the Creative Commons Attribution 4.0 License.
the Creative Commons Attribution 4.0 License.
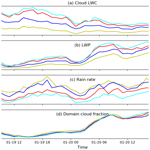
Effects of aerosol in simulations of realistic shallow cumulus cloud fields in a large domain
George Spill
Philip Stier
Paul R. Field
Guy Dagan
Previous study of shallow convection has generally suffered from having to balance domain size with resolution, resulting in high-resolution studies which do not capture large-scale behaviour of the cloud fields. In this work we hope to go some way towards addressing this by carrying out cloud-resolving simulations on large domains. Simulations of trade wind cumulus are carried out using the Met Office Unified Model (UM), based on a case study from the Rain In Cumulus over the Ocean (RICO) field campaign. The UM is run with a nested domain of 500 km with 500 m resolution, in order to capture the large-scale behaviour of the cloud field, and with a double-moment interactive microphysics scheme. Simulations are run using baseline aerosol profiles based on observations from RICO, which are then perturbed. We find that the aerosol perturbations result in changes to the convective behaviour of the cloud field, with higher aerosol leading to an increase (decrease) in the number of deeper (shallower) clouds. However, despite this deepening, there is little increase in the frequency of higher rain rates. This is in contrast to the findings of previous work making use of idealised simulation setups. In further contrast, we find that increasing aerosol results in a persistent increase in domain mean liquid water path and decrease in precipitation, with little impact on cloud fraction.
- Article
(1415 KB) - Full-text XML
- BibTeX
- EndNote
Shallow cumuli are the most common cloud type on Earth (Rossow and Schiffer, 1999; Sassen and Wang, 2008); they are ubiquitous throughout the trade winds, yet their behaviour is still poorly understood. These small, warm, shallow convective clouds have an important part in regulating the thermodynamics and dynamics of their environment; warming the cloud layer through condensation, transporting moisture to the inversion layer above, and cooling both the inversion and the sub-cloud layer through the evaporation of detraining cloud droplets and precipitation (Hartmann et al., 1992; Zhu and Bretherton, 2004; Neggers et al., 2007).
Trade wind shallow cumuli are of great interest in the context of a changing climate. In particular due to their coupling to circulation, as well as their radiative properties; reflecting shortwave radiation whilst emitting longwave radiation at a similar temperature to the surface due to their low, warm cloud tops. The myriad of ways in which they interact with their environment means there is still much uncertainty in how they may respond to perturbations to the climate. Indeed, low-cloud feedbacks are responsible for most of the uncertainty in climate sensitivity (Bony et al., 2004; Bony and Dufresne, 2005; Medeiros et al., 2008, 2015; Vial et al., 2013; Boucher et al., 2013).
Aerosol particles in the atmosphere can act as cloud condensation nuclei (CCN), allowing the formation of cloud droplets (Köhler, 1936). Changes in aerosol concentration can therefore have significant impacts on the properties of clouds. For example, for a given liquid water content, an increase in CCN will lead to a greater number of smaller droplets. Smaller, more numerous droplets scatter more shortwave radiation back to space, and thus this results in an increase in the cloud albedo (Twomey, 1977). Additionally, the shift in the droplet size distribution may affect the formation of precipitation in shallow clouds by inhibiting the development of larger droplets (Albrecht, 1989).
Aerosol-induced changes in the precipitation efficiency of clouds can also lead to impacts on convection. Suppressed precipitation can result in increased condensation warming the lower part of the cloud layer, and increased evaporation of detraining droplets cooling the upper part. This destabilisation of the cloud layer can lead to an invigoration and deepening of the convection (Albrecht, 1993; Stevens and Feingold, 2009; Dagan et al., 2016; Sheffield et al., 2015).
Cloud fields may be affected in other ways: changing precipitation characteristics may affect the formation of cold pools, for example, which can have an impact on the development of new convection and contribute to the mesoscale organisation of the field of shallow clouds (Seifert and Heus, 2013; Seigel, 2014; Seifert et al., 2015).
A number of studies (Xue et al., 2008; Jiang et al., 2010) have seen significant aerosol effects such as those described above. However, several others (van den Heever et al., 2011; Seifert et al., 2015) have also shown effects where parts of the system respond to perturbations in such a way as to offset the initial aerosol effect. Stevens and Feingold 2009 described these as buffering effects, and proposed possible buffers that may be relevant for cloud–aerosol interactions, including, for example, convective deepening and invigoration. They describe a mechanism for the deepening of shallow cumuli by increasing aerosol, whereby higher droplet numbers delay the onset of precipitation and increase evaporation at the cloud top. This destabilises the cloud layer, enabling greater vertical development of the cloud, which can then produce heavier rain, potentially compensating for the initial reduction in precipitation.
There have been a number of observational studies showing an invigoration effect on shallow clouds (Kaufman et al., 2005; Yuan et al., 2011; Koren et al., 2014), while modelling studies have shown seemingly conflicting results. Jiang and Feingold (2005) and Xue et al. (2008) both find that increasing aerosol actually suppresses convection in warm, shallow clouds, while Dagan et al. (2017) and Altaratz et al. (2014) argue for a “turning point” between suppression and invigoration of convection, depending on local conditions and specific cloud properties. Van den Heever et al. (2011) find that even within a cloud field the response varies: with shallower clouds being suppressed and deeper clouds penetrating the trade inversion experiencing invigoration. A similar result is obtained by Seifert et al. (2015), who find a reduction in the number of small clouds due to an evaporative feedback from aerosol-suppression of precipitation. Both van den Heever et al. (2011) and Seifert et al. (2015) find that, though there are aerosol effects on cloud populations and properties such as rain rate, over a large area and after a long time these effects are minor. In contrast, Saleeby et al. (2015) find that a reduction in shallower cumuli and stratocumulus, along with an increase in deeper cumuli, leads to a reduction in domain-accumulated precipitation with increased aerosol.
