the Creative Commons Attribution 4.0 License.
the Creative Commons Attribution 4.0 License.
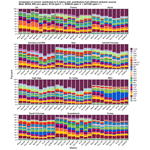
Source attribution of European surface O3 using a tagged O3 mechanism
Aurelia Lupaşcu
Tim Butler
Tropospheric ozone (O3) is an important air pollutant that affects human health, ecosystems, and climate. The contributions of O3 precursor emissions from different geographical source regions to the O3 concentration can help to quantify the effects of local versus remotely transported precursors on the O3 concentration in a certain area. This study presents a “tagging” approach within the WRF-Chem model that attributes O3 concentration in several European receptor regions to nitrogen oxide (NOx) emissions from within and outside of Europe during April–September 2010. We also examine the contribution of these different precursor sources to various O3 metrics and their exceedance events. Firstly, we show that the spatial distributions of simulated monthly mean MDA8 from tagged O3 source regions and types for late spring, summer, and early autumn 2010 varies with season. For summer conditions, O3 production is dominated by national and intra-European sources, while in the late spring and early autumn intercontinental transported O3 is an important contributor to the total O3 concentration. We have also identified shipping activities in the Mediterranean Sea as an important source of O3 for the Mediterranean countries, as well as the main contributor to high modelled MDA8 O3 concentration in the Mediterranean Basin itself. Secondly, to have a better understanding of the origin of MDA8 O3 exceedances, we compare modelled and observed values of MDA8 O3 concentration in the Po Valley and Germany–Benelux receptor regions, revealing that the contribution from local sources is about 41 % and 38 % of modelled MDA8 O3 during the exceedance days, respectively. By examining the relative contributions of remote NOx sources to modelled and observed O3 exceedance events, we determine that model underrepresentation of long-range O3 transport could be contributing to a general underestimation of modelled O3 exceedance events in the Germany–Benelux receptor region. Thirdly, we quantify the impact of local vs. non-local NOx precursors on O3 production for each European receptor region using different O3 metrics. The comparison between mean, MDA8 and 95th percentile O3 metrics accentuates the importance of large contributions from locally emitted NOx precursors to the high end of the O3 distribution. When we compare the vegetation and health metrics, we notice that the SOMO35 and AOT40 indexes exhibit rather similar behaviour, while the W126 index accentuates the importance of local emissions. Overall, this study highlights the importance of a tagging approach to quantify the contribution of local and remote sources to the MDA8 O3 concentration during several periods as well to different O3 metrics. Moreover, this method could be applied to assess different mitigation options.
- Article
(2935 KB) - Full-text XML
-
Supplement
(1070 KB) - BibTeX
- EndNote
Tropospheric ozone (O3) is formed primarily during the oxidation of volatile organic compounds (VOCs) in the presence of nitrogen oxides (NOx) and sunlight. Ground-level O3 is an important air pollutant that damages human health (Fleming et al., 2018) and vegetation (Mills et al., 2018). It also affects the radiative forcing (e.g. Ramaswamy et al., 2001; Stevenson et al., 2013) and therefore contributes to climate change. Impacts of O3 on human health are associated with lung disease, chronic disease, and death from respiratory ailments. To protect human populations from exposure to high levels of O3, the World Health Organization (WHO, 2006, 2017) recommended an air quality guideline for ozone in which the maximum daily average 8 h (MDA8) for O3 should not exceed 100 µg m−3. The European Environmental Agency (EEA, 2017a) reported that the EU long-term objective target concentration of 120 µg m−3 is often exceeded and that more than 90 % of the urban population of the European Union was exposed to O3 levels higher than the stricter recommendation set by the WHO. A 2010 report from HTAP (HTAP, 2010) shows that the observed baseline O3 concentrations (concentrations without the contribution from local anthropogenic emissions) have increased throughout the last several decades since overall global anthropogenic emissions of O3 precursors have increased. However, a more recent study by Gaudel et al. (2018) has established that the global surface O3 trends exhibit high variability and depend on several factors such as season, region, elevation, and proximity to fresh ozone precursor emissions. However, since the network capable of monitoring ozone levels is sparse, it is difficult to quantify the O3 changes on a global scale. Satellite-derived O3 measurements can be used to quantify changing levels of O3, but Gaudel et al. (2018) showed that these products are not capable of quantifying significant trends. Surface O3 pollution due to urbanisation and motorisation processes are serious challenges for large cities (e.g. Chan and Yao, 2008; Folberth et al., 2015; Li et al., 2017, 2019). Paoletti et al. (2014) showed that in Europe and the United States of America, the average O3 concentration in the cities has increased at a faster rate than that observed in rural areas. Fleming et al. (2018) showed that the fourth highest daily maximum 8 h O3 (4MDA8) is more ubiquitous at urban sites than at non-urban sites. This leads to a worsening of general air quality that, ultimately, affects human health and ecosystems (Paoletti et al., 2014; Monks et al., 2015; WHO, 2017; Fleming et al., 2018; Mills et al., 2018). To improve the air quality in certain areas, it is important to know the extent to which different precursors (NOx and VOCs) contribute to tropospheric O3 concentrations.
Information regarding levels of NOx and VOC emissions and weather conditions enhances our ability to predict the formation of tropospheric O3. The continuous development of chemical transport models can lead to a better understanding of the processes that contribute to high-O3 episodes. Knowing the impacts of NOx and VOC emissions from sources such as surface anthropogenic activities, fires, soil, and the stratosphere on total O3 production can help authorities develop strategies aimed at reducing the impact of high levels of O3 on the well-being of both humans and ecosystems. Several approaches have been used to determine the extent to which individual sources contribute to total levels of O3. For example, perturbation of different emission categories has allowed scientists to make estimations regarding the contributions of individual sources of O3 to total O3 levels (e.g. Fiore et al., 2009).
Tagging techniques have also been used in modelling studies to determine source–receptor relationships and how individual sources of pollutants contribute to total pollution levels at given locations. Pollutants with relatively low chemical reactivities, such as carbon monoxide (CO), can be “tagged” according to their emission sectors or regions for attribution studies (e.g. Pfister et al., 2011). Sudo and Akimoto (2007) and Derwent et al. (2015) used O3 tracers tagged by their region of formation to show that intercontinental transport of O3 can occur from polluted source regions, such as North America and East Asia, and appears to be the most important source of tropospheric O3 in Europe. Other studies, including those of Wang et al. (2009) and Grewe et al. (2010, 2012, 2017), have used tagging methods to identify the contribution of individual sources of O3 to overall levels. This method is especially useful since it can track emitted NOx species during transport and chemical processing. Moreover, Grewe et al. (2012) showed the impact of the tagging method on mitigation measures, while Dahlmann et al. (2011) examined the contribution of O3 sources to O3 radiative forcing. Work by Emmons et al. (2012) and Butler et al. (2018) describes a procedure for tagging O3 produced from NOx sources through updates to the MOZART chemical mechanism, and Butler et al. (2018) expanded the tagging technique to account for VOC sources.
Based on the work of Emmons et al. (2012), Pfister et al. (2013) and Safieddine et al. (2014) were able to use the WRF-Chem regional model to quantify the contribution of inflow (tagged O3 and odd nitrogen species entering into the regional domain at the lateral boundaries) and of anthropogenic NOx precursors (named NOx in the following) on the surface O3 levels. Using a slightly different methodology, Gao et al. (2016) have implemented within the WRF-Chem framework a tagging method based on Ozone Source Apportionment Technology (OSAT) (Yarwood et al., 1996) incorporated in the Comprehensive Air quality Model with extensions (CAMx).
Much effort has been focused on understanding the origin of tropospheric O3 and the key role played by the intercontinental transport and the contribution of stratospheric O3 intrusion and of different emissions sources to tropospheric O3 concentration in a wide range of receptor regions. To better understand the changes in air pollution levels, it is necessary to know the relationship between levels of an emitted species and its atmospheric concentration. When this information is available, it is possible to quantify the contribution of different emission precursor sources to overall O3 concentration levels at a particular receptor location. For this purpose, we followed a strategy outlined in Emmons et al. (2012) and Butler et al. (2018) to implement a tagging technique into the regional WRF-Chem model. The model can be used to quantify source contributions to the tropospheric O3 concentration, by tagging NOx emissions, and corresponding products so that they can be traced to the final production of O3.
When studying the effects of O3, the impact of the compound on humans and vegetation is of the utmost importance. Therefore, several exposure indexes have been defined to describe the relationship between O3 and both human health and agricultural crop yield that are based on hourly averaged data. Musselman et al. (2006), Agathokleous et al. (2018), and Lefohn et al. (2018) review literature describing O3 metrics. Additionally, work by Paoletti et al. (2007) has provided a list of common O3 exposure metrics used to assess risk to human health and vegetation. Here we use some well-known O3 metrics, such as MDA8, SOMO35, AOT40, and W126. The MDA8 index has been defined as the maximum daily average 8 h (MDA8) O3 values (ppb) (Lefohn et al., 2018). SOMO35 (WHO, 2001) has been determined by European protocols (EU directive 2008/50/EC, 2008) and is defined as the annual sum of MDA8 O3 with a cut-off of values of 35 ppb. Both MDA8 and SOMO34 are health-related metrics. The AOT40 and W126 vegetation metrics have been used to regulate air pollution in both Europe (EU directive 2008/50/EC, 2008) and the United States (U.S. EPA regulations https://www.gpo.gov/fdsys/pkg/FR-2015-10-26/pdf/2015-26594.pdf, last access: 15 April 2019). The European legislation (EU directive 2008/50/EC, 2008) AOT40 metric is measured throughout daytime periods from May to July (growth season) and has a defined target limit of 18 000 µg m−3 h (9000 ppb ⋅ h) and a long-term objective of 6000 µg m−3 h (3000 ppb ⋅ h). A standard of 15 ppm ⋅ h has been defined for the seasonal W126 index, which is averaged over 3 years. These metrics have been used to assess the impact of mitigation strategies (Avnery et al., 2013), the impact of industry on air quality management issues (Vijayaraghavan et al., 2016), and the impact of high O3 levels and temperatures on crops (Tai and Val Martin, 2017).
In this paper, we use a tagged O3 mechanism in the WRF-Chem model to understand the contribution of emitted O3 precursors from different geographical source regions and types on the modelled O3 concentration in several European receptor regions. In Sect. 2 we discuss the details of implementing this tagging technique and describe changes made to both the chemical mechanism and WRF-Chem code. Section 2 also describes the WRF-Chem configuration, simulation design, and input data used in the study. An analysis of the WRF-Chem simulation is presented in Sect. 3, while Sect. 4 summarises our findings.
2.1 Tagging technique
To perform a WRF-Chem model simulation using a tagging approach, several changes must be implemented in the model code to accommodate additional tracers and reactions representing tagged constituents. Butler et al. (2018) describe in detail how the tagging technique was implemented in the Community Earth System Model. The tagging technique used in this study is based on the same approach and uses the same modified version of the MOZART chemical mechanism. Further details on how the chemical mechanism was extended can be found in Butler et al. (2018).
To use the NOx tagging mechanism, a new chemistry option was added in the namelist.input file: chem_opt =113 and through the code. The coupling of the new chemical scheme with microphysics and radiative processes requires several modifications to the code: (1) the first step is to create a new chemistry option. The package mozart_tag_kpp (chemopt ) has been added to ∼/WRFV3/Registry/registry.chem together with new model variables for tagged NOx species (e.g. O3_X_INI, O3_X_STR). For this purpose, the preprocessing software described in Butler et al. (2018) was adapted to produce a new chemical mechanism; (2) the new chemistry package is a KPP option. Therefore, we created a new subdirectory in ∼/WRFV3/chem/KPP/mechanisms/ directory containing the files (*.spc, *.eqn, *.kpp, and *.def), which defined the chemical model species and constants, chemical reactions in KPP format, model description, computer language, precision, and integrator.
