the Creative Commons Attribution 4.0 License.
the Creative Commons Attribution 4.0 License.
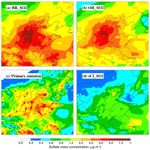
Effects of stabilized Criegee intermediates (sCIs) on sulfate formation: a sensitivity analysis during summertime in Beijing–Tianjin–Hebei (BTH), China
Lang Liu
Naifang Bei
Jiarui Wu
Suixin Liu
Jiamao Zhou
Xia Li
Qingchuan Yang
Tian Feng
Junji Cao
Xuexi Tie
Guohui Li
Sulfate aerosols have profound impacts on the climate, ecosystem, visibility, and public health, but the sulfate formation pathway remains elusive. In the present study, a source-oriented WRF-Chem model is applied to simulate a persistent air pollution episode from 4 to 15 July 2015 in Beijing–Tianjin–Hebei (BTH), China, to study the contributions of four pathways to sulfate formation. When comparing simulations to measurements in BTH, the index of agreement (IOA) of meteorological parameters, air pollutants, and aerosol species generally exceeds 0.6. On average in BTH, the heterogeneous reaction of SO2 involving aerosol water and the SO2 oxidation by OH constitutes the two most important sulfate sources, with a contribution of about 35 %–38 % and 33 %–36 %, respectively. Primary sulfate emissions account for around 22 %–24 % of the total sulfate concentration. SO2 oxidation by stabilized Criegee intermediates (sCIs) also plays an appreciable role in sulfate formation, with a contribution of around 9 % when an upper limit of the reaction rate constant of sCIs with SO2 ( cm3 s−1) and a lower limit of the reaction rate constant of sCIs with H2O ( cm3 s−1) are used. Sensitivity studies reveal that there are still large uncertainties in the sulfate contribution of SO2 oxidation by sCIs. The sulfate contribution of the reaction is decreased to less than 3 % when is decreased to cm3 s−1. Furthermore, when is increased to cm3 s−1 based on the reported ratio of to (), the sulfate contribution becomes insignificant at less than 2 %. Further studies need to be conducted to better determine and to evaluate the effects of sCI chemistry on sulfate formation.
As a major component of fine particulate matter (PM2.5) in the atmosphere, sulfate aerosols not only directly and indirectly influence regional and global climate, but they also impair the ecosystem, visibility, and potentially public health (e.g., Wang and Hao, 2012; Guo et al., 2014; Gao et al., 2016; Tao et al., 2017). Sulfate aerosols are primarily formed through homogeneous and heterogeneous oxidations of sulfur dioxide (SO2) emitted from anthropogenic and natural sources (Seinfeld and Pandis, 2006). The sulfate formation pathway via SO2 oxidation includes aqueous reactions in cloud or fog droplets, heterogeneous reactions associated with aerosol water, and gas-phase reactions with hydroxyl radicals (OH) and stabilized Criegee intermediates (sCIs) (Seinfeld and Pandis, 2006; Wang et al., 2016; Li et al., 2017). Recent studies have revealed that SO2 oxidation by sCIs could constitute an important sulfate source in the atmosphere (Welz et al., 2012; Mauldin et al., 2012; Boy et al., 2013; Pierce et al., 2013; Percival et al., 2013).
Carbonyl oxide intermediates formed in the ozonolysis reaction of alkenes, often known as sCIs, are proposed to be important radicals in the atmosphere. In the gas phase, sCIs can act as an additional atmospheric oxidant. Laboratory studies in the 1970s showed that SO2 oxidation is enhanced in the presence of alkenes and ozone, providing the first evidence that sCIs could react with SO2 (Cox and Penkett, 1971). In the 2010s, Welz et al. (2012) used photoionization mass spectrometry to make the first direct measurement of individual sCI isomers. They found that the reaction rate of the simplest sCI, H2COO, with SO2 is faster than expected by up to 3 orders of magnitude, whereas the removal of sCIs by water vapor is comparatively slow. The result has also indicated that sCI chemistry potentially contributes substantially to SO2 oxidation and has profound effects on sulfate formation. Based on laboratory experiments and theoretical considerations, Mauldin et al. (2012) reported that the reaction rate of sCIs originated from the ozonolysis reaction of α-pinene and limonene with SO2 under boundary layer atmospheric conditions. The new reaction rates are slower than those found in Welz et al. (2012) but still about 1 order of magnitude faster than previously used (Jenkin et al., 1997).
Further studies have been conducted to evaluate the contributions of SO2 oxidation by sCIs to the sulfate in the atmosphere based on the results of Welz et al. (2012) and Mauldin et al. (2012). Boy et al. (2013) examined the effects of the increased reaction rate of sCIs with SO2 on the atmospheric sulfuric acid (H2SO4) concentration at two stations, showing that the reaction contributes as much as 33 %–46 % of H2SO4 concentrations at the ground level. Using the results of Welz et al. (2012), Sarwar et al. (2013) showed that SO2 oxidation by sCIs does not substantially influence sulfate concentrations in the USA due to the competing reaction of sCIs with water vapor. However, when using the high reaction rate constant of sCIs with SO2 () and the low reaction rate constant of sCIs with H2O () simultaneously, SO2 oxidation by sCIs considerably enhances sulfate formation (Sarwar et al., 2014). Li et al. (2013) demonstrated that SO2 oxidation by sCIs contributes about 18 % of the sulfate concentration during summertime in the eastern USA when using the reported by Welz et al. (2012). Pierce et al. (2013) used the same in simulations of the GEOS-Chem model, showing that the reaction increases H2SO4 production globally by 4 %, and the induced H2SO4 enhancement is almost entirely distributed over forested continental regions with large fluxes of biogenic alkene emissions.
With rapid industrialization and urbanization, heavy air pollution with high levels of PM2.5 and/or ozone (O3) frequently occurs in Beijing–Tianjin–Hebei (BTH), and sulfate aerosols have become a main component of PM2.5 (e.g., Zhang et al., 2012; Zhao et al., 2013; Sun et al., 2015; Li et al., 2017; Wu et al., 2017). Considering the high alkene emissions and increasing trend of O3 concentrations during summertime in BTH, it is imperative to assess the effects of sCI chemistry on sulfate formation. In the present study, a source-oriented WRF-Chem model has been developed and applied to study the contribution of different pathways to sulfate formation in BTH during the summer of 2015. The model configuration and methodology are described in Sect. 2. Results and a discussion are presented in Sect. 3. The conclusions and a summary are drawn in Sect. 4.
2.1 WRF-Chem model and configuration
A specific version of the WRF-Chem model (Grell et al., 2005) developed by Li et al. (2010, 2011a, b, 2012) at the Molina Center for Energy and the Environment is used in the present study. A detailed model description can be found in previous studies (Li et al., 2018; Wu et al., 2017; Feng et al., 2016; Xing et al., 2019). Briefly, the model includes a new flexible gas-phase chemical module and the Community Multi-scale Air Quality (CMAQ) aerosol module developed by the US EPA (Binkowski and Roselle, 2003). The wet deposition uses the method in the CMAQ module, and the dry deposition of chemical species is parameterized following Wesely (1989). The photolysis rates are calculated using the Fast Tropospheric Ultraviolet and Visible Radiation Model (FTUV; Li et al., 2005; Tie et al., 2003), with the aerosol and cloud effects on the photochemistry (Li et al., 2011a). The ISORROPIA version 1.7 is applied to calculate the inorganic components (Nenes et al., 1998). The secondary organic aerosol (SOA) is simulated using a nontraditional module, including the volatility basis set (VBS) modeling approach and SOA contributions from glyoxal and methylglyoxal. The anthropogenic emission inventory with a horizontal resolution of 6 km is developed by Zhang et al. (2009), with the base year of 2013, including industry, transportation, power plant, residential, and agriculture sources. The Model of Emissions of Gases and Aerosols from Nature (MEGAN) is used to calculate the biogenic emissions online (Guenther et al., 2006).
