the Creative Commons Attribution 4.0 License.
the Creative Commons Attribution 4.0 License.
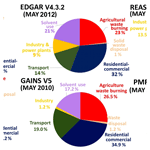
Source apportionment of volatile organic compounds in the northwest Indo-Gangetic Plain using a positive matrix factorization model
Vinayak Sinha
In this study we undertook quantitative source apportionment for 32 volatile organic compounds (VOCs) measured at a suburban site in the densely populated northwest Indo-Gangetic Plain using the US EPA PMF 5.0 model. Six sources were resolved by the PMF model. In descending order of their contribution to the total VOC burden these are “biofuel use and waste disposal” (23.2 %), “wheat-residue burning”(22.4 %), “cars” (16.2 %), “mixed daytime sources”(15.7 %) “industrial emissions and solvent use”(11.8 %), and “two-wheelers” (8.6 %).
Wheat-residue burning is the largest contributor to the total ozone formation potential (32.4 %). For the emerging contaminant isocyanic acid, photochemical formation from precursors (37 %) and wheat-residue burning (25 %) were the largest contributors to human exposure. Wheat-residue burning was also the single largest source of the photochemical precursors of isocyanic acid, namely, formamide, acetamide and propanamide, indicating that this source must be most urgently targeted to reduce human concentration exposure to isocyanic acid in the month of May. Our results highlight that for accurate air quality forecasting and modeling it is essential that emissions are attributed only to the months in which the activity actually occurs. This is important for emissions from crop residue burning, which occur in May and from mid-October to the end of November.
The SOA formation potential is dominated by cars (36.9 %) and two-wheelers (21.1 %), which also jointly account for 47% of the human class I carcinogen benzene in the PMF model. This stands in stark contrast to various emission inventories which estimate only a minor contribution of the transport sector to the benzene exposure (∼10 %) and consider residential biofuel use, agricultural residue burning and industry to be more important benzene sources. Overall it appears that none of the emission inventories represent the regional emissions in an ideal manner. Our PMF solution suggests that transport sector emissions may be underestimated by GAINSv5.0 and EDGARv4.3.2 and overestimated by REASv2.1, while the combined effect of residential biofuel use and waste disposal emissions as well as the VOC burden associated with solvent use and industrial sources may be overestimated by all emission inventories. The agricultural waste burning emissions of some of the detected compound groups (ketones, aldehydes and acids) appear to be missing in the EDGARv4.3.2 inventory.
- Article
(6329 KB) - Full-text XML
-
Supplement
(2955 KB) - BibTeX
- EndNote
Volatile organic compounds (VOCs) have diverse natural (760 Tg(C) yr−1 Sindelarova et al., 2014) and anthropogenic sources (127 Tg yr−1 average value; IPCC, 2013). Certain VOCs emitted primarily by anthropogenic sources, such as benzene and isocyanic acid, have direct adverse impacts on human health even at low ppb concentration exposures (Chandra and Sinha, 2016). In densely populated regions like the Indo-Gangetic Plain (IGP), reactive anthropogenic VOCs contribute significantly to the formation of health-relevant secondary pollutants such as ozone and secondary organic aerosol (Chandra and Sinha, 2016; Sarkar et al., 2016). At our study site, a representative suburban site in the NW IGP, the 8 h average NAAQS (national ambient air quality standard) for ozone of 100 µg m−3 was exceeded on 29 out of 31 d during May 2012 (Sinha et al., 2014), while the 24 h average NAAQS for PM2.5 of 60 µg m−3 was exceeded during 27 out of 31 d in the same period. It has been shown that wheat-residue burning results in significant enhancement (by 19 ppb) of the daytime ozone mixing ratios in pre-monsoon season (Kumar et al., 2016) and long-range transport in the form of dust storms from the Arabian Peninsula brings extremely high PM2.5 mass loadings (with peak PM2.5 mass loadings of 950 µg m−3 on 17 May 2012) (Sinha et al., 2014; Pawar et al., 2015) and enhances the PM2.5 mass by ∼30 %.
However, ozone mixing ratios exceed the NAAQS even during the non-fire-influenced days of the pre-monsoon season, and the NAAQS for PM2.5 is exceeded 60 % of the time for air masses with no history of long-range transport (Kumar et al., 2016; Pawar et al., 2015). This indicates that local ozone and PM2.5 precursor emissions deserve further study.
Previous source receptor modeling studies of VOC emission from India (Srivastava, 2004; Srivastava et al., 2005; Majumdar et al., 2009) produced results that conflicted strongly with the bottom-up emission inventories, all of which contain significant emissions from residential fuel usage even when filtered for the New Delhi National Capital Region (41 %–45 %), Greater Mumbai (32 %–36 %) and Greater Kolkata (33 %–59 %). Transport sector emissions, according to the bottom-up emission inventories contribute only 15 %–35 %, 17 %–43 % and 6 %–14 % to the total VOC emissions in New Delhi National Capital Region, Greater Mumbai and Greater Kolkata, respectively. All previous studies employed a chemical mass balance (CMB) technique for ambient VOC source attribution and identified the transport sector as the main source in the form of evaporative emissions (40 %–87 %) in Mumbai (Srivastava, 2004), diesel internal combustion engines (26 %–58 %) in Delhi (Srivastava et al., 2005) and roadway/refuelling exhaust (40 %) in Kolkata city (Majumdar et al., 2009). Except for the study performed in Kolkata which found a contribution of <10 % from wood combustion, residential fuel usage was not identified as a potential VOC source in those source receptor modeling studies. The observed discrepancy could be partially caused by the fact that a CMB is not necessarily an ideal tool for conducting source receptor modeling studies in understudied environments, as the model needs to be initialized with locally measured source profiles of all potentially significant sources. However, it is unlikely that this is the only reason for the discrepancies between source receptor modeling outcomes and emission inventories. The only other source receptor modeling study in South Asia was conducted using a positive matrix factorization model (EPA PMF5.0) with data collected in the Kathmandu valley, Nepal, as part of the SUSKAT campaign and attributed a negligible fraction of the anthropogenic VOC burden to residential biofuel usage (14 %). Instead different industrial sources including brick kilns (jointly 52 %) and the transport sector (21 %) were identified as the dominant VOC sources in the Kathmandu valley.
Different bottom-up emission inventories have large discrepancies between each other when extracted for the NW IGP. For our study region (27.4–34.9 ∘N, 72–79.8 ∘E), EDGARv4.3.2 (Huang et al., 2017) estimates that the road transport sector contributes only 18 % of the total anthropogenic VOC emissions (440 Gg yr−1), while REAS v2.1 (Kurokawa et al., 2013) attributes 35.8 % of the total anthropogenic VOC emissions (1227 Gg yr−1) to this sector. For industrial emissions and solvent use, GAINS (Amann et al., 2011) has the lowest (540 Gg yr−1) and EDGARv4.3.2 the highest absolute emissions (900 Gg yr−1). Crop residue burning as a VOC source is missing in REAS but accounted for a 6 % (145 Gg yr−1) and 7 % (163 Gg yr−1) share of the annual VOC emissions in EDGARv4.3.2 and GAINS, respectively. Considering the large discrepancies between bottom-up inventories and different source receptor modeling studies, more source receptor modeling studies using robust statistical tools and better tracers for different biomass burning sources are necessary.
In the present study, we applied the US EPA's PMF 5.0 model in constrained mode for source apportionment of 32 VOCs measured at IISER Mohali Atmospheric Chemistry Facility in May 2012 with the objective of quantifying the most important sources of ozone and SOA precursors, the human class I carcinogen benzene, and the emerging contaminant isocyanic acid (Chandra and Sinha, 2016), so that strategies for air pollution mitigation can benefit from quantitative evidence concerning the contribution of major sources. The month of May is of special interest, as it is affected by widespread wheat-residue burning in the NW IGP. In the present study, we quantify the contribution of this important area source to the VOC burden at a downwind site. Our analysis includes several rarely reported nitrogen-containing compounds which appear to have strong pyrogenic sources in this particular study region. Compounds such as amines, amides and isocyanic acid are presently not included in global emission inventories and the default atmospheric chemistry mechanisms, despite their potential importance for secondary aerosol formation and human health. We compare our source receptor modeling output with several emission inventories such as REAS v2.1, EDGARv4.3.2 and GAINS v5 to assess which emission inventory is most consistent with the results of our source receptor modeling study that employs in situ observations.