The new chemistry option considers a large number of species and reactions; therefore we exceeded hard-coded limits that the KPP chemical preprocessor version 2.1 (Sandu and Sander, 2006) allows. To overcome these limits, we increased MAX_EQN and MAX_SPECIES in the header file gdata.h, located in ∼/WRFV3/chem/KPP/kpp/kpp-2.1/src. Further, we updated the subroutines in the ∼/WRFV3/chem directory to consider the new chemistry package. The modules that we modified are described in Appendix A.
Although WRF-Chem uses the Advanced Research WRF (ARW) dynamic core in this simulation, which conserves mass and scalar mass (Grell et al., 2005), the tagged O3 species are advected independently. Thus, numerical errors associated with the advection scheme led to gradients in the sum of tagged species concentration compared to the “real” concentration; therefore, the relationship between these variables is not conserved. Since the advection scheme fails to reproduce the expected solution (in which the sum of the tagged species concentration at each grid point must be equal to the real concentration), we solve this by fixing all undershoots and/or overshoots assuming that the sum of tagged species mass is proportional to the real concentration. This technique was also applied in Flemming et al. (2015) and Gromov et al. (2010).
Compared to Pfister et al. (2013) and Safieddine et al. (2014), the expanded tagging technique used in this study has the advantage that multiple tags can be defined in each model run.
2.2 Experimental setup
WRF-Chem version 3.7.1 was used for this study to account for the impact of different global and European O3 precursor source regions to several European receptor regions during the April–September 2010 period. A single domain, which covers the area between 32 and 70∘ N and 29∘ W and 57∘ E, was used with 50 km grid spacing and 35 vertically stretched layers from the ground up to 50 hPa. The physics options used for this study include the Morrison double-moment microphysics scheme (Morrison et al., 2009), the Grell–Freitas cumulus parameterisation (Grell and Freitas, 2014), the Rapid Radiative Transfer Model (Iacono et al., 2008) for longwave radiation and Goddard shortwave scheme (Chou and Suarez, 1994), the Yonsei University boundary-layer parameterisation (Hong et al., 2006), and the Monin–Obukhov scheme for the surface layer (Jiménez et al., 2012). The initial and boundary conditions for meteorological fields are taken from the European Centre for Medium-Range Weather Forecasts (ECMWF) reanalysis. Anthropogenic emissions were obtained from the TNO-MACC III emission inventory for Europe (Kuenen et al., 2014). Because the model domain extends beyond the edges of the TNO-MACC III inventory, we used for completion emissions from the HTAP V2 inventory (http://edgar.jrc.ec.europa.eu/htap_v2, last access: 15 June 2018). Biogenic emissions were computed online using the Model of Emissions of Gases and Aerosols from Nature (MEGAN) (Guenther et al., 2006). The biomass burning emissions are based on the Fire INventory from NCAR (FINN) (Wiedinmyer et al., 2011).
For this WRF-Chem simulation, the tagged MOZART chemical mechanism for NOx emissions (Butler et al., 2018) is used to represent the gas-phase chemistry. The photolysis rates were computed using the Fast Tropospheric Ultraviolet and Visible (FTUV) Radiation Model (Tie et al., 2003; Li et al., 2005). The dry deposition was calculated following the Wesely (1989) resistance method, while the wet removal scheme for the tagged MOZART chemistry is based on Neu and Prather (2012).
NOx emitted by several source regions and types is tagged and explicitly tracked using additional tagged reactions and tracers. Thus, we follow the contribution to the total ozone concentration from each specific emission source and type, from both within and outside the European model domain. Table 1 summarises tagged source regions and types that are used in this study. Using a division of source regions within the European model domain, 15 geographical source regions are specified in Table 1 and depicted in Fig. 1. A similar division of European regions has been used by Christensen and Christensen (2007) and Otero et al. (2018) to address the main sources of uncertainty in regional climate simulations, as well as during the AQMEII project (i.e. Struzewska et al., 2015). Except for ALP, the source regions within the European domain are identical to receptor regions. Given the complex topography of the ALP source region, we split this region into two receptor regions: the Po Valley region and the high Alps (regions above 1500 m elevation).
To represent the impact of transported O3 from different regions outside of the domain, we used chemical boundary conditions derived from the extended CAM-Chem version 1.2 global simulations. Butler et al. (2019) used the tagging approach within the CAM-Chem model for several HTAP2 source regions such as ASI (Asia), NAF (north Africa), NAM (North America), OCN (Oceanic sources), RBU (Russia, Belarus, Ukraine), and RST (rest of the world), as well as for several other source types: BIO (biogenic emissions), BMB (biomass burning emissions), LGT (lightning), and STR (stratospheric O3). No overlap of source regions or types is allowed.
The BIO, BMB, LGT, and STR source types are also included in the tagged chemical mechanism used in this simulation, but without including them in the division of source regions; we refer to these sources as “other global source types” from here on. Ozone due to these other global source types can originate both from long-range transport from remote source regions through the lateral model boundaries and from precursor emissions within the European model domain.
For each receptor region, we analyse the impact of the NOx emissions coming from different source regions and types on the total O3 concentration.
2.3 Ozone metrics
Using different metrics to assess the impact of O3, we can determine which precursor sources most highly influence the accumulation of O3 in different receptor regions and thus to provide insights into which type of mitigation measures will be useful for a particular geographic area. These metrics include the mean O3 concentration, the mean of MDA8, the cumulative exposure to mixing ratios above 35 ppb (SOMO35) (Colette et al., 2012), and the 95th percentile for surface O3. Neither the impact of O3 exposure on trees, plants, and ecosystems (W126) (Lapina et al., 2014) nor the AOT40 accumulation metric (the threshold is 40 ppb) was used to assess risk to vegetation from O3 exposure (UNECE, 2010).
The European Air Quality Directive (EU directive 2008/50/EC, 2008) specifies that O3 exposure should remain below a target MDA8 O3 value of 120 µg m−3, which can be exceeded up to 25 d per calendar year averaged over 3 years. The modelled daytime AOT40 (during local daylight hours 08:00–19:00 LT for all times throughout) was calculated according to Eq. (1).
According to European legislation (EU directive 2008/50/EC, 2008), the AOT40 metric is accumulated over the daytime period from May to July (growth season) and it has a defined target limit of 18 000 µg m−3 h (9000 ppb ⋅ h) and a long-term objective of 6000 µg m−3 h (3000 ppb ⋅ h). W126, however, is described according to U.S. EPA regulations (https://www.gpo.gov/fdsys/pkg/FR-2015-10-26/pdf/2015-26594.pdf, last access: 15 June 2018). A standard of 15 ppm ⋅ h is defined for the seasonal W126 index, which is an average over a 3-year period. For this study, the hourly surface O3 tagged outputs for April through September are used to calculate the highest 3-month W126 index values (see Eq. 2):
According to Lefohn et al. (1988), the W126 index includes all hourly O3 values within the specified time range, although a lower weight is given to hourly O3 concentrations below the inflection point of 65 ppb, while values above 90 ppb are weighted with a factor of almost 1. SOMO35 (WHO, 2001) is defined as the sum of the MDA8 O3 with a cut-off of 35 ppb (see Eq. 3). For this metric, the EU air quality directives do not prescribe a limit or a target value.
The contribution of tagged O3 is based on formulations of each metric and is calculated from the model output. In the case of the MDA8 and 95th percentile metrics, we searched for the specific period in which calculated values of total O3 concentration meet the requirements for the formulation of these metrics. Once this is identified, tagged O3 concentrations are extracted for the same period, which can then be used for further analysis. However, the contribution of concentration of tagged O3 to cumulative metrics is slightly different; a large proportion of each tagged species is used to determine total O3, as illustrated below for AOT40 at a specific time period:
Based on their formulation, we grouped metrics into either non-cumulative (mean O3, MDA8, and the 95th percentile) or cumulative (SOMO35, W126, and AOT40) categories. Since the latter metrics have different formulations (including hourly O3 values above a specific threshold) and do not cover the same periods, to facilitate a more direct comparison between findings from multiple O3 metrics, an analysis of the relative contribution of different source regions to total O3 in each receptor region was performed. This was done using averaged values for non-cumulative metrics and 6-month sums for cumulative metrics (SOMO35, AOT40) useful for evaluating effects on crops (cumulated over the May–July period) as well as a maximum of 3-month sums for every consecutive 3-month period determined using the W126 index.
Our discussion of the results of the model is focused on the April–September 2010 period. We first briefly evaluate the ability of WRF-Chem to reproduce meteorological parameters using measurements from the Global Weather Observation (GWO) dataset provided by the British Atmospheric Data Centre (BADC) and observed O3 concentrations using the measurements included in AirBase, a European air quality database (EEA, 2017b). We then provided a more detailed analysis of the contribution of different source regions and types to MDA8 values describing total O3 for the analysed period.
3.1 Evaluation of meteorology and chemistry
Since the accurate simulation of meteorological parameters represents a key factor affecting the concentrations of trace gases, we briefly compare the modelled mean sea level pressure (MSLP), 2 m temperature (T2M), and 10 m wind speed (WS10M) and direction (WD10M) variables against GWO measurement. Predicted model variables were then evaluated against observations using statistical scores that include normalised mean bias (NMB) and the correlation factor between simulated and measured values (r).
An extensive evaluation of WRF-Chem using the MOZART chemical mechanism to predict long-term meteorological data and O3 levels has been presented previously (Mar et al., 2016). The main differences between the set-up used in this study and the model described by Mar et al. (2016) include differences between the versions of the model used (3.7.1 vs. 3.5.1, respectively), horizontal resolutions (50 km×50 km vs. 45 km×45 km, respectively), microphysics (Morrison vs. Lin, respectively) and cumulus schemes (Grell–Freitas vs. Grell 3-D, respectively), simulation years (2010 vs. 2007, respectively), anthropogenic emissions inventory (TNO-MACC III vs. TNO-MACC II, respectively), and chemical input and boundary conditions (extended CAM-Chem version 1.2 with MOZART-4 vs. MOZART-4/GEOS-5 simulations found at https://www.acom.ucar.edu/wrf-chem/mozart.shtml, last access: 15 June 2018, respectively).
Due to the coarse resolution of our domain, the air parcel dynamics associated with the complex topography of mountainous areas was not properly reproduced. Thus, we assessed the ability of the model to reproduce the meteorological variables using only those sites located below 1500 m above sea level. MSLP data were well reproduced over the entire period (NMB =0 % and r=0.98). The model predicted T2M values well (r=0.91); however temperature was underestimated by 3 % (see Table 2). WS10M was also fairly well reproduced in terms of both spatial and temporal variability (NMB =8 %, r=0.63). Yet, WD10M data could not be predicted as well as other meteorological variables (NMB =13 %, r=0.47); behaviour could be related to the existence of unresolved topography features (Jiménez and Dudhia, 2012). However, the model performance is similar to Mar et al. (2016) and Tuccella et al. (2012).
Table 2Observed mean and simulation summary statistics for meteorological parameters. The normalised mean bias (NMB) and correlation coefficient (R) are calculated between simulated and observed meteorological observation from GWO during the April–September 2010 period.