Traditionally, the brute force method (BFM) is generally used to quantify the formation pathway of particulate matter and chemical compounds in modeling studies (Dunker et al., 1996). The BFM evaluates the importance of a certain formation pathway by including and excluding the pathway in simulations, but it lacks a consideration of interactions of the complicated physical and chemical processes in the atmosphere (Zhang and Ying, 2011). The source-oriented method introduces additional chemical species to represent formations from different pathways, providing a direct and quantitative determination of the contributions of different pathways (Ying and Krishnan, 2010). Coupled source-oriented air quality models have been widely used to study the source apportionment of particulate matter and chemical compounds. A detailed description of the method can be found in previous studies (Ying and Kleeman, 2006; Ying and Krishnan, 2010; Zhang and Ying, 2011). In the present study, four reactive tagged species are introduced to track sulfate formation pathways.
A persistent air pollution episode with high levels of O3 and PM2.5 from 4 to 15 July 2015 in BTH is simulated in association with the observation of air pollutants and secondary aerosols. Detailed information about the episode can be found in Wu et al. (2017). Figure 1 shows the WRF-Chem model simulation domain, and Table 1 presents the model configuration.
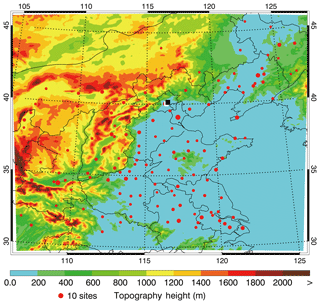
Figure 1WRF-Chem simulation domain with topography height. The red filled circles show the locations of the cities with ambient air quality monitoring sites, and the size of the circles represents the number of sites in each city. The white and black filled rectangles denote the weather station and the NCNST observation site in Beijing.
2.2 Simulations for sulfate aerosols
Four sulfate formation pathways are considered in the WRF-Chem model, including (1) the heterogeneous reaction of SO2 involving aerosol water (hereafter referred to as HR_SO2), (2) SO2 oxidation by OH (hereafter referred to as OH_SO2), (3) primary emissions, and (4) SO2 oxidation by sCIs (hereafter referred to as sCI_SO2). The sulfate formed in the four pathways is tagged and traced in the model to study their contributions to sulfate formation. It is worth noting that the WRF-Chem model cannot resolve clouds formed in the planetary boundary layer (PBL) well, so aqueous SO2 oxidation in cloud or fog droplets is not considered in the study, which might cause sulfate underestimation; on the other hand, it might overestimate the contributions of the four pathways to the sulfate concentration. The HR_SO2 is parameterized as a 1st-order irreversible uptake of SO2 by aerosol water, with a reactive uptake coefficient of , assuming that alkalinity is sufficient to maintain the high iron-catalyzed reaction rate in BTH (Li et al., 2017). Aerosol hygroscopic growth is directly predicted by ISORROPIA in the model, and the aerosol water surface area is scaled from the calculated wet aerosol surface area using the third moment of aerosol species.
The effects of sCI chemistry on sulfate formation depend on and , as well as the sCI precursor concentration. In the study, sCIs are assumed to result from the ozonolysis reaction of five alkenes based on the SAPRC99 mechanism, including ethene (ETHE), terminal olefin (OLE1), internal olefin (OLE2), isoprene (ISOP), and monoterpenes (TERPs). Detailed information about the sCI chemistry associated with sulfate formation can be found in Table 2.
CH2OO (sCI1) is used to represent sCIs produced from the ozonolysis reaction of ETHE and OLE1, and the sCI yield of the two reactions is described in Sarwar et al. (2013). CH3CHOO (sCI2) is formed from the ozonolysis reaction of OLE2 and proposed to have two isomers: syn-CH3CHOO and ant-CH3CHOO (Anglada et al., 2011). The reported reaction rate constants of syn-CH3CHOO and ant-CH3CHOO with H2O are and cm3 s−1, respectively. We use syn-CH3CHOO (sCI2) to represent sCIs from the ozonolysis reaction of OLE2 to minimize the removal of sCIs by water vapor and maximize sulfate production following Ying et al. (2014). sCI3 is used to represent sCIs from the ozonolysis reaction of isoprene and monoterpenes, and the detailed chemistry of sCI3 (syn-CH3-anti-(cis-CH=CH2)CHOO) is described in Sarwar et al. (2013, 2014).
In the base case (hereafter referred to as the B case) simulation used to compare with observations in BTH, we use a single reported by Welz et al. (2012) for reactions of SO2 with . For the removal of by water vapor, we employ the suggested by Ying et al. (2014). While not important, the reaction of with NO2 is also implemented in the model, and the rate constant is taken from Welz et al. (2012).
2.3 Observations
Simulations are compared to available meteorological and air pollutant observations to validate the model performance. The meteorological parameters, including surface temperature (TSFC), relative humidity (RH), and wind speed and direction with a 3 h interval, are obtained from the website http://www.meteomanz.com (last access: 28 October 2019). The hourly measurements of PM2.5, O3, SO2, and NO2 used in this study are downloaded from the website http://www.aqistudy.cn (last access: 28 October 2019). Submicron sulfate, nitrate, ammonium, and organic particulate matter are observed by the Aerodyne Aerosol Chemical Speciation Monitor (ACSM) at the National Center for Nanoscience and Technology (NCNST), Chinese Academy of Sciences, Beijing (116.39∘ E, 39.99∘ N). A detailed description of the methods to obtain the primary organic aerosol (POA) and secondary organic aerosol (SOA) mass concentration from the results of ACSM are given in Wu et al. (2017). Figure 1 shows the locations of the ambient air quality monitoring sites and the NCNST observation site.
2.4 Statistical methods for model evaluation
In this study, the mean bias (MB), root mean square error (RMSE), and index of agreement (IOA) are used to evaluate the model performance.
Pi and Oi are the simulated and observed variables, respectively. N is the total number of simulations for comparison, and denotes the average of the observation. The IOA ranges from 0 to 1, with 1 showing a perfect agreement of the simulation with the observation.
3.1 Model evaluation
3.1.1 Meteorological parameter simulations in Beijing
Considering the key role of meteorological conditions in air pollution simulations (Bei et al., 2012, 2017), Fig. 2 shows the temporal profiles of observed and simulated TSFC, RH, wind speed, and wind direction from 4 to 15 July 2015 at the weather station in Beijing (Fig. 1). The WRF-Chem model generally replicates the temporal variation of the TSFC well during the whole episode compared to observations, with an MB and IOA of 0.7 ∘C and 0.95, respectively. The model considerably overestimates TSFC on 4 July and in the evening of 13 and 14 July. The model also performs reasonably well in simulating the RH against observations, with an MB and IOA of −5.3 % and 0.84, respectively. Observed high RH exceeding 75 % during nighttime is generally well captured, except on 4 and 14 July when the TSFC is overestimated. In addition, the model also tracks the temporal variations of the wind speed and direction reasonably well compared to the observations, with an IOA of around 0.60. In general, the reasonable simulations of meteorological fields provide a reliable basis for modeling the O3 and PM2.5 pollution episode in the present study.
