the Creative Commons Attribution 4.0 License.
the Creative Commons Attribution 4.0 License.
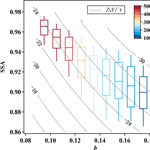
Aerosol optical properties at SORPES in Nanjing, east China
Yicheng Shen
Jiaping Wang
Xuguang Chi
Ximeng Qi
Xin Huang
Qiang Liu
Longfei Zheng
Zheng Xu
Tuukka Petäjä
Pasi P. Aalto
Congbin Fu
Markku Kulmala
Aerosol optical properties (AOPs) and supporting parameters – particle number size distributions, PM2.5 mass concentrations, and the concentrations of trace gases (NOx and NOy) – were measured at SORPES, a regional background station in Nanjing, China from June 2013 to May 2015. The aerosol was highly scattering: the average scattering coefficient was σsp=403 ± 314 Mm−1, the absorption coefficient σap=26 ± 19 Mm−1, and the single-scattering albedo SSA = 0.93 ± 0.03 for green light. The SSA in Nanjing appears to be slightly higher than published values from several other sites in China and elsewhere. The average Ångström exponent of absorption (AAE) for the wavelength range 370–950 nm was 1.04 and the AAE range was 0.7–1.4. These AAE values can be explained with different amounts of non-absorbing coating on pure black carbon (BC) cores and different core sizes rather than contribution by brown carbon. The AOPs had typical seasonal cycles with high σsp and σap in winter and low ones in summer: the averages were σsp=544 ± 422 and σap=36 ± 24 Mm−1 in winter and σsp=342 ± 281 and σap=20 ± 13 Mm−1 in summer. The intensive AOPs had no clear seasonal cycles, the variations in them were rather related to the evolution of pollution episodes. The diurnal cycles of the intensive AOPs were clear and in agreement with the cycle of the particle number size distribution. The diurnal cycle of SSA was similar to that of the air photochemical age, suggesting that the darkest aerosol originated from fresh traffic emissions. A Lagrangian retroplume analysis showed that the potential source areas of high σsp and σap are mainly in eastern China. Synoptic weather phenomena dominated the cycle of AOPs on a temporal scale of 3–7 days. During pollution episodes, modeled boundary layer height decreased, whereas PM2.5 concentrations and σsp and σap typically increased gradually and remained high during several days but decreased faster, sometimes by even more than an order of magnitude within some hours. During the growth phase of the pollution episodes the intensive AOPs evolved clearly. The mass scattering efficiency MSE of PM2.5 grew during the extended pollution episodes from ∼ 4 to ∼ 6 m2 g−1 and the mass fraction of BCe decreased from ∼ 10 to ∼ 3 % during the growth phase of the episodes. Particle growth resulted in the backscatter fraction decreasing from more than 0.16 to less than 0.10, SSA growing from less than 0.9 to more than 0.95, and radiative forcing efficiency (RFE) changing from less than −26 W m−2 to more than −24 W m−2, which means that the magnitude of RFE decreased. The RFE probability distribution at SORPES was clearly narrower than at a clean background site which is in agreement with a published RFE climatology.
- Article
(5367 KB) - Full-text XML
-
Supplement
(2533 KB) - BibTeX
- EndNote
Atmospheric aerosols alter the radiation budget of the earth system directly by scattering and absorbing solar radiation and indirectly by acting as cloud condensation nuclei (CCN), thus affecting cloud formation, cloud optical properties, and cloud lifetime (IPCC, 2013). Radiation forcing of aerosol exerts significant impacts on the climate system and contributes the largest uncertainty in the assessment of climate change both regionally and globally (IPCC, 2013). Such uncertainty is not only due to highly inhomogeneous spatial distributions and temporal variations in aerosol but also due to limited measurements of aerosol chemical composition and size distributions which aerosol optical properties (AOPs) depend on. Black carbon (BC) aerosols are especially important due to their strong capability of light absorption. It has been estimated that the radiative and climate impacts of BC is the second strongest contributor to global warming (Ramanathan et al., 2007) and can also influence rainfall, large-scale circulation, and hydrological cycles (Menon et al., 2002). Recent studies also indicate that the absorbing aerosols heat the air and change the vertical temperature profile, and thus have an influence on the planetary boundary layer (PBL) structure (e.g., Ding et al., 2013a, 2016a; Wilcox et al., 2017; Wang et al., 2018). Light scattering aerosol over polluted continental areas mainly consist of secondary aerosols produced by heterogeneous reactions, which greatly increase the aerosol scattering coefficient and play an important role in haze events in China (Huang et al., 2014; Wang et al., 2018).
During recent decades, many comprehensive studies of aerosol optical properties have been conducted in China, especially in the three large urban agglomerations of the North China Plain (NCP), the Pearl River Delta (PRD), and the Yangtze River Delta (YRD) regions (e.g., Kim et al., 2004; Andreae et al., 2008; Yan et al., 2008; Cheng et al., 2008; Yang et al., 2009; Ma et al., 2011; Zhuang et al., 2014, 2016; Wang et al., 2017). These three regions are highly industrialized with an extremely high population density. Due to the complex anthropogenic emissions and consequent chemical reactions, these three regions not only have some of the highest aerosol loadings worldwide but also some of the greatest uncertainties in radiative forcing of aerosols. The fast urbanization and industrialization process along with new technology and policy make the emission characteristics change year by year.
To reduce the uncertainties in aerosol climatic effects, long-term continuous measurements of aerosol optical properties, particle size distributions, and other relevant parameters including trace gas concentrations and meteorological parameters have been conducted at the Station for Observing Regional Processes of the Earth System (SORPES, in operation since 2011), a regional background station in the YRD (Ding et al, 2013b, 2016b). SORPES is located in the northwestern part of the YRD area and to the northeast of the Nanjing urban area. The complex monsoon and synoptic weather together with the surrounding complex emission sources may have important impacts on aerosols in this region (Ding et al., 2013b). On the other hand, the AOPs influence radiative transfer and further modify meteorological processes, such as the PBL and weather (Ding et al., 2013a; Wang et al., 2018). Ding et al. (2016a) and Petäjä et al. (2016) studied the interactions of pollutants and the PBL and found that high PM and especially BC concentrations enhance the stability of a polluted boundary layer by modifying the PBL temperature profile and by decreasing the surface heat flux. In these papers, a few typical cases were discussed; however, there is a lack of analysis on the long-term data and no detailed analysis of how particle size distributions and AOPs evolve during extreme haze pollution episodes. Part of the AOP data measured at SORPES were earlier used for a technical analysis of the interpretation of absorption photometer data (Virkkula et al., 2015). In this work, we will present 2 years of AOP observations at the SORPES station and a comprehensive analysis of their temporal variations and relationships with particle size distributions and transport.
