the Creative Commons Attribution 4.0 License.
the Creative Commons Attribution 4.0 License.
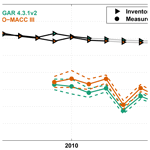
Emissions of methane in Europe inferred by total column measurements
Debra Wunch
Dylan B. A. Jones
Geoffrey C. Toon
Nicholas M. Deutscher
Frank Hase
Justus Notholt
Ralf Sussmann
Thorsten Warneke
Jeroen Kuenen
Hugo Denier van der Gon
Jenny A. Fisher
Joannes D. Maasakkers
Using five long-running ground-based atmospheric observatories in Europe, we demonstrate the utility of long-term, stationary, ground-based measurements of atmospheric total columns for verifying annual methane emission inventories. Our results indicate that the methane emissions for the region in Europe between Orléans, Bremen, Białystok, and Garmisch-Partenkirchen are overestimated by the state-of-the-art inventories of the Emissions Database for Global Atmospheric Research (EDGAR) v4.2 FT2010 and the high-resolution emissions database developed by the Netherlands Organisation for Applied Scientific Research (TNO) as part of the Monitoring Atmospheric Composition and Climate project (TNO-MACC_III), possibly due to the disaggregation of emissions onto a spatial grid. Uncertainties in the carbon monoxide inventories used to compute the methane emissions contribute to the discrepancy between our inferred emissions and those from the inventories.
Recent global policy agreements have led to renewed efforts to reduce greenhouse gas emissions to cap global temperature rise (e.g., Conference of the Parties 21, COP 21; UNFCCC, 2015; Kona et al., 2016). This, in turn, has motivated countries to seek methods of reducing their greenhouse gas emissions. In Europe, methane emissions account for a significant fraction (about 11 % by mass of CO2 equivalent) of the total greenhouse gas emissions (UNFCCC, 2017). The lifetime of atmospheric methane is significantly shorter than for carbon dioxide, its 100-year global warming potential is significantly larger, and it is at near steady state in the atmosphere; therefore, significant reductions in methane emissions are an effective short-term strategy for reducing greenhouse gas emissions (Dlugokencky et al., 2011). Emission reduction strategies that include both methane emission reductions and carbon dioxide reductions are thought to be among the most effective at slowing the increase in global temperatures (Shoemaker et al., 2013). Thus, it is important to know exactly how much methane is being emitted and the geographic and temporal source of the emissions. This requires an approach that combines state-of-the-art emissions inventories that contain information about the specific point and area sources of the known emissions and timely and long-term measurements of greenhouse gases in the atmosphere to verify that the emissions reduction targets are met.
Because atmospheric methane is well-mixed and has a lifetime of about 12 years (Stocker et al., 2013), it is transported far from its emission source, making source attribution efforts challenging from atmospheric measurements alone. Atmospheric measurements are often assimilated into “flux inversion” models to locate the sources of the emissions (e.g., Houweling et al., 2014) but rely on model wind fields to drive transport, and they also tend to have spatial resolutions that do not resolve subregional scales. Methane measurement schemes that constrain emissions on local and regional scales are thus important to help identify the sources of the emissions and to verify inventory analyses. Regional- or national-scale emissions are important to public policy as those emissions are reported annually to the United Nations Framework Convention on Climate Change (UNFCCC).
The atmospheric measurement techniques that are used to estimate methane emissions include measurements made in situ, either on the ground, from tall towers, or from aircraft. Remote sensing techniques are also used, either from space or from the ground. The spatial scale of the sensitivity to emissions differs with the measurement technique: surface in situ measurements provide information about local emissions on urban scales (e.g., McKain et al., 2015; Hopkins et al., 2016), and aircraft in situ measurements can provide information about regional- and synoptic-scale fluxes (e.g., Jacob et al., 2003; Kort et al., 2008, 2010; Wofsy, 2011; Baker et al., 2012; Frankenberg et al., 2016; Karion et al., 2016). Satellite remote sensing techniques provide information useful for extracting emission information on larger scales (regional to global) (e.g., Silva et al., 2013; Schneising et al., 2014; Alexe et al., 2015; Turner et al., 2015) and for large point or urban sources (e.g., Kort et al., 2012, 2014; Nassar et al., 2017). Several studies have shown the importance of simultaneous measurements of co-emitted species (e.g., C2H6 and CH4 or CO and CO2, Aydin et al., 2011; Simpson et al., 2012; Peischl et al., 2013; Silva et al., 2013; Hausmann et al., 2016; Wunch et al., 2016; Jeong et al., 2017) or co-located measurements (e.g., Wunch et al., 2009, 2016), showing the added analytical power of the combination of atmospheric tracer information. Ground-based remote sensing instruments have been used to estimate methane emissions on urban (e.g., Wunch et al., 2009; Hase et al., 2015; Wunch et al., 2016) and sub-urban (e.g., Chen et al., 2016; Viatte et al., 2017) scales. In Hase et al. (2015), Viatte et al. (2017), and Chen et al. (2016), the authors have placed mobile ground-based remote sensing instruments around a particular emitter of interest (e.g., a city, dairy, or neighborhood) and have designed short-term campaigns to measure the difference between upwind and downwind atmospheric methane abundances. From these differences the authors have computed emission fluxes. However, there is a network of nonmobile ground-based remote sensing instruments that have been collecting long-term measurements of atmospheric greenhouse gas abundances. These instruments were not placed intentionally around an emitter of interest, but collectively they ought to contain information about nearby emissions. To date, there have been no studies that have attempted to extract regional methane emission information from these existing ground-based remote sensing observatories.
In this paper, we will describe our methods for computing the emissions of methane using five stationary ground-based remote sensing instruments located in Europe in Sect. 2. Our results and comparisons to the state-of-the-art inventories are shown in Sect. 3, and we summarize our results in Sect. 4.
Our study area is the region between five long-running atmospheric observatories situated in Europe. Three of the stations are in Germany: Bremen (Notholt et al., 2014), Karlsruhe (Hase et al., 2014), and Garmisch-Partenkirchen (Sussmann and Rettinger, 2014). The other two are in Poland (Białystok, Deutscher et al., 2017) and France (Orléans, Warneke et al., 2014). Each station measures the vertical column-averaged dry-air mole fraction of carbon dioxide (), carbon monoxide (XCO), methane (), and other trace gas species. The locations are shown in Fig. 1, overlaid on a nighttime light image from the National Aeronautics and Space Administration (NASA) to provide a sense of the population density of the area. These observatories are part of the Total Carbon Column Observing Network (TCCON, Wunch et al., 2011) and have been tied to the World Meteorological Organization trace-gas scale through comparisons with vertically integrated, calibrated in situ profiles over the observatories (Wunch et al., 2010, 2015; Messerschmidt et al., 2011; Geibel et al., 2012).
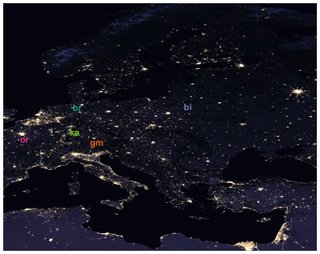
Figure 1The locations of the TCCON observatories overlaid on a NASA nighttime light image. From west to east, the stations are Orléans (or, pink), Karlsruhe (ka, green), Bremen (br, blue-green), Garmisch-Partenkirchen (gm, orange), and Białystok (bi, purple).
Following a similar method to Wunch et al. (2009, 2016), we estimate emissions of methane from the data recorded from the TCCON observatories, coupled with gridded inventories of carbon monoxide within the region. We compute changes (or “anomalies”) in and XCO that we will refer to as and ΔXCO, and we then compute the slopes relating to ΔXCO. From the computed slopes (α), we can infer emissions of methane (E) if emissions of carbon monoxide (ECO, in mass per unit time) are known, using the following relationship:
where is the ratio of the molecular masses of CH4 and CO.