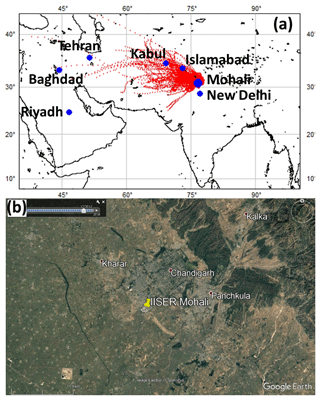
Figure 1(a) Mohali, located on the Indian Subcontinent, with the overlaid 72 h air mass back trajectories for May 2012 at 09:00 and 23:00 LT (UTC+5:30) (b) Precise location of IISER-Mohali Atmospheric chemistry facility (30.667 ∘N, 76.729 ∘E, 310 m above mean sea level, a.m.s.l.) with nearby cities on Google Earth© imagery. The campus of IISER Mohali is outlined in white.
2.1 Receptor site
The measurement facility is situated inside the Indian Institute of Science Education and Research Mohali (IISER Mohali) campus (Fig. 1a), which is a suburban site (30.667 ∘N, 76.729 ∘E, 310 m a.m.s.l) in Mohali near Chandigarh in India (Fig. 1b). Collectively the metropolitan area of Chandigarh–Mohali–Panchkula forms a tri-city with a total population of 1 941 118 (Census, 2011). The main air transport toward the site was from the northwest and the period studied was impacted by wheat-residue burning, a dust storm and strong photochemistry (Sinha et al., 2014). Figure 1a shows 72 h HYSPLIT back trajectories arriving at the site. With average wind speeds of 5.6 m s−1 during the study period (range 1–20 m s−1) the meteorological conditions were conducive for capturing the contribution of regional emission sources. The measurement site, the meteorology and the primary dataset acquired during May 2012 have been discussed in detail elsewhere (Sinha et al., 2014).
2.2 VOCs and other auxiliary measurements
We used hourly data of 32 measured organic ions which were assigned to volatile organic compounds (Supplement Table S1) based on PTR-TOF-MS studies conducted by our group within the South Asian environment (Sarkar et al., 2016) to initialize the US EPA PMF 5.0 model and employed CO, SO2, O3 and NOy as independent tracers to validate the results. As described in greater detail in Sinha et al. (2014), ambient air sampling was performed continuously through a Teflon inlet line protected by an in-line Teflon filter. A high-sensitivity proton transfer reaction quadrupole mass spectrometer (PTR-QMS; HS Model 11-07HS-088, Ionicon Analytik Gesellschaft, Austria) was operated at drift tube pressure of 2.2 mbar, a drift tube temperature of 60 ∘C and a drift tube voltage of 600 V, which resulted in an operating E∕N ratio of ∼135 Td. Carbon monoxide (CO), sulfur dioxide (SO2), ozone (O3) and NOy (NO, NO2 and other nitrogen species converted to NO by a molybdenum converter such as nitric acid and PAN) were measured using Thermo Fischer Scientific 48i (IR filter correlation based spectroscopy), 43i (pulsed UV fluorescence), 49i (UV absorption photometry) and 42i trace level air quality analyzers (chemiluminescence), respectively.
2.3 Positive matrix factorization model
In the current study, the US EPA PMF 5.0 receptor model (Norris et al., 2014) was applied to the ambient VOC dataset (in µg m−3) from May 2012 measured at the IISER-Mohali Atmospheric chemistry facility, comprising a data matrix of 721 samples (rows) and 32 species (columns). The EPA PMF 5.0 receptor model (Paatero et al., 2014; Norris et al., 2014) is a multivariate factor analysis tool (Paatero and Tapper, 1994; Paatero, 1997), which decomposes the data matrix xij with i number of samples and j number of measured VOCs into two matrices, the factor contribution matrix gik (which provides the mass g contributed by each factor to the individual sample) and the factor profiles matrix fkj (which provides the source profile/fingerprint of each individual source). Both matrices are established for a user-defined number of sources p from the existing intrinsic variability in the dataset, leaving behind a matrix of residuals eij.
A detailed description of the model can be found elsewhere (Paatero and Tapper, 1994; Paatero, 1997; Paatero et al., 2014; Norris et al., 2014). The two primary advantages of the PMF over other source receptor modeling tools are its inherent non-negative constraints (Hopke, 2016) and its capability to optimally weigh individual data points and assign uncertainties, which makes it possible to include less robust species that can be useful for defining real sources. The EPAv5.0 model is superior when compared to other source receptor modeling tools as it contains advanced rotational features (Paatero and Hopke, 2009) which allow the rotational ambiguity to be constrained in a manner that pushes the PMF solution towards the real world space.
All 32 species were assigned a fixed 20 % in the uncertainty, which represents the largest uncertainty estimated for strong compounds, to avoid a situation where the difference in the assigned uncertainty drives the PMF to dedicate a separate factor towards minimizing the Q of a single compound with low uncertainty (toluene) by taking it out of all other source profiles and opening a separate factor profile containing just a single compound. A total of 18 were identified as weak based on the signal-to-noise ratio and the presence of potential isobaric interferences as detailed in Table S2. For weak species, the PMF model triples the stated uncertainty to reduce their impact on the model's solution. Designating sources with isobaric interferences as weak is warranted, because when two sources with different temporal profiles (nighttime combustion and daytime biogenic emission or nighttime combustion and daytime photochemistry) could potentially contribute different compounds to the same m∕z ratio, zero values are almost absent in that particular column of the matrix and the tracer is affected by additional uncertainty not appropriately expressed by merely looking at the instrumental measurement error and the signal-to-noise ratio. When this column is made “weak” and given a higher uncertainty, other “strong” tracers, representing a single compound, define source profiles and this reduces the rotational ambiguity of the model. The “weak” compounds with isobaric interferences tend to be distributed among the source profiles available as per the solution that minimizes Q but they do not define any of the profiles. The extra modeling uncertainty was kept to zero and missing values (<5 %) were excluded. For every base model run, we used 20 runs with random seeds. Stable Q values were obtained for all runs. The model was run with 3 to 7 factors, to identify the appropriate number of factors as discussed in the Supplement in greater detail. Figure 2 shows the percentage contribution of the identified sources to the VOC burden for these runs. Figure S4 a, b and c show how the factor profile, percentage of each VOC originating from a certain source, and the factor contribution change while increasing the number of factors in the model. Figure 2 shows that a seven-factor solution provides little advantage over a six-factor solution, while a five-factor solution does not resolve the wheat-residue burning source, which is independently verified by MODIS (Moderate Resolution Imaging Spectroradiometer) fire counts over the region. The residuals for all species for the six-factor solution depicted a normal curve and fall within −3.3σ and +3.3σ for all species, indicating a good model fit. The constraints feature of the 5.0 version of the model was utilized to improve the performance of the model further, as described in greater detail in the Supplement. The constrained model operation of the PMF version 5.0 allows the rotational ambiguity of the model to be reduced using external knowledge. For example, if a source is inactive for a particular period (as is photochemistry at night), then the source contribution (gik) due to that factor during that time period can be pulled to zero in the model to provide more robust output. Similarly, a compound that is known to be present only in primary emissions can be pulled down in the source composition (fkj) matrix of the photochemistry factor. A list of the constraints applied is provided in Table S3. A detailed discussion of the use of constraints in a receptor model has been provided in previous studies (Paatero et al., 2002, 2014; Paatero and Hopke, 2009; Norris et al., 2014; Sarkar et al., 2016). Bootstrap model runs (Brown et al., 2015) were performed to assess the model uncertainty. For bootstrapping, model inputs were initialized to random seed, 100 number of bootstrap runs and default values for both block size (10) and minimum correlation R value (0.6). Bootstrap analysis resulted in no unmapped factors. Except for the car and two-wheeler factor (R=0.6) for which a certain degree of co-linearity is expected, none of the other factors showed cross-correlation with each other (R<0.3) and the g-space plot even of this factor pair is well filled. The constraint mode was unable to force the PMF model to separate the wheat-residue burning factor in a five-factor solution without imposing a split between the car and two-wheeler factor, indicating that these two indeed represent distinct source profiles.
2.4 Validation of the PMF output
The PMF generates two matrices from the intrinsic variability in the dataset: a factor contribution matrix and a factor profile matrix.
Traditionally the PMF output has been validated by cross-correlating the factor contribution matrix with independent tracers which were not used to initialize the model but are considered useful tracers for the respective source (Brown et al., 2015; Leuchner and Rappenglück, 2010; Gaimoz et al., 2011; Bon et al., 2011; Sarkar et al., 2016). We perform this validation step for all six source factors resolved by the PMF model. These were identified as “biofuel use and waste disposal”, “wheat-residue burning”, “four-wheelers”, “two-wheelers”, “industrial emissions and solvent use” and “mixed daytime sources”. The factor contribution for four-wheelers (R=0.7) and two-wheelers (R=0.6) correlated best with the independent tracer NOy, which is considered to be a vehicular exhaust marker (Ramanathan et al., 1985). The factor contribution of the domestic fuel usage and waste disposal factor correlated best with the independent tracer CO (R=0.9), a proxy for inefficient combustion, while the factor contribution of the industrial emission factor correlated best with the independent tracer SO2 (R=0.6). The wheat-residue burning factor days showed a moderate cross-correlation with MODIS fire counts with an R=0.4 and a lag of 2 d. O3 (R=0.8) was the best independent tracer for the mixed daytime factor.
