the Creative Commons Attribution 4.0 License.
the Creative Commons Attribution 4.0 License.
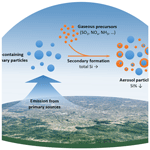
Unraveling the role of silicon in atmospheric aerosol secondary formation: a new conservative tracer for aerosol chemistry
Dawei Lu
Jihua Tan
Xuezhi Yang
Xu Sun
Guibin Jiang
Aerosol particles are ubiquitous in the atmosphere and affect the quality of human life through their climatic and health effects. The formation and growth of aerosol particles involve extremely complex reactions and processes. Due to limited research tools, the sources and chemistry of aerosols are still not fully understood, and until now have normally been investigated by using chemical species of secondary aerosols (e.g., , , , SOC) as tracers. Here we investigated the role of silicon (Si), an ubiquitous but relatively inert element, during the secondary aerosol formation process. We analyzed the correlation of Si in airborne fine particles (PM2.5) collected in Beijing – a typical pollution region – with the secondary chemical species and secondary particle precursors (e.g., SO2 and NOx). The total mass of Si in PM2.5 was found to be uncorrelated with the secondary aerosol formation process, which suggested that Si is a new conservative tracer for the amount of primary materials in PM2.5 and can be used to estimate the relative amount of secondary and primary compounds in PM2.5. This finding enables the accurate estimation of secondary aerosol contribution to PM2.5 by using Si as a single tracer rather than the commonly used multiple chemical tracers. In addition, we show that the correlation analysis of secondary aerosols with the Si isotopic composition of PM2.5 can further reveal the sources of the precursors of secondary aerosols. Therefore, Si may provide a new tool for aerosol chemistry studies.
- Article
(2007 KB) - Full-text XML
-
Supplement
(1249 KB) - BibTeX
- EndNote
Atmospheric particulate pollution is a global environmental issue that seriously threatens human health and sustainable development. The fine particulate matter less than 2.5 µm (PM2.5) in size is of great concern because it poses significant risks to human health and is a major cause of the haze phenomenon (Pope et al., 2009, 2002). Understanding the sources and chemistry of aerosols is critical for air pollution control. The aerosol particles can be directly emitted from primary sources (primary particles) or secondarily formed from gaseous precursors (e.g., SO2, NOx, NH3, and volatile organic compounds – VOCs) through complex chemical reactions and processes in the atmosphere (secondary particles) (Zhang et al., 2012b, 2015). Particularly, secondary aerosol (SA) is of great importance as it contributes to a large portion of particulate pollution in most pollution regions (e.g., China and India) (Huang et al., 2014). However, until now, the formation mechanism of secondary aerosols has not been fully understood and it is difficult to accurately estimate secondary aerosols due to the extreme complexity of aerosol chemistry and limited research tools. The traditional method is based on the combined estimates of secondary inorganic aerosol (SIA) and secondary organic aerosol (SOA) using multiple chemical components indicative of secondary chemistry, e.g., , , and for SIA (Bi et al., 2007), and organic carbon (OC) and elemental carbon (EC) for SOA (Docherty et al., 2008). However, the accuracy of the method is still controversial and measurements of these multiple tracers are laborious (Schmid et al., 2001; Watson et al., 2005).
As the second highest abundant element in the Earth's crust, silicon (Si) is ubiquitous in terrestrial systems, as well as in atmospheric aerosols. The roles of Si in continental and marine environments have been well clarified in biogeochemical studies (Basile-Doelsch, 2006). However, despite the ubiquitousness of Si, the research on atmospheric Si is rather limited (Bzdek et al., 2014; Lu et al., 2018). We note that Si is a special element compared with other high-abundance ones (e.g., O, C, N, and S). It is relatively inert, normally forms bonds only with oxygen (in SiIV), and does not form volatile compounds in the natural environment (Savage et al., 2014). In fact, over 90 % of Si in the Earth's crust is composed of nonvolatile silicate minerals that should not be able to serve as gaseous precursors for secondary aerosols (an exception is synthetic organosilicons that will be discussed later). Therefore, Si may provide a different route to aerosol chemistry from that using traditional tracers.
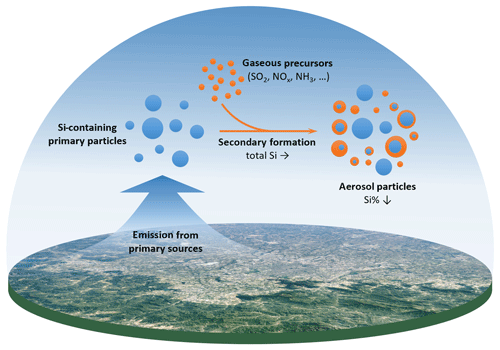
Figure 1Scheme showing the role of Si during the secondary formation process of aerosol particles. Note: this scheme only reflects the role of Si but does not show all reactions involved in the secondary aerosol formation.
In this study, we aimed to clarify the role of Si during the secondary aerosol formation process in the atmosphere. Based on the nature of Si, we hypothesize that Si in PM2.5 is unaffected by the secondary particle formation process and predominantly emitted from primary sources (as depicted in Fig. 1). Therefore, the particular properties of Si (i.e., high abundance and chemical inactiveness) may make it a new conservative tracer for aerosol chemistry studies. To specify this point, we herein show the estimation of secondary aerosols based on the dilution effect of Si during the secondary aerosol formation process by using Si as a single tracer, and we compare this with the traditional method that uses multiple chemical tracers.
2.1 Chemicals and reagents
The standard reference material of urban atmospheric particulate matter (NIST-1648a) and the Si isotope standard (NIST SRM-8546) were purchased from the National Institute of Standards and Technology (Gaithersburg, MD). The Si isotope standard IRMM-017 was purchased from the Institute for Reference Materials and Measurements (GEEL, Belgium). The element calibration standard solution was from Agilent (Santa Clara, CA). Sodium hydroxide was from Beijing Ruikai Electronic Co. (Beijing, China). Nitric acid was from Merck (Darmstadt, Germany). Hydrochloric acid was from Beijing Chemical Works (Beijing, China). Hydrogen peroxide was from Sinopharm Chemical Reagent Co. (Shanghai, China). Ultrapure water (18.3 MΩ⋅cm) obtained from a Milli-Q gradient system (Millipore, Bedford) was used.