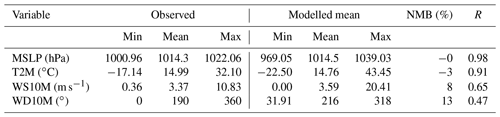
We also compared modelled MDA8 O3 concentrations with observations provided by the publicly available AirBase dataset. The relatively coarse resolution of the domain may not be representative of changes in local emissions when the measurements are taken from urban areas; therefore, to aid in the analysis, we used only those stations characterised as rural. As can be seen in Table 3, evaluation of the model over the entire period revealed that the model performs quite well with respect to the prediction of concentration and temporal evolution. Mar et al. (2016) reported a mean bias (MB) value of 15.85 µg m−3 and an NMB of 17 % for the June–August 2007 period when the MOZART mechanism was used to assess the chemical performances of the model. For the same time period, we obtained an MB value of −5.92 µg m−3 and an NMB value of −6.3 %. Tuccella et al. (2012) reported an annual MB of −1.4 µg m−3 when the RADM2 chemical mechanism was used to simulate a period throughout 2007. Month-to-month analysis (Table 3) shows that the model reproduces the O3 concentration well compared to Mar et al. (2016) and Tuccella et al. (2012). Even though the performance of the model in terms of temporal variation is relatively good (r values fall between 0.58 and 0.71), it mostly underestimated concentrations of O3, except in September, when the model overestimated concentrations (NMB =4.6 %). Errors of the model may be explained by a wide range of uncertainties related to modelled physical and chemical processes such as grid resolution, vertical and horizontal transport, boundary-layer mixing, emission inventory, chemistry and photolysis rates, dry deposition, wet scavenging, etc. It is also possible that uncertainties in measurements contribute to observed errors. Since the focus of this study is on the contribution of different sources of precursors to the total tropospheric O3 concentration of a particular area, a more thorough analysis of the ability of the model to reproduce the observed meteorological variables is beyond the scope of this paper.
Table 3Observed mean and simulation summary statistics for MDA8 O3 concentrations (µg m−3) at rural background sites. The normalised mean bias (NMB) and correlation coefficient (R) are calculated between simulated and observed O3 concentrations from the AirBase dataset during the April–September 2010 period.
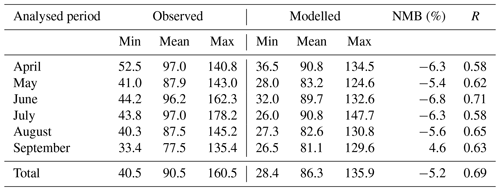
3.2 Contribution of tagged precursor sources to the MDA8 O3 mixing ratios
Figure 2 shows the spatial distributions of simulated monthly mean MDA8 values from tagged O3 source regions and other global source types throughout late spring in 2010. The receptor regions shown were mainly influenced by the overseas combination of NAM, ASI, OCN, and RST sources that combined contribute from 23 % in the Po Valley to up to 53.6 % in the UKI region (see Table S1 in the Supplement). O3 from RST (a 7.5 %–15 % contribution) is the main source from overseas. O3 from shipping NOx emissions advected through the model boundaries combined with O3 produced from shipping NOx emissions in the Atlantic Ocean mostly affects Atlantic coastal countries (up to a 16.1 % contribution in the UKI region), yet a small contribution of ∼4 %–5 % was also observed within inland regions. Long-range transport of O3 from Asia and North America contributes significantly to total observed O3 in Europe, accounting for 9.6 % of the total observed O3 in ITA and up to ∼22 % in UKI and SCA. After intercontinental transport, O3 produced within Europe is an important source of O3 in receptor regions, followed by O3 coming from other global source types (LGT, BIO, and BMB). In general, for the April–May 2010 period, the contribution from the local sources to the total MDA8 O3 mixing ratio in receptor regions falls within a range from 8.5 % (SCA) to 21 % (RBU) (see Table S1). Emissions from local sources do not only affect local O3 mixing ratios, but also impact O3 levels of bordering countries due to strong horizontal pollution transport. In all receptor regions, local anthropogenic sources have a lower contribution to MDA8 O3 mixing ratios than the sum of O3 due to anthropogenic sources in other European source regions and long-range transport of ozone from intercontinental source regions. The contribution of intercontinental transport to the total MDA8 O3 mixing ratio in Europe is consistent with previously reported results, i.e. Fiore et al. (2009) and Karamchandani et al. (2017), while this study allows us to identify which anthropogenic sources exert a strong influence on MDA8 O3 predicted in different regions. Using observations, Danielsen (1968) and Thouret et al. (2006) showed that the transport of O3 from the stratosphere also contributes to tropospheric O3. Here, stratospheric O3 contributes up to 7 ppb (12.5 % in SCA) to the total MDA8 O3 mixing ratio, which is a finding similar to that reported by Derwent et al. (2015). A similar tagged system for predicting O3 levels, using the CAM-Chem model (Butler et al., 2018), has also shown that stratospheric O3 significantly contributes to the total tropospheric O3 mixing ratio. The MOZART chemical mechanism used in this study does not explicitly treat stratospheric chemistry; thus surface stratospheric O3 could be attributed to the vertical and horizontal transport of stratospheric O3 and stratospheric tagged precursor species concentrations coming from the CAM-Chem extended model that enters the domain through lateral boundaries.
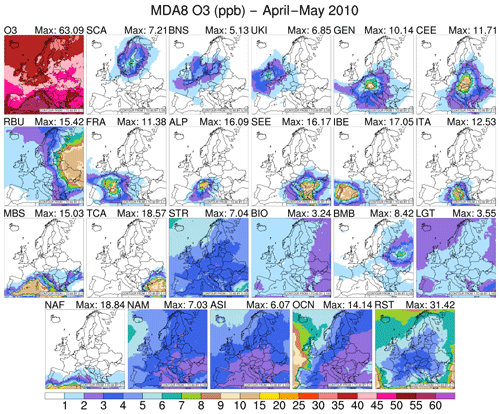
Figure 2Contribution to MDA8 O3 (ppb) of each O3 source region and global source type for the April–May 2010 period.
During June–August 2010, western Europe was mostly influenced by a high-pressure system centred over the Atlantic (see Fig. S1 in the Supplement). In the upper troposphere, a ridge influenced the vertical atmospheric structure, especially over southern Europe. Therefore, these “usual summer conditions” favoured the intrusion of warm air coming from Africa and the Arabian peninsula and led to a warm and dry climate characterised by subsidence, stability, clear skies, and high-intensity solar radiation. Hence, the photochemical formation of O3 was enhanced and influenced the stronger contribution of local emissions to the total mixing ratio compared to the previous period examined. Figure 3 depicts the average MDA8 O3 for June–August 2010. For most regions, we notice that levels of O3 produced from local sources from June to August compared with April–May were enhanced (Fig. 2). Local sources can contribute to more than 20 % of the mean MDA8 O3 mixing ratio (from 14.6 % in SCA to 35.7 % in the Po Valley; see Table S1). This shows that local sources play a strong role in the formation of O3 throughout the June–August period, as has been previously shown by Jiménez et al. (2006) and Querol et al. (2018). Compared with late spring, the relative contribution of overseas sources decreased in summer, varying from 10.9 % in the Po Valley receptor region to 44.8 % in the UKI region in the month of July (Figs. 2 and 3; Table S1). We noticed the spread of O3 produced from European anthropogenic precursors over bordering regions compared with late spring 2010 (Figs. 2 and 3). The increase in average temperature combined with stable atmospheric conditions led to an enhancement of the biogenic NO emitted into the atmosphere, especially in southeastern and eastern Europe; thus, the BIO global source type contributes up to ∼9 ppb (13.2 % of MDA8 O3) in the RBU receptor region (see Fig. 3). The vegetation fires that took place across Russia in July and August (Gilbert, 2010; Huijnen et al., 2012) as well as in Portugal and Spain (European Commission, 2011) lead to increases in the contribution of O3 coming from BMB of up to 29 ppb (16 %) in the RBU receptor region and up to 8.5 ppb (2.3 %) in the IBE receptor region. BMB emissions contribute domain-wide more than 3 % (Po Valley), with the greatest impacts modelled over RBU, IBE, SEE, SCA, and TCA. The enhanced photochemical activity during summer combined with the weakening of stratospheric–tropospheric exchange reduce the influence of stratospheric O3 from a domain-wide mean MDA8 O3 mixing ratio of 4.4 ppb in the spring to 1.3 ppb in the summer (Figs. 2 and 3).
The decrease in photochemical activity in September 2010 is reflected in decreases in total O3 mixing ratios compared with the summer of the same year as well as in a reduction associated with the local source contribution to the total O3 mixing ratio (Fig. 4). Thus, only in IBE, TCA, FRA, Po Valley, the high Alps, and RBU regions was contribution of local sources to total MDA8 O3 higher than 20 % (Table S1). On the other hand, we noticed an increase in O3 coming from anthropogenic overseas sources and from lightning in autumn, stressing that seasonal variations exist within the outflow from other continents. There is also variation in the lifetime of O3, which is shortest during the summer as a result of enhanced photolytic activity.
Although we have seen that long-range transport plays a major role in total O3 mixing ratios, the tagging technique helps to gain more insight into which region of the world dominates these mixing ratios in spring or autumn. In early autumn, the western European receptor regions exhibit a slight increase of 1.6 % in O3 mixing ratios coming from North America compared with spring, while the contribution of O3 mixing ratios coming from other overseas sources decreases. This could be linked to the prevailing westerly wind and the synoptic conditions seen during the first period of September, when the Azores High extended far to the east and north (Fig. S1). This phenomenon creates conditions that are conducive to the transatlantic transport of American pollution in the eastern direction. For example, in autumn periods within the RBU receptor region, North American and oceanic sources account for up to 14.6 % in spring and 11.4 % in autumn of the MDA8 O3 mixing ratios.
Apart from local and other global source types, NOx emissions from shipping activities in the Atlantic Ocean combined with the oceanic O3 from boundary conditions are an important source of O3 that explains up to 16 % in late spring, 21 % in summer, and 12 % in early autumn of the MDA8 O3 mixing ratio in the UKI, IBE, FRA, GEN, CEE, and SCA regions. Butler et al. (2018) showed that O3 from oceanic sources reaches a minimum level in the North Atlantic Ocean during the summer, yet this study shows that in the UKI, IBE, FRA, GEN, CEE, and SCA receptor regions the oceanic O3 contribution peaks in the summer. This implies that the nearby shipping emissions have a greater impact on oceanic bordering countries rather than oceanic O3 from boundary conditions. Furthermore, the NOx emissions from shipping activities in the Mediterranean and Black seas account for up to 14 % in late spring, 19 % in summer, and 11 % in early autumn of the MDA8 O3 mixing ratio predicted in the receptor regions situated along the shore of the Mediterranean Sea, such as IBE, ITA, SEE, and FRA.
Our model results have shown that the highest MDA8 O3 mixing ratios are predicted to occur over the Mediterranean Basin. This is due to the presence of favourable conditions for O3 formation including the presence of small deposition sinks and intense photochemistry (Figs. 2–4). Several studies, such as Safieddine et al. (2014), Tagaris et al. (2017), Mertens et al. (2018), Querol et al. (2018) and the references therein, have used source attribution methods to establish the origin of tropospheric O3 observed over the Mediterranean Basin. The tagging technique used here shows that the O3 from shipping activities in the Mediterranean and Black seas (MBS) explains, on average, 15 % in late spring, 20 % in summer, and 12 % in early autumn of total MDA8 O3 predicted to accumulate within the MBS receptor region. These findings are similar to those of Aksoyoglu et al. (2016) that showed these emissions accounted for 10 %–20 % of the mean O3 in the Mediterranean in the summer of 2006. Moreover, Tagaris et al. (2017) have shown that shipping emissions explain up to 30 % of the MDA8 O3 simulated for July 2006 over the Mediterranean Sea. This study has shown that the shipping activities likely accounted for up to 35 % of the MDA8 O3 near the Strait of Gibraltar (see Fig. 5) during the April–September 2010 period. Shipping emissions contribute most highly to total O3 in the western basin of the Mediterranean Sea. Aside from shipping activities, the other European source regions have a localised contribution to total MDA8 O3 predicted in the Mediterranean Sea. Thus, ITA, ALP, and GEN source regions contribute mostly to the central basin; IBE and FRA are the main contributors in the western basin and SEE and TCA predominantly contribute to the eastern basin. Natural sources contribute on average up to 10 % of MDA8 O3 in the western basin and up to approximately 25 % of MDA8 O3 in the eastern basin. The long range of O3 transport contributes up to 45 % along the north African shore and it exhibits a zonal pattern, with low mixing ratios occurring in the north and high mixing ratios occurring south of the Mediterranean Sea, a trend mostly due to O3 mixing ratios from NAF and RST sources.