Much of the behaviour of convective clouds is constrained or driven by local conditions – heating, water budgets, or large-scale subsidence for example – many of which may contribute to so-called buffering effects (Seifert et al., 2012), raising the possibility that cloud responses to aerosol are regime dependent or regionally dependent.
Despite much work on the subject, there is still a great deal of uncertainty and debate over the response of shallow convection to perturbations such as changes in aerosol (Tao et al., 2012). Typical modelling studies of shallow convection make use of high-resolution large eddy simulations (LESs) or cloud-resolving models (CRMs). These models explicitly resolve convection but until recently have only been run on limited area domains, of the order of tens of kilometres, due to computational limitations.
In this work we begin to extend the investigation of shallow convection by making use of the Met Office Unified Model's capabilities to run high-resolution simulations on large domains in order to study the effect of aerosol perturbations over entire cloud fields on spatial scales of the order of hundreds of kilometres. Additionally, the use of a double-moment cloud microphysics scheme, described below, allows aerosol concentration to be perturbed directly, rather than using cloud droplet number as a proxy. We aim to investigate the character of the response of shallow convection to aerosol perturbations in simulations of realistic weather systems and whether and why this may differ from that seen in idealised simulations.
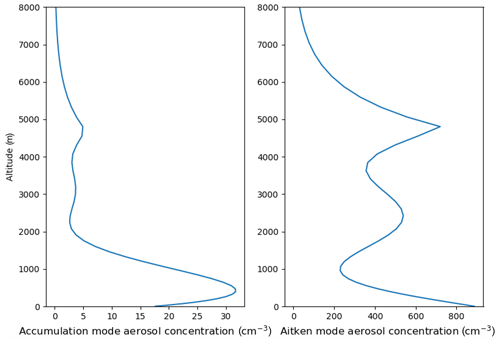
Figure 1Vertical profiles of accumulation and Aitken mode aerosol concentration used in the baseline simulation.
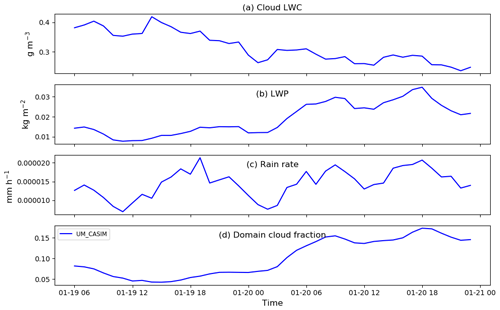
Figure 2Time series of domain-averaged (a) cloud liquid water content (in-cloud only), (b) liquid water path, (c) rain rate, and (d) cloud fraction, all for the baseline case UM_CASIM. A liquid water content threshold of 0.01 g m−3 is used to define a cloudy grid box.
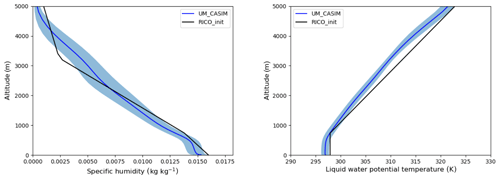
Figure 3Domain-averaged vertical profiles of liquid water potential temperature and specific humidity for the baseline case, compared to the RICO initial setup, with the standard deviation of the baseline shaded.
The Rain in Cumulus over the Ocean (Rauber et al., 2007) campaign was carried out over a period of November 2004–January 2005 in a region of the trade winds in the western Atlantic off the Caribbean. This has been, and is, an ideal region for studies of shallow cumuli due to their prevalence, as well as the absence of upstream islands meaning that clouds observed here are likely to be highly representative examples of shallow cumuli.
Aircraft and shipborne measurements across the campaign region were supported by ground-based systems as well as radiosondes. The aerosol profiles used in this work were based on measurements from one of the NSF/NCAR C-130Q campaign aircraft flights from 19 January 2005 (Stossmeister, 2008). Vertical profiles, shown in Fig. 1, of Aitken and accumulation mode aerosol number concentrations were derived from a fit to these data, and allowed to decay exponentially with height (e-folding height = 1 km) above 5 km.