However, our study goes one step further than all previous studies in validating the PMF output. For five out of six factors we validated the factor profiles generated by the PMF model against grab samples collected at the source. Factor profiles were cross-correlated with the fingerprints of source samples collected from a number of potential sources including wheat-residue fires (Chandra et al., 2017; Kumar et al., 2018), ambient air samples from a busy traffic junction (Chandra et al., 2017) and an industrial area (this study), tail-pipes of various vehicles (this study), waste burning (Sharma et al., 2019), leaf litter burning (this study), domestic biofuel use (Stockwell et al., 2016), and brick kilns (Zhong et al., 2019) to identify the sources. Figure 3 shows the factor profiles obtained from the PMF run (in dark blue), the percentage of each species explained by the respective PMF factor (red squares) and the source profiles of those sources which best matched the factor profile (in various colors as indicated in the legend). The factor profile of residential fuel usage and waste disposal correlates most strongly with the measured VOC source speciation profiles of domestic cooking (R=0.8), leaf-litter burning (R=0.7) and smoldering garbage fires (R=0.6), the wheat-residue burning factor with flaming wheat-residue burning (R=0.9), the four-wheeler factor with the tailpipe exhaust of petrol-fueled cars (R=0.5), gasoline evaporation headspace for diesel (R=0.5) and urban traffic junction grab samples (R=0.8), and the two-wheeler factor with the tailpipe exhaust of petrol-fuelled four-stroke two-wheelers (R=0.6). The industrial emissions correlated best with the source profile of brick kilns (R=0.5) and ambient air samples collected in an industrial area (0.6). For mixed daytime sources no source profile sampling is possible.
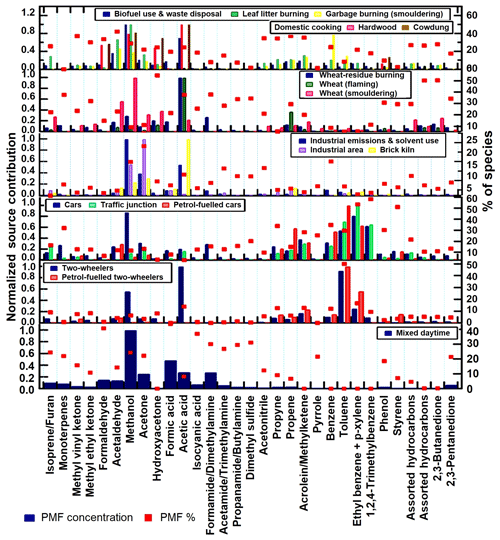
Figure 3Factor profile composition for 6 PMF resolved factors at IISER-Mohali. It displays the normalized source fingerprints of the PMF factors (dark blue) and samples collected at the source (in various colors) in bar-chart form. The value of the normalized species contribution is depicted on the left-hand axis. The percentage of each species explained by each of the PMF factors is displayed in the form of a red square to be read from the right-hand axis.
2.5 Conditional probability function analysis
We perform a conditional probability function (CPF) analysis (Leuchner and Rappenglück, 2010) which aids in identifying physical locations of different PMF source factors without using back trajectories (Xie and Berkowitz, 2006) The CPF is computed using the factor contribution of the PMF model in combination with the wind direction at the receptor site. It quantifies the probability of factor contributions surpassing a certain threshold (75th percentile) for a particular wind direction sector, thereby highlighting the directional dependency of source factors, and is defined as follows:
where mΔθ represents the number of data points in the wind direction bin Δθ which exceeded the threshold criterion and nΔθ represents the total number of data points from the same wind direction bin. Δθ was assigned a value of 30∘.
2.6 Calculation of the ozone formation potential and SOA formation potential
Ozone production potential (O3PP) for each of the PMF-derived source factors was calculated based on the method used by Sinha and co-workers (Sinha et al., 2012) using the following equation:
where n stands for the number of ozone molecules produced in the oxidation of VOCi using n=2 and [OH] =106 molecules cm−3. The values were summed up for all the VOCs for obtaining the ozone production potential corresponding to each of the PMF-derived factors for the daytime hours (07:00–18:00 LT).
Secondary organic aerosol (SOA) potential was calculated for the PMF source factors using the literature SOA yields (Derwent et al., 2010) under low-NOx conditions for benzene, toluene, ethylbenzene, trimethylbenzene, styrene, methanol, isoprene, formaldehyde, acetaldehyde, acetone, formic acid and acetic acid using the equation given below for 07:00–18:00 LT.
2.7 Methodology for the comparison of PMF source factors with existing emission inventories
The Global Emission Database for Global Atmospheric Research (EDGARv4.3.2) inventory for the year 2012 (Huang et al., 2017) and two regional emission inventories, the Regional Emission inventory in Asia (REAS v2.1) for the year 2008 (Kurokawa et al., 2013) and the Greenhouse Gas and Air Pollution Interactions and Synergies model (GAINS) (Amann et al., 2011) for the year 2010 (Stohl et al., 2015), were compared with our PMF output. The gridded inventory was filtered for latitude 27.4–34.9 ∘N and longitude 72–79.8 ∘E, i.e., the fetch region from which the air mass trajectories reach the receptor site within 1 d. This filtering is required because compounds with photochemical lifetimes of less than a day (e.g., styrene, C-8 and C-9 aromatics) feature prominently in several source profiles, indicating that most of the transport sector emission, were less than a day old when they reached the receptor site. Other compounds with longer lifetimes such as toluene (2 d), benzene (6 d) or acetonitrile (months) can reach the site from more distant sources. The wheat-residue burning source shows the highest cross-correlation with the regional fire counts for a lag time of 2 d, indicating that emissions from distant sources can and do impact the site with a time lag. The chosen fetch region includes the areas where the maximum number of wheat-residue burning fire counts are observed while avoiding a size that is too large to be consistent with the relatively unaltered signature of some of the other PMF source profiles.
Annual emissions were available for EDGAR (2012) and GAINS (2010), whereas REAS provided monthly data (May 2008). However, Fig. S6 shows that despite providing monthly data, the REAS emission inventory has very little seasonality for any of the sources.
To facilitate the comparison of the PMF output of the month of May, which is affected by a strongly seasonal source (crop residue burning), with emission inventories that provide only annual data as of now, we calculate hypothetical pie charts which attribute annual crop residue burning emissions over the region only to the 2.5 months when crop residue burning actually occurs (middle of October to end of November and May).
3.1 Split up of VOC emission sources in Mohali and their contribution to ozone and SOA formation potential
Figure 4a shows the percent contribution of the different sectors to ambient VOC mass concentration loadings during May 2012 in Mohali, while Fig. S7 shows a time series of the total VOC mass contributed by the individual factors to the overall mass. The two traffic factors combined together were found to be the strongest contributors to the total VOC mass concentration (25.1 %) followed by biofuel use and waste disposal factor (23.2 %), wheat-residue burning (22.4 %), the mixed daytime factor (15.7 %) and industrial emissions (11.8 %), with the residual unapportioned VOC mass only amounting to 1.7 % of the total. Early source receptor modeling studies from India attributed a slightly larger share (26 %–58 %) of the total VOC mass to traffic-related emissions (Srivastava, 2004; Srivastava et al., 2005), suggesting that the progression to the emission norms Bharat stage III and IV (which are equivalent to Euro 3 and Euro 4 norms, http://cpcb.nic.in/vehicular-exhaust/, last access: 28 April 2019) may have brought down VOC emissions from the traffic sector.
Figure 4b shows the contribution of the different sectors to the ozone formation potential during May 2012 in Mohali. The wheat-residue burning factor was found to be the largest contributor to the ozone formation potential (32.4 %) and has been shown to enhance ambient tropospheric ozone mixing ratios by 19 ppb (Kumar et al., 2016). Both traffic sources combined, the mixed daytime sources, biofuel use and waste disposal, and industrial emissions and solvent use contributed 21.9 %, 20.3 %, 18.1 % and 7.3 %, respectively, to the ozone formation potential. It is clear that in order to bring ozone levels into compliance with the NAAQS, the wheat-residue burning source of ozone precursors deserves the most attention at this point, but the transport sector and biofuel use and waste disposal should not be neglected either.