3.1.2 Air pollutant simulations in BTH
Figure 3 shows the diurnal profiles of measured and simulated PM2.5, O3, NO2, SO2, and CO mass concentrations averaged over all ambient monitoring stations in BTH during the episode. The WRF-Chem model exhibits good performance in simulating the temporal variations of PM2.5 and O3 mass concentrations against observations in BTH, with IOAs of around 0.90. However, the model fails to capture the observed high PM2.5 concentration on 11 and 12 July and frequently overestimates the O3 concentration in the evening, with an MB of 1.2 µg m−3. The simulated temporal variation of NO2 mass concentrations is also generally consistent with observations in BTH, but the model frequently overestimates NO2 concentrations against observations during nighttime, which might be caused by the low simulated planetary boundary layer (PBL) height or the O3 overestimation. Simulations of the SO2 mass concentration are not as good as those of other pollutants in BTH during the episode, with an IOA of 0.45. During summertime, SO2 is principally emitted by point sources, including power plants and agglomerated industrial zones, so the uncertainties of simulated wind fields substantially affect the SO2 simulations. Additionally, the model overestimation of SO2 concentrations is also considerable during nighttime, which is perhaps due to the simulated low PBL height. It is worth noting that NH3 plays an important role in sulfate formation (Wang et al., 2016; Cheng et al., 2016), so it is imperative to validate NH3 simulations using measurements. However, due to a lack of routine measurements of NH3 in BTH, a validation of the NH3 simulation is not provided in the study.
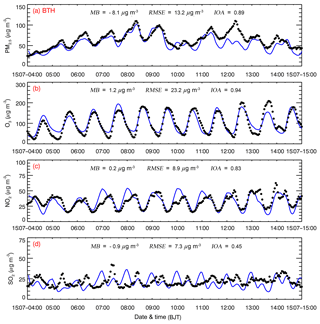
Figure 3Comparison of observed (black dots) and simulated (blue line) diurnal profiles of near-surface hourly (a) PM2.5, (b) O3, (c) NO2, and (d) SO2 averaged over all ambient monitoring stations in BTH from 4 to 15 July 2015.
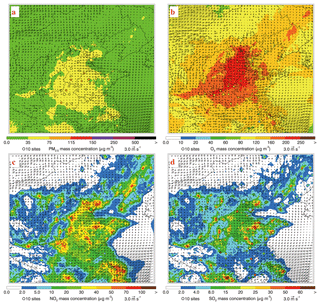
Figure 4Spatial distributions of average (a) PM2.5, (b) peak O3, (c) NO2, and (d) SO2 mass concentrations from 4 to 15 July 2015. Colored dots, colored contour, and black arrows are observations of air pollutants, simulations of air pollutants, and simulated surface winds, respectively.
Figure 4 presents the distributions of simulated and observed near-surface mass concentrations of PM2.5, O3, NO2, and SO2 along with the simulated wind fields averaged from 4 to 15 July 2015. Generally, the simulated wind in BTH is weak during the episode and the easterly wind prevails, which is favorable for the accumulation of air pollutants, particularly along the Taihang and Yan Mountains due to the blocking effect. The model generally reproduces the spatial distribution of PM2.5 concentrations well against observations, with the PM2.5 concentration exceeding 35 µg m−3 in the plain area of BTH (Fig. 4a). The average simulated peak O3 concentrations are more than 200 µg m−3 during the episode in the plain area of BTH, consistent with measurements showing severe O3 pollution (Fig. 4b). High levels of O3 indicate a strong atmospheric oxidation capacity (AOC) facilitating photochemical reactions over BTH (Fig. 4b). The simulated high NO2 and SO2 concentrations are generally concentrated in cities and their surrounding areas, in agreement with the measurements (Fig. 4c and d). However, the model considerably overestimates the NO2 concentrations against measurements in Beijing, Shijiazhuang, and Handan city. In addition, the SO2 concentrations in BTH are much lower than those during wintertime (Li et al., 2018; Xing et al., 2019), generally less than 30 µg m−3. Reduced SO2 concentrations in BTH during summertime are caused by the efficient removal of gas-phase oxidation due to the high AOC, the reduction of residential coal combustion, and the increased PBL height.
3.1.3 Aerosol species simulations in Beijing
Figure 5 presents the temporal variations of simulated and observed submicron nitrate, ammonium, SOA, and POA mass concentrations at the NCNST site in Beijing from 4 to 15 July 2015. The WRF-Chem model reasonably reproduces the diurnal variation of the nitrate concentration compared to observations, with an MB and IOA of −0.7 µg m−3 and 0.81, respectively (Fig. 5a). Nitrate formation is sensitive to the air temperature, and its variation is generally negatively correlated with that of temperature. When the temperature is lowest in the early morning, the nitrate concentration reaches its peak; when the temperature is up to 30 ∘C in the afternoon, the nitrate concentration is generally less than 1 µg m−3. The simulated ammonium profile is generally in agreement with observations, with an IOA of 0.71, but the model biases are still large. The model underestimation of the ammonium concentration is considerable on 11 and 12 July, and the overestimation is also noticeable on 6 and 7 July. The model reasonably reproduces the temporal variation of the SOA and POA concentrations compared to measurements at the NCNST site, with an IOA of around 0.60. The observed SOA concentration exhibits rather large fluctuations from 11 to 15 July 2015, which are not well tracked by the model. The model fails to capture the observed large fluctuations of POA concentrations. The POA concentration in Beijing is primarily contributed by direct emissions from vehicles, cooking, coal combustion, biomass burning, and transboundary transport from outside Beijing (Wu et al., 2017, 2018), and the uncertainties in various anthropogenic sources and simulated meteorological fields substantially affect the POA simulations (Bei et al., 2017, 2016).
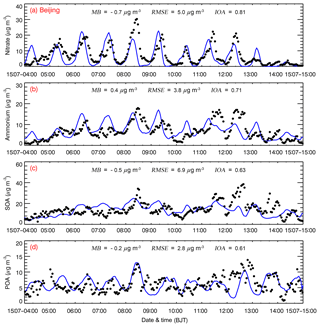
Figure 5Comparison of observed (black dots) and simulated (blue line) diurnal profiles of hourly submicron (a) nitrate, (b) ammonium, (c) SOA, and (d) POA mass concentrations at the NCNST site in Beijing from 4 to 15 July 2015.
In summary, the WRF-Chem model performs reasonably well in simulating meteorological fields, air pollutants, and aerosol species, providing an underlying basis for further evaluation of the sulfate formation pathway.
3.2 Contributions of four pathways to sulfate formation in Beijing and BTH
Figure 6a provides the diurnal profile of simulated and observed submicron sulfate concentrations at the NCNST site in Beijing from 4 to 15 July 2015. The model tracks temporal variations of the observed sulfate concentration reasonably well, with an MB and IOA of −0.9 µg m−3 and 0.71, respectively. During 11 to 12 July 2015, the model noticeably underestimates the high sulfate concentrations against the measurements. As mentioned in Sect. 2, the aqueous oxidation of SO2 in cloud or fog droplets is not considered in the simulation, which might be one of the reasons for the underestimation.
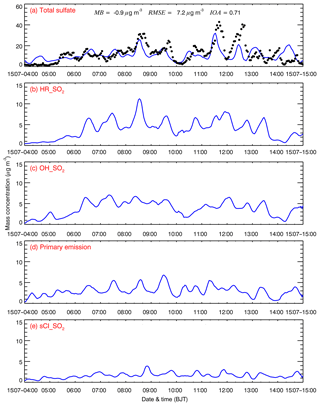
Figure 6(a) Comparison of observed (black dots) and simulated (blue line) diurnal profiles of the hourly submicron sulfate mass concentration and the temporal variation of the simulated sulfate contribution of (b) HR_SO2, (c) OH_SO2, (d) primary emissions, and (e) sCI_SO2 to the sulfate concentration at the NCNST site in Beijing from 4 to 15 July 2015.
Figure 6b–e present the contributions of the four pathways to sulfate formation during the episode at the NCNST site in Beijing. On average, HR_SO2 plays the most important role in sulfate formation, with a contribution of about 32.1 % (Fig. 6b). Li et al. (2017) have also shown that HR_SO2 is the dominant sulfate source, contributing around 58.4 % to sulfate concentrations in Beijing during wintertime due to the very humid conditions and inefficient sulfate formation from gas-phase SO2 oxidation due to the low AOC. In this study, the average simulated RH is not high in Beijing, less than 50 % during the episode, and high O3 concentrations enhance the AOC to facilitate gas-phase SO2 oxidation, causing the decreased sulfate contribution of HR_SO2. It is worth noting that HR_SO2 relies on the assumption that alkalinity is sufficient to maintain the high iron-catalyzed reaction rate (Li et al., 2017). Figure 7 presents the temporal variation of the average simulated aqueous pH in Beijing during the episode. The simulated pH generally fluctuates between 5 and 7, with an average of 6.2, demonstrating an efficient iron-catalyzed reaction involving aerosol water. High O3 concentrations substantially increase sulfate formation efficiency through SO2 oxidation by OH and sCIs. OH_SO2 play a considerable role in sulfate formation, with a contribution of 30.8 % (Fig. 6c). It is worth noting that the primary emission pathway contributes 24.0 % of the sulfate concentration at the NCNST site.
The sCI_SO2 accounts for about 13.1 % of sulfate concentrations at the NCNST site, which is less than the contribution of the other three pathways. The effects of sCI_SO2 on sulfate formation depend on the . The reported from previous studies spans orders of magnitude (Welz et al., 2012; Maudlin et al., 2012; Jenkin et al., 1997). In the present study, an upper limit of ( cm3 s−1) is used in the B case, which is reported by Welz et al. (2012). Furthermore, the reaction of sCIs with H2O is the main loss pathway for sCIs in the atmosphere, and the used in this study represents a lower limit ( cm3 s−1) (Ying et al., 2014). Therefore, the contribution of sCI_SO2 to sulfate formation might be overestimated in the present study.
Figure 8b–e present the simulated temporal variations of the four pathways to the sulfate concentration averaged over the whole BTH from 4 to 15 July 2015. On average, HR_SO2 is still the dominant sulfate source, with a contribution of 35.3 % (Fig. 8b). OH_SO2 plays an important role in sulfate formation, accounting for 33.1 % of sulfate mass (Fig. 8c). The primary emission pathway and sCI_SO2 contribute 22.5 % and 9.1 % of the sulfate concentration, respectively (Fig. 8d and e).
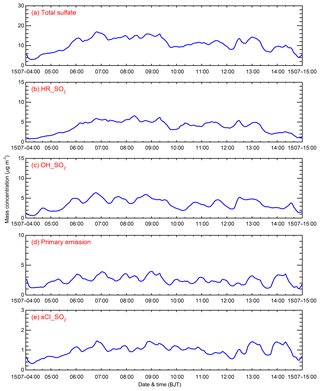
Figure 8(a) Simulated diurnal profiles of the total sulfate mass concentration and contributions of (b) HR_SO2, (c) OH_SO2, (d) primary emissions, and (e) sCI_SO2 to the sulfate concentration averaged over BTH from 4 to 15 July 2015.
Figure 9 presents the spatial distribution of the contributions of the four pathways to sulfate formation averaged during the episode. The contribution of HR_SO2 to sulfate formation is substantial in BTH, particularly in the plain area, with the sulfate contribution exceeding 4.0 and being up to 7.0 µg m−3 (Fig. 9a). Under conditions of high O3 concentrations, OH_SO2 oxidation also becomes an important sulfate source, with a sulfate contribution of more than 3.0 µg m−3 in the plain area of BTH, which is comparable to the heterogeneous pathway (Fig. 9b). Similar to the spatial distribution of SO2 concentrations, the sulfate contribution of primary emissions is mainly concentrated in cities and their downwind areas (Fig. 4c). In the plain area of BTH, the sulfate contribution of primary emissions is more than 2.0 µg m−3 on average and exceeds 7 µg m−3 in highly industrialized cities, such as Shijiazhuang, Tangshan, and Xingtai. The sulfate contribution of sCI_SO2 is not as important as the other three sources; it is more than 0.8 µg m−3 in the plain area of BTH and the most striking in Beijing, with a contribution exceeding 1.2 µg m−3 (Fig. 9d).
3.3 Sensitivity studies
Figure 10 shows the diurnal profiles of sCI1, sCI2, and sCI3 concentrations in BTH from 4 to 15 July 2015. The average concentration of sCI1, sCI2, and sCI3 is 1.8, 2.0, and 1.9×104 molecules cm−3, respectively. Novelli et al. (2017) estimated the concentration of sCIs in the lower troposphere based on observations in a boreal forest in Finland and in rural southern Germany. The results show that the average concentration of sCIs is about 5.0×104 molecules cm−3 with an order of magnitude uncertainty, generally consistent with our study. Dominant sCI peaks frequently occur during nighttime, mainly caused by the low PBL facilitating the accumulation of alkenes and sCIs as well as low reaction rates of sCIs with other species (Smith et al., 2015; Stone et al., 2014; Taatjes, 2017). It is worth noting that sCIs are predominantly quenched by reactions with water vapor in the atmosphere.
Considering the large variation of the reported and the importance of water vapor in sCI removal, sensitivity studies are further conducted to evaluate the sulfate contribution of the sCI_SO2 in BTH when the different and are used in simulations. In the B-case simulation, an upper limit of and a lower limit of are used. The simulated average sulfate concentration is 10.8 µg m−3 in BTH, constituting a major component of PM2.5. HR_SO2 and OH_SO2 constitute the two most important sulfate sources, with a sulfate contribution of 35 % and 33 %, respectively. Primary emissions make up about 23 % of the sulfate in BTH, caused by high SO2 emissions. The sulfate contribution of sCI_SO2 is about 1.0 µg m−3 or 9.1 %, which is less than that in Beijing with a higher O3 concentration. Pierce et al. (2013) used the same in the GEOS-Chem model as the B case in this study, revealing that the H2SO4 concentration is increased by 4 % due to sCI_SO2 on global average. The H2SO4 enhancement is 10 %–25 % over forested regions in the Northern Hemisphere (up to 100 % in July), but it is generally negligible elsewhere. The sulfate contribution of sCI_SO2 in BTH is close to that over forested regions in Pierce et al. (2013), which is primarily caused by the increasing trend of O3 and the high precursor emissions of sCIs such as ethene, isoprene, and monoterpenes during summertime in the region. Additionally, Boy et al. (2013) employed the reported by Mauldin et al. (2012) and Welz et al. (2012) to verify the sulfate contribution of sCI_SO2 in Europe, showing an H2SO4 contribution of as much as 33 %–46 % at the ground level. These different sulfate contributions of sCI_SO2 are mainly caused by the variation of the reaction constant of sCIs with SO2, NO2, and H2O, as well as the sCI precursor concentration and the atmospheric conditions in the simulations (Taatjes, 2017).