A global configuration of the Unified Model (UM) vn10.8 (Walters et al., 2017), GA6.1, at resolution N768 (∼25 km × ∼17 km at midlatitudes) is run from operational analysis initial conditions and used as a driving model to provide the lateral boundary conditions for a ∼500 km × ∼500 km nested region, centred on 17.5∘ N, 61.8∘ W. The nested region has a horizontal resolution of ∼500 m × ∼500 m and a stretched vertical coordinate system with 70 levels below 40 km. This nested configuration allows for the simulations to capture the transient features and forcing for the specific case, due to the open boundaries and driving global model. The resolution in the nested region is expected to resolve most of the relevant convection, and inspection of the simulations shows that this is indeed the case. A model time step of 15 s is used with prognostic and diagnostic radiation time steps of 900 and 300 s. The simulations are initialised for 00:00 UTC 19 January 2005 and are run for 48 h. The nested simulations are run without a parameterised convection scheme, and the operational microphysics scheme is replaced in favour of the double-moment Cloud AeroSol Interactive Microphysics (CASIM) scheme (Shipway and Hill, 2012; Grosvenor et al., 2017; Miltenberger et al., 2018). A number of size modes for insoluble and soluble aerosol are available; however, we use only the soluble Aitken and accumulation modes, with the profiles shown in Fig. 1. These profiles are used to initialise the domain and as lateral boundary conditions. Aerosol may be advected through the domain but we do not include processing such as activation scavenging or precipitation washout. Here, CASIM is run with a sub-grid cloud fraction scheme based on that of Smith (1990), which parameterise the sub-grid variability in relative humidity. Its implementation in CASIM is described in Grosvenor et al. (2017). Additionally, we choose to apply the droplet activation scheme from Shipway (2015) rather than CASIM's default scheme from Abdul-Razzak and Ghan (2000). This decision was based on the findings of a number of studies that the latter consistently underestimates the number of activated droplets for very high aerosol concentrations, and has too much competition for water vapour (Simpson et al., 2014; Connolly et al., 2014; Shipway, 2015). Shipway (2015) shows that this is particularly apparent for typical marine aerosol scenarios.
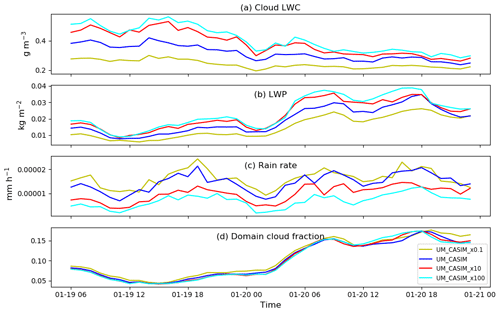
Figure 5Time series of domain-averaged (a) cloud liquid water content (in-cloud only), (b) liquid water path (LWP), (c) rain rate, and (d) cloud fraction. A liquid water content threshold of 0.01 g m−3 is used to define a cloudy grid box.
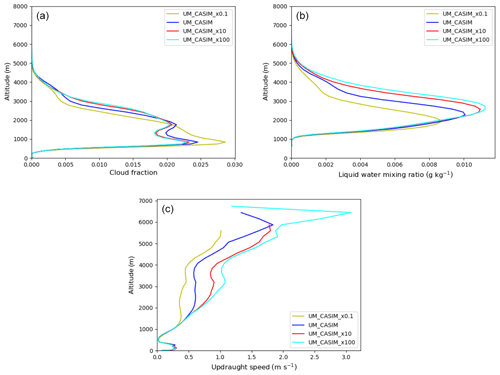
Figure 6Vertical profiles of domain-averaged liquid water mixing ratio (a) and cloud fraction (b), calculated for all columns, and updraught speed (c), calculated for cloudy columns only.
Four simulations with different aerosol number concentrations were carried out: a baseline case, UM_CASIM, and three with the aerosol profiles perturbed by factors of 0.1, 10, and 100, labelled as UM_CASIM_0.1, UM_CASIM_10, and UM_CASIM_100, respectively.
3.1 Structure and evolution of simulation
Figure 2 shows the evolution of a number of domain-averaged quantities over the simulation period for the baseline case, not including a 6 h spin-up. Average profiles of liquid water potential temperature and specific humidity are shown in Fig. 3, which compare well to those used as initial profiles used in the GEWEX Cloud System Study (GCSS) RICO model intercomparison study (vanZanten et al., 2011), as well as those shown in Nuijens et al. (2009) and those obtained from simulations such as in Seifert and Heus (2013). Qualitative visual inspection reveals the transient meteorological features and characteristics of the cloud field over the simulated period. Some example snapshots are shown in Fig. 4.
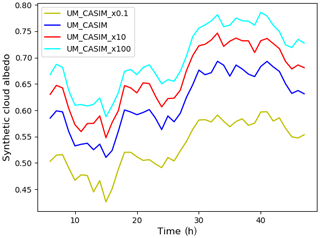
Figure 7Time series of mean synthetic cloud albedo. This is calculated for cloudy columns only using an estimate of the cloud optical depth, as shown in Eq. (1).
3.2 Aerosol perturbations
In Fig. 5, time series of a number of domain-averaged quantities, excluding an initial 6 h spin-up period, show that even when considered across a large domain there is a marked response to the aerosol perturbations.
Figure 5a shows the domain average of in-cloud liquid water with a liquid water threshold of 0.01 g m−3 used to define a cloud. The cloud liquid water content (LWC) and domain liquid water path (LWP) both increase monotonically as the aerosol concentration is increased, while the rain rate decreases. Despite significant effects on other domain-wide parameters, there seems to be only a modest reduction in the domain-wide cloud fraction from aerosol perturbations.
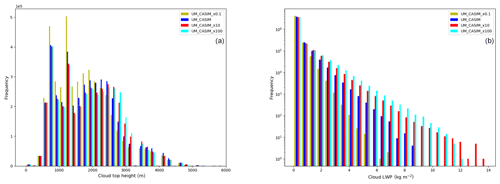
Figure 9Histograms of cloud top height (a), calculated as the highest cloudy grid box in a cloudy column, and of total column liquid water path (b), calculated using only columns containing cloud.