2.2 Sampling of PM2.5 samples
The PM2.5 and primary source samples were collected around the Beijing region, China, as described in a previous report (Lu et al., 2018). Briefly, the PM2.5 samples were collected in an urban district of Beijing in 2013 by using a low-volume air sampler (Partisol 2025i, Thermo Fisher, USA) at a flow rate of 16.7 L min−1. The sampling site was ca. 20 m above the ground and surrounded by office and residential buildings (40.0481∘ N, 116.4254∘ E). We selected the days with relatively high PM2.5 concentrations in each week in 2013 (n=63) to reflect the haze pollution condition of the whole year. Several haze events over consecutive days were also included to investigate the SA formation process. The sampling dates with the PM2.5 concentrations and meteorological parameters are detailed in Table S1 in the Supplement. The PM2.5 samples were collected onto Whatman 3–5 Teflon membrane filters (Ø = 47 mm, Maidstone, UK) or Munktell MK360 quartz filters (Ø = 90 mm, Maidstone, UK) and weighed by the giant gravimetric balance method (Yang et al., 2015). Different filters were chosen depending on target analytes, e.g., the analysis of Si and water soluble inorganic ions must be performed with Teflon filters, while quartz filters should be used for OC and EC analysis.
2.3 Sample preparation procedures
For the analysis of Si concentration and isotopic composition, the samples were digested and purified as reported previously (Georg et al., 2006; Zambardi and Poitrasson, 2011). Briefly, the sample was first dried in a silver crucible in a muffle furnace at 1000 K for 10 min. Then, high-purity solid NaOH was added to the sample at a ratio of 1:20 and the mixture was heated at 1000 K for another 10 min, followed by cooling down to room temperature. The obtained fusion cake was dissolved with 2 mL of water followed by 24 h incubation, and then the solution was acidized by an HCl solution to pH ∼ 2.
To eliminate the interference from the matrix, the samples were purified by cation-exchange column chromatography. The cation resin (Dowex 50WX8, 200–400 mesh) in H+ form was packed in to a 1.8 mL resin bed in a BioRad column and then rinsed with HCl, HNO3 solution, and water until the eluate reached pH ∼ 7. Afterwards, 1 mL of the sample solution was loaded to the resin and eluted with 2 mL of water. Because Si in the solution is present mostly in the form of nonionic Si(OH)4 and anionic (Georg et al., 2006), cationic species could be removed from Si species by the cation-exchange resin. The recovery during the column purification procedures with the IRMM-017 was >95 %. The recovery of Si during the whole sample preparation procedures with the NIST-1648 was 89.9 %–96.2 %.
2.4 Measurement of Si concentration
The concentration of Si was measured on an Agilent 8800 inductively coupled plasma mass spectrometer (Santa Clara, CA, USA). The clean blank membrane filters have also been analyzed using the same procedures to subtract the background signals from the samples.
2.5 Estimation of secondary aerosols in PM2.5 by using the Si-dilution method
Providing the total Si in PM2.5 (Si) remains unchanged during the secondary growth of PM2.5, we can obtain
where , mpri, and ms represent the mass of PM2.5, primary particles, and secondary particles, respectively; Si represent the total Si mass in PM2.5; and Cpri represent Si abundance in PM2.5 and primary particles, respectively. Thus, the contribution of secondary aerosols to PM2.5 (fs) can be estimated by the Eq. (3):
The can be obtained by direct measurement of the collected PM2.5 samples, and the Cpri can be estimated from the mixed Si abundances of primary sources:
where Ci and fi represent the Si abundance and mass fraction of different primary sources (). In Eq. (4), the Ci can be obtained by measuring the collected primary source samples. In order to obtain fi, we need to know the emission amount (mi) of each source. The mi of anthropogenic sources in the Beijing region was adopted from the Multi-resolution Emission Inventory for China (MEIC) database (Li et al., 2017). For the mi of natural sources (i.e., dust), it can be calculated based on the isotopic mass balance of Si in PM2.5:
where δ30Si represents Si isotopic composition of PM2.5 and δ30Sii represents Si isotopic signatures of different primary sources. The mi values for different primary sources used in the calculation are given in Table 1.
Table 1Parameters for primary sources used in the estimation of secondary aerosol contribution to PM2.5.

a Data from the MEIC database (MEIC). b Since the MEIC database does not include data for biomass burning, the mi of biomass burning was estimated from the data of other sources according to source apportionment results of 2013 reported previously (Tian et al., 2016). c In the calculation, soil, construction, and urban fugitive dust are treated as a single source considering their similarity in Si abundance and Si isotopic signatures (Lu et al., 2018). The mi of dust was calculated by using isotopic mass balance of Si in PM2.5 (see Methodology). d The Si abundance and natural Si isotopic signatures of primary sources around Beijing are adopted from a previous study (Lu et al., 2018).
2.6 Uncertainty analysis in the estimation of secondary aerosols
The uncertainty of secondary aerosol contribution estimated by the Si-dilution method was obtained by the error propagation calculation. The general formula for the error propagation calculation is as follows (Ku, 1966; Larsen et al., 2012):
where Sf represents the standard deviation of the function f, xi represents the variables in the function f, and Si represents the standard deviation of xi. The Eq. (6) was applied to each step of the calculation of secondary aerosol contribution (fs) to obtain the uncertainty of the final result. Briefly, the uncertainty of fs is dependent on several variables in the calculation, including the measured Si abundance in PM2.5 (, Si abundance (Ci) and mass fraction of primary sources (fi), and Si isotopic composition of PM2.5 (δ30Si and primary sources (δ30Sii). The errors of , Ci, δ30Si, and δ30Sii can be directly obtained in the experimental measurements. The fi is given by the MEIC database (MEIC; Li et al., 2017). Note that the MEIC is a public emission inventory database that has been widely applied and well validated in air pollution research in China (Jiang et al., 2015; Zheng et al., 2015; Guan et al., 2014; Lin et al., 2014; Zhang et al., 2012a), but it does not include error information for the data. So, the error of fi was not included in the calculation. In this way, the uncertainty of the annual mean fs in 2013 was calculated to be 26.1 %. This value included both the method uncertainty and the variations on different dates. Nevertheless, it should be noted that the emission inventory actually affected the uncertainty of the estimate result. For example, if the uncertainty of the emission inventory was assumed to be 5 %, the uncertainty of the annual mean fs in 2013 would be increased to 29.3 %. On the other hand, the MEIC database used here can only provide yearly emission mass data, so using a high temporally resolved emission inventory may further increase the accuracy of the result.
