the Creative Commons Attribution 4.0 License.
the Creative Commons Attribution 4.0 License.
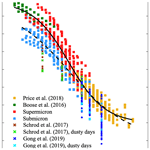
Size-dependent ice nucleation by airborne particles during dust events in the eastern Mediterranean
Naama Reicher
Carsten Budke
Lukas Eickhoff
Shira Raveh-Rubin
Ifat Kaplan-Ashiri
Thomas Koop
The prediction of cloud ice formation in climate models remains a challenge, partly due to the complexity of ice-related processes. Mineral dust is a prominent aerosol in the troposphere and is an important contributor to ice nucleation in mixed-phase clouds, as dust can initiate ice heterogeneously at relatively low supercooling conditions. We characterized the ice nucleation properties of size-segregated mineral dust sampled during dust events in the eastern Mediterranean. The sampling site allowed us to compare the properties of airborne dust from several sources with diverse mineralogy that passed over different atmospheric paths. We focused on particles with six size classes determined by the Micro-Orifice Uniform Deposit Impactor (MOUDI) cutoff sizes: 5.6, 3.2, 1.8, 1.0, 0.6 and 0.3 µm. Ice nucleation experiments were conducted in the Weizmann Supercooled Droplets Observation on a Microarray (WISDOM) setup, whereby the particles are immersed in nanoliter droplets using a microfluidics technique. We observed that the activity of airborne particles depended on their size class; supermicron and submicron particles had different activities, possibly due to different composition. The concentrations of ice-nucleating particles and the density of active sites (ns) increased with the particle size and particle concentration. The supermicron particles in different dust events showed similar activity, which may indicate that freezing was dominated by common mineralogical components. Combining recent data of airborne mineral dust, we show that current predictions, which are based on surface-sampled natural dust or standard mineral dust, overestimate the activity of airborne dust, especially for the submicron class. Therefore, we suggest including information on particle size in order to increase the accuracy of ice formation modeling and thus weather and climate predictions.
- Article
(4120 KB) - Full-text XML
-
Supplement
(3299 KB) - BibTeX
- EndNote
Cloud droplets can supercool to 238 K before homogeneous freezing occurs (Koop and Murray, 2016; Rosenfeld and Woodley, 2000). At warmer temperatures, heterogeneous ice nucleation (HIN), whereby the presence of aerosol particles lowers the required energy barrier to form a stable ice nucleus, is the common pathway of ice formation (Murray et al., 2012; Pruppacher and Klett, 1997; Khvorostyanov and Curry, 2004; Hoose and Möhler, 2012). These ice-nucleating particles (INPs) can be activated at subzero temperatures and subsequently lower humidity conditions, mainly by interaction with supercooled droplets. INPs are relatively rare particles and comprise only about 10−5 of the total ambient particles in the free troposphere (Rogers et al., 1998). Yet, their interaction with clouds can greatly influence climate (Gettelman et al., 2012; Tan et al., 2016; Lohmann and Feichter, 2005). Therefore, it is important to represent them well in weather and climate models (DeMott et al., 2010). Currently, ice formation is a source of great uncertainty in cloud and climate models, partly due to the complexity of ice processes and the insufficient understanding of the key surface properties that determine an INP (IPCC, 2013). To improve the predictions of models, great effort is invested in the characterization of INPs and in the development of parameterizations based on their physical and chemical properties (Cantrell and Heymsfield, 2005; Niemand et al., 2012; Ullrich et al., 2017).
One of the most abundant INPs in the atmosphere is mineral dust, which originates in dryland zones such as deserts (Middleton, 2017; DeMott et al., 2003b). Field observations have identified an increase in INP concentrations and ice cloud formation in the presence of mineral dust (Ansmann et al., 2008; Rosenfeld et al., 2001; DeMott et al., 2003b; Cziczo et al., 2004; Sassen et al., 2003). Ice residuals often contain mineral particles (Cziczo et al., 2013; Cziczo and Froyd, 2014; Twohy and Poellot, 2005). Mineral dust has high spatial and temporal variability, impacting atmospheric, oceanic, biological, terrestrial and human systems (Garrison et al., 2003; Gat et al., 2017; Jickells et al., 2005; Mahowald et al., 2014; Mazar et al., 2016; Middleton and Goudie, 2001). Each year, gigatons of dust are transported globally over long distances, dominating the atmospheric aerosol mass and aerosol optical depth (AOD) (Chiapello et al., 1999; Tegen and Fung, 1994; Ben-Ami et al., 2010; Prospero, 1999; Koren et al., 2006). Though the exact property of an aerosol that determines its ice nucleation ability remains unclear, it was consistently shown that the mineral composition plays an important role (Kanji et al., 2017) and that for a certain mineral type, larger particles are more effective heterogeneous INPs than small ones (Archuleta et al., 2005; Lüönd et al., 2010; Welti et al., 2019). Local surface features such as steps, cracks and cavities, a close match of the surface lattice with that of ice, or surface hydroxyl groups (Freedman, 2015; Marcolli, 2014; Zielke et al., 2015; Kiselev et al., 2017; Fletcher, 1969; Tunega et al., 2004; Anderson and Hallett, 1976; Pruppacher and Klett, 1997) are believed to be the responsible factors for the ice nucleation ability of mineral surfaces.
Natural mineral dust particles are often chemically similar but differ in their mineralogy (Engelbrecht et al., 2009), and the particles are often composed of a mixture of minerals (internally mixed), such as clays, quartz, feldspars and calcites (Claquin et al., 1999). Other common minerals are palygorskite, hematite, halite, gypsum, gibbsite and goethite (Ganor et al., 1991; Perlwitz et al., 2015; Kandler et al., 2007; Mahowald et al., 2014). The mineralogy of mineral dust is set by its source region and is considered to be an important factor that determines its freezing characteristics (Zimmermann et al., 2008; Augustin-Bauditz et al., 2014). Traditionally, clay minerals were thought to be responsible for atmospheric ice nucleation because they compose much of the dust fraction. However, using standard mineral particles, Atkinson et al. (2013) showed that K feldspar is the most efficient type and suggested that it could dominate atmospheric ice formation at relatively high temperatures above 258 K. This was further supported by measurements of natural mineral dust from desert surfaces worldwide, wherein the importance of quartz mineral was also indicated (Boose et al., 2016b).
Airborne mineral dust (AMD) can experience chemical and physical modifications during its atmospheric transport that may alter dust's ability to nucleate ice (Kanji et al., 2013). It was shown that atmospheric aging processes can change the size, morphology and surface chemistry of the particles. This includes, for example, the adsorption of organic components on AMD (Murphy et al., 2006; DeMott et al., 2003a; Falkovich et al., 2004) or coatings of nitrates, chlorides and sulfates that enhance the hygroscopicity of the particles (Krueger et al., 2004; Laskin et al., 2005; Li and Shao, 2009). Levin et al. (1996) found that AMD particles transported over the Mediterranean Sea were often coated with sulfate and other soluble materials, which affect cloud microphysical properties and can eventually result in enhanced ice nucleation. In addition, mineral dust carries biological components, such as bacteria and fungi, which are known to have the ability to induce ice nucleation at relatively high temperatures (Gat et al., 2017; Mazar et al., 2016; Pratt et al., 2009; O'Sullivan et al., 2016). Further modifications that can occur during AMD atmospheric transport are the differentiation of size and mineralogy. These can occur due to gravitational sedimentation, for example, whereby larger particles sediment faster than smaller ones. Near source regions, dust samples were richer in components that are more abundant in the coarse fractions, such as quartz and potassium feldspars, while in remote locations, higher amounts of clay minerals and sodium–calcium feldspar were observed (Murray et al., 2012; Schepanski, 2018).
While there are few measurements of AMD close to source regions (Price et al., 2018; Boose et al., 2016a; Ardon-Dryer and Levin, 2014; Schrod et al., 2017), parameterizations of ice formation in climate models are often based on the freezing properties of natural dust or soil samples collected from deserts or standard dust particles (Niemand et al., 2012; Connolly et al., 2009; Ullrich et al., 2017; Atkinson et al., 2013; Broadley et al., 2012) that may not sufficiently represent AMD (Boose et al., 2016b; Spichtinger and Cziczo, 2010). Natural dust samples showed higher ice nucleation ability than AMD samples, possibly due to atmospheric processing of AMD that may lead to deactivation and possibly due to laboratory processes, such as milling or sieving, that were applied to the natural dust samples and may have enhanced its activity (Boose et al., 2016b).
In this study, we sampled airborne particles during dust events in the eastern Mediterranean and investigated their ice nucleation abilities. The eastern Mediterranean is located in the strip of the world's main deserts and experiences the transport of desert dust from different sources. The main source is the Sahara in North Africa. It is estimated that about 100 million tons of dust per year is lifted from the Sahara towards the eastern Mediterranean during late winter and spring (Ganor, 1994; Ganor and Mamane, 1982; Ganor et al., 2010). In autumn, local dust is transported, commonly from the Arabian Peninsula and the Syrian Desert (Dayan et al., 1991; Ganor, 1994). The dust events are often associated with the regional eastern Mediterranean synoptic systems, such as winter lows and Red Sea troughs (Ganor et al., 2010). Our sampling site was located in Israel, where Saharan dust is transported over North Africa and/or the Mediterranean Sea and Syrian and Arabian dust is transported over land from the east (Ganor et al., 1991). These distinct sources and paths allow for the investigation of the ice nucleation properties of AMD with diverse origins and transport paths.
The ability of the collected particles to initiate immersion freezing was studied using the Weizmann Supercooled Droplets Observation on a Microarray (WISDOM) instrument (Reicher et al., 2018), and one of the dust events was studied using the Bielefeld Ice Nucleation ARraY (BINARY) instrument (Budke and Koop, 2015). We characterized the concentrations and the density of ice-nucleation-active sites (INASs) of AMD in different size classes for several dust cases, as well as combining recent literature and available AMD data to understand how well AMD is represented in models based on recent parameterizations.
2.1 Sampling
Airborne particles were sampled during six dust events in 2016 and 2017, detailed in Table 1. Sampling started when visibility was reduced due to increasing concentrations of particulate matter (PM). The sampling site is located on the roof of a three-story building in Rehovot, Israel (31.9∘ N, 34.8∘ E; about 80 m a.s.l.). The location is often impacted by mineral dust storms, transported from nearby and distant geographical locations, mainly from the Sahara and Arabia deserts and less frequently from the Syrian Desert depending on the season and the synoptic conditions (Dayan, 1986; Ganor et al., 2010; Kalderon-Asael et al., 2009).
Table 1Summary of the investigated dust storm events. The events are denoted by their geographic origin: Saharan dust storm (SDS), Syrian dust storm (SyDS), mixed contribution of the two (MDS) and mix of dust event with a dust-free period (CSDS).
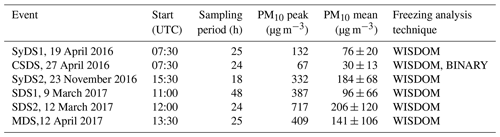
Particles were collected on polycarbonate filters (47 mm Cyclopore, 0.1 µm Isopore; Whatman) using the Micro-Orifice Uniform Deposit Impactor (MOUDI) (model 110-R). The MOUDI is a 10-stage impactor with an 18 µm cut-point inlet stage followed by size-segregating stages with cut points (D50) between 0.056 and 10 µm in aerodynamic diameter (Marple et al., 1991). The particles are collected on the different stages as a function of their aerodynamic diameter. The collection efficiency for each particle size is described in Marple et al. (1991). Sampling time ranged between 17 and 48 h with a 30 L min−1 sample flow rate, similar to previous studies (Huffman et al., 2013; Mason et al., 2015).
2.2 Air mass back trajectories
Back trajectories were calculated by a Lagrangian method using LAGRANTO 2.0 (Sprenger and Wernli, 2015). The calculation of air mass trajectories was based on wind data from the European Centre for Medium-Range Weather Forecasts ERA-Interim reanalysis (Dee et al., 2011), available every 6 h at 1∘ × 1∘ horizontal grid with 60 vertical hybrid levels. For each 6 h time step during each event, 72 h back trajectories were calculated from all available data grid points with pressure larger than 850 hPa, resulting in 11 trajectories, which end their path in the lower troposphere for each calculation. In a second step, the Eulerian densities of the resulting trajectories were computed by gridding the trajectories for each event, smoothed by using a radius of 100 km and interpolated to 1 h. Finally, the trajectory density was summed over the entire event duration and normalized by the maximum trajectory count.
2.3 Dust column mass density maps
Time-averaged maps of dust column mass density (hourly 0.5∘ × 0.625∘) reanalysis data were obtained from the Modern-Era Retrospective analysis for Research and Applications (MERRA-2). Maps were produced using NASA's Global Modeling and Assimilation Office (GMAO) (Gelaro et al., 2017) for a period of up to 72 h prior to the sampled event.
2.4 Particulate matter data
Particulate matter mass data were obtained from the Israeli Ministry of Environment website. Concentrations of particles with aerodynamic diameters smaller than 10 µm (PM10) were measured in the Rehovot station, located about 1 km from our sampling site. The 5 min mean data were used to calculate peak and mean concentrations of the sampled dust events.
2.5 Particle number size and surface area distributions
Particle size distribution and concentrations between 0.25 and 32 µm were measured on site by an optical particle counter (OPC; GRIMM Technologies model 1.109) in parallel to the MOUDI sampling. In order to estimate the total surface area that was collected on the different stages, we assumed that the particles are spheres and used the diameter of the GRIMM midpoint of the different GRIMM channels as the particle diameter.
2.6 Conversion of GRIMM channels to MOUDI stages
To determine the total surface area collected on MOUDI's filter, a conversion matrix between the GRIMM channels and the MOUDI stages was applied. The conversion was based on the particle collection efficiency curves of MOUDI and interstage particle losses reported in Marple et al. (1991). Figure 1 demonstrates the fraction of particles that are collected on the stages based on their aerodynamic diameter. Freezing analyses focused on stage no. 2 (D50=5.6 µm), stage no. 3 (D50=3.2 µm), stage no. 4 (D50=1.8 µm), stage no. 5 (D50=1.0 µm), stage no. 6 (D50=0.6 µm) and stage no. 7 (D50=0.3 µm). For example, most of the particles with an optical diameter >8.5 µm will be collected on stage no. 2 (D50=5.6 µm), whereas all the particles with an optical diameter >17.5 µm are assumed to be collected on former stages (inlet and stage no. 1). In some cases, particles in a certain size are likely to impact two different MOUDI stages. For example, a small fraction of particles with 0.5 µm optical diameter are collected on stage no. 5 (D50=0.6 µm), and most of them impact stage no. 6 (D50=0.3 µm). The initial particle concentration that was used is the accumulated sum of all particles for the entire sampling period.
2.7 Ice-freezing experiments and quantification
2.7.1 WISDOM
The immersion freezing activity of the sampled ambient mineral dust was measured using suspensions of the collected particles that were extracted from the filters by dry sonication (VialTweeter, model UP200St; Hielcher). This type of sonication method is more effective than an ultrasonic bath in which most of the energy dissipates in the surrounding water. A quarter filter was inserted into a 1.5 mL Eppendorf vial with 0.3 mL of deionized water and sonicated in three 30 s cycles to avoid heating produced during intense sonication. The suspension was immediately used for droplet production and freezing experiments in WISDOM as detailed in Reicher et al. (2018). Briefly, an array of 0.5 nL monodispersed droplets (∼100 µm diameter, suspended in an oil mixture) was generated in a microfluidic device that was cooled by a commercial cooling stage (THMS600, Linkam) under a microscope (BX-51 with 10× magnification, Olympus) coupled to a charge-couple device (CCD) camera. The device was first cooled at a faster constant rate of 10 K min−1 from room temperature to 263 K, since freezing events were not expected and indeed were never observed in that temperature range. Then a constant cooling rate of 1 K min−1 was used until all the droplets froze. The temperature uncertainty was ±0.3 K based on error propagation between the calibrated droplet temperature and the uncertainty of the temperature sensor that is located in the cooling stage (see Reicher et al., 2018, for more details).
2.7.2 BINARY
The Bielefeld Ice Nucleation ARraY (BINARY) is an optical freezing array of droplets pipetted on a hydrophobic substrate in separated sealed compartments and cooled in a Linkam cooling stage (LTS120) (Budke and Koop, 2015). In the present study an array of 64 droplets of 0.6 µL was employed. Suspensions were prepared by extracting a quarter filter in 1.5 mL of double-distilled water (that is, 5 times more diluted than WISDOM suspensions) using a bath sonicator (Elma Transsonic Digital, TP 670/H) for 30 min. The bath temperature increased during sonication from about 288 to 308 K. The obtained suspensions were used directly and further diluted (1:10) for another set of measurements with reduced surface area of the particles in the droplets. For the freezing experiments, the droplets were cooled at a rate of 1 K min−1. Temperature uncertainty was ±0.3 K.
2.7.3 Quantification of freezing properties
The cumulative concentration of INPs present in a volume of solvent, V, at temperature T was derived using the fraction of frozen droplets (fice(T)) that was obtained directly from the freezing experiments (Vali, 1971):
For control experiments, a quarter of blank filter was immersed in pure water, similar to freezing experiments for the airborne samples, and the concentration of the background impurities (Kimp(T)) was subtracted from the concentrations that were detected for airborne samples.
The atmospheric concentrations of INPs per unit volume of air as a function of temperature, INP(T), were determined by incorporating the sampling and solvent parameters into Eq. (2) (Hader et al., 2014):
where Vsolvent is the volume of the water used for extraction, Vair is the total sampled air volume and f is the fraction of filter that was used in the extraction.
For a comparison of the ice nucleation activity of the different dust events, the INP concentration in the liquid was converted to the number of active sites per unit surface area of INPs, i.e., the surface density of sites ns active above temperature T (Vali, 1971):
where A is the surface area immersed in a single droplet of the experiment based on the total surface area of particles in the suspension.
2.8 Scanning electron microscopy
A quarter of selected filters were coated with iridium for analyzing the chemical composition of airborne particles using a scanning electron microscope (SEM; Supra 55VP, LEO) equipped with an energy-dispersive X-ray spectroscopy (EDX) detector for elemental microanalysis. The analysis was done at a voltage of 5 kV using the Quantax software (Bruker).
3.1 Air mass back trajectories and the origin of the dust storms
The density of air mass back trajectories for a 72 h period prior to the sampling for all events is shown in Fig. 2. The sampling site and the surrounding main deserts are shown as well. During the sampled events, the air mass trajectories were diverse. In some cases, the air masses traveled directly to the sampling site from the source region, while in other cases, they traveled a longer distance. In most events, the air mass had either an easterly or westerly component and was often concentrated in the same geographical area.
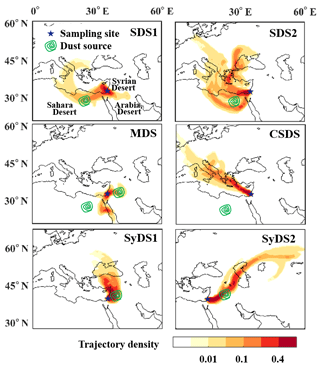
Figure 2Air mass origin and atmospheric paths of the dust events. Colors represent the density of 72 h backward air mass trajectories (normalized to the total trajectory counts). The green contours represent the geographic locations where a high mass of dust occurred during the air mass transition, which is defined as the potential origin of the dust. Abbreviations in the top right of each panel indicate the particular dust event.