3.3 Tagged ozone precursor contributions to exceedances of MDA8 target values – case study
As previously mentioned, the European Air Quality Directive (EU directive 2008/50/EC, 2008) has defined a target value of 120 µg m−3 for the MDA8 O3 concentration, which can be exceeded up to 25 d per calendar year (over a 3-year span). In the following, we refer to values that surpass 120 µg m−3 as exceedances and values below 120 µg m−3 as non-exceedances. Figure S2 shows the spatial distribution of the number of exceedances observed and calculated throughout the April–September 2010 period for the AirBase rural stations. The observed MDA8 O3 exceeds the target limits locally in Po Valley, Austria, and Germany; in coastal areas of Portugal, Spain, France, and Italy; and inland areas of Poland and Slovakia. However, the modelled exceedances do not exhibit the same spatial pattern or intensity as observed values. Our use of tags allows for the identification of main source contributors to exceedances of modelled MDA8 O3. Given the high number of stations that measure O3, for simplicity, we will discuss the source contribution to the MDA8 O3 exceedances only for the Po Valley, high Alps, and GEN receptor regions.
Figure 6 exhibits the contribution of each tagged source and type to modelled and to observed MDA8 O3 values. Samples were, in all cases, taken at the location of the measurement stations, throughout the April–September 2010 period. Figure 6 shows the average conditions during the exceedance of the MDA8 O3 target value, and also, at times, occurred when the target value was not exceeded. To perform the source attribution for the observed values, we have scaled these values proportionally by the relative concentrations of each tagged O3 tracer in our model output.
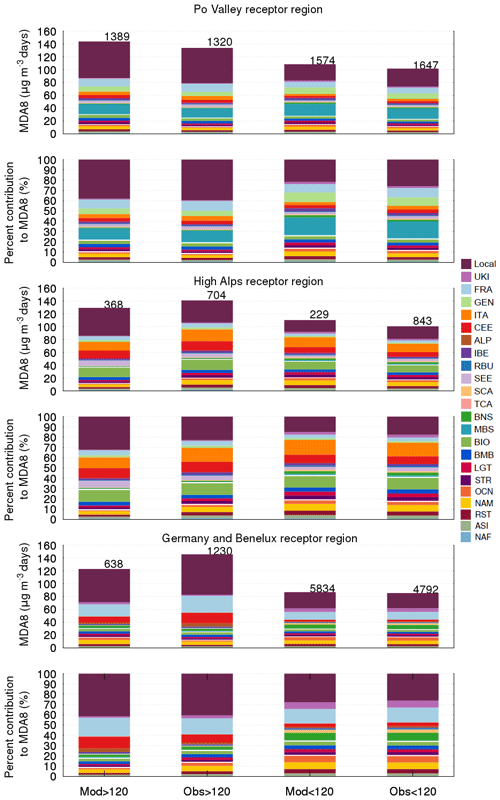
Figure 6Mean modelled and observed MDA8 O3 mixing ratio filtered by a threshold of 120 µg m−3 for the Po Valley, (top panel) high Alps (third panel from top), and GEN (fifth panel from top) and percent contribution to MDA8 O3 from different emissions sources and types for the Po Valley (second panel), high Alps (fourth panel) and GEN (bottom panel) during the April–September 2010 period. In each case the contributions of tagged sources to the total O3 are shown. The tagged contributions of local and other European sources, HTAP2 source regions, and other global source types to observed O3 are obtained by scaling the observed O3 by the relative contributions of these tagged sources to modelled O3. The total number of exceedances (and non-exceedances) of the MDA8 O3 target value is indicated at the top of each column.
The relative contribution of emissions from different source regions to modelled and to observed MDA8 O3 values, after being scaled to account for the contribution of modelled sources of O3 types, is generally similar for the Po Valley and GEN receptor regions (see Fig. 6). In the Po Valley, we can pinpoint the main remote contributor as being MBS (see Fig. 6), followed by GEN and FRA, suggesting a dominant westerly and northerly air flow. The recirculation of air masses in the Gulf of Genoa could accentuate the sea breeze, and therefore more O3 coming from NOx associated with shipping activities in the Mediterranean will be transported to the coastal and inland stations.
The high Alps receptor region is less influenced by ALP emissions than the Po Valley, and it is more influenced by remote sources (see Fig. 6). The increased contribution of O3 from CEE, ITA, and FRA to both exceedance and non-exceedance days in the high Alps receptor region compared with the Po Valley receptor region highlights the impact of the transboundary transport of O3 and its precursors. Furthermore, the contribution of stratospheric as well as long-range sources was generally 6 % higher in this receptor region than in the Po Valley receptor region.
In GEN, the main remote source regions are FRA and CEE during the exceedance days and FRA and UKI during non-exceedance days (Fig. 6). Opposite to the Po Valley, in GEN the model predicts fewer MDA8 O3 exceedance days. Comparing the source contribution to both modelled and observed exceedance days, we noticed that the model underestimates O3 concentrations associated with long-range transport and natural sources. Further, the model predicted higher levels of O3 from CEE and FRA than observed. Underestimation of long-range-transported O3 into the GEN region in our model could be explained by the fact that the number of modelled MDA8 O3 exceedances in GEN is half of the observed number of exceedances (Fig. 6).
This kind of analysis can be applied to improve our knowledge of the origin of O3 precursors and their contribution to MDA8 O3 health metrics. Hence, by using this tagging technique, policymakers can identify future actions required to control the NOx emissions at local and regional levels.
3.4 Tagged ozone precursor contributions to regulatory ozone metrics
In this section, we discuss the contribution of O3 mixing ratios from diverse emissions sources and types to several metrics that quantify the O3 exposures of humans and ecosystems. From modelled hourly mixing ratios of tagged O3 sources and other global types, we have calculated different O3 metrics, including non-cumulative (mean, MDA8, and the 95th percentile O3) and cumulative (SOMO35, W126, and AOT40) metrics. We have chosen not to analyse the performance of the calculated cumulative metrics in comparison with measured values, as was done in previous work by Tong et al. (2009). Their work showed that the poor performance of the cumulative metrics is closely related to the sensitivity of these metrics to the threshold values or weighting factors.
Figure 7 and Table S2 include the percentage of the contribution of different sources of emissions and other global types to total O3 as calculated using health and vegetation metrics. The non-cumulative O3 metrics employed in this study have displayed similar patterns for most of the receptor regions. The contribution of local and European sources to the total O3 mixing ratios has been low when we applied to mean O3 metric and high when using the 95th percentile metric. These findings emphasise the importance of O3 produced by local and neighbouring sources to the high end of the O3 mixing ratio distribution.
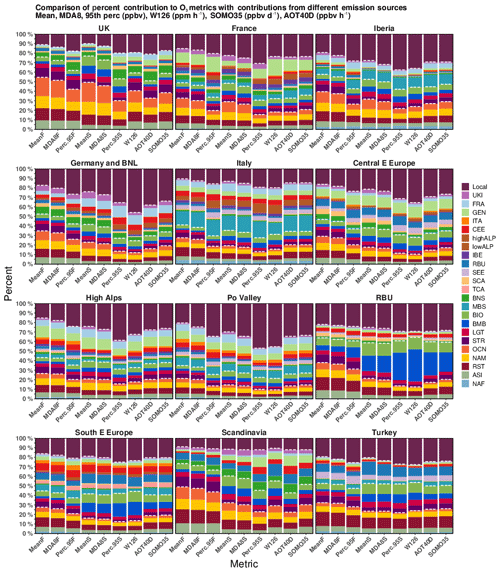
Figure 7Comparison of percent contribution of local and other European sources, HTAP2 source regions, and other global source types to different O3 metrics. The metrics analysed are mean, MDA8, and 95th percentile (ppb) for the early “F” and late “S” simulation periods, W126 (ppm ⋅ h), SOMO35, and AOT40 (ppb ⋅ h). The white dashed lines in each panel separate different categories (intercontinental transport, other global source types, and local and other European sources).
Splitting the non-cumulative metrics into early (April–June) and late (July–September) simulation periods clearly illustrates that the European receptor regions are more prone to be influenced by intercontinental transport during the early period than the late period. The contribution of inter-continentally transported O3 to mean O3 values in different receptor regions is higher during the early period and it spans between 22.8 % and 54.3 % of total O3. In the late period it accounts for between 16 % and 48.9 % of total O3 (see Fig. 7 and Table S2). Since O3 associated with intercontinental transport comes, in this case, solely from boundary conditions, errors in boundary conditions affect the predicted mixing ratio of various chemical species and, consequently, the contribution of overseas sources of O3 to levels observed in Europe O3 (Tang et al., 2007; Giordano et al., 2015; Im et al., 2018).
The shorter lifespan of O3 over remote ocean regions throughout the warm season, combined with synoptic conditions, has led to decreased levels of inter-continentally transported O3 to Europe. Thus, for most receptor regions, the O3 coming from Asia and the rest of the world was reduced by more than half when compared with the cold period. The O3 mixing ratio from the stratosphere is, in general, 2.5 times higher in the cold season than in the warm season, which is consistent with the findings of a study by Butler et al. (2018), which showed that the stratospheric O3 mixing ratio varies with altitude and its lifetime is influenced by season and latitude. The tagging technique also helps to quantify the impact of biogenic and biomass burning emissions of NOx on tropospheric O3. The impact of biogenic NOx emissions on mean O3 mixing ratios is between 3.3 % in the Po Valley and 5.9 % in TCA in the early season, while during the late season it is between 5.4 % in the Po Valley and 13.4 % in RBU. The biomass burning emissions account for variable percentages of mean O3 mixing ratios. These span between 1.6 % in ITA and 5.3 % in RBU during the early season and between 3.8 % in the Po Valley and 16.3 % in RBU during the late season. Natural sources do not usually vary greatly when different non-cumulative metrics are applied. An exception would be for the biomass burning emissions in RBU during the late season. Thus, BMB in RBU contributes to 16.3 %, 17.6 %, and 28.8 % of the mean, MDA8, and 95th percentile, respectively.