In Wunch et al. (2009, 2016), measurements from a single atmospheric observatory were used to infer emissions because the unique dynamics of the region advected the polluted air mass into and out of the study area diurnally. In this paper, we rely on several stations to provide measurements of the boundary of the study region to measure CO and CH4 emitted between the stations. This analysis relies on a few assumptions about the nature of the emissions. First, that the lifetimes of the gases of interest are longer than the transport time within the region. This is the case both for methane, which has an atmospheric lifetime of 12 years, and for carbon monoxide, which has an atmospheric lifetime of a few weeks. Second, we assume that typical emissions are consistent over time periods longer than a few days so that they are advected together. The nature of the emissions in this region (mostly residential and industrial energy needs) supports this assumption. Third, we assume that the spatial distribution of the emissions is similar for CH4 and CO, as confirmed by the inventory maps (Fig. A3). This method does not require carbon monoxide and methane to be co-emitted (as they generally do not have the same emissions sources).
To compute anomalies and slopes, we first filter the data to minimize the impact of data sparsity and air mass differences between stations (Appendix Appendix A). Then, for each station, the daily median value is subtracted from each measurement. This reduces the impact of the station altitude and any background seasonal cycle from aliasing into the results. Subsequently, we compute the differences in the and XCO abundances measured at the same solar zenith and solar azimuth angles on the same day at two TCCON stations. By computing anomalies at the same solar zenith angles, we minimize any impact that air-mass-dependent biases could have on the calculated anomalies. This analysis is repeated for all combinations of pairs of stations within the study area. The vertical sensitivity of the TCCON measurements is explicitly taken into account by dividing the anomalies by the surface layer column averaging kernel value, as we assume that the anomalies are due to emissions near the surface. The slopes computed for each year and each pair of stations are shown in Fig. 2.
The farthest distance between the European TCCON stations included in this study is between Orléans and Białystok (1580 km). Climatological annual mean surface wind speeds from the National Centers for Environmental Prediction (NCEP) and National Center for Atmospheric Research (NCAR) reanalysis (Kalnay et al., 1996) within the study area are about 6 km h−1 (Fig. A1). The air from Orléans will quickly mix vertically from the surface where the winds aloft are more rapid than at the surface (see Appendix Appendix B). Thus, air from Orléans would normally reach Białystok in a few days. To determine whether these anomalies are consistent throughout the transport time through the study area, we compute anomalies between sites lagged by up to 14 days. The slopes of the anomalies do not change significantly or systematically with the lag time (Appendix Appendix B; Fig. A2), presumably because the atmospheric composition within the study area is relatively well-mixed or because the emissions are relatively consistent from day to day within the study area.
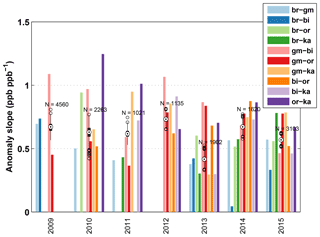
Figure 2The bars show the methane to carbon monoxide anomaly slopes for each site pair. The method of computing these anomaly slopes is detailed in Sect. 2 of the main text. The black targets indicate the median value of the slope for that year, when all site pairs are considered simultaneously, and the 25th and 75th quartiles of the median value are indicated by the vertical black bars. Outliers are indicated by open black circles.
Previous papers have used carbon dioxide instead of carbon monoxide to infer methane emissions. We choose to compute emissions using measurements of XCO instead of in this work because the natural CO2 fluxes in the region are large compared with the anthropogenic emissions, and they have a strong diurnal and seasonal cycle. The distance between the stations is large enough that local (sub-daily) uptake of CO2 differs from station to station, significantly obscuring the relationships between methane and carbon dioxide, and thus the anomaly slopes, especially in the summer months. While the emissions inventory of anthropogenic CO2 may be more accurate than the CO inventory in the region, the presence of these large natural fluxes of CO2 precludes its use in the anomaly slope calculation. The accuracy of our method, therefore, is limited by the accuracy of the carbon monoxide emission inventory. Fires could provide a large flux of CO without a large CH4 flux, and this should also be taken into consideration in these types of analyses. In our study area fluxes from fires are small.
2.1 Inventories
To obtain an estimate of carbon monoxide emissions (ECO) within the study area, we use gridded inventories and sum the emissions within the study area to compare with our emissions inferred from the TCCON measurements (see Appendix Appendix C and Fig. A3 for details). The two inventories employed here are the Emissions Database for Global Atmospheric Research (EDGAR) and the Netherlands Organisation for Applied Scientific Research (TNO) high-resolution emissions database developed as part of the Monitoring Atmospheric Composition and Climate project (TNO-MACC_III). The EDGAR version v4.3.1_v2 of January 2016 annual gridded inventory is available at spatial resolution and reports global emissions from the year 2000 to 2010 (Olivier et al., 1994; EC-JRC and PBL, 2016). The TNO-MACC_III inventory is a Europe-specific air quality emissions inventory, available on a grid, and reports emissions for 2000–2011 (Kuenen et al., 2014). Both EDGAR and TNO-MACC_III provide spatially and temporally coincident methane inventories which we use to compare with our inferred emissions. We use the EDGAR version v4.2 FT2010 and the TNO-MACC_III methane inventories.
Using country-level emissions reported through 2015 from the European Environment Agency (EEA, 2015), we extrapolate the EDGAR and TNO-MACC_III gridded inventory CO emissions for the study area through 2015. This facilitates more direct comparisons with the TCCON measurements, which begin with sufficient data for our study in 2009. We extrapolate the emissions by scaling the total emissions from the countries that are intersected by the area of interest (Germany, Poland, Belgium, France, Luxembourg, and the Czech Republic) to the last reported year of emissions from the inventory. We then assume that the same scaling factor applies for each subsequent year. The details of the extrapolation method are in Appendix Appendix D and Figs. A4 and A5.
The time series of the reported emissions from 2000 to 2015 are shown in Fig. 3. The inventories and scaled country-level reported emissions for this region suggest that emissions of CO and CH4 have decreased by about 40 % and 20 %, respectively, between 2000 and 2015. The TNO-MACC_III carbon monoxide emissions are on average 15 % higher than the EDGAR v.4.3.1 emissions in the study area. The total TNO-MACC_III and EDGAR methane emissions agree to within 2 % in the study area.
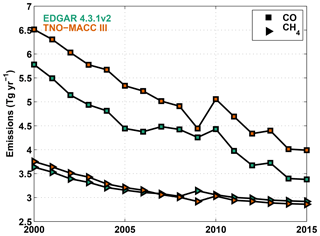
Figure 3This figure shows the summed EDGAR (green) and TNO-MACC_III (orange) emissions within the study area for CO (squares) and CH4 (triangles). The study area is defined in Fig. 1. All emissions are shown in units of Tg yr−1. Extrapolation begins after 2010 for EDGAR and 2011 for TNO-MACC_III.
An earlier version of the EDGAR carbon monoxide inventory was evaluated by Stavrakou and Müller (2006) and Fortems-Cheiney et al. (2009), who assimilated satellite measurements of CO using the EDGAR v3.3FT2000 CO emissions inventory as the a priori. Stavrakou and Müller (2006) found that, over Europe, the a posteriori emissions increase by less than 15 % when assimilating carbon monoxide from the Measurements of Pollution in the Troposphere (MOPITT) satellite instrument (Emmons et al., 2004). Fortems-Cheiney et al. (2009) assimilated Infrared Atmospheric Sounding Interferometer (IASI) CO (Clerbaux et al., 2009) and MOPITT CO and found that the a posteriori emissions increase by 16 % and 45 %, respectively.