The dust origins were identified based on back trajectory analysis, integrated with reanalysis data from remote sensing of atmospheric dust. We followed the dust mass concentration prior to the sampling period, as detailed in Fig. S1 in the Supplement. Locations that contained high levels of suspended dust that overlapped with the air mass trajectories were identified as possible sources of dust. The green contours in Fig. 2 represent the assigned dust origin for each sampled event based on the reanalysis data. Note that in two events, there was no overlap between the dust origin and air mass trajectories. These events will be further discussed below. Two events, denoted by SDS1 and SDS2, originated in the northern Sahara. The source of SDS1 was near the border of Egypt and Libya, and the source of SDS2 was in Egypt, east of SDS1. The dust traveled over the Mediterranean Sea and was potentially affected by the marine environment, possibly obtaining a sea salt or anthropogenic sulfate coating (Levin et al., 1996). Two other events, denoted by SyDS1 and SyDS2, originated from the Syrian Desert, from western Iraq and southern Syria. Compared to the Saharan events, the dust mass density in the Syrian Desert events was relatively low.
Another event was defined as a “mixed dust” event (MDS) because it was more complicated and included contributions of different sources: the analysis indicates that there is one possible dust origin east of the sampling site in the Syrian Desert and another one southwest of the sampling site in the Sahara. However, the air mass trajectories did not overlap the Saharan dust origin but indicated that the air mass was transported from the Red Sea. Further analysis of the air mass trajectories prior to the sampling period in the Red Sea showed that both Sahara and Arabia dusts were transported to the Red Sea (see the Supplement, Fig. S2a). Another event did not show overlap between the air mass trajectories and the dust origin. Further analysis of air mass back trajectories in the days prior to the sampling period showed that dust was transported to the Mediterranean Sea from the region of Libya in the Sahara, towards Turkey, and was deflected eastward by westerly winds to the sampling site (see the Supplement, Fig. S2b). The dusty air masses rapidly cleared up, and relatively non-dusty air masses arrived at the sampling site, as inferred from PM10 concentrations and the OPC size distributions; see Sect. 3.2. This event was defined as a “clean and Saharan dust storm” and is denoted by CSDS. Table 1 summarizes the sampled events, their sampling periods, and the peak and mean PM10 concentrations during sampling. Peak values ranged from 67 µg m−3 in CSDS and 132 µg m−3 in SyDS1 to 717 µg m−3 in SDS2, which was the strongest dust event in this study. In SDS1, MDS and SyDS2, the values ranged between ∼300 and 400 µg m−3. When comparing the mean PM10 concentrations during the entire sampling periods, CSDS was categorized as a non-dusty event, with the lowest concentrations of 30±13 µg m−3, i.e., below the threshold of 42 µg m−3 for dusty conditions (Krasnov et al., 2014). The mean values in the rest of the events ranged from 76 to 206 µg m−3, and they were therefore categorized as dust storms.
3.2 Particle number size distributions
Figure 3a describes the mean particle number size distributions of sampled air during the dust events, as detected by the GRIMM OPC. The lowest channel of the GRIMM includes particles that are larger than 0.25 µm. This channel possibly underestimates the total particle count since the counting efficiency is less than 100 %.
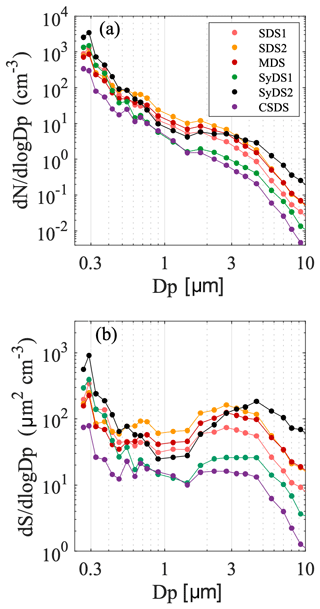
Figure 3Particle size distributions. Particle number size (a) and surface area size (b) distributions averaged over the entire sampling periods of the events as monitored by GRIMM OPC during the studied events. Dp is the diameter of the particles and set at the center of each GRIMM channel.
The number size distributions had similar patterns in all the events. The highest particle number concentrations were in the submicron size range, decreasing towards larger particles. Events SDS1, SDS2 and MDS had a rather similar particle concentration distribution. Event SyDS1 showed similar particle concentrations in the submicron range, but the particle concentrations in the supermicron range were about an order of magnitude lower, which was also apparent in the PM10 data. CSDS, a predominantly non-dusty event, had the lowest particle concentrations in comparison to the rest of the sampled events, as also indicated by the PM10 data. In the SyDS2 event, exceptionally high concentrations in the supermicron range above 3 µm were observed, and the peak extended towards larger particle sizes combined with relatively high particle concentrations. Note that prior to and during this event, a series of biomass burning events occurred in Israel extending to about 100 km north and 50 km east of the sampling site. Therefore, this peak may also include contributions from biomass burning particles. This is further supported by the SEM-EDX analysis of the filters from this event, which in comparison with the other events contained super-aggregates in the supermicron range, typically observed in biomass burning emissions (Chakrabarty et al., 2014), with distinct morphologies and elemental composition (shown in the Supplement in Fig. S3).
The surface area size distributions shown in Fig. 3b compare the contribution of supermicron and submicron particles to the available ambient surface area. Ice nucleation initiated on the surface of the particles, and therefore their surface area concentration is an important parameter in addition to number concentrations. Here it is clearly seen that the potential contribution of supermicron particles to ice nucleation may be significant when compared to the submicron particles, although their number concentrations were up to 2 orders of magnitude lower.
3.3 Airborne INP concentrations
The cumulative INP concentration spectra for the six dust events are shown in Fig. 4. In each event, different particle size classes are marked by different colors. Freezing was observed between 255 and 238 K, and the INP concentrations spanned 4 orders of magnitude from 10−1 to 103 L−1 of air.
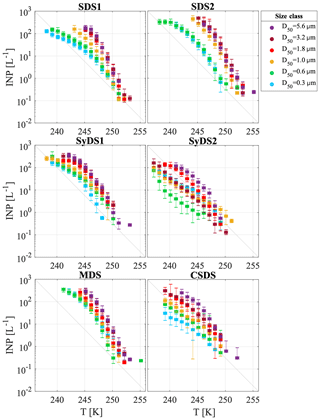
Figure 4Airborne INP concentrations measured during dust events. INP concentrations per liter of air as a function of temperature are presented in different colors for the different particle size classes. Uncertainty in temperature is 0.3 K. The grey diagonal line is presented for orientation only.
A particle size dependence of the freezing temperature and INP concentration was observed. Larger particles froze at warmer temperatures with a higher number of INPs. The variation between the six size classes ranged from 1 to 2 orders of magnitude, and in some cases the smallest particles had behavior similar to the large ones. For example, in event SDS2, size classes D50=0.6 µm and D50=0.3 µm were less ice-active than the rest of the size classes, while in MDS, all size classes showed similar activity. As an exception, event SyDS2 showed a weaker size dependence in comparison to the other dust events and in some size classes lower INP concentrations. In comparison, in the relatively non-dusty event CSDS, the variability between the different size classes was higher, especially at lower temperatures. In Fig. 5, similar to Fig. 4, INP concentrations are presented but arranged according to the different size classes. The variability within each size class was relatively high and spans over 2 orders of magnitude; for example, at size class D50=0.3 µm near 245 K, the INP concentration ranged from about 1 to almost 102 L−1 of air. It is clearly seen that INP concentrations in dusty conditions (SDS1, SDS2, MDS and SyDS1) were higher than in non-dusty conditions (CSDS) for the supermicron range but similar in the submicron range. Previous studies also pointed out the significant contribution of supermicron particles to the INP population. Mason et al. (2016) studied the immersion freezing abilities of airborne particles in North America and Europe and found that supermicron particles dominated the freezing, especially at relatively high temperature (258 K). Recent measurements in a coastal tropical site conducted by Ladino et al. (2019) also found high concentrations of INPs at relatively high temperatures (>258 K) due to supermicron particles. In these studies, however, mineral dust is not expected to dominate the samples, and bioaerosol particles are thought to dominate the freezing at high temperatures (>258 K). At lower temperatures (below 253 K), Ladino et al. (2019) suggested that mineral dust dominated the freezing. Moreover, DeMott et al. (2010) found that INP concentrations are correlated with particles >0.5 µm. Other studies, such as Rosinski et al. (1986) and Huffman et al. (2013), also found that supermicron particles were responsible for most of the INP population in some cases, while when changing the freezing mode that was analyzed or the measurement meteorological conditions, their contribution was reduced. Vali (1966), in contrast, found that submicron particles dominate freezing in hail melt samples.
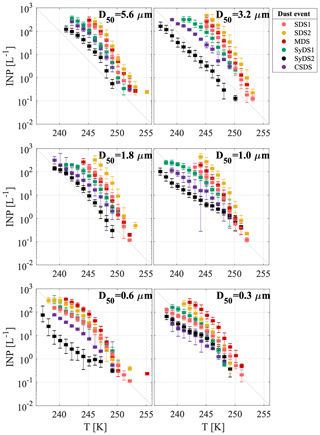
Figure 5Airborne INP concentrations for various size classes. INP concentrations per liter of air as a function of temperature are presented in different colors for the different dust events that were sampled. Uncertainty in temperature is 0.3 K. The grey diagonal line is presented for orientation only.
3.4 Size dependence of ice active site density (ns(T))
Figure 6 presents the ns(T) curves for the different dust events spanning a range of 106 m−2 at 253 K to 1011 m−2 at 238 K. In general, ns(T) increased with particle size. The highest ns values were observed in the supermicron range D50=5.6 µm, followed by D50=3.2, 1.8 and 1.0 µm. The activity of the latter three classes was similar within measurement uncertainties. In the submicron range, stages D50=0.6 and 0.3 µm, the ns(T) values were lower than in the supermicron range and showed higher variability between the different events, except for the MDS event, which had similar activity in the submicron and the supermicron range. While INP concentrations may generally vary due to experimental parameters, such as particle concentration in the droplet or droplet size, ns(T) accounts for these differences since it is normalized by the total surface area of particles immersed in the droplet. Therefore, the effect of particle size diminishes using the ns(T) curves if the particles' ice nucleation ability is indeed similar. Hence, the analysis presented in Fig. 6 indicates that the supermicron particles are better INPs than the submicron ones, implying they have more active sites and/or active sites that nucleate ice at higher temperatures.