Although sCI_SO2 is not an important sulfate source, its contribution might be overestimated. The used in the B case is only measured for the smallest sCI, H2COO, but the larger sCIs (such as those produced from typical larger alkenes in the atmosphere) might have a lower reaction rate with SO2 and produce stable low-volatility species such as sulfur-bearing secondary ozonides (Spracklen et al., 2011; Vereecken et al., 2012). Additionally, Welz et al. (2012) measured at low pressure (4 Torr), making it unclear if those rates are appropriate for atmospheric conditions.
In the S1 case, the reported ( cm3 s−1) by Mauldin et al. (2012) is used, which is deduced from the ozonolysis of α-pinene under boundary layer atmospheric conditions, and the and are the same as those in the B case. The sulfate contribution of sCI_SO2 becomes insignificant; it is around 0.3 µg m−3 or less than 3 % of the total simulated sulfate concentration on average during the episode in BTH (Fig. 11). Compared to the B case, the sulfate contribution of sCI_SO2 is decreased by more than 70 % in the S1 case. The substantial reduction reveals that further studies are needed to precisely determine .
One of the largest uncertainties concerning the lifetime of sCIs is related to , but few studies have been conducted to directly measure . The reported varies widely, ranging from to cm3 s−1 (Hatakeyama and Akimoto, 1994), and several studies show that needs to be adjusted when is adjusted (Li et al., 2013; Calvert et al., 1978; Suto et al., 1985). In order to evaluate the effect of water vapor on sCI_SO2, in the S2 case, is increased to cm3 s−1 based on the reported ratio of to () (Calvert et al., 1978), and the and are the same as those in the B case. The average sulfate contribution of sCI_SO2 in BTH is decreased to 0.2 µg m−3 or less than 2 % of the total simulated sulfate concentration due to the competition of water vapor with SO2 for sCIs (Fig. 11). Additionally, if a low is used in the S2 case, the effect of water vapor on sCI removal becomes more substantial. In the S1 case and S2 case, there is a dominant peak of sulfate concentrations on 10 July, which might be caused by the reaction rate constants used in the two cases. We have performed an additional sensitivity study (S3 case), in which the reported ( cm3 s−1) and ( cm3 s−1) suggested by Stone et al. (2014) and Smith et al. (2015) are used. The results show that the average sulfate contribution of sCI_SO2 becomes more insignificant; it is about 0.06 µg m−3 or less than 0.6 %. However, there is no obvious peak around 10 July (Fig. 11), indicating that the large uncertainty in contributions of sCI_SO2 to sulfate mass is due to the different value of and used in the sensitivity studies.
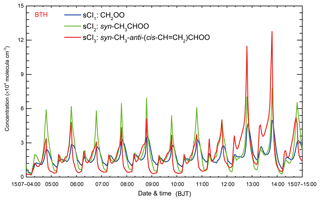
Figure 10Temporal variations of the simulated concentration of different sCIs (blue line: sCI1; green line: sCI2; red line: sCI3) in BTH from 4 to 15 July 2015.
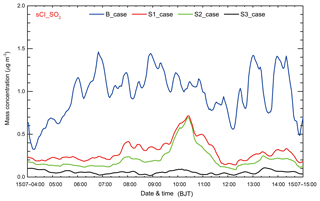
Figure 11Temporal variations of the simulated average sulfate concentration contributed by sCI_SO2 (blue line: B case; red line: S1 case; green line: S2 case; black line: S3 case) in BTH from 4 to 15 July 2015.
It is worth noting that the uncertainty in HR_SO2, as the most important sulfate source, also influences the sulfate contribution of sCI_SO2. Several factors influence the heterogeneous reactions of SO2 on aerosol surfaces, including aerosol water surface area, aerosol acidity, and organic coating. In the B case, the predicted average aerosol liquid water and wet surface areas are 18.8 µg m−3 and m2 m−3 in the BTH during the episode, and the uptake coefficient of SO2 by aerosols (γ) is assumed as . To investigate the sensitivity of sCI_SO2 sulfate contributions to uncertainties in HR_SO2, we perform sensitivity simulations with γ of and . The sulfate concentration of HR_SO2 is considerably affected by the variation of γ, with an average change of −18.3 % and 25.6 % in BTH during the episode when the γ is assumed as and , respectively. However, the effects of the γ change on the sulfate contributions of sCI_SO2 are not significant, with a change of −4.4 % and 3.9 % when the γ is assumed as and , respectively.
In the present study, a persistent air pollution episode with high O3 and PM2.5 concentrations from 4 to 15 July 2015 in BTH is simulated using a source-oriented WRF-Chem model to study the contributions of four pathways to sulfate formation. The four sulfate formation pathways include the heterogeneous reaction of SO2 involving aerosol water (HR_SO2), SO2 oxidation by OH (OH_SO2), primary emissions, and SO2 oxidation by sCIs (sCI_SO2).
The WRF-Chem model reasonably reproduces the temporal variations of the meteorological parameters compared to observations at the weather station in Beijing. The model performs reasonably well in simulating the temporal profiles and spatial distributions of air pollutant mass concentrations against observations at monitoring sites in BTH. In addition, the simulated diurnal variations of submicron nitrate, ammonium, POA, SOA, and sulfate mass concentrations are generally in good agreement with the measurements at the NCNST site in Beijing.
On average in BTH during the simulation episode, HR_SO2 plays the most important role in sulfate formation, with a sulfate contribution of about 35 %. Under conditions of high O3 concentrations during summertime, OH_SO2 also constitutes a major sulfate source comparable to HR_SO2, accounting for about 33 % of the total simulated sulfate concentration in BTH. Due to high SO2 emissions, primary emissions contribute about 23 % of the sulfate concentration in BTH, mainly concentrated in cities and their downwind regions.
When an upper limit for ( cm3 s−1) and a lower limit for ( cm3 s−1) are used, sCI_SO2 plays an appreciable role in sulfate formation, with a contribution of around 9 %. However, there are still large uncertainties in the contributions of sCI_SO2 to sulfate formation. Sensitivity studies reveal that the sulfate contribution of sCI_SO2 is substantially decreased to less than 3 % when is decreased to cm3 s−1 but the lower limit of remains. Furthermore, when is increased to cm3 s−1 based on the reported ratio of to ( cm3 s−1) but the upper limit of remains, the sulfate contribution of the reaction becomes insignificant at less than 2 %. Future studies still need to be conducted to measure and under atmospheric conditions to better evaluate the effects of sCI chemistry on sulfate formation. Additionally, as a potentially important atmospheric oxidant, sCIs may enhance ozone formation and ultimately OH formation. The indirect effects of sCIs on sulfate formation by promoting the OH_SO2 pathway should also be investigated further.
Real-time PM2.5, O3, NO2, SO2, and CO are accessible for the public on the website http://106.37.208.233:20035 (China MEP, 2013a). One can also access the historic profile of observed ambient pollutants by visiting http://www.aqistudy.cn (China MEP, 2013b).
GL, as the contact author, provided the ideas and financial support, verified the conclusions, and revised the paper. LL conducted research, designed the experiments, carried out the methodology, performed the simulation, processed the data, prepared the data visualization, and prepared the paper with contributions from all authors. NB, JW, and XL provided the treatment of meteorological data, analyzed the study data, validated the model performance, and reviewed the paper. SL, QY, TF, and JZ provided the observation data used in the study, synthesized the observation, and reviewed the paper. XT and JC provided critical reviews in the pre-publication stage.
The authors declare that they have no conflict of interest.
This article is part of the special issue “Regional transport and transformation of air pollution in eastern China”. It is not associated with a conference.
This work is financially supported by the National Key R&D Plan (Quantitative Relationship and Regulation Principle between Regional Oxidation Capacity of Atmospheric and Air Quality (2017YFC0210000)) and the National Research Program for Key Issues in Air Pollution Control (DQGG0105).
This research has been supported by the National Key R&D Plan (grant no. 2017YFC0210000) and the National Research Program for Key Issues in Air Pollution Control (grant no. DQGG0105).
This paper was edited by Yuan Wang and reviewed by three anonymous referees.
Anglada, J. M., González, J., and Torrent-Sucarrat, M.: Effects of the substituents on the reactivity of carbonyl oxides. A theoretical study on the reaction of substituted carbonyl oxides with water, Phys. Chem. Chem. Phys., 13, 13034–13045, https://doi.org/10.1039/C1CP20872A, 2011.
Bei, N., Li, G., and Molina, L. T.: Uncertainties in SOA simulations due to meteorological uncertainties in Mexico City during MILAGRO-2006 field campaign, Atmos. Chem. Phys., 12, 11295–11308, https://doi.org/10.5194/acp-12-11295-2012, 2012.
Bei, N., Li, G., Huang, R.-J., Cao, J., Meng, N., Feng, T., Liu, S., Zhang, T., Zhang, Q., and Molina, L. T.: Typical synoptic situations and their impacts on the wintertime air pollution in the Guanzhong basin, China, Atmos. Chem. Phys., 16, 7373–7387, https://doi.org/10.5194/acp-16-7373-2016, 2016.
Bei, N., Wu, J., Elser, M., Feng, T., Cao, J., El-Haddad, I., Li, X., Huang, R., Li, Z., Long, X., Xing, L., Zhao, S., Tie, X., Prévôt, A. S. H., and Li, G.: Impacts of meteorological uncertainties on the haze formation in Beijing–Tianjin–Hebei (BTH) during wintertime: a case study, Atmos. Chem. Phys., 17, 14579–14591, https://doi.org/10.5194/acp-17-14579-2017, 2017.
Binkowski, F. S. and Roselle, S. J.: Models-3 Community Multiscale Air Quality (CMAQ) model aerosol component 1. Model description, J. Geophys. Res.-Atmos., 108, 335–346, https://doi.org/10.1029/2001JD001409, 2003.
Boy, M., Mogensen, D., Smolander, S., Zhou, L., Nieminen, T., Paasonen, P., Plass-Dülmer, C., Sipilä, M., Petäjä, T., Mauldin, L., Berresheim, H., and Kulmala, M.: Oxidation of SO2 by stabilized Criegee intermediate (sCI) radicals as a crucial source for atmospheric sulfuric acid concentrations, Atmos. Chem. Phys., 13, 3865–3879, https://doi.org/10.5194/acp-13-3865-2013, 2013.
Calvert, J. G., Su, F., Bottenheim, J. W., and Strausz, O. P.: Mechanism of the homogeneous oxidation of sulfur dioxide in the troposphere, Atmos. Environ., 12, 197–226, https://doi.org/10.1016/0004-6981(78)90201-9, 1978.
Chen, F. and Dudhia, J.: Coupling an advanced land surface-hydrology model with the Penn State-NCAR MM5 modeling system. Part I: Model implementation and sensitivity, Mon. Weather Rev., 129, 569–585, https://doi.org/10.1175/1520-0493(2001)129<0569:CAALSH>2.0.CO;2, 2001.
Cheng, Y., Zheng, G., Wei, C., Mu, H., Zheng, B., Wang, Z., Gao, M., Zhang, Q., He, K., Carmichael, G., Pöschl, U., and Su, H.: Reactive nitrogen chemistry in aerosol water as a source of sulfate during haze events in China, Sci. Adv., 2, e1601530, https://doi.org/10.1126/sciadv.1601530, 2016.
China MEP (Ministry of Environmental Protection, China): Air Quality Observation Real-time Release Platform of MEP Data Center, available at: http://106.37.208.233:20035 (last access: 28 October 2019), 2013a.
China MEP (Ministry of Environmental Protection, China): Online Monitoring and Analysis Platform of China Air Quality, available at: https://www.aqistudy.cn (last access: 28 October 2019), 2013b.
Chou, M. D. and Suarez, M. J.: A solar radiation parameterization for atmospheric studies, edited by: Suarez, M. J., No. NASA/TM-1999-10460, NASA Technique Report, Greenbelt, USA, 1999.
Chou, M. D., Suarez, M. J., Liang, X. Z., Yan, M. H., and Cote, C.: A Thermal Infrared Radiation Parameterization for Atmospheric Studies, edited by: Suarez, M. J., No. NASA/TM-2001-104606, NASA Technique Report, Greenbelt, USA, 2001.
Cox, R. A. and Penkett, S. A.: Oxidation of atmospheric SO2 by products of the Ozone-Olefin reaction, Nature, 230, 321–322, https://doi.org/10.1038/230321a0, 1971.
Dunker, A. M., Morris, R. E., Pollack, A. K., Schleyer, C. H., and Yarwood, G.: Photochemical modeling of the impact of fuels and vehicles on urban ozone using auto oil program data, Environ. Sci. Technol., 30, 787–801, https://doi.org/10.1021/es950175m, 1996.
Feng, T., Li, G., Cao, J., Bei, N., Shen, Z., Zhou, W., Liu, S., Zhang, T., Wang, Y., Huang, R.-J., Tie, X., and Molina, L. T.: Simulations of organic aerosol concentrations during springtime in the Guanzhong Basin, China, Atmos. Chem. Phys., 16, 10045–10061, https://doi.org/10.5194/acp-16-10045-2016, 2016.
Gao, M., Carmichael, G. R., Wang, Y., Saide, P. E., Yu, M., Xin, J., Liu, Z., and Wang, Z.: Modeling study of the 2010 regional haze event in the North China Plain, Atmos. Chem. Phys., 16, 1673–1691, https://doi.org/10.5194/acp-16-1673-2016, 2016.
Grell, G. A., Peckham, S. E., Schmitz, R., McKeen, S. A., Frost, G., Skamarock, W. C., and Eder, B.: Fully coupled “online” chemistry within the WRF model, Atmos. Environ., 39, 6957–6975, https://doi.org/10.1016/j.atmosenv.2005.04.027, 2005.
Guenther, A., Karl, T., Harley, P., Wiedinmyer, C., Palmer, P. I., and Geron, C.: Estimates of global terrestrial isoprene emissions using MEGAN (Model of Emissions of Gases and Aerosols from Nature), Atmos. Chem. Phys., 6, 3181–3210, https://doi.org/10.5194/acp-6-3181-2006, 2006.
Guo, S., Hu, M., Zamora, M. L., Peng, J., Shang, D., Zheng, J., Du, Z., Wu, Z., Shao, M., and Zeng, L.: Elucidating severe urban haze formation in China, P. Natl. Acad. Sci. USA, 111, 17373–17378, https://doi.org/10.1073/pnas.1419604111, 2014.
Hatakeyama, S. and Akimoto, H.: Reactions of Criegee Intermediates in the gas phase, Res. Chem. Intermed., 20, 503–524, https://doi.org/10.1163/156856794X00432, 1994.
Hong, S. Y. and Lim, J. O. J.: The WRF Single-Moment 6-Class Microphysics Scheme (WSM6), Asia-Pacif. J. Atmos. Sci., 42, 129–151, 2006.