The more recent EDGAR v4.3.1 CO emissions in our study are 24 % lower than the EDGAR v3.3FT2000 CO emissions for the year 2000, so it may be that the EDGAR v4.3.1 CO emissions are significantly underestimated. However, assimilations of CO are known to be very sensitive to the chemistry described in the model: most notably the OH chemistry (Protonotariou et al., 2010; Yin et al., 2015). Therefore, it is difficult to determine how much of the discrepancy between versions of the model is from the inventory or the model chemistry.
The EDGAR methane inventory has been evaluated in several previous studies. It has been shown to overestimate regional CH4 emissions (e.g., Wunch et al., 2009; Wecht et al., 2014) but to underestimate oil and gas emissions (e.g., Miller et al., 2013; Buchwitz et al., 2017). However, recent methane isotope analysis by Röckmann et al. (2016) has suggested that the EDGAR inventory overestimates fossil-fuel-related emissions. The study area of interest here has little oil and gas production, except for some test sites in Poland (USEIA, 2015), no commercial shale gas industry, and few pipelines.
2.2 Model experiment
To test whether the anomaly method described in Sect. 2 can accurately infer methane emissions, we conducted a modeling experiment using version v12.1.0 of the GEOS-Chem model (http://www.geos-chem.org, last access: 4 January 2019) to simulate methane and carbon monoxide for the year 2010. The model is driven by the Modern-Era Retrospective analysis for Research and Applications, version 2 (MERRA-2) meteorology from the NASA Global Modeling and Assimilation Office. The native resolution of the meteorological fields is , with 72 vertical levels from the surface to 0.01 hPa, which we degraded to and 47 vertical levels. We use the linear CO-only and CH4-only simulations of GEOS-Chem, with prescribed monthly mean OH fields. In the CO-only simulation, global anthropogenic emissions are from EDGAR v4.3.1, which are overwritten regionally with the following emissions: the Cooperative Programme for Monitoring and Evaluation of the Long-range Transmission of Air Pollutants in Europe (EMEP), the U.S. Environmental Protection Agency National Emission Inventory for 2011 (NEI2011), the MIX inventory for Asia, the Visibility Observational (BRAVO) Study Emissions Inventory for Mexico, and the criteria air contaminants (CAC) inventory for Canada. The sources of CO from the oxidation of CH4 and volatile organic compounds (VOCs) are prescribed following Fisher et al. (2017). For the CH4-only simulation, the emissions are as described in Maasakkers et al. (2019). Global anthropogenic emissions are from EDGAR v4.3.2, but the US emissions were replaced with those from Maasakkers et al. (2016), and emissions from wetlands are from WetCHARTs version 1.0 (Bloom et al., 2017). For both CO and CH4 simulations, emissions from biomass burning are from the Quick Fire Emissions Dataset (QFED) (Darmenov and Silva, 2015). The biomass burning in the study area produces less than 2 % of the total anthropogenic emissions of CO.
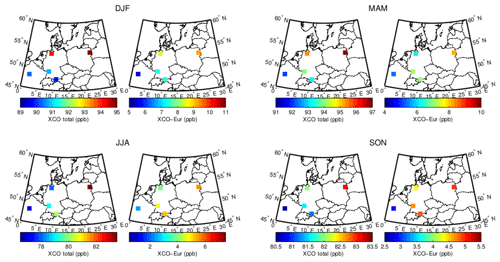
Figure 4This figure compares seasonally averaged modeled total XCO with the XCO contribution from emissions in Europe. Each season has two maps: the left map shows the total XCO and the right map shows the contribution from European emissions (XCO−Eur). The spatial pattern of the gradients in modeled XCO between the TCCON stations is reflected in the European contribution.
We used identical OH fields (from version v7-02-03 of GEOS-Chem) for the CO and CH4 simulations, so that the chemical losses of methane and carbon monoxide are consistent, and ran tagged CO experiments so that we could identify the source of the emissions. The model atmospheric carbon monoxide and methane profiles were integrated to compute simulated XCO and . To illustrate the sensitivity of the modeled fields to European emissions, we show the seasonal means of the modeled XCO sampled at the five TCCON stations in Fig. 4. Also plotted is the column contribution (XCO−Eur) from CO emissions only in Europe (defined as the broader region between 0–45∘ E and 45–55∘ N). As can be seen, the spatial pattern of the differences in modeled XCO between the TCCON stations is reflected in XCO−Eur. We calculated the anomalies in XCO and XCO−Eur, using the same approach employed with the atmospheric data, and found that the anomalies in XCO−Eur, which represent the direct influence of European emissions on atmospheric CO, account for about 35 % of the anomalies in XCO. This confirms that the XCO anomalies between the TCCON stations are sensitive to European emissions.
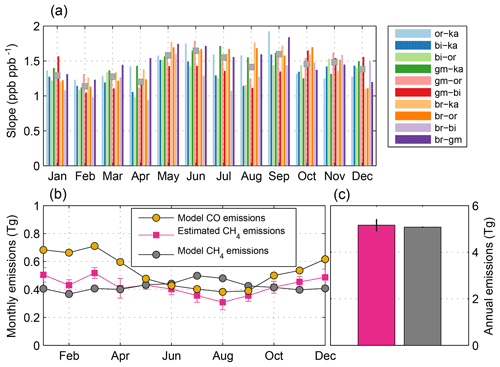
Figure 5This figure shows the results from the modeling experiment using GEOS-Chem. Panel (a) shows the model –ΔXCO slopes for each month and pair of stations (indicated by the colors). The median slopes for each month are overlaid with grey squares. Panel (b) shows the model carbon monoxide emissions (excluding VOC and methane oxidation) and the model methane emissions. The inferred methane emissions from our tracer–tracer slope method are plotted in pink squares. Panel (c) shows the annual methane emissions from the tracer–tracer slope method and the model.
To estimate the modeled CH4 emissions using the modeled CO, the modeled XCO and were interpolated to the locations of the TCCON stations and anomalies and slopes were computed. We then applied Eq. (1) to our anomaly slopes to compute methane emissions from the known CO emissions, accounting for only the CO emissions from anthropogenic, biomass burning, and biofuel sources. We neglect sources of CO emissions from the oxidation of CH4 and VOCs because the column enhancements for those emissions are relatively spatially uniform across this region of Europe, and thus they should not contribute significantly to the anomalies. The resulting annual CH4 emissions agree well with the model emissions: the inferred emissions from the anomaly analysis are higher than the model emissions by less than 2 % (Fig. 5).
While the inferred annual emissions agree well with the modeled annual emissions, the seasonal pattern of the emissions inferred from the anomaly analysis differs from that of the model. The anomaly analysis overestimates emissions in the winter and underestimates emissions in the summer. This may be due to small spatial inhomogeneities in the column enhancements from VOC (biogenic) emissions that influence the anomaly analysis most in summertime when VOC emissions are largest. Including the VOC emissions in the total carbon monoxide emissions leads us to infer annual methane emissions that are overestimated by 15 %, increasing the inferred summertime emissions without significantly changing the inferred wintertime emissions.
The seasonal analysis suggests that the 2 % agreement in the annual emission estimate may reflect the compensating effects of discrepancies over the seasonal cycle, and improving the seasonal estimate may require a better treatment of the VOC contribution to atmospheric CO. Nevertheless, the results here suggest that for this region of Europe, where VOC and methane oxidation emissions lead to relatively spatially uniform column enhancements and fire emissions are small, we can successfully use the anomaly method described in Sect. 2 to infer annual methane emissions.