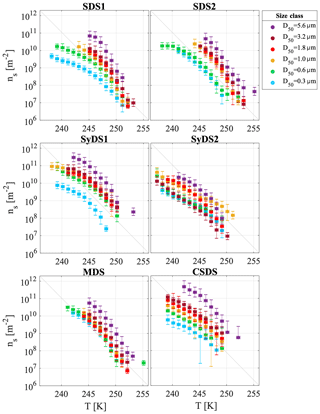
Figure 6Ice-active site density as a function of temperature, ns(T), for airborne particles dominated by mineral dust are presented individually for each dust event. The different colors represent the different size classes that were investigated. SDS, SyDS and MDS represent Saharan, Syrian and mixed dust events, respectively (see text for more details). The linear grey line is identical in each panel to facilitate comparison.
Figure 7 displays the same ns(T) curves as Fig. 6 but now arranged according to the different size classes. It is observed that in the supermicron range, all ns(T) curves from the different events merge (with the exception of SyDS2), suggesting that freezing was dominated by a common component. While the freezing activity decreases with decreasing particle size, the shape of the curves is preserved, suggesting that the abundance of this common component decreases with particle size. One possible explanation for this observation may be mineralogy segregation, known to occur with particle size: larger particles contain more primary minerals, such as K feldspar, whereas smaller particles contain more secondary minerals, such as clays and quartz that are common in all particle sizes (Perlwitz et al., 2015; Claquin et al., 1999). Therefore, the reduced activity in the submicron range and the higher variability between the dust events, especially at D50=0.3 µm, may be attributed to a different mineralogical composition of the particles or to the lack of the important ice-inducing component. Alternatively, it is also possible that the submicron particles are mixed with other particle types that are more common in this size range, such as urban pollution (Li et al., 2016), and therefore freezing may not be dominated exclusively by mineral dust. Moreover, due to their larger surface-to-volume ratio, submicron particles are more sensitive to atmospheric processing than supermicron particles, which can lead to further deactivation of their ice-active sites (Boose et al., 2016a). These considerations may explain the variability in the activity between different events. For example, we propose that the passage of SDS1 and SDS2 over the Mediterranean Sea can contribute to their reduced activity in the submicron range, while for the MDS event, a shorter and relatively direct transport path resulted in less atmospheric processing. Although speculative, these considerations may possibly explain why the freezing activity of submicron particles converged with those of the supermicron particles, but we acknowledge that further measurements are needed to confirm these suggestions.
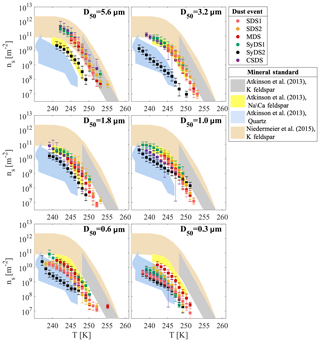
Figure 7Ice-active site density during dust events in different particle size classes. Dust events from the Sahara (SDS), Syrian Desert (SyDS) or both (MDS) are marked by the different colors. Data for D50=3.2, 1.8 and 1.0 µm of SDS no. 2 adopted from Reicher et al. (2018). Relevant standard minerals scaled to ambient values are shown: K feldspar, Na–Ca feldspar and quartz from Atkinson et al. (2013), as well as K feldspar from Niedermeier et al. (2015).
In Fig. 7, we also compare a few relevant ns(T) curves of standard minerals, as derived by Atkinson et al. (2013) and Niedermeier et al. (2015), together with our measured ns(T) curves. The standard curves of K feldspar, Na–Ca feldspar and quartz were scaled to the estimated fraction of these minerals in AMD (see Table S1) and are typically used for the prediction of AMD ice-nucleating activity. A good agreement of the absolute ns values was observed in the relevant temperature range, and the slopes of the curves were similar to those of the feldspars, especially for the supermicron range. A good agreement was also observed with the standard ns(T) curve of quartz, suggesting that it contributes to the freezing of submicron particles in the lower temperature range. Note that the standard ns(T) curves of clay minerals and calcite were not plotted here despite their large abundance in AMD because there was no overlap with the ice nucleation activity in this study. Only the freezing activity of the largest particles (D50=5.6 µm) overlapped the K feldspar prediction of Atkinson et al. (2013), indicating that this prediction possibly overestimates the freezing activity of the entire size distribution of AMD. For the particles in the size range of µm, there is an overlap in activity with the K feldspar prediction of Niedermeier et al. (2015) and Na–Ca feldspar of Atkinson et al. (2013). However, in all cases, the feldspar predictions overestimate the freezing activity of AMD in the submicron range.
The ns(T) curves of SyDS2 display moderate slopes and lower ice nucleation activity in comparison with the other dust events in all size classes, except for the smallest particles with D50=0.3 µm. As already mentioned, these particles were most likely mixed with smoke particles from biomass burning events that occurred during the same period, and the filters from this event were covered with super-aggregate particles in the supermicron size, rich with potassium, similar to particles seen in other biomass burning events (Chakrabarty et al., 2014).
3.5 Comparison of WISDOM and BINARY measurements for event CSDS
A complementary analysis for the CSDS event using BINARY is shown in Fig. 8. BINARY probes droplets with larger volumes and it is thus more sensitive to less common ice-nucleating sites that may not show a signal in WISDOM. In the BINARY experiments, two suspensions were tested with different dilution factors to extend our sensitivity. The higher total dust surface area per droplet sample that was investigated in the BINARY experiments, the yellow markers in Fig. 8, demonstrates the warmest freezing temperatures ranging from 255 to 246 K, and the ns(T) values ranged from 106 to 109 m−2. The 1:10 diluted samples (purple markers) showed freezing at lower temperatures, ranging from about 251 to 244 K, with higher ns(T) values ranging from 108 to 1011 m−2. In some of the dilute cases of the BINARY experiments, the data were at the limit of the background impurities (see the Supplement, Fig. S5). In order to include only data that are significantly different from the background, a criterion was set in which only data points that are larger by at least 2 standard deviations than the mean background impurities were further considered in Fig. 8. If data were below that threshold, they were considered not significant and were thus removed (e.g., the data for D50=0.6 and 0.3 µm for the diluted BINARY samples).
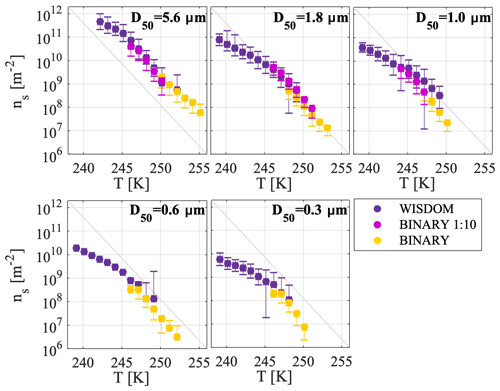
Figure 8Complementary measurements of WISDOM and BINARY for CSDS. Analysis in BINARY was performed to increase the detection sensitivity of ice-active site densities. Two suspensions with different dilution factors were analyzed by BINARY and are compared here to the WISDOM data for the different size classes.
Figure 8 shows a very good agreement between the BINARY and WISDOM data because the ns(T) curves merged nicely onto each other for each size class. Whereas BINARY was more sensitive than WISDOM to the warmer and relatively rare active sites, WISDOM detected the more common active sites in the low temperature range. Overall, the dependence of the freezing activity temperature range on the immersed surface area per droplet is well demonstrated here, whereby a reduction in the surface area of the different experiments (WISDOM < BINARY diluted < BINARY) decreased the probability to observe freezing at higher temperatures. This was also previously demonstrated in studies of standard mineral dust (Broadley et al., 2012; Marcolli et al., 2007; Reicher et al., 2018). Overall, the data shown in Fig. 8 indicate the added value when using experimental techniques of different sensitivity for the purpose of measuring the concentration and active site density of INPs in field studies (e.g., Atkinson et al., 2013; Chen et al., 2018; Harrison et al., 2018).
3.6 Comparison of supermicron and submicron ranges with AMD measurements and predictions
The particle surface area that was used to derive ns(T) represents the total airborne particles that were collected for each sample, regardless of particle composition. When mineral dust dominated the composition, as in a dust event case (see, for example, Fig. S4 in the Supplement), we treat ns(T) as representative for AMD freezing. Figure 9a compiles the ns(T) results of AMD from a few recent studies that focused on airborne particles (albeit not size-selected) during dust events. Results from our current study, excluding the events SyDS2 and CSDS that were not dominated by AMD, are presented alongside those of Price et al. (2018) and Boose et al. (2016b). Price et al. (2018) collected airborne particles in flights west of the Sahara over the tropical Atlantic at altitudes up to 3.5 km. Boose et al. (2016b) analyzed airborne particles that were deposited in the eastern Mediterranean region in Egypt, Cyprus and the Peloponnese (Greece) during dust events. Boose et al. (2016b) also sampled airborne particles during dust events over Tenerife, off West Africa. In addition, we present measurements that were also conducted in the eastern Mediterranean region in Cyprus. Schrod et al. (2017) measured INPs in the lower troposphere using an unmanned aircraft system, and Gong et al. (2019) measured INPs at ground level. Both studies measured the immersion freezing of the sampled particles during different atmospheric conditions that included a few dust plumes from the Sahara. Note that we present only immersion freezing measurements by Schrod et al. (2017) here and not all the data. Also note that the presented data are not necessarily dominated by mineral dust, in contrast to the current study, Price et al. (2018) and Boose et al. (2016b). The specific cases in which the samples were taken during the passage of dust plumes and are possibly dominated by mineral dust are marked in Fig. 9a in green for Schrod et al. (2017) and cyan for Gong et al. (2019). The supermicron data presented in this paper are about 1 to 2 orders of magnitude higher, while our submicron data are in relatively good agreement with Schrod et al. (2017), except for the lowest temperature (243 K) points at which 1 to 3 orders of magnitude differences were observed. The Gong et al. (2019) data are lower by 1 to 3 orders of magnitude, but there is some overlap with this study and with Price et al. (2018).
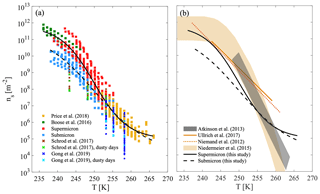
Figure 9Heterogeneous ice nucleation by airborne particles during dusty conditions. (a) Active site densities of supermicron and submicron size classes from this study are shown together with flight data (Schrod et al., 2017, and Price et al., 2018) and deposited or in situ data (Boose et al., 2016b, and Gong et al., 2019). New parameterizations, which were derived in this work based on the combined AMD data of the different studies, are shown for supermicron and submicron classes. (b) The new parameterizations derived in this study based on all AMD data are shown next to recent parameterizations for desert dust (Ullrich et al., 2017, and Niemand et al., 2012) and K feldspar predictions (Atkinson et al., 2013 and Niedermeier et al., 2015).
This compilation of the data that were dominated by mineral dust (i.e., this study, Price et al., 2018, and Boose et al., 2016b) shows that ns(T) curves from the different studies exhibit great similarities over a wide range of temperatures (236–265 K) for dust from different locations and geographic sources, with varying atmospheric paths and altitudes. This similarity may have significant implications for modeling ice nucleation activity by AMD, since it suggests that parameterizations can be simplified, for example, by neglecting the complication of accounting for the mineralogy of different geographical sources. Due to the different behavior of submicron and supermicron particles, we also suggest that accounting for the particle size class will improve the prediction of ice cloud formation. For that purpose, we derived two basic parameterizations (Eq. 4), for supermicron and submicron particles, based on the combined AMD data (including data from this study, Price et al., 2018, and Boose et al., 2016b, and excluding SyDS2), which cover a wide range of temperatures and spread more than 5 orders of magnitudes in ns(T) values. These parameterizations are the best mathematical fit for a Hill-type equation, which is normally used for fitting S-shaped data as they are observed in this compilation:
where the coefficients (95 % confidence bounds) for supermicron range particles are set to
Those for the submicron range are
Parameterizations for each individual size class can be found in Table S2 in the Supplement.
In Fig. 9b, the parameterizations derived here are presented next to the recent parameterizations of the ice nucleation of desert dust by Ullrich et al. (2017) and Niemand et al. (2012). These parameterizations are predominantly based on natural surface-collected dust samples, but they also contained one sample of AMD from Israel and agree within an order of magnitude with our supermicron data in the low temperature range (243–247 K). However, they overpredict ns(T) by more than an order of magnitude when compared to our submicron data and to the Price et al. (2018) data at warmer temperatures (247–259 K). This emphasizes that AMD ice nucleation may not be correctly represented when based on desert dust sampled from the surface, consistent with the conclusions of Boose et al. (2016b), who showed that the average freezing activity of AMD is reduced when compared to the activity of surface-collected desert dust. K feldspar parameterizations by Atkinson et al. (2013) and Niedermeier et al. (2015) are also shown here and, as mentioned before, overpredict the freezing activity of AMD at temperatures lower than about 255 K.
We characterized the INP activity of particles collected during several mineral dust events in the eastern Mediterranean. Dust from the Sahara, the major source for atmospheric dust, together with dust from the Arabian and Syrian deserts were included. Six size classes were studied that cover both the supermicron and submicron size ranges. The INP concentrations ranged from 10−1 L−1 of air in the relatively weak dust events to 103 L−1 of air in the strongest event. The ns values ranged from 106 to 1011 m−2 in the temperature range of 238–255 K. A size dependence was observed in both the INP concentration and ns values. Larger particles were more active INPs, exhibiting higher INP concentrations and a higher number of nucleating sites per surface area at higher temperatures. Comparison between freezing results of WISDOM with BINARY showed good agreement and strengthened previous studies that observed how freezing activity could depend on the technical properties and limitations of the instrumentation used, thereby emphasizing the importance of using complementary instruments.
The dust events studied here represent a range of dust loads, different dust origins and atmospheric paths. Yet, the supermicron particles in these events exhibited similar freezing abilities. This may indicate that there is a unique component that is responsible for freezing activity, as was previously suggested (Atkinson et al., 2013; Boose et al., 2016b; Kaufmann et al., 2016; Price et al., 2018). Our measurements showed that the activity of the supermicron particles was in the range of standard particles of feldspar mineral and that the activity of the submicron particles was in the range of standard quartz. Therefore, we suggest that these may be the two most important components that dominate freezing by atmospheric mineral dust (AMD) and may therefore be important for heterogeneous ice nucleation in atmospheric clouds. The submicron particles showed higher variability between events, possibly due to different composition of the particles or higher sensitivity to atmospheric processing during long-range transport. In general, supermicron particles contributed the most to the INP concentration, in agreement with other previous studies (Mason et al., 2016; Huffman et al., 2013; Ladino et al., 2019). However, our current study is probably the only case in which mineral dust dominated the samples. Nevertheless, all of these studies highlight the importance of the supermicron size class of AMD for atmospheric ice nucleation.
Mineral dust is important both on a regional scale near its source region and on a global scale, since it remains ice-active even after long transport in the atmosphere and thus over considerable distances (DeMott et al., 2003b; Chou et al., 2011). With distance from the dust source, supermicron particles will settle, and submicron particles may then dominate ice nucleation on the global scale (Ryder et al., 2013; Murray et al., 2012). However, recent airborne measurements found coarse and giant particles in the vicinity of and also far from source regions (Ryder et al., 2018). Therefore, including the particle size class in INP parameterizations can improve predictions of ice formation in clouds. Moreover, information on airborne INP size distributions may be helpful in identifying the dominant INP sources (Mason et al., 2016). The overprediction of AMD freezing ability demonstrated in this study, as well as by the Atkinson et al. (2013), Niedermeier et al. (2015), Niemand et al. (2012) and Ullrich et al. (2017) parameterizations, especially for submicron particles, emphasizes the importance of future studies to better quantify the changes in the ice-nucleating properties of AMD by atmospheric processing.
Data are available upon request to the first author.
The supplement related to this article is available online at: https://doi.org/10.5194/acp-19-11143-2019-supplement.
NR and YR designed the experiments, carried out the field measurements, conducted freezing experiments in WISDOM and wrote the paper. CB, LE and TK designed and performed freezing experiments in BINARY. SRR performed back trajectory analyses. NR and IKA performed the chemical analyses of filters. All authors contributed to the discussion, analysis of data and the writing of the paper.
The authors declare that they have no conflict of interest.
This article is part of the special issue “Fifth International Workshop on Ice Nucleation (FIN)”. It is not associated with a conference.
This study was funded by the Israel Science Foundation (grant no. 236/16). The authors are grateful for funding from the German Research Foundation (DFG) through the research unit FOR 1525 (INUIT) under KO 2944/2-2 for Carsten Budke and Thomas Koop, as well as a Mercator Fellowship for Yinon Rudich; the authors acknowledge support from the Helen Kimmel Center for Planetary Sciences and the de Botton Center for Marine Sciences. Analyses and visualizations of MERRA data in this study were produced with the Giovanni online data system, developed and maintained by the NASA GES DISC (http://giovanni.sci.gsfc.nasa.gov/Giovanni, last access: August 2018). PM10 data are available from the Israel Ministry of Environmental Protection website (http://www.svivaaqm.net/Default.rtl.aspx, last access: August 2018). Other data used in this study can be retrieved from https://osf.io/gpuqt (last access: August 2018).
This research has been supported by the Israel Science Foundation (grant no. 236/16), the German Research Foundation (DFG) through the research unit FOR 1525 (INUIT grant no. KO 2944/2-2), the Helen Kimmel Center for Planetary Sciences and the de Botton Center for Marine Sciences.
This paper was edited by Allan Bertram and reviewed by two anonymous referees.
Anderson, B. and Hallett, J.: Supersaturation and time dependence of ice nucleation from the vapor on single crystal substrates, J. Atmos. Sci., 33, 822–832, 1976.
Ansmann, A., Tesche, M., Althausen, D., Müller, D., Seifert, P., Freudenthaler, V., Heese, B., Wiegner, M., Pisani, G., Knippertz, P., and Dubovik, O.: Influence of Saharan dust on cloud glaciation in southern Morocco during the Saharan Mineral Dust Experiment, J. Geophys. Res.-Atmos., 113, D04210, https://doi.org/10.1029/2007JD008785, 2008.
Archuleta, C. M., DeMott, P. J., and Kreidenweis, S. M.: Ice nucleation by surrogates for atmospheric mineral dust and mineral dust/sulfate particles at cirrus temperatures, Atmos. Chem. Phys., 5, 2617–2634, https://doi.org/10.5194/acp-5-2617-2005, 2005.
Ardon-Dryer, K. and Levin, Z.: Ground-based measurements of immersion freezing in the eastern Mediterranean, Atmos. Chem. Phys., 14, 5217–5231, https://doi.org/10.5194/acp-14-5217-2014, 2014.
Atkinson, J. D., Murray, B. J., Woodhouse, M. T., Whale, T. F., Baustian, K. J., Carslaw, K. S., Dobbie, S., O'Sullivan, D., and Malkin, T. L.: The importance of feldspar for ice nucleation by mineral dust in mixed-phase clouds, Nature, 498, 355–358, https://doi.org/10.1038/nature12278, 2013.
Augustin-Bauditz, S., Wex, H., Kanter, S., Ebert, M., Niedermeier, D., Stolz, F., Prager, A., and Stratmann, F.: The immersion mode ice nucleation behavior of mineral dusts: A comparison of different pure and surface modified dusts, Geophys. Res. Lett., 41, 7375–7382, 2014.
Ben-Ami, Y., Koren, I., Rudich, Y., Artaxo, P., Martin, S. T., and Andreae, M. O.: Transport of North African dust from the Bodélé depression to the Amazon Basin: a case study, Atmos. Chem. Phys., 10, 7533–7544, https://doi.org/10.5194/acp-10-7533-2010, 2010.
Boose, Y., Sierau, B., García, M. I., Rodríguez, S., Alastuey, A., Linke, C., Schnaiter, M., Kupiszewski, P., Kanji, Z. A., and Lohmann, U.: Ice nucleating particles in the Saharan Air Layer, Atmos. Chem. Phys., 16, 9067–9087, https://doi.org/10.5194/acp-16-9067-2016, 2016a.
Boose, Y., Welti, A., Atkinson, J., Ramelli, F., Danielczok, A., Bingemer, H. G., Plötze, M., Sierau, B., Kanji, Z. A., and Lohmann, U.: Heterogeneous ice nucleation on dust particles sourced from nine deserts worldwide – Part 1: Immersion freezing, Atmos. Chem. Phys., 16, 15075–15095, https://doi.org/10.5194/acp-16-15075-2016, 2016b.
Broadley, S. L., Murray, B. J., Herbert, R. J., Atkinson, J. D., Dobbie, S., Malkin, T. L., Condliffe, E., and Neve, L.: Immersion mode heterogeneous ice nucleation by an illite rich powder representative of atmospheric mineral dust, Atmos. Chem. Phys., 12, 287–307, https://doi.org/10.5194/acp-12-287-2012, 2012.
Budke, C. and Koop, T.: BINARY: an optical freezing array for assessing temperature and time dependence of heterogeneous ice nucleation, Atmos. Meas. Tech., 8, 689–703, https://doi.org/10.5194/amt-8-689-2015, 2015.
Cantrell, W. and Heymsfield, A.: Production of Ice in Tropospheric Clouds: A Review, B. Am. Meteorol. Soc., 86, 795–807, https://doi.org/10.1175/bams-86-6-795, 2005.
Chakrabarty, R. K., Beres, N. D., Moosmüller, H., China, S., Mazzoleni, C., Dubey, M. K., Liu, L., and Mishchenko, M. I.: Soot superaggregates from flaming wildfires and their direct radiative forcing, Sci. Rep.-UK, 4, 5508, https://doi.org/10.1038/srep05508, 2014.
Chen, J., Pei, X., Wang, H., Chen, J., Zhu, Y., Tang, M., and Wu, Z.: Development, Characterization, and Validation of a Cold Stage-Based Ice Nucleation Array (PKU-INA), Atmosphere, 9, 357, https://doi.org/10.3390/atmos9090357, 2018.
Chiapello, I., Bergametti, G., Chatenet, B., Dulac, F., Jankowiak, I., Liousse, C., and Soares, E. S.: Contribution of the different aerosol species to the aerosol mass load and optical depth over the northeastern tropical Atlantic, J. Geophys. Res.-Atmos., 104, 4025–4035, https://doi.org/10.1029/1998JD200044, 1999.
Chou, C., Stetzer, O., Weingartner, E., Jurányi, Z., Kanji, Z. A., and Lohmann, U.: Ice nuclei properties within a Saharan dust event at the Jungfraujoch in the Swiss Alps, Atmos. Chem. Phys., 11, 4725–4738, https://doi.org/10.5194/acp-11-4725-2011, 2011.
Claquin, T., Schulz, M., and Balkanski, Y.: Modeling the mineralogy of atmospheric dust sources, J. Geophys. Res.-Atmos., 104, 22243–22256, 1999.
Connolly, P. J., Möhler, O., Field, P. R., Saathoff, H., Burgess, R., Choularton, T., and Gallagher, M.: Studies of heterogeneous freezing by three different desert dust samples, Atmos. Chem. Phys., 9, 2805–2824, https://doi.org/10.5194/acp-9-2805-2009, 2009.
Cziczo, D., Murphy, D., Hudson, P., and Thomson, D.: Single particle measurements of the chemical composition of cirrus ice residue during CRYSTAL-FACE, J. Geophys. Res.-Atmos., 109, D04201, https://doi.org/10.1029/2003JD004032, 2004.
Cziczo, D. J. and Froyd, K. D.: Sampling the composition of cirrus ice residuals, Atmos. Res., 142, 15–31, 2014.
Cziczo, D. J., Garimella, S., Raddatz, M., Hoehler, K., Schnaiter, M., Saathoff, H., Moehler, O., Abbatt, J. P., and Ladino, L. A.: Ice nucleation by surrogates of Martian mineral dust: What can we learn about Mars without leaving Earth?, J. Geophys. Res.-Planet., 118, 1945–1954, 2013.
Dayan, U.: Climatology of Back Trajectories from Israel Based on Synoptic Analysis, J. Clim. Appl. Meteorol., 25, 591–595, https://doi.org/10.1175/1520-0450(1986)025<0591:cobtfi>2.0.co;2, 1986.
Dayan, U., Heffter, J., Miller, J., and Gutman, G.: Dust intrusion events into the Mediterranean basin, J. Appl. Meteorol., 30, 1185–1199, 1991.
Dee, D. P., Uppala, S., Simmons, A., Berrisford, P., Poli, P., Kobayashi, S., Andrae, U., Balmaseda, M., Balsamo, G., and Bauer, d. P.: The ERA-Interim reanalysis: Configuration and performance of the data assimilation system, Q. J. Roy. Meteor. Soc., 137, 553–597, 2011.
DeMott, P. J., Cziczo, D., Prenni, A., Murphy, D., Kreidenweis, S., Thomson, D., Borys, R., and Rogers, D.: Measurements of the concentration and composition of nuclei for cirrus formation, P. Natl. Acad. Sci., 100, 14655–14660, 2003a.
DeMott, P. J., Sassen, K., Poellot, M. R., Baumgardner, D., Rogers, D. C., Brooks, S. D., Prenni, A. J., and Kreidenweis, S. M.: African dust aerosols as atmospheric ice nuclei, Geophys. Res. Lett., 30, 1732, https://doi.org/10.1029/2003GL017410, 2003b.
DeMott, P. J., Prenni, A. J., Liu, X., Kreidenweis, S. M., Petters, M. D., Twohy, C. H., Richardson, M. S., Eidhammer, T., and Rogers, D. C.: Predicting global atmospheric ice nuclei distributions and their impacts on climate, P. Natl. Acad. Sci. USA, 107, 11217–11222, https://doi.org/10.1073/pnas.0910818107, 2010.
Engelbrecht, J. P., McDonald, E. V., Gillies, J. A., “Jay” Jayanty, R., Casuccio, G., and Gertler, A. W.: Characterizing mineral dusts and other aerosols from the Middle East – Part 2: Grab samples and re-suspensions, Inhal. Toxicol., 21, 327–336, 2009.
Falkovich, A. H., Schkolnik, G., Ganor, E., and Rudich, Y.: Adsorption of organic compounds pertinent to urban environments onto mineral dust particles, J. Geophys. Res.-Atmos., 109, D02208, https://doi.org/10.1029/2003JD003919, 2004.
Fletcher, N.: Active sites and ice crystal nucleation, J. Atmos. Sci., 26, 1266–1271, 1969.
Freedman, M. A.: Potential sites for ice nucleation on aluminosilicate clay minerals and related materials, J. Phys. Chem. Lett., 6, 3850–3858, 2015.
Ganor, E.: The frequency of Saharan dust episodes over Tel Aviv, Israel, Atmos. Environ., 28, 2867–2871, 1994.
Ganor, E. and Mamane, Y.: Transport of Saharan dust across the eastern Mediterranean, Atmos. Environ., 16, 581–587, 1982.
Ganor, E., Foner, H. A., Brenner, S., Neeman, E., and Lavi, N.: The chemical composition of aerosols settling in Israel following dust storms, Atmos. Environ. A-Gen., 25, 2665–2670, https://doi.org/10.1016/0960-1686(91)90196-E, 1991.
Ganor, E., Osetinsky, I., Stupp, A., and Alpert, P.: Increasing trend of African dust, over 49 years, in the eastern Mediterranean, J. Geophys. Res.-Atmos., 115, D07201, https://doi.org/10.1029/2009JD012500, 2010.
Garrison, V. H., Shinn, E. A., Foreman, W. T., Griffin, D. W., Holmes, C. W., Kellogg, C. A., Majewski, M. S., Richardson, L. L., Ritchie, K. B., and Smith, G. W.: African and Asian Dust: From Desert Soils to Coral Reefs, BioScience, 53, 469–480, https://doi.org/10.1641/0006-3568(2003)053[0469:AAADFD]2.0.CO;2, 2003.
Gat, D., Mazar, Y., Cytryn, E., and Rudich, Y.: Origin-Dependent Variations in the Atmospheric Microbiome Community in Eastern Mediterranean Dust Storms, Environ. Sci. Technol., 51, 6709–6718, https://doi.org/10.1021/acs.est.7b00362, 2017.
Gelaro, R., McCarty, W., Suárez, M. J., Todling, R., Molod, A., Takacs, L., Randles, C. A., Darmenov, A., Bosilovich, M. G., and Reichle, R.: The modern-era retrospective analysis for research and applications, version 2 (MERRA-2), J. Climate, 30, 5419–5454, 2017.
Gettelman, A., Liu, X., Barahona, D., Lohmann, U., and Chen, C.: Climate impacts of ice nucleation, J. Geophys. Res.-Atmos., 117, D20201, https://doi.org/10.1029/2012JD017950, 2012.
Gong, X., Wex, H., Müller, T., Wiedensohler, A., Höhler, K., Kandler, K., Ma, N., Dietel, B., Schiebel, T., Möhler, O., and Stratmann, F.: Characterization of aerosol properties at Cyprus, focusing on cloud condensation nuclei and ice nucleating particles, Atmos. Chem. Phys. Discuss., https://doi.org/10.5194/acp-2019-198, in review, 2019.
Hader, J. D., Wright, T. P., and Petters, M. D.: Contribution of pollen to atmospheric ice nuclei concentrations, Atmos. Chem. Phys., 14, 5433–5449, https://doi.org/10.5194/acp-14-5433-2014, 2014.
Harrison, A. D., Whale, T. F., Rutledge, R., Lamb, S., Tarn, M. D., Porter, G. C. E., Adams, M. P., McQuaid, J. B., Morris, G. J., and Murray, B. J.: An instrument for quantifying heterogeneous ice nucleation in multiwell plates using infrared emissions to detect freezing, Atmos. Meas. Tech., 11, 5629–5641, https://doi.org/10.5194/amt-11-5629-2018, 2018.
Hoose, C. and Möhler, O.: Heterogeneous ice nucleation on atmospheric aerosols: a review of results from laboratory experiments, Atmos. Chem. Phys., 12, 9817–9854, https://doi.org/10.5194/acp-12-9817-2012, 2012.
Huffman, J. A., Prenni, A. J., DeMott, P. J., Pöhlker, C., Mason, R. H., Robinson, N. H., Fröhlich-Nowoisky, J., Tobo, Y., Després, V. R., Garcia, E., Gochis, D. J., Harris, E., Müller-Germann, I., Ruzene, C., Schmer, B., Sinha, B., Day, D. A., Andreae, M. O., Jimenez, J. L., Gallagher, M., Kreidenweis, S. M., Bertram, A. K., and Pöschl, U.: High concentrations of biological aerosol particles and ice nuclei during and after rain, Atmos. Chem. Phys., 13, 6151–6164, https://doi.org/10.5194/acp-13-6151-2013, 2013.
IPCC: Climate Change 2013, The Physical Science Basis, Cambridge University Press, Cambridge, UK and New York, NY, USA, 2013.
Jickells, T. D., An, Z. S., Andersen, K. K., Baker, A. R., Bergametti, G., Brooks, N., Cao, J. J., Boyd, P. W., Duce, R. A., Hunter, K. A., Kawahata, H., Kubilay, N., laRoche, J., Liss, P. S., Mahowald, N., Prospero, J. M., Ridgwell, A. J., Tegen, I., and Torres, R.: Global Iron Connections Between Desert Dust, Ocean Biogeochemistry, and Climate, Science, 308, 67–71, https://doi.org/10.1126/science.1105959, 2005.
Kalderon-Asael, B., Erel, Y., Sandler, A., and Dayan, U.: Mineralogical and chemical characterization of suspended atmospheric particles over the east Mediterranean based on synoptic-scale circulation patterns, Atmos. Environ., 43, 3963–3970, https://doi.org/10.1016/j.atmosenv.2009.03.057, 2009.
Kandler, K., Benker, N., Bundke, U., Cuevas, E., Ebert, M., Knippertz, P., Rodríguez, S., Schütz, L., and Weinbruch, S.: Chemical composition and complex refractive index of Saharan Mineral Dust at Izana, Tenerife (Spain) derived by electron microscopy, Atmos. Environ., 41, 8058–8074, 2007.
Kanji, Z. A., Welti, A., Chou, C., Stetzer, O., and Lohmann, U.: Laboratory studies of immersion and deposition mode ice nucleation of ozone aged mineral dust particles, Atmos. Chem. Phys., 13, 9097–9118, https://doi.org/10.5194/acp-13-9097-2013, 2013.
Kanji, Z. A., Ladino, L. A., Wex, H., Boose, Y., Burkert-Kohn, M., Cziczo, D. J., and Krämer, M.: Overview of ice nucleating particles, Meteorol. Mon., 58, 1.1–1.33, 2017.
Kaufmann, L., Marcolli, C., Hofer, J., Pinti, V., Hoyle, C. R., and Peter, T.: Ice nucleation efficiency of natural dust samples in the immersion mode, Atmos. Chem. Phys., 16, 11177–11206, https://doi.org/10.5194/acp-16-11177-2016, 2016.
Khvorostyanov, V. I. and Curry, J. A.: The theory of ice nucleation by heterogeneous freezing of deliquescent mixed CCN. Part I: Critical radius, energy, and nucleation rate, J. Atmos. Sci., 61, 2676–2691, 2004.
Kiselev, A., Bachmann, F., Pedevilla, P., Cox, S. J., Michaelides, A., Gerthsen, D., and Leisner, T.: Active sites in heterogeneous ice nucleation – the example of K-rich feldspars, Science, 355, 367–371, 2017.
Koop, T. and Murray, B. J.: A physically constrained classical description of the homogeneous nucleation of ice in water, J. Chem. Phys., 145, 211915, https://doi.org/10.1063/1.4962355, 2016.
Koren, I., Yoram, J. K., Richard, W., Martin, C. T., Yinon, R., Martins, J. V., and Daniel, R.: The Bodélé depression: a single spot in the Sahara that provides most of the mineral dust to the Amazon forest, Environ. Res. Lett., 1, 014005, https://doi.org/10.1088/1748-9326/1/1/014005, 2006.
Krasnov, H., Katra, I., Koutrakis, P., and Friger, M. D.: Contribution of dust storms to PM10 levels in an urban arid environment, J. Air Waste Manage., 64, 89–94, https://doi.org/10.1080/10962247.2013.841599, 2014.
Krueger, B. J., Grassian, V. H., Cowin, J. P., and Laskin, A.: Heterogeneous chemistry of individual mineral dust particles from different dust source regions: the importance of particle mineralogy, Atmos. Environ., 38, 6253–6261, 2004.
Ladino, L. A., Raga, G. B., Alvarez-Ospina, H., Andino-Enríquez, M. A., Rosas, I., Martínez, L., Salinas, E., Miranda, J., Ramírez-Díaz, Z., Figueroa, B., Chou, C., Bertram, A. K., Quintana, E. T., Maldonado, L. A., García-Reynoso, A., Si, M., and Irish, V. E.: Ice-nucleating particles in a coastal tropical site, Atmos. Chem. Phys., 19, 6147–6165, https://doi.org/10.5194/acp-19-6147-2019, 2019.
Laskin, A., Wietsma, T. W., Krueger, B. J., and Grassian, V. H.: Heterogeneous chemistry of individual mineral dust particles with nitric acid: A combined CCSEM/EDX, ESEM, and ICP-MS study, J. Geophys. Res.-Atmos., 110, D10208, https://doi.org/10.1029/2004JD005206, 2005.
Levin, Z., Ganor, E., and Gladstein, V.: The effects of desert particles coated with sulfate on rain formation in the eastern Mediterranean, J. Appl. Meteorol., 35, 1511–1523, 1996.
Li, W. J. and Shao, L. Y.: Observation of nitrate coatings on atmospheric mineral dust particles, Atmos. Chem. Phys., 9, 1863–1871, https://doi.org/10.5194/acp-9-1863-2009, 2009.
Lohmann, U. and Feichter, J.: Global indirect aerosol effects: a review, Atmos. Chem. Phys., 5, 715–737, https://doi.org/10.5194/acp-5-715-2005, 2005.
Lüönd, F., Stetzer, O., Welti, A., and Lohmann, U.: Experimental study on the ice nucleation ability of size-selected kaolinite particles in the immersion mode, J. Geophys. Res.-Atmos., 115, D14201, https://doi.org/10.1029/2009JD012959, 2010.
Mahowald, N., Albani, S., Kok, J. F., Engelstaeder, S., Scanza, R., Ward, D. S., and Flanner, M. G.: The size distribution of desert dust aerosols and its impact on the Earth system, Aeolian Res., 15, 53–71, https://doi.org/10.1016/j.aeolia.2013.09.002, 2014.
Marcolli, C.: Deposition nucleation viewed as homogeneous or immersion freezing in pores and cavities, Atmos. Chem. Phys., 14, 2071–2104, https://doi.org/10.5194/acp-14-2071-2014, 2014.
Marcolli, C., Gedamke, S., Peter, T., and Zobrist, B.: Efficiency of immersion mode ice nucleation on surrogates of mineral dust, Atmos. Chem. Phys., 7, 5081–5091, https://doi.org/10.5194/acp-7-5081-2007, 2007.
Marple, V. A., Rubow, K. L., and Behm, S. M.: A Microorifice Uniform Deposit Impactor (MOUDI): Description, Calibration, and Use, Aerosol Sci. Tech., 14, 434–446, https://doi.org/10.1080/02786829108959504, 1991.
Mason, R. H., Chou, C., McCluskey, C. S., Levin, E. J. T., Schiller, C. L., Hill, T. C. J., Huffman, J. A., DeMott, P. J., and Bertram, A. K.: The micro-orifice uniform deposit impactor–droplet freezing technique (MOUDI-DFT) for measuring concentrations of ice nucleating particles as a function of size: improvements and initial validation, Atmos. Meas. Tech., 8, 2449–2462, https://doi.org/10.5194/amt-8-2449-2015, 2015.
Mason, R. H., Si, M., Chou, C., Irish, V. E., Dickie, R., Elizondo, P., Wong, R., Brintnell, M., Elsasser, M., Lassar, W. M., Pierce, K. M., Leaitch, W. R., MacDonald, A. M., Platt, A., Toom-Sauntry, D., Sarda-Estève, R., Schiller, C. L., Suski, K. J., Hill, T. C. J., Abbatt, J. P. D., Huffman, J. A., DeMott, P. J., and Bertram, A. K.: Size-resolved measurements of ice-nucleating particles at six locations in North America and one in Europe, Atmos. Chem. Phys., 16, 1637–1651, https://doi.org/10.5194/acp-16-1637-2016, 2016.