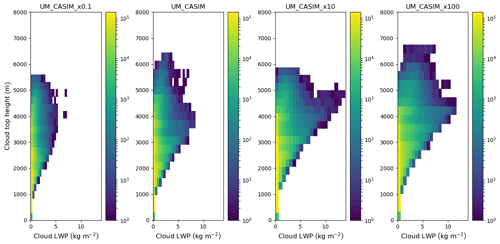
Figure 10Joint histograms of liquid water path and cloud top height for each simulation, with aerosol increasing from left to right along the figure.
Concurrently with the responses in average cloud LWC, we see similar trends in the domain-averaged vertical profiles of liquid water mixing ratio in Fig. 6. The peak in liquid water is increased and is shifted to a higher altitude. Additionally, the liquid water mixing ratio becomes significantly greater at higher altitudes. The changes in these profiles indicate a deepening response to increasing aerosol. This may also be inferred from the profiles of cloud fraction (Fig. 6). As aerosol is increased, the cloud fraction is reduced at lower altitudes but increases at higher altitudes. Additionally, the lowest aerosol case, UM_CASIM_0.1, produces a cloud fraction profile which does not have the same pronounced double peaks seen in the other cases. An invigoration response is also evident in the profiles of updraught speed in Fig. 6. The updraught speeds show little change below 1.5 km; however, there are marked responses above 1.5 km with updraughts increasing in strength with aerosol. This increase, along with smaller droplets under the higher aerosol conditions having smaller fall velocities, leads to more water being lifted higher in the atmosphere (Koren et al., 2015), as can be seen in the vertical profiles of liquid water.
An increase in aerosol loading will generally lead to an increase in the cloud droplet number concentration. Under such an increase, combined with the increase in liquid water path, we would expect the cloud albedo to also increase. In Fig. 7 we show the synthetic cloud albedo, calculated following Seifert and Heus (2013), as
where τ is an estimate of optical depth given by 0.19LWP, depending on the cloud liquid water path, LWP, and cloud droplet number concentration, N (Zhang et al., 2005). This shows that there is indeed a significant increase in the cloud albedo with higher aerosol loads. This change is sufficient to lead to an increase in the domain-wide scene albedo, in spite of the slight reduction in cloud fraction with higher aerosol. This is shown in Fig. 8, where the scene albedo is calculated as
where C is the cloud fraction and Ab is the background albedo. Following Seifert et al. (2015), we assume this to be the albedo of the sea surface at high zenith angles and set it to be 0.05.
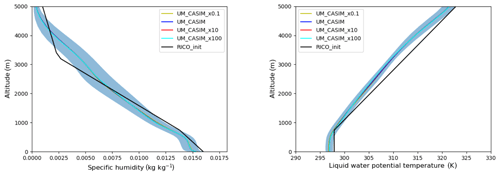
Figure 12Average vertical profiles of liquid water potential temperature and specific humidity for each aerosol concentration, compared to the RICO initial setup as in Fig. 2, with the standard deviation of the baseline case shaded.
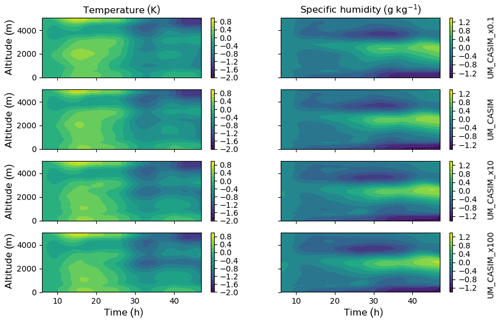
Figure 13Hovmöller plots showing the temporal evolution of domain mean temperature (left) and specific humidity (right). These show the difference between the mean temperature or specific humidity at each time in the simulation and the first time point after the 6 h spin-up. Each row is for a different simulation, with aerosol increasing from top to bottom down the figure.
The distributions of cloud top height (CTH) shown in Fig. 9 also indicate a shift in the convective behaviour with aerosol perturbations: with increasing aerosol resulting in a suppression of the frequency of occurrence of clouds with lower CTHs and an increase in the prevalence of higher CTHs.
The distributions of LWP in Fig. 9 also indicate a deepening response to aerosol: as the aerosol is increased, low LWPs become less frequent, while the tail of the distribution grows and extends to higher values.
The joint histograms of cloud top height and liquid water path also shown in Fig. 10 give a clearer view of the effect: with higher aerosol concentrations come higher peak LWPs, indicating deeper clouds, as well as larger numbers of higher LWP clouds. However, it is also clear from these histograms that throughout all the simulations the cloud fields are dominated in terms of occurrence by the shallowest clouds with lower CTHs.
With the change in the convective behaviour of these clouds comes an effect on the precipitation. As shown in Fig. 11, lower aerosol concentrations result in higher frequencies of drizzle and lower rain rates, while these are suppressed for higher aerosol concentrations, as is the onset of precipitation. This effect is responsible for the reduction in the domain-averaged precipitation in Fig. 2. There does not appear to be a consistent response in the frequency of the highest rain rates; however, due to the rarity of these events it is difficult to draw firm conclusions.
