simulated in WRF-HR because bigger snow grains in WRF-HR lead to lower clean albedo and thus smaller percentage reduction in albedo compared to STC-MODDRFS. Moreover, we have assumed spherical shaped snow grains in our simulations. Recently, microscopic-level studies show that uncertainties associated with simplified snow grain shape treatment in model parameterization can solely contribute to large biases in SNICAR-Δα estimates and thus the LAP–snow albedo radiative and snowmelt feedback processes (Liou et al., 2014; Dang et al., 2016; He et al., 2017). Thirdly, the fact that the persistent cloud cover over HMA during the winter season can induce a lot of uncertainty in the STC-MODSCAG and STC-MODDRFS estimates is also equally important.
At the same time, uncertainties regarding aerosol emission, transport and deposition to the snow layers are also significant. It is well known that the transport and deposition of black carbon from Indian landmass to the Himalayas increases in the afternoon with the evolution of the boundary layer over the IGP region (Dumka et al., 2015; Raatikainen et al., 2014). This feature is well simulated by the model (not shown). As STC-MODDRFS estimates are representative of 10:00 LT, but simulated values are sampled in the midday (10:00–14:00 LT), positive biases in aerosol transport and deposition in snowpacks (i.e., higher Δα values) might be simulated in WRF-HR runs, especially during pre-monsoon months. At the same time, GOCART dust emission parameterization (used here) is dependent on near-surface wind speed. Previous studies have evaluated and illustrated inherent uncertainties in dust emission by this parameterization, mostly underestimation over the Indian region (Dipu et al., 2013; Kumar et al., 2014). Thus, the uncertainty in local dust emission fluxes over HMA can also contribute to the biases in simulated Δα values. Also, large biases in LAP values may be simulated due to model uncertainties in enhanced wet scavenging fluxes in winter. An overestimation in LAP concentration can lead to an overestimation of snow darkening and melting, resulting in an underestimation of NSD (Fig. S4). The large biases in Δα values (>20 %) simulated by WRF-HR towards late spring could be attributed to both underestimation in fSCA and overestimation of LAP concentration in the model.
Although a better quantification of these model biases requires evaluation against in situ measurements, it is worth mentioning here that no in situ measurements are available for a direct comparison of these high WRF-HR Δα values over the western Himalayas (Gertler et al., 2016). Nonetheless, the high values simulated during pre-monsoon months are close to previously reported values over other HMA regions. Kaspari et al. (2014) used the offline SNICAR model to report that BC concentrations in pre-monsoon snow/ice samples at Mera Glacier were large enough to reduce albedo by 6 %–10 % and the reduction in albedo was 40 %–42 % relative to clean snow when dust is included in the calculation. Recently, Zhang et al. (2018) combined a large dataset of LAP measurements in surface snow with the offline SNICAR model to illustrate that Δα can be >35 % over the Tibetan Plateau. Moreover, the composite effect of this discrepancy on seasonal and annual mean values is minimal as the snowpack is at its minimum near the end of pre-monsoon season. Similar high daily variability, huge radiative forcing values (LAPRF∼200 W m−2) and sudden decline in snow depth in late pre-monsoon months is also reported over the upper Colorado River basin (Skiles et al., 2015; Skiles and Painter, 2017)
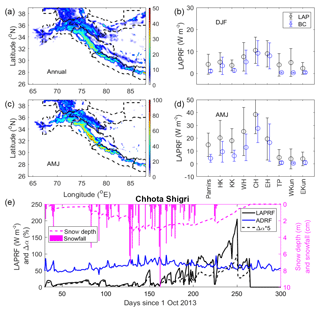
Figure 10(a) Spatial distribution of annual mean snow-mediated LAP-induced radiative forcing (LAPRF) from WRF-HR and (b) spatial distribution of seasonal mean LAPRF over snow-covered areas for the pre-monsoon season (AMJ) of the water year 2013–2014. Panels (c) and (d) illustrate the distribution of LAPRF (blue) and BC-only LAPRF (black) over each subregion identified by glacier classification following the Randolph Glacier Inventory for winter and pre-monsoon seasons, respectively. The circle and vertical legs represent mean±SD over each region for the entire year. Here, the Hindu Kush, Karakoram, western Himalayan, central Himalayan, eastern Himalayan, Tibetan Plateau, West Kunlun and East Kunlun regions are abbreviated as HK, KK, WH, CH, EH, TP, WKun and EKun, respectively. Panel (e) shows time series of daily midday LAPRF (blue) and aerosol direct radiative forcing at the surface (ADRF, blue) over the Chhota Shigri region (marked in Fig. 9a) of the western Himalayan region. The simulated LAP-induced change in snow albedo is also shown as a hashed black line. Also, snow depth and snowfall from WRF-HR over the same grid are included.
In agreement with the spatial variation in Δα values, the annual mean snow-mediated LAP-induced radiative forcing (LAPRF) from WRF-HR over grids in the western Himalayas and central Himalayas is highest followed by that from the Karakoram, Hindu Kush, eastern Himalayan and Pamir regions (Fig. 10a). The spatial distribution of pre-monsoon mean LAPRF values from WRF-HR (Fig. 10b) is similar to that of the annual mean LAPRF values, but the pre-monsoon magnitudes are higher by an order of magnitude throughout most of the domain. This is mainly due to the large increase in Δα values in pre-monsoon values when LAPs aggregate on the surface compared with winter months when LAPs are continuously covered by new snow (Fig. 9). Spatiotemporal variability in LAPRF is evident in the seasonal and regional distribution plots (Fig. 10c, d). LAPRF values over the edges of HMA are greater than the highland TP region in both winter and pre-monsoon months probably due to greater LAP deposition simulated over the Hindu Kush, Karakoram and Himalayan regions (Fig. S7) from the close proximity of dust sources. Also, it is clearly visible that the maximum LAPRF values within the HMA region are present over grids in Himalayan ranges (during both winter and pre-monsoon) with annual mean values >50 W m−2 (seen in Fig. 10b, d) and maximum instantaneous values higher than 150 W m−2 (not shown). The time series of midday mean LAPRF values (Fig. 10e) over the same grids in the Chhota Shigri glacier region in the western Himalayas is plotted to ascertain possible daily variability in LAPRF for midday over the region. Corresponding LAP-induced albedo reduction, snow depth and snowfall values are also plotted to show how LAPRF can affect local snow melting. The daily snow depth increases to 3.6 m in winter (30–150 d) followed by gradual reduction and snow cover melting in pre-monsoon months (150–270 d). The mean midday LAPRF value is ∼10 W m−2 in the winter season, but the magnitude increased gradually during March (18 W m−2), April (44 W m−2), May (86 W m−2) and June (123.5 W m−2), eventually terminating with a peak value of 202 W m−2 in mid-June. The temporal evolution in Δα values closely followed the LAPRF values with a variation in the range of 1 %–20 %. The shortwave aerosol direct radiative forcing (ADRF) during midday at the surface over the same grid is also shown (as a blue curve) in Fig. 10e. The momentarily high values of ADRF during the period indicate dust storms or sudden increase in aerosol loading over the grid. A closer look illustrates that the large daily variations in LAPRF and Δα are associated with the variability in ADRF and daily snowfall occurrence over the grid. Fresh snowfall feedbacks result in subsequent reduction in LAPRF and enhancement in snow depth (Fig. 10e), while higher aerosol loading over aged snow is followed by a clear increase in LAPRF. During melting, our model considers that only a fraction of LAP is washed away with meltwater, which results in enhanced concentration of LAP during the end stages of the snowpack. This snow–albedo feedback along with the momentary high aerosol loading (on the 250th day) can explain the very high values of albedo reduction and LAPRF that were simulated during the last days of the snow cover over this grid. Higher LAPRF values indicate more energy being absorbed by the snowpack and thus more snow melting. Therefore, LAP-induced snow melting effect over the Himalayas is very significant and is the largest relative to other areas within HMA.