Even though the SOMO35 and AOT40 metrics are not accumulated over the same period (SOMO35 is accumulated over the entire simulated period, and the AOT40 metric is accumulated over the May–July period) and do not use same input data (daily MDA8 O3 for SOMO35 vs. daytime O3 mixing ratios for AOT40), since they are based on threshold exceedances and are designed to measure exposure to high O3 levels of humans (SOMO35) and vegetation (AOT40), there is a way to directly compare data from each metric type. As shown in Fig. 7 and Table S2, the contribution of different sources of emissions and types as a proportion of total SOMO35 and AOT40 metrics is similar for most of the European receptor regions. Their spatial distribution (not shown) is also comparable, with minimum values over the UK, NW Europe, and Scandinavia and maximum values over Italy, the Alps, south of Spain, east of Turkey, and in the metropolitan area of Moscow, Russia. These results are consistent with previous studies performed by Aksoyoglu et al. (2014) and Anav et al. (2016). The overseas sources contribute similarly to SOMO35 and AOT40 indexes (usually less than 30 %) for most of the receptor regions used in this study. However, in UKI the overseas sources account for 32 % of AOT40 and 38 % of SOMO35, and in SCA they contribute to ∼22 % of AOT40 and 30 % of SOMO35. This suggests that these metrics are more sensitive with respect to the O3 mixing ratios from remote sources in areas having a low level of O3 pollution. In the RBU receptor region, these indicators are sensitive to O3 coming from biomass burning emissions (20 % of SOMO35 and 24 % of AOT40), whereas for the remaining receptor regions the contribution of natural sources to SOMO35 and AOT40 is similar. Local sources account for a range of ∼12 % (SCA) to ∼38 % (GEN) of these metrics. These data highlight the occurrence of increased O3 production from local sources in comparison with northern European countries as well as large emissions of NOx in the GEN source region. Since the difference between AOT40 and SOMO35 is only a few percentage points, regardless of the receptor region, we were able to conclude that they behave similarly, according to thresholds used to define these metrics.
The tagging method allows a better understanding of the main precursor sources responsible for exceedances of regulatory O3 metrics. This information can help to inform further modelling studies aimed at investigating the effects of emission reduction strategies and ultimately inform air quality policy. For example, in the Po Valley receptor region, the modelled AOT40 is up to 3.4 times higher than the target limit given by EU legislation (on average 31 218 ppb ⋅ h). The observed and calculated AOT40 values depicted in Fig. S3 exhibit the exceedance of target limits in the Po Valley. O3 coming from local sources can explain 35.0 % of this value (an average of 10 909 ppb ⋅ h). After local sources, the main European anthropogenic sources contributing to high AOT40 values in the Po Valley region are from FRA (6.6 %), GEN (7 %), and MBS (8.8 %) (Table S2). Generally, the O3 mixing ratio and its precursors transported from other anthropogenic European sources into the Po Valley receptor regions account for ∼39.5 %, while natural sources account for ∼12.3 % and long-range transport accounts for ∼13.4 % of the remaining AOT40 mixing ratios. Thus, to reach at least the target limit in the Po Valley receptor region, considerable emission reductions will still be needed, not only on a local scale but also on the European scale, especially within the MBS, GEN, and FRA source regions.
Figure 7 also shows the percentage that different types of emissions and emission regions contribute to the W126 index. Interestingly, for most of the receptor regions, local NOx anthropogenic emissions cause the largest response in W126 values compared with the other cumulative metrics used in this study. Thus, local NOx explains from 10.9 % (0.1 ppm ⋅ h) in SCA to more than 40 % of W126 in GEN (45.9 %; 2.48 ppm ⋅ h) and the Po Valley (45.4 %; 8.7 ppm ⋅ h) of W126 index values calculated for each region. The effect of European transported plumes is also enhanced when using the W126 index compared with the other metrics for most of the downwind receptor regions. This behaviour is related to how these metrics have been defined. Due to its sigmoidal weighted formulation, as discussed in Westenbarger and Frisvold (1995) and Lapina et al. (2014), W126 includes all daytime values rather than O3 levels above a certain threshold, as is done using SOMO35 and AOT40; therefore lower weighting factors of less than 0.5 are given to low O3 values and weighting factors above 0.5 are given to O3 values situated above the inflection point of 67 ppb.
The modelled mean AOT40 and W126 values in the Po Valley receptor region exceeded standards (26 368 ppb ⋅ h for AOT40 and 28.9 ppm ⋅ h for W126) during the May–July 2010 period, and, as shown in Fig. 7 and Table S2, local sources are an important contributor to these metrics. To better understand why the W126 index is mainly influenced by local sources compared with the other cumulative metrics, we thoroughly compared AOT40 and W126 values for the Po Valley receptor region. As shown in Fig. 8, a temporal series of hourly daylight values for mean O3, W126, and AOT40 values averaged over the Po Valley receptor region are given. Since the W126 unit is ppm ⋅ h, a more direct comparison with the W126 index would require values to be expressed in ppb ⋅ h. Further, all metrics showed a similar level of temporal variation in which they peaked in the first half of July. Also, whenever the averaged O3 mixing ratio was lower than 60 ppb (Fig. 8a), the W126 value was lower than AOT40 (Fig. 8d). This way of acting was most probably due to the weighting factor being less than 0.3, and above this mixing ratio W126 tends to be higher than AOT40. This behaviour is closely linked to the definition of these metrics. If the O3 mixing ratio is less than 40 ppb, W126 has a weighting factor lower than 0.03, while AOT40 has a weighting factor of 0. Above this threshold, AOT40 has a weighting factor of 1, while in the case of W126 only O3 values higher than 100 ppb have a weighting factor of 1. Due to the way these metrics are defined, predicted O3 values in each grid cell are taken into account for the W126 but may not be taken into account for the AOT40 index.
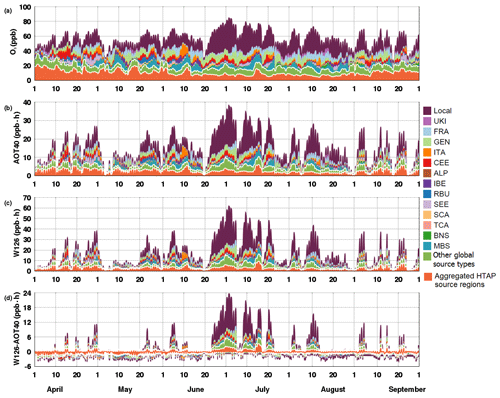
Figure 8April–September 2010 time series of daytime (a) hourly O3 (ppb), (b) hourly AOT40 index (ppb ⋅ h), (c) hourly W126 index (ppb ⋅ h), and (d) differences between W126 and AOT40 indexes (ppb ⋅ h) averaged over the Po Valley receptor region. The colour bars indicate the O3 source categories – aggregated HTAP2 regions (ASI, NAM, NAF, OCN, and RST), the other global source types (STR, LGT, BMB, and BIO), and the European source regions. The white dashed lines in each panel separate different categories (intercontinental transport, other global source types, and local and other European sources).
In addition, visual analysis of the time series also revealed that when the O3 mixing ratios from local sources are ∼20 ppb, these mixing ratios have a higher contribution to W126 than AOT40. To better understand this observation, we have further analysed the relationship between mean O3 values from ALP sources (O3-ALP) and the percent contribution of these O3 tracers to mean O3, W126, and AOT40 metrics. Figure 9 shows scatter plots for O3-ALP that relate the contributions of these mixing ratios to mean O3, W126, and AOT40. In addition, the linear regressions of Y vs. X () using all datasets have been applied. We saw that in general, high mean O3-ALP mixing ratios have a higher contribution to W126 than to AOT40; this was also confirmed by the linear regression between O3-ALP and W126 that yields a slope of 1.52 compared to a slope of 1.36 obtained when the linear regression was applied to AOT40 vs. O3-ALP. Averaged O3-ALP and mean O3 as well as O3-ALP and W126 were highly correlated (r=0.96 and r=0.93, respectively), while O3-ALP and AOT40 are correlated more loosely (0.88). The high level of correlation between O3-ALP and both mean O3 and W126 could be related to the fact that these metrics account for all modelled values, whilst AOT40 considers only O3 values above 40 ppb.
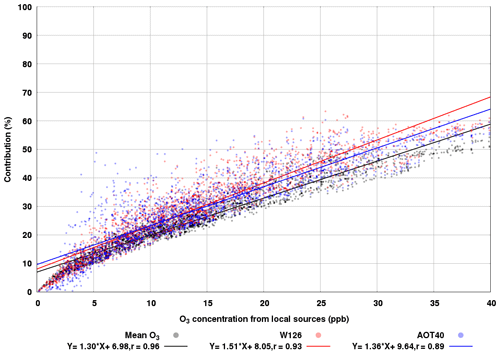
Figure 9Scatter plots showing the ozone concentration from local sources versus the contribution to mean O3 (black dots), W126 (red dots), and AOT40 (blue dots). The solid lines are the lines of best fit.
Extending this analysis to all receptor regions, we can explain why the W126 index is more sensitive to O3 coming from local sources compared with the other cumulative metrics. In addition, W126 accentuates the contribution of BIO and BMB in RBU, TCA, and SEE, most likely because the metric includes all daytime values and not just those above a certain threshold. Thus, the use of W126 highlights the considerable impacts of BIO and BMB emissions on total O3 mixing ratios throughout the summer and from burning vegetation that ultimately influence the extent to which O3 causes damage to vegetation.
We have seen that the contribution of NOx to total O3 varies depending on metrics and regions considered. Hence, the tagging method could help design different emission control strategies in specific source regions depending on which impacts need to be reduced in specific receptor regions.
Here, we implemented a new chemical mechanism within the WRF-Chem model to account for source attribution of O3 from NOx. We investigated the origin of surface O3 using the tagging technique from April to September 2010, as well as the contribution of different sources to O3 metrics and their exceedance events.
Using tagged simulation from WRF-Chem, we show the spatial distribution of simulated monthly mean MDA8 from tagged O3 source regions and other global types throughout late spring, summer, and early autumn of 2010. The contribution of different sources to O3 production varies with season. We have identified intercontinentally transported O3 as an important contributor to the total O3 mixing ratio, especially in the late spring and early autumn. During summer, however, the O3 production is dominated by national and intra-European sources. We have also identified shipping activities in the Mediterranean Sea as an important source of O3 for the IBE, ITA, SEE, and FRA peripheral maritime receptor regions. We also analysed the main sources of MDA8 O3 over the Mediterranean Basin and we have identified the main factors that contribute to MDA8 O3 mixing ratios to the greatest degree. These were mainly shipping activities and the localised contribution from the bordering countries.
To better understand the origin of MDA8 O3 exceedances, we compared modelled and observed values of MDA8 O3 concentration in the Po Valley, high Alps, Germany, and Benelux receptor regions. Throughout days exceeding the recommended thresholds of 120 µg, the contribution from local sources was ∼41 %, 34 %, and 38 % of modelled MDA8 O3 for the Po Valley, high Alps, and GEN, respectively. Throughout days not exceeding recommended thresholds, local emissions explain ∼27 %, 16 %, and 23 % of modelled MDA8 O3 for the Po Valley, high Alps, and GEN, respectively. Moreover, this tagging approach revealed that the main remote sources of MDA8 O3 are MBS, GEN, and FRA for the Po Valley receptor region and are FRA, CEE, and UKI for the Germany and Benelux receptor region. In addition, these analyses identified a persistently high contribution of transboundary sources to background O3 concentration in the high Alps receptor region. Furthermore, by showing that the contribution of precursor sources to modelled O3 target value exceedances in the GEN region is systematically different from the contribution of precursor sources to modelled O3 when exceedances are observed but not modelled, we have identified a possible reason (underestimation of long-range transport) for the poor performance of our model with respect to reproducing the observed number of O3 target value exceedances in the GEN region.
Through comparisons with different O3 metrics, we quantified the impact of local vs. non-local NOx on O3 production for each European receptor region. The comparison between mean, MDA8, and 95th percentile O3 metrics accentuates the importance of large contributions from different NOx sources to the high end of the O3 distribution. By analysing these metrics for two periods (April–June and July–September), we can clearly distinguish the contribution of different NOx to total O3 mixing ratios in each region and throughout different times of the year. When we compare the cumulative metrics, we noticed that the SOMO35 and AOT40 indexes exhibit rather similar behaviour. Considering that these metrics are not calculated over the same period nor do they use the same input data, the similar behaviour is likely due to the similar threshold values applied to define these metrics.