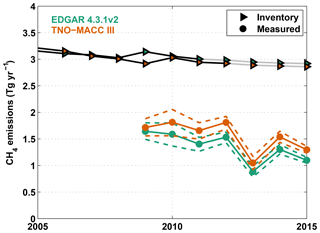
Figure 6The black line is the summed EDGAR (green) and TNO-MACC_III (orange) methane emissions within the study area shown in Fig. 1. The grey lines indicate the projected emissions based on scaling the country-level emissions reported by the UNFCCC (UNFCCC, 2017) to the area emissions in 2010 for EDGAR and 2011 for TNO-MACC_III. The lower solid lines show the emissions inferred from the TCCON anomaly analysis using CO emissions from the two models, and the dashed lines indicate the 5th and 95th percentiles.
To compute methane emissions, we apply Eq. (1) to our anomaly slopes and the inventory-reported carbon monoxide emissions in the study region (Fig. 6). If we choose the mean of the reported CO emissions from EDGAR v4.3.1 and TNO-MACC_III, the methane emissions we compute within the study area based on the TCCON measurements are 1.7±0.3 Tg yr−1 in 2009, with a non-monotonic decrease to 1.2±0.3 Tg yr−1 in 2015. The uncertainties quoted here are from the standard errors on the data slope fitting only; we have not included uncertainties from the inventories. The magnitude of methane emissions we compute from the TCCON data are, on average, about 2.3 times lower than the methane emissions reported by EDGAR and about 2 times lower than the methane emissions reported by TNO-MACC_III.
Our method of inferring methane emissions depends critically on the carbon monoxide inventory. The carbon monoxide emissions for 2010 in the study area from our GEOS-Chem model run, derived from EMEP emissions, were 6.4 Tg, about 35 % higher than the average of the EDGAR and TNO-MACC_III emissions for that year. This magnitude underestimate has also been suggested by Stavrakou and Müller (2006) and Fortems-Cheiney et al. (2009) using independent data. Using the GEOS-Chem carbon monoxide emissions increases the methane emissions inferred by the anomaly analysis to 2.4±0.3 Tg in 2010. This value remains lower than the EDGAR and TNO-MACC_III methane emissions estimates for 2010, which are 3 Tg, but by only 20 %. Therefore, we find that the inventories likely overestimate methane emissions, but the accuracy of our results relies on the accuracy of the carbon monoxide inventory.
Although the EDGAR and TNO-MACC_III inventories agree to within 15 % in carbon monoxide emissions and 2 % in methane emissions in the study region, they spatially distribute these emissions differently. Maps of the spatial differences between the TNO-MACC_III and EDGAR emissions are shown in Fig. 7 for carbon monoxide and Fig. 8 for methane. EDGAR estimates larger emissions of carbon monoxide from the main cities in the study region and the surrounding areas. This is clearly visible from the difference map (Fig. 7), where cities such as Hamburg, Berlin, Prague, Wrocław, Warsaw, Munich, Paris, and Vienna appear in blue. However, the overall carbon monoxide emissions from TNO-MACC_III in the study area are higher than EDGAR, and this comes from regions between the main cities, particularly in Poland and eastern France.
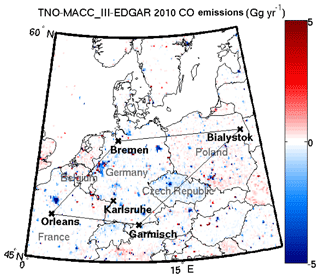
Figure 7This map shows the difference between the TNO-MACC_III carbon monoxide emissions and the EDGAR emissions for the year 2010. The black straight lines delineate the study area from the surrounding region. The TCCON stations included in this study are marked with black “x” symbols and labeled in black bold font. The countries intersected by or contained within the study area are labeled in grey. Warm (red) colors indicate that the TNO-MACC_III inventory is larger than the EDGAR inventory; cool (blue) colors indicate that the EDGAR inventory is larger than TNO-MACC_III.
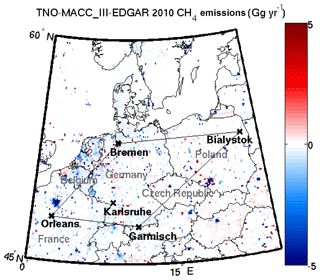
Figure 8This map shows the difference between the TNO-MACC_III methane emissions and the EDGAR emissions for the year 2010. The labeling and coloring follows that in Fig. 7.
The differences between EDGAR and TNO-MACC_III methane emissions also show that the EDGAR emissions estimates near large cities are significantly larger (Fig. 8). In contrast to the carbon monoxide spatial distribution, the TNO-MACC_III methane emissions are generally smaller everywhere, except for discrete point sources.
Comparing country-level carbon monoxide emissions reported in 2010 with the inventories shows reasonable agreement, which is expected since the inventories use country-level reports as input. The sum of the carbon monoxide emissions within the entire countries of Germany, Poland, France, Luxembourg, Belgium, and the Czech Republic differ between EDGAR and TNO-MACC_III by 18 %, with EDGAR estimates lower than those from TNO-MACC_III. Emissions from Germany, most of which are included in the study area, differ by only 6 % between EDGAR and TNO-MACC_III, again with EDGAR estimates lower than TNO-MACC_III. The national carbon monoxide emissions reported to the Convention on Long-range Transboundary Air Pollution (LRTAP Convention, https://www.eea.europa.eu/ds_resolveuid/0156b7a0ca47485593e7754c52c24afd, last access: 15 November 2017, EEA, 2015) agree to within a few percent of the TNO-MACC_III country-level emissions (e.g., 5.5 % for Germany in 2010).
The differences between 2010 country-level emissions estimates are larger for methane: EDGAR estimates are larger than TNO-MACC_III estimates by 36 % when summing all countries intersected by the study area and 8 % when considering only German emissions. The TNO-MACC_III country-level emissions estimates agree to within a few percent of the UNFCCC (http://di.unfccc.int/time_series, last access: 15 November 2017) country-level reported methane emissions (e.g., 8 % for Germany in 2010).
The differences between the EDGAR and TNO-MACC_III inventories suggest that the spatial distribution of emissions is less certain than the larger-scale emissions, since the total carbon monoxide and methane emissions between the inventories agree to within 15 % and 2 %, respectively, in the study area, but these estimates can disagree by a factor of 2 on city-level scales.
If we assume that the national-scale methane emissions are correctly reported in EDGAR and TNO-MACC_III, our results indicate that the methane emissions in the region are incorrectly spatially distributed in the inventories. It could be that point or urban sources outside the study area but within the countries intersected by the study area emit a larger proportion of the country-level emissions than previously thought.
Using co-located measurements of methane and carbon monoxide from five long-running ground-based atmospheric observing stations, we have shown that in the area of Europe between Orléans, Bremen, Białystok, and Garmisch-Partenkirchen, the inventories likely overestimate methane emissions and point to a large uncertainty in the spatial distribution (i.e., the spatial disaggregation) of country-level emissions. However, the magnitude of our inferred methane emissions relies heavily on the EDGAR v4.3.1 and TNO-MACC_III carbon monoxide inventories, and thus there is a need for rigorous validation of the carbon monoxide inventories.
This study demonstrates the potential of clusters of long-term ground-based stations monitoring total columns of atmospheric greenhouse and tracer gases. It also shows the potential of having co-located measurements of multiple pollutants to derive better estimates of emissions. These types of observing systems can help policy makers verify that greenhouse gas emissions are reducing at a rate necessary to meet regulatory obligations. The atmospheric measurements are agnostic to the source (and country of origin) of the methane, measuring only what is emitted into the atmosphere in a given area. Thus, they can help validate and reveal inadequacies in the current inventories, and, in particular, how country-wide emission reports are disaggregated on a grid. To enhance these results, simultaneous measurements of complementary atmospheric trace gases, such as ethane, acetylene, nitrous oxide, nitrogen dioxide, ammonia, and isotopes, would help distinguish between sources of methane. This would provide additional valuable information that would likely improve inventory disaggregation.