3.1 Correlation of Si with the secondary species in PM2.5
To verify the role of Si as depicted in Fig. 1, we analyzed Si abundance and secondary aerosol tracers (e.g., , , , OC, and EC) in PM2.5 samples collected around Beijing, China, a typical particulate pollution region, on haze days (n=63) in 2013, in which year the particulate pollution in this region reached an unprecedentedly high level (Huang et al., 2014). We found that the PM2.5 concentration showed a clear seasonal trend (higher in spring–winter than in summer–autumn) within the range of 21.7–337.1 µg m−3 (Fig. S1 and Table S1 in the Supplement). The PM2.5 profile of the year 2013 was consistent with that reported previously (Lu et al., 2018), confirming the representativeness of the data. All secondary species in PM2.5 showed a similar seasonal trend with the PM2.5 concentration (Fig. S1). However, the total Si in PM2.5 (expressed as Si relative to air volume in µg m−3) did not show any seasonal trends (Fig. S2).
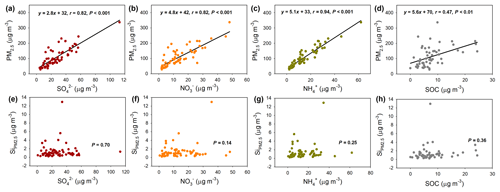
Figure 2Correlation analysis of PM2.5 concentration and its Si content (Si) with the secondary species in PM2.5. (a–d). Correlation of PM2.5 concentration with (a), (b), (c), and SOC (d). (e–h). Correlation of Si with (e), (f), (g), and SOC (h).
Furthermore, Fig. 2 shows the correlation of PM2.5 and its Si content with the secondary species in PM2.5. It can be seen that the PM2.5 concentration was highly linearly correlated with the secondary species (P<0.01). These chemical species are directly indicative of secondary chemistry: sulfate is mainly converted from atmospheric SO2 primarily emitted from coal combustion (Seinfeld and Pandis, 2016), nitrate originates from NOx emitted mainly from vehicle exhaust and power plants (Seinfeld and Pandis, 2016), and secondary organic carbon (SOC), as an indicator of SOA, derives from complex gaseous precursors (Hallquist et al., 2009). Specifically, PM2.5 showed a higher correlation with SIA (P<0.001) than with SOA (P<0.01), probably due to the higher contribution of SIA to SA than SOA (Fig. S3). However, as a stark contrast to the significant correlation in Fig. 2a–d, the Si showed no significant correlation with any of the secondary species (P>0.14); additionally, no obvious trend in Si was observed with the increase of secondary species. These results strongly evidenced that the Si was inactive during the secondary chemical process of aerosols.
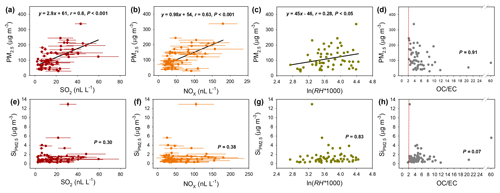
Figure 3Correlation analysis of PM2.5 concentration and Si with typical secondary aerosol precursors and relative humidity (RH). (a–d). Correlation of PM2.5 concentration with SO2 (a), NOx (b), RH (c), and the OC ∕ EC ratio (d). (d–f). Correlation of Si with SO2 (e), NOx (f), RH (g), and the OC ∕ EC ratio (h). The red dotted lines in (d) and (h) represent OC ∕ EC =2, which can be used to indicate the formation of secondary aerosols at OC ∕ EC >2 (Chow et al., 1994).
3.2 Correlation of Si with the secondary precursors and relative humidity
To further demonstrate the role of Si in secondary aerosols, we analyzed the correlation of Si with the secondary precursors (SO2 and NOx) and relative humidity (RH). RH is an important meteorological parameter that affects the secondary aerosol formation (Tang et al., 2016). As shown in Fig. 3a–c, PM2.5 was positively correlated with the atmospheric concentration of SO2 and NOx (P<0.001) and RH (P<0.05). It is worth noting that all PM2.5 samples yielded an OC ∕ EC ratio >2 (Fig. 3d), which indicated the generation of secondary aerosols (Chow et al., 1994), while Si was not significantly correlated with any of the secondary precursors or RH (P>0.3; Fig. 3e–g), again demonstrating that the Si was not affected by the secondary chemistry of aerosols. Furthermore, we also found that the Si was not correlated with the PM2.5 concentration (Fig. S4).