Figure 4c shows the contribution of the different sectors to the SOA formation potential (∼32 µg m−3) under low-NOx conditions. Traffic is the single largest contributor and is responsible for contributing 59.0 % of the SOA formation potential followed by biofuel use and waste disposal (14.9 %), wheat-residue burning (13.9 %), industrial emissions and solvent use (10.1 %), and the mixed daytime factor (2.2 %). While the calculated SOA formation potential, particularly from transport sector emissions (Ensberg et al., 2014) and aromatic compounds (Li et al., 2017; Li and Cocker III, 2018), is affected by large uncertainties and may depend in a non-linear fashion on NOx and VOC concentrations (Xu et al., 2015) our calculated SOA formation potential seem to indicate that SOA formation could contribute significantly to the average PM2.5 mass loading (104 µg m−3).
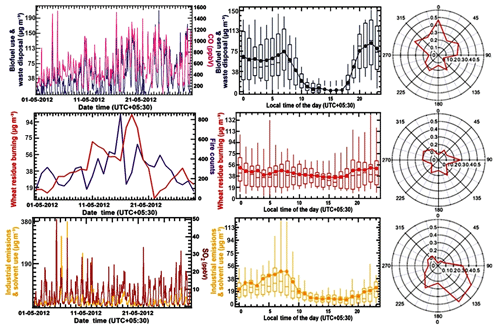
Figure 5Factor contribution time series, factor diel variability and CPF plot for PMF factor 1 (biofuel use and waste disposal), PMF factor 2 (wheat-residue burning) and PMF factor 3 (industrial emissions and solvent use) for May 2012. The time series of PMF factor's hourly mass (in µg m−3) is plotted against independent tracer species CO (in ppbv) for the biofuel use and waste disposal factor, daily fire counts for the wheat-residue burning factor and SO2 (in ppbv) for the industrial emission and solvent use factor. The diel box-and-whisker plot shows the statistical parameters of factor's hourly mass contribution (in µg m−3) for every hour of the day plotted against the start time of the hour. The width of the box gives 25th and 75th percentiles, 50th percentile partitions the box; whiskers represent 10th and 90th percentiles of the dataset and average values are given by solid circles.
3.2 Factor 1 – biofuel use and waste disposal
The biofuel use and waste disposal factor combines two sources with similar source profiles and high spatiotemporal overlap into one factor. As discussed previously for other South Asian atmospheric environments (Sarkar et al., 2017), the source contributions of domestic biofuel use and domestic waste burning are difficult to segregate. Figure 5 shows a weak bimodal behavior with an early morning and late evening peak for this factor, as both domestic biofuel use and waste disposal fires peak in the early morning and in the evening hours (Nagpure et al., 2015). The highest conditional probability for this factor is from the north (>0.4), the direction of the Dadu Majra landfill in Chandigarh, followed by the wind direction NW where a large village (Mauli Baidwan) can be found within 1 km of the receptor, and NE, the direction of Panchkula’s garbage dump in Sector 23. This and the fact that the average contribution of this factor remains above 56 µg m−3 throughout the night indicates that garbage burning contributes significantly to the biofuel use and waste disposal factor.
Figures 3 and 6 show that this factor explains a significant share of the mass of acetonitrile (a biomass burning tracer), aldehydes, ketones, acids, propyne and propene in the PMF model. For propene (60 %), aldehydes (85 %) and ketones (68 %) the residential sector is the dominant source in the most recent speciated emission inventory EDGARv4.3.2. The percentage share for aldehydes and ketones in the inventory is higher than its share in the PMF because the agricultural residue burning source of these compounds is currently missing in the inventory. For acids, however, the residential fuel usage source in the inventory (0.5 %) is dwarfed by solvent-use-associated emissions (96 %), while in the PMF the two biomass burning sources (residential biofuel use and waste disposal and wheat-residue burning) account for almost 69 % of the total acids in the model. High emission of oxygenated VOCs have been reported previously for source profiles of biofuel stoves (Wang et al., 2009; Paulot et al., 2011; Stockwell et al., 2016), open waste burning (Sharma et al., 2019), and PMF factors' results on the residential biofuel use and waste disposal factor in Kathmandu, Nepal (Sarkar et al., 2017).
It should be noted that this factor is responsible for approximately 25 % of the total benzene emissions in our PMF model, while emission inventories attribute a larger share (39 %–74 %) of this compound to this source. Since benzene is an identified Group-1 carcinogen (IARC, 1987) and emissions occur within the household itself (domestic cooking) or within close proximity of the house (waste disposal) this factor deserves special attention in programs targeted at emission reductions. However, the impact of such emission reductions in the residential and waste management sector on human benzene exposure is likely to be overestimated by modeling studies using present-day emission inventories, as the inventories attribute 39 %–74 % of the benzene emissions to residential fuel usage and waste disposal, while the PMF suggests the transport sector is the largest benzene source (Fig. S8a). Direct emission of isocyanic acid, a highly toxic emerging contaminant, and its photochemical precursors (Alkyl amines and Amides) was observed from this source and explained 18 % of the isocyanic acid mass concentration and 7 %–15 % of all the alkyl amines and amides in the PMF model, respectively.
3.3 Factor 2 – wheat-residue burning
Wheat-residue burning takes place every year in the NW IGP in the post-harvest season and generally peaks in the month of May. It has been shown that wheat-residue burning has a major impact on both ozone mixing ratios (Kumar et al., 2016) and VOC mixing ratios and hydroxyl radical reactivity (Kumar et al., 2018) and results in a large suite of unknown (∼40 %) and poorly quantified reactive gaseous emissions. Wheat-residue burning emissions are transported to the receptor site from a large fetch region and often with a significant lag time. Hence, there is no strong conditional probability for enhancements from any specific wind direction (Fig. 5).
Figures 3 and 6 show that the wheat-residue burning factor explains a significant share of all acids, amines/amides, several ketones, aldehydes, isoprene/furan, monoterpenes, acetonotrile, propene, styrene and phenol in the PMF model. This makes wheat-residue burning the largest contributor to the human exposure to isocyanic acid in the month of May both through direct emissions of isocyanic acid and by virtue of being the largest source for its photochemical precursors.
In the EDGARv4.3.2 the agricultural residue burning source of ketones, aldehydes and acids is missing. On the other hand agricultural waste burning appears to be the dominant anthropogenic isoprene source (94 %) in the EDGARv4.3.2 inventory, while in our PMF model residential biofuel usage and the transport sector are equally important contributors to the isoprene/furan mass. The monoterpene emissions from agricultural residue burning (6 %) in the EDGARv4.3.2 inventory are dwarfed by emissions from solvent use (90 %), while in our PMF solution wheat-residue burning and the transport sector appear to be the dominant anthropogenic sources of signals at m∕z 81 and 137.
3.4 Factor 3 – industrial emissions and solvent use
The source fingerprint of the industrial emissions and solvent use factor is dominated by methanol (7.3 µg m−3), acetic acid (3.9 µg m−3) and acetone (2.9 µg m−3). This points towards solvent use (Gaimoz et al., 2011) and/or polymer manufacturing (Sarkar et al., 2017) contributing to the industrial emission and solvent use factor. In addition, Figs. 3 and 6 show that this factor explains a significant fraction of the benzene (20 %) and acetonitrile (17 %) mass in the PMF model. While both are known for their use as solvents (Brown et al., 2007), they can also be emitted from the combustion. The EDGARv4.3.2 emission inventory has a strong industrial and solvent source of toluene, xylenes, acids, formaldehyde and monoterpenes which is not reflected with equal strength in our PMF solution.
The correlation of the industrial emissions and solvent use factor with the SO2 time series (R=0.6), indicates that the emissions of coal or biofuel burning in industrial units and/or coal-fired power plants may also be contributing to this factor profile. Figure 5 shows that the highest conditional probability of this factor is to the southeast direction (120–150 ∘ wind sector). The receptor site is downwind of a 600 MW coal-fired power plant located in Jagadhri (80 km SE) as well as downwind of several industrial areas and brick kiln clusters located around Dera Bassi (15 km), Lalru (20 km) and Jagadhari (80 km) when the wind blows from this direction. In the Kathmandu valley, biofuel co-fired brick kilns explained a significant fraction of the benzene and acetonitrile mass (Sarkar et al., 2017) and the factor profile shows a moderate correlation with the source signature of brick kiln emissions (R=0.5), and hence a combustion contribution from brick kilns to the factor profile cannot be ruled out. The diel profile broadly reflects boundary layer dynamics, with factor contributions increasing continuously throughout the night, indicating a buildup of constant emissions in the nocturnal boundary layer. Factor contributions peak in the early morning (32–49 µg m−3 between 05:00–09:00 local time, LT) and the factor contribution of this factor decreases from 09:00 LT onwards after the breakup of the nocturnal boundary layer. This factor has a higher average than the median factor contributions at night due to strong plumes (∼375 µg m−3) reaching the receptor when it is downwind of the industrial sector but not during other nights when the wind direction is from rural Punjab (NW) or the urban sector (NE).