TCCON data are available from the TCCON archive, hosted by the California Institute of Technology at https://tccondata.org. Karlsruhe data were obtained from https://doi.org/10.14291/tccon.ggg2014.karlsruhe01.R1/1182416 (Hase et al., 2014). Bremen data were obtained from https://doi.org/10.14291/tccon.ggg2014.bremen01.R0/1149275 (Notholt et al., 2014). Garmisch data were obtained from https://doi.org/10.14291/tccon.ggg2014.garmisch01.R0/1149299 (Sussmann and Rettinger, 2014). Orléans data were obtained from https://doi.org/10.14291/tccon.ggg2014.orleans01.R0/1149276 (Warneke et al., 2014). Bialystok data were obtained from https://doi.org/10.14291/tccon.ggg2014.bialystok01.R1/1183984 (Deutscher et al., 2017). The Emissions Database for Global Atmospheric Research (EDGAR) inventory is available from the European Commission Joint Research Centre (JRC) and the Netherlands Environmental Assessment Agency (PBL), http://edgar.jrc.ec.europa.eu (last access: 7 April 2017). The GEOS-Chem v12.1.0 model is available from https://doi.org/10.5281/zenodo.1553349 (The International GEOS-Chem User Community, 2018).
DW designed the study, performed the analysis, and wrote the paper. DBAJ ran the GEOS-Chem model, supported by the CO and CH4 work of JAF and JDM. JK and HDvdG provided the TNO-MACC_III inventory. GCT helped refine the data analysis methodology. NMD, FH, JN, RS, and TW provided TCCON data. All coauthors read and provided feedback on the contents of the paper and helped interpret the results.
The authors declare no competing interests.
The NASA Earth Observatory images were prepared by Joshua Stevens, using Suomi National Polar-orbiting Partnership (NPP) Visible Infrared Imaging Radiometer (VIIRS) data from Miguel Román, at NASA's Goddard Space Flight Center. The authors would like to thank two anonymous reviewers for thoughtful comments and suggestions that significantly strengthened the paper.
This paper was edited by William Lahoz and reviewed by two anonymous referees.
Alexe, M., Bergamaschi, P., Segers, A., Detmers, R., Butz, A., Hasekamp, O., Guerlet, S., Parker, R., Boesch, H., Frankenberg, C., Scheepmaker, R. A., Dlugokencky, E., Sweeney, C., Wofsy, S. C., and Kort, E. A.: Inverse modelling of CH4 emissions for 2010–2011 using different satellite retrieval products from GOSAT and SCIAMACHY, Atmos. Chem. Phys., 15, 113–133, https://doi.org/10.5194/acp-15-113-2015, 2015. a
Aydin, M., Verhulst, K. R., Saltzman, E. S., Battle, M. O., Montzka, S. A., Blake, D. R., Tang, Q., and Prather, M. J.: Recent decreases in fossil-fuel emissions of ethane and methane derived from firn air, Nature, 476, 198–201, https://doi.org/10.1038/nature10352, 2011. a
Baker, A. K., Schuck, T. J., Brenninkmeijer, C. A. M., Rauthe-Schöch, A., Slemr, F., van Velthoven, P. F. J., and Lelieveld, J.: Estimating the contribution of monsoon-related biogenic production to methane emissions from South Asia using CARIBIC observations, Geophys. Res. Lett., 39, L10813, https://doi.org/10.1029/2012GL051756, 2012. a
Bloom, A. A., Bowman, K. W., Lee, M., Turner, A. J., Schroeder, R., Worden, J. R., Weidner, R., McDonald, K. C., and Jacob, D. J.: A global wetland methane emissions and uncertainty dataset for atmospheric chemical transport models (WetCHARTs version 1.0), Geosci. Model Dev., 10, 2141–2156, https://doi.org/10.5194/gmd-10-2141-2017, 2017. a
Buchwitz, M., Schneising, O., Reuter, M., Heymann, J., Krautwurst, S., Bovensmann, H., Burrows, J. P., Boesch, H., Parker, R. J., Somkuti, P., Detmers, R. G., Hasekamp, O. P., Aben, I., Butz, A., Frankenberg, C., and Turner, A. J.: Satellite-derived methane hotspot emission estimates using a fast data-driven method, Atmos. Chem. Phys., 17, 5751–5774, https://doi.org/10.5194/acp-17-5751-2017, 2017. a
Chen, J., Viatte, C., Hedelius, J. K., Jones, T., Franklin, J. E., Parker, H., Gottlieb, E. W., Wennberg, P. O., Dubey, M. K., and Wofsy, S. C.: Differential column measurements using compact solar-tracking spectrometers, Atmos. Chem. Phys., 16, 8479–8498, https://doi.org/10.5194/acp-16-8479-2016, 2016. a, b
Clerbaux, C., Boynard, A., Clarisse, L., George, M., Hadji-Lazaro, J., Herbin, H., Hurtmans, D., Pommier, M., Razavi, A., Turquety, S., Wespes, C., and Coheur, P.-F.: Monitoring of atmospheric composition using the thermal infrared IASI/MetOp sounder, Atmos. Chem. Phys., 9, 6041–6054, https://doi.org/10.5194/acp-9-6041-2009, 2009. a
Darmenov, A. S. and Silva, A.: The Quick Fire Emissions Dataset (QFED): Documentation of versions 2.1, 2.2 and 2.4, NASA Technical Report Series on Global Modeling and Data Assimilation, NASA/TM-2015-104606, Tech. Rep. September, NASA, Goddard Space Flight Center, Greenbelt, Maryland, 2015. a
Deutscher, N. M., Notholt, J., Messerschmidt, J., Weinzierl, C., Warneke, T., Petri, C., and Grupe, P.: TCCON data from Bialystok (PL), Release GGG2014.R1, CaltechDATA, https://doi.org/10.14291/tccon.ggg2014.bialystok01.r1/1183984, 2017. a, b
Dlugokencky, E. J., Nisbet, E. G., Fisher, R., and Lowry, D.: Global atmospheric methane: budget, changes and dangers, Philos. T. R. Soc. A., 369, 2058–72, https://doi.org/10.1098/rsta.2010.0341, 2011. a
EC-JRC and PBL: Emission Database for Global Atmospheric Research (EDGAR), release EDGAR v4.3.1_v2 (1970–2010), available at: http://edgar.jrc.ec.europa.eu (last access: 16 October 2017), 2016. a
EEA: National emissions reported to the Convention on Long-range Transboundary Air Pollution (LRTAP Convention) (database), Tech. rep., available at: http://www.eea.europa.eu/data-and-maps/data/national-emissions- reported-to-the-convention-on-long-range-transboundary-air-pollution-lrtap-convention-9 (last access: 15 November 2017), 2015. a, b
Emmons, L. K., Deeter, M. N., Gille, J. C., Edwards, D. P., Attié, J.-L., Warner, J., Ziskin, D., Francis, G., Khattatov, B., Yudin, V., Lamarque, J.-F., Ho, S.-P., Mao, D., Chen, J. S., Drummond, J., Novelli, P., Sachse, G., Coffey, M. T., Hannigan, J. W., Gerbig, C., Kawakami, S., Kondo, Y., Takegawa, N., Schlager, H., Baehr, J., and Ziereis, H.: Validation of Measurements of Pollution in the Troposphere (MOPITT) CO retrievals with aircraft in situ profiles, J. Geophys. Res.-Atmos., 109, D03309, https://doi.org/10.1029/2003JD004101, 2004. a