3.3 Effects of synthetic organosilicons and dry deposition
Based on the aforementioned results, we infer that Si should mainly be present in primary particles or act as nuclei during the formation of secondary particles. This was consistent with the nonvolatile nature of most Si-containing compounds in the natural environment. An exception is synthetic low-mass organic Si compounds (e.g., methylsiloxanes; Schweigkofler and Niessner, 1999; Ahrens et al., 2014; Xu et al., 2012), which may be able to transform into aerosol nanoparticles via hydroxyl-radical-mediated oxidative cleavage of Si-C bonds in the upper atmosphere (Atkinson, 1991; Sommerlade et al., 1993; Atkinson et al., 1995; Wu and Johnston, 2017). However, previous studies on the impact of low-mass organosilicons on the air quality have demonstrated that organosilicons did not contribute to the lower atmosphere aerosol formation (Graiver et al., 2003; Sommerlade et al., 1993). As a result, the US Environmental Protection Agency (EPA) has excluded organosilicons from the regulation concerning restriction of VOCs in the atmosphere. Recently, Janechek et al. (2017) found that the oxidative product concentration of cyclic volatile methylsiloxanes was ∼ 10–40 times lower than their parent compounds in the US (Janechek et al., 2017). Another study by Wu and Johnston reported that secondary aerosol yields of siloxane oxidation might reach 15 % (Wu and Johnston, 2017), suggesting that the contribution of organosilicons to secondary aerosols might vary in different regions. Thus, it may be necessary to consider the secondary Si production to determine whether it should be included in the secondary aerosol calculation. Note that the secondary Si production depends on the atmospheric concentration of both organosilicons and hydroxyl radicals. Up to now, no data about atmospheric organosilicons in the studied region were reported. Despite that, some recent studies reported the measurement and modeling results of atmospheric hydroxyl radicals in the North China Plain (Tan et al., 2017; Tham et al., 2016). The daily maximum concentration of atmospheric hydroxyl radicals was in the range of (5–15) × 106 cm−3 (∼ 10−10 mol m−3). Since hydroxyl radical is a crucial condition for the siloxane oxidation, the extremely low concentration of hydroxyl radicals in the atmosphere could greatly limit the secondary Si production from siloxanes in the studied region, suggesting that the secondary Si production could be negligible compared with the high-abundance Si in primary particles. This deduction has also been experimentally verified by the irrelevance of Si with the secondary chemistry of aerosols in Figs. 2 and 3.
We have also considered the influence of dry deposition on the Si abundance in PM2.5. The dry deposition is a size-dependent process that can cause coarse particles to fall more quickly than fine particles. However, it was found that the average Si abundance did not change significantly when the particle size was smaller than 2.5 µm (Tan et al., 2016). That is to say, for fine particles (<2.5 µm), the dry deposition rate would not significantly affect the Si abundance. Thus, the Si-dilution effect with PM2.5 should be predominantly controlled by the secondary aerosol formation.
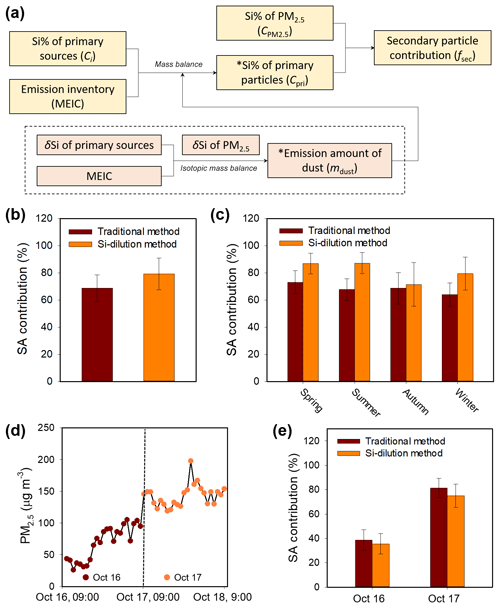
Figure 4Comparison of the SA estimate results between the Si-dilution method and the traditional method. (a) A flow chart showing how the fs was obtained by the Si-dilution method. The boxes with estimations from emission inventories are labeled with asterisk. (b) Annual mean SA contribution to PM2.5 in 2013 in Beijing. (c) Seasonal mean SA contribution in 2013 in Beijing. The error bars for the Si-dilution method include both method uncertainty and the variations of samples on different dates (see Sect. 2.6), while for the traditional method the error bars only represent the variations of samples on different dates (without method uncertainty). Please see text for a more detailed discussion on the uncertainties. (d–e) Analysis of SA contribution during a special haze episode. (d) Monitoring of PM2.5 concentration variations during 16 to 17 October 2013. (e) Daily SA contributions during the episode estimated by using the two different methods.
3.4 Secondary aerosol estimation using the Si-dilution method
The special role of Si in PM2.5 enables us to gain insights into the secondary particles in PM2.5 by using Si as a new conservative tracer. Here, as an example, we show the estimation of secondary aerosol contribution to PM2.5, which was thought of as a complicated and difficult task. Providing the Si remains unchanged during the secondary growth of PM2.5, the secondary formation can cause a dilution of the Si abundance in PM2.5. Thus, it is possible to estimate the secondary aerosol contribution to PM2.5 (fs) simply by the dilution factor (see Eq. 3 in Sect. 2.5). To this end, the theoretical Si abundance in primary particles (Cpri) and the final Si abundance in PM2.5 ( need to be known. The can be directly measured with the collected PM2.5 samples. In this study, an annual mean value of 1.56 % (n=63) was obtained. The Cpri can be derived from the mixed Si abundance of primary sources based on individual Si abundance and emission amounts of primary sources: the former can be measured with the collected primary source samples, and the latter can be given by a public emission inventory (e.g., the MEIC database). It should be noted that the emission inventory does not include the emission amount of natural primary sources (i.e., dust) (MEIC), which can be estimated by the isotopic mass balance of Si in PM2.5 based on the emission data of other sources and Si isotopic signatures of primary sources (see Table 1) (Lu et al., 2018). All estimation procedures are shown in Fig. 4a. In this way, the theoretical mean Si abundance in primary particles was calculated to be 7.51 %. That is, the theoretical Si abundance in primary particles (7.51 %) was diluted by the secondary aerosol formation to be 1.56 %. Based on these values, the mean contribution of secondary particles to PM2.5 on haze days in 2013 in the studied region was easily calculated to be 79.2 % (Fig. 4b). In the same way, we have also estimated the secondary aerosol contribution to PM2.5 in different seasons (Fig. 4c).
Note that the MEIC database only provides yearly emission data of primary sources. Thus, using a higher temporally resolved emission inventory in future studies may further increase the accuracy of the result. In addition, the interregional atmospheric transport of aerosols may also affect the estimate result. Here the PM2.5 was assumed to mainly derive from local emission of primary particles and SA precursors. In fact, a portion of it might also come from adjacent cities and regions via atmospheric transport, which could cause uncertainties in the result. Therefore, using primary source information and emission inventory covering adjacent cities and regions in the calculation may also improve the accuracy of the result.