We can attempt to gain a greater insight into the change in convection by inspecting the thermodynamic environment in which the clouds are developing. In Fig. 12 we see that the variation in the mean specific humidity and liquid water potential temperature is far less across the aerosol perturbations than the standard deviation in the baseline case. We can visualise the change in thermodynamic structure through the simulation in more detail using the plots shown in Fig. 13. Here we show the difference between the domain-averaged temperature or specific humidity at a given time and that at the beginning of the analysis period. We can see that though there are some differences between the simulations they are not very large. The minor changes reflect the deepening of convection but also demonstrate that the deepening and invigoration is not sufficient to significantly affect the thermodynamic structure in such a way as to promote further deepening.
We have presented results from a set of large-domain simulations with perturbed aerosol loadings. Simulations based on particular, realistic days of the RICO field campaign were run using the Met Office Unified Model in a 500 km × 500 km domain with 500 m resolution, nested in a global driving model. Our findings show that for a large domain without periodic boundary conditions, with realistic synoptic weather subject to large-scale forcing and energy and water budgets, changes in aerosol concentration can have significant effects. The impacts of aerosols on cloud microphysics are sufficient to result in persistent changes in the behaviour of the cloud field. We find that increasing aerosol suppresses the onset of precipitation and leads to deepening and invigoration of convection. Increased aerosol loading results in a suppression of the shallow mode of convection and invigoration of mid-level and deeper clouds. There is little change, however, in the updraught strength at low altitudes, in contrast to the substantially increased updraught speeds higher in the atmosphere. In spite of the convective deepening and invigoration, domain-averaged precipitation is still reduced throughout the simulations, with little discernible change to the frequency of high rain rates. Examination of the thermodynamic structure of the simulations reveals that it is in fact highly resilient and is not significantly affected by the changes to the cloud field.
Previous studies of the effects of aerosols on convection, which have made use of more idealised modelling setups, making use of prescribed forcings and periodic boundaries, have also found invigoration and deepening as a result of increasing aerosol, as well as suppression of the shallowest clouds. However, our results differ from these in several ways. Van den Heever et al. (2011) and Seifert et al. (2015) both find similar suppression and invigoration effects on different parts of the cloud population, as well as on characteristics of the precipitation rate distribution. However, they conclude that the domain-wide effect is minimal, with small impacts on domain-averaged or equilibrium properties. This is in agreement with our own findings for cloud cover but is in contrast to those for precipitation, where we find a persistent decrease in rain rates with higher aerosol. Further, while Seifert et al. (2015) find that reduced cloud cover with higher aerosol compensates for the Twomey effect to produce only a minor change in scene albedo in equilibrium conditions, we find that there is a clear increase in scene albedo with increasing aerosol. Lee et al. (2012) argue that differences in aerosol have significant effects on the thermodynamic environment and development of instability, which in turn affects development of the cloud field. Our findings, however, are of thermodynamic conditions which are not significantly affected by aerosol.
Additionally, Dagan et al. (2017) find that there exists an optimum aerosol loading for convective invigoration and deepening, above which the trend reverses and increasing aerosol leads to suppression. In contrast, we find monotonic deepening and invigoration. Our results here do not preclude the possibility of an optimum loading or turning point, although in this case it is likely that such a point would be far above realistic aerosol concentrations, given the perturbations we applied.
It is important to note that the standard picture of buffering of aerosol effects on shallow convection appears to require some equilibrium state of the cloud field. In idealised simulations this state is reached under different aerosol loadings by affecting the convective development and precipitation characteristics to varying degrees. However, in the real atmosphere with constantly varying cloud fields subject to large-scale advection no such equilibrium exists. Dagan et al. (2018) show that the characteristic timescale of shallow convective cloud fields is less than 12 h, much less than the time required to reach an equilibrium state (Seifert et al., 2015). Here we have presented simulations of such a transient case, which suggest a quite different response: one in which cloud fields do not respond dramatically to restore an equilibrium but instead are altered persistently within the constraints of the transient thermodynamic conditions.
Given the apparent differences between idealised, limited-area large eddy simulations, and those presented here, it seems clear that work is required to elucidate the sources of these differences. LES studies performed on large domains will be necessary, as well as direct comparison of idealised and realistic model setups. It will be important to discern which differences are due to the choice of model and which are due to the idealised or realistic nature of the simulations. In future work we aim to make such a comparison through the use of idealised and realistic configurations of the same model. We hope that the simulations and results we have discussed here will provide a starting point for this direction of investigating anthropogenic perturbations to shallow cumuli, and ultimately the climate.
The simulation data used in this study are available from George Spill upon request and will be made available through the Centre for Environmental Data Analysis (CEDA) Archive.
GS carried out the simulations and analyses presented. PRF assisted with the simulations. PS, GD, and PRF assisted with the design and interpretation of the analyses. GS prepared the manuscript with contributions from all co-authors.
The authors declare that they have no competing interests.
George Spill acknowledges funding from the Natural Environment Research Council with grant reference number 1796357, and from the UK Met Office, and the use of the Monsoon2 system, a collaborative facility supplied under the Joint Weather and Climate Research Programme, a strategic partnership between the Met Office and the Natural Environment Research Council. Guy Dagan and Philip Stier acknowledge funding from the European Research Council project RECAP under the European Union’s Horizon 2020 research and innovation programme with grant agreement no. 724602. Philip Stier additionally acknowledges funding from the Natural Environment Research Council project NE/L01355X/1 (CLARIFY) and from and NE/P013406/1 (A-CURE).