Kona, A., Melica, G., Koffi, B., Iancu, A., Zancanella, P., Rivas Calvete, S., Bertoldi, P., Janssens-Maenhout, G., and Monforti-Ferrario, F.: Covenant of Mayors: Greenhouse Gas Emissions Achievement and Projections, EUR 28155 EN, Publications Office of the European Union, Luxembourg, https://doi.org/10.2790/11008, 2016. a
European Environment Agency: Annual European Union greenhouse gas inventory 1990–2014 and inventory report 2016, Tech. rep., available at: http://www.eea.europa.eu/publications/european-union-greenhouse-gas-inventory-2013 (last access: 26 July 2018), 2016. a
Fisher, J. A., Murray, L. T., Jones, D. B. A., and Deutscher, N. M.: Improved method for linear carbon monoxide simulation and source attribution in atmospheric chemistry models illustrated using GEOS-Chem v9, Geosci. Model Dev., 10, 4129–4144, https://doi.org/10.5194/gmd-10-4129-2017, 2017. a
Fortems-Cheiney, A., Chevallier, F., Pison, I., Bousquet, P., Carouge, C., Clerbaux, C., Coheur, P.-F., George, M., Hurtmans, D., and Szopa, S.: On the capability of IASI measurements to inform about CO surface emissions, Atmos. Chem. Phys., 9, 8735–8743, https://doi.org/10.5194/acp-9-8735-2009, 2009. a, b, c
Frankenberg, C., Thorpe, A. K., Thompson, D. R., Hulley, G., Kort, E. A., Vance, N., Borchardt, J., Krings, T., Gerilowski, K., Sweeney, C., Conley, S., Bue, B. D., Aubrey, A. D., Hook, S., and Green, R. O.: Airborne methane remote measurements reveal heavy-tail flux distribution in Four Corners region, P. Natl. Acad. Sci. USA, 113, 35, 9734–9739, https://doi.org/10.1073/pnas.1605617113, 2016. a
Geibel, M. C., Messerschmidt, J., Gerbig, C., Blumenstock, T., Chen, H., Hase, F., Kolle, O., Lavrič, J. V., Notholt, J., Palm, M., Rettinger, M., Schmidt, M., Sussmann, R., Warneke, T., and Feist, D. G.: Calibration of column-averaged CH4 over European TCCON FTS sites with airborne in-situ measurements, Atmos. Chem. Phys., 12, 8763–8775, https://doi.org/10.5194/acp-12-8763-2012, 2012. a
Hase, F., Blumenstock, T., Dohe, S., Gross, J., and Kiel, M.: TCCON data from Karlsruhe (DE), Release GGG2014R1, TCCON data archive, hosted by CaltechDATA, https://doi.org/10.14291/tccon.ggg2014.karlsruhe01.R1/1182416, 2014. a, b
Hase, F., Frey, M., Blumenstock, T., Groß, J., Kiel, M., Kohlhepp, R., Mengistu Tsidu, G., Schäfer, K., Sha, M. K., and Orphal, J.: Application of portable FTIR spectrometers for detecting greenhouse gas emissions of the major city Berlin, Atmos. Meas. Tech., 8, 3059–3068, https://doi.org/10.5194/amt-8-3059-2015, 2015. a, b
Hausmann, P., Sussmann, R., and Smale, D.: Contribution of oil and natural gas production to renewed increase in atmospheric methane (2007–2014): top-down estimate from ethane and methane column observations, Atmos. Chem. Phys., 16, 3227–3244, https://doi.org/10.5194/acp-16-3227-2016, 2016. a
Hopkins, F. M., Kort, E. A., Bush, S. E., Ehleringer, J. R., Lai, C.-T., Blake, D. R., and Randerson, J. T.: Spatial patterns and source attribution of urban methane in the Los Angeles Basin, J. Geophys. Res.-Atmos., 121, 2490–2507, https://doi.org/10.1002/2015JD024429, 2016. a
Houweling, S., Krol, M., Bergamaschi, P., Frankenberg, C., Dlugokencky, E. J., Morino, I., Notholt, J., Sherlock, V., Wunch, D., Beck, V., Gerbig, C., Chen, H., Kort, E. A., Röckmann, T., and Aben, I.: A multi-year methane inversion using SCIAMACHY, accounting for systematic errors using TCCON measurements, Atmos. Chem. Phys., 14, 3991–4012, https://doi.org/10.5194/acp-14-3991-2014, 2014. a
Jacob, D. J.: Introduction to Atmospheric Chemistry, Princeton University Press, Princeton, New Jersey, 1999. a
Jacob, D. J., Crawford, J. H., Kleb, M. M., Connors, V. S., Bendura, R. J., Raper, J. L., Sachse, G. W., Gille, J. C., Emmons, L., and Heald, C. L.: Transport and Chemical Evolution over the Pacific (TRACE-P) aircraft mission: Design, execution, and first results, J. Geophys. Res., 108, 9000, https://doi.org/10.1029/2002JD003276, 2003. a
Jeong, S., Cui, X., Blake, D. R., Miller, B., Montzka, S. A., Andrews, A., Guha, A., Martien, P., Bambha, R. P., LaFranchi, B., Michelsen, H. A., Clements, C. B., Glaize, P., and Fischer, M. L.: Estimating methane emissions from biological and fossil-fuel sources in the San Francisco Bay Area, Geophys. Res. Lett., 44, 1, 486–495, https://doi.org/10.1002/2016GL071794, 2017. a
Kalnay, E., Kanamitsu, M., Kistler, R., Collins, W., Deaven, D., Gandin, L., Iredell, M., Saha, S., White, G., Woollen, J., Zhu, Y., Leetmaa, A., Reynolds, R., Chelliah, M., Ebisuzaki, W., Higgins, W., Janowiak, J., Mo, K. C., Ropelewski, C., Wang, J., Jenne, R., and Joseph, D.: The NCEP/NCAR 40-Year Reanalysis Project, B. Am. Meteorol. Soc., 77, 437–471, https://doi.org/10.1175/1520-0477(1996)077<0437:TNYRP>2.0.CO;2, 1996. a, b
Karion, A., Sweeney, C., Miller, J. B., Andrews, A. E., Commane, R., Dinardo, S., Henderson, J. M., Lindaas, J., Lin, J. C., Luus, K. A., Newberger, T., Tans, P., Wofsy, S. C., Wolter, S., and Miller, C. E.: Investigating Alaskan methane and carbon dioxide fluxes using measurements from the CARVE tower, Atmos. Chem. Phys., 16, 5383–5398, https://doi.org/10.5194/acp-16-5383-2016, 2016. a
Kort, E. A., Eluszkiewicz, J., Stephens, B. B., Miller, J. B., Gerbig, C., Nehrkorn, T., Daube, B. C., Kaplan, J. O., Houweling, S., and Wofsy, S. C.: Emissions of CH4 and N2O over the United States and Canada based on a receptor-oriented modeling framework and COBRA-NA atmospheric observations, Geophys. Res. Lett., 35, 1–5, https://doi.org/10.1029/2008GL034031, 2008. a
Kort, E. A., Andrews, A. E., Dlugokencky, E. J., Sweeney, C., Hirsch, A., Eluszkiewicz, J., Nehrkorn, T., Michalak, A. M., Stephens, B. B., Gerbig, C., Miller, J. B., Kaplan, J., Houweling, S., Daube, B. C., Tans, P. P., and Wofsy, S. C.: Atmospheric constraints on 2004 emissions of methane and nitrous oxide in North America from atmospheric measurements and a receptor-oriented modeling framework, J. Integr. Environ. Sci., 7, 125–133, https://doi.org/10.1080/19438151003767483, 2010. a