On the other hand, VOCs including siloxanes are likely to become increasingly significant in the SA formation as aerosols originating from fossil fuels may become less important over time (McDonald et al., 2018). The method present here is based on the dilution effect of Si in primary particles during the SA formation, and therefore it should be suitable for the environment where secondary Si production is minimal (e.g., in heavily polluted urban regions with a large fossil fuel contribution like Beijing). Nevertheless, the method also remains flexible enough to be modified to be applicable in the cases in which the secondary Si production is not negligible. In that case, the secondary Si production mass needs to be estimated and included in the mass balance calculations (Sect. 2.5).
3.5 Uncertainty analysis and comparison with the traditional method
The uncertainty of the estimate result could be obtained by the error propagation calculation (Ku, 1966; Larsen et al., 2012) from the errors of variables used in the calculation (see Sect. 2.6 for details). In this way, the uncertainty of the annual mean SA contribution in 2013 was calculated to be 26.1 %. This value included the method uncertainty and the variations on different dates but did not include the effect of the emission inventory because the MEIC database does not include error information for the data (see Sect. 2.6). Thus, the effect of the uncertainty of emission inventory should be borne in mind when understanding the uncertainty of the result. As a comparison, the traditional method uses more variables (e.g., , , , EC, and OC) in the calculation, which may bring in large uncertainties to the result. For example, Schmid et al. (2001) reported that the RSD in the EC measurement could reach 36.6 %–45.5 %, which would cause large uncertainties in calculating the ratio of OC ∕ EC and consequently cause the SOC to be biased by up to 64 % (Guo et al., 2014). Meanwhile, the approach to estimating SOA by multiplying the SOC by an empirical coefficient is still controversial (Docherty et al., 2008). However, it is difficult to make a direct comparison of uncertainties between the Si-dilution method and traditional multitracer method due to the absence of error information from the emission inventory. Despite that, considering that the present method uses only a single tracer, it seems easier to control the uncertainties with the present method than with the traditional multitracer method.
We also compared the estimate results of the Si-dilution method with the traditional multitracer method (Table S2). As shown in Fig. 4b–c, generally, the SA contribution estimated by the Si-dilution method was slightly higher than that obtained by the traditional method. Such a difference can be explained by the different strategies of the methods: the traditional method is based on a limited number of species rather than all active secondary species in PM2.5, and thus the SA may be prone to underestimation, while the Si-dilution method only uses an inactive species (i.e., Si), and possible loss of Si-containing components during the sample pretreatment procedures may cause the result to be overestimated. Despite that, the results between the two methods were close. These two methods were further compared with a special haze episode during 16 to 17 October in 2013 in Beijing. During this episode, the PM2.5 concentration burst from 26.1 to 197.8 µg m−3 (Fig. 4d). The Si-dilution method showed that the SA contribution increased remarkably from 35.6 % for 16 October to 75.0 % for 17 October, which was highly consistent with that obtained with the traditional method (Fig. 4e). This could also verify the accuracy of the Si-dilution method.
3.6 Sources of secondary particle precursors revealed by Si isotopic signatures
In addition to the SA formation estimation, the correlation analysis of Si isotopic composition of PM2.5 with the SA can further reveal the sources of the precursors of SA. Note that the Si isotopic composition is independent of Si abundance of PM2.5, and it can actually reflect the primary sources of PM2.5, because different primary sources have different Si isotopic signatures (e.g., coal burning and industrial emission are 30Si-depleted sources, while vehicle emission and dust are 30Si-enriched sources) (Lu et al., 2018). From Fig. S5, the secondary aerosols were negatively correlated with the δ30Si of PM2.5, suggesting that 30Si-depleted primary sources (e.g., coal burning and industrial emission) contributed more precursors to secondary aerosols than 30Si-enriched sources. Specifically, from Fig. 5a, the δ30Si showed a declining trend with the increase in concentration (P=0.006), suggesting that the 30Si-depleted primary sources contributed more precursor (i.e., SO2) to the species than 30Si-enriched sources. This was consistent with the fact that SO2 mainly derives from coal burning, which is a 30Si-depleted source (Lu et al., 2018). The and SOC concentration was also negatively correlated with the δ30Si (Fig. 5c, d), suggesting that the precursors of these secondary species were also largely contributed by 30Si-depleted primary sources. However, the concentration did not show any correlation with the δ30Si (P=0.63; Fig. 5b). This could be explained by the fact that the sources of were more uncertain and involved both 30Si-enriched (e.g., vehicle exhaust) and 30Si-depleted primary sources (e.g., power plants) (Seinfeld and Pandis, 2016).
To further show the correlation of Si isotopic signatures with the SA precursors, we also analyzed two typical haze episodes in 2013 in Beijing (Fig. S6). In the episode during 30 November to 1 December 2013 (Fig. S6a–j), the PM2.5 rapidly increased from 26.1 to 197.8 µg m−3, during which the SIA, SOA, and their major constituents (i.e., , , , EC, and SOC) synchronously increased and the SA precursors (NOx and SO2) also showed a rapid rise. Meanwhile, the δ30Si of PM2.5 shifted negatively with the SA formation, suggesting that the SA precursors were largely contributed by 30Si-depleted sources. In another haze episode during 6 to 7 December 2013 (Fig. S6k–t), similar phenomena were observed, and the δ30Si of PM2.5 shifted negatively with the SA formation. Thus, all results mentioned above suggested that 30Si-depleted primary sources (e.g., coal burning and industrial emission) contributed more SA precursors than 30Si-enriched sources. This deduction was consistent with previous knowledge that coal burning and industrial emission contributed more precursors to SA (e.g., SO2, NOx, and VOCs) (Sun et al., 2016). Accordingly, we show that the Si isotopic composition not only indicates the primary sources, but can also reveal the sources of precursors of secondary species of PM2.5.