The use of the W126 index accentuates the importance of local emissions. To confirm this, we investigated the behaviour of modelled mean AOT40 and W126 values in the Po Valley receptor region. We noticed that when the local sources contribute to more than 20 ppb of the O3 mixing ratios, these mixing ratios have a higher contribution to W126 than they do to AOT40, and we determined that the difference was mostly due to the definition of W126, which takes into account all O3 values, not only those that are above a certain threshold.
Overall, this study has identified local and remote factors that contribute to the MDA8 O3 mixing ratio during several periods as well as within different O3 metrics. Furthermore, the method applied here could be used to design improved emission control strategies depending on which impacts need to be reduced.
The WRF-Chem model is publicly available at http://www2.mmm.ucar.edu/wrf/users/download/get_source.html (last access: 15 June 2018). The modifications introduced and described in Sect. 2 are available online via Zenodo at https://doi.org/10.5281/zenodo.3501963 (Lupascu and Butler, 2019). The model data can be provided upon request to the corresponding author.
-
chemics_init.F;
-
module_input_chem_data.F;
-
module_plumerise1.F and module_add_emiss_burn.F to account for the source attribution of biomass burning emissions to O3 concentration;
-
module_emissions_anthropogenics.F to account for the impact of anthropogenic emissions on O3 concentration;
-
module_bioemi_megan2.F and module_data_mgn2mech.F to see the impact of biogenic emissions on O3 concentration;
-
module_lightning_nox_driver.F for lightning-generated nitrogen oxides;
-
Dry and wet deposition of tagged trace gases are treated by module_dep_simple.F and module_mozcart_wetscav.F; thus all tagged species have the same dry deposition velocities and wet removal rates with the corresponding non-tagged species;
-
module_ftuv_driver.F to consider the photolytical reaction of the new packages;
-
emissions_driver.F;
-
chem_driver.F.
The supplement related to this article is available online at: https://doi.org/10.5194/acp-19-14535-2019-supplement.
AL and TB designed the research. AL adapted the automatic mechanism-rewriting and code-generation tools and implemented them into the WRF-Chem source code. AL performed the model runs and subsequent analysis. AL wrote the paper with contributions from TB.
The authors declare that they have no conflict of interest.
The authors would like to thank Kathleen Mar for helping with the emissions preprocessing as well as to Jane Coates for her help with some of the plots.
This work was hosted by IASS Potsdam, with financial support provided by the Federal Ministry of Education and Research of Germany (FBMBF) and the Ministry for Science, Research and Culture of the state of Brandenburg (MWFK).
This paper was edited by Maria Kanakidou and reviewed by three anonymous referees.
Agathokleous, E., Kitao, M., and Kinose, Y. .: A Review Study on Ozone Phytotoxicity Metrics for Setting Critical Levels in Asia, Asian Journal of Atmospheric Environment, 12, 1–16, https://doi.org/10.5572/ajae.2018.12.1.001, 2018. a
Aksoyoglu, S., Keller, J., Ciarelli, G., Prévôt, A. S. H., and Baltensperger, U.: A model study on changes of European and Swiss particulate matter, ozone and nitrogen deposition between 1990 and 2020 due to the revised Gothenburg protocol, Atmos. Chem. Phys., 14, 13081–13095, https://doi.org/10.5194/acp-14-13081-2014, 2014. a
Aksoyoglu, S., Baltensperger, U., and Prévôt, A. S. H.: Contribution of ship emissions to the concentration and deposition of air pollutants in Europe, Atmos. Chem. Phys., 16, 1895–1906, https://doi.org/10.5194/acp-16-1895-2016, 2016. a
Anav, A., De Marco, A., Proietti, C., Alessandri, A., Dell'Aquila, A., Cionni, I., Friedlingstein, P., Khvorostyanov, D., Menut, L., Paoletti, E., Sicard, P., Sitch, S., Vitale, M., Anav, A., De Marco, A., and Proietti, C.: Comparing concentration-based (AOT40) and stomatal uptake (PODY) metrics for ozone risk assessment to European forests, Glob. Change Biol., 22, 1608–1627, https://doi.org/10.1111/gcb.13138, 2016. a
Avnery, S., Mauzerall, D. L., and Fiore, A. M.: Increasing global agricultural production by reducing ozone damages via methane emission controls and ozone-resistant cultivar selection, Glob. Change Biol., 19, 1285–1299, https://doi.org/10.1111/Gcb.12118, 2013. a
Butler, T., Lupascu, A., Coates, J., and Zhu, S.: TOAST 1.0: Tropospheric Ozone Attribution of Sources with Tagging for CESM 1.2.2, Geosci. Model Dev., 11, 2825–2840, https://doi.org/10.5194/gmd-11-2825-2018, 2018. a, b, c, d, e, f, g, h, i, j
Chan, C. K. and Yao, X.: Air pollution in mega cities in China, Atmos. Environ., 42, 1–42, https://doi.org/10.1016/j.atmosenv.2007.09.003, 2008. a
Chou, M.-D. and Suarez, M. J.: An efficient thermal infrared radiation parametrization for use in general circulation models, NASA Tech. Memo., 104606, 85 pp., 1994. a
Christensen, J. H. and Christensen, O. B.: A summary of the PRUDENCE model projections of changes in European climate by the end of this century, Climatic Change, 81, 7–30, https://doi.org/10.1007/s10584-006-9210-7, 2007. a
Colette, A., Granier, C., Hodnebrog, Ø., Jakobs, H., Maurizi, A., Nyiri, A., Rao, S., Amann, M., Bessagnet, B., D'Angiola, A., Gauss, M., Heyes, C., Klimont, Z., Meleux, F., Memmesheimer, M., Mieville, A., Rouïl, L., Russo, F., Schucht, S., Simpson, D., Stordal, F., Tampieri, F., and Vrac, M.: Future air quality in Europe: a multi-model assessment of projected exposure to ozone, Atmos. Chem. Phys., 12, 10613–10630, https://doi.org/10.5194/acp-12-10613-2012, 2012. a
Dahlmann, K., Grewe, V., Ponater, M., and Matthes, S.: Quantifying the contributions of individual NOx sources to the trend in ozone radiative forcing, Atmos. Environ., 45, 2860–2868, https://doi.org/10.1016/j.atmosenv.2011.02.071, 2011. a
Danielsen, E. F.: Stratospheric-Tropospheric Exchange Based on Radioactivity, Ozone and Potential Vorticity. J. Atmos. Sci., 25, 502–518, https://doi.org/10.1175/1520-0469(1968)025<0502:STEBOR>2.0.CO;2, 1968. a
Derwent, R. G., Utember, S. R., Jenkin, M. E., and Shallcross, D. E.: Tropospheric ozone production regions and the intercontinental origins of surface ozone over Europe, Atmos. Environ., 112, 216–224, 2015. a, b
EEA: Air quality in Europe-2017 report, EEA Report, No 13/2017, ISBN 978-92-9213-920-9, 80 pp., Publications Office ofthe European Union, Luxembourg, available at: https://www.eea.europa.eu/publications/air-quality-in-europe-2017 (last access: 26 June 2019), 2017a. a
EEA: AirbAse – The European air quality database, available at: https://www.eea.europa.eu/data-and-maps/data/aqereporting-8 (last access: 12 September 2018), 2017b. a
Emmons, L. K., Hess, P. G., Lamarque, J.-F., and Pfister, G. G.: Tagged ozone mechanism for MOZART-4, CAM-chem and other chemical transport models, Geosci. Model Dev., 5, 1531–1542, https://doi.org/10.5194/gmd-5-1531-2012, 2012. a, b, c
EU directive 2008/50/EC: EU directive 2008/50/EC of the European parliament and of the council on Ambient Air Quality and Cleaner Air for Europe, available at: https://eur-lex.europa.eu/eli/dir/2008/50/2015-09-18 (last access: 6 April 2018), 2008. a, b, c, d, e, f
European Commission: Forest Fires in Europe 2010, Luxembourg: Publications Office of the European Union, EUR 24910 EN, ISBN 978-92-79-20919-2, 2011. a
Fiore, A., Dentener, F. J., Wild, O., Cuvelier, C., Schultz, M. G., Hess, P., Textor, C., Schulz, M., Doherty, R. M., Horowitz, L. W., MacKenzie, I. A., Sanderson, M. G., Shindell, D. T., Stevenson, D. S., Szopa, S., Dingenen, R. V., Zeng, G., Atherton, C., Bergmann, D., Bey, I., Carmichael, G., Collins, W. J., Duncan, B. N., Faluvegi, G., Folberth, G., Gauss, M., Gong, S., Hauglustaine, D., Holloway, T., Isaksen, I. S. A., Jacob, D. J., Jonson, J. E., Kaminski, J. W., Keating, T. J., Lupu, A., Marmer, E., Montanaro, V., Park, R. J., Pitari, G., Pringle, K. J., Pyle, J. A., Schroeder, S., Vivanco, M. G., Wind, P., Wojcik, G., Wu, S., and Zuber, A.: Multimodel estimates of intercontinental source-receptor relationships for ozone pollution, J. Geophys. Res., 114, D04301, https://doi.org/10.1029/2008JD010816, 2009. a, b
Fleming, Z., Doherty, R., Von Schneidemesser, E., Malley, C., Cooper, O., Pinto, J., Colette, A., Xu, X., Simpson, D., Schultz, M., Lefohn, A., Hamad, S., Moolla, R., Solberg, S., and Feng, Z.: Tropospheric Ozone Assessment Report: Present-day ozone distribution and trends relevant to human health, Elementa, 6, 12, https://doi.org/10.1525/elementa.273, 2018. a, b, c
Flemming, J., Huijnen, V., Arteta, J., Bechtold, P., Beljaars, A., Blechschmidt, A.-M., Diamantakis, M., Engelen, R. J., Gaudel, A., Inness, A., Jones, L., Josse, B., Katragkou, E., Marecal, V., Peuch, V.-H., Richter, A., Schultz, M. G., Stein, O., and Tsikerdekis, A.: Tropospheric chemistry in the Integrated Forecasting System of ECMWF, Geosci. Model Dev., 8, 975–1003, https://doi.org/10.5194/gmd-8-975-2015, 2015. a
Folberth, G. A., Butler, T. M., Collins, W. J., and Rumbold, S. T.: Megacities and climate change – A brief overview, Environ. Pollut., 203, 235–242, https://doi.org/10.1016/j.envpol.2014.09.004, 2015. a
Gao, J., Zhu, B., Xiao, H., Kang, H., Hou, X., and Shao, P.: A case study of surface ozone source apportionment during a high concentration episode, under frequent shifting wind conditions over the Yangtze River Delta, China, Sci. Total Environ., 544, 853–863, https://doi.org/10.1016/j.scitotenv.2015.12.039, 2016. a
Gaudel, A., Cooper, O. R., Ancellet, G., Barret, B., Boynard, A., Burrows, J. P., Clerbaux, C., Coheur, P. ., Cuesta, J., Cuevas, E., Doniki, S., Dufour, G., Ebojie, F., Foret, G., Garcia, O., Granados-Muñoz, M. J., Hannigan, J. W., Hase, F., Hassler, B., Huang, G., Hurtmans, D., Jaffe, D., Jones, N., Kalabokas, P., Kerridge, B., Kulawik, S., Latter, B., Leblanc, T., Le Flochmoën, E., Lin, W., Liu, J., Liu, X., Mahieu, E., McClure-Begley, A., Neu, J. L., Osman, M., Palm, M., Petetin, H., Petropavlovskikh, I., Querel, R., Rahpoe, N., Rozanov, A., Schultz, M. G., Schwab, J., Siddans, R., Smale, D., Steinbacher, M., Tanimoto, H., Tarasick, D. W., Thouret, V., Thompson, A. M., Trickl, T., Weatherhead, E., Wespes, C., Worden, H. M., Vigouroux, C., Xu, X., Zeng, G., and Ziemke, J.: Tropospheric Ozone Assessment Report: Present-day distribution and trends of tropospheric ozone relevant to climate and global atmospheric chemistry model evaluation, Elementa, 6, 39, https://doi.org/10.1525/elementa.291, 2018. a, b
Gilbert, N.: Russia counts environmental cost of wildfires, Nature News, https://doi.org/10.1038/news.2010.404, 2010. a
Giordano, L., Brunner, D., Flemming, J., Hogrefe, C., Im, U., Bianconi, R., Badia, A., Balzarini, A., Baró, R., Chemel, C., Curci, G., Forkel, R., Jiménez-Guerrero, P., Hirtl, M., Hodzic, A., Honzak, L., Jorba, O., Knote, C., Kuenen, J. J. P., Makar, P. A., Manders-Groot, A., Neal, L., Pérez, J. L., Pirovano, G., Pouliot, G., San José, R., Savage, N., Schroder, W., Sokhi, R. S., Syrakov, D., Torian, A., Tuccella, P., Werhahn, J., Wolke, R., Yahya, K., Žabkar, R., Zhang, Y., and Galmarini, S.: Assessment of the MACC re-analysis and its influence as chemical boundary conditions for regional air quality modeling in AQMEII-2, Atmos. Environ., 115, 371–388, 2015. a
Grell, G. A. and Freitas, S. R.: A scale and aerosol aware stochastic convective parameterization for weather and air quality modeling, Atmos. Chem. Phys., 14, 5233-5250, https://doi.org/10.5194/acp-14-5233-201, 2014. a
Grell, G. A., Peckham, S. E., Schmitz, R., McKeen, S. A., Frost, G., Skamarock, W. C., and Eder, B.: Fully coupled online chemistry within the WRF model, Atmos. Environ., 39, 6957–6975, 2005. a
Grewe, V., Tsati, E., and Hoor, P.: On the attribution of contributions of atmospheric trace gases to emissions in atmospheric model applications, Geosci. Model Dev., 3, 487–499, https://doi.org/10.5194/gmd-3-487-2010, 2010. a
Grewe, V., Dahlmann, K., Matthes, S., and Steinbrecht, W.: Attributing ozone to NOx emissions: Implications for climate mitigation measures, Atmos. Environ., 59, 102–107, https://doi.org/10.1016/j.atmosenv.2012.05.002, 2012. a, b
Grewe, V., Tsati, E., Mertens, M., Frömming, C., and Jöckel, P.: Contribution of emissions to concentrations: the TAGGING 1.0 submodel based on the Modular Earth Submodel System (MESSy 2.52), Geosci. Model Dev., 10, 2615–2633, https://doi.org/10.5194/gmd-10-2615-2017, 2017. a
Gromov, S., Jöckel, P., Sander, R., and Brenninkmeijer, C. A. M.: A kinetic chemistry tagging technique and its application to modelling the stable isotopic composition of atmospheric trace gases, Geosci. Model Dev., 3, 337–364, https://doi.org/10.5194/gmd-3-337-2010, 2010. a
Guenther, A., Karl, T., Harley, P., Wiedinmyer, C., Palmer, P. I., and Geron, C.: Estimates of global terrestrial isoprene emissions using MEGAN (Model of Emissions of Gases and Aerosols from Nature), Atmos. Chem. Phys., 6, 3181–3210, https://doi.org/10.5194/acp-6-3181-2006, 2006. a
Hong, S.-Y., Noh, Y., and Dudhia, J.: A new vertical diffusion package with an explicit treatment of entrainment processes, Mon. Weather Rev., 134, 2318–2341, https://doi.org/10.1175/MWR3199.1, 2006. a
HTAP: Hemispheric Transport of Air Pollution 2010, Part A: Ozone and Particulate Matter, Air Pollution Studies, No. 17, Geneva, Switzerland, 2010. a
Huijnen, V., Flemming, J., Kaiser, J. W., Inness, A., Leitão, J., Heil, A., Eskes, H. J., Schultz, M. G., Benedetti, A., Hadji-Lazaro, J., Dufour, G., and Eremenko, M.: Hindcast experiments of tropospheric composition during the summer 2010 fires over western Russia, Atmos. Chem. Phys., 12, 4341–4364, https://doi.org/10.5194/acp-12-4341-2012, 2012. a
Iacono, M., Delamere, J., Mlawer, E., Shephard, M., Clough, S., and Collins, W.: Radiative forcing by long-lived greenhouse gases: Calculations with the AER radiative transfer models, J. Geophys. Res., 113, D13103, https://doi.org/10.1029/2008JD009944, 2008. a
Im, U., Christensen, J. H., Geels, C., Hansen, K. M., Brandt, J., Solazzo, E., Alyuz, U., Balzarini, A., Baro, R., Bellasio, R., Bianconi, R., Bieser, J., Colette, A., Curci, G., Farrow, A., Flemming, J., Fraser, A., Jimenez-Guerrero, P., Kitwiroon, N., Liu, P., Nopmongcol, U., Palacios-Peña, L., Pirovano, G., Pozzoli, L., Prank, M., Rose, R., Sokhi, R., Tuccella, P., Unal, A., Vivanco, M. G., Yarwood, G., Hogrefe, C., and Galmarini, S.: Influence of anthropogenic emissions and boundary conditions on multi-model simulations of major air pollutants over Europe and North America in the framework of AQMEII3, Atmos. Chem. Phys., 18, 8929–8952, https://doi.org/10.5194/acp-18-8929-2018, 2018. a
Jiménez, P. A. and Dudhia, J.: Improving the Representation of Resolved and Unresolved Topographic Effects on Surface Wind in the WRF Model, J. Appl. Meteorol. Clim., 51, 300–316, https://doi.org/10.1175/JAMC-D-11-084.1, 2012. a
Jiménez, P., Lelieveld, J., and Baldasano, J. M.: Multiscale modeling of air pollutants dynamics in the northwestern Mediterranean basin during a typical summertime episode, J. Geophys. Res., 111, D18306, https://doi.org/10.1029/2005JD006516, 2006. a
Jiménez, P., Dudhia, J., González-Rouco, J., Navarro, J., Montávez, J., and García-Bustamante, E.: A revised scheme for the WRF surface layer formulation, Mon. Weather Rev., 140, 898–918, https://doi.org/10.1175/MWR-D-11-00056.1, 2012. a
Karamchandani, P., Long, Y., Pirovano, G., Balzarini, A., and Yarwood, G.: Source-sector contributions to European ozone and fine PM in 2010 using AQMEII modeling data, Atmos. Chem. Phys., 17, 5643–5664, https://doi.org/10.5194/acp-17-5643-2017, 2017. a
Kuenen, J. J. P., Visschedijk, A. J. H., Jozwicka, M., and Denier van der Gon, H. A. C.: TNO-MACC_II emission inventory; a multi-year (2003–2009) consistent high-resolution European emission inventory for air quality modelling, Atmos. Chem. Phys., 14, 10963–10976, https://doi.org/10.5194/acp-14-10963-2014, 2014. a
Lapina, K., Henze, D. K., Milford, J. B., Huang, M., Lin, M., Fiore, A. M., Carmichael, G., Pfister, G. G., and Bowman, K.: Assessment of source contributions to seasonal vegetative exposure to ozone in the U.S., J. Geophys. Res.-Atmos., 119, 324–340, https://doi.org/10.1002/2013JD020905, 2014. a, b
Lefohn, A., Malley, C., Smith, L., Wells, B., Hazucha, M., Simon, H., Naik, V., Mills, G., Schultz, M., Paoletti, E., De Marco, A., Xu, X., Zhang, L., Wang, T., Neufeld, H., Musselman, R., Tarasick, D., Brauer, M., Feng, Z., Tang, H., Kobayashi, K., Sicard, P., Solberg, S., and Gerosa, G.: Tropospheric ozone assessment report: Global ozone metrics for climate change, human health, and crop/ecosystem research, Elementa, 6, 28, https://doi.org/10.1525/elementa.279, 2018. a, b
Lefohn, A. S., Laurence, J. A., and Kohut, R. J.: A comparison of indices that describe the relationship between exposure to ozone and reduction in the yield of agricultural crops, Atmos. Environ., 22, 1229–1240, https://doi.org/10.1016/0004-6981(88)90353-8, 1988. a
Li, G., Zhang, R., Fan, J., and Tie, X.: Impacts of black carbon aerosol on photolysis and ozone, J. Geophys. Res., 110, D23206, https://doi.org/10.1029/2005JD005898, 2005. a
Li, G., Bei, N., Cao, J., Wu, J., Long, X., Feng, T., Dai, W., Liu, S., Zhang, Q., and Tie, X.: Widespread and persistent ozone pollution in eastern China during the non-winter season of 2015: observations and source attributions, Atmos. Chem. Phys., 17, 2759–2774, https://doi.org/10.5194/acp-17-2759-2017, 2017. a
Li, Y., Zhang, J., Sailor, D. J., and Ban-Weiss, G. A.: Effects of urbanization on regional meteorology and air quality in Southern California, Atmos. Chem. Phys., 19, 4439–4457, https://doi.org/10.5194/acp-19-4439-2019, 2019. a
Lupascu, A. and Butler, T.: Lupascu_Butler_ACP_WRFChem v1.0, Zenodo, https://doi.org/10.5281/zenodo.3501963, 2019. a
Mar, K. A., Ojha, N., Pozzer, A., and Butler, T. M.: Ozone air quality simulations with WRF-Chem (v3.5.1) over Europe: model evaluation and chemical mechanism comparison, Geosci. Model Dev., 9, 3699–3728, https://doi.org/10.5194/gmd-9-3699-2016, 2016. a, b, c, d, e
Mertens, M., Grewe, V., Rieger, V. S., and Jöckel, P.: Revisiting the contribution of land transport and shipping emissions to tropospheric ozone, Atmos. Chem. Phys., 18, 5567–5588, https://doi.org/10.5194/acp-18-5567-2018, 2018. a
Mills, G., Pleijel, H., Malley, C., Sinha, B., Cooper, O., Schultz, M., Neufeld, H., Simpson, D., Sharps, K., Feng, Z., Gerosa, G., Harmens, H., Kobayashi, K., Saxena, P., Paoletti, E., Sinha, V., and Xu, X.: Tropospheric ozone assessment report: Present-day tropospheric ozone distribution and trends relevant to vegetation, Elementa, 6, 47, https://doi.org/10.1525/elementa.302, 2018. a, b
Monks, P. S., Archibald, A. T., Colette, A., Cooper, O., Coyle, M., Derwent, R., Fowler, D., Granier, C., Law, K. S., Mills, G. E., Stevenson, D. S., Tarasova, O., Thouret, V., von Schneidemesser, E., Sommariva, R., Wild, O., and Williams, M. L.: Tropospheric ozone and its precursors from the urban to the global scale from air quality to short-lived climate forcer, Atmos. Chem. Phys., 15, 8889–8973, https://doi.org/10.5194/acp-15-8889-2015, 2015. a
Morrison, H., Thompson, G., and Tatarskii, V.: Impact of cloud microphysics on the development of trailing stratiform precipitation in a simulated squall line: Comparison of one- and two-moment schemes, Mon. Weather Rev., 137, 991–1007, https://doi.org/10.1175/2008MWR2556.1, 2009. a
Musselman, R., Lefohn, A., Massman, W., and Heath, R.: A critical review and analysis of the use of exposure- and flux-based ozone indices for predicting vegetation effects, Atmos. Environ., 40, 1869–1888, https://doi.org/10.1016/j.atmosenv.2005.10.064, 2006. a
Neu, J. L. and Prather, M. J.: Toward a more physical representation of precipitation scavenging in global chemistry models: cloud overlap and ice physics and their impact on tropospheric ozone, Atmos. Chem. Phys., 12, 3289–3310, https://doi.org/10.5194/acp-12-3289-2012, 2012. a
Otero, N., Sillmann, J., Mar, K. A., Rust, H. W., Solberg, S., Andersson, C., Engardt, M., Bergström, R., Bessagnet, B., Colette, A., Couvidat, F., Cuvelier, C., Tsyro, S., Fagerli, H., Schaap, M., Manders, A., Mircea, M., Briganti, G., Cappelletti, A., Adani, M., D'Isidoro, M., Pay, M.-T., Theobald, M., Vivanco, M. G., Wind, P., Ojha, N., Raffort, V., and Butler, T.: A multi-model comparison of meteorological drivers of surface ozone over Europe, Atmos. Chem. Phys., 18, 12269–12288, https://doi.org/10.5194/acp-18-12269-2018, 2018. a
Paoletti, E., De Marco, A., and Racalbuto, S.: Why should we calculate complex indices of ozone exposure? Results from Mediterranean background stations, Environ. Monit. Assess., 128, 19–30, https://doi.org/10.1007/s10661-006-9412-5., 2007. a
Paoletti, E., De Marco, A., Beddows, D., Harrison, R., and Manning, W.: Ozone levels in European and USA cities are increasing more than at rural sites, while peak values are decreasing, Environ. Pollut., 192, 295–299, https://doi.org/10.1016/j.envpol.2014.04.040, 2014. a, b
Pfister, G. G., Avise, J., Wiedinmyer, C., Edwards, D. P., Emmons, L. K., Diskin, G. D., Podolske, J., and Wisthaler, A.: CO source contribution analysis for California during ARCTAS-CARB, Atmos. Chem. Phys., 11, 7515–7532, https://doi.org/10.5194/acp-11-7515-2011, 2011. a
Pfister, G. G., Walters, S., Emmons, L. K., Edwards, D. P., and Avise, J.: Quantifying the contribution of inflow on surface ozone over California during summer 2008, J. Geophys. Res.-Atmos., 118, 12282–12299, https://doi.org/10.1002/2013JD020336, 2013. a, b
Querol, X., Alastuey, A., Gangoiti, G., Perez, N., Lee, H. K., Eun, H. R., Park, Y., Mantilla, E., Escudero, M., Titos, G., Alonso, L., Temime-Roussel, B., Marchand, N., Moreta, J. R., Revuelta, M. A., Salvador, P., Artíñano, B., García dos Santos, S., Anguas, M., Notario, A., Saiz-Lopez, A., Harrison, R. M., Millán, M., and Ahn, K.-H.: Phenomenology of summer ozone episodes over the Madrid Metropolitan Area, central Spain, Atmos. Chem. Phys., 18, 6511–6533, https://doi.org/10.5194/acp-18-6511-2018, 2018. a, b
Ramaswamy, V., Boucher, O., Haigh, J., Hauglustaine, D., Haywood, J., Myhre, G., Nakajima, T., Shi, G., and 2001, S. S.: Radiative forcing of climate change, in: Climate Change 2001: The Scientific Basis, Contribution of WG1 to the Third Assessment Report of the IPCC, edited by: Houghton, J. T., Dingis, Y., Griggsis, D. J., Nogueris, M., van der Lindenis, P. J., Daiis, X., Maskellis, K., and Johnsonis, C. A., Cambridge University Press, England, 2001. a
Safieddine, S., Boynard, A., Coheur, P.-F., Hurtmans, D., Pfister, G., Quennehen, B., Thomas, J. L., Raut, J.-C., Law, K. S., Klimont, Z., Hadji-Lazaro, J., George, M., and Clerbaux, C.: Summertime tropospheric ozone assessment over the Mediterranean region using the thermal infrared IASI/MetOp sounder and the WRF-Chem model, Atmos. Chem. Phys., 14, 10119–10131, https://doi.org/10.5194/acp-14-10119-2014, 2014. a, b, c
Sandu, A. and Sander, R.: Technical note: Simulating chemical systems in Fortran90 and Matlab with the Kinetic PreProcessor KPP-2.1, Atmos. Chem. Phys., 6, 187–195, https://doi.org/10.5194/acp-6-187-2006, 2006. a
Stevenson, D. S., Young, P. J., Naik, V., Lamarque, J.-F., Shindell, D. T., Voulgarakis, A., Skeie, R. B., Dalsoren, S. B., Myhre, G., Berntsen, T. K., Folberth, G. A., Rumbold, S. T., Collins, W. J., MacKenzie, I. A., Doherty, R. M., Zeng, G., van Noije, T. P. C., Strunk, A., Bergmann, D., Cameron-Smith, P., Plummer, D. A., Strode, S. A., Horowitz, L., Lee, Y. H., Szopa, S., Sudo, K., Nagashima, T., Josse, B., Cionni, I., Righi, M., Eyring, V., Conley, A., Bowman, K. W., Wild, O., and Archibald, A.: Tropospheric ozone changes, radiative forcing and attribution to emissions in the Atmospheric Chemistry and Climate Model Intercomparison Project (ACCMIP), Atmos. Chem. Phys., 13, 3063–3085, https://doi.org/10.5194/acp-13-3063-2013, 2013. a
Struzewska, J., Zdunek, M., Kaminski, J. W., Łobocki, L., Porebska, M., Jefimow, M., and Gawuc, L.: Evaluation of the GEM-AQ model in the context of the AQMEII Phase 1 project, Atmos. Chem. Phys., 15, 3971–3990, https://doi.org/10.5194/acp-15-3971-2015, 2015. a
Sudo, K. and Akimoto, H.: Global source attribution of tropospheric ozone: Long-range transport from various source regions, J. Geophys. Res., 112, D12302, https://doi.org/10.1029/2006JD007992, 2007. a
Tagaris, E., Stergiou, I., and Sotiropoulou, R.: Impact of shipping emissions on ozone levels over Europe: assessing the relative importance of the Standard Nomenclature for Air Pollution (SNAP) categories, Environ. Sci. Pollut. R., 24, 14903–14909, https://doi.org/10.1007/s11356-017-9046-x, 2017. a, b
Tai, A. P. and Val Martin, M.: Impacts of ozone air pollution and temperature extremes on crop yields: Spatial variability, adaptation and implications for future food security, Atmos. Environ., 169, 11–21, https://doi.org/10.1016/j.atmosenv.2017.09.002, 2017. a
Tang, Y., Carmichael, G., Thongboonchoo, N., Chai, T., Horowitz, L., Pierce, R., Al-Saadi, J., Pfister, G., Vukovich, J., Avery, M., Sachse, G., Ryerson, T., Holloway, J., Atlas, E., Flocke, F., Weber, R., Huey, L., Dibb, J., Streets, D., and Brune, W.: Influence of lateral and top boundary conditions on regional air quality prediction: A multiscale study coupling regional and global chemical transport models, J. Geophys. Res., 112, D10S18, https://doi.org/10.1029/2006JD007515, 2007. a
Thouret, V., Cammas, J.-P., Sauvage, B., Athier, G., Zbinden, R., Nédélec, P., Simon, P., and Karcher, F.: Tropopause referenced ozone climatology and inter-annual variability (1994–2003) from the MOZAIC programme, Atmos. Chem. Phys., 6, 1033–1051, https://doi.org/10.5194/acp-6-1033-2006, 2006. a
Tie, X., Madronich, S., Walters, S., Zhang, R., Rasch, P., and Collins, W.: Effect of clouds on photolysis and oxidants in the troposphere, J. Geophys. Res., 108, 4642, https://doi.org/10.1029/2003JD003659, 2003. a
Tong, D., Mathur, R., Kang, D., Yu, S., Schere, K., and Pouliot, G.: Vegetation exposure to ozone over the continental United States: Assessment of exposure indices by the Eta-CMAQ air quality forecast model, Atmos. Environ., 43, 724–733, https://doi.org/10.1016/j.atmosenv.2008.09.084, 2009. a
Tuccella, P., Curci, G., Visconti, G., Bessagnet, B., Menut, L., and Park, R.: Modeling of gas and aerosol with WRF/Chem over Europe: Evaluation and sensitivity study, J. Geophys. Res., 117, D03303, https://doi.org/10.1029/2011JD016302, 2012. a, b, c
UNECE: Mapping Critical Levels for Vegetation, International Cooperative Programme on Effects of Air Pollution on Natural Vegetation and Crops, Bangor, UK, 2010. a
Vijayaraghavan, K., Cho, S., Morris, R., Spink, D., Jung, J., Pauls, R., and Duffett, K.: Photochemical model evaluation of the ground-level ozone impacts on ambient air quality and vegetation health in the Alberta oil sands region: Using present and future emission scenarios, Atmos. Environ., 141, 209–218, https://doi.org/10.1016/j.atmosenv.2016.06.053, 2016. a
Wang, Z., Chien, C.-J., and Tonnesen, G.: Development of a tagged species source apportionment algorithm to characterize three-dimensional transport and transformation of precursors and secondary pollutants, J. Geophys. Res., 114, D21206, https://doi.org/10.1029/2008JDO10846, 2009. a
Wesely, M.: Parameterization of surface resistances to gaseous dry deposition in regional-scale numerical models, Atmos. Environ., 23, 1293–1304, https://doi.org/10.1016/0004-6981(89)90153-4, 1989. a
Westenbarger, D. A. and Frisvold, G.: Air pollution and farm level crop yields: an empirical analysis of corn and soybeans, Agricultural and Resource Economics Review, 24, 156–165, 1995. a
WHO: Quantification of health effects of exposure to air pollution, EUR/01/5026342, E74256, WHO Regional office for Europe, Copenhagen, available at: http://www.euro.who.int/document/e74256.pdf (last access: 20 April 2018), 2001. a
WHO: Air quality guidelines. Global update 2005. Particulate matter, ozone, nitrogen dioxide and sulfur dioxide, World Health Organizations 2006, ix+484 pp., ISBN 9289021926, available at: http://www.euro.who.int/__data/assets/pdf_file/0005/78638/E90038.pdf?ua=1 (last access: 18 April 2016), 2006. a
WHO: Evolution of WHO air quality guidelines: past, present and future, World Health Organizations, ISBN 9789289052306, vi+32 pp., 2017. a, b
Wiedinmyer, C., Akagi, S. K., Yokelson, R. J., Emmons, L. K., Al-Saadi, J. A., Orlando, J. J., and Soja, A. J.: The Fire INventory from NCAR (FINN): a high resolution global model to estimate the emissions from open burning, Geosci. Model Dev., 4, 625–641, https://doi.org/10.5194/gmd-4-625-2011, 2011. a
Yarwood, G., Morris, R., Yocke, M., Hogo, H., and Chico, T.: Development of a Methodology for Source Apportionment of Ozone Concentrations Estimates From a Photo-chemical Grid Model, Air and Waste Management Association, 89th AWNA Annual Meeting, Nashville Tenn., p. 15222, 1996. a
- Abstract
- Introduction
- Model simulation
- Results and discussions
- Conclusions
- Code and data availability
- Appendix A: List of chemistry modules changed to account for the new chemical mechanism
- Author contributions
- Competing interests
- Acknowledgements
- Financial support
- Review statement
- References
- Supplement
- Abstract
- Introduction
- Model simulation
- Results and discussions
- Conclusions
- Code and data availability
- Appendix A: List of chemistry modules changed to account for the new chemical mechanism
- Author contributions
- Competing interests
- Acknowledgements
- Financial support
- Review statement
- References
- Supplement