Kort, E. A., Frankenberg, C., Miller, C. E., and Oda, T.: Space-based Observations of Megacity Carbon Dioxide, Geophys. Res. Lett., 39, 1–5, https://doi.org/10.1029/2012GL052738, 2012. a
Kort, E. A., Frankenberg, C., Costigan, K. R., Lindenmaier, R., Dubey, M. K., and Wunch, D.: Four corners: The largest US methane anomaly viewed from space, Geophys. Res. Lett., 41, 6898–6903, https://doi.org/10.1002/2014GL061503, 2014. a
Kuenen, J. J. P., Visschedijk, A. J. H., Jozwicka, M., and Denier van der Gon, H. A. C.: TNO-MACC_II emission inventory; a multi-year (2003–2009) consistent high-resolution European emission inventory for air quality modelling, Atmos. Chem. Phys., 14, 10963–10976, https://doi.org/10.5194/acp-14-10963-2014, 2014. a
Maasakkers, J. D., Jacob, D. J., Sulprizio, M. P., Turner, A. J., Weitz, M., Wirth, T., Hight, C., DeFigueiredo, M., Desai, M., Schmeltz, R., Hockstad, L., Bloom, A. A., Bowman, K. W., Jeong, S., and Fischer, M. L.: Gridded National Inventory of U.S. Methane Emissions, Environ. Sci. Technol., 50, 13123–13133, https://doi.org/10.1021/acs.est.6b02878, 2016. a
Maasakkers, J. D., Jacob, D. J., Sulprizio, M. P., Scarpelli, T. R., Nesser, H., Sheng, J.-X., Zhang, Y., Hersher, M., Bloom, A. A., Bowman, K. W., Worden, J. R., Janssens-Maenhout, G., and Parker, R. J.: Global distribution of methane emissions, emission trends, and OH concentrations and trends inferred from an inversion of GOSAT satellite data for 2010–2015, Atmos. Chem. Phys. Discuss., https://doi.org/10.5194/acp-2018-1365, in review, 2019. a
McKain, K., Down, A., Raciti, S. M., Budney, J., Hutyra, L. R., Floerchinger, C., Herndon, S. C., Nehrkorn, T., Zahniser, M. S., Jackson, R. B., Phillips, N., and Wofsy, S. C.: Methane emissions from natural gas infrastructure and use in the urban region of Boston, Massachusetts, P. Natl. Acad. Sci. USA, 112, 1941–1946, https://doi.org/10.1073/pnas.1416261112, 2015. a
Messerschmidt, J., Geibel, M. C., Blumenstock, T., Chen, H., Deutscher, N. M., Engel, A., Feist, D. G., Gerbig, C., Gisi, M., Hase, F., Katrynski, K., Kolle, O., Lavrič, J. V., Notholt, J., Palm, M., Ramonet, M., Rettinger, M., Schmidt, M., Sussmann, R., Toon, G. C., Truong, F., Warneke, T., Wennberg, P. O., Wunch, D., and Xueref-Remy, I.: Calibration of TCCON column-averaged CO2: the first aircraft campaign over European TCCON sites, Atmos. Chem. Phys., 11, 10765–10777, https://doi.org/10.5194/acp-11-10765-2011, 2011. a
Miller, S. M., Wofsy, S. C., Michalak, A. M., Kort, E. A., Andrews, A. E., Biraud, S. C., Dlugokencky, E. J., Eluszkiewicz, J., Fischer, M. L., Janssens-Maenhout, G., Miller, B. R., Miller, J. B., Montzka, S. A., Nehrkorn, T., and Sweeney, C.: Anthropogenic emissions of methane in the United States., P. Natl. Acad. Sci. USA, 110, 20018–20022, https://doi.org/10.1073/pnas.1314392110, 2013. a
Nassar, R., Hill, T. G., McLinden, C. A., Wunch, D., Jones, D. B. A., and Crisp, D.: Quantifying CO2 emissions from individual power plants from space, Geophys. Res. Lett., 44, 19, 10045–10053, https://doi.org/10.1002/2017GL074702, 2017. a
Notholt, J., Petri, C., Warneke, T., Deutscher, N. M., Buschmann, M., Weinzierl, C., Macatangay, R., and Grupe, P.: TCCON data from Bremen (DE), Release GGG2014R0, TCCON data archive, hosted by CaltechDATA, https://doi.org/10.14291/tccon.ggg2014.bremen01.R0/1149275, 2014. a, b
Olivier, J. G. J., Bouwman, A. F., van der Maas, C. W. M., and Berdowski, J. J. M.: Emission database for global atmospheric research (Edgar), Environ. Monit. Assess., 31–31, 93–106, https://doi.org/10.1007/BF00547184, 1994. a
Peischl, J., Ryerson, T. B., Brioude, J., Aikin, K. C., Andrews, A. E., Atlas, E., Blake, D. R., Daube, B. C., de Gouw, J. A., Dlugokencky, E. J., Frost, G. J., Gentner, D. R., Gilman, J. B., Goldstein, A., Harley, R. A., Holloway, J. S., Kofler, J., Kuster, W. C., Lang, P. M., Novelli, P. C., Santoni, G. W., Trainer, M., Wofsy, S. C., and Parrish, D. D.: Quantifying sources of methane using light alkanes in the Los Angeles basin, California, J. Geophys. Res.-Atmos., 118, 10, 4974–4990, https://doi.org/10.1002/jgrd.50413, 2013. a
Protonotariou, A., Tombrou, M., Giannakopoulos, C., Kostopoulou, E., and Le Sager, P.: Study of CO surface pollution in Europe based on observations and nested-grid applications of GEOS-CHEM global chemical transport model, Tellus B, 62, 209–227, https://doi.org/10.1111/j.1600-0889.2010.00462.x, 2010. a
Röckmann, T., Eyer, S., van der Veen, C., Popa, M. E., Tuzson, B., Monteil, G., Houweling, S., Harris, E., Brunner, D., Fischer, H., Zazzeri, G., Lowry, D., Nisbet, E. G., Brand, W. A., Necki, J. M., Emmenegger, L., and Mohn, J.: In situ observations of the isotopic composition of methane at the Cabauw tall tower site, Atmos. Chem. Phys., 16, 10469–10487, https://doi.org/10.5194/acp-16-10469-2016, 2016. a
Saad, K. M., Wunch, D., Toon, G. C., Bernath, P., Boone, C., Connor, B., Deutscher, N. M., Griffith, D. W. T., Kivi, R., Notholt, J., Roehl, C., Schneider, M., Sherlock, V., and Wennberg, P. O.: Derivation of tropospheric methane from TCCON CH4 and HF total column observations, Atmos. Meas. Tech., 7, 2907–2918, https://doi.org/10.5194/amt-7-2907-2014, 2014. a
Schneising, O., Reuter, M., Buchwitz, M., Heymann, J., Bovensmann, H., and Burrows, J. P.: Terrestrial carbon sink observed from space: variation of growth rates and seasonal cycle amplitudes in response to interannual surface temperature variability, Atmos. Chem. Phys., 14, 133–141, https://doi.org/10.5194/acp-14-133-2014, 2014. a
Shoemaker, J. K., Schrag, D. P., Molina, M. J., and Ramanathan, V.: What Role for Short-Lived Climate Pollutants in Mitigation Policy?, Science, 342, 1323–1324, https://doi.org/10.1126/science.1240162, 2013. a
Silva, S. J., Arellano, A. F., and Worden, H. M.: Toward anthropogenic combustion emission constraints from space-based analysis of urban CO2∕CO sensitivity, Geophys. Res. Lett., 40, 4971–4976, https://doi.org/10.1002/grl.50954, 2013. a, b
Simpson, I. J., Sulbaek Andersen, M. P., Meinardi, S., Bruhwiler, L., Blake, N. J., Helmig, D., Rowland, F. S., and Blake, D. R.: Long-term decline of global atmospheric ethane concentrations and implications for methane, Nature, 488, 490–494, https://doi.org/10.1038/nature11342, 2012. a
Stavrakou, T. and Müller, J. F.: Grid-based versus big region approach for inverting CO emissions using Measurement of Pollution in the Troposphere (MOPITT) data, J. Geophys. Res.-Atmos., 111, 1–21, https://doi.org/10.1029/2005JD006896, 2006. a, b, c
Stocker, T., Qin, D., Plattner, G.-K., Tignor, M., Allen, S., Boschung, J., Nauels, A., Xia, Y., Bex, V., and Midgley, P.: Climate change 2013: The Physical Science Basis. Contribution of Working Group I to the Fifth Assessment Report of the Intergovernmental Panel on Climate Change, p. 1535, Cambridge University Press, Cambridge, UK and New York, NY, USA, available at: https://www.ipcc.ch/report/ar5/wg1/ (last access: 25 March 2019), 2013. a
Sussmann, R. and Rettinger, M.: TCCON data from Garmisch (DE), Release GGG2014R0, TCCON data archive, hosted by CaltechDATA, https://doi.org/10.14291/tccon.ggg2014.garmisch01.R0/1149299, 2014. a, b
The International GEOS-Chem User Community: geoschem/geos-chem: GEOS-Chem 12.1.0 (Version 12.1.0), Zenodo, https://doi.org/10.5281/zenodo.1553349, 2018. a
Turner, A. J., Jacob, D. J., Wecht, K. J., Maasakkers, J. D., Lundgren, E., Andrews, A. E., Biraud, S. C., Boesch, H., Bowman, K. W., Deutscher, N. M., Dubey, M. K., Griffith, D. W. T., Hase, F., Kuze, A., Notholt, J., Ohyama, H., Parker, R., Payne, V. H., Sussmann, R., Sweeney, C., Velazco, V. A., Warneke, T., Wennberg, P. O., and Wunch, D.: Estimating global and North American methane emissions with high spatial resolution using GOSAT satellite data, Atmos. Chem. Phys., 15, 7049–7069, https://doi.org/10.5194/acp-15-7049-2015, 2015. a
UNFCCC: Paris Climate Change Conference – November 2015, COP 21, Adoption of the Paris Agreement. Proposal by the President, 21932, 32, available at: https://undocs.org/FCCC/CP/2015/L.9/Rev.1 (last access: 26 July 2018), 2015. a
UNFCCC: GHG Data – Time series, Annex I, available at: http://di.unfccc.int/time_series, last access: 15 November 2017 a, b
USEIA: World Shale Resource Assessments, available at: https://www.eia.gov/analysis/studies/worldshalegas/\%0A (last access: 20 September 2017), 2015. a
Viatte, C., Lauvaux, T., Hedelius, J. K., Parker, H., Chen, J., Jones, T., Franklin, J. E., Deng, A. J., Gaudet, B., Verhulst, K., Duren, R., Wunch, D., Roehl, C., Dubey, M. K., Wofsy, S., and Wennberg, P. O.: Methane emissions from dairies in the Los Angeles Basin, Atmos. Chem. Phys., 17, 7509–7528, https://doi.org/10.5194/acp-17-7509-2017, 2017. a, b
Warneke, T., Messerschmidt, J., Notholt, J., Weinzierl, C., Deutscher, N. M., Petri, C., Grupe, P., Vuillemin, C., Truong, F., Schmidt, M., Ramonet, M., and Parmentier, E.: TCCON data from Orléans (FR), Release GGG2014R0, TCCON data archive, hosted by CaltechDATA, https://doi.org/10.14291/tccon.ggg2014.orleans01.R0/1149276, 2014. a, b
Washenfelder, R. A., Wennberg, P. O., and Toon, G. C.: Tropospheric methane retrieved from ground-based near-IR solar absorption spectra, Geophys. Res. Lett., 30, 1–5, https://doi.org/10.1029/2003GL017969, 2003. a
Wecht, K. J., Jacob, D. J., Frankenberg, C., Jiang, Z., and Blake, D. R.: Mapping of North American methane emissions with high spatial resolution by inversion of SCIAMACHY satellite data, J. Geophys. Res.-Atmos., 119, 7741–7756, https://doi.org/10.1002/2014JD021551, 2014. a
Wofsy, S. C.: HIAPER Pole-to-Pole Observations (HIPPO): fine-grained, global-scale measurements of climatically important atmospheric gases and aerosols, Philos. T. R. Soc. A., 369, 1943, 2073–2086, https://doi.org/10.1098/rsta.2010.0313, 2011. a
Wunch, D., Wennberg, P. O., Toon, G. C., Keppel-Aleks, G., and Yavin, Y. G.: Emissions of greenhouse gases from a North American megacity, Geophys. Res. Lett., 36, 1–5, https://doi.org/10.1029/2009GL039825, 2009. a, b, c, d, e
Wunch, D., Toon, G. C., Wennberg, P. O., Wofsy, S. C., Stephens, B. B., Fischer, M. L., Uchino, O., Abshire, J. B., Bernath, P., Biraud, S. C., Blavier, J.-F. L., Boone, C., Bowman, K. P., Browell, E. V., Campos, T., Connor, B. J., Daube, B. C., Deutscher, N. M., Diao, M., Elkins, J. W., Gerbig, C., Gottlieb, E., Griffith, D. W. T., Hurst, D. F., Jiménez, R., Keppel-Aleks, G., Kort, E. A., Macatangay, R., Machida, T., Matsueda, H., Moore, F., Morino, I., Park, S., Robinson, J., Roehl, C. M., Sawa, Y., Sherlock, V., Sweeney, C., Tanaka, T., and Zondlo, M. A.: Calibration of the Total Carbon Column Observing Network using aircraft profile data, Atmos. Meas. Tech., 3, 1351–1362, https://doi.org/10.5194/amt-3-1351-2010, 2010. a
Wunch, D., Toon, G. C., Blavier, J.-F. L., Washenfelder, R. A., Notholt, J., Connor, B. J., Griffith, D. W. T., Sherlock, V., and Wennberg, P. O.: The Total Carbon Column Observing Network, Philos. T. R. Soc. A., 369, 2087–2112, https://doi.org/10.1098/rsta.2010.0240, 2011. a
Wunch, D., Toon, G. C., Sherlock, V., Deutscher, N. M., Liu, C., Feist, D. G., and Wennberg, P. O.: The Total Carbon Column Observing Network's GGG2014 Data Version, Tech. rep., California Institute of Technology, Pasadena, California, https://doi.org/10.14291/tccon.ggg2014.documentation.R0/1221662, 2015. a
Wunch, D., Toon, G. C., Hedelius, J. K., Vizenor, N., Roehl, C. M., Saad, K. M., Blavier, J.-F. L., Blake, D. R., and Wennberg, P. O.: Quantifying the loss of processed natural gas within California's South Coast Air Basin using long-term measurements of ethane and methane, Atmos. Chem. Phys., 16, 14091–14105, https://doi.org/10.5194/acp-16-14091-2016, 2016. a, b, c, d, e
Yin, Y., Chevallier, F., Ciais, P., Broquet, G., Fortems-Cheiney, A., Pison, I., and Saunois, M.: Decadal trends in global CO emissions as seen by MOPITT, Atmos. Chem. Phys., 15, 13433–13451, https://doi.org/10.5194/acp-15-13433-2015, 2015. a