3.7 Implications for air pollution control policies
The SA contribution obtained here (79.2±26.1 % for all of 2013 and 88.7±8.9 % for January 2013; Fig. 4) was higher overall than that in a previous report (30 %–77 % for four cities in China in January 2013), probably due to the different sampling dates (Huang et al., 2014). It is worth noting that these results have been verified by two independent methods (Fig. 4). Thus, more strict policies should be enforced to reduce the emission of secondary particle precursors including SO2, NOx, and VOCs for haze pollution control, especially for NOx and VOCs, which have not aroused enough attention in current pollution control strategies (Wang et al., 2013). Furthermore, the 30Si-depleted primary sources (e.g., coal burning and industrial emissions) should be regulated because they contribute considerably to both primary particles and secondary particle precursors.
In summary, we investigated the role of Si during aerosol secondary formation and showed that the high abundance and chemical inactiveness of Si make it a new conservative tracer in investigating the aerosol formation process. Based on the Si-dilution effect, we have proposed a new method to estimate the secondary aerosol contribution to PM2.5 by using Si as a single tracer. In addition, the sources of the precursors of secondary aerosols can be revealed by the correlation analysis of secondary aerosols with the Si isotopic composition of PM2.5. Overall, this study not only enriches our understanding of the role of Si during the aerosol formation process, but also adds a new alternative tool into the toolbox of aerosol chemistry research. However, it should be noted that this method is expected to be useful only in regions where crustal particles represent a measurable amount of PM2.5, and in areas dominated by biogenic aerosols (e.g., forests) its usefulness is still questionable. Meanwhile, some measures may further improve the accuracy of the method, such as increasing the size and representativeness of primary source sample sets. Considering the interregional transport of PM2.5, future efforts should also be made to include primary sources and emission inventories of adjacent cities and regions in the calculation.
The data in this study can be obtained from the corresponding author upon request.
The supplement related to this article is available online at: https://doi.org/10.5194/acp-19-2861-2019-supplement.
QL designed the research. GJ supervised the project. DL performed most of experiments. JT measured the concentration of secondary species and calculated the secondary aerosol contribution using the traditional method. XY helped with the measurements. XS measured the atmospheric concentration of SO2 and NOx and RH. QL and DL analyzed the data and wrote the paper.
The authors declare that they have no conflict of interest.
This work was financially supported by the National Natural Science
Foundation of China (no. 21825403, 91843301, 91543104), the Chinese Academy
of Sciences (XDB14010400, QYZDB-SSW-DQC018), and the National Basic Research
Program of China (2015CB931903, 2015CB932003).
Edited by: Sergey A. Nizkorodov
Reviewed by: two anonymous referees
Ahrens, L., Harner, T., and Shoeib, M.: Temporal variations of cyclic and linear volatile methylsiloxanes in the atmosphere using passive samplers and high-volume air samplers, Environ. Sci. Technol., 48, 9374–9381, https://doi.org/10.1021/es502081j, 2014.
Atkinson, R.: Kinetics of the gas-phase reactions of a series of organosilicon compounds with OH and NO3 Radicals and O3 at 297±2 K, Environ. Sci. Technol., 25, 863–866, https://doi.org/10.1021/Es00017a005, 1991.
Atkinson, R., Tuazon, E. C., Kwok, E. S. C., Arey, J., Aschmann, S. M., and Bridier, I.: Kinetics and products of the gas-phase reactions of (CH3)4Si, (CH3)3SiCH2OH, (CH3)3SiOSi(CH3)3 and (CD3)3SiOSi(CD3)3 with Cl atoms and OH radicals, J. Chem. Soc. Faraday Trans., 91, 3033–3039, https://doi.org/10.1039/Ft9959103033, 1995.
Basile-Doelsch, I.: Si stable isotopes in the Earth's surface: A review, J. Geochem. Explor., 88, 252–256, https://doi.org/10.1016/j.gexplo.2005.08.050, 2006.
Bi, X. H., Feng, Y. C., Wu, J. H., Wang, Y. Q., and Zhu, T.: Source apportionment of PM10 in six cities of northern China, Atmos. Environ., 41, 903–912, https://doi.org/10.1016/j.atmosenv.2006.09.033, 2007.
Bzdek, B. R., Horan, A. J., Pennington, M. R., Janechek, N. J., Baek, J., Stanier, C. O., and Johnston, M. V.: Silicon is a frequent component of atmospheric nanoparticles, Environ. Sci. Technol., 48, 11137–11145, https://doi.org/10.1021/es5026933, 2014.
Chow, J. C., Watson, J. G., Fujita, E. M., Lu, Z. Q., Lawson, D. R., and Ashbaugh, L. L.: Temporal and spatial variations of PM2.5 and PM10 aerosol in the Southern California air quality study, Atmos. Environ., 28, 2061–2080, https://doi.org/10.1016/1352-2310(94)90474-X, 1994.
Docherty, K. S., Stone, E. A., Ulbrich, I. M., DeCarlo, P. F., Snyder, D. C., Schauer, J. J., Peltier, R. E., Weber, R. J., Murphy, S. M., Seinfeld, J. H., Grover, B. D., Eatough, D. J., and Jimenez, J. L.: Apportionment of Primary and Secondary Organic Aerosols in Southern California during the 2005 Study of Organic Aerosols in Riverside (SOAR-1), Environ. Sci. Technol., 42, 7655–7662, https://doi.org/10.1021/es8008166, 2008.
Georg, R. B., Reynolds, B. C., Frank, M., and Halliday, A. N.: New sample preparation techniques for the determination of Si isotopic compositions using MC-ICPMS, Chem. Geol., 235, 95–104, https://doi.org/10.1016/j.chemgeo.2006.06.006, 2006.
Graiver, D., Farminer, K. W., and Narayan, R.: A review of the fate and effects of silicones in the environment, J. Polym. Environ., 11, 129–136, https://doi.org/10.1023/A:1026056129717, 2003.
Guan, D. B., Klasen, S., Hubacek, K., Feng, K. S., Liu, Z., He, K. B., Geng, Y., and Zhang, Q.: Determinants of stagnating carbon intensity in China, Nat. Clim. Change, 4, 1017–1023, https://doi.org/10.1038/Nclimate2388, 2014.
Guo, S., Hu, M., Guo, Q. F., and Shang, D. J.: Comparison of Secondary Organic Aerosol Estimation Methods, Acta Chim. Sinica, 72, 658–666, https://doi.org/10.6023/A14040254, 2014.