Snow-mediated radiative forcing only due to BC deposition is shown in Fig. 10c, d. Although a similar spatiotemporal pattern in LAPRF and BC-only LAPRF is simulated, contrasting features in the context of BC contribution to LAPRF are present in between the western part and eastern part of HMA. During winter, the magnitude of BC-only LAPRF values is similar to that of total LAPRF over the western part of the HMA region (i.e., Pamirs, Karakoram, Hindu Kush and western Himalayas) suggesting that BC is a dominant contributor to net LAPRF (Fig. 10c). However, large differences between LAPRF and BC-only LAPRF are present during the pre-monsoon season over the western regions, indicating that dust contribution is more or less equal to that of BC contribution over these regions (Fig. 10d). In contrast, the dust contribution to LAPRF values over the eastern domain (TP and Kunlun regions) is significant during the winter season (Fig. 10c, d). The spatiotemporal variability in dust and BC contribution has been reported previously and is mainly linked with the seasonal variability in meteorology and associated advection of South Asian emissions (Zhang et al., 2015; Wang et al., 2015; Niu et al., 2018). The ADRF-induced surface cooling effect may nullify the effects of LAPRF-induced warming effect on snowpack melting. However, the drastic increase in LAPRF values in April through June causes the magnitude of LAPRF to be twice that of ADRF over the western Himalayas during the snow melting period, highlighting dominance of LAPRF (as also seen in Fig. 10e).
The simulated annual mean, pre-monsoon mean and BC-only LAPRF values from WRF-HR are in general higher compared to previously reported estimates of LAPRF from model studies at coarser resolution. For example, Ménégoz et al. (2014) have reported annual mean LAPRF of ∼1–3 W m−2 over the Himalayas using an online simulation at a 50 km resolution grid. Similarly, Qian et al. (2011) used the Community Atmosphere Model version 3.1 at coarser spatial resolution to show that simulated aerosol-induced snow albedo perturbations can generate LAPRF values of 5–25 W m−2 during pre-monsoon months over HMA. Also, coarsely resolved GEOS-Chem-simulated BC-only LAPRF can vary from 5 to 15 W m−2 in the snow-covered regions over the TP (Kopacz et al., 2011). Recently, a decade-long simulation using the RegCM model at 50 km spatial resolution also estimated maximum BC-only LAPRF values of 5–6 W m−2 over the Himalayas and southeastern TP averaged over the non-monsoon season (Ji, 2016). However, the comparison of WRF-HR and WRF-CR simulations provided in this study clearly shows that the magnitudes of snow macro- and micro-properties, aerosol loading and LAP-induced albedo darkening over the Himalayas improved significantly with finer spatial resolution. Thus, the global model-simulated LAPRF values are likely underestimated. In agreement, recently, Zhang et al. (2018) estimated BC-only LAPRF of 20–35 W m−2 using an offline SNICAR calculation forced with a greater coverage of measurements of surface snow content. He et al. (2018) have also reported similar high BC-only LAPRF values after implementing a realistic snow grain size parameterization in offline SNICAR calculations over HMA.
In this study, we use the SNICAR model coupled with the WRF-Chem regional model at high spatial resolution (WRF-HR; 12 km) to simulate the transport, deposition and radiative forcing of light-absorbing particles (LAPs; mainly black carbon and dust) over the high mountains of Asia (HMA) during the water year 2013–2014. The snow grain sizes and LAP-induced snow darkening were evaluated, for the first time, against comprehensive satellite retrievals (the MODSCAG and MODDRFS spatial and temporally complete retrieved satellite observations) over HMA. The atmospheric aerosol loading is evaluated against satellite and ground-based AOD measurements over the HMA region. Results from another simulation which employ the same model configuration but a coarser spatial resolution (WRF-CR; 1∘) are also compared with WRF-HR to illustrate the significance of a better representation of terrain on snowpack and aerosol simulation over HMA. The main conclusions from our study are as follows.
-
The simulated macro- and microphysical properties and the duration of snowpacks over HMA improve significantly due to the use of fine spatial resolution, especially over the southern slopes of the Hindu Kush and Himalayan ranges.
-
Simulated aerosol loading over HMA is also more realistic in WRF-HR than in WRF-CR, which leads to a reduction in biases of annual mean LAP concentration in snow. This improvement is attributed to a more realistic simulation of wet deposition (due to a better simulation of snowpack) and dry deposition of LAPs (associated with a better representation of terrain) in WRF-HR.
-
WRF-HR captures the magnitude of LAP-induced snow albedo reduction (Δα) over the Himalayas and Hindu Kush region relatively well compared to the STC-MODDRFS retrievals during pre-monsoon months. However, during winter, large biases in modeled Δα values are present. This is probably due to inherent uncertainties in model parameterizations and satellite retrievals associated with the cloud cover over HMA in the winter period.
-
The glaciers and snow cover regions located in the Himalayas have the highest LAPRF within HMA, i.e., annual mean LAPRF at ∼20 W m−2 and pre-monsoon mean LAPRF at ∼40 W m−2. This is consistent with similar high values of Δα over Himalayan ranges, i.e., annual mean Δα values of ∼2 %–4 % and pre-monsoon mean Δα values of ∼4 %–8 %. The annual mean LAP concentration in snow values (200–300 mg kg−1) is also high. Thus, the Himalayas (more specifically, the western Himalayas) are most vulnerable to LAP-induced snow melting within HMA.
Ramanathan and Carmichael (2008) suggest that atmospheric warming from LAPs (∼20 W m−2) may be just as important as greenhouse gases in the melting of snowpack and glaciers over Asia. In this context, the high magnitudes of LAPRF values in pre-monsoon months over HMA (∼40 W m−2) clearly show that snow-mediated aerosol forcing on snow melting over HMA can be 2-fold the significance of the atmospheric forcing. Nonetheless, the differences in snow surface properties between WRF-HR and satellite observations indicate probable uncertainties in model parameterizations. At the same time, the STC-MODDRFS retrievals themselves may have an uncertainty of ∼5 % in instantaneous Δα measurements. Thus, efforts to further reduce the LAPRF uncertainties in the model are warranted in the future by using in situ observations (i.e., field campaigns), specifically over the most-affected western Himalayas, where relevant measurements are largely absent (Gertler et al., 2016). Moreover, satellite retrievals will be markedly improved in the coming decade with the NASA Decadal Survey Surface Biology and Geology imaging spectrometer mission, which includes as a core measurement snow albedo and its controls. These retrievals, visible through a shortwave infrared imaging spectrometer, have uncertainties an order of magnitude smaller (Painter et al., 2013) than those from multispectral sensors such as MODIS and will provide a more accurate constraint on the physically based modeling pursued here.
Online chemistry coupled Weather Research and Forecasting model (WRF-Chem) is a community model available for download at http://www2.mmm.ucar.edu/wrf/users/download/get_sources.html (last access: 1 October 2017). MODSCAG–MODDRFS data are available from https://snow.jpl.nasa.gov/portal/data/help_modscag (last access: 1 October 2017). The modified version of the model code used in this study and processed satellite product can be obtained from the authors upon request. Aerosol optical depth data are freely available from https://aeronet.gsfc.nasa.gov/ (last access: 1 October 2017) and http://atmos3.cr.chiba-u.jp/skynet/merak/merak.html (last access: 1 October 2017).