Mazar, Y., Cytryn, E., Erel, Y., and Rudich, Y.: Effect of dust storms on the atmospheric microbiome in the Eastern Mediterranean, Environ. Sci. Technol., 50, 4194–4202, 2016.
Middleton, N.: Desert dust hazards: A global review, Aeolian Res., 24, 53–63, 2017.
Middleton, N. J. and Goudie, A. S.: Saharan dust: sources and trajectories, T. I. Brit. Geogr., 26, 165–181, https://doi.org/10.1111/1475-5661.00013, 2001.
Murphy, D., Cziczo, D., Froyd, K., Hudson, P., Matthew, B., Middlebrook, A., Peltier, R., Sullivan, A., Thomson, D., and Weber, R.: Single-particle mass spectrometry of tropospheric aerosol particles, J. Geophys. Res.-Atmos., 111, D23S32, https://doi.org/10.1029/2006JD007340, 2006.
Murray, B. J., O'Sullivan, D., Atkinson, J. D., and Webb, M. E.: Ice nucleation by particles immersed in supercooled cloud droplets, Chem. Soc. Rev., 41, 6519–6554, https://doi.org/10.1039/C2CS35200A, 2012.
Niedermeier, D., Augustin-Bauditz, S., Hartmann, S., Wex, H., Ignatius, K., and Stratmann, F.: Can we define an asymptotic value for the ice active surface site density for heterogeneous ice nucleation?, J. Geophys. Res.-Atmos., 120, 5036–5046, 2015.
Niemand, M., Möhler, O., Vogel, B., Vogel, H., Hoose, C., Connolly, P., Klein, H., Bingemer, H., DeMott, P., Skrotzki, J., and Leisner, T.: A Particle-Surface-Area-Based Parameterization of Immersion Freezing on Desert Dust Particles, J. Atmos. Sci., 69, 3077–3092, https://doi.org/10.1175/jas-d-11-0249.1, 2012.
O'Sullivan, D., Murray, B. J., Ross, J. F., and Webb, M. E.: The adsorption of fungal ice-nucleating proteins on mineral dusts: a terrestrial reservoir of atmospheric ice-nucleating particles, Atmos. Chem. Phys., 16, 7879–7887, https://doi.org/10.5194/acp-16-7879-2016, 2016.
Perlwitz, J. P., Pérez García-Pando, C., and Miller, R. L.: Predicting the mineral composition of dust aerosols – Part 1: Representing key processes, Atmos. Chem. Phys., 15, 11593–11627, https://doi.org/10.5194/acp-15-11593-2015, 2015.
Pratt, K. A., DeMott, P. J., French, J. R., Wang, Z., Westphal, D. L., Heymsfield, A. J., Twohy, C. H., Prenni, A. J., and Prather, K. A.: In situ detection of biological particles in cloud ice-crystals, Nat. Geosci., 2, 398–401, https://doi.org/10.1038/ngeo521, 2009.
Price, H., Baustian, K., McQuaid, J., Blyth, A., Bower, K., Choularton, T., Cotton, R., Cui, Z., Field, P., and Gallagher, M.: Atmospheric Ice-Nucleating Particles in the Dusty Tropical Atlantic, J. Geophys. Res.-Atmos., 123, 2175–2193, 2018.
Prospero, J. M.: Long-range transport of mineral dust in the global atmosphere: Impact of African dust on the environment of the southeastern United States, P. Natl. Acad. Sci. USA, 96, 3396–3403, 1999.
Pruppacher, H. and Klett, J.: Microphysics of Clouds and Precipitation: With an Introduction to Cloud Chemistry and Cloud Electricity, 954 pp., Springer, New York, 1997.
Reicher, N., Segev, L., and Rudich, Y.: The WeIzmann Supercooled Droplets Observation on a Microarray (WISDOM) and application for ambient dust, Atmos. Meas. Tech., 11, 233–248, https://doi.org/10.5194/amt-11-233-2018, 2018.
Rogers, D. C., DeMott, P. J., Kreidenweis, S. M., and Chen, Y.: Measurements of ice nucleating aerosols during SUCCESS, Geophys. Res. Lett., 25, 1383–1386, 1998.
Rosenfeld, D. and Woodley, W. L.: Deep convective clouds with sustained supercooled liquid water down to −37.5 ∘C, Nature, 405, 440–442, 2000.
Rosenfeld, D., Rudich, Y., and Lahav, R.: Desert dust suppressing precipitation: A possible desertification feedback loop, P. Natl. Acad. Sci. USA, 98, 5975–5980, 2001.
Rosinski, J., Haagenson, P., Nagamoto, C., and Parungo, F.: Ice-forming nuclei of maritime origin, J. Aerosol Sci., 17, 23–46, 1986.
Ryder, C., Highwood, E., Lai, T., Sodemann, H., and Marsham, J.: Impact of atmospheric transport on the evolution of microphysical and optical properties of Saharan dust, Geophys. Res. Lett., 40, 2433–2438, 2013.
Ryder, C. L., Marenco, F., Brooke, J. K., Estelles, V., Cotton, R., Formenti, P., McQuaid, J. B., Price, H. C., Liu, D., Ausset, P., Rosenberg, P. D., Taylor, J. W., Choularton, T., Bower, K., Coe, H., Gallagher, M., Crosier, J., Lloyd, G., Highwood, E. J., and Murray, B. J.: Coarse-mode mineral dust size distributions, composition and optical properties from AER-D aircraft measurements over the tropical eastern Atlantic, Atmos. Chem. Phys., 18, 17225–17257, https://doi.org/10.5194/acp-18-17225-2018, 2018.
Sassen, K., DeMott, P. J., Prospero, J. M., and Poellot, M. R.: Saharan dust storms and indirect aerosol effects on clouds: CRYSTAL-FACE results, Geophys. Res. Lett., 30, 1633, https://doi.org/10.1029/2003GL017371, 2003.
Schepanski, K.: Transport of mineral dust and its impact on climate, Geosciences, 8, 151, https://doi.org/10.3390/geosciences8050151, 2018.
Schrod, J., Weber, D., Drücke, J., Keleshis, C., Pikridas, M., Ebert, M., Cvetković, B., Nickovic, S., Marinou, E., Baars, H., Ansmann, A., Vrekoussis, M., Mihalopoulos, N., Sciare, J., Curtius, J., and Bingemer, H. G.: Ice nucleating particles over the Eastern Mediterranean measured by unmanned aircraft systems, Atmos. Chem. Phys., 17, 4817–4835, https://doi.org/10.5194/acp-17-4817-2017, 2017.
Spichtinger, P. and Cziczo, D. J.: Impact of heterogeneous ice nuclei on homogeneous freezing events in cirrus clouds, J. Geophys. Res.-Atmos., 115, D14208, https://doi.org/10.1029/2009JD012168, 2010.
Sprenger, M. and Wernli, H.: The LAGRANTO Lagrangian analysis tool – version 2.0, Geosci. Model Dev., 8, 2569–2586, https://doi.org/10.5194/gmd-8-2569-2015, 2015.
Tan, I., Storelvmo, T., and Zelinka, M. D.: Observational constraints on mixed-phase clouds imply higher climate sensitivity, Science, 352, 224–227, https://doi.org/10.1126/science.aad5300, 2016.
Tegen, I. and Fung, I.: Modeling of mineral dust in the atmosphere: Sources, transport, and optical thickness, J. Geophys. Res.-Atmos., 99, 22897–22914, https://doi.org/10.1029/94JD01928, 1994.
Tunega, D., Gerzabek, M. H., and Lischka, H.: Ab initio molecular dynamics study of a monomolecular water layer on octahedral and tetrahedral kaolinite surfaces, J. Phys. Chem. B, 108, 5930–5936, 2004.
Twohy, C. H. and Poellot, M. R.: Chemical characteristics of ice residual nuclei in anvil cirrus clouds: evidence for homogeneous and heterogeneous ice formation, Atmos. Chem. Phys., 5, 2289–2297, https://doi.org/10.5194/acp-5-2289-2005, 2005.
Ullrich, R., Hoose, C., Möhler, O., Niemand, M., Wagner, R., Höhler, K., Hiranuma, N., Saathoff, H., and Leisner, T.: A New Ice Nucleation Active Site Parameterization for Desert Dust and Soot, J. Atmos. Sci., 74, 699–717, https://doi.org/10.1175/jas-d-16-0074.1, 2017.
Vali, G.: Sizes of atmospheric ice nuclei, Nature, 212, 384–385, https://doi.org/10.1038/212384a0, 1966.
Vali, G.: Quantitative Evaluation of Experimental Results an the Heterogeneous Freezing Nucleation of Supercooled Liquids, J. Atmos. Sci., 28, 402–409, https://doi.org/10.1175/1520-0469(1971)028<0402:qeoera>2.0.co;2, 1971.
Welti, A., Lohmann, U., and Kanji, Z. A.: Ice nucleation properties of K-feldspar polymorphs and plagioclase feldspars, Atmos. Chem. Phys. Discuss., https://doi.org/10.5194/acp-2018-1271, in review, 2019.
Zielke, S. A., Bertram, A. K., and Patey, G.: Simulations of ice nucleation by kaolinite (001) with rigid and flexible surfaces, J. Phys. Chem. B, 120, 1726–1734, 2015.
Zimmermann, F., Weinbruch, S., Schütz, L., Hofmann, H., Ebert, M., Kandler, K., and Worringen, A.: Ice nucleation properties of the most abundant mineral dust phases, J. Geophys. Res.-Atmos., 113, D23204, https://doi.org/10.1029/2008JD010655, 2008.