Horowitz, L. W., Walters, S., Mauzerall, D. L., Emmons, L. K., Rasch, P. J., Granier, C., Tie, X., Lamarque, J. F., Schultz, M. G., Tyndall, G. S., Orlando, J. J., and Brasseur, G. P.: A global simulation of tropospheric ozone and related tracers: Description and evaluation of MOZART, version 2, J. Geophys. Res.-Atmos., 108, 4784, https://doi.org/10.1029/2002JD002853, 2003.
Janjić, Z. I.: Nonsingular Implementation of the Mellor-Yamada Level 2.5 Scheme in the NCEP Meso Model, NCEP Office Note #437, NCEP, Camp Springs, USA, 2002.
Jenkin, M. E., Saunders, S. M., and Pilling, M. J.: The tropospheric degradation of volatile organic compounds: a protocol for mechanism development, Atmos. Environ., 31, 81–104, https://doi.org/10.1016/S1352-2310(96)00105-7, 1997.
Li, G., Zhang, R., Fan, J., and Tie, X.: Impacts of black carbon aerosol on photolysis and ozone, J. Geophys. Res.-Atmos., 110, D23206, https://doi.org/10.1029/2005JD005898, 2005.
Li, G., Lei, W., Zavala, M., Volkamer, R., Dusanter, S., Stevens, P., and Molina, L. T.: Impacts of HONO sources on the photochemistry in Mexico City during the MCMA-2006/MILAGO Campaign, Atmos. Chem. Phys., 10, 6551–6567, https://doi.org/10.5194/acp-10-6551-2010, 2010.
Li, G., Bei, N., Tie, X., and Molina, L. T.: Aerosol effects on the photochemistry in Mexico City during MCMA-2006/MILAGRO campaign, Atmos. Chem. Phys., 11, 5169–5182, https://doi.org/10.5194/acp-11-5169-2011, 2011a.
Li, G., Zavala, M., Lei, W., Tsimpidi, A. P., Karydis, V. A., Pandis, S. N., Canagaratna, M. R., and Molina, L. T.: Simulations of organic aerosol concentrations in Mexico City using the WRF-CHEM model during the MCMA-2006/MILAGRO campaign, Atmos. Chem. Phys., 11, 3789–3809, https://doi.org/10.5194/acp-11-3789-2011, 2011b.
Li, G., Lei, W., Bei, N., and Molina, L. T.: Contribution of garbage burning to chloride and PM2.5 in Mexico City, Atmos. Chem. Phys., 12, 8751–8761, https://doi.org/10.5194/acp-12-8751-2012, 2012.
Li, G., Bei, N., Cao, J., Huang, R., Wu, J., Feng, T., Wang, Y., Liu, S., Zhang, Q., Tie, X., and Molina, L. T.: A possible pathway for rapid growth of sulfate during haze days in China, Atmos. Chem. Phys., 17, 3301–3316, https://doi.org/10.5194/acp-17-3301-2017, 2017.
Li, J., Ying, Q., Yi, B., and Yang, P.: Role of stabilized Criegee Intermediates in the formation of atmospheric sulfate in eastern United States, Atmos. Environ., 79, 442–447, https://doi.org/10.1016/j.atmosenv.2013.06.048, 2013.
Li, X., Wu, J., Elser, M., Feng, T., Cao, J., El-Haddad, I., Huang, R., Tie, X., Prévôt, A. S. H., and Li, G.: Contributions of residential coal combustion to the air quality in Beijing–Tianjin–Hebei (BTH), China: a case study, Atmos. Chem. Phys., 18, 10675–10691, https://doi.org/10.5194/acp-18-10675-2018, 2018.
Mauldin, R. L., Berndt, T., Sipilä, M., Paasonen, P., Petäjä, T., Kim, S., Kurtén, T., Stratmann, F., Kerminen, V. M., and Kulmala, M.: A new atmospherically relevant oxidant of sulphur dioxide, Nature, 488, 193–196, https://doi.org/10.1038/nature11278, 2012.
Nenes, A., Pandis, S. N., and Pilinis, C.: ISORROPIA: A new thermodynamic equilibrium model for multiphase multicomponent inorganic aerosols, Aquat. Geochem., 4, 123–152, https://doi.org/10.1023/a:1009604003981, 1998.
Novelli, A., Hens, K., Tatum Ernest, C., Martinez, M., Nölscher, A. C., Sinha, V., Paasonen, P., Petäjä, T., Sipilä, M., Elste, T., Plass-Dülmer, C., Phillips, G. J., Kubistin, D., Williams, J., Vereecken, L., Lelieveld, J., and Harder, H.: Estimating the atmospheric concentration of Criegee intermediates and their possible interference in a FAGE-LIF instrument, Atmos. Chem. Phys., 17, 7807–7826, https://doi.org/10.5194/acp-17-7807-2017, 2017.
Percival, C. J., Welz, O., Eskola, A. J., Savee, J. D., Osborn, D. L., Topping, D. O., Lowe, D., Utembe, S. R., Cooke, M. C., Taatjes, C. A., and Shallcross, D. E.: Regional and global impacts of Criegee intermediates on atmospheric sulphuric acid concentrations and first steps of aerosol formation, Faraday Discuss., 165, 45–73, https://doi.org/10.1039/C3FD00048F, 2013.
Pierce, J. R., Evans, M. J., Scott, C. E., D'Andrea, S. D., Farmer, D. K., Swietlicki, E., and Spracklen, D. V.: Weak global sensitivity of cloud condensation nuclei and the aerosol indirect effect to Criegee + SO2 chemistry, Atmos. Chem. Phys., 13, 3163–3176, https://doi.org/10.5194/acp-13-3163-2013, 2013.
Sarwar, G., Fahey, K., Kwok, R., Gilliam, R. C., Roselle, S. J., Mathur, R., Xue, J., Yu, J., and Carter, W. P.: Potential impacts of two SO2 oxidation pathways on regional sulfate concentrations: Aqueous-phase oxidation by NO2 and gas-phase oxidation by Stabilized Criegee Intermediates, Atmos. Environ., 68, 186–197, https://doi.org/10.1016/j.atmosenv.2012.11.036, 2013.
Sarwar, G., Simon, H., Fahey, K., Mathur, R., Goliff, W. S., and Stockwell, W. R.: Impact of sulfur dioxide oxidation by Stabilized Criegee Intermediate on sulfate, Atmos. Environ., 85, 204–214, https://doi.org/10.1016/j.atmosenv.2013.12.013, 2014.
Seinfeld, J. H. and Pandis, S. N.: Atmospheric Chemistry and Physics: From Air Pollution to Climate Change, 2nd Edn., John Wiley & Sons Inc., New York, 2006.
Smith, M., Chang, C-H., Chao, W., Lin, L-C., Takahashi, K., Boering, K., and Lin, J. J.-M.: Strong negative temperature dependence of the simplest Criegee Intermediate CH2OO reaction with water dimer, J. Phys. Chem. Lett., 6, 2708–2713, https://doi.org/10.1021/acs.jpclett.5b01109, 2015.
Spracklen, D. V., Jimenez, J. L., Carslaw, K. S., Worsnop, D. R., Evans, M. J., Mann, G. W., Zhang, Q., Canagaratna, M. R., Allan, J., Coe, H., McFiggans, G., Rap, A., and Forster, P.: Aerosol mass spectrometer constraint on the global secondary organic aerosol budget, Atmos. Chem. Phys., 11, 12109–12136, https://doi.org/10.5194/acp-11-12109-2011, 2011.
Stone, D., Blitz, M., Daubney, L., Howes, N., and Seakins, P.: Kinetics of CH2OO reactions with SO2, NO2, NO, H2O and CH3CHO as a function of pressure, Phys. Chem. Chem. Phys., 16, 1139–1149, https://doi.org/10.1039/c3cp54391a, 2014.
Sun, Y. L., Wang, Z. F., Du, W., Zhang, Q., Wang, Q. Q., Fu, P. Q., Pan, X. L., Li, J., Jayne, J., and Worsnop, D. R.: Long-term real-time measurements of aerosol particle composition in Beijing, China: seasonal variations, meteorological effects, and source analysis, Atmos. Chem. Phys., 15, 10149–10165, https://doi.org/10.5194/acp-15-10149-2015, 2015.
Suto, M., Manzanares, E. R., and Lee, L. C.: Detection of sulfuric-acid aerosols by ultraviolet scattering, Environ. Sci. Technol., 19, 815–820, https://doi.org/10.1021/es00139a008, 1985.
Taatjes, C. A.: Criegee intermediates: What direct production and detection can teach us about reactions of carbonyl oxides, Annu. Rev. Phys. Chem., 68, 183–207, https://doi.org/10.1146/annurev-physchem-052516-050739, 2017.
Tao, J., Zhang, L., Cao, J., and Zhang, R.: A review of current knowledge concerning PM2.5 chemical composition, aerosol optical properties and their relationships across China, Atmos. Chem. Phys., 17, 9485–9518, https://doi.org/10.5194/acp-17-9485-2017, 2017.
Tie, X., Madronich, S., Walters, S., Zhang, R., Rasch, P., and Collins, W.: Effect of clouds on photolysis and oxidants in the troposphere, J. Geophys. Res.-Atmos., 108, 4642, https://doi.org/10.1029/2003JD003659, 2003.
Vereecken, L., Harder, H., and Novelli, A.: The reaction of Criegee intermediates with NO, RO2, and SO2, and their fate in the atmosphere, Phys. Chem. Chem. Phys., 14, 14682–14695, https://doi.org/10.1039/c2cp42300f, 2012.
Wang, S. and Hao, J.: Air quality management in China: Issues, challenges, and options, J. Environ. Sci., 24, 2–13, https://doi.org/10.1016/S1001-0742(11)60724-9, 2012.
Wang, G., Zhang, R., Gomez, M. E., Yang, L., Levy Zamora, M., Hu, M., Lin, Y., Peng, J., Guo, S., Meng, J., Li, J., Cheng, C., Hu, T., Ren, Y., Wang, Y., Gao, J., Cao, J., An, Z., Zhou, W., Li, G., Wang, J., Tian, P., Marrero-Ortiz, W., Secrest, J., Du, Z., Zheng, J., Shang, D., Zeng, L., Shao, M., Wang, W., Huang, Y., Wang, Y., Zhu, Y., Li, Y., Hu, J., Pan, B., Cai, L., Cheng, Y., Ji, Y., Zhang, F., Rosenfeld, D., Liss, P. S., Duce, R. A., Kolb, C. E., and Molina, M. J.: Persistent sulfate formation from London Fog to Chinese haze, P. Natl. Acad. Sci. USA, 113, 13630–13635, https://doi.org/10.1073/pnas.1616540113, 2016.
Welz, O., Savee, J. D., Osborn, D. L., Vasu, S. S., Percival, C. J., Shallcross, D. E., and Taatjes, C. A: Direct kinetic measurements of Criegee Intermediate (CH2OO) formed by reaction of CH2I with O2, Science, 335, 204–207, https://doi.org/10.1126/science.1213229, 2012.
Wesely, M. L.: Parameterization of surface resistances to gaseous dry deposition in regional-scale numerical models, Atmos. Environ., 23, 1293–1304, https://doi.org/10.1016/0004-6981(89)90153-4, 1989.
Wu, J., Li, G., Cao, J., Bei, N., Wang, Y., Feng, T., Huang, R., Liu, S., Zhang, Q., and Tie, X.: Contributions of trans-boundary transport to summertime air quality in Beijing, China, Atmos. Chem. Phys., 17, 2035–2051, https://doi.org/10.5194/acp-17-2035-2017, 2017.
Wu, J., Bei, N., Li, X., Cao, J., Feng, T., Wang, Y., Tie, X., and Li, G.: Widespread air pollutants of the North China Plain during the Asian summer monsoon season: a case study, Atmos. Chem. Phys., 18, 8491–8504, https://doi.org/10.5194/acp-18-8491-2018, 2018.
Xing, L., Wu, J., Elser, M., Tong, S., Liu, S., Li, X., Liu, L., Cao, J., Zhou, J., El-Haddad, I., Huang, R., Ge, M., Tie, X., Prévôt, A. S. H., and Li, G.: Wintertime secondary organic aerosol formation in Beijing–Tianjin–Hebei (BTH): contributions of HONO sources and heterogeneous reactions, Atmos. Chem. Phys., 19, 2343–2359, https://doi.org/10.5194/acp-19-2343-2019, 2019.
Ying, Q. and Kleeman, M. J.: Source contributions to the regional distribution of secondary particulate matter in California, Atmos. Environ., 40, 736–752, https://doi.org/10.1016/j.atmosenv.2005.10.007, 2006.
Ying, Q. and Krishnan, A.: Source contribution of volatile organic compounds to ozone formation in southeast Texas, J. Geophys. Res.-Atmos., 115, D17306, https://doi.org/10.1029/2010JD013931, 2010.
Ying, Q., Cureno, I. V., Chen, G., Ali, S., Zhang, H., Malloy, M., Bravo, H. A., and Sosa, R.: Impacts of stabilized Criegee Intermediates, surface uptake processes and higher aromatic secondary organic aerosol yields on predicted PM2.5 concentrations in the Mexico City Metropolitan Zone, Atmos. Environ., 94, 438–447, https://doi.org/10.1016/j.atmosenv.2014.05.056, 2014.
Zhang, H. and Ying, Q.: Secondary organic aerosol formation and source apportionment in Southeast Texas, Atmos. Environ., 45, 3217–3227, https://doi.org/10.1016/j.atmosenv.2011.03.046, 2011.
Zhang, Q., Streets, D. G., Carmichael, G. R., He, K. B., Huo, H., Kannari, A., Klimont, Z., Park, I. S., Reddy, S., Fu, J. S., Chen, D., Duan, L., Lei, Y., Wang, L. T., and Yao, Z. L.: Asian emissions in 2006 for the NASA INTEX-B mission, Atmos. Chem. Phys., 9, 5131–5153, https://doi.org/10.5194/acp-9-5131-2009, 2009.
Zhang, X. Y., Wang, Y. Q., Niu, T., Zhang, X. C., Gong, S. L., Zhang, Y. M., and Sun, J. Y.: Atmospheric aerosol compositions in China: spatial/temporal variability, chemical signature, regional haze distribution and comparisons with global aerosols, Atmos. Chem. Phys., 12, 779–799, https://doi.org/10.5194/acp-12-779-2012, 2012.
Zhao, P. S., Dong, F., He, D., Zhao, X. J., Zhang, X. L., Zhang, W. Z., Yao, Q., and Liu, H. Y.: Characteristics of concentrations and chemical compositions for PM2.5 in the region of Beijing, Tianjin, and Hebei, China, Atmos. Chem. Phys., 13, 4631–4644, https://doi.org/10.5194/acp-13-4631-2013, 2013.