The supplement related to this article is available online at: https://doi.org/10.5194/acp-19-7105-2019-supplement.
CS performed the modeling and analysis and wrote the paper under the supervision of YQ. KR, KJB and THP created the STC-MODSCAG and STC-MODDRFS datasets. All authors provided advice and feedback throughout the analysis, drafting and submission process.
The authors declare that they have no conflict of interest.
This research was supported by the NASA High Mountain Asia Project. The Pacific Northwest National Laboratory (PNNL) is operated for the DOE by Battelle Memorial Institute under contract DE-AC06-76RLO 1830. Part of this work was performed at the Jet Propulsion Laboratory, California Institute of Technology under contract with NASA. Hui Wang acknowledges support from the U.S. Department of Energy (DOE), Office of Science, Biological and Environmental Research as part of the Earth System Modeling program. We thank the PIs of the selected AERONET and SKYNET stations for providing the data used in this study. The AERONET data are obtained from the AERONET website, http://aeronet.gsfc.nasa.gov/ (last access: 1 October 2017). We also thank the PI of the selected SKYNET station for providing the data used in this study. The SKYNET data are obtained from the website http://atmos3.cr.chiba-u.jp/skynet/merak/merak.html/ (last access: 1 October 2017).
This paper was edited by Yafang Cheng and reviewed by three anonymous referees.
Amante, C. and Eakins, B. W.: ETOPO1 1 Arc-Minute Global Relief Model: Procedures, Data Sources and Analysis, NOAA Technical Memorandum NESDIS, NGDC-24, 19 pp., 2009.
Azam, M. F., Ramanathan, A., Wagnon, P., Vincent, C., Linda, A., Berthier, E., Sharma, P., Mandal, A., Angchuk, T., Singh, V. B., and Pottakkal, J. G.: Meteorological conditions, seasonal and annual mass balances of Chhota Shigri Glacier, western Himalaya, India, Ann. Glaciol., 57, 328–338, https://doi.org/10.3189/2016AoG71A570, 2016.
Bair, E. H., Rittger, K., Davis, R. E., Painter, T. H., and Dozier, J.: Validating reconstruction of snow water equivalent in California's Sierra Nevada using measurements from the NASA Airborne Snow Observatory, Water Resour. Res., 52, 8437–8460, https://doi.org/10.1002/2016WR018704, 2016.
Bair, E. H., Davis, R. E., and Dozier, J.: Hourly mass and snow energy balance measurements from Mammoth Mountain, CA USA, 2011–2017, Earth Syst. Sci. Data, 10, 549–563, https://doi.org/10.5194/essd-10-549-2018, 2018.
Barnard, J. C., Fast, J. D., Paredes-Miranda, G., Arnott, W. P., and Laskin, A.: Technical Note: Evaluation of the WRF-Chem “Aerosol Chemical to Aerosol Optical Properties” Module using data from the MILAGRO campaign, Atmos. Chem. Phys., 10, 7325–7340, https://doi.org/10.5194/acp-10-7325-2010, 2010.
Barnett, T. P., Adam, J. C., and Lettenmaier, D. P.: Potential impacts of a warming climate on water availability in snow-dominated regions, Nature, 438, 303–309, https://doi.org/10.1038/nature04141, 2005.
Binkowski, F. S. and Shankar, U.: The Regional Particulate Matter Model. 1. Model description and preliminary results, J. Geophys. Res., 100, 26191–26209, https://doi.org/10.1029/95JD02093, 1995.
Bolch, T., Kulkarni, A., Kääb, A., Huggel, C., Paul, F., Cogley, J. G., Frey, H., Kargel, J. S., Fujita, K., Scheel, M., Bajracharya, S., and Stoffel, M.: The state and fate of himalayan glaciers, Science, 336, 310–314, https://doi.org/10.1126/science.1215828, 2012.
Bollasina, M. A., Ming, Y., and Ramaswamy, V.: Anthropogenic Aerosols and the Weakening of the South Asian Summer Monsoon, Science, 334, 502–505, https://doi.org/10.1126/science.1204994, 2011.
Bonasoni, P., Laj, P., Marinoni, A., Sprenger, M., Angelini, F., Arduini, J., Bonafè, U., Calzolari, F., Colombo, T., Decesari, S., Di Biagio, C., di Sarra, A. G., Evangelisti, F., Duchi, R., Facchini, MC., Fuzzi, S., Gobbi, G. P., Maione, M., Panday, A., Roccato, F., Sellegri, K., Venzac, H., Verza, GP., Villani, P., Vuillermoz, E., and Cristofanelli, P.: Atmospheric Brown Clouds in the Himalayas: first two years of continuous observations at the Nepal Climate Observatory-Pyramid (5079 m), Atmos. Chem. Phys., 10, 7515–7531, https://doi.org/10.5194/acp-10-7515-2010, 2010.
Bond, T. C., Doherty, S. J., Fahey, D. W., Forster, P. M., Berntsen, T., Deangelo, B. J., Flanner, M. G., Ghan, S., Kärcher, B., Koch, D., Kinne, S., Kondo, Y., Quinn, P. K., Sarofim, M. C., Schultz, M. G., Schulz, M., Venkataraman, C., Zhang, H., Zhang, S., Bellouin, N., Guttikunda, S. K., Hopke, P. K., Jacobson, M. Z., Kaiser, J. W., Klimont, Z., Lohmann, U., Schwarz, J. P., Shindell, D., Storelvmo, T., Warren, S. G., and Zender, C. S.: Bounding the role of black carbon in the climate system: A scientific assessment, J. Geophys. Res.-Atmos., 118, 5380–5552, https://doi.org/10.1002/jgrd.50171, 2013.
Brandt, R. E., Warren, S. G., and Clarke, A. D.: A controlled snowmaking experiment testing the relation between black carbon content and reduction of snow albedo, J. Geophys. Res.-Atmos., 116, D08109, https://doi.org/10.1029/2010JD015330, 2011.
Brown, R. D. and Robinson, D. A.: Northern Hemisphere spring snow cover variability and change over 1922–2010 including an assessment of uncertainty, The Cryosphere, 5, 219–229, https://doi.org/10.5194/tc-5-219-2011, 2011.
Campanelli, M., Estellés, V., Tomasi, C., Nakajima, T., Malvestuto, V., and Martínez-Lozano, J. A.: Application of the SKYRAD Improved Langley plot method for the in situ calibration of CIMEL Sun-sky photometers, Appl. Optics, 46, 2688–702, https://doi.org/10.1364/AO.46.002688, 2007.
Chapman, E. G., Gustafson Jr., W. I., Easter, R. C., Barnard, J. C., Ghan, S. J., Pekour, M. S., and Fast, J. D.: Coupling aerosol-cloud-radiative processes in the WRF-Chem model: Investigating the radiative impact of elevated point sources, Atmos. Chem. Phys., 9, 945–964, https://doi.org/10.5194/acp-9-945-2009, 2009.
Conway, H., Gades, A., and Raymond, C. F.: Albedo of dirty snow during conditions of melt, Water Resour. Res., 32, 1713–1718, https://doi.org/10.1029/96WR00712, 1996.
Dang, C., Fu, Q., and Warren, S. G.: Effect of Snow Grain Shape on Snow Albedo, J. Atmos. Sci., 73, 3573–3583, https://doi.org/10.1175/JAS-D-15-0276.1, 2016.
Dang, C., Warren, S. G., Fu, Q., Doherty, S. J., Sturm, M., and Su, J.: Measurements of light-absorbing particles in snow across the Arctic, North America, and China: Effects on surface albedo, J. Geophys. Res.-Atmos., 122, 10149–10168, https://doi.org/10.1002/2017JD027070, 2017.
Dentener, F., Kinne, S., Bond, T., Boucher, O., Cofala, J., Generoso, S., Ginoux, P., Gong, S., Hoelzemann, J. J., Ito, A., Marelli, L., Penner, J. E., Putaud, J.-P., Textor, C., Schulz, M., van der Werf, G. R., and Wilson, J.: Emissions of primary aerosol and precursor gases in the years 2000 and 1750 prescribed data-sets for AeroCom, Atmos. Chem. Phys., 6, 4321–4344, https://doi.org/10.5194/acp-6-4321-2006, 2006.
Dipu, S., Prabha, T. V., Pandithurai, G., Dudhia, J., Pfister, G., Rajesh, K., and Goswami, B. N.: Impact of elevated aerosol layer on the cloud macrophysical properties prior to monsoon onset, Atmos. Environ., 70, 454–467, 2013.
Doherty, S. J., Warren, S. G., Grenfell, T. C., Clarke, A. D., and Brandt, R. E.: Light-absorbing impurities in Arctic snow, Atmos. Chem. Phys., 10, 11647–11680, https://doi.org/10.5194/acp-10-11647-2010, 2010.
Doherty, S. J., Grenfell, T. C., Forsström, S., Hegg, D. L., Brandt, R. E., and Warren, S. G.: Observed vertical redistribution of black carbon and other insoluble light-absorbing particles in melting snow, J. Geophys. Res.-Atmos., 118, 5553–5569, https://doi.org/10.1002/jgrd.50235, 2013.
Dozier, J., Painter, T. H., Rittger, K., and Frew, J. E.: Time-space continuity of daily maps of fractional snow cover and albedo from MODIS, Adv. Water Resour., 31, 1515–1526, https://doi.org/10.1016/j.advwatres.2008.08.011, 2008.
Dubovik, O., Smirnov, A., Holben, B. N., King, M. D., Kaufman, Y. J., Eck, T. F., and Slutsker, I.: Accuracy assessments of aerosol optical properties retrieved from Aerosol Robotic Network (AERONET) Sun and sky radiance measurements, J. Geophys. Res.-Atmos., 105, 9791–9806, https://doi.org/10.1029/2000JD900040, 2000.
Dumka, U. C., Kaskaoutis, D. G., Srivastava, M. K., and Devara, P. C. S.: Scattering and absorption properties of near-surface aerosol over Gangetic–Himalayan region: the role of boundary-layer dynamics and long-range transport, Atmos. Chem. Phys., 15, 1555–1572, https://doi.org/10.5194/acp-15-1555-2015, 2015.
Dyurgerov, M. B.: Mountain glaciers at the end of the twentieth century: Global analysis in relation to climate and water cycle, Polar Geogr., 25, 241–336, https://doi.org/10.1080/10889370109377717, 2001.
Easter, R. C., Ghan, S. J., Zhang, Y., Saylor, R. D., Chapman, E. G., Laulainen, N. S., Abdul-Razzak, H., Leung, L. R., Bian, X., and Zaveri, R. A.: MIRAGE: Model description and evaluation of aerosols and trace gases, J. Geophys. Res.-Atmos., 109, D20210, https://doi.org/10.1029/2004JD004571, 2004.
Fan, J., Wang, Y., Rosenfeld, D., and Liu, X.: Review of Aerosol–Cloud Interactions: Mechanisms, Significance, and Challenges, J. Atmos. Sci., 73, 4221–4252, https://doi.org/10.1175/JAS-D-16-0037.1, 2016.
Fast, J. D., Gustafson, W. I., Easter, R. C., Zaveri, R. A., Barnard, J. C., Chapman, E. G., Grell, G. A., and Peckham, S. E.: Evolution of ozone, particulates, and aerosol direct radiative forcing in the vicinity of Houston using a fully coupled meteorology-chemistry-aerosol model, J. Geophys. Res.-Atmos., 111, D21305, https://doi.org/10.1029/2005JD006721, 2006.
Flanner, M. G. and Zender, C. S.: Snowpack radiative heating: Influence on Tibetan Plateau climate, Geophys. Res. Lett., 32, 1–5, https://doi.org/10.1029/2004GL022076, 2005.
Flanner, M. G., Zender, C. S., Randerson, J. T., and Rasch, P. J.: Present-day climate forcing and response from black carbon in snow, J. Geophys. Res.-Atmos., 112, D11202, https://doi.org/10.1029/2006JD008003, 2007.
Flanner, M. G., Zender, C. S., Hess, P. G., Mahowald, N. M., Painter, T. H., Ramanathan, V., and Rasch, P. J.: Springtime warming and reduced snow cover from carbonaceous particles, Atmos. Chem. Phys., 9, 2481–2497, https://doi.org/10.5194/acp-9-2481-2009, 2009.
Flanner, M. G., Liu, X., Zhou, C., Penner, J. E., and Jiao, C.: Enhanced solar energy absorption by internally-mixed black carbon in snow grains, Atmos. Chem. Phys., 12, 4699–4721, https://doi.org/10.5194/acp-12-4699-2012, 2012.
Gautam, R., Hsu, N. C., Lau, W. K. M., and Yasunari, T. J.: Satellite observations of desert dust-induced Himalayan snow darkening, Geophys. Res. Lett., 40, 988–993, https://doi.org/10.1002/grl.50226, 2013.
Gertler, C. G., Puppala, S. P., Panday, A., Stumm, D., and Shea, J.: Black carbon and the Himalayan cryosphere: A review, Atmos. Environ., 125, 404–417, https://doi.org/10.1016/j.atmosenv.2015.08.078, 2016.
Ghatak, D., Sinsky, E., and Miller, J.: Role of snow-albedo feedback in higher elevation warming over the Himalayas, Tibetan Plateau and Central Asia, Environ. Res. Lett., 9, 114008, https://doi.org/10.1088/1748-9326/9/11/114008, 2014.
Ginot, P., Dumont, M., Lim, S., Patris, N., Taupin, J.-D., Wagnon, P., Gilbert, A., Arnaud, Y., Marinoni, A., Bonasoni, P., and Laj, P.: A 10 year record of black carbon and dust from a Mera Peak ice core (Nepal): variability and potential impact on melting of Himalayan glaciers, The Cryosphere, 8, 1479–1496, https://doi.org/10.5194/tc-8-1479-2014, 2014.
Ginoux, P., Chin, M., Tegen, I., Prospero, J. M., Holben, B., Dubovik, O., and Lin, S.-J.: Sources and distributions of dust aerosols simulated with the GOCART model, J. Geophys. Res.-Atmos., 106, 20255–20273, https://doi.org/10.1029/2000JD000053, 2001.
Gustafson, W. I., Chapman, E. G., Ghan, S. J., Easter, R. C., and Fast, J. D.: Impact on modeled cloud characteristics due to simplified treatment of uniform cloud condensation nuclei during NEAQS 2004, Geophys. Res. Lett., 34, L19809, https://doi.org/10.1029/2007GL030021, 2007.
Hadley, O. L. and Kirchstetter, T. W.: Black-carbon reduction of snow albedo, Nat. Clim. Chang., 2, 437–440, https://doi.org/10.1038/nclimate1433, 2012.
Hansen, J. and Nazarenko, L.: Soot climate forcing via snow and ice albedos, P. Natl. Acad. Sci. USA, 101, 423–428, https://doi.org/10.1073/pnas.2237157100, 2004.
Hansen, J., Sato, M., and Ruedy, R.: Radiative forcing and climate response, J. Geophys. Res.-Atmos., 102, 6831–6864, https://doi.org/10.1029/96JD03436, 1997.
He, C., Li, Q., Liou, K.-N., Takano, Y., Gu, Y., Qi, L., Mao, Y., and Leung, L. R.: Black carbon radiative forcing over the Tibetan Plateau, Geophys. Res. Lett., 41, 7806–7813, https://doi.org/10.1002/2014GL062191, 2014.
He, C., Takano, Y., Liou, K.-N., Yang, P., Li, Q., and Chen, F.: Impact of Snow Grain Shape and Black Carbon–Snow Internal Mixing on Snow Optical Properties: Parameterizations for Climate Models, J. Climate, 30, 10019–10036, https://doi.org/10.1175/JCLI-D-17-0300.1, 2017.
He, C., Flanner, M. G., Chen, F., Barlage, M., Liou, K.-N., Kang, S., Ming, J., and Qian, Y.: Black carbon-induced snow albedo reduction over the Tibetan Plateau: uncertainties from snow grain shape and aerosol–snow mixing state based on an updated SNICAR model, Atmos. Chem. Phys., 18, 11507–11527, https://doi.org/10.5194/acp-18-11507-2018, 2018.
Hess, M., Koepke, P., and Schult, I.: Optical Properties of Aerosols and Clouds: The Software Package OPAC, B. Am. Meteorol. Soc., 79, 831–844, https://doi.org/10.1175/1520-0477(1998)079<0831:OPOAAC>2.0.CO;2, 1998.
Holben, B. N., Eck, T. F., Slutsker, I., Tanré, D., Buis, J. P., Setzer, A., Vermote, E., Reagan, J. A., Kaufman, Y. J., Nakajima, T., Lavenu, F., Jankowiak, I., and Smirnov, A.: AERONET – A Federated Instrument Network and Data Archive for Aerosol Characterization, Remote Sens. Environ., 66, 1–16, https://doi.org/10.1016/S0034-4257(98)00031-5, 1998.
Hu, Z., Zhao, C., Huang, J., Leung, L. R., Qian, Y., Yu, H., Huang, L., and Kalashnikova, O. V.: Trans-Pacific transport and evolution of aerosols: evaluation of quasi-global WRF-Chem simulation with multiple observations, Geosci. Model Dev., 9, 1725–1746, https://doi.org/10.5194/gmd-9-1725-2016, 2016.
Immerzeel, W. W., Van Beek, L. P. H., and Bierkens, M. F. P.: Climate change will affect the asian water towers, Science, 328, 1382–1385, https://doi.org/10.1126/science.1183188, 2010.
IPCC: Climate Change 2013 – The Physical Science Basis: Working Group I Contribution to the Fifth Assessment Report of the Intergovernmental Panel on Climate Change, Cambridge University Press, Cambridge, https://doi.org/10.1017/CBO9781107415324, 2014.
Jacobi, H.-W., Lim, S., Ménégoz, M., Ginot, P., Laj, P., Bonasoni, P., Stocchi, P., Marinoni, A., and Arnaud, Y.: Black carbon in snow in the upper Himalayan Khumbu Valley, Nepal: observations and modeling of the impact on snow albedo, melting, and radiative forcing, The Cryosphere, 9, 1685–1699, https://doi.org/10.5194/tc-9-1685-2015, 2015.
Jayarathne, T., Stockwell, C. E., Bhave, P. V., Praveen, P. S., Rathnayake, C. M., Islam, Md. R., Panday, A. K., Adhikari, S., Maharjan, R., Goetz, J. D., DeCarlo, P. F., Saikawa, E., Yokelson, R. J., and Stone, E. A.: Nepal Ambient Monitoring and Source Testing Experiment (NAMaSTE): emissions of particulate matter from wood- and dung-fueled cooking fires, garbage and crop residue burning, brick kilns, and other sources, Atmos. Chem. Phys., 18, 2259–2286, https://doi.org/10.5194/acp-18-2259-2018, 2018.
Ji, Z. M.: Modeling black carbon and its potential radiative effects over the Tibetan Plateau, Adv. Clim. Chang. Res., 7, 139–144, https://doi.org/10.1016/j.accre.2016.10.002, 2016.
Kaser, G., Grosshauser, M., and Marzeion, B.: Contribution potential of glaciers to water availability in different climate regimes, P. Natl. Acad. Sci. USA, 107, 20223–20227, https://doi.org/10.1073/pnas.1008162107, 2010.
Kaspari, S., Painter, T. H., Gysel, M., Skiles, S. M., and Schwikowski, M.: Seasonal and elevational variations of black carbon and dust in snow and ice in the Solu-Khumbu, Nepal and estimated radiative forcings, Atmos. Chem. Phys., 14, 8089–8103, https://doi.org/10.5194/acp-14-8089-2014, 2014.
Khan, A. A., Pant, N. C., Sarkar, A., Tandon, S. K., Thamban, M., and Mahalinganathan, K.: The Himalayan cryosphere: A critical assessment and evaluation of glacial melt fraction in the Bhagirathi basin, Geosci. Front., 8, 107–115, https://doi.org/10.1016/j.gsf.2015.12.009, 2017.
Kopacz, M., Mauzerall, D. L., Wang, J., Leibensperger, E. M., Henze, D. K., and Singh, K.: Origin and radiative forcing of black carbon transported to the Himalayas and Tibetan Plateau, Atmos. Chem. Phys., 11, 2837–2852, https://doi.org/10.5194/acp-11-2837-2011, 2011.
Kulkarni, A. V., Rathore, B. P., and Singh, S. K.: Distribution of seasonal snow cover in central and western Himalaya, Ann. Glaciol., 51, 123–128, https://doi.org/10.3189/172756410791386445, 2010.
Kumar, R., Barth, M. C., Pfister, G. G., Naja, M., and Brasseur, G. P.: WRF-Chem simulations of a typical pre-monsoon dust storm in northern India: influences on aerosol optical properties and radiation budget, Atmos. Chem. Phys., 14, 2431–2446, https://doi.org/10.5194/acp-14-2431-2014, 2014.
Lau, K. M., Kim, M. K., and Kim, K. M.: Asian summer monsoon anomalies induced by aerosol direct forcing: The role of the Tibetan Plateau, Clim. Dynam., 26, 855–864, https://doi.org/10.1007/s00382-006-0114-z, 2006.
Lau, W. K. M., Kim, M. K., Kim, K. M., and Lee, W. S.: Enhanced surface warming and accelerated snow melt in the Himalayas and Tibetan Plateau induced by absorbing aerosols, Environ. Res. Lett., 5, 025204, https://doi.org/10.1088/1748-9326/5/2/025204, 2010.
Levy, R. C., Remer, L. A., Mattoo, S., Vermote, E. F., and Kaufman, Y. J.: Second-generation operational algorithm: Retrieval of aerosol properties over land from inversion of Moderate Resolution Imaging Spectroradiometer spectral reflectance, J. Geophys. Res.-Atmos., 112, D13211, https://doi.org/10.1029/2006JD007811, 2007.
Levy, R. C., Remer, L. A., Kleidman, R. G., Mattoo, S., Ichoku, C., Kahn, R., and Eck, T. F.: Global evaluation of the Collection 5 MODIS dark-target aerosol products over land, Atmos. Chem. Phys., 10, 10399–10420, https://doi.org/10.5194/acp-10-10399-2010, 2010.
Li, Z., Lau, W. K. M., Ramanathan, V., Wu, G., Ding, Y., Manoj, M. G., Liu, J., Qian, Y., Li, J., Zhou, T., Fan, J., Rosenfeld, D., Ming, Y., Wang, Y., Huang, J., Wang, B., Xu, X., Lee, S. S., Cribb, M., Zhang, F., Yang, X., Zhao, C., Takemura, T., Wang, K., Xia, X., Yin, Y., Zhang, H., Guo, J., Zhai, P. M., Sugimoto, N., Babu, S. S., and Brasseur, G. P.: Aerosol and monsoon climate interactions over Asia, Rev. Geophys., 54, 866–929, https://doi.org/10.1002/2015RG000500, 2016.
Liou, K. N., Takano, Y., He, C., Yang, P., Leung, L. R., Gu, Y., and Lee, W. L.: Stochastic parameterization for light absorption by internally mixed BC/dust in snow grains for application to climate models, J. Geophys. Res.-Atmos., 119, 7616-7632, https://doi.org/10.1002/2014JD021665, 2014.
Marcq, S., Laj, P., Roger, J. C., Villani, P., Sellegri, K., Bonasoni, P., Marinoni, A., Cristofanelli, P., Verza, G. P., and Bergin, M.: Aerosol optical properties and radiative forcing in the high Himalaya based on measurements at the Nepal Climate Observatory-Pyramid site (5079 m a.s.l.), Atmos. Chem. Phys., 10, 5859–5872, https://doi.org/10.5194/acp-10-5859-2010, 2010.
Ménégoz, M., Krinner, G., Balkanski, Y., Boucher, O., Cozic, A., Lim, S., Ginot, P., Laj, P., Gallée, H., Wagnon, P., Marinoni, A., and Jacobi, H. W.: Snow cover sensitivity to black carbon deposition in the Himalayas: from atmospheric and ice core measurements to regional climate simulations, Atmos. Chem. Phys., 14, 4237–4249, https://doi.org/10.5194/acp-14-4237-2014, 2014.
Menon, S., Koch, D., Beig, G., Sahu, S., Fasullo, J., and Orlikowski, D.: Black carbon aerosols and the third polar ice cap, Atmos. Chem. Phys., 10, 4559–4571, https://doi.org/10.5194/acp-10-4559-2010, 2010.
Ming, J., Cachier, H., Xiao, C., Qin, D., Kang, S., Hou, S., and Xu, J.: Black carbon record based on a shallow Himalayan ice core and its climatic implications, Atmos. Chem. Phys., 8, 1343–1352, https://doi.org/10.5194/acp-8-1343-2008, 2008.
Ming, J., Du, Z., Xiao, C., Xu, X., and Zhang, D.: Darkening of the mid-Himalaya glaciers since 2000 and the potential causes, Environ. Res. Lett., 7, 014021, https://doi.org/10.1088/1748-9326/7/1/014021, 2012.
Mlawer, E. J., Taubman, S. J., Brown, P. D., Iacono, M. J., and Clough, S. A.: Radiative transfer for inhomogeneous atmospheres: RRTM, a validated correlated-k model for the longwave, J. Geophys. Res.-Atmos., 102, 16663–16682, https://doi.org/10.1029/97JD00237, 1997.
Nair, V. S., Babu, S. S., Moorthy, K. K., Sharma, A. K., Marinoni, A., and Ajai: Black carbon aerosols over the Himalayas: Direct and surface albedo forcing, Tellus B, 65, 1, https://doi.org/10.3402/tellusb.v65i0.19738, 2013.
Nakajima, T., Tonna, G., Rao, R., Boi, P., Kaufman, Y., and Holben, B.: Use of sky brightness measurements from ground for remote sensing of particulate polydispersions, Appl. Optics, 35, 2672, https://doi.org/10.1364/AO.35.002672, 1996.
Ningombam, S. S., Srivastava, A. K., Bagare, S. P., Singh, R. B., Kanawade, V. P., and Dorjey, N.: Assessment of aerosol optical and micro-physical features retrieved from direct and diffuse solar irradiance measurements from Skyradiometer at a high altitude station at Merak: Assessment of aerosol optical features from Merak, Environ. Sci. Pollut. Res., 22, 16610–16619, https://doi.org/10.1007/s11356-015-4788-9, 2015.
Niu, H., Kang, S., Wang, H., Zhang, R., Lu, X., Qian, Y., Paudyal, R., Wang, S., Shi, X., and Yan, X.: Seasonal variation and light absorption property of carbonaceous aerosol in a typical glacier region of the southeastern Tibetan Plateau, Atmos. Chem. Phys., 18, 6441–6460, https://doi.org/10.5194/acp-18-6441-2018, 2018.
Oleson, K. W., Lawrence, D. M., Gordon, B., Flanner, M. G., Kluzek, E., Peter, J., Levis, S., Swenson, S. C., Thornton, E., and Feddema, J.: Technical description of version 4.0 of the Community Land Model (CLM), NCAR Technical Note NCAR/TN-478+STR, https://doi.org/10.5065/D6FB50WZ, 2010.
Painter, T. H., Rittger, K., McKenzie, C., Slaughter, P., Davis, R. E., and Dozier, J.: Retrieval of subpixel snow covered area, grain size, and albedo from MODIS, Remote Sens. Environ., 113, 868–879, https://doi.org/10.1016/j.rse.2009.01.001, 2009.
Painter, T. H., Bryant, A. C., and McKenzie Skiles, S.: Radiative forcing by light absorbing impurities in snow from MODIS surface reflectance data, Geophys. Res. Lett., 39, L17502, https://doi.org/10.1029/2012GL052457, 2012.
Painter, T. H., Seidel, F. C., Bryant, A. C., McKenzie Skiles, S., and Rittger, K.: Imaging spectroscopy of albedo and radiative forcing by light-absorbing impurities in mountain snow, J. Geophys. Res.-Atmos., 118, 9511–9523, https://doi.org/10.1002/jgrd.50520, 2013.
Pfeffer, W. T., Arendt, A. A., Bliss, A., Bolch, T., Cogley, J. G., Gardner, A. S., Hagen, J. O., Hock, R., Kaser, G., Kienholz, C., Miles, E. S., Moholdt, G., Mölg, N., Paul, F., Radić, V., Rastner, P., Raup, B. H., Rich, J., Sharp, M. J., Andreassen, L. M., Bajracharya, S., Barrand, N. E., Beedle, M. J., Berthier, E., Bhambri, R., Brown, I., Burgess, D. O., Burgess, E. W., Cawkwell, F., Chinn, T., Copland, L., Cullen, N. J., Davies, B., De Angelis, H., Fountain, A. G., Frey, H., Giffen, B. A., Glasser, N. F., Gurney, S. D., Hagg, W., Hall, D. K., Haritashya, U. K., Hartmann, G., Herreid, S., Howat, I., Jiskoot, H., Khromova, T. E., Klein, A., Kohler, J., König, M., Kriegel, D., Kutuzov, S., Lavrentiev, I., Le Bris, R., Li, X., Manley, W. F., Mayer, C., Menounos, B., Mercer, A., Mool, P., Negrete, A., Nosenko, G., Nuth, C., Osmonov, A., Pettersson, R., Racoviteanu, A., Ranzi, R., Sarikaya, M. A., Schneider, C., Sigurdsson, O., Sirguey, P., Stokes, C. R., Wheate, R., Wolken, G. J., Wu, L. Z., and Wyatt, F. R.: The randolph glacier inventory: A globally complete inventory of glaciers, J. Glaciol., 60, 537–552, https://doi.org/10.3189/2014JoG13J176, 2014.
Prasad, A. K., Yang, K.-H. S., El-Askary, H. M., and Kafatos, M.: Melting of major Glaciers in the western Himalayas: evidence of climatic changes from long term MSU derived tropospheric temperature trend (1979–2008), Ann. Geophys., 27, 4505–4519, https://doi.org/10.5194/angeo-27-4505-2009, 2009.
Qian, Y., Gong, D., Fan, J., Ruby Leung, L., Bennartz, R., Chen, D., and Wang, W.: Heavy pollution suppresses light rain in China: Observations and modeling, J. Geophys. Res.-Atmos., 114, D00K02, https://doi.org/10.1029/2008JD011575, 2009.
Qian, Y., Flanner, M. G., Leung, L. R., and Wang, W.: Sensitivity studies on the impacts of Tibetan Plateau snowpack pollution on the Asian hydrological cycle and monsoon climate, Atmos. Chem. Phys., 11, 1929–1948, https://doi.org/10.5194/acp-11-1929-2011, 2011.
Qian, Y., Wang, H., Zhang, R., Flanner, M. G., and Rasch, P. J.: A sensitivity study on modeling black carbon in snow and its radiative forcing over the Arctic and Northern China, Environ. Res. Lett., 9, 064001, https://doi.org/10.1088/1748-9326/9/6/064001, 2014.
Qian, Y., Yasunari, T. J., Doherty, S. J., Flanner, M. G., Lau, W. K. M., Ming, J., Wang, H., Wang, M., Warren, S. G., and Zhang, R.: Light-absorbing particles in snow and ice: Measurement and modeling of climatic and hydrological impact, Adv. Atmos. Sci., 32, 64–91, https://doi.org/10.1007/s00376-014-0010-0, 2015.
Raatikainen, T., Hyvärinen, A. P., Hatakka, J., Panwar, T. S., Hooda, R. K., Sharma, V. P., and Lihavainen, H.: The effect of boundary layer dynamics on aerosol properties at the Indo-Gangetic plains and at the foothills of the Himalayas, Atmos. Environ., 89, 548–555, https://doi.org/10.1016/j.atmosenv.2014.02.058, 2014.
Ramanathan, V. and Carmichael, G.: Global and regional climate changes due to black carbon, Nat. Geosci., 1, 221–227, https://doi.org/10.1038/ngeo156, 2008.
Ramanathan, V., Crutzen, P. J., Kiehl, J. T., and Rosenfeld, D.: Aerosol, climate and the hydrological cycle, Science, 294, 2119–2124, 2001.
Ramanathan, V., Ramana, M. V., Roberts, G., Kim, D., Corrigan, C., Chung, C., and Winker, D.: Warming trends in Asia amplified by brown cloud solar absorption, Nature, 448, 575–578, https://doi.org/10.1038/nature06019, 2007.
Ren, D. and Karoly, D.: Comparison of glacier-inferred temperatures with observations and climate model simulations, Geophys. Res. Lett., 33, L23710, https://doi.org/10.1029/2006GL027856, 2006.
Ren, J., Jing, Z., Pu, J., and Qin, X.: Glacier variations and climate change in the central Himalaya over the past few decades, Ann. Glaciol., 43, 218–222, 2006.
Rittger, K., Painter, T. H., and Dozier, J.: Assessment of methods for mapping snow cover from MODIS, Adv. Water Resour., 51, 367–380, https://doi.org/10.1016/j.advwatres.2012.03.002, 2013.
Rittger, K., Bair, E. H., Kahl, A., and Dozier, J.: Spatial estimates of snow water equivalent from reconstruction, Adv. Water Resour., 94, 345–363, https://doi.org/10.1016/j.advwatres.2016.05.015, 2016.
Santra, S., Verma, S., Fujita, K., Chakraborty, I., Boucher, O., Takemura, T., Burkhart, J. F., Matt, F., and Sharma, M.: Simulations of black carbon (BC) aerosol impact over Hindu Kush Himalayan sites: validation, sources, and implications on glacier runoff, Atmos. Chem. Phys., 19, 2441–2460, https://doi.org/10.5194/acp-19-2441-2019, 2019.
Sarangi, C., Tripathi, S. N., Kanawade, V. P., Koren, I., and Pai, D. S.: Investigation of the aerosol–cloud–rainfall association over the Indian summer monsoon region, Atmos. Chem. Phys., 17, 5185–5204, https://doi.org/10.5194/acp-17-5185-2017, 2017.
Schmale, J., Flanner, M., Kang, S., Sprenger, M., Zhang, Q., Guo, J., Li, Y., Schwikowski, M., and Farinotti, D.: Modulation of snow reflectance and snowmelt from Central Asian glaciers by anthropogenic black carbon, Sci. Rep. UK, 7, 40501, https://doi.org/10.1038/srep40501, 2017.
Shrestha, A. B., Wake, C. P., Mayewski, P. A., and Dibb, J. E.: Maximum temperature trends in the Himalaya and its vicinity: An analysis based on temperature records from Nepal for the period 1971–94, J. Climate, 12, 2775–2786, https://doi.org/10.1175/1520-0442(1999)012<2775:MTTITH>2.0.CO;2, 1999.
Simard, M., Pinto, N., Fisher, J. B., and Baccini, A.: Mapping forest canopy height globally with spaceborne lidar, J. Geophys. Res.-Biogeo., 116, G04021, https://doi.org/10.1029/2011JG001708, 2011.
Singh, P. and Bengtsson, L.: Hydrological sensitivity of a large Himalayan basin to climate change, Hydrol. Process., 18, 2363–2385, https://doi.org/10.1002/hyp.1468, 2004.
Skiles, S. M., Painter, T. H., Deems, J. S., Bryant, A. C., and Landry, C. C.: Dust radiative forcing in snow of the Upper Colorado River Basin: 2. Interannual variability in radiative forcing and snowmelt rates, Water Resour. Res., 48, W07522, https://doi.org/10.1029/2012WR011986, 2012.
Skiles, S. M., Painter, T. H., Belnap, J., Holland, L., Reynolds, R. L., Goldstein, H. L., and Lin, J.: Regional variability in dust-on-snow processes and impacts in the Upper Colorado River Basin, Hydrol. Process., 29, 5397–5413, https://doi.org/10.1002/hyp.10569, 2015.
Skiles, S. M. K. and Painter, T.: Daily evolution in dust and black carbon content, snow grain size, and snow albedo during snowmelt, Rocky Mountains, Colorado, J. Glaciol., 63, 118–132, https://doi.org/10.1017/jog.2016.125, 2017.
Svensson, J., Ström, J., Kivekäs, N., Dkhar, N. B., Tayal, S., Sharma, V. P., Jutila, A., Backman, J., Virkkula, A., Ruppel, M., Hyvärinen, A., Kontu, A., Hannula, H.-R., Leppäranta, M., Hooda, R. K., Korhola, A., Asmi, E., and Lihavainen, H.: Light-absorption of dust and elemental carbon in snow in the Indian Himalayas and the Finnish Arctic, Atmos. Meas. Tech., 11, 1403–1416, https://doi.org/10.5194/amt-11-1403-2018, 2018.
Thind, P. S., Chandel, K. K., Sharma, S. K., Mandal, T. K., and John, S.: Light-absorbing impurities in snow of the Indian Western Himalayas: impact on snow albedo, radiative forcing, and enhanced melting, Environ. Sci. Pollut. Res. Int., 26, 7566–7578, https://doi.org/10.1007/s11356-019-04183-5, 2019.
Toon, O. B., McKay, C. P., Ackerman, T. P., and Santhanam, K.: Rapid calculation of radiative heating rates and photodissociation rates in inhomogeneous multiple scattering atmospheres, J. Geophys. Res., 94, 16287, https://doi.org/10.1029/JD094iD13p16287, 1989.
Tripathi, S. N., Dey, S., Chandel, A., Srivastava, S., Singh, R. P., and Holben, B. N.: Comparison of MODIS and AERONET derived aerosol optical depth over the Ganga Basin, India, Ann. Geophys., 23, 1093–1101, https://doi.org/10.5194/angeo-23-1093-2005, 2005.
van der Werf, G. R., Randerson, J. T., Giglio, L., Collatz, G. J., Mu, M., Kasibhatla, P. S., Morton, D. C., DeFries, R. S., Jin, Y., and van Leeuwen, T. T.: Global fire emissions and the contribution of deforestation, savanna, forest, agricultural, and peat fires (1997–2009), Atmos. Chem. Phys., 10, 11707–11735, https://doi.org/10.5194/acp-10-11707-2010, 2010.
Wake, C. P., Mayewski, P. A., Li, Z., Han, J., and Qin, D.: Modern eolian dust deposition in central Asia, Tellus B, 46, 220–233, https://doi.org/10.3402/tellusb.v46i3.15793, 1994.
Wang, B., Bao, Q., Hoskins, B., Wu, G., and Liu, Y.: Tibetan Plateau warming and precipitation changes in East Asia, Geophys. Res. Lett., 35, L14702, https://doi.org/10.1029/2008GL034330, 2008.
Wang, M., Xu, B., Cao, J., Tie, X., Wang, H., Zhang, R., Qian, Y., Rasch, P. J., Zhao, S., Wu, G., Zhao, H., Joswiak, D. R., Li, J., and Xie, Y.: Carbonaceous aerosols recorded in a southeastern Tibetan glacier: analysis of temporal variations and model estimates of sources and radiative forcing, Atmos. Chem. Phys., 15, 1191–1204, https://doi.org/10.5194/acp-15-1191-2015, 2015.
Warren, S. G.: Can black carbon in snow be detected by remote sensing?, J. Geophys. Res.-Atmos., 118, 779–786, https://doi.org/10.1029/2012JD018476, 2013.
Warren, S. G. and Wiscombe, W. J.: A Model for the Spectral Albedo of Snow. II: Snow Containing Atmospheric Aerosols, J. Atmos. Sci., 37, 2734–2745, https://doi.org/10.1175/1520-0469(1980)037<2734:AMFTSA>2.0.CO;2, 1980.
Xu, B., Yao, T., Liu, X., and Wang, N.: Elemental and organic carbon measurements with a two-step heating-gas chromatography system in snow samples from the Tibetan Plateau, Ann. Glaciol., 43, 257–262, https://doi.org/10.3189/172756406781812122, 2006.
Xu, B., Cao, J., Hansen, J., Yao, T., Joswia, D. R., Wang, N., Wu, G., Wang, M., Zhao, H., Yang, W., Liu, X., and He, J.: Black soot and the survival of Tibetan glaciers, P. Natl. Acad. Sci. USA, 106, 22114–22118, https://doi.org/10.1073/pnas.0910444106, 2009.
Xu, J., Grumbine, R. E., Shrestha, A., Eriksson, M., Yang, X., Wang, Y., and Wilkes, A.: The melting Himalayas: Cascading effects of climate change on water, biodiversity, and livelihoods, Conserv. Biol., 23, 520–530, https://doi.org/10.1111/j.1523-1739.2009.01237.x, 2009.
Yao, T., Pu, J., Lu, A., Wang, Y., and Yu, W.: Recent Glacial Retreat and Its Impact on Hydrological Processes on the Tibetan Plateau, China, and Surrounding Regions, Arctic, Antarct. Alp. Res., 39, 642–650, https://doi.org/10.1657/1523-0430(07-510)[YAO]2.0.CO;2, 2007.
Yasunari, T. J., Bonasoni, P., Laj, P., Fujita, K., Vuillermoz, E., Marinoni, A., Cristofanelli, P., Duchi, R., Tartari, G., and Lau, K.-M.: Estimated impact of black carbon deposition during pre-monsoon season from Nepal Climate Observatory – Pyramid data and snow albedo changes over Himalayan glaciers, Atmos. Chem. Phys., 10, 6603–6615, https://doi.org/10.5194/acp-10-6603-2010, 2010.
Yasunari, T. J., Tan, Q., Lau, K. M., Bonasoni, P., Marinoni, A., Laj, P., Ménégoz, M., Takemura, T., and Chin, M.: Estimated range of black carbon dry deposition and the related snow albedo reduction over Himalayan glaciers during dry pre-monsoon periods, Atmos. Environ., 78, 259–267, https://doi.org/10.1016/j.atmosenv.2012.03.031, 2013.
Zaveri, R. A. and Peters, L. K.: A new lumped structure photochemical mechanism for large-scale applications, J. Geophys. Res.-Atmos., 104, 30387–30415, https://doi.org/10.1029/1999JD900876, 1999.
Zaveri, R. A., Easter, R. C., Fast, J. D., and Peters, L. K.: Model for Simulating Aerosol Interactions and Chemistry (MOSAIC), J. Geophys. Res.-Atmos., 113, https://doi.org/10.1029/2007JD008782, 2008.
Zhang, Q., Streets, D. G., Carmichael, G. R., He, K. B., Huo, H., Kannari, A., Klimont, Z., Park, I. S., Reddy, S., Fu, J. S., Chen, D., Duan, L., Lei, Y., Wang, L. T., and Yao, Z. L.: Asian emissions in 2006 for the NASA INTEX-B mission, Atmos. Chem. Phys., 9, 5131–5153, https://doi.org/10.5194/acp-9-5131-2009, 2009.
Zhang, R., Wang, H., Qian, Y., Rasch, P. J., Easter, R. C., Ma, P.-L., Singh, B., Huang, J., and Fu, Q.: Quantifying sources, transport, deposition, and radiative forcing of black carbon over the Himalayas and Tibetan Plateau, Atmos. Chem. Phys., 15, 6205–6223, https://doi.org/10.5194/acp-15-6205-2015, 2015.
Zhang, Y., Kang, S., Sprenger, M., Cong, Z., Gao, T., Li, C., Tao, S., Li, X., Zhong, X., Xu, M., Meng, W., Neupane, B., Qin, X., and Sillanpää, M.: Black carbon and mineral dust in snow cover on the Tibetan Plateau, The Cryosphere, 12, 413–431, https://doi.org/10.5194/tc-12-413-2018, 2018.
Zhao, C., Liu, X., Ruby Leung, L., and Hagos, S.: Radiative impact of mineral dust on monsoon precipitation variability over West Africa, Atmos. Chem. Phys., 11, 1879–1893, https://doi.org/10.5194/acp-11-1879-2011, 2011.
Zhao, C., Chen, S., Leung, L. R., Qian, Y., Kok, J. F., Zaveri, R. A., and Huang, J.: Uncertainty in modeling dust mass balance and radiative forcing from size parameterization, Atmos. Chem. Phys., 13, 10733–10753, https://doi.org/10.5194/acp-13-10733-2013, 2013a.
Zhao, C., Leung, L. R., Easter, R., Hand, J., and Avise, J.: Characterization of speciated aerosol direct radiative forcing over California, J. Geophys. Res.-Atmos., 118, 2372–2388, https://doi.org/10.1029/2012JD018364, 2013b.
Zhao, C., Hu, Z., Qian, Y., Ruby Leung, L., Huang, J., Huang, M., Jin, J., Flanner, M. G., Zhang, R., Wang, H., Yan, H., Lu, Z., and Streets, D. G.: Simulating black carbon and dust and their radiative forcing in seasonal snow: a case study over North China with field campaign measurements, Atmos. Chem. Phys., 14, 11475–11491, https://doi.org/10.5194/acp-14-11475-2014, 2014.