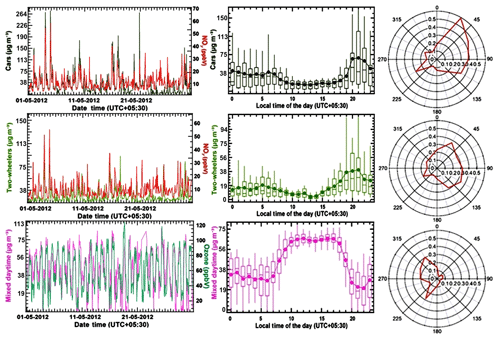
Figure 7Factor contribution time series, factor diel variability and CPF plot for PMF factor 4 and factor 5 (cars and two-wheelers) and PMF factor 6 (mixed daytime) for May 2012. The time series of PMF factor's hourly mass (in µg m−3) is plotted against independent tracer species NOy (in ppbv) for the car and two-wheeler factor and O3 (in ppbv) for the mixed daytime factor. The diel box-and-whisker plot shows the statistical parameters of factor's hourly mass contribution (in µg m−3) for every hour of the day plotted against the start time of the hour. The width of the box gives 25th and 75th percentiles, 50th percentile partitions the box; whiskers represent 10th and 90th percentiles of the dataset and average values are given by solid circles.
3.5 Factor 4 and 5 – cars and two-wheelers
The factor profile of the four-wheeler factor explains a significant share of all aromatic compounds in the PMF model. The factor represents a mixture of multiple components contributed by fuel exhaust and fuel evaporative running losses from vehicles and resembles ambient air samples from a busy traffic intersection. Similar profiles have been observed during field measurements in Beirut, Lebanon (Salameh et al., 2014, 2016) and Hong Kong (Ho et al., 2004). The highest conditional probability (Fig. 7) is observed for the Chandigarh wind sector (0–90 ∘). As reported previously from Mexico City during the Milagro campaign (Bon et al., 2011), a significant mass of methanol (4.3 µg m−3) and other oxygenated VOCs were present in the traffic emissions factor. The fact that this factor explains 28 % of the total m∕z 57 is consistent with the gasoline additive MTBE (which is still in use in India) being detected at this m∕z ratio as an interference to acrolein/methylketone (Karl et al., 2003; Warneke et al., 2003, 2005; Rogers et al., 2006). Signals at m∕z 31, 47, 59, 61, 73 and 87 in aged traffic plumes can be attributed to formaldehyde, formic acid, glyoxal, acetic acid, methylglyoxal and 2-butanedione, which are products of the gas phase oxidation of toluene, C-8 and C-9 aromatic compounds (Bethel et al., 2000; Ervens et al., 2004). In addition, car exhaust also explained 34 % of the propyne mass in the model.
Factor 5, two-wheeler exhaust, explains 50 % of the total toluene mass as well as 17 %, 12 % and 9 % of the total C-8 aromatics, benzene and C-9 aromatics in the PMF model, respectively. The factor shows a signal at m∕z 61 (acetic acid) which may partially be due to fragmentation of octane or ethyl acetate (Warneke et al., 2003; Rogers et al., 2006) which could be present in fuel. The mass has also been attributed to acetic acid in a previous study of diesel tailpipe emissions (Jobson et al., 2005). Nevertheless, it still seems that the two-wheeler factor profile has a higher contribution from oxidized compounds compared to the car factor profile, indicating that the plumes are typically more aged. Figure 7 shows that this factor displays higher conditional probability than the car factor towards the towns Kharar (8 km N), Dera Bassi (15 km SE) and Lalru (20 km SE), and a lower conditional probability than the car factor towards Chandigarh (NE), indicating two-wheelers are more abundant in small towns, while cars dominate the traffic emissions in urban Chandigarh. This is independently supported by vehicle registration data (http://mospi.nic.in/statistical-year-book-india/2018/189, last access: 28 April 2019).
Figure 7 illustrates that both the traffic factors show bimodal peaks in morning (19 µg m−3 at 05:00–09:00 LT) and evening (38 µg m−3 at 19:00–21:00 LT) during peak traffic hours. Mass loadings during evening rush hour are higher than during morning rush hour, because peak morning traffic occurs after the breakup of the nocturnal boundary layer, while in the evening emissions accumulate in the shallow nocturnal boundary layer. When the wind blows from the urban sector (0–90 ∘) during peak traffic hours (19:00–21:00 LT) peak factor contributions of >260 µg m−3 for cars and >150 µg m−3 for two-wheelers are observed.
As can be seen from Fig. 6, the two traffic factors jointly explain 47 %, 80 %, 70 % and 67 % of the total benzene, toluene, C-8 and C-9 aromatic compounds in the model, consistent with findings from the Kathmandu valley that traffic, not residential biofuel use and waste disposal, is the more important source of aromatic compounds in South Asia. It is also clear that despite stringent regulations, the transport sector in the region is still the largest contributor to human benzene exposure. It can be seen from Fig. S8a–d that at present, various emission inventories consider the transport sector to be a minor source of benzene (10 %–16 %). The EDGARv4.3.2 emission inventory also considers the transport sector to be only a minor source of toluene (11 %–15 %) and xylenes (17 %–22 %). Residential fuel usage, industry and solvent use are considered to be the most significant year-round source of benzene, toluene and xylenes in EDGARv4.3.2. Agricultural residue burning becomes the most significant source of all aromatic compounds in the EDGARv4.3.2 emission inventory when crop residue burning emissions are treated as occurring during crop residue burning season only, which may imply that the annual emissions of aromatic compounds from the stubble burning may be overestimated. REAS v.2.1 appears to be overestimating the residential fuel burning contribution to benzene and toluene emissions and the solvent usage contribution to toluene emissions. However, it captures the contribution of the transport sector to xylenes and trimethylbenzenes emissions well.
3.6 Factor 6 – mixed daytime sources
Figures 4 and 6 show that mixed daytime sources comprising biogenic emissions and photochemically formed compounds explained 22 % of the monoterpenes and 25 % of the measured isoprene, respectively. Isoprene has a short chemical lifetime of 1.5 h during the day and 16 % and 11 % of its first-generation oxidation products MVK and MEK (Kesselmeier and Staudt, 1999) were also attributed to this factor. In addition, the mixed daytime factor explains 41 %, 44 %, 24 % and 22 % of the total formaldehyde, formic acid/ethanol, methanol and acetone mass, respectively. Photochemically formed isocyanic acid, formamide, acetamide and propanamide explain a slightly lower fraction (27 %–37 %) of the total mass concentration of these compounds compared to what has been reported from the Kathmandu valley in wintertime (36 %–41 %). Figure 7 illustrates that the mixed daytime factor peaks between 09:00 and 16:00 LT and shows a slightly enhanced conditional probability for the 180–330∘ rural wind sector (0.2–0.3) due to agroforestry plantations of poplar in the rural landscape.
3.7 Comparison of PMF source factors with existing emission inventories
Figure 8 shows pie charts depicting the contribution of different sectors to the total VOC mass burden for the emission inventories and our PMF output. Biofuel use and waste disposal were responsible for 28.1 % of the mass in our PMF but 39 %, 44.2 % and 41.7 % of the mass in EDGARv4.3.2, GAINS and REASv2.1 respectively. The contribution of crop residue burning (27.1 %) to the VOC mass in the month of May would be highly underestimated by both GAINS (7 %) and EDGARv4.3.2 (6 %) if the annual emissions are attributed equally to all months of the year. However, if both emission inventories would attribute their annual crop residue burning emissions over the region only to the 2.5 months when crop residue burning actually occurs (middle of October to end of November and May), these emission inventories could be reconciled with the PMF solution, as emissions in May would amount to 26.5 % and 23 % of the monthly VOC emissions for the month of May for GAINS and EDGARv4.3.2, respectively, as shown in Fig. 8. At the same time the percentage share of domestic fuel use and waste disposal would drop to 32 % and 35 % in EDGARv4.3.2 and GAINS, respectively, and the contribution of industrial emissions and solvent use would drop to 18 % in GAINS and 30 % in EDGAR. Our PMF (14.3 %) solution indicates that industrial emissions and solvent usage (14.3 %) are currently overestimated in all emission inventories but are closest to GAINS (540 Gg yr−1, 18 %) for industrial emissions and solvent use. For domestic biofuel use and waste disposal EDGARv4.3.2 (968 Gg yr−1, 32 %) appears to agree best with our PMF solution. For wheat-residue burning GAINS agrees well with our PMF output, while the agricultural waste burning emissions of some of the detected compound groups (ketones, aldehydes and acids) appear to be missing in the EDGARv4.3.2 inventory. Our PMF solution for road transport sector emissions (30.5 %) lies in between the estimates of GAINS (558 Gg yr−1, 24 %) and REAS (1230 Gg yr−1, 36.2 %), possibly because not all pre-2000 super-emitters for which the 20-year vehicle lifetime has been exceeded have been retired as planned.