2.1 Site description
The measurements were conducted at the SORPES station (32∘07′14′ N, 118∘57′10′ E; ∼ 40 m a.s.l.) from July 2013 to May 2015. The station is located on the top of a small hill, ∼ 30 m a.g.l., inside Nanjing University Xianlin Campus, 20 km northeast of downtown Nanjing (Ding et al., 2013b, 2016b; Xie et al., 2015). SORPES is surrounded by several campuses and residential areas and there is no industry within 3 km from the station. A new highway ∼ 1 km to the west of SORPES was opened to traffic in 2014. The prevailing easterly wind, i.e., northeast in winter and southeast in summer (Ding et al., 2013b), minimizes the effect from downtown Nanjing and from the highway. With some consideration on the data analysis, the site can still be considered as a regional background station with little local influence (Ding et al., 2016b).
2.2 Measurements
2.2.1 Aerosol optical measurements
The scattering and backscattering coefficients (σsp and σbsp, respectively) were measured at three wavelengths (λ=450, 525, and 635 nm) using an integrating nephelometer (Aurora 3000, Ecotech). The sample air was taken through a 2 m stainless steel tube, the top of which is 1 m above the roof. The inlet has a rain cap and an external heater to prevent condensation. The zero of the nephelometer was checked by filtered air every day and the span by Tetrafluoroethane (R134a) every 2 weeks. The monthly average relative humidity (RH) at SORPES varies from 65 to 80 % (Ding et al., 2013b) and the aerosol hygroscopic growth is usually significant when RH increases above 50 % (Baltensberger et al., 2003; Zhang et al. 2015; WMO, 2016). We use an internal heater to reduce the RH of the sample air below 50 % for most of the time. Since the internal heater of the nephelometer often malfunctioned, ∼ 25–30 % of data suffered from high RH. The respective data were corrected as will be discussed below. Light absorption was measured using a 7-wavelength Aethalometer (AE-31, Magee Scientific) at λ=370, 470, 520, 590, 660, 880, and 950 nm. The Aethalometer is a filter-based instrument that measures light attenuation from which light absorption can be calculated. The detailed calculation will be discussed below. The Aethalometer shares the same PM2.5 cyclone inlet with several trace gas analyzers. The sample air is taken through a stainless steel tube to the instruments. The flow rate for the Aethalometer was set to 5.0 L min−1 for the whole period. An internal flowmeter records the real-time flow rate continuously, and flow checks were conducted twice a year using a bubble flowmeter (Gilibrator system, Gilian). The time resolution of the Aethalometer data was set to 5 min and it was set to change the sampling spot when the maximum attenuation was 125. These settings were used for the whole period.
2.2.2 Supporting measurements
Supporting measurements were conducted for the same period (Ding et al., 2013b, 2016b). Particle number size distributions were measured using a custom-made differential mobility particle sizer (DMPS) in the size range of 6–800 nm (mobility diameter) and an aerodynamic particle sizer (APS, TSI model 3321) in the size range of 0.52–20 µm (aerodynamic diameter). More details can be seen in Qi et al. (2015). The number size distributions were used here for modeling scattering coefficients, for calculating effective diameters, and for estimating particle mass concentrations as will be discussed below.
Mass concentrations of particles smaller than 2.5 µm (PM2.5) were measured with an online analyzer based on the light scattering and beta ray absorption (Thermo Scientific, 5030 SHARP, USA). The trace gas measurements (NOx and NOy) used in this work were conducted with a NO-NO2-NOx Analyzer (model 42i, Thermo Scientific, USA) and a NO-DIF-NOy Analyzer (model 42i-Y, Thermo Scientific, USA). These data were used for estimating the photochemical age of air masses. More details for PM2.5, trace gases, water soluble ions, and meteorological parameters can be found in Ding et al. (2013b, 2016b) and Xie et al. (2015).
2.3 Data processing
2.3.1 Corrections for scattering coefficient
First the truncation error of the scattering measurements was corrected according to Müller et al. (2011). In addition, since 28.6 % of total data were measured when the sample air relative humidity RHsample>50 %, due to an intermittent fault of the internal heater of the nephelometer, the scattering coefficients were corrected for hygroscopic growth in order to maximize the data availability when the internal heater of the nephelometer was malfunctioning. Hygroscopic aerosols take up water as humidity increases thus increasing σsp. The impact of relative humidity on σsp is defined as the scattering enhancement factor f (RH, λ):
where σsp (dry, λ) and σsp (RH, λ) represent scattering coefficients at wavelength λ in dry and humid conditions, respectively. We used the parameterization with the equation
in this study. The constants c and g for total scattering (backscattering) coefficient were set to 0.72(0.87) and 0.65(0.34), respectively, according to Carrico et al. (2003) who derived them from measurements during ACE-Asia, a well-recognized aerosol experiment in Asia. Here we choose the value for “polluted” condition in their study. All total scattering coefficients and backscattering coefficients measured with sample relative humidity RHsample>50 % were corrected according to Eq. (2) to RH = 50 %. By considering that the RH sensor inside the nephelometer may not be as accurate as the one at the meteorological station, we recalculated the sample RH using the Clausius–Clapeyron equation. It was assumed that the absolute humidity of the sample air and at the RH sensor 20 m away from the inlet are the same. The pressure and temperature of the nephelometer were used for correcting the scattering coefficients to standard temperature and pressure (STP) conditions (T=273.15 K, p=1013 hPa). All aerosol optical properties discussed in this paper use STP condition unless otherwise specified. A brief comparison between non-corrected scattering coefficients and data corrected with the two parameterizations is presented in the Supplement Sect. S1.
2.3.2 Absorption coefficient
The Aethalometer does not measure absorption coefficient directly, instead it measures the light attenuation (ATN) of aerosol-loaded spots on quartz filters. The attenuation coefficient σatn at wavelength λ is calculated from
where Q is the flow rate, A is the spot size, and ΔATN is the change of attenuation during the time step Δt. The Aethalometer firmware converts σatn to equivalent black carbon (BCe; Petzold et al., 2013) mass concentration by dividing it with a wavelength-dependent mass attenuation coefficient of 14 625 m2 g−1λ(nm)−1. However, it is not as straightforward to calculate the absorption coefficient σap. Several algorithms for calculating σap from Aethalometer data have been presented, e.g., Weingartner et al. (2003), Arnott et al. (2005), Schmid et al. (2006), Virkkula et al. (2007), and Collaud Coen et al. (2010). In principle they can all be presented in the form of
or
where f and R are functions that correct for the loading, s and s′ are the fraction of scattering coefficient that results in a change of ATN and would be interpreted as absorption and BCe if not taken into account, and Cref is the multiple-scattering correction factor. There are no unambiguous forms for f, R, s, s′, and Cref. A recent analysis suggested that f is influenced by the aerosol backscatter fraction (Virkkula et al., 2015). Arnott et al. (2005) and Schmid et al. (2006) suggest that Cref is wavelength-dependent, whereas Collaud Coen et al. (2010) used a non-wavelength-dependent Cref. In this study, we used the Collaud Coen et al. (2010) algorithm with Cref=4.26, the value obtained from measurements in a flat region near populated and industrialized areas at Cabauw, the Netherlands (Collaud Coen et al., 2010).
2.3.3 Intensive aerosol optical properties
Light absorption and scattering depend on wavelength λ approximately as λ−AAE and λ−SAE, where AAE and SAE are the Ångström exponents of absorption and scattering, respectively. SAE can be calculated from σsp measured at two wavelengths λ1 and λ2 from
For multiple wavelengths, SAE can also be calculated by taking the logarithm of scattering coefficients and the respective wavelengths and SAE is the slope obtained from their linear regression as was done by Virkkula et al. (2011). SAE is typically considered to be associated with the dominating particle size so that large values (SAE > 2) indicate a large contribution of small particles and small values (SAE < 1) a large contribution of large particles. However, this relationship is not quite unambiguous, as discussed by, for example, Schuster et al. (2006) and Virkkula et al. (2011). In this study, unless otherwise specified, we use σsp at λ=635 and 450 nm to calculate SAE; For the tables, other wavelength pairs were also used. To calculate absorption coefficient according to Eg. (4), σsp measured at the nephelometer wavelengths were interpolated and extrapolated to the Aethalometer wavelengths according to (λ1/λx)SAE .
AAE can be calculated from Eq. (6) by using σap instead of σsp. AAE is an indicator of the dominant light absorber so that values around 1 indicate absorption by BC (e.g., Bond and Bergstrom, 2006; Bond et al., 2013) and clearly larger values by other absorbers. For example, light absorbing organics may yield AAE in the range 3–7 (e.g., Kirchstetter and Thatcher, 2012). The interpretation is ambiguous since AAE not only depends on the dominant absorber but also on the size and internal structure of the particles. For instance, for pure BC particles, AAE variation may have AAE < 1 and BC particles coated with non-absorbing material may have AAE in the range from < 1 to almost 2 (e.g., Gyawali et al., 2009; Lack and Cappa, 2010). In this study, unless otherwise specified, we use σap at λ=370 and 950 nm to estimate AAE; For the tables, other wavelength pairs were also used.
The ratio of scattering to extinction is the single scattering albedo (SSA)
It is a measure of the darkness of aerosols. At low SSA aerosols heat the atmosphere and at high values they cool it, depending also on other parameters (e.g., Haywood and Shine, 1995). SSA is ∼ 0.3 for pure BC particles (e.g., Schnaiter et al., 2003) and 1 for purely scattering aerosols. SSA was calculated for the Aethalometer wavelengths. The backscatter fraction,
was calculated at λ=450, 525, and 635 nm. It is a measure related to the angular distribution of light scattered by aerosol particles. For very small particles, b approaches the value 0.5 (e.g., Wiscombe and Grams, 1976; Horvath et al., 2016) and decreases with increasing particle size. From b, it is possible to estimate the average up-scatter fraction β, one of the properties controlling the aerosol direct radiative forcing (e.g., Andrews et al., 2006). The larger b is, the more aerosols scatter light to space and cool the atmosphere or heat it less if the aerosol is so dark that it heats the atmosphere as shown in the formula for the top of the atmosphere aerosol radiative forcing efficiency (RFE = ΔF∕τ), i.e., aerosol forcing per unit optical depth (τ):
where D is the fractional day length, So is the solar constant, Tat is the atmospheric transmission, Ac is the fractional cloud amount, Rs is the surface reflectance, and β is the average up-scatter fraction calculated from b. If the non-aerosol-related factors are kept constant and if it is assumed that β has no zenith angle dependence, this formula can be used for assessing the intrinsic radiative forcing efficiency by aerosols (e.g., Sheridan and Ogren, 1999; Delene and Ogren, 2002). The constants used were D=0.5, So=1370 W m−2, Tat=0.76, Ac=0.6, and Rs=0.15 as suggested by Haywood and Shine (1995), and β was calculated from β = 0.0817 + 1.8495b−2.9682b2 (Delene and Ogren, 2002).
2.3.4 Properties calculated from the particle number size distributions
The size distributions measured with the DMPS were used to calculate three weighted mean diameters, the geometric mean diameter (GMD),
the surface mean diameter (SMD),
and volume mean diameter (VMD),
where i is number of size bin, Dp,i is mobility diameter, and Ni is number concentration. The mass concentration of particles smaller than 0.8 µm was calculated from , where the density of particles ρp was assumed to be 1.7 g cm−3. The DMPS measures the mobility diameter which for spherical particles equals the physical diameter Dp. The aerodynamic diameter of spherical particles with density ρp is , where ρ0 is the density of water. For Dp=0.8 µm and ρp=1.7 g cm−3, this yields Da=1.0 µm. In the results, therefore, the mass concentration calculated from the number size distributions will be denoted as PM1. The reasoning for the use of a density of 1.7 g cm−3 was presented by Wang et al. (2017). The PM1 concentrations were calculated by using the density of 1.7 g cm−3 even though it was not determined with any physical measurements so an explanation is needed. The density of the major inorganic aerosol compounds ammonium sulfate and ammonium nitrate are 1.76 and 1.725 g cm−3, respectively (e.g., Tang, 1996). The density of sulfuric-acid-coated soot has been estimated to be 1.7 g cm−3 (Zhang et al., 2008). Ambient aerosol particles also contain many unknown compounds such as organics and also some water even at RH < 50 %. Densities of real atmospheric aerosols have been published from several regions. Just to name some, the mean apparent particle density of 1.6 ± 0.5 g cm−3 was determined for urban aerosol by Pitz et al. (2003) and at a boreal forest site the average density was 1.66 ± 0.13 g cm−3 (Saarikoski et al., 2005). Based on the above, it is reasonable to use the density of 1.7 g cm−3 for the estimation of aerosol mass concentration from the number size distributions. It is clear, however, that this value is uncertain and also that in reality it is not constant as the chemical composition of aerosols varies.
The DMPS measurements were continuous throughout the whole study period, while the APS for a few months only. We estimated the contribution of particles smaller than 800 nm to scattering by using the DMPS and APS data measured during one month in July–August 2014. Scattering coefficients were calculated from
where Qsp is the scattering efficiency that depends on particle size (Dp), wavelength (λ) and refractive index (m) of the particles. The results showed that the contribution of particles in the DMPS size range was ∼ 91 % of the whole integrated σsp. Since the APS data were short we did not model scattering for the whole period, but only discuss the weighted mean diameters from DMPS data.
2.4 Use of the trace gas data
The NOx and NOy concentrations can be used as a semi-quantitative indicator of the age of air masses, i.e., oxidation time passed since the emission of NOx from its sources, mainly traffic. NOx is the sum of NO and NO2 and NOy the sum of NOx and its oxidation products (e.g., HNO3; peroxyacetyl nitrate, PAN; and organic nitrates). The photochemical age, denoted as PA below, can be described using the ratio between NOx and NOy (Olszyna et al., 1994; Kleinman et al., 2008) and it is usually calculated as the negative logarithm of this ratio, i.e., PA = −log(NOx / NOy). If NOx = NOy then −log(NOx / NOy) = 0 and higher when NOx has had time to be oxidized. The −log(NOx / NOy) is close to 0 for the freshest traffic emission, a subsequent increase from 0 to 1 (1 indicates very aged) takes around 1 day without mixing any fresh emission (Kleinman et al., 2008). A value of 1 is usually considered as aged and the measured value seldom exists with this value. Since −log(NOx / NOy) is on a lognormal scale, such photochemical age is very sensitive to the fraction of fresh emission.
2.5 Modeling
To estimate the source regions of air masses, backward Lagrangian particle dispersion modeling (LPDM) was conducted by using the Hybrid Single-Particle Lagrangian Integrated Trajectory (HYSPLIT) model developed in the Air Resource Laboratory of the National Oceanic and Atmospheric Administration (Draxler and Hess, 1998; Stein et al., 2015). Thus 48 h back-trajectories and 72 h backward retroplumes (i.e., surface residence time of backward simulation for particles released at a specific location) starting from the altitude of 100 m above SORPES were calculated using HYSPLIT with GDAS meterological data based on a method developed and evaluated by Ding et al. (2013c). In this study, a retroplume (the unit is m−3 h) refers to the concentration (in m−3) averaged between 0 and 100 m and integrated for 1 h. In each run, HYSPLIT was set to model the release of 3000 particles each hour at SORPES, and track the backward trajectory of each particle. The “concentration” indicates the location of all those particles at a given moment. The “retroplume” as an integrated concentration, however, also gives information on the residence time of the particles within a certain grid cell (Ding et al., 2013c). The 48 h back-trajectories were also clustered by using HYSPLIT. Description of the clustering method is found at the NOAA Air Resources Laboratory (ARL) web page and will not be described here.
The planetary boundary layer height (PBLH) was calculated by WRF modeling (Weather Research and Forecasting) using the Yonsei University (YSU) PBL scheme. The PBL top is determined using a critical bulk Richardson number of zero, so it is effectively dependent on the buoyancy profile, and the PBL top is defined at the maximum entrainment layer (Hong et al., 2006).
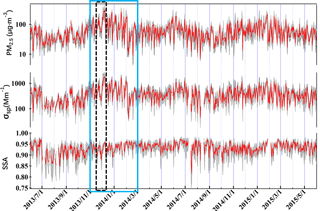
Figure 1Daily averaged PM2.5 concentrations, scattering coefficients (σsp, λ=525 nm), absorption coefficients σap, λ=520 nm), and single-scattering albedo (SSA, λ = 520 nm) at SORPES in June 2013–May 2015. The grey shaded areas show the 10th to 90th percentile range of hourly-averaged data. The blue box shows the period discussed in Sect. 3.5 (and plotted in the Supplement, Fig. S11), the black box shows the period of Fig. 9.
3.1 Overview of aerosol optical properties
The daily average and range of mass concentration of particles with an aerodynamic diameter of less than or equal to 2.5 µm (PM2.5), scattering coefficients (σsp), and SSA of green light during the whole period discussed in the paper are presented in Fig. 1. The extensive aerosol properties (PM2.5, σsp) measured with two independent instruments, the mass monitor and the nephelometer, followed each other. They often varied by almost an order of magnitude between consecutive days. A “pollution episode” is here defined as a time period that meets the following criteria: (1) lasting for 1–2 synoptic periods, i.e., 3–14 days; (2) PM2.5 concentrations both at the start and at the end of the period are significantly lower than the maxima during the episode; and (3) only one sharp/flat peak during the whole period. There were approximately 2–6 clear pollution episodes a month. In these episodes, PM2.5 concentrations and scattering and absorption coefficients typically increased and remained high during several days but decreased faster and remained at the lower level for a shorter period than at the higher level. This will be discussed more below.
The contribution of scattering to total extinction ( was high enough to keep SSA > 0.9 most of the time. Daily averages with very high SSA > 0.95 were observed throughout the year but especially in summer 2014. On the other hand, in summer 2013 there were several days with SSA < 0.9. A careful comparison of the time series shown in Fig. 1 shows that generally SSA was the highest at the peak PM2.5 concentration. It will be shown below that this is typically associated with particle growth.
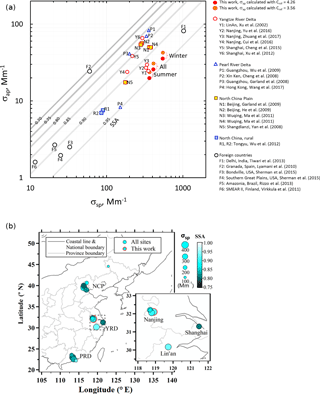
Figure 2 (a) Average absorption coefficient (σap) vs. average scattering coefficient (σsp) of green light at SORPES (this work) and selected Chinese and foreign sites. For SORPES, the averages for the whole period (Table 1) and for summer and winter (Table 3) are presented with two symbols: the solid red circles and the circles with the red cross for which σap was calculated by using Cref=4.26 and 3.56, respectively. For details of measurement methods, periods, wavelengths, and references of the comparison sites see Table 2. Constant single-scattering albedo (SSA) is depicted by the solid grey lines. (b) the locations of some of the studies mentioned in Table 2, the size and color of each cycle represent the scattering coefficient and single-scattering albedo, respectively, the circle with a red margin is the SORPES site.
Table 1Statistical summary of aerosol optical properties and associated supporting parameters measured at SORPES, Nanjing, in July 2013–May 2015. N∕Ntot: fraction of hours with valid observations, σap: absorption coefficient, σsp: scattering coefficient, SSA: single-scattering albedo, AAE: Ångström exponent of absorption, SAE: Ångström exponent of scattering, b: backscatter fraction, ΔF∕τ: radiative forcing efficiency, PM2.5: mass concentration of particles smaller than 2.5 µm aerodynamic diameter, GMD: geometric mean diameter, and VMD: volume mean diameter.
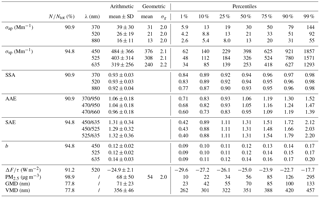
Table 1 shows a statistical overview of the aerosol optical properties and the related supporting parameters, PM2.5 concentrations, GMD, and VMD during the period discussed in this paper. The overall data coverage for absorption and scattering coefficients are 91.2 and 95.0 %, respectively. Since absorption coefficients can only be derived when both the nephelometer and Aethalometer are working properly, the data coverage for absorption coefficient (91.2 %) is also the fraction of time when all aerosol optical properties have valid values. The average ± standard deviation of σsp and σap were 403 ± 322 and 26 ± 19 Mm−1 at λ=525 nm and λ=520 nm, respectively. The geometric mean (and geometric standard deviation) of σsp and σap were 308(2.1) and 21 Mm−1(2.0). These two are plotted against each other in Fig. 2 together with respective published values observed at selected Chinese and foreign sites presented in Table 2. The results from some foreign sites are included in Fig. 2 to put the SORPES data into a more global context. At most sites, σsp and σap were neither measured with similar instruments nor at the same wavelengths as at SORPES, which complicates the comparison. To make the results as comparable as possible, only green wavelength results are shown. The σap averages were interpolated to the same wavelength at which the green σsp was measured at the same site. In this interpolation, it was assumed that AAE = 1 which undoubtedly increases the uncertainty in the comparison.
Table 2Published scattering coefficients (σsp), absorption coefficients (σap), and single-scattering albedos (SSA) at selected Chinese and foreign sites. The numbers in parentheses show the wavelength of the respective parameter in nm.
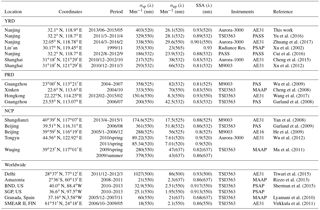
The overall average σsp and σap measured at SORPES were comparable to those at Lin'an in November 1999 and at Pukou (in Nanjing) from 1 March to 30 April 2011. These two sites are both in the YRD area. In the center of Nanjing, the annual averages were very close to those at SORPES, even though σap was slightly higher and σsp lower in the center of the city, respectively, which can be explained by the proximity to fresh traffic emissions. Another difference is that Zhuang et al. (2017) used the Cref=3.56 at λ=520 nm for calculating σap from Aethalometer data, whereas the value Cref=4.26 was used for all wavelengths in the present study. For comparison, the average σap at SORPES was also calculated by using the same Cref=3.56 at λ=520 nm and plotted against σsp during the above-mentioned periods in Fig. 2. When the same Cref is used for processing both sites' data, the average σap appears to be slightly higher at SORPES than in the center of the city.
The scatter plot also reveals some noteworthy differences between the sites. In the coastal city of Shanghai, σsp was lower and σap was higher than at the inland sites of the YRD (Lin'an and Nanjing) in any of the published results. One possible reason for the difference is that when wind blows from the NE (the prevailing wind direction in the YRD) there is less time for the formation and condensation of scattering material on fresh BC particles in Shanghai than at the inland sites. The scattering coefficient at the YRD inland sites is also comparable with published values observed in Beijing and Wuqing, a site between Beijing and Tianjin in the NCP, and in Guangzhou in the PRD region in China. On the other hand, σap is lower at the YRD inland sites than at the NCP and PRD sites, which together with high σsp leads to higher SSA than at the other polluted sites. This suggests that there may be differences in the local and regional emissions.
Shangdianzi is a global atmosphere watch (GAW) regional station 100 km northeast of Beijing (Yan et al., 2008). Tongyu is a regional background station located in the semi-arid area of northeastern China about 750 km even further NE of Beijing (Wu et al., 2012). Both Shangdianzi and Tongyu are partially influenced by highly polluted air from SW and cleaner air from remote areas so the average σap and σsp levels are clearly lower than in Beijing or YRD. But at both sites the average SSA is slightly lower than at SORPES. The Hong Kong measurements were conducted at the Hok Tsui monitoring station on the southeast tip of Hong Kong Island facing the South China Sea. Even though it is not far from the city, its aerosols are dominated by sea-salt particles which explains the high SSA (Wang et al., 2017).
In Delhi, India, σap was the highest, suggesting high BC emissions. However, there σsp was also so high that the SSA was in the same range as at the YRD inland sites. Grenada, an urban site in Spain, is an obvious outlier in the comparison, there the aerosol is the darkest, σap is at the same level as in Nanjing but σsp clearly lower. Lyamani et al. (2008) suggested that part of the explanation of low SSA in Grenada is the transport of light-absorbing Saharan dust in Grenada. The lowest σap and σsp in this comparison were at two continental background sites in the USA, the Amazonian site in Brazil, and at the boreal forest site of SMEAR II in Finland. Note that there, SSA was also slightly lower than at the YRD inland sites.
The overall SAE average ± standard deviation for the wavelength pair 450/635 nm was 1.31 ± 0.34 (Table 1), which is very close to the respective value of 1.32 ± 0.41 in the center of Nanjing (Zhuang et al., 2017). The overall average backscatter fraction b=0.12 ± 0.02 at λ=525 nm (Table 1) is also very similar to that at several sites around the world (e.g., Delene and Ogren, 2002; Andrews et al., 2011; Virkkula et al., 2011).
The average AAE for the wavelength pair 370/950 nm was 1.06 ± 0.18 and 98 % of the values varied in the range of about 0.7–1.5. Using the wavelength pairs 370/880 nm and 470/660 nm yielded slightly different AAE distributions (Table 1). Note, however, that these values were calculated with the algorithm of Collaud Coen (2010) with a non-wavelength-dependent Cref. Algorithms that assume wavelength-dependent Cref (Arnott et al., 2005; Schmid et al., 2006) would yield higher AAE. For instance, Zhuang et al. (2017) used the latter algorithm and reported AAE = 1.58 ± 0.23 for the measurements in the center of Nanjing. This is a significantly higher value than in the present work so the difference needs some discussion.
It is simple to show that if the Cref depends on wavelength as /λ0)α then AAE(Cref,λ) = AAE(Cref,0) + α, where AAE(Cref,0) is the AAE calculated from σap that were calculated from Eq. (4) using a constant Cref = Cref,0. The Cref of Arnott et al. (2005) has a wavelength dependency of /520 nm)0.181 which leads to AAE values of AAE(AAE(Cref,0) + 0.181 so that the average AAE would be 1.24 instead of 1.06 shown in Table 1. The above reasoning is an approximation only because the scattering corrections in Eq. (4) were not taken into account. These corrections also affect the AAE but an evaluation of them is out of the scope of the present paper. However, the AAE uncertainty further affects estimates of the sources of BC. The AAE has recently been used for estimating the contributions of burning fossil fuel and wood to BC. Zotter et al. (2017) used Aethalometer data with a constant Cref and found that the AAE calculated from the wavelength pair 370 and 950 nm (AAE(370/950)) was ∼ 0.9 and ∼ 2.09 for BC from traffic and wood burning emissions, respectively, by analyzing data collected in Switzerland. For the wavelength pair 470/950, the respective AAEs were ∼ 0.9 and ∼ 1.68 for BC from traffic and wood burning. By using these values and the average value of AAE(370/950) = 1.06, the model by Zotter et al. (2017) yields an estimate of ∼ 8 % of BC originating from wood burning and 92 % from traffic. By using the wavelength pair 470/950, the average AAE of 1.04 with the same model yields also ∼ 8 % contribution by wood burning. If a wavelength-dependent Cref were used that yields AAE values larger by 0.18, as discussed above, the average AAE(370/950) would be ∼ 1.24 and the fraction of BC from wood burning ∼ 18 %. Zhuang et al. (2017) used a wavelength-dependent Cref for analyzing Aethalometer data collected in the center of Nanjing and obtained the average AAE = 1.58 for the wavelength pair 470 and 660 nm. By using the same values and model this AAE would yield an estimated contribution of ∼ 43 % by wood burning. The difference of these results suggests that further, harmonized studies should be conducted.
However, it should not be forgotten that aerosol optics creates a further source of uncertainty in the interpretation of AAE. The AAE range 0.7–1.5 is also explainable with different amounts of non-absorbing coating on pure BC cores and different core sizes (Gyawali et al., 2009; Lack and Cappa, 2010). If this is the explanation the data does not suggest any significant contribution to absorption by brown carbon.
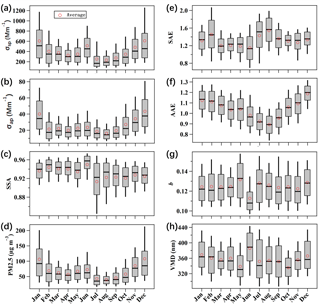
Figure 3Seasonal cycle of selected aerosol optical properties and supporting parameters at SORPES. (a) Scattering coefficient at 525 nm, (b) absorption coefficient at 520 nm, (c) backscatter fraction at 525 nm, (d) single-scattering albedo at 520 nm, (e) scattering Ångström exponent between 450 and 635 nm, (f) absorption Ångström exponent between 370 and 950 nm, (g) PM2.5 concentration and (h) volume mean diameter for 6–800 nm particles. The solid lines represent median values, red cycles stand for averages, the boxes are 25 and 75th percentiles, and the whiskers represent the 10th and 90th percentiles.
3.2 Seasonal variation of aerosol optical properties
We used the hourly-averaged data measured from June 2013 to May 2015 for the analyses of seasonal cycles of AOPs and the influencing factors at SORPES. The four seasons are defined as follows: spring: March–May, summer: June–August, autumn: September–November and winter: December–February. The seasonal cycles of 8 parameters: σsp, σap, b, SSA, SAE, AAE, PM2.5, and GMD are presented in Fig. 3 and in Table 3. Both scattering and absorption coefficients have a clear seasonal cycle. In general, they were clearly higher in late autumn and winter than in summer. Both coefficients reached the peak monthly averages in December, σsp=610 Mm−1 at λ=525 nm and σap=43.6 Mm−1 at λ=520 nm, more than twice as high as those in August, σsp=232 Mm−1 and σap=16.2 Mm−1. Such a seasonal cycle agrees with the seasonal cycle of PM2.5 mass concentrations. Several possible explanations are the following: (1) in winter the prevailing wind from the YRD region or the NCP brings polluted air masses continuously, which enhances the pollution while wind blows from different, cleaner directions by the summer monsoon in summer (Ding et al., 2013c demonstrate this point from LPDM simulation); (2) more efficient vertical mixing of the aerosol to higher altitudes, which dilutes the aerosol loading in the boundary layer in summer (in other words, winter aerosols are confined into a thinner mixing layer than in summer, which leads to a higher particle concentration in winter; Ding et al., 2016a); (3) both in-cloud scavenging and precipitation scavenging are stronger in summer than winter because of more precipitation in summer than in winter; and (4) the anthropogenic emissions have obvious seasonal variations. A detailed discussion of the seasonal variations in emissions and their potential effects on the AOPs is presented in the Supplement (Sect. S5).
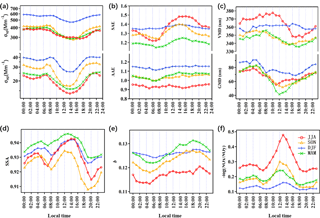
Figure 4Diurnal variations in (a) scattering coefficient (σsp) at λ=525 nm and absorption coefficient (σap) at λ=520 nm, (b) Ångström exponents of scattering (SAE) and absorption (AAE), (c) volume mean diameter (VMD) and geometric mean diameter (GMD), (d) single scattering albedo (SSA) at λ=520 nm, (e) backscatter fraction (b) at λ=525 nm, and (f) photochemical age of air mass (−log(NOx / NOy) in the four seasons. The values are the averages of the corresponding hour in the four seasons coded by the colors as green: spring, red: summer, yellow: autumn, blue: winter.
Contrary to the otherwise relatively low scattering coefficients in summer, the average σsp in June (513 Mm−1) was the third highest among all 12 months, just below 610 Mm−1 in December and 603 Mm−1 in January. The median σsp in June was 453 Mm−1 almost the same as the median value of December (456 Mm−1). Moreover, in June the 25th percentile of σsp (294 Mm−1) was the highest among all 12 months. This indicates that the high monthly average scattering coefficient was not caused by some short episodes. The absorption coefficient was only a little bit higher than in the adjacent months (Fig. 3b). However, the average SSA in June was 0.95 at 520 nm, much higher than in any other month. Both backscatter fraction (b) and SAE reached the lowest monthly averages in June, even though their seasonal variation was otherwise different. The independent parameters PM2.5 and the geometric mean diameter and the volume mean diameter were also higher in June than in the adjacent months, both of which are consistent with the higher σsp and the high mean diameters also with the low b and SAE. There is a possibility that the high scattering coefficients in June were due to high RH and insufficient humidity correction with the parameterization described above. To evaluate this possibility, AOPs were also modeled with a Mie code and the DMPS data. The DMPS was measuring dry size distributions and yet the modeled AOPs present the same seasonality, supporting the above discussion. The details are in the Supplement Sect. S4. The high σsp and PM2.5 values in June were possibly due to biomass burning since in June there were more fire spots observed than in any other month within a 300 km range from SORPES (Ding et al., 2013a, b). A more detailed discussion of the period is omitted from the present paper.
3.3 Diurnal cycles
The hourly-averaged data were classified according to the hour of the day in the four seasons. Figure 4 presents diurnal cycles of aerosol optical properties σsp, σap, SSA, and b as well as the diurnal cycles of the supporting parameters photochemical age and the geometric and volume mean diameters (GMD and VMD). The averaging was conducted so that the value for 00:00 is the average of 5 min data between 00:00 and 01:00 and analogically for all hours of the day. All times discussed in this section are local time (LT). Diurnal cycles of different percentiles for these parameters as well as averaged particle size distribution patterns are presented in the Supplement (Fig. S6).
A clear diurnal variation in σsp and σap was observed in all seasons (Fig. 4a). In each season, there was an evident minimum in the afternoon around 14:00 and a continuous almost flat peak from 20:00 to 08:00 the next day. Within these 12 h flat peaks, there were two maxima, one around 20:00–23:00 in the evening and another at around 08:00. We call them the “evening peak” and the “morning peak” here. Horvath et al. (1997) and Lyamani et al. (2010) found a similar cycle with two maxima in early morning and afternoon. Notably, even though the minimum in the early morning is only 0–5 % lower than the two peaks, we use two maxima and two minima to divide the whole cycle into four stages: (1) in the early morning, SSA (Fig. 4d) started to decrease and reached a minimum around 07:00 and 08:00. This decrease indicates that relatively more light-absorbing aerosol was emitted around that period and possibly influenced by vehicle emission during rush hours. (2) Then SSA increased significantly and reached the highest value of the day at around 14:00. The SSA at λ=520 nm even exceeded 0.94 in summer and spring. (3) After reaching the maximum, SSA decreased rapidly and reached the lowest daily values at around 19:00–20:00, which can be considered as a combined influence by deceleration of secondary aerosol formation at dusk and an additional soot emission during rush hours. (4) SSA increased gradually from 20:00 to early morning the next day.
The diurnal cycles are influenced by the variations in the PBL height, anthropogenic activities and photochemical reactions. The strong decrease in σsp and σap after the morning peak can be associated with the boundary layer development in daytime, which enhances convective activity and decreases the particle loading at ground level. Both σsp and σap reached minima at around 14:00 and then increased again as vertical mixing got weaker. The increase in absorption in the afternoon was approximately as fast as the decrease after the morning peak: σap at 20:00 was almost the same as at 08:00. On the other hand, the increase in scattering after 14:00 was somewhat slower than the decrease from 08:00 to 14:00. The maximum σsp was reached later than the maximum σap and as a result SSA decreased to a minimum at about 19:00–20:00.
The diurnal cycle of SSA (Fig. 4d) followed very closely the diurnal cycle of the photochemical age (Fig. 4f): the air masses with the lowest photochemical age contained the aerosol with the lowest SSA, which suggests that in these air masses there were BC particles that had not been coated with as thick a coating with light-scattering material as in the aged air masses. The most probable source for such intensive NOx emission in the morning is the rush-hour traffic. For stage 2, we notice that the start time for the increase is 1 hour earlier in summer and spring than in winter and autumn. An opposite time difference can be observed in the evening. The obvious explanation is the seasonal variation in the length of the day: the average sunrise (and sunset) times are approximately 07:00 (17:30), 05:30 (18:30), 05:15 (19:00), and 06:15 (19:30) for winter, spring, summer, and autumn, respectively.
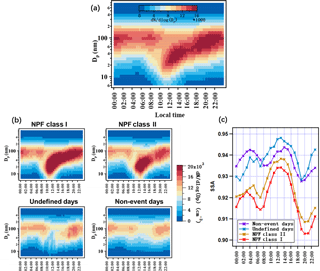
Figure 5Diurnal cycles of particle number size distributions and SSA classified into NPF classes. (a) Averaged particle number size distribution of the whole measurement period, (b) diurnal number size distributions averaged over NPF class I and NPF class II days, undefined days, and non-event days, and (c) average diurnal cycle of SSA by using the dates classified as in the NPF classification.
Kulmala et al. (2016) estimated that it may be possible that about half of the particles in the accumulation mode, i.e., the optically relevant size range originate, from new particle formation (NPF) at SORPES so it is worth estimating whether it affects the SSA diurnal cycle. On average, at about 10:00–12:00 when the photochemical reactions are active and solar radiation is strong, NPF occurred at SORPES as discussed in detail by Qi et al. (2015). NPF produces small particles that are initially too small to affect the total light scattering. However, at the time of the NPF some of the older, larger particles still remained which resulted in a bimodal size distribution with a fast growing nucleation mode and an Aitken mode in the particle diameter range of about 70–90 nm (Fig. 5). The species that condense on the newly formed particles are typically light scattering inorganic and organic species and they condense also on the Aitken-mode particles. At about 12:00–14:00 the newly formed particles had grown into the size range of about 20–50 nm, which has still a very small scattering cross section (, where Qs is the scattering efficiency) compared with that of the larger mode that at this time had grown by condensation of light-scattering species to ∼ 100 nm or larger. This mode was probably only partially responsible for the high SSA. At the same time the boundary layer height (not shown) and the photochemical age reached the diurnal maximum (Fig. 4f). This suggests that vertical transport of aged aerosol from upper levels contributes to the high SSA when the vertical mixing is at its strongest. It is also likely that the aged aerosol has a mixture of accumulation-mode particles transported from further away and more local aerosol that were formed and grown during the previous day and remained in a residual layer. Even though the above discussion shows that particles formed by NPF cannot be responsible for the growth of SSA during the same day it is worth noting that NPF and SSA diurnal cycles do have a connection in our data. During the clearest NPF days, classified into the class I and II NPF according to the classification used by Qi et al. (2015), the SSA diurnal cycles were also the clearest and largest (Fig. 5b, c). On average, NPF took place around 10:00 after which particles grew and reached optically significant sizes only late in the evening when SSA reached the minimum. By using the above reasoning this suggests that both the condensation on existing particles and the vertical mixing were then the strongest.
In the afternoon, the boundary layer started to decrease and the air masses contained less aged and more fresh aerosols and lower SSA (Fig. 4d, f). The minimum photochemical ages and SSA were observed at stage 3 at about 18:00–20:00 LT, depending on the season. At that time the size distributions show that the number concentrations of Aitken-mode particles increased more than by a straightforward growth of the particles formed earlier during the day (Fig. 5). This suggests that there was an injection of fresh BC particles into the boundary layer during the evening rush hour. Very probably, aerosol was then an external mixture of those grown after the NPF and the more freshly emitted BC. At stage 4, during the course of the night, σap decreased slowly indicating weaker BC emissions. However, the particles kept growing both by condensation and coagulation as seen by the growing GMD, VMD, and σsp (Fig. 4a, c). Also, SSA grew all night long, which suggests that the main mechanism of the growth was again condensation of light-scattering species. The formation of these species in the absence of light may be due to NO3 radical chemistry, as was suggested to be the explanation of a similar increase in SSA at night in Sao Paolo, Brazil (Backman et al., 2012). Possible diurnal cycles of emissions, not only including traffic, and diurnal cycles of air mass transport may also affect the diurnal cycles of AOPs. However, we are not able to figure out whether and how they would cause the afternoon SSA peak.
The diurnal cycle of SAE (Fig. 4b), a usual qualitative indicator for the dominating particle size, suggests that the size was the smallest during 14:00–16:00 when SAE reached maximum values of each seasonal diurnal cycle, approximately 1–2 h later than SSA. The interpretation of the inverse relationship between SAE and particle size is not correct when the size distribution consists of multiple modes (e.g., Schuster et al., 2006). This is consistent with the comparison between the diurnal cycles of the GMD and SAE: the GMD was the smallest during 10:00–12:00 when the newly formed particles formed the nucleation mode at the same time the Aitken mode was present. After about 16:00–18:00, the size distribution became close to unimodal and the relationship inverse: GMD grew almost steadily and SAE decreased almost steadily during the night until the morning peak. Then the σap grew and GMD decreased but so did SAE still until about 08:00 the next day.
The relationship of the backscatter fraction b and particle size is basically similar to that of SAE and particle size: for small particles b is large – for Rayleigh scattering, i.e., for gas molecules b=0.5 – and it decreases with increasing particle size. For real atmospheric size distributions, the interpretation of b gets complicated when multiple modes are present, as for SAE. The diurnal cycles of b suggest that particles were the largest just before the morning peak at about 05:00, after which the optically dominating particle size decreased and b grew until 12:00–19:00 depending on season (Fig. 4e). After the peak value, b decreased almost steadily during the night in agreement with the growing GMD (Fig. 4c), in principle very much like SAE. There are also some obvious differences between b and SAE diurnal cycles. The b peak values were reached later than SAE in winter, spring, and autumn but earlier in summer. Another difference is that in summer b remained at a steady high value for several hours, from 12:00 to 21:00, contrary to SAE.
The range of AAE values during the diurnal cycle was not very large (Fig. 4b). AAE was usually the smallest during the morning peak and then it grew slowly. The largest diurnal growth was in spring: from ∼ 1.00 to ∼ 1.09. The average AAE diurnal variation is in a range that suggests it can be attributed to variations in the diameter of a BC core and a light-scattering shell coating it (e.g., Gyawali et al., 2009; Lack and Cappa, 2010; Wang et al.,2016). A detailed analysis of this would require measurements with an instrument that can actually measure the BC core and shell such as the single-particle soot photometer (SP2; e.g., Schwarz et al., 2008).
3.4 Aerosols in different air masses
3.4.1 Aerosol optical properties as a function of wind speed and direction
Figures 6a and b present the scattering and absorption coefficients and Fig. 6c the photochemical age as −log(NOx / NOy) observed at the different wind directions and speeds by using polar coordinates. Figure 6d presents a standard wind rose for the 2 year measurement period. The prevailing wind direction is E and NE. Only a small fraction of wind blew from the SW and NW. There was no big difference in wind speed from the different directions: the largest and lowest average wind speed (WS) was 3.9 and 2.0 m s−1 from the directions 100–105 and 160–165∘, respectively.
Generally, both σsp and σap decreased with increasing wind speed when WS > 1 m s−1 in all directions (Figs. 6a, b, S7). This suggests that at strong winds, air was generally more diluted with cleaner air from upper altitudes by turbulent mixing. The effect of horizontal transport, increased dispersion, and shorter residence time within emission areas may also contribute to the decrease in σsp and σap. We do not make further analyses on the possible explanations, it would require extensive modeling that is out of the scope of the present paper. An exception was the west-northwesterly (WNW) wind direction (WD ≈ 285 ± 15∘) sector since σsp did not decