the Creative Commons Attribution 4.0 License.
the Creative Commons Attribution 4.0 License.
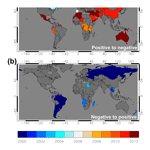
Trends and trend reversal detection in 2 decades of tropospheric NO2 satellite observations
Aristeidis K. Georgoulias
Ronald J. van der A
Piet Stammes
K. Folkert Boersma
Henk J. Eskes
In this work, a ∼21-year global dataset from four different satellite sensors with a mid-morning overpass (GOME/ERS-2, SCIAMACHY/ENVISAT, GOME-2/Metop-A, and GOME-2/Metop-B) is compiled to study the long-term tropospheric NO2 patterns and trends. The Global Ozone Monitoring Experiment (GOME) and GOME-2 data are “corrected” relative to the SCanning Imaging Absorption spectroMeter for Atmospheric CHartographY (SCIAMACHY) data to produce a self-consistent dataset that covers the period April 1996–September 2017. The highest tropospheric NO2 concentrations are seen over urban, industrialized, and highly populated areas and over ship tracks in the oceans. Tropospheric NO2 has generally decreased during the last 2 decades over the industrialized and highly populated regions of the western world (a total decrease of the order of ∼49 % over the US, the Netherlands, and the UK; ∼36 % over Italy and Japan; and ∼32 % over Germany and France) and increased over developing regions (a total increase of ∼160 % over China and ∼33 % over India). It is suggested here that linear trends cannot be used efficiently worldwide for such long periods. Tropospheric NO2 is very sensitive to socioeconomic changes (e.g., environmental protection policies, economic recession, warfare, etc.) which may cause either short-term changes or even a reversal of the trends. The application of a method capable of detecting the year when a reversal of trends happened shows that tropospheric NO2 concentrations switched from positive to negative trends and vice versa over several regions around the globe. A country-level analysis revealed clusters of countries that exhibit similar positive-to-negative or negative-to-positive trend reversals, while 29 out of a total of 64 examined megacities and large urban agglomerations experienced a trend reversal at some point within the last 2 decades.
- Article
(22551 KB) - Full-text XML
-
Supplement
(19584 KB) - BibTeX
- EndNote
Nitrogen dioxide (NO2) constitutes one of the most important air pollutants in the atmosphere, being responsible for the air quality degradation in many regions across the Earth. It plays a major role in a number of processes in the troposphere, such as the photochemical production of ozone (O3) and the formation of nitric acid (HNO3; Seinfeld and Pandis, 2016, and references therein) and the formation of nitrate aerosols (Basset and Seinfeld, 1983) and modifies the radiative balance in the atmosphere either directly (by absorbing solar radiation; Solomon et al., 1999) or indirectly (e.g., by the formation of ozone or the modification of the greenhouse gases' lifetime such as that of methane; Isaksen et al., 2014). In addition, NO2 has a diverse effect on human health, being toxic at high concentrations. Long exposure to NO2 may lead to the development of asthma and increase susceptibility to respiratory infections (WHO, 2003).
As NO2 is largely produced by anthropogenic activities (e.g., transportation, industry, domestic heating, power plants, and smelters) it is mostly abundant in urban environments. A small part of the global NO2 concentrations is produced by natural sources such as biomass burning, lightning flashes, and soil microbial activity (Hilboll et al., 2013, and references therein). Socioeconomic changes from the beginning of the industrial revolution until today had a critical impact on the NO2 levels over various locations around the planet (Vestreng et al., 2009). At the same time, the continuous growth of the global population and its concentration into urban agglomerations (cities, megacities, and conurbations) led to the development of major NO2 hotspots which can be detected from space (Schneider et al., 2015).
It has been more than 2 decades now that a series of sensors aboard sun-synchronous orbit satellites has continuously measured the tropospheric NO2 vertical column density (VCD) at nearly the same time (Equator crossing time in mid-morning), offering global coverage at timescales ranging from 1–6 d. The first sensor to measure NO2 VCDs was the Global Ozone Monitoring Experiment (GOME; Burrows et al., 1999) aboard the European Space Agency's (ESA's) satellite ERS-2. GOME flew on a sun-synchronous near-polar orbit from mid-1995, delivering NO2 measurements at a nominal spatial resolution of 320 km × 40 km2 (Equator crossing time: 10:30 LT) until June 2003, when the ERS-2 tape recorder failed, leading to a very low global coverage. Until June 2003, daily coverage was achieved every 3 d. Except from the nominal GOME operation, narrow swath-mode measurements were taken for 3 d each month at a spatial resolution that was 4 times higher (80 km × 40 km2; Beirle et al., 2004). In this mode, global coverage was achieved using 12 d of data. Due to a saturation of the visible channels under certain circumstances during the first months of its operation, GOME had a smaller ground pixel (80 × 40 km2; worse ground coverage) until March 1996, when the problem was solved.
GOME was succeeded by the SCanning Imaging Absorption spectroMeter for Atmospheric CHartographY (SCIAMACHY; Burrows et al., 1995; Bovensmann et al., 1999) aboard the ESA's satellite ENVISAT. SCIAMACHY was on a sun-synchronous near-polar orbit, delivering NO2 measurements at a spatial resolution of 60 × 30 km2 (Equator crossing time: 10:00 LT) from August 2002 until April 2012, when contact was lost. The sensor's global coverage time was 6 d.
SCIAMACHY was succeeded by two GOME-2 satellite instruments (Munro et al., 2016) aboard Metop-A (October 2006) and Metop-B (September 2012) with morning Equator crossing times, which were developed by the ESA and are operated by the European Organisation for the Exploitation of Meteorological Satellites (EUMETSAT). GOME-2A flies on a sun-synchronous near-polar orbit with an Equator crossing time of 09:30 LT. Originally, GOME-2A had a 1920 km swath and a 80 × 40 km2 footprint covering the Earth once every 1.5 d. In July 2013 the GOME-2A swath was changed to 960 km, and its footprint was changed to 40 × 40 km2. GOME-2B shares the same characteristics of GOME-2A before July 2013 (Munro et al., 2016). GOME-2A delivers NO2 measurements from January 2007, and GOME-2B delivers NO2 measurements from January 2013 onwards.
The instruments mentioned above are different by means of their technical characteristics, calibration, and their spatial resolution, which makes the use of their observations as one single dataset very challenging. Several studies in the past made use of the tropospheric NO2 data from the aforementioned sensors simultaneously; however, in most cases, the datasets were either used separately (e.g., van der A et al., 2006; Valks et al., 2011; Schneider et al., 2012) or they were downgraded to a low spatial resolution (e.g., van der A et al., 2008; Konovalov et al., 2010). Some studies have suggested a method that accounts for the spatial resolution difference between GOME and SCIAMACHY observations (e.g., Konovalov et al., 2006, 2008) in order to preserve the high spatial resolution. On top of this correction, Hilboll et al. (2013) suggested a method that accounts for all the other instrumental differences, including instrument-dependent offsets in a fitted trend function. They applied their method on GOME, SCIAMACHY, OMI (Ozone Monitoring Instrument), and GOME-2A data. Geddes et al. (2016) followed a similar approach by applying a spatial resolution and a shift correction on data from GOME, SCIAMACHY, and GOME-2A sensors.
Here, we proceed to the compilation of a ∼21-year self-consistent dataset, using morning data from GOME/ERS-2, SCIAMACHY/ENVISAT, GOME-2/Metop-A, and GOME-2/Metop-B, by following a three-step procedure. It has to be noted that the OMI (Levelt et al., 2018) aboard the EOS Aura (2004–today) has also been measuring tropospheric NO2 since October 2004; however, its Equator crossing time is in the afternoon. Taking into account the fact that tropospheric NO2 is characterized by a significant diurnal variability (Boersma et al., 2008), the use of mixed morning and afternoon measurements might insert large uncertainties, and hence we decided to focus on morning measurements only. Details about the datasets, which are merged along with a description of the methodology followed, are given in Sect. 2. The joint dataset is used for a detailed global trend analysis (trend calculation method described in Sect. 2). The long-term tropospheric NO2 global patterns and trends are presented in Sect. 3.1. A method that detects trend reversals was developed (see Sect. 2) in order to show that a single linear trend cannot be used efficiently worldwide for such long periods. The method is applied to a global scale, on a country basis, and for a number of megacities and large urban agglomerations around the world, and the year of the reversal and the trends for the period before and after the reversals are reported (Sect. 3.2, 3.3, and 3.4). The coincidence of the trend reversals with different socioeconomic changes is also examined. At the end of the paper, the main findings and conclusions of this research are summarized.
2.1 Satellite data
In this work, we use tropospheric NO2 VCD data from the GOME (April 1996–June 2003), SCIAMACHY (July 2002–March 2012), GOME-2A (January 2007– September 2017), and GOME-2B (January 2013–September 2017) TM4NO2A v. 2.3 datasets, which are available from the Royal Netherlands Meteorological Institute (KNMI). The retrieval scheme consists of three steps. First, the NO2 slant column density (SCD) is retrieved, applying the differential optical absorption spectroscopy (DOAS) method (Platt, 1994), then the stratospheric and tropospheric contribution to the NO2 SCD is calculated with a data assimilation approach (Dirksen et al., 2011), and finally the tropospheric SCD is converted into tropospheric VCD using a calculated air mass factor (AMF; Boersma et al., 2004). With DOAS, the reflectance spectrum in the wavelength range from 425 to 450 nm, measured by the sensors, is fitted by a model that takes into account absorption cross sections for NO2, O3, O2-O2, and H2O and the Ring effect, while a low-order polynomial accounts for the scattering from aerosols and clouds and for the Rayleigh scattering (Vandaele et al., 2005). The separation between the stratospheric and tropospheric SCD is achieved by assimilating the total SCD retrieved with the DOAS into the TM4 chemistry transport model (Dirksen et al., 2011). The AMFs which are used in step 3 for the conversion of the tropospheric SCDs from step 2 into tropospheric VCDs are pre-calculated using the Doubling-Adding KNMI (DAK) radiative transfer model (Stammes, 2001). The retrieval scheme uses satellite-based surface albedo climatological data (Boersma et al., 2004), while cloud fraction and cloud top height is retrieved using the FRESCO algorithm (Koelemeijer et al., 2001). Specifically, the TM4NO2A v. 2.3 dataset used here is the result of a major update that was implemented during the switch from TM4NO2A v. 1.1 to TM4NO2A v. 2.0 (new altitude-dependent AMF lookup table, a more realistic surface albedo dataset from MERIS sensor aboard the ENVISAT satellite, an improved terrain height dataset, and better sampling of TM4 profiles) and the correction of minor retrieval errors thereafter (details in Boersma et al., 2011, and on TEMIS website: http://www.temis.nl/index.php, last access: 19 March 2019). Single-pixel GOME, SCIAMACHY, GOME-2A, and GOME-2B retrievals were attributed to a standard grid of , and the observations were averaged on a monthly basis. When averaging, each observation is weighted by its fractional area (%) within the grid cell. For each valid observation, the cloud radiance fraction has to be less than 50 % (cloud fraction less than about 20 %) and the surface albedo not higher than 0.3, while observations with a solar zenith angle higher than 80∘ are filtered out. In addition, there is no limitation to the number of observations used, negative columns are taken into account, and the observational error is ignored in the averaging process (e.g., Schneider et al., 2015).
2.2 Methodology
In order to produce a self-consistent tropospheric NO2 VCD monthly gridded dataset from GOME, SCIAMACHY, GOME-2A, and GOME-2B for the period March 1996 to September 2017, we followed a three-step methodology based on the methods of Hilboll et al. (2013) and Geddes et al. (2016). A basic difference to Hilboll et al. (2013) is that we first produced a self-consistent dataset applying all the necessary corrections, and then we proceeded to a trend analysis instead of fitting part of the corrections during the trend analysis. In addition, the trend analysis is applied to monthly data instead of annual data, contrary to Geddes et al. (2016). The SCIAMACHY dataset was used as a reference, as SCIAMACHY shared common periods of measurements with both GOME and GOME-2A.
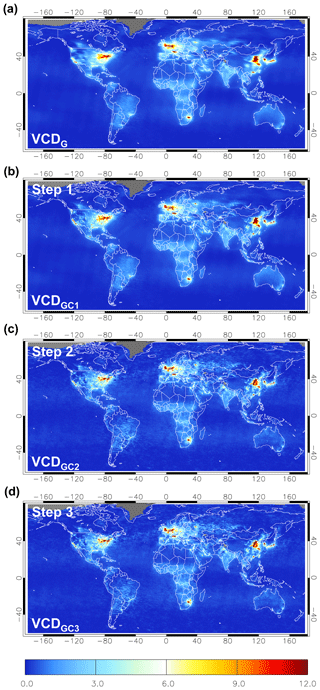
Figure 1GOME tropospheric NO2 VCD (in 1015 molecules cm−2) patterns for the whole GOME period from the original VCDG data (a), from the VCDGC1 data corrected in step 1 (b), from the VCDGC2 data corrected in step 2 (c), and from the VCDGC3 corrected in step 3 data (d).
The GOME data were first corrected for the low horizontal resolution they exhibit relative to SCIAMACHY (320 × 40 km2 vs. 60 × 30 km2; step 1; see also Hilboll et al., 2013). Of course the grid cell size is the same () for the GOME and SCIAMACHY monthly datasets; however, this does not impact the fact that the information included in a larger swath corresponds to a larger area. Hence, the gridded data which are produced from larger pixels (320 × 40 km2 for GOME) will be of “lower resolution” than the ones produced from smaller pixels (60 × 30 km2 for SCIAMACHY), and the resulting maps will be much smoother. As the GOME nominal resolution is nearly 3 times lower than that of SCIAMACHY at the horizontal dimension, following the reasoning of Geddes et al. (2016), we may assume that each grid cell of the GOME gridded dataset will correspond to an area nearly 3 times larger. Hence, in step 1, the SCIAMACHY monthly gridded VCD data (VCDSC) were first smoothed in the horizontal dimension in order to match GOME's horizontal resolution. This was achieved by using a boxcar algorithm with an averaging window of (3.25∘) in longitudinal direction (Eq. 1) similarly to Geddes et al. (2016). The correction is applied in the horizontal dimension only as the along-track dimensions are close in the two datasets (40 km vs. 30 km) and are also close to the latitudinal dimension of the gridded dataset (0.25∘). Then climatological monthly values for the full 9-year period (January 2003–December 2011) were calculated from the original and the smoothed SCIAMACHY (VCDSCsm) dataset on a grid cell basis (Eq. 2). The ratio of the original and the smoothed climatological values is defined as spatial resolution climatological correction factor (CF1: 1 value for each month of the year for a total of 12 values for each grid cell; see also Hilboll et al., 2013). To avoid having unreasonably large CF1 values due to very low tropospheric NO2 levels, CF1 was set equal to 1 in cases of VCDSCsm values lower than 0.1×1015 molecules cm−2, which corresponds to SCIAMACHY's precision. The total and the monthly mean CF1 values for the whole globe as well as for North America, Europe, and southeastern Asia can be seen in Figs. S1–S8 in the Supplement. CF1 is unitless and exhibits characteristic spatial patterns with values greater than and lower than 1 over and adjacent to pollution hotspots, respectively. The CF1 patterns are pretty persistent throughout the year. The original GOME gridded data (VCDG) were multiplied with the corresponding CF1 values to produce a GOME dataset (VCDGC1) with apparently higher spatial resolution (see Eq. 3). This method generally assumes that the relative spatial structure of the central dataset (SCIAMACHY) persists during the GOME period. The VCDG and VCDGC1 patterns for the whole GOME period are shown in Fig. 1a and b, respectively:
where x and y are the central longitude and latitude of a grid cell (in degrees) and t is the time in 1-month steps (from January 2003 to December 2011), while , (a total of 13 values), and
where is the month for which the climatological monthly values VCD) and VCD) are calculated, and
The different spatial resolution leads to different spatial and temporal sampling by the two instruments, which affects the observed NO2 levels, the seasonal variability, and its amplitude. The spatial resolution correction is expected to correct only part of those biases, and hence further corrections were applied. Following Hilboll et al. (2013), who used a trend model that explicitly accounts for a level shift between the two instruments and for a change in the amplitude of the seasonal variation, we applied a shift correction (step 2) and a seasonal amplitude correction (step 3) successively, on top of the spatial resolution correction (step 1). More specifically, the corrected GOME data (VCDGC1) for the 11-month GOME–SCIAMACHY common period of August 2002–June 2003 were compared against SCIAMACHY data (VCDsc) for the same period, and a shift correction was further applied to account for the instrumental bias between the two sensors (step 2). The shift correction factor (CF2: 1 value for each grid cell) is equal to the difference between the two datasets for the common period and was calculated on a grid cell basis (Eq. 4), similarly to Geddes et al. (2016). CF2 (in 1015 molecules cm−2) takes higher positive and negative values over several pollution hotspots (absolute values higher than 0.5), showing that further corrections should be applied to the data. The global CF2 patterns as well as the corresponding CF2 values for North America, Europe, and southeastern Asia are shown in Figs. S9–S12. CF2 was added to the GOME data corrected by the spatial resolution to produce a further corrected GOME dataset (VCDGC2; Eq. 5). The VCDGC2 patterns for the whole GOME period are shown in Fig. 1c:
where t is the time in 1-month steps for the common GOME-SCIAMACHY period (t1: August 2002 to t2: June 2003) of n=11 months, and
Finally, the GOME data were brought closer to the SCIAMACHY data by applying a correction for the different seasonal amplitudes that may still exist after the first two corrections (step 3). The normalized-to-long-term-average seasonal variability (climatological monthly values were divided by the long-term average) of the SCIAMACHY data (VCDsc) for the whole SCIAMACHY period is divided by the normalized-to-long-term-average seasonal variability of the twice-corrected GOME data (VCDGC2) for the whole GOME period. The seasonal amplitude correction factor (CF3: 1 value for each month of the year for a total of 12 values for each grid cell) is equal to the ratio of the SCIAMACHY and GOME normalized seasonal variability, and it is unitless (Eq. 6). Like in the case of CF1, to avoid having unreasonably large values due to very low tropospheric NO2 levels, CF3 was set equal to 1 for grid cells with VCDSC or VCDGC2 levels lower than 0.1×1015 molecules cm−2. The total and the monthly mean CF3 values for the whole globe as well as for North America, Europe, and southeastern Asia can be seen in Figs. S13–S20. The CF3 patterns are pretty patchy and cannot be connected to areas with low or high tropospheric NO2, contrary to the case of CF1 and CF2. The already twice-corrected GOME data (VCDGC2) were multiplied with the CF3 on a monthly basis to produce the final GOME dataset (VCDGC3; see Eq. 7). The VCDGC3 patterns for the whole GOME period are shown in Fig. 1d. As SCIAMACHY and GOME-2 have a comparable spatial resolution, in the case of GOME-2 data, step 1 was omitted. The GOME-2A and 2B data were averaged on a monthly basis to produce a common GOME-2 dataset, which is then corrected following steps 2 and 3. The GOME-2A and GOME-2B data were assumed to be of equal quality and resolution in the averaging process:
where t is the time in 1-month steps for the whole SCIAMACHY period (tSC1: August 2002 to tSC2: March 2012) of nSC=116 months and for the whole GOME period (tG1: April 1996 to tG2: June 2003) of nG=87 months, and
The self-consistent GOME–SCIAMACHY–GOME-2 time series were fitted by using a model with a linear trend and a Fourier-based seasonal component (see Eqs. 8 and 9). The method is based on Weatherhead et al. (1998) and has been frequently used in previous studies to calculate the trends of trace gases, aerosols, surface solar radiation, etc. (e.g., van der A et al., 2008; De Smedt et al., 2010; de Meij et al., 2012; Pozzer et al., 2015; Georgoulias et al., 2016; Alexandri et al., 2017) and check whether they are statistically significant at the 95 % confidence level (Eq. 10). Due to the systematic lack of valid tropospheric NO2 retrievals (due to clouds, snow and ice cover, etc.), especially over areas at high latitudes, only trends calculated for time series with at least 8 months per year are considered reliable and hence are shown (see also Pozzer et al., 2015):
where Yt is the monthly mean value for month t, Xt is the number of the month after the first month of the time series, A is the monthly mean of the first month of the time series, and B is the trend. The seasonal component contains the amplitudes an and bn; T is the period and Nt is the difference between the modeled and the measured value, usually defined as the remainder:
where φ is the autocorrelation in the remainder and εt is the white noise. Autocorrelation φ affects the precision of the trend σB, which is given as a function of φ, the length of the dataset in years m, and the variance σN of the remainder for small autocorrelations:
The calculated trend B is considered to be statistically significant at the 95 % confidence level if .
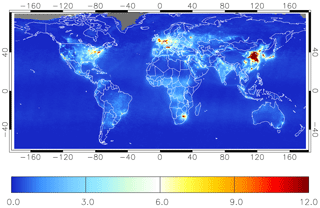
Figure 2Tropospheric NO2 VCD (in 1015 molecules cm−2) patterns as seen using the self-consistent GOME, SCIAMACHY, and GOME-2 dataset for the combined period April 1996–September 2017.
In order to detect trend reversals in the self-consistent GOME–SCIAMACHY–GOME-2 time series, a method similar to the one that was originally suggested in a solar dimming and brightening study (Cermak et al., 2010) was used. The method is capable of finding the year when a reversal from positive to negative trends or from negative to positive trends happened, with a very limited error of 0.5 %–1 %. The trend reversal method is based on the minimization of a value S(t), which is calculated for each year t of the period [t1=2000, tn=2012]:
where p(Bl) and p(Br) express the probability that the trends Bl and Br for the short periods on the left [] and on the right [] of the year t are statistically insignificant (1-significance level of the trend), and is the standard error of the trend for the combined sub-periods []. We use a time window of 4 in our calculations so that each trend is calculated for at least 5 years, and hence we can search for a trend reversal only within the period 2000–2012. The year tr, when S takes its lower value and there is a switch from a positive trend to a negative trend or from a negative trend to a positive trend, is considered to be a potential trend reversal year. The trends with the corresponding significance levels and probabilities are calculated here using least-squares linear regression (TREND function in IDL). In this study, only when the trend Bb for the whole period before tr (including tr) [] or the trend Ba for the whole period after tr (including tr) [] is statistically significant at the 95 % confidence level, a trend reversal is reported. Specifically, for the four extended regions of interest, the country, and the megacities and large urban agglomeration analyses performed in this paper, the Bb and Ba trends are calculated from the monthly time series using the method presented in the previous paragraph (Eqs. 8, 9, and 10) in order to be consistent with the trends for the whole time period (April 1996–September 2017) which are reported in the paper.
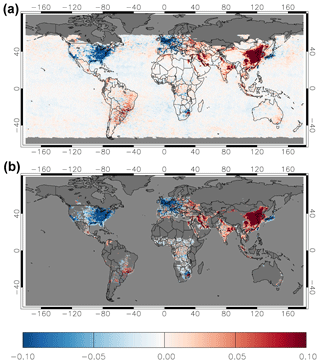
Figure 3(a) Satellite-based trends of tropospheric NO2 VCD (in 1015 molecules cm−2 yr−1) for the period April 1996–September 2017. Only trends calculated for time series with at least 8 months per year are taken into consideration; (b) same as (a) but for statistically significant trends at the 95 % confidence level and for grid cells with a long-term tropospheric NO2 VCD mean of at least 1×1015 molecules cm−2.
3.1 Long-term NO2 patterns and linear trends
The multi-year average tropospheric NO2 VCD patterns from the combined GOME-SCIAMACHY-GOME-2 dataset for the period April 1996–September 2017 are shown in Fig. 2. It is obvious that the highest NO2 concentrations are confined over urban, industrialized, and highly populated areas. A careful look over oceanic regions reveals local maxima over ship tracks (e.g., the Indian Ocean, Mediterranean Sea, and Red Sea), highlighting the contribution of ship emissions to the global NO2 burden. The highest tropospheric NO2 VCDs appear over an extended area located in eastern China. This area encloses the Beijing–Tianjin–Hebei (BTH) and the Yangtze River Delta (YRD) urban clusters, which have experienced an unprecedented population growth and a rapid industrial development over the last 2 decades (see Kourtidis et al., 2015; Krotkov et al., 2016; de Foy et al., 2016). Some very striking NO2 hotspots that can be seen on the map (red color) are the Pearl River Delta (PRD), southern China; Seoul, South Korea; Tokyo, Japan; Tehran, Iran; Moscow, Russia; the Highveld, South Africa; the Po Valley, northern Italy; the area covering the triangle of the Netherlands–Belgium–Germany; Paris, France; London, UK; New York, US; and others (see also van der A, 2008; Schneider et al., 2015; Krotkov et al., 2016 and references therein). Despite the fact that NO2 is transported from one region to another and that there is transboundary transport, due to the short NO2 lifetime (from a few hours up to a day), its concentrations are generally representative of the local NO2 emission strength.
During the last 2 decades various socioeconomic changes that impacted the local NO2 concentrations have taken place over different areas around the globe. In Fig. 3, the linear trends of the tropospheric NO2 VCD are shown in 1015 molecules cm−2 yr−1. More specifically, in Fig. 3a, all the grid cells are shown, while in Fig. 3b, only grid cells with statistically significant trends at the 95 % confidence level and a long-term tropospheric NO2 VCD mean of at least 1×1015 molecules cm−2 are shown, as in Schneider et al. (2012). To exclude the existence of a large systematic bias in the trends, a remote region located in the south of the Pacific Ocean (40–50∘ S, 130–150∘ W) with near-zero mean tropospheric NO2 levels ( molecules cm−2 for the period of interest) was examined. Indeed, a very low negative trend of molecules cm−2 yr−1 was found, which is well below SCIAMACHY's precision of 0.1×1015 molecules cm−2 (Hilboll et al., 2013) and can be considered negligible. Taking into account this and following previous studies, the trend values given in the paper are rounded to two decimal places, except for Tables 2 and 3 for intercomparison reasons between the various countries and megacities (see Sect. 3.3 and 3.4).
Strong statistically significant positive trends appear over extended areas in southeastern Asia (e.g., eastern China, India, Thailand, and Indonesia), the Middle East (e.g., Iraq, Iran, the Persian Gulf, and the eastern coast of the Red Sea), eastern Europe (e.g., the northern Balkans, the Black Sea, and the continental areas around it), northern Africa (e.g., regions around the Nile, Morocco, and northern Algeria), South Africa (the eastern part of the Highveld Plateau), South America (e.g., the region around Rio in Brazil, central Argentina, the region around Santiago in Chile, northern Colombia, and Venezuela), and central America (the region of Mexico City). The trend values over these areas are higher than 0.05×1015 molecules cm−2 yr−1, with a maximum value of 2.18×1015 molecules cm−2 yr−1 appearing within the BTH urban cluster in eastern China. On the contrary, strong statistically significant negative trends appear over the largest part of the US (especially the eastern US and the state of California), western and central Europe, Japan and Taiwan in southeastern Asia, and the region around the Johannesburg–Pretoria conurbation in South Africa. The absolute trend values over these areas are higher than 0.05×1015 molecules cm−2 yr−1, with a maximum trend of molecules cm−2 yr−1 appearing close to the city of Los Angeles in the western US.
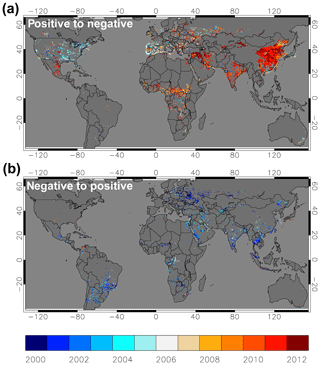
Figure 4Year of tropospheric NO2 VCD trend reversal from positive to negative (a) and from negative to positive (b). Only grid cells with a statistically significant trend at the 95 % confidence level for the period before or after the year of reversal and with a long-term tropospheric NO2 VCD mean of at least 1×1015 molecules cm−2 are shown.
In general, the trend patterns here resemble the ones appearing in previous satellite-based studies for shorter periods (e.g., van der A et al., 2008; Schneider et al., 2012; Hilboll et al., 2013; Krotkov et al., 2016). In conclusion, our results confirm that tropospheric NO2 concentrations have generally decreased during the last ∼21 years over the industrialized and highly populated regions of the so-called western world and increased over developing regions. Indicatively, according to the calculated trends, the tropospheric NO2 levels have decreased by ∼49 % during the whole period of interest (relative to the fitted mean of the first year) over the US, the Netherlands, and the UK; by ∼36 % over Italy and Japan; and by ∼32 % over Germany and France during this period and increased over regions like China (an average increase of ∼160 %, with an increase of 200 %–300 % over specific areas of eastern China) or India (∼33 %).
3.2 Trend reversals
As shown in the previous paragraph, the tropospheric NO2 linear trends during the last 2 decades appear to be strong and statistically robust over different regions around the world. However, it has been reported in previous studies that the implementation of environmental protection policies (e.g., van der A et al., 2017, for eastern China), economic recession (e.g., Castellanos and Boersma, 2012, for Europe; Vrekoussis et al., 2013, for Greece; and Cuevas et al., 2014, for Spain), warfare (e.g., Lelieveld et al., 2015, for the Middle East), and other events (e.g., Mijling et al., 2009, for the Beijing Olympic Games) may have led to temporal or persistent changes to trace gases' (e.g., SO2 and NO2) concentrations. This study mostly focuses on persistent changes which have led to a significant trend reversal of tropospheric NO2 at some point during the last ∼21 years. As shown in Fig. 4, trend reversals may indeed be detected over several regions around the globe. Figure 4a shows the year when a reversal from positive to negative trends started, and Fig. 4b shows the year when a reversal from negative to positive trends started. Only grid cells with a statistically significant trend at the 95 % confidence level for the period before or after the year of the reversal and a long-term tropospheric NO2 VCD mean of at least 1×1015 molecules cm−2 are shown.
Extended areas over eastern China exhibit a clear reversal from positive to negative trends, mostly in 2011 (see Fig. 4a), while the same areas are characterized by strong statistically significant positive trends in Fig. 3b. It becomes more than obvious that by using a linear trend model for the whole period of interest, one cannot depict the change in tropospheric NO2. A smaller area with a persistent reversal from positive to negative trends in 2012 appears in northwestern China. These striking features are in accordance with recent studies focusing on eastern Asia; van der A et al. (2017) showed that NOx emissions in eastern China reached a peak in 2012 and slowly decreased thereafter while the economy kept growing. Similar results were recently shown for aerosols (Sogacheva et al., 2018). This situation is attributed to the installation of NOx filtering systems at power plants and heavy industry. With the 12th 5-year plan (ChinaFAQs project, 2012), China set the target to reduce NOx emissions by 10 % during the period 2010–2015 and seems to have achieved it (de Foy et al., 2016). Selective catalytic reduction (SCR) systems were installed in this period, growing from a penetration of about 18 % (2011) to 86 % (2015; Liu et al., 2016). The use of SCR technology in power plants is expected to cause a reduction of the emissions by at least 70 % (ICAC, 2009). This is the most significant measure taken by the Chinese state and largely coincides with the reversal years appearing in Fig. 4a. In the meantime, China also introduced several new national emission standards for cars, switching from the China 3 to China 4 standard in 2011 (Wu et al., 2017). The maximum allowed amount of on-road-vehicle NOx emissions was reduced by 50 %. Stricter regulations were implemented on a city level for on-road vehicles (e.g., a ban on older polluting cars in Beijing). The approval of the first national environmental standard for limiting the concentrations of fine particles in the atmosphere by China's State Council accelerated the implementation of various measures after 2012, particularly over the urban clusters of BTH, YRD, and PRD (Zhao et al., 2013), which generally exhibit a trend reversal in 2011 (see Fig. 4a). The stricter and faster implementation of environmental policies in the capital city of Beijing and other key regions might explain the 1-year lag observed in the trend reversal over eastern China (2011) and northwestern China (2012; see Fig. 4a).
Similarly to eastern China, large parts of India experienced a reversal from positive to negative trends, mostly in 2011. On the contrary, areas in central–southern India experienced a reversal from negative to positive trends at some point in the period 2000–2006. India experienced a population growth of ∼37 % (relative to 1996) during the period 1996–2017, mostly in urban areas, which was accompanied by a gross domestic product (GDP) increase of ∼29 % (World Bank, 2019). NOx emissions generally increased as a result of large-scale urbanization (rural population decreased from ∼73 % of the total population in 1996 to ∼66 % in 2017), with industrialization and economic growth, energy production, industry, and transportation being the main contributors to the emissions (Ghude et al., 2013, and references therein). The Indian economy started developing at much higher rates after 2002 (World Bank, 2019), which might explain the observed negative-to-positive trend reversals appearing in the years 2000–2006 over specific areas (e.g., increase in tropospheric NO2 in the greater Ballari region due to the rapid growth of the steel industry, especially after 2006). India's economic growth experienced a slowdown after 2011 (GDP still increased but at a lower rate), which might explain part of the observed positive-to-negative trend reversals over specific areas. Hilboll et al. (2017) also observed a stagnation of tropospheric NO2 over India, attributing it to a combination of a slowdown in Indian economic development, the implementation of cleaner technology (e.g., Bansal and Bandivadekar, 2013), meteorological factors (see Voulgarakis et al., 2010), and changes in tropospheric chemistry. However, it has to be noted that the way in which all these parameters may influence the tropospheric NO2 levels and trends over India is pretty complicated and should be studied in more detail in the future.
Another region with widely spread positive-to-negative trend reversals is the Iberian Peninsula (see Fig. 4a). Not only the continental areas but also the coastal areas around the Iberian Peninsula (outside and inside the Mediterranean Basin) experienced this trend reversal, mostly during the period 2003–2007. We observe an early trend reversal over the Madrid and Valencia regions in Spain in the period 2000–2002, which is in accordance with NO2 ground concentration measurements. More specifically, in Cuevas et al. (2014), a continuous drop in surface NO2 is seen after 1999–2000 over the two cities. A reversal with a time lag of few years (2009–2011) is observed over areas in the communities of Extremadura and Catalonia. The observed differences are probably connected to the different economic and political characteristics of each area and the fact that the NO2 changes are driven by different reasons. The decline in the tropospheric NO2 levels in the first half of the 2000s when the economy was rising might be attributed to the implementation of environmental measures and the optimization of combustion processes (EEA-APFS-Spain., 2014). Following the European Union directives, Spain introduced its First National Emission Reduction Program in 2003, setting stringent combustion emission standards (IEA, 2017). This was updated and revised afterwards, leading to the Second National Emission Reduction Program in 2008. The decline in NO2 in the late 2000s to early 2010s might be due to the financial recession that started in 2008 (Cuevas et al., 2014). Similar differences are observed over areas in Portugal. For example, the areas around Santarém, in the northeast of Lisbon, exhibit a trend reversal in 2004–2005 (EEA-APFS-Portugal., 2014), while areas around other important cities, such as Evora and Coimbra, exhibit a trend reversal in the late 2000s to early 2010s, probably due to the 2008 financial recession.
The Middle East is another region with a persistent positive-to-negative trend reversal. Almost the whole of Syria (officially the Syrian Arab Republic) along with large parts of Iraq experienced a trend reversal during the period 2011–2012 (Fig. 4a) as a consequence of the Syrian Civil War, which broke out in 2011. Large parts of Iran experienced a similar trend reversal around 2011. This is mostly a result of the extension in 2010 of sanctions which were first imposed by the United Nations Security Council in 2006 (Lelieveld et al., 2015), while a decrease in transboundary transport of NO2 from neighboring countries due to the warfare cannot be ruled out. Similarly, oceanic and continental areas around the Persian Gulf experienced a trend reversal in 2011 or earlier. Lelieveld et al. (2015) attributed this to air quality control in the Persian Gulf states from the mid-2000s to late 2000s onwards. Within the Middle East, there are also sporadic areas (e.g., in Iran, Iraq, areas around the Persian Gulf, and areas around the eastern coast of the Red Sea in Saudi Arabia and the Nile River in Egypt) with a reversal from negative to positive trends in the early 2000s (2000–2003), probably due to changes in power generation and industrial, transport, and shipping emissions (Krotkov et al., 2016; Fig. 4b).
Extended areas with a persistent positive-to-negative trend reversal are also located in central Africa and Mexico (late 2000s) and in the US (early 2000s; Fig. 4a). On the contrary, areas with a persistent negative-to-positive trend reversal in the early 2000s can be seen in South America (highly populated, industrialized areas in Brazil and Argentina; Fig. 4b). The reversal points coincide with socioeconomic changes that took place in these two countries. Specifically, Argentina experienced a great economic depression during the period 1998–2002. The country's GDP declined by ∼11 % and the industrial production declined by ∼22 % in 2002 relative to 2001 (Cline, 2013), while the economy started reviving afterwards. Similarly, Brazil's GDP declined from 1997 to 2002, increased by a factor of ∼5 by 2011, and then declined again, reaching values close to those in 2009 in 2016 (World Bank, 2019). However, it has to be highlighted that in 2009, Brazil, specifically Rio de Janeiro (also known as Rio), won the bid to host the 2016 Olympic Games. This, despite the country's GDP decline, is expected to have given a boost to construction activities in Rio and the other host cities (São Paulo, Belo Horizonte, Salvador, Brasilia, and Manaus) and hence to NOx emissions. Indicative is the almost-uninterrupted increase in CO2 emissions from 2002 onwards (World Bank, 2019).
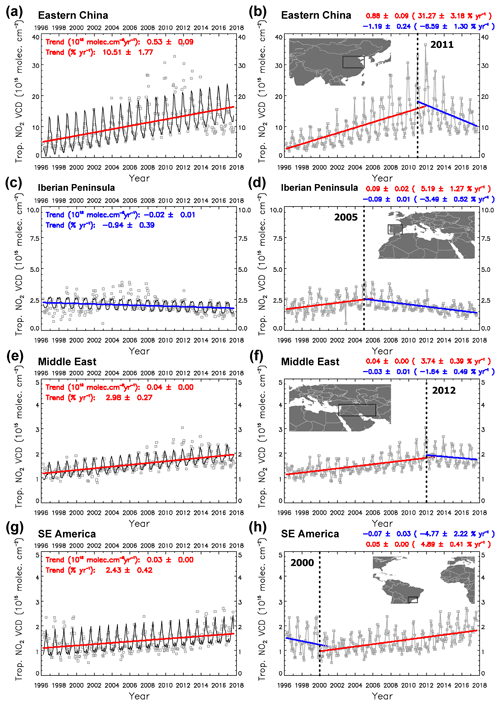
Figure 5(a, c, e, g) Satellite-based time series (grey points) of tropospheric NO2 VCD for the period April 1996–September 2017 for eastern China (a), the Iberian Peninsula (c), the Middle East (e), and southeastern America (g). The black line depicts the fitted time series, and the thick line depicts the trend (solid for statistically significant trends and dashed for statistically insignificant trends at the 95 % confidence level; red color for positive trends and blue color for negative trends). The absolute trends (in 1015 molecules cm−2 yr−1) and the trends relative to the fitted mean of the first year (in % yr−1) with the corresponding uncertainties (±1σ) are given in the plots. (b, d, f, h) Satellite-based time series (grey points and lines) of tropospheric NO2 VCD for the same regions. The thick lines, similarly to the left column panels, depict the trend for the sub-period before and the sub-period after the detected trend reversal (the year of reversal is included in both sub-periods). The year of the trend reversal is indicated with a black thick dotted line. The absolute trends and the trends relative to the fitted mean of the first year of the sub-periods (in parentheses) with the corresponding uncertainties are given in the plots (upper and lower lines correspond to the fist and the second sub-period, respectively). The regions of interest are also shown in the panels.
Table 1Absolute trends (in 1015 molecules cm−2 yr−1) and trends relative to the fitted mean of the first year (in % yr−1) with the corresponding uncertainties (±1σ) for the period April 1996–September 2017 for four regions of interest: ECH (eastern China), IPE (Iberian Peninsula), MEA (the Middle East), and SAM (southeastern America). The year when a trend reversal was detected and the absolute and relative trend for the sub-period before and the sub-period after the detected trend reversal are also given. The year of reversal is included in both sub-periods, while the relative trends are calculated relative to the fitted mean of each sub-period's first year. Bold characters are used to indicate the year of reversal and the statistically significant trends at the 95 % confidence level.
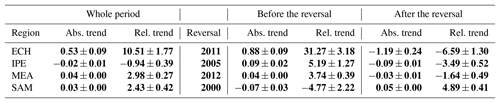
To demonstrate the need for a different approach when looking into long-term linear trends of tropospheric NO2, in Fig. 5, we present the time series and the trend for the whole period of measurements (April 1996–September 2017) and for the period before and after the trend reversal for four different regions of interest around the globe, i.e., eastern China (ECH; 30–40∘ N, 107–122∘ E), the Iberian Peninsula (IPE; 36–44∘ N, 10–0∘ W), the Middle East (MEA; 28–38∘ N, 34–60∘ E), and southeastern America (SAM; 29–19∘ S, 52–42∘ W; see also embedded maps in Fig. 5). The four regions were selected because they represent areas that experienced a trend reversal in different periods and for different reasons (see Fig. 5 and discussion above). The absolute (in 1015 molecules cm−2 yr−1) and relative trends (relative to the fitted mean of the period or sub-period first year; in % yr−1) for each region are given in Fig. 5 and Table 1.
Table 2The same as Table 1 but for countries. In order to save space, only countries that exhibit a trend reversal are shown here, while results for all the world countries are given in Table S1 of the Supplement. Bold characters are used to indicate the year of reversal and the statistically significant trends at the 95 % confidence level.
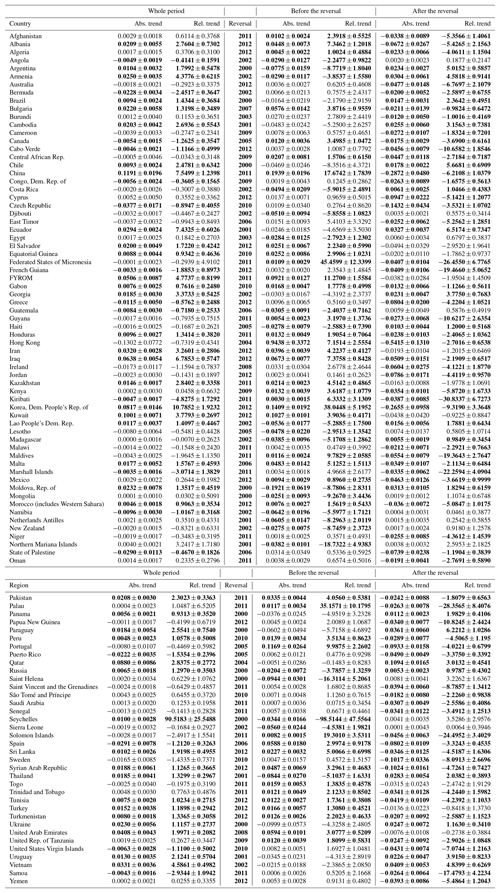
While ECH exhibits a statistically significant positive trend for the whole period of interest, a clear trend reversal is observed in 2011, with a statistically significant positive trend during the period April 1996–December 2011 and a statistically significant negative trend during the period January 2011–September 2017 (Fig. 5a, b). Following the discussion above, the observed trend reversal in ECH may be attributed to the implementation of environmental protection policies. In addition, while IPE exhibits a statistically significant negative trend for the whole period (Fig. 5c), a clear trend reversal is observed in 2005, with a statistically significant positive trend during the period April 1996–December 2005 and a statistically significant negative trend during the period January 2005–September 2017 (Fig. 5d). The 2005 trend reversal in IPE might be attributed to a combination of environmental measures and optimization in combustion processes. Similarly to ECH, MEA exhibits a statistically significant positive trend for the whole period of interest, with a clear trend reversal in 2012 (Fig. 5e). A statistically significant positive trend is observed during the period April 1996–December 2012 and a statistically significant negative trend during the period January 2012–September 2017 (Fig. 5f) which is attributed to the war that takes place in the area since 2011. Finally, SAM exhibits a statistically significant positive trend for the whole period of interest. A clear trend reversal is observed in 2000 with a statistically significant negative trend during the period April 1996–December 2000 and a statistically significant positive trend during the period January 2000–September 2017. The trend reversal in SAM might be attributed to a revival of the economy after ∼2000 in combination with the preparations for the Rio Olympic Games (see discussion above).
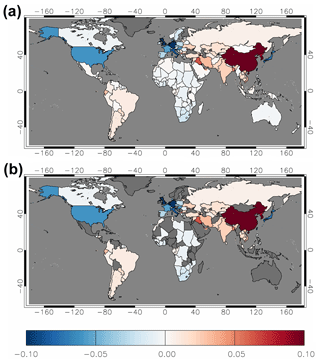
Figure 6(a) Satellite-based trends of tropospheric NO2 VCD (in 1015 molecules cm−2 yr−1) on a country basis for the period April 1996–September 2017. Only trends calculated for time series with at least 8 months per year are taken into consideration; (b) same as (a) but for statistically significant trends at the 95 % confidence level.
3.3 Countries
The same analysis was repeated on a country-level basis, which allows for safer interpretations of the observed trend reversals, as the environmental policies, the socioeconomic changes, and consequently NOx emission changes are unique within each country. As country-level averages are used here, there is no discrimination between national hotspots and background areas, while transboundary transport cannot be excluded as well. In Fig. 6a, the linear trend of the tropospheric NO2 VCD (in 1015 molecules cm−2 yr−1) for the period April 1996–September 2017 is shown for each country. In Fig. 6b, only countries with a statistically significant trend at the 95 % confidence level are shown. In line with Fig. 3, the US and Canada in North America, countries in central and western Europe (the UK, Spain, France, Switzerland, Belgium, the Netherlands, Germany, Denmark, Poland, the Czech Republic, Slovakia, Hungary, Romania, Italy, Slovenia, and Croatia), Japan and Taiwan in southeastern Asia, and several countries in Africa (Libya, Chad, Sudan – the region encompassing both the Republic of the Sudan and South Sudan, Ethiopia, Mali, Guinea, Liberia, Côte d'Ivoire, Ghana, the Democratic Republic of the Congo, Angola, Namibia, Botswana, Zimbabwe, Mozambique, and South Africa) exhibit a statistically significant negative trend. On the contrary, statistically significant positive trends can be seen over countries in eastern Europe and the Middle East and over almost the whole of Asia and South America.
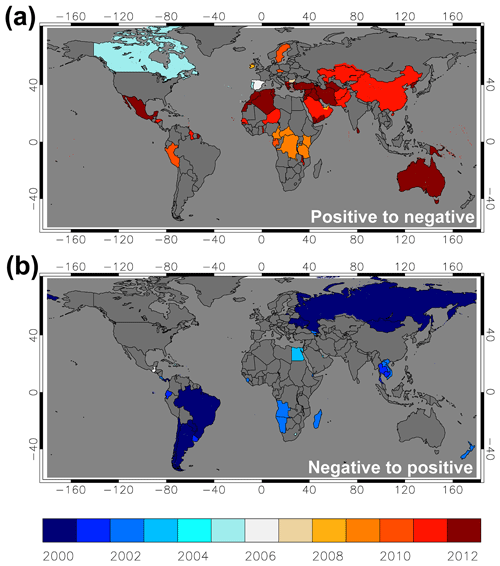
Figure 7Year of tropospheric NO2 VCD trend reversal from positive to negative (a) and from negative to positive (b) on a country level. Only countries with a statistically significant trend at the 95 % confidence level for the period before or after the year of reversal are shown.
The countries with the highest statistically significant negative trends (deep blue color in Fig. 6: absolute values higher than 0.1×1015 molecules cm−2 yr−1) in the world are the Netherlands ( molecules cm−2 yr % yr−1), Belgium ( molecules cm−2 yr % yr−1), the UK ( molecules cm−2 yr % yr−1), Taiwan ( molecules cm−2 yr % yr−1), and Germany ( molecules cm−2 yr % yr−1), while the countries with the highest statistically significant positive trends (deep red color in Fig. 6: values higher than 0.1×1015 molecules cm−2 yr−1) in the world are Swaziland (a sovereign state in southern Africa; molecules cm−2 yr % yr−1), Lebanon ( molecules cm−2 yr % yr−1), China ( molecules cm−2 yr % yr−1), Bahrain ( molecules cm−2 yr % yr−1), South Korea ( molecules cm−2 yr % yr−1), and Kuwait ( molecules cm−2 yr % yr−1). These values along with the absolute (in 1015 molecules cm−2 yr−1) and relative trends (relative to the fitted mean of the first year; in % yr−1) for all the world countries are given in Table S1 in the Supplement.
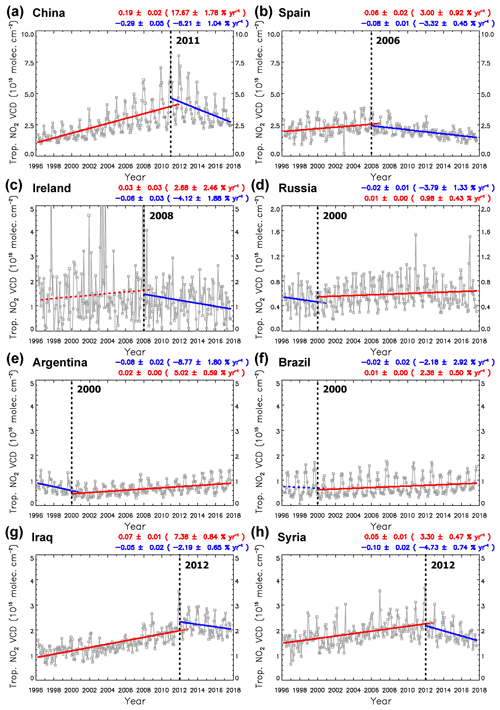
Figure 8Satellite-based time series (grey points and lines) of tropospheric NO2 VCD for the period April 1996–September 2017 for China (a), Spain (b), Ireland (c), Russia (d), Argentina (e), Brazil (f), Iraq (g), and Syria (h). The thick lines depict the trend (solid for statistically significant trends and dashed for statistically insignificant trends at the 95 % confidence level; red color for positive trends and blue color for negative trends) for the sub-period before and the sub-period after the detected trend reversal (the year of reversal is included in both sub-periods). The year of the trend reversal is indicated with a thick black dotted line. The absolute trends (in 1015 molecules cm−2 yr−1) and the trends (in % yr−1) relative to the fitted mean of the first year of the sub-periods (in parentheses) with the corresponding uncertainties (±1σ) are given on the plots (upper and lower lines correspond to the fist and the second sub-period, respectively)
Table 2 includes the absolute and relative trends only for countries that experienced a trend reversal. In the same Table, the year of trend reversal along with the absolute and relative trends for the period before the trend reversal (including the reversal year) and after the trend reversal (including the reversal year) are shown. In addition, Fig. 7a shows the year when a reversal from positive to negative trends was observed, and Fig. 7b shows the year when a reversal from negative to positive trends started on a country basis. Only countries with a statistically significant trend at the 95 % confidence level for the period before or after the year of the reversal are shown.
In several regions around the world, we can see clusters of countries that exhibit a reversal from positive to negative NO2 trends in the years 2011–2012. For example Kazakhstan, China, and North Korea in central–eastern Asia; Australia and Papua New Guinea in Oceania; Pakistan, Afghanistan, and Turkmenistan in central Asia; Iran, Iraq, Syria, Jordan, Saudi Arabia, Yemen, and Oman in the Middle East and the Arabian Peninsula; Greece, Cyprus, Turkey, Albania, and FYROM in the eastern Mediterranean and the Balkan Peninsula; Morocco, Algeria, and Tunisia in northwestern Africa; and Mexico, El Salvador, and Honduras in central America. Another cluster of countries that exhibit similar trend reversals, but for the years 2009–2010, is located in central Africa (Gabon, Equatorial Guinea, Cameroon, the Central African Republic, the Democratic Republic of the Congo, Tanzania, Malawi, and Kenya). There are also other individual countries around the world that exhibit a reversal from positive to negative trends within the period 2009–2012 (e.g., Sweden in Europe, Peru in South America, Sri Lanka in southern Asia, etc.) and also countries that exhibit such a trend reversal earlier than this (e.g., Canada and Portugal in 2005, Spain in 2006, Bulgaria in 2007, and Ireland in 2008).
On the contrary, we can also see clusters of countries around the world that exhibit a reversal from negative to positive NO2 trends in the years 2000–2002. For example Mongolia, Russia, Ukraine, Moldova, Georgia, and Armenia in Eurasia; Chile, Argentina, Paraguay, Uruguay, and Brazil in South America; and Thailand, Cambodia, Laos, and Vietnam in southeastern Asia. As discussed in Sect. 3.2, despite the fact that trend reversals appear in the same year over different regions (here countries), the driving reasons may be completely different. Similarly to Fig. 4, Fig. 8 presents the time series for the period before and after the trend reversal for eight countries of interest (China, Spain, Ireland, Russia, Argentina, Brazil, Iraq, and Syria). These countries were selected according to the results from the global analysis so as to be representative of different driving reasons.
As discussed above, the reversal from positive to negative trends over China in 2011 (Fig. 8a) is related to the extended implementation of environmental protection policies, while the reversal from positive to negative trends over Spain in 2006 (Fig. 8b) is probably related to a combination of environmental measures and optimization in combustion processes (see Sect. 3.2 and references therein). The reversal from positive to negative trends in Ireland in 2008 (Fig. 8c) coincides with the global financial crisis, which is also reflected in the sharp decline of Ireland's GDP (by ∼18 % relative to 2008) during the period 2008–2012 (World Bank, 2019). The annual mean tropospheric NO2 levels are almost stable after 2012, which is in line with Ireland's Environmental Protection Agency (EPA) report on air pollutant emissions (EPA, 2018). As shown in Fig. 8d, Russia exhibits a reversal from negative to positive trends in 2000. This is apparently connected to the economic boom in Russia after 1999, as the Russian GDP increased by a factor of 10 during the period 1999–2013 (World Bank, 2019). Similarly to Russia, Argentina and Brazil also exhibit a reversal from negative to positive tropospheric NO2 trends in 2000 (Fig. 8e and f). As discussed in Sect. 3.2, this reversal point coincides with a revival from the economic recessions that the two countries experienced in the years around 2000. In the case of Brazil, the preparations for the 2016 Olympic Games may have affected the tropospheric NO2 levels after 2009 and consequently played a role in the positive trends observed after 2000 (see Sect. 3.2 and references therein). Finally, Iraq and Syria exhibit a reversal from positive to negative trends in 2012 as a consequence the Syrian Civil War, which broke out in 2011 (see also Sect. 3.2 and references therein) and largely affected the economic and industrial activities in those countries. Indicatively, for Syria, it has been estimated that during the period 2011–2016 the cumulative GDP loss was 226 billion US dollars (4 times the Syrian GDP in 2010; World Bank Group, 2017).
3.4 Megacities and large urban agglomerations
The same analysis was repeated for a total of 64 megacities (population of more than 10 million inhabitants) and large urban agglomerations (population of more than ∼5 million inhabitants). The list of megacities and large urban agglomerations (hereafter denoted also as population hotspots – PHSs) used here is taken from Schneider et al. (2015). Such areas are characterized by extensive human activities (transportation, industry, domestic heating, etc.). Hence, trace gas emissions are expected to be more sensitive to socioeconomic changes and trend reversals are expected to be sharper. In Fig. 9 the linear trend of the tropospheric NO2 VCD (in 1015 molecules cm−2 yr−1) for the period April 1996–September 2017 is shown for 64 PHSs out of a total of 66 PHSs appearing in the Schneider et al. (2015) list, as only trends calculated for time series with at least 8 months per year are reported. Statistically significant trends at the 95 % confidence level are marked with a black outline.
As shown in Fig. 9, the majority of the PHSs with the highest statistically significant negative trends are located in Europe and the US, while the PHSs with the highest statistically significant positive trends are mostly confined to southeastern Asia (e.g., China and India), the Middle East and the Arabian Peninsula, and South America. More specifically, the PHSs with the highest statistically significant negative trends (deep blue color in Fig. 9: absolute values higher than 0.4×1015 molecules cm−2 yr−1) in the world are Los Angeles ( molecules cm−2 yr % yr−1), New York ( molecules cm−2 yr % yr−1), Boston ( molecules cm−2 yr % yr−1), the Po Valley ( molecules cm−2 yr % yr−1), Chicago ( molecules cm−2 yr % yr−1), and Philadelphia ( molecules cm−2 yr % yr−1). On the contrary the PHSs with the highest statistically significant positive trends (deep red color in Fig. 9: values higher than 0.4×1015 molecules cm−2 yr−1) are Tianjin ( molecules cm−2 yr % yr−1), Beijing ( molecules cm−2 yr % yr−1), Shenyang ( molecules cm−2 yr % yr−1), Chongqing ( molecules cm−2 yr % yr−1), Tehran ( molecules cm−2 yr % yr−1), Chengdu ( molecules cm−2 yr % yr−1), Shanghai ( molecules cm−2 yr % yr−1), Wuhan ( molecules cm−2 yr % yr−1), and Baghdad ( molecules cm−2 yr % yr−1). These values along with the trends for all the PHSs examined in this work can be found in Table 3.
Table 3The same as Table 1 but for megacities and large urban agglomerations. Bold characters are used to indicate the year of reversal and the statistically significant trends at the 95 % confidence level.
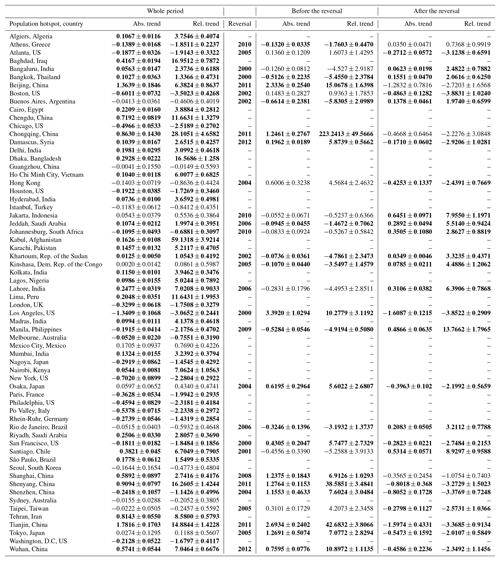
29 out of the 64 examined PHSs exhibit a trend reversal within the period of interest. Figure 10a shows the year when a reversal from positive to negative trends started (Atlanta, Beijing, Boston, Chongqing, Damascus, Hong Kong, Los Angeles, Osaka, San Francisco, Shanghai, Shenyang, Shenzhen, Taipei, Tianjin, Tokyo, and Wuhan), and Fig. 10b shows the year when a reversal from negative to positive trends started (Athens, Bangaluru, Bangkok, Buenos Aires, Jakarta, Jeddah, Johannesburg, Khartoum, Kinshasa, Lahore, Manila, Rio de Janeiro, and Santiago). Only PHSs with a statistically significant trend at the 95 % confidence level for the period before or after the year of the reversal are shown. The year of trend reversal along with the absolute and relative trends for the period before the trend reversal (including the reversal year) and after the trend reversal (including the reversal year) are given in Table 3.
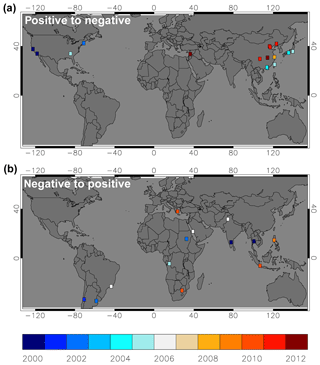
Figure 10Year of tropospheric NO2 VCD trend reversal from positive to negative (a) and from negative to positive (b) for megacities and large urban agglomerations of the world. Only spots with a statistically significant trend at the 95 % confidence level for the period before or after the year of reversal are shown.
On top of the discussions above, time series for the period before and after the trend reversal for six selected PHSs are presented in Fig. 11. Beijing, the capital of China, exhibits a sharp reversal from positive to negative (statistically insignificant) trends in 2011 as a result of emission control policies (see discussion in Sect. 3.2 and 3.3). Similarly, Los Angeles exhibits a sharp reversal from positive to negative trends in 2000, probably due to the combined effect of efficient emission control measures in California, especially after the late 1990s to early 2000s, and the economic activity slowdown following the 2008 global financial crisis (Russell et al., 2012; Hilboll et al., 2013; Lurmann et al., 2015). A reversal from negative to positive trends is observed in 2002 in Buenos Aires (capital city and financial, industrial, and commercial center of Argentina) which coincides with the period in which the country started recovering from the great economic depression of 1998–2002 (see also Sect. 3.2 and 3.3). Rio de Janeiro exhibits a sharp reversal from negative to positive trends in 2006, a bit later than the whole of Brazil (trend reversal in 2000). The trend reversal coincides with the revival of Brazil's economy and with the preparations for the 2014 World Cup and the 2016 Olympic Games (see also Sect. 3.2 and 3.3). Athens, the capital city and financial center of Greece, where half the country's population lives, exhibits a reversal from negative to positive (statistically insignificant) trends in 2010. Vrekoussis et al. (2013) reported a 30 %–40 % decrease in tropospheric NO2 in Athens during the period 2008–2012 as a result of the unprecedented economic crisis that the country experienced from 2008 onwards. Our results suggest that there may be a stabilization of the tropospheric NO2 levels after the rapid decline that was observed in the first years of the crisis. Finally, Damascus, the capital city and financial and industrial center of Syria, exhibits a sharp reversal from positive to negative trends in 2012 as a result of the Syrian Civil War, which broke out in 2011 (see Sect. 3.2 and 3.3 and references therein).
In this work, a self-consistent GOME, SCIAMACHY, and GOME-2 tropospheric NO2 VCD dataset is compiled for the period April 1996–September 2017. The GOME and GOME-2A/GOME-2B data are corrected relative to the SCIAMACHY data following a three-step procedure, and the multi-satellite dataset is then used to study the long-term global tropospheric NO2 patterns and trends and search for possible trend reversals during this ∼21-year period. The main findings of the present study are summarized in the following:
-
The highest tropospheric NO2 concentrations are seen over urban, industrialized, and highly populated areas and over ship tracks in the oceans. Tropospheric NO2 has generally decreased during the last 2 decades over the industrialized and highly populated regions of the western world and increased over developing regions. Statistically significant negative trends appear over the largest part of the US, western and central Europe, Japan and Taiwan in southeastern Asia, and the region around the Johannesburg–Pretoria conurbation in South Africa. Strong statistically significant positive trends appear over areas in southeastern Asia, the Middle East, eastern Europe, northern Africa, South Africa, and South and central America. Indicatively, during the last ∼21 years, the tropospheric NO2 levels have decreased by ∼49 % during the whole period of interest (relative to the fitted mean of the first year) over the US, the Netherlands and the UK; by ∼36 % over Italy and Japan; and by ∼32 % over Germany and France, while they increased over regions like China (an average increase of ∼160 %, with an increase of 200 %–300 % over the eastern part of the country), or India (∼33 %).
-
The application of a trend reversal detection method on a global scale revealed that extended areas over eastern China exhibit a clear reversal from positive to negative trends, mostly in 2011, while a smaller area in northwestern China exhibits a reversal from positive to negative trends in 2012. Similarly to eastern China, large parts of India experienced a reversal from positive to negative trends, mostly in 2011, while areas in central–southern India experienced a reversal from negative to positive trends during the first half of the 2000s. Other regions with widely spread positive-to-negative trend reversals are the Iberian Peninsula (mostly during the first half of the 2000s) and the Middle East (2011–2012), despite the fact that within the Middle East there are sporadic areas with a reversal from negative to positive trends in the early 2000s. A similar negative-to-positive trend reversal is observed over the region of Nile River in Egypt. Extended areas with a persistent positive-to-negative trend reversal are also seen in central Africa and Mexico (late 2000s) and in the US (early 2000s), while areas with a persistent negative-to-positive trend reversal in the early 2000s can be seen in South America, mostly in Brazil and Argentina.
-
A country-level analysis showed clusters of countries that exhibit a reversal from positive to negative tropospheric NO2 trends in the years 2011–2012 in central–eastern Asia (Kazakhstan, China, and North Korea), Oceania (Australia and Papua New Guinea), central Asia (Pakistan, Afghanistan, and Turkmenistan), the Middle East and the Arabian Peninsula (Iran, Iraq, Syria, Jordan, Saudi Arabia, Yemen, and Oman), the eastern Mediterranean and the Balkan Peninsula (Greece, Cyprus, Turkey, Albania, and FYROM), northwestern Africa (Morocco, Algeria, and Tunisia) and central America (Mexico, El Salvador, and Honduras). Another cluster of countries that exhibit similar trend reversals, but for the years 2009–2010, is located in central Africa (Gabon, Equatorial Guinea, Cameroon, the Central African Republic, the Democratic Republic of the Congo, Tanzania, Malawi, and Kenya). There are also individual countries around the world that exhibit a reversal from positive to negative trends within the period 2009–2012 (e.g., Sweden, Peru, and Sri Lanka) and countries that exhibit such a trend reversal earlier than this (Canada and Portugal in 2005, Spain in 2006, Bulgaria in 2007, and Ireland in 2008). On the contrary, we can see clusters of countries around the world that exhibit a reversal from negative to positive NO2 trends in the years 2000–2002 in Eurasia (Mongolia, Russia, Ukraine, Moldova, Georgia, and Armenia), South America (Chile, Argentina, Paraguay, Uruguay, and Brazil) and southeastern Asia (Thailand, Cambodia, Laos, and Vietnam).
-
The application of the trend reversal detection method on 64 megacities and large urban agglomerations revealed that 29 of them exhibit a tropospheric NO2 trend reversal. A reversal from negative to positive trends was observed for Athens, Greece; Bangaluru, India; Bangkok, Thailand; Buenos Aires, Argentina; Jakarta, Indonesia; Jeddah, Saudi Arabia; Johannesburg, South Africa; Khartoum, Republic of the Sudan; Kinshasa, the Democratic Republic of the Congo; Lahore, Pakistan; Manila, Philippines; Rio de Janeiro, Brazil; and Santiago, Chile, while a reversal from positive to negative trends was observed for Atlanta, US; Beijing, China; Boston, US; Chongqing, China; Damascus, Syria; Hong Kong; Los Angeles, US; Osaka, Japan; San Francisco, US; Shanghai, China; Shenyang, China; Shenzhen, China; Taipei, Taiwan; Tianjin, China; Tokyo, Japan; and Wuhan, China.
-
It is shown that the observed tropospheric NO2 trend reversals over different regions, countries and megacities and large urban agglomerations can be associated with various socioeconomic changes (environmental policies, economic recession, warfare, etc.) that possibly had a direct impact on NOx emissions. For example, the reversal from positive to negative trends in 2011–2012 over extended areas in China (including Beijing and other megacities) can be attributed to the efficient implementation of environmental protection policies. The reversal from positive to negative trends over Spain in 2006 might be attributed to a combination of environmental measures and optimization in combustion processes, while the reversal from positive to negative trends in Ireland in 2008 coincides with the bursting of the global financial crisis. Russia's reversal from negative to positive trends in 2000 might be connected to the economic boom of the country after 1999. Similarly, Argentina and Brazil's reversal from negative to positive trends in 2000 coincides with a revival from the economic recession that both the countries experienced during the years around year 2000. The megacities Buenos Aires in Argentina and Rio de Janeiro in Brazil exhibit a similar trend reversal a bit later than the corresponding countries. In the case of Brazil, the preparations for the 2016 Olympic Games might have affected the tropospheric NO2 levels after 2009 and consequently played a role in the positive trends observed after 2000. Iraq and Syria (including Damascus) exhibit a reversal from positive to negative trends in 2012 which is profoundly due to the Syrian Civil War, which broke out in 2011 and largely affected the economic and industrial activities. Athens, Greece, exhibits a reversal from negative to positive (statistically insignificant) trends in 2010, pointing towards a stabilization of tropospheric NO2 after the rapid decline that was observed during the first years of the Greek economic crisis. Finally, a positive-to-negative trend reversal is seen in Los Angeles, US, in 2000 which might be attributed to the combined effect of efficient emission control measures in California, especially after the late 1990s to early 2000s, and the economic activity slowdown following the 2008 global financial crisis.
In the coming years, tropospheric NO2 time series will be extended from new more sophisticated satellite sensors such as the recently launched Tropospheric Monitoring Instrument (TROPOMI) aboard the ESA's Sentinel-5 Precursor (S-5P) satellite (Veefkind et al., 2012). Hence, the need to develop similar methods in the future that will be able to incorporate both morning and afternoon measurements (e.g., from OMI and TROPOMI) and detect more than one trend reversal point in improved tropospheric NO2 products (e.g., QA4ECV v. 1.1; Boersma et al., 2018) is acknowledged.
The self-consistent multi-satellite tropospheric NO2 dataset compiled here and the original GOME, SCIAMACHY, GOME-2A, and GOME-2B data are available through the TEMIS website (http://www.temis.nl, last access: 19 March 2019, TEMIS, 2019).
The supplement related to this article is available online at: https://doi.org/10.5194/acp-19-6269-2019-supplement.
AKG, RJvdA, and PS designed the study with feedback from the other co-authors. AKG performed the data analysis and wrote the paper with comments from the co-authors. All the authors contributed to the interpretation of the results.
The authors declare that they have no conflict of interest.
This research has been implemented within the framework of the SCIAvisie project funded by the Netherlands Space Office (NSO).
This paper was edited by Ilse Aben and reviewed by Maria-Elissavet Koukouli and one anonymous referee.
Alexandri, G., Georgoulias, A. K., Meleti, C., Balis, D., Kourtidis, K. A., Sanchez-Lorenzo, A., Trentmann, J., and Zanis, P.: A high resolution satellite view of surface solar radiation over the climatically sensitive region of Eastern Mediterranean, Atmos. Res., 188, 107–121, https://doi.org/10.1016/j.atmosres.2016.12.015, 2017.
Bansal, G. and Bandivadekar, G.: Overview of India's vehicle emissions control program, Tech. Rep., The International Council on Clean Transportation, Washington, D.C., 2013.
Basset, M. and Seinfeld, J. H.: Atmospheric Equilibrium Model of Sulfate and Nitrate Aerosols, Atmos. Environ., 17, 2237–2252, 1983.
Beirle, S., Platt, U., Wenig, M., and Wagner, T.: Highly resolved global distribution of tropospheric NO2 using GOME narrow swath mode data, Atmos. Chem. Phys., 4, 1913–1924, https://doi.org/10.5194/acp-4-1913-2004, 2004.
Boersma, K. F., Eskes, H. J., and Brinksma, E. J.: Error analysis for tropospheric NO2 retrieval from space, J. Geophys. Res., 109, D04311, https://doi.org/10.1029/2003JD003962, 2004.
Boersma, K. F., Jacob, D. J., Eskes, H. J., Pinder, R. W., and Wang, J.: Intercomparison of SCIAMACHY and OMI tropospheric NO2 columns: Observing the diurnal evolution of chemistry and emissions from space, J. Geophys. Res., 113, D16S26, https://doi.org/10.1029/2007JD008816, 2008.
Boersma, K. F., Eskes, H. J., Dirksen, R. J., van der A, R. J., Veefkind, J. P., Stammes, P., Huijnen, V., Kleipool, Q. L., Sneep, M., Claas, J., Leitão, J., Richter, A., Zhou, Y., and Brunner, D.: An improved tropospheric NO2 column retrieval algorithm for the Ozone Monitoring Instrument, Atmos. Meas. Tech., 4, 1905–1928, https://doi.org/10.5194/amt-4-1905-2011, 2011.
Boersma, K. F., Eskes, H. J., Richter, A., De Smedt, I., Lorente, A., Beirle, S., van Geffen, J. H. G. M., Zara, M., Peters, E., Van Roozendael, M., Wagner, T., Maasakkers, J. D., van der A, R. J., Nightingale, J., De Rudder, A., Irie, H., Pinardi, G., Lambert, J.-C., and Compernolle, S. C.: Improving algorithms and uncertainty estimates for satellite NO2 retrievals: results from the quality assurance for the essential climate variables (QA4ECV) project, Atmos. Meas. Tech., 11, 6651–6678, https://doi.org/10.5194/amt-11-6651-2018, 2018.
Bovensmann, H., Burrows, J. P., Buchwitz, M., Frerick, F., Nöel, S., and Rozanov, V. V.: SCIAMACHY: mission objectives and measurement modes, J. Atmos. Sci., 56, 127–150, https://doi.org/10.1175/1520-0469(1999)056<0127:SMOAMM>2.0.CO;2, 1999.
Burrows, J. P., Hölzle, E., Goede, A., Visser, H., and Fricke, W.: SCIAMACHY – scanning imaging absorption spectrometer for atmospheric chartography, Acta Astronaut., 35, 445–451, https://doi.org/10.1016/0094-5765(94)00278-T, 1995.
Burrows, J. P.,Weber, M., Buchwitz, M., Rozanov, V. V., Ladstätter-Weißenmayer, A., Richter, A., DeBeek, R., Hoogen, R., Bramstedt, K., Eichmann, K.-U., and Eisinger, M.: The Global Ozone Monitoring Experiment (GOME): mission concept and first scientific results, J. Atmos. Sci., 56, 151–175, https://doi.org/10.1175/1520-0469(1999)056<0151:TGOMEG>2.0.CO;2, 1999.
Castellanos, P. and Boersma, K. F.: Reductions in nitrogen oxides over Europe driven by environmental policy and economic recession, Sci. Rep., 2, 265, https://doi.org/10.1038/srep00265, 2012.
Cermak, J., Wild, M., Knutti, R., Mishchenko, M. I., and Heidinger, A. K.: Consistency of global satellite-derived aerosol and cloud data sets with recent brightening observations, Geophys. Res. Lett., 37, L21704, https://doi.org/10.1029/2010GL044632, 2010.
ChinaFAQs Project: China Adopts World-Class Pollutant Emissions Standards for Coal Power Plants, available at: http://www.chinafaqs.org/library/chinafaqs-china-adopts-world-class-pollutant-emissions-standards-coal-power-plants (last access: 15 September 2018), 2012.
Cline, W. R.: Restoring economic growth in Argentina (English), Working paper; no. 9/03, Washington, DC: World Bank, available at: http://documents.worldbank.org/curated/en/8055014687692776 47/Restoring-economic-growth-in-Argentina (last access: 19 March 2019), 2003.
Cuevas, C. A., Notario, A., Adame, J. A., Hilboll, A., Richter, A., Burrows, J. P., and Saiz-Lopez, A.: Evolution of NO2 levels in Spain from 1996 to 2012, Sci. Rep., 4, 5887, https://doi.org/10.1038/srep05887, 2014.
de Foy, B., Lu, Z., and Streets, D. G.: Satellite NO2 retrievals suggest China has exceeded its NOx reduction goals from the twelfth Five-Year Plan, Scientific Reports, 6, 35912, https://doi.org/10.1038/srep35912, 2016.
de Meij, A., Pozzer, A., and Lelieveld, J.: Trend analysis in aerosol optical depths and pollutant emission estimates between 2000 and 2009, Atmos. Environ., 51, 75–85, https://doi.org/10.1016/j.atmosenv.2012.01.059, 2012.
De Smedt, I., Stavrakou, T., Muller, J.-F., van der A, R. J., and Van Roozendael, M.: Trend detection in satellite observations of formaldehyde tropospheric columns, Geophys. Res. Lett., 37, L18808, https://doi.org/10.1029/2010GL044245, 2010.
Dirksen, R. J., Boersma, K. F., Eskes, H. J., Ionov, D. V., Bucsela, E. J., Levelt, P. F., and Kelder, H. M.: Evaluation of stratospheric NO2 retrieved from the Ozone Monitoring Instrument: intercomparison, diurnal cycle and trending, J. Geophys. Res., 116, D08305, https://doi.org/10.1029/2010JD014943, 2011.
EEA-APFS-Portugal: European Environment Agency – Air pollution fact sheet – Portugal, available at: https://www.eea.europa.eu/themes/air/air-pollution-country-fact-sheets-2014/portugal-air-pollutant-emissions-country-fact sheet/at_download/file (last access: 19 March 2019), 2014.
EEA-APFS-Spain: European Environment Agency – Air pollution fact sheet – Spain, available at: https://www.eea.europa.eu/themes/air/air-pollution-country-fact-sheets-2014/spain-air-pollutant-emissions-country-factshe et/at_download/file (last access: 19 March 2019), 2014.
EPA: Ireland's Air Pollutant Emissions 2016, available at: http://www.epa.ie/pubs/reports/air/airemissions/ irelandsairpollutantemissions2016.html (last access: 19 March 2019), 2018.
Geddes, J. A., Martin, R. V., Boys, B. L., and van Donkelaar, A.: Long-term trends worldwide in ambient NO2 concentrations inferred from satellite observations, Environ. Health Persp., 124, 281–289, https://doi.org/10.1289/ehp.1409567, 2016.
Georgoulias, A. K., Alexandri, G., Kourtidis, K. A., Lelieveld, J., Zanis, P., and Amiridis, V.: Differences between the MODIS Collection 6 and 5.1 aerosol datasets over the greater Mediterranean region, Atmos. Environ., 147, 310–319, https://doi.org/10.1016/j.atmosenv.2016.10.014, 2016.
Ghude, S. D., Pfister, G. G., Jena, C., van der A, R., Emmons, L. K., and Kumar, R.: Satellite constraints of nitrogen oxide (NOx) emissions from India based on OMI observations and WRF-Chem simulations, Geophys. Res. Lett., 40, 423–428, https://doi.org/10.1002/grl.50065, 2013.
Hilboll, A., Richter, A., and Burrows, J. P.: Long-term changes of tropospheric NO2 over megacities derived from multiple satellite instruments, Atmos. Chem. Phys., 13, 4145–4169, https://doi.org/10.5194/acp-13-4145-2013, 2013.
Hilboll, A., Richter, A., and Burrows, J. P.: NO2 pollution over India observed from space – the impact of rapid economic growth, and a recent decline, Atmos. Chem. Phys. Discuss., https://doi.org/10.5194/acp-2017-101, in review, 2017.
ICAC: Institute of Clean Air Companies, Selective Catalytic Reduction (SCR) control of NOx emissions from fossil fuel-fired electric power plants, available at: https://c.ymcdn.com/sites/icac.site-ym.com/resource/resmgr/ Standards_WhitePapers/SCR_WhitePaper_final_2009.pdf (last access: 19 March 2019), 2009.
IEA: Clean Coal Center report, available at: https://www.iea-coal.org/wp-content/uploads/2017/12/spain-1 (last access: 19 March 2019), 2017.
Isaksen, I. S. A., Berntsen, T. K., Dalsøren, S. B., Eleftheratos, K., Orsolini, Y., Rognerud, B., Stordal, F., Søvde, O. A., Zerefos, C., and Holmes, C. D.: Atmospheric Ozone and Methane in a Changing Climate, Atmosphere, 5, 518–535, https://doi.org/10.3390/atmos5030518, 2014.
Koelemeijer, R. B. A., Stammes, P., Hovenier, J. W., and de Haan, J. F.: A fast method for retrieval of cloud parameters using oxygen A band measurements from the Global Ozone Monitoring Experiment, J. Geophys. Res., 106, 3475–3490, https://doi.org/10.1029/2000JD900657, 2001.
Konovalov, I. B., Beekmann, M., Richter, A., and Burrows, J. P.: Inverse modelling of the spatial distribution of NOx emissions on a continental scale using satellite data, Atmos. Chem. Phys., 6, 1747–1770, https://doi.org/10.5194/acp-6-1747-2006, 2006.
Konovalov, I. B., Beekmann, M., Burrows, J. P., and Richter, A.: Satellite measurement based estimates of decadal changes in European nitrogen oxides emissions, Atmos. Chem. Phys., 8, 2623–2641, https://doi.org/10.5194/acp-8-2623-2008, 2008.
Konovalov, I. B., Beekmann, M., Richter, A., Burrows, J. P., and Hilboll, A.: Multi-annual changes of NOx emissions in megacity regions: nonlinear trend analysis of satellite measurement based estimates, Atmos. Chem. Phys., 10, 8481–8498, https://doi.org/10.5194/acp-10-8481-2010, 2010.
Kourtidis, K., Stathopoulos, S., Georgoulias, A. K., Alexandri, G., and Rapsomanikis, S.: A study of the impact of synoptic weather conditions and water vapor on aerosol-cloud relationships over major urban clusters of China, Atmos. Chem. Phys., 15, 10955–10964, https://doi.org/10.5194/acp-15-10955-2015, 2015.
Krotkov, N. A., McLinden, C. A., Li, C., Lamsal, L. N., Celarier, E. A., Marchenko, S. V., Swartz, W. H., Bucsela, E. J., Joiner, J., Duncan, B. N., Boersma, K. F., Veefkind, J. P., Levelt, P. F., Fioletov, V. E., Dickerson, R. R., He, H., Lu, Z., and Streets, D. G.: Aura OMI observations of regional SO2 and NO2 pollution changes from 2005 to 2015, Atmos. Chem. Phys., 16, 4605–4629, https://doi.org/10.5194/acp-16-4605-2016, 2016.
Lelieveld, J., Beirle, S., Hörmann, C., Stenchikov, G., and Wagner, T.: Abrupt recent trend changes in atmospheric nitrogen dioxide over the Middle East, Sci. Adv., 1, 2–6, https://doi.org/10.1126/sciadv.1500498, 2015.
Levelt, P. F., Joiner, J., Tamminen, J., Veefkind, J. P., Bhartia, P. K., Stein Zweers, D. C., Duncan, B. N., Streets, D. G., Eskes, H., van der A, R., McLinden, C., Fioletov, V., Carn, S., de Laat, J., DeLand, M., Marchenko, S., McPeters, R., Ziemke, J., Fu, D., Liu, X., Pickering, K., Apituley, A., González Abad, G., Arola, A., Boersma, F., Chan Miller, C., Chance, K., de Graaf, M., Hakkarainen, J., Hassinen, S., Ialongo, I., Kleipool, Q., Krotkov, N., Li, C., Lamsal, L., Newman, P., Nowlan, C., Suleiman, R., Tilstra, L. G., Torres, O., Wang, H., and Wargan, K.: The Ozone Monitoring Instrument: overview of 14 years in space, Atmos. Chem. Phys., 18, 5699–5745, https://doi.org/10.5194/acp-18-5699-2018, 2018.
Liu, F., Zhang, Q., Ronald, J. van der A., Zheng, B., Tong, D., Yan, L., Zheng, Y., and He, K.: Recent reduction in NOx emissions over China: synthesis of satellite observations and emission inventories, Environ. Res. Lett., 11, 114002, https://doi.org/10.1088/1748-9326/11/11/114002, 2016.
Lurmann, F., Avol, E., and Gilliland, F.: Emissions reduction policies and recent trends in Southern California's ambient air quality, J. Air Waste Manage., 65, 324–335, https://doi.org/10.1080/10962247.2014.991856, 2015.
Mijling, B., van der A, R. J., Boersma, K. F., Van Roozendael, M., De Smedt, I., and Kelder, H. M.: Reductions in NO2 detected from space during the 2008 Beijing Olympic Games, Geophys. Res. Lett., 36, L13801, https://doi.org/10.1029/2009GL038943, 2009.
Munro, R., Lang, R., Klaes, D., Poli, G., Retscher, C., Lindstrot, R., Huckle, R., Lacan, A., Grzegorski, M., Holdak, A., Kokhanovsky, A., Livschitz, J., and Eisinger, M.: The GOME-2 instrument on the Metop series of satellites: instrument design, calibration, and level 1 data processing – an overview, Atmos. Meas. Tech., 9, 1279–1301, https://doi.org/10.5194/amt-9-1279-2016, 2016.
Platt, U.: Differential optical absorption spectroscopy (DOAS), in: Air Monitoring by Spectroscopic Techniques, edited by: Sigrist, M. W., Vol. 127, John Wiley & Sons, Hoboken, N.J., USA, 27–83, 1994.
Pozzer, A., de Meij, A., Yoon, J., Tost, H., Georgoulias, A. K., and Astitha, M.: AOD trends during 2001–2010 from observations and model simulations, Atmos. Chem. Phys., 15, 5521–5535, https://doi.org/10.5194/acp-15-5521-2015, 2015.
Russell, A. R., Valin, L. C., and Cohen, R. C.: Trends in OMI NO2 observations over the United States: effects of emission control technology and the economic recession, Atmos. Chem. Phys., 12, 12197–12209, https://doi.org/10.5194/acp-12-12197-2012, 2012.
Schneider, P. and van der A, R. J.: A global single-sensor analysis of 2002–2011 tropospheric nitrogen dioxide trends observed from space, J. Geophys. Res., 117, 1–17, https://doi.org/10.1029/2012JD017571, 2012.
Schneider, P., Lahoz, W. A., and van der A, R.: Recent satellite-based trends of tropospheric nitrogen dioxide over large urban agglomerations worldwide, Atmos. Chem. Phys., 15, 1205–1220, https://doi.org/10.5194/acp-15-1205-2015, 2015.
Seinfeld, J. H. and Pandis, S. N.: Atmospheric chemistry and physics: from air pollution to climate change, Wiley, New Jersey, USA, 2016.
Sogacheva, L., Rodriguez, E., Kolmonen, P., Virtanen, T. H., Saponaro, G., de Leeuw, G., Georgoulias, A. K., Alexandri, G., Kourtidis, K., and van der A, R. J.: Spatial and seasonal variations of aerosols over China from two decades of multi-satellite observations – Part 2: AOD time series for 1995–2017 combined from ATSR ADV and MODIS C6.1 and AOD tendency estimations, Atmos. Chem. Phys., 18, 16631–16652, https://doi.org/10.5194/acp-18-16631-2018, 2018.
Solomon, S., Portmann, R. W., Sanders, R. W., and Daniels, J. S.: On the role of nitrogen dioxide in the absorption of solar radiation, J. Geophys. Res., 104, 12047–12058, https://doi.org/10.1029/1999JD900035, 1999.
Stammes, P.: Spectral radiance modelling in the UV-Visible range, in: IRS 2000: Current Problems in Atmospheric Radiation, edited by: Smith, W. L. and Timofeyev, Y. M., A. Deepak Publ., Hampton, VA, 385–388, 2001.
TEMIS: Tropospheric Emission Monitoring Internet Service, available at: http://www.temis.nl, last access: 19 March 2019.
Valks, P., Pinardi, G., Richter, A., Lambert, J.-C., Hao, N., Loyola, D., Van Roozendael, M., and Emmadi, S.: Operational total and tropospheric NO2 column retrieval for GOME-2, Atmos. Meas. Tech., 4, 1491–1514, https://doi.org/10.5194/amt-4-1491-2011, 2011.
van der A, R. J., Peters, D. H. M. U., Eskes, H., Boersma, K. F., Van Roozendael, M., De Smedt, I., and Kelder, H. M.: Detection of the trend and seasonal variation in tropospheric NO2 over China, J. Geophys. Res., 111, 1–10, https://doi.org/10.1029/2005JD006594, 2006.
van der A, R. J., Eskes, H. J., Boersma, K. F., van Noije, T. P. C., Van Roozendael, M., De Smedt, I., Peters, D. H. M. U., and Meijer, E. W.: Trends, seasonal variability and dominant NOx source derived from a ten year record of NO2 measured from space, J. Geophys. Res., 113, 1–12, https://doi.org/10.1029/2007JD009021, 2008.
van der A, R. J., Mijling, B., Ding, J., Koukouli, M. E., Liu, F., Li, Q., Mao, H., and Theys, N.: Cleaning up the air: effectiveness of air quality policy for SO2 and NOx emissions in China, Atmos. Chem. Phys., 17, 1775–1789, https://doi.org/10.5194/acp-17-1775-2017, 2017.
Vandaele, A. C., Fayt, C., Hendrick, F., Hermans, C., Humbled, F., Van Roozendael, M., Gil, M., Navarro, M., Puentedura, O., Yela, M., Braathen, G., Stebel, K., Tørnkvist, K., Johnston, P., Kreher, K., Goutail, F., Mieville, A., Pommereau, J.-P., Khaikine, S., Richter, A., Oetjen, H., Wittrock, F., Bugarski, S., Friess, U., Pfeilsticker, K., Sinreich, R., Wagner, T., Corlett, G., and Leigh, R.: An intercomparison campaign of ground-based UV-visible measurements of NO2, BrO, and OClO slant columns: Methods of analysis and results for NO2, J. Geophys. Res., 110, D08305, https://doi.org/10.1029/2004JD005423, 2005.
Veefkind, J. P., Aben, I., McMullan, K., Forster, H., de Vries, J., Otter, G., Claas, J., Eskes, H. J., de Haan, J. F., Kleipool, Q., van Weele, M., Hasekamp, O., Hoogeveen, R., Landgraf, J., Snel, R., Tol, P., Ingmann, P., Voors, R., Kruizinga, B., Vink, R., Visser, H., and Levelt, P. F.: TROPOMI on the ESA Sentinel-5 Precursor: a GMES mission for global observations of the atmospheric composition for climate, air quality and ozone layer applications, Remote Sens. Environ., 120, 70–83, https://doi.org/10.1016/j.rse.2011.09.027, 2012.
Vestreng, V., Ntziachristos, L., Semb, A., Reis, S., Isaksen, I. S. A., and Tarrasón, L.: Evolution of NOx emissions in Europe with focus on road transport control measures, Atmos. Chem. Phys., 9, 1503–1520, https://doi.org/10.5194/acp-9-1503-2009, 2009.
Vrekoussis, M., Richter, A., Hilboll, A., Burrows, J. P., Gerasopoulos, E., Lelieveld, J., Barrie, L., Zerefos, C., and Mihalopoulos, N.: Economic Crisis Detected from Space: Air Quality observations over Athens/Greece, Geophys. Res. Lett., 40, 458–463, https://doi.org/10.1002/grl.50118, 2013.
Voulgarakis, A., Savage, N. H., Wild, O., Braesicke, P., Young, P. J., Carver, G. D., and Pyle, J. A.: Interannual variability of tropospheric composition: the influence of changes in emissions, meteorology and clouds, Atmos. Chem. Phys., 10, 2491–2506, https://doi.org/10.5194/acp-10-2491-2010, 2010.
Weatherhead, E. C., Reinsel, G. C., Tiao, G. C., Meng, X.-L., Choi, D., Cheang, W.-K., Keller, T., DeLuisi, J., Wuebbles, D. J., Kerr, J. B., Miller, A. J., Oltmans, S. J., and Frederick, J. E.: Factors affecting the detection of trends: Statistical considerations and applications to environmental data, J. Geophys. Res., 103, 17149, https://doi.org/10.1029/98JD00995, 1998.
WHO: Health Aspects of Air Pollution with Particulate Matter, Ozone and Nitrogen Dioxide, World Health Organization, Bonn, Germany, 2003.
World Bank Group: The Toll of War: The Economic and Social Consequences of the Conflict in Syria, available at: https://openknowledge.worldbank.org/bitstream/handle/10986/27541/The20Toll20of20War.pdf (last access: 19 March 2019), 2017.
World Bank: available at: https://data.worldbank.org (last access: 19 March 2019), 2019.
Wu, Y., Zhang, S., Hao, J., Liu, H., Wu, X., Hu, J., Walsh, M. P., Wallington, T. J., and Zhang, K. M. S.: Stevanovic, On-road vehicle emissions and their control in China: A review and outlook, Sci. Total Environ., 574, 332–349, https://doi.org/10.1016/j.scitotenv.2016.09.040, 2017.
Zhao, B., Wang, S. X., Liu, H., Xu, J. Y., Fu, K., Klimont, Z., Hao, J. M., He, K. B., Cofala, J., and Amann, M.: NOx emissions in China: historical trends and future perspectives, Atmos. Chem. Phys., 13, 9869–9897, https://doi.org/10.5194/acp-13-9869-2013, 2013.