This research has been supported by the Natural Environment Research Council (grant no. 1796357), the Met Office Academic Partnership (grant no. 1796357), the Horizon 2020 (grant no. RECAP (724602)), and the Natural Environment Research Council (grant nos. NE/L01355X/1 and NE/P013406/1).
This paper was edited by Pedro Jimenez-Guerrero and reviewed by two anonymous referees.
Abdul-Razzak, H. and Ghan, S. J.: A parameterization of aerosol activation: 2. Multiple aerosol types, J. Geophys. Res.-Atmos., 105, 6837–6844, https://doi.org/10.1029/1999JD901161, 2000. a
Albrecht, B. A.: Aerosols, Cloud Microphysics, and Fractional Cloudiness, Science, 245, 1227–1230, https://doi.org/10.1126/science.245.4923.1227, 1989. a
Albrecht, B. A.: Effects of precipitation on the thermodynamic structure of the trade wind boundary layer, J. Geophys. Res., 98, 7327–7337, 1993. a
Altaratz, O., Koren, I., Remer, L. A., and Hirsch, E.: Review: Cloud invigoration by aerosols – Coupling between microphysics and dynamics, Atmos. Res., 140, 38–60, https://doi.org/10.1016/j.atmosres.2014.01.009, 2014. a
Bony, S. and Dufresne, J.-L.: Marine boundary layer clouds at the heart of tropical cloud feedback uncertainties in climate models, Geophys. Res. Lett., 32, https://doi.org/10.1029/2005GL023851, 2005. a
Bony, S., Dufresne, J.-L., Le Treut, H., Morcrette, J.-J., and Senior, C.: On dynamic and thermodynamic components of cloud changes, Clim. Dynam., 22, 71–86, https://doi.org/10.1007/s00382-003-0369-6, 2004. a
Boucher, O., Randall, D., Artaxo, P., Bretherton, C., Feingold, G., Forster, P., Kerminen, V.-M., Kondo, Y., Liao, H., Lohmann, U., Rasch, P., Satheesh, S. K., Sherwood, S., Stevens, B., and Zhang, X. Y.: Clouds and aerosols, 571–657, Cambridge University Press, Cambridge, UK, https://doi.org/10.1017/CBO9781107415324.016, 2013. a
Connolly, P. J., Topping, D. O., Malavelle, F., and McFiggans, G.: A parameterisation for the activation of cloud drops including the effects of semi-volatile organics, Atmos. Chem. Phys., 14, 2289–2302, https://doi.org/10.5194/acp-14-2289-2014, 2014. a
Dagan, G., Koren, I., Altaratz, O., and Heiblum, R. H.: Aerosol effect on the evolution of the thermodynamic properties of warm convective cloud fields, Sci. Rep., 6, 38769 EP, https://doi.org/10.1038/srep38769, 2016. a
Dagan, G., Koren, I., Altaratz, O., and Heiblum, R. H.: Time-dependent, non-monotonic response of warm convective cloud fields to changes in aerosol loading, Atmos. Chem. Phys., 17, 7435–7444, https://doi.org/10.5194/acp-17-7435-2017, 2017. a, b
Dagan, G., Koren, I., Altaratz, O., and Lehahn, Y.: Shallow Convective Cloud Field Lifetime as a Key Factor for Evaluating Aerosol Effects, iScience, 10, 192–202, https://doi.org/10.1016/j.isci.2018.11.032, 2018. a
Grosvenor, D. P., Field, P. R., Hill, A. A., and Shipway, B. J.: The relative importance of macrophysical and cloud albedo changes for aerosol-induced radiative effects in closed-cell stratocumulus: insight from the modelling of a case study, Atmos. Chem. Phys., 17, 5155–5183, https://doi.org/10.5194/acp-17-5155-2017, 2017. a, b
Hartmann, D. L., Ockert-Bell, M. E., and Michelsen, M. L.: The Effect of Cloud Type on Earth's Energy Balance: Global Analysis, J. Climate, 5, 1281–1304, https://doi.org/10.1175/1520-0442(1992)005<1281:TEOCTO>2.0.CO;2, 1992. a
Jiang, H. and Feingold, G.: Effect of aerosol on warm convective clouds: Aerosol-cloud-surface flux feedbacks in a new coupled large eddy model, J. Geophys. Res.-Atmos., 111, D01202, https://doi.org/10.1029/2005JD006138, 2005. a
Jiang, H., Feingold, G., and Sorooshian, A.: Effect of Aerosol on the Susceptibility and Efficiency of Precipitation in Warm Trade Cumulus Clouds, J. Atmos. Sci., 67, 3525–3540, https://doi.org/10.1175/2010JAS3484.1, 2010. a
Kaufman, Y. J., Koren, I., Remer, L. A., Rosenfeld, D., and Rudich, Y.: The effect of smoke, dust, and pollution aerosol on shallow cloud development over the Atlantic Ocean, P. Natl. Acad. Sci., 102, 11207–11212, https://doi.org/10.1073/pnas.0505191102, 2005. a
Köhler, H.: The nucleus in and the growth of hygroscopic droplets, Transactions of the Faraday Society, 1152–1161, 1936. a