Hallquist, M., Wenger, J. C., Baltensperger, U., Rudich, Y., Simpson, D., Claeys, M., Dommen, J., Donahue, N. M., George, C., Goldstein, A. H., Hamilton, J. F., Herrmann, H., Hoffmann, T., Iinuma, Y., Jang, M., Jenkin, M. E., Jimenez, J. L., Kiendler-Scharr, A., Maenhaut, W., McFiggans, G., Mentel, Th. F., Monod, A., Prévôt, A. S. H., Seinfeld, J. H., Surratt, J. D., Szmigielski, R., and Wildt, J.: The formation, properties and impact of secondary organic aerosol: current and emerging issues, Atmos. Chem. Phys., 9, 5155–5236, https://doi.org/10.5194/acp-9-5155-2009, 2009.
Huang, R. J., Zhang, Y., Bozzetti, C., Ho, K. F., Cao, J. J., Han, Y., Daellenbach, K. R., Slowik, J. G., Platt, S. M., Canonaco, F., Zotter, P., Wolf, R., Pieber, S. M., Bruns, E. A., Crippa, M., Ciarelli, G., Piazzalunga, A., Schwikowski, M., Abbaszade, G., Schnelle-Kreis, J., Zimmermann, R., An, Z., Szidat, S., Baltensperger, U., El Haddad, I., and Prevot, A. S.: High secondary aerosol contribution to particulate pollution during haze events in China, Nature, 514, 218–222, https://doi.org/10.1038/nature13774, 2014.
Janechek, N. J., Hansen, K. M., and Stanier, C. O.: Comprehensive atmospheric modeling of reactive cyclic siloxanes and their oxidation products, Atmos. Chem. Phys., 17, 8357–8370, https://doi.org/10.5194/acp-17-8357-2017, 2017.
Jiang, X. J., Zhang, Q., Zhao, H. Y., Geng, G. N., Peng, L. Q., Guan, D. B., Kan, H. D., Huo, H., Lin, J. T., Brauer, M., Martin, R. V., and He, K. B.: Revealing the Hidden Health Costs Embodied in Chinese Exports, Environ. Sci. Technol., 49, 4381–4388, https://doi.org/10.1021/es506121s, 2015.
Ku, H. H.: Notes on the use of propagation of error formulas, J. Res. Natl. Bureau Stand., 70, 263–273, 1966.
Larsen, B. R., Gilardoni, S., Stenstrom, K., Niedzialek, J., Jimenez, J., and Belis, C. A.: Sources for PM air pollution in the Po Plain, Italy: II. Probabilistic uncertainty characterization and sensitivity analysis of secondary and primary sources, Atmos. Environ., 50, 203–213, https://doi.org/10.1016/j.atmosenv.2011.12.038, 2012.
Li, M., Zhang, Q., Kurokawa, J.-I., Woo, J.-H., He, K., Lu, Z., Ohara, T., Song, Y., Streets, D. G., Carmichael, G. R., Cheng, Y., Hong, C., Huo, H., Jiang, X., Kang, S., Liu, F., Su, H., and Zheng, B.: MIX: a mosaic Asian anthropogenic emission inventory under the international collaboration framework of the MICS-Asia and HTAP, Atmos. Chem. Phys., 17, 935–963, https://doi.org/10.5194/acp-17-935-2017, 2017.
Lin, J. T., Pan, D., Davis, S. J., Zhang, Q., He, K. B., Wang, C., Streets, D. G., Wuebbles, D. J., and Guan, D. B.: China's international trade and air pollution in the United States, P. Natl. Acad. Sci. USA, 111, 1736–1741, https://doi.org/10.1073/pnas.1312860111, 2014.
Lu, D., Liu, Q., Yu, M., Yang, X., Fu, Q., Zhang, X., Mu, Y., and Jiang, G.: Natural Silicon Isotopic Signatures Reveal the Sources of Airborne Fine Particulate Matter, Environ. Sci. Technol., 52, 1088–1095, 2018.
McDonald, B. C., Gouw, J. A. d., Gilman, J. B., Jathar, S. H., Akherati, A., Cappa, C. D., Jimenez, J. L., Lee-Taylor, J., Hayes, P. L., McKeen, S. A., Cui, Y. Y., Kim, S.-W., Gentner, D. R., Isaacman-VanWertz, G., Goldstein, A. H., Harley, R. A., Frost, G. J., Roberts, J. M., Ryerson, T. B., and Trainer, M.: Volatile chemical products emerging as largest petrochemical source of urban organic emissions, Science, 359, 760–764, https://doi.org/10.1126/science.aaq0524, 2018.
Multi-resolution Emission Inventory for China, available at: http://meicmodel.org/, last access: 31 December, 2018.
Pope, C. A., Burnett, R. T., Thun, M. J., Calle, E. E., Krewski, D., Ito, K., and Thurston, G. D.: Lung cancer, cardiopulmonary mortality, and long-term exposure to fine particulate air pollution, J. Am. Med. Assoc., 287, 1132–1141, 2002.
Pope, C. A., Ezzati, M., and Dockery, D. W.: Fine-particulate air pollution and life expectancy in the United States, N. Engl. J. Med., 360, 376–386, https://doi.org/10.1056/NEJMsa0805646, 2009.
Savage, P. S., Armytage, R. M. G., Georg, R. B., and Halliday, A. N.: High temperature silicon isotope geochemistry, Lithos, 190, 500–519, https://doi.org/10.1016/j.lithos.2014.01.003, 2014.
Schmid, H., Laskus, L., Abraham, H. J., Baltensperger, U., Lavanchy, V., Bizjak, M., Burba, P., Cachier, H., Crow, D., Chow, J., Gnauk, T., Even, A., ten Brink, H. M., Giesen, K. P., Hitzenberger, R., Hueglin, C., Maenhaut, W., Pio, C., Carvalho, A., Putaud, J. P., Toom-Sauntry, D., and Puxbaum, H.: Results of the “carbon conference” international aerosol carbon round robin test stage I, Atmos. Environ., 35, 2111–2121, https://doi.org/10.1016/S1352-2310(00)00493-3, 2001.
Schweigkofler, M. and Niessner, R.: Determination of siloxanes and VOC in landfill gas and sewage gas by canister sampling and GC-MS/AES analysis, Environ. Sci. Technol., 33, 3680–3685, https://doi.org/10.1021/Es9902569, 1999.
Seinfeld, J. H. and Pandis, S. N.: Atmospheric Chemistry and Physics: From Air Pollution to Climate Change, 3 Edn., ISBN 978-1-118-94740-1, Wiley, 2016.
Sommerlade, R., Parlar, H., Wrobel, D., and Kochs, P.: Product analysis and kinetics of the gas-phase reactions of selected organosilicon compounds with OH radicals using a smog chamber-mass spectrometer system, Environ. Sci. Technol., 27, 2435–2440, https://doi.org/10.1021/Es00048a019, 1993.
Sun, Y., Du, W., Fu, P., Wang, Q., Li, J., Ge, X., Zhang, Q., Zhu, C., Ren, L., Xu, W., Zhao, J., Han, T., Worsnop, D. R., and Wang, Z.: Primary and secondary aerosols in Beijing in winter: sources, variations and processes, Atmos. Chem. Phys., 16, 8309–8329, https://doi.org/10.5194/acp-16-8309-2016, 2016.
Tan, J., Duan, J., Zhen, N., He, K., and Hao, J.: Chemical characteristics and source of size-fractionated atmospheric particle in haze episode in Beijing, Atmos. Res., 167, 24–33, 2016.
Tan, Z., Fuchs, H., Lu, K., Hofzumahaus, A., Bohn, B., Broch, S., Dong, H., Gomm, S., Häseler, R., He, L., Holland, F., Li, X., Liu, Y., Lu, S., Rohrer, F., Shao, M., Wang, B., Wang, M., Wu, Y., Zeng, L., Zhang, Y., Wahner, A., and Zhang, Y.: Radical chemistry at a rural site (Wangdu) in the North China Plain: observation and model calculations of OH, HO2 and RO2 radicals, Atmos. Chem. Phys., 17, 663–690, https://doi.org/10.5194/acp-17-663-2017, 2017.
Tang, M., Cziczo, D. J., and Grassian, V. H.: Interactions of water with mineral dust aerosol: Water adsorption, hygroscopicity, cloud condensation, and ice nucleation, Chem. Rev., 116, 4205–4259, https://doi.org/10.1021/acs.chemrev.5b00529, 2016.
Tham, Y. J., Wang, Z., Li, Q., Yun, H., Wang, W., Wang, X., Xue, L., Lu, K., Ma, N., Bohn, B., Li, X., Kecorius, S., Größ, J., Shao, M., Wiedensohler, A., Zhang, Y., and Wang, T.: Significant concentrations of nitryl chloride sustained in the morning: investigations of the causes and impacts on ozone production in a polluted region of northern China, Atmos. Chem. Phys., 16, 14959–14977, https://doi.org/10.5194/acp-16-14959-2016, 2016.
Tian, S. L., Pan, Y. P., and Wang, Y. S.: Size-resolved source apportionment of particulate matter in urban Beijing during haze and non-haze episodes, Atmos. Chem. Phys., 16, 1–19, https://doi.org/10.5194/acp-16-1-2016, 2016.
Wang, Y., Zhang, Q. Q., He, K., Zhang, Q., and Chai, L.: Sulfate-nitrate-ammonium aerosols over China: response to 2000–2015 emission changes of sulfur dioxide, nitrogen oxides, and ammonia, Atmos. Chem. Phys., 13, 2635–2652, https://doi.org/10.5194/acp-13-2635-2013, 2013.
Watson, J. G., Chow, J. C., and Chen, L.-W. A.: Summary of organic and elemental carbon/black carbon analysis methods and intercomparisons, Aerosol Air Qual. Res., 5, 65–102, 2005.
Wu, Y. and Johnston, M. V.: Aerosol formation from OH oxidation of the volatile cyclic methyl siloxane (cVMS) decamethylcyclopentasiloxane, Environ. Sci. Technol., 51, 4445–4451, 2017.
Xu, L., Shi, Y. L., Wang, T., Dong, Z. R., Su, W. P., and Cai, Y. Q.: Methyl siloxanes in environmental matrices around a siloxane production facility, and their distribution and elimination in plasma of exposed population, Environ. Sci. Technol., 46, 11718–11726, https://doi.org/10.1021/es3023368, 2012.
Yang, J., Fu, Q., Guo, X., Chu, B., Yao, Y., Teng, Y., and Wang, Y.: Concentrations and seasonal variation of ambient PM2.5 and associated metals at a typical residential area in Beijing, China, B. Environ. Contam. Tox., 94, 232–239, 2015.
Zambardi, T. and Poitrasson, F.: Precise determination of silicon isotopes in silicate rock reference materials by MC-ICP-MS, Geostand. Geoanal. Res., 35, 89–99, https://doi.org/10.1111/j.1751-908X.2010.00067.x, 2011.
Zhang, Q., He, K. B., and Huo, H.: Cleaning China's air, Nature, 484, 161–162, 2012a.
Zhang, R., Khalizov, A., Wang, L., Hu, M., and Xu, W.: Nucleation and growth of nanoparticles in the atmosphere, Chem. Rev., 112, 1957–2011, https://doi.org/10.1021/cr2001756, 2012b.
Zhang, R., Wang, G., Guo, S., Zamora, M. L., Ying, Q., Lin, Y., Wang, W., Hu, M., and Wang, Y.: Formation of urban fine particulate matter, Chem. Rev., 115, 3803–3855, https://doi.org/10.1021/acs.chemrev.5b00067, 2015.
Zheng, B., Zhang, Q., Zhang, Y., He, K. B., Wang, K., Zheng, G. J., Duan, F. K., Ma, Y. L., and Kimoto, T.: Heterogeneous chemistry: a mechanism missing in current models to explain secondary inorganic aerosol formation during the January 2013 haze episode in North China, Atmos. Chem. Phys., 15, 2031–2049, https://doi.org/10.5194/acp-15-2031-2015, 2015.