Overall it appears that none of the emission inventories is ideal at the present. Our PMF solution suggests that transport sector emissions may be underestimated by GAINS and EDGARv4.3.2, while the combined effect of residential biofuel use and waste disposal emissions as well as the VOC burden associated with solvent use may be overestimated by all emission inventories. Similar results have been reported previously. Sarkar and co-workers (Sarkar et al., 2017) reported an underestimation of transport sector emissions for the REAS and EDGAR emission inventory for the Kathmandu valley in Nepal and an overestimation of the residential biofuel use and waste disposal source in all emission inventories, while Gaimoz and co-workers (Gaimoz et al., 2011) reported an overestimation of the VOC emissions from solvent use in Paris.
Our results highlight that for accurate air quality forecasting and modeling it is essential that emissions are attributed only to the months in which the activity actually occurs. This is important for emissions from crop residue burning (which occur in May and from mid-October to the end of November). Annually averaged emissions are unlikely to yield accurate air quality forecast in regions affected by such seasonal events. At present, more specialized fire emission inventories such as FINN (Wiedinmyer et al., 2011) must be used to account for the full seasonality and day-to-day variations of open burning emissions. We also demonstrate that the source profiles obtained as PMF output can be validated and matched against samples collected at the potential sources to validate the factor identification.
For the human class I carcinogen benzene, the traffic factor alone contributed to 47 % of the total benzene mass at this receptor site followed by residential biofuel use and waste disposal (25 %) and industrial emissions and solvent use (20 %). This stands in stark contrast to various emission inventories which estimate the transport sector contribution to the benzene exposure to be 10 % and consider residential biofuel use, agricultural residue burning and industry to be more important benzene sources. Since the annual NAAQS for benzene is exceeded at this receptor site (Chandra and Sinha, 2016), all three sectors must be targeted for emission reductions.
For the emerging contaminant isocyanic acid, photochemical formation from precursors (37 %), wheat-residue burning (25 %), and biofuel usage and waste disposal (18 %) were the largest contributors to human exposure. The monthly average isocyanic acid mixing ratio of 1.4 ppb exceeds concentrations that can, after dissociation at blood pH, result in blood cyanate ion concentrations (Roberts et al., 2011) high enough to produce significant health effects in humans (Wang et al., 2007) such as atherosclerosis, cataracts and rheumatoid arthritis due to protein damage. Peak mixing ratios of this compound exceed 3 ppb in some nighttime wheat-residue burning plumes. Wheat-residue burning was also the single largest source of the photochemical precursors of isocyanic acid, namely, formamide, acetamide and propanamide, indicating that this source must be most urgently targeted to reduce human concentration exposure to isocyanic acid.
Overall it appears that none of the emission inventories is ideal at the present. Our PMF solution suggests that transport sector emissions may be underestimated by GAINSv5.0 and EDGARv4.3.2, while the combined effect of residential biofuel use and waste disposal emissions as well as the VOC burden associated with solvent use may be overestimated by all emission inventories. Agricultural waste burning emissions of some of the detected compound groups (ketones, aldehydes and acids) are currently missing in the EDGARv4.3.2 inventory, while aromatic emissions from the same source appear to be overestimated. Thus, large improvements are required in existing emission inventories for correct source attribution and inclusion of missing compounds over this densely populated region of the world.
Data are available from the corresponding author upon request.
The supplement related to this article is available online at: https://doi.org/10.5194/acp-19-15467-2019-supplement.
P performed the analysis and wrote the first draft of the paper. BS conceived the analysis and revised the paper draft. VS collected the data and commented on the paper draft.
The authors declare that they have no conflict of interest.
We acknowledge the IISER Mohali Atmospheric Chemistry facility for data and the Ministry of Human Resource Development (MHRD), India, for funding the facility. Pallavi acknowledges IISER Mohali for Institute PhD fellowship.
This research has been supported by the National Mission on Strategic knowledge for Climate Change (NMSKCC) MRDP Program of the Department of Science and Technology, India, DST Climate Change Program (SPLICE) (grant no. DST/CCP/MRDP/100/2017(G)).
This paper was edited by James Roberts and reviewed by two anonymous referees.
Amann, M., Bertok, I., Borken-Kleefeld, J., Cofala, J., Heyes, C., Hoeglund-Isaksson, L., Klimont, Z., Nguyen, B., Posch, M., Rafaj, P., Sandler, R., Schoepp, W., Wagner, F., and Winiwarter, W.: Cost-effective control of air quality and greenhouse gases in Europe: Modeling and policy applications, Environ. Model Softw., 26, 1489–1501., https://doi.org/10.1016/j.envsoft.2011.07.012, 2011. a, b
Bethel, H. L., Atkinson, R., and Arey, J.: Products of the gas-phase reactions of OH radicals with p-xylene and 1, 2, 3- and 1, 2, 4-trimethylbenzene: effect of NO2 concentration, J. Phys. Chem. A, 104, 8922–8929, https://doi.org/10.1021/jp001161s, 2000. a
Bon, D. M., Ulbrich, I. M., de Gouw, J. A., Warneke, C., Kuster, W. C., Alexander, M. L., Baker, A., Beyersdorf, A. J., Blake, D., Fall, R., Jimenez, J. L., Herndon, S. C., Huey, L. G., Knighton, W. B., Ortega, J., Springston, S., and Vargas, O.: Measurements of volatile organic compounds at a suburban ground site (T1) in Mexico City during the MILAGRO 2006 campaign: measurement comparison, emission ratios, and source attribution, Atmos. Chem. Phys., 11, 2399–2421, https://doi.org/10.5194/acp-11-2399-2011, 2011. a, b
Brown, S. G., Frankel, A., and Hafner, H. R.: Source apportionment of VOCs in the Los Angeles area using positive matrix factorization, Atmos. Environ., 41, 227–237, https://doi.org/10.1016/j.atmosenv.2006.08.021, 2007. a
Brown, S. G., Eberly, S., Paatero, P., and Norris, G. A.: Methods for estimating uncertainty in PMF solutions: Examples with ambient air and water quality data and guidance on reporting PMF results, Sci. Total Environ., 518, 626–635, https://doi.org/10.1016/j.scitotenv.2015.01.022, 2015. a, b
Census: Government of India, Ministry of Home Affairs, Office of the Registrar General & Census Commissioner, India, available at: http://www.censusindia.gov.in/pca/Searchdata.aspx (last access: 25 July 2018), 2011. a
Chandra, B., Sinha, V., Hakkim, H., and Sinha, B.: Storage stability studies and field application of low cost glass flasks for analyses of thirteen ambient VOCs using proton transfer reaction mass spectrometry, Int. J. Mass Spectrom., 419, 11–19, https://doi.org/10.1016/j.ijms.2017.05.008, 2017. a, b
Chandra, B. P. and Sinha, V.: Contribution of post-harvest agricultural paddy residue fires in the NW Indo-Gangetic Plain to ambient carcinogenic benzenoids, toxic isocyanic acid and carbon monoxide, Environ. Int., 88, 187–197, https://doi.org/10.1016/j.envint.2015.12.025, 2016. a, b, c, d
Derwent, R. G., Jenkin, M. E., Utembe, S. R., Shallcross, D. E., Murrells, T. P., and Passant, N. R.: Secondary organic aerosol formation from a large number of reactive man-made organic compounds, Sci. Total Environ., 408, 3374–3381, https://doi.org/10.1016/j.scitotenv.2010.04.013, 2010. a
Ensberg, J. J., Hayes, P. L., Jimenez, J. L., Gilman, J. B., Kuster, W. C., de Gouw, J. A., Holloway, J. S., Gordon, T. D., Jathar, S., Robinson, A. L., and Seinfeld, J. H.: Emission factor ratios, SOA mass yields, and the impact of vehicular emissions on SOA formation, Atmos. Chem. Phys., 14, 2383–2397, https://doi.org/10.5194/acp-14-2383-2014, 2014. a
Ervens, B., Feingold, G., Frost, G. J., and Kreidenweis, S. M.: A modeling study of aqueous production of dicarboxylic acids: 1. Chemical pathways and speciated organic mass production, J. Geophys. Res.-Atmos., 109, D15205, https://doi.org/10.1029/2003JD004387, 2004. a
Gaimoz, C., Sauvage, S., Gros, V., Herrmann, F., Williams, J., Locoge, N., Perrussel, O., Bonsang, B., d’Argouges, O., and Sarda-Estève, R.: Volatile organic compounds sources in Paris in spring 2007. Part II: source apportionment using positive matrix factorisation, Environ. Chem., 8, 91–103, https://doi.org/10.1071/EN10067, 2011. a, b, c
Ho, K., Lee, S., Guo, H., and Tsai, W.: Seasonal and diurnal variations of volatile organic compounds (VOCs) in the atmosphere of Hong Kong, Sci. Total Environ., 322, 155–166, https://doi.org/10.1016/j.scitotenv.2003.10.004, 2004. a
Hopke, P.: Review of receptor modeling methods for source apportionment, J. Air Waste Manage., 66, 237–259, https://doi.org/10.1080/10962247.2016.1140693, 2016. a
Huang, G., Brook, R., Crippa, M., Janssens-Maenhout, G., Schieberle, C., Dore, C., Guizzardi, D., Muntean, M., Schaaf, E., and Friedrich, R.: Speciation of anthropogenic emissions of non-methane volatile organic compounds: a global gridded data set for 1970–2012, Atmos. Chem. Phys., 17, 7683–7701, https://doi.org/10.5194/acp-17-7683-2017, 2017. a, b
IARC: International Agency for Research on Cancer. Overall Evaluations of Carcinogenicity: An Updating of IARC Monographs Volumes 1 to 42, Supplement 7, available at: https://monographs.iarc.fr/ENG/Monographs/suppl7/Suppl7.pdf (last access: 1 April 2019), 1987. a
IPCC: Climate Change 2013: The Physical Science Basis. Contribution of Working Group I to the Fifth Assessment Report of the Intergovernmental Panel on Climate Change, edited byL Stocker, T. F., Qin, D., Plattner, G.-K., Tignor, M., Allen, S. K., Boschung, J., Nauels, A., Xia, Y., Bex, V., and Midgley, P. M., https://doi.org/10.1017/CBO9781107415324, 2013. a
Jobson, B., Alexander, M. L., Maupin, G. D., and Muntean, G. G.: Online analysis of organic compounds in diesel exhaust using a proton transfer reaction mass spectrometer (PTR-MS), Int. J. Mass Spectrom., 245, 78–89, https://doi.org/10.1016/j.ijms.2005.05.009, 2005. a
Karl, T., Jobson, T., Kuster, W. C., Williams, E., Stutz, J., Shetter, R., Hall, S. R., Goldan, P., Fehsenfeld, F., and Lindinger, W.: Use of proton transfer reaction mass spectrometry to characterize volatile organic compound sources at the La Porte super site during the Texas Air Quality Study 2000, J. Geophys. Res.-Atmos., 108, 4508, https://doi.org/10.1029/2002JD003333, 2003. a
Kesselmeier, J. and Staudt, M.: Biogenic volatile organic compounds (VOC): an overview on emission, physiology and ecology, J. Atmos. Chem., 33, 23–88, https://doi.org/10.1023/A:1006127516791, 1999. a
Kumar, V., Sarkar, C., and Sinha, V.: Influence of post-harvest crop residue fires on surface ozone mixing ratios in the NW IGP analyzed using 2 years of continuous in situ trace gas measurements, J. Geophys. Res.-Atmos., 121, 3619–3633, https://doi.org/10.1002/2015JD024308, 2016. a, b, c, d
Kumar, V., Chandra, B., and Sinha, V.: Large unexplained suite of chemically reactive compounds present in ambient air due to biomass fires, Sci. Rep., 8, 626, https://doi.org/10.1038/s41598-017-19139-3, 2018. a, b
Kurokawa, J., Ohara, T., Morikawa, T., Hanayama, S., Janssens-Maenhout, G., Fukui, T., Kawashima, K., and Akimoto, H.: Emissions of air pollutants and greenhouse gases over Asian regions during 2000–2008: Regional Emission inventory in ASia (REAS) version 2, Atmos. Chem. Phys., 13, 11019–11058, https://doi.org/10.5194/acp-13-11019-2013, 2013. a, b
Leuchner, M. and Rappenglück, B.: VOC source–receptor relationships in Houston during TexAQS-II, Atmos. Environ., 44, 4056–4067, https://doi.org/10.1016/j.atmosenv.2009.02.029, 2010. a, b
Li, J., Zhang, M., Wu, F., Sun, Y., and Tang, G.: Assessment of the impacts of aromatic VOC emissions and yields of SOA on SOA concentrations with the air quality model RAMS-CMAQ, Atmos. Environ., 158, 105–115, https://doi.org/10.1016/j.atmosenv.2017.03.035, 2017. a
Li, W. and Cocker III, D. R.: Assessment of the impacts of aromatic VOC emissions and yields of SOA on SOA concentrations with the air quality model RAMS-CMAQ, Atmos. Environ., 184, 17–23, https://doi.org/10.1016/j.atmosenv.2018.03.059, 2018. a
Majumdar, D., Mukherjee, A., and Sen, S.: Apportionment of Sources to Determine Vehicular Emission Factors of BTEX in Kolkata, India, Water Air Soil Pollut., 209, 379–388, https://doi.org/10.1007/s11270-008-9951-1, 2009. a, b
Nagpure, A. S., Ramaswami, A., and Russell, A.: Characterizing the spatial and temporal patterns of open burning of municipal solid waste (MSW) in Indian cities, Environ. Sci. Technol., 49, 12904–12912, https://doi.org/10.1021/acs.est.5b03243, 2015. a
Norris, G., Duvall, R., Brown, S., and Bai, S.: EPA Positive Matrix Factorization (PMF) 5.0 Fundamentals and User Guide, available at: https://www.epa.gov/sites/production/files/2015-02/documents/pmf_5.0_user_guide.pdf (last access: 31 October 2019), 2014. a, b, c, d
Paatero, P.: Least squares formulation of robust non-negative factor analysis, Chemom. Intell. Lab. Syst., 37, 23–35, https://doi.org/10.1016/S0169-7439(96)00044-5, 1997. a, b
Paatero, P. and Hopke, P. K.: Rotational tools for factor analytic models, J. Chemometr., 23, 91–100, https://doi.org/10.1002/cem.1197, 2009. a, b
Paatero, P. and Tapper, U.: Positive matrix factorization: A non-negative factor model with optimal utilization of error estimates of data values, Environmetrics, 5, 111–126, https://doi.org/10.1002/env.3170050203, 1994. a, b
Paatero, P., Hopke, P. K., Song, X. H., and Ramadan, Z.: Understanding and controlling rotations in factor analytic models, Chemometr. Intell. Lab., 60, 253–264, https://doi.org/10.1016/S0169-7439(01)00200-3, 2002. a
Paatero, P., Eberly, S., Brown, S. G., and Norris, G. A.: Methods for estimating uncertainty in factor analytic solutions, Atmos. Meas. Tech., 7, 781–797, https://doi.org/10.5194/amt-7-781-2014, 2014. a, b, c
Paulot, F., Wunch, D., Crounse, J. D., Toon, G. C., Millet, D. B., DeCarlo, P. F., Vigouroux, C., Deutscher, N. M., González Abad, G., Notholt, J., Warneke, T., Hannigan, J. W., Warneke, C., de Gouw, J. A., Dunlea, E. J., De Mazière, M., Griffith, D. W. T., Bernath, P., Jimenez, J. L., and Wennberg, P. O.: Importance of secondary sources in the atmospheric budgets of formic and acetic acids, Atmos. Chem. Phys., 11, 1989–2013, https://doi.org/10.5194/acp-11-1989-2011, 2011. a
Pawar, H., Garg, S., Kumar, V., Sachan, H., Arya, R., Sarkar, C., Chandra, B. P., and Sinha, B.: Quantifying the contribution of long-range transport to particulate matter (PM) mass loadings at a suburban site in the north-western Indo-Gangetic Plain (NW-IGP), Atmos. Chem. Phys., 15, 9501–9520, https://doi.org/10.5194/acp-15-9501-2015, 2015. a, b
Ramanathan, V., Cicerone, R. J., Singh, H. B., and Kiehl, J. T.: Trace gas trends and their potential role in climate change, J. Geophys. Res.-Atmos., 90, 5547–5566, https://doi.org/10.1029/JD090iD03p05547, 1985. a
Roberts, J. M., Veres, P. R., Cochran, A. K., Warneke, C., Burling, I. R., Yokelson, R. J., Lerner, B., Gilman, J. B., Kuster, W. C., Fall, R., and de, G. J.: Isocyanic acid in the atmosphere and its possible link to smoke-related health effects, P. Natl. Acad. Sci. USA, 108, 8966–8971, 2011. a
Rogers, T., Grimsrud, E., Herndon, S., Jayne, J., Kolb, C. E., Allwine, E., Westberg, H., Lamb, B., Zavala, M., and Molina, L.: On-road measurements of volatile organic compounds in the Mexico City metropolitan area using proton transfer reaction mass spectrometry, Int. J. Mass Spectrom., 252, 26–37, https://doi.org/10.1016/j.ijms.2006.01.027, 2006. a, b
Salameh, T., Afif, C., Sauvage, S., Borbon, A., and Locoge, N.: Speciation of non-methane hydrocarbons (NMHCs) from anthropogenic sources in Beirut, Lebanon, Environ. Sci. Pollut. Res., 21, 10867–10877, https://doi.org/10.1007/s11356-014-2978-5, 2014. a
Salameh, T., Sauvage, S., Afif, C., Borbon, A., and Locoge, N.: Source apportionment vs. emission inventories of non-methane hydrocarbons (NMHC) in an urban area of the Middle East: local and global perspectives, Atmos. Chem. Phys., 16, 3595–3607, https://doi.org/10.5194/acp-16-3595-2016, 2016. a
Sarkar, C., Sinha, V., Kumar, V., Rupakheti, M., Panday, A., Mahata, K. S., Rupakheti, D., Kathayat, B., and Lawrence, M. G.: Overview of VOC emissions and chemistry from PTR-TOF-MS measurements during the SusKat-ABC campaign: high acetaldehyde, isoprene and isocyanic acid in wintertime air of the Kathmandu Valley, Atmos. Chem. Phys., 16, 3979–4003, https://doi.org/10.5194/acp-16-3979-2016, 2016. a, b, c, d
Sarkar, C., Sinha, V., Sinha, B., Panday, A. K., Rupakheti, M., and Lawrence, M. G.: Source apportionment of NMVOCs in the Kathmandu Valley during the SusKat-ABC international field campaign using positive matrix factorization, Atmos. Chem. Phys., 17, 8129–8156, https://doi.org/10.5194/acp-17-8129-2017, 2017. a, b, c, d, e
Sharma, G., Sinha, B., Jangra, P., Hakkim, H., Chandra, B. P., Kumar, A., and Sinha, V.: Gridded emissions of CO, NOx, SO2, CO2, NH3, HCl, CH4, PM2.5, PM10, BC and NMVOC from open municipal waste burning in India, Environ. Sci. Technol., 53, 4765–4774, 2019. a, b
Sindelarova, K., Granier, C., Bouarar, I., Guenther, A., Tilmes, S., Stavrakou, T., Müller, J.-F., Kuhn, U., Stefani, P., and Knorr, W.: Global data set of biogenic VOC emissions calculated by the MEGAN model over the last 30 years, Atmos. Chem. Phys., 14, 9317–9341, https://doi.org/10.5194/acp-14-9317-2014, 2014. a
Sinha, V., Williams, J., Diesch, J. M., Drewnick, F., Martinez, M., Harder, H., Regelin, E., Kubistin, D., Bozem, H., Hosaynali-Beygi, Z., Fischer, H., Andrés-Hernández, M. D., Kartal, D., Adame, J. A., and Lelieveld, J.: Constraints on instantaneous ozone production rates and regimes during DOMINO derived using in-situ OH reactivity measurements, Atmos. Chem. Phys., 12, 7269–7283, https://doi.org/10.5194/acp-12-7269-2012, 2012. a
Sinha, V., Kumar, V., and Sarkar, C.: Chemical composition of pre-monsoon air in the Indo-Gangetic Plain measured using a new air quality facility and PTR-MS: high surface ozone and strong influence of biomass burning, Atmos. Chem. Phys., 14, 5921–5941, https://doi.org/10.5194/acp-14-5921-2014, 2014. a, b, c, d, e
Srivastava, A.: Source apportionment of ambient VOCS in Mumbai city, Atmos. Environ., 38, 6829–6843, https://doi.org/10.1016/j.atmosenv.2004.09.009, 2004. a, b, c
Srivastava, A., Sengupta, B., and Dutta, S.: Source apportionment of ambient VOCs in Delhi City, Sci. Total Environ., 343, 207–220, https://doi.org/10.1016/j.scitotenv.2004.10.008, 2005. a, b, c
Stockwell, C. E., Christian, T. J., Goetz, J. D., Jayarathne, T., Bhave, P. V., Praveen, P. S., Adhikari, S., Maharjan, R., DeCarlo, P. F., Stone, E. A., Saikawa, E., Blake, D. R., Simpson, I. J., Yokelson, R. J., and Panday, A. K.: Nepal Ambient Monitoring and Source Testing Experiment (NAMaSTE): emissions of trace gases and light-absorbing carbon from wood and dung cooking fires, garbage and crop residue burning, brick kilns, and other sources, Atmos. Chem. Phys., 16, 11043–11081, https://doi.org/10.5194/acp-16-11043-2016, 2016. a, b
Stohl, A., Aamaas, B., Amann, M., Baker, L. H., Bellouin, N., Berntsen, T. K., Boucher, O., Cherian, R., Collins, W., Daskalakis, N., Dusinska, M., Eckhardt, S., Fuglestvedt, J. S., Harju, M., Heyes, C., Hodnebrog, Ø., Hao, J., Im, U., Kanakidou, M., Klimont, Z., Kupiainen, K., Law, K. S., Lund, M. T., Maas, R., MacIntosh, C. R., Myhre, G., Myriokefalitakis, S., Olivié, D., Quaas, J., Quennehen, B., Raut, J.-C., Rumbold, S. T., Samset, B. H., Schulz, M., Seland, Ø., Shine, K. P., Skeie, R. B., Wang, S., Yttri, K. E., and Zhu, T.: Evaluating the climate and air quality impacts of short-lived pollutants, Atmos. Chem. Phys., 15, 10529–10566, https://doi.org/10.5194/acp-15-10529-2015, 2015. a
Wang, S., Wei, W., Du, L., Li, G., and Hao, J.: Characteristics of gaseous pollutants from biofuel-stoves in rural China, Atmos. Environ., 43, 4148–4154, https://doi.org/10.1016/j.atmosenv.2009.05.040, 2009. a
Wang, Z., Nicholls, S. J., Rodriguez, E. R., Kummu, O., Hörkkö, S., Barnard, J., Reynolds, W. F., Topol, E. J., DiDonato, J. A., and Hazen, S. L.: Protein carbamylation links inflammation, smoking, uremia and atherogenesis, Nat. Med., 13, 1176–1184, 2007. a
Warneke, C., De Gouw, J. A., Kuster, W. C., Goldan, P. D., and Fall, R.: Validation of atmospheric VOC measurements by proton transfer reaction mass spectrometry using a gas-chromatographic preseparation method, Environ. Sci. Technol., 37, 2494–2501, https://doi.org/10.1021/es026266i, 2003. a, b
Warneke, C., Kato, S., de Gouw, J. A., Goldan, P. D., Kuster, W. C., Shao, M., Lovejoy, E. R., Fall, R., and Fehsenfeld, F. C.: Online volatile organic compound measurements using a newly developed proton transfer ion trap mass spectrometry instrument during New England Air Quality Study Intercontinental Transport and Chemical Transformation 2004: Performance, intercomparison, and compound identification, Environ. Sci. Technol., 39, 5390–5397, https://doi.org/10.1021/es050602o, 2005. a
Wiedinmyer, C., Akagi, S. K., Yokelson, R. J., Emmons, L. K., Al-Saadi, J. A., Orlando, J. J., and Soja, A. J.: The Fire INventory from NCAR (FINN): a high resolution global model to estimate the emissions from open burning, Geosci. Model Dev., 4, 625–641, https://doi.org/10.5194/gmd-4-625-2011, 2011. a
Xie, Y. and Berkowitz, C. M.: The use of positive matrix factorization with conditional probability functions in air quality studies: an application to hydrocarbon emissions in Houston, Texas, Atmos. Environ., 40, 3070–3091, https://doi.org/10.1016/j.atmosenv.2005.12.065, 2006. a
Xu, J., Griffin, R. J., Liu, Y., Nakao, S., and Cocker III, D. R.: Simulated impact of NOx on SOA formation from oxidation of toluene and m-xylene, Atmos. Environ., 101, 217e225, https://doi.org/10.1016/j.atmosenv.2014.11.008, 2015. a
Zhong, M., Saikawa, E., Avramov, A., Chen, C., Sun, B., Ye, W., Keene, W. C., Yokelson, R. J., Jayarathne, T., Stone, E. A., Rupakheti, M., and Panday, A. K.: Nepal Ambient Monitoring and Source Testing Experiment (NAMaSTE): emissions of particulate matter and sulfur dioxide from vehicles and brick kilns and their impacts on air quality in the Kathmandu Valley, Nepal, Atmos. Chem. Phys., 19, 8209–8228, https://doi.org/10.5194/acp-19-8209-2019, 2019. a