Koren, I., Dagan, G., and Altaratz, O.: From aerosol-limited to invigoration of warm convective clouds, Science, 344, 1143–1146, https://doi.org/10.1126/science.1252595, 2014. a
Koren, I., Altaratz, O., and Dagan, G.: Aerosol effect on the mobility of cloud droplets, Environ. Res. Lett., 10, 104011, https://doi.org/10.1088/1748-9326/10/10/104011, 2015. a
Lee, S.-S., Feingold, G., and Chuang, P. Y.: Effect of Aerosol on Cloud-Environment Interactions in Trade Cumulus, J. Atmos. Sci., 69, 3607–3632, https://doi.org/10.1175/JAS-D-12-026.1, 2012. a
Medeiros, B., Stevens, B., Held, I. M., Zhao, M., Williamson, D. L., Olson, J. G., and Bretherton, C. S.: Aquaplanets, Climate Sensitivity, and Low Clouds, J. Climate, 21, 4974–4991, https://doi.org/10.1175/2008JCLI1995.1, 2008. a
Medeiros, B., Stevens, B., and Bony, S.: Using aquaplanets to understand the robust responses of comprehensive climate models to forcing, Clim. Dynam., 44, 1957–1977, https://doi.org/10.1007/s00382-014-2138-0, 2015. a
Miltenberger, A. K., Field, P. R., Hill, A. A., Rosenberg, P., Shipway, B. J., Wilkinson, J. M., Scovell, R., and Blyth, A. M.: Aerosol-cloud interactions in mixed-phase convective clouds – Part 1: Aerosol perturbations, Atmos. Chem. Phys., 18, 3119–3145, https://doi.org/10.5194/acp-18-3119-2018, 2018. a
Neggers, R. A. J., Neelin, J. D., and Stevens, B.: Impact Mechanisms of Shallow Cumulus Convection on Tropical Climate Dynamics, J. Climate, 20, 2623–2642, https://doi.org/10.1175/JCLI4079.1, 2007. a
Nuijens, L., Stevens, B., and Siebesma, A. P.: The Environment of Precipitating Shallow Cumulus Convection, J. Atmos. Sci., 66, 1962–1979, https://doi.org/10.1175/2008JAS2841.1, 2009. a
Rauber, R. M., Stevens, B., Ochs, H. T., Knight, C., Albrecht, B. A., Blyth, A. M., Fairall, C. W., Jensen, J. B., Lasher-Trapp, S. G., Mayol-Bracero, O. L., Vali, G., Anderson, J. R., Baker, B. A., Bandy, A. R., Burnet, E., Brenguier, J.-L., Brewer, W. A., Brown, P. R. A., Chuang, R., Cotton, W. R., Di Girolamo, L., Geerts, B., Gerber, H., Göke, S., Gomes, L., Heikes, B. G., Hudson, J. G., Kollias, P., Lawson, R. R., Krueger, S. K., Lenschow, D. H., Nuijens, L., O'Sullivan, D. W., Rilling, R. A., Rogers, D. C., Siebesma, A. P., Snodgrass, E., Stith, J. L., Thornton, D. C., Tucker, S., Twohy, C. H., and Zuidema, P.: Rain in Shallow Cumulus Over the Ocean: The RICO Campaign, B. Am. Meteorol. Soc., 88, 1912–1928, https://doi.org/10.1175/BAMS-88-12-1912, 2007. a
Rossow, W. B. and Schiffer, R. A.: Advances in understanding clouds from ISCCP, B. Am. Meteorol. Soc., 80, 2261–2288, https://doi.org/10.1175/1520-0477(1999)080<2261:AIUCFI>2.0.CO;2, 1999. a
Saleeby, S. M., Herbener, S. R., van den Heever, S. C., and L’Ecuyer, T.: Impacts of Cloud Droplet-Nucleating Aerosols on Shallow Tropical Convection, J. Atmos. Sci., 72, 1369–1385, https://doi.org/10.1175/JAS-D-14-0153.1, 2015. a
Sassen, K. and Wang, Z.: Classifying clouds around the globe with the CloudSat radar: 1-year of results, Geophys. Res. Lett., 35, L04805, https://doi.org/10.1029/2007GL032591, 2008. a
Seifert, A. and Heus, T.: Large-eddy simulation of organized precipitating trade wind cumulus clouds, Atmos. Chem. Phys., 13, 5631–5645, https://doi.org/10.5194/acp-13-5631-2013, 2013. a, b, c
Seifert, A., Köhler, C., and Beheng, K. D.: Aerosol-cloud-precipitation effects over Germany as simulated by a convective-scale numerical weather prediction model, Atmos. Chem. Phys., 12, 709–725, https://doi.org/10.5194/acp-12-709-2012, 2012. a
Seifert, A., Heus, T., Pincus, R., and Stevens, B.: Large-eddy simulation of the transient and near-equilibrium behavior of precipitating shallow convection, J. Adv. Model. Earth Syst., 7, 1918–1937, https://doi.org/10.1002/2015MS000489, 2015. a, b, c, d, e, f, g, h
Seigel, R. B.: Shallow Cumulus Mixing and Subcloud-Layer Responses to Variations in Aerosol Loading, J. Atmos. Sci., 71, 2581–2603, https://doi.org/10.1175/JAS-D-13-0352.1, 2014. a
Sheffield, A. M., Saleeby, S. M., and Heever, S. C.: Aerosol-induced mechanisms for cumulus congestus growth, J. Geophys. Res.-Atmos., 120, 8941–8952, https://doi.org/10.1002/2015JD023743, 2015. a
Shipway, B. J.: Revisiting Twomey's approximation for peak supersaturation, Atmos. Chem. Phys., 15, 3803–3814, https://doi.org/10.5194/acp-15-3803-2015, 2015. a, b, c
Shipway, B. J. and Hill, A. A.: Diagnosis of systematic differences between multiple parametrizations of warm rain microphysics using a kinematic framework, Q. J. Roy. Meteorol. Soc., 138, 2196–2211, https://doi.org/10.1002/qj.1913, 2012. a
Simpson, E., Connolly, P., and McFiggans, G.: An investigation into the performance of four cloud droplet activation parameterisations, Geosci. Model Dev., 7, 1535–1542, https://doi.org/10.5194/gmd-7-1535-2014, 2014. a
Smith, R. N. B.: A scheme for predicting layer clouds and their water content in a general circulation model, Q. J. Roy. Meteorol. Soc., 116, 435–460, https://doi.org/10.1002/qj.49711649210, 1990. a
Stevens, B. and Feingold, G.: Untangling aerosol effects on clouds and precipitation in a buffered system, Nature, 461, 607EP, https://doi.org/10.1038/nature08281, 2009. a, b, c
Stossmeister, G.: GOES-East 1KM RICO-Domain HDF data. Version 1.0, available at: https://data.eol.ucar.edu/dataset/87.021 (last access: 29 May 2018), Data provided by NCAR/EOL under the sponsorship of the National Science Foundation, available at: https://data.eol.ucar.edu/ (last access: 29 May 2018), 2008. a
Tao, W.-K., Chen, J.-P., Li, Z., Wang, C., and Zhang, C.: Impact of aerosols on convective clouds and precipitation, Rev. Geophys., 50, RG2001, https://doi.org/10.1029/2011RG000369, 2012. a
Twomey, S.: The Influence of Pollution on the Shortwave Albedo of Clouds, J. Atmos. Sci., 34, 1149–1152, https://doi.org/10.1175/1520-0469(1977)034<1149:TIOPOT>2.0.CO;2, 1977. a
van den Heever, S. C., Stephens, G. L., and Wood, N. B.: Aerosol Indirect Effects on Tropical Convection Characteristics under Conditions of Radiative-Convective Equilibrium, J. Atmos. Sci., 68, 699–718, https://doi.org/10.1175/2010JAS3603.1, 2011. a, b, c, d
vanZanten, M. C., Stevens, B., Nuijens, L., Siebesma, A. P., Ackerman, A. S., Burnet, F., Cheng, A., Couvreux, F., Jiang, H., Khairoutdinov, M., Kogan, Y., Lewellen, D. C., Mechem, D., Nakamura, K., Noda, A., Shipway, B. J., Slawinska, J., Wang, S., and Wyszogrodzki, A.: Controls on precipitation and cloudiness in simulations of trade-wind cumulus as observed during RICO, J. Adv. Model. Earth Syst., 3, M06001, https://doi.org/10.1029/2011MS000056, 2011. a
Vial, J., Bony, S., Dufresne, J.-L., and Roehrig, R.: Coupling between lower-tropospheric convective mixing and low-level clouds: Physical mechanisms and dependence on convection scheme, J. Adv. Model. Earth Syst., 8, 1892–1911, https://doi.org/10.1002/2016MS000740, 2013. a
Walters, D., Boutle, I., Brooks, M., Melvin, T., Stratton, R., Vosper, S., Wells, H., Williams, K., Wood, N., Allen, T., Bushell, A., Copsey, D., Earnshaw, P., Edwards, J., Gross, M., Hardiman, S., Harris, C., Heming, J., Klingaman, N., Levine, R., Manners, J., Martin, G., Milton, S., Mittermaier, M., Morcrette, C., Riddick, T., Roberts, M., Sanchez, C., Selwood, P., Stirling, A., Smith, C., Suri, D., Tennant, W., Vidale, P. L., Wilkinson, J., Willett, M., Woolnough, S., and Xavier, P.: The Met Office Unified Model Global Atmosphere 6.0/6.1 and JULES Global Land 6.0/6.1 configurations, Geosci. Model Dev., 10, 1487–1520, https://doi.org/10.5194/gmd-10-1487-2017, 2017. a
Xue, H., Feingold, G., and Stevens, B.: Aerosol Effects on Clouds, Precipitation, and the Organization of Shallow Cumulus Convection, J. Atmos. Sci., 65, 392–406, https://doi.org/10.1175/2007JAS2428.1, 2008. a, b
Yuan, T., Remer, L. A., and Yu, H.: Microphysical, macrophysical and radiative signatures of volcanic aerosols in trade wind cumulus observed by the A-Train, Atmos. Chem. Phys., 11, 7119–7132, https://doi.org/10.5194/acp-11-7119-2011, 2011. a
Zhang, Y., Stevens, B., and Ghil, M.: On the diurnal cycle and susceptibility to aerosol concentration in a stratocumulus-topped mixed layer, Q. J. Roy. Meteorol. Soc., 131, 1567–1583, 2005. a
Zhu, P. and Bretherton, C. S.: A Simulation Study of Shallow Moist Convection and Its Impact on the Atmospheric Boundary Layer, Mon. Weather Rev., 132, 2391–2409, https://doi.org/10.1175/1520-0493(2004)132<2391:ASSOSM>2.0.CO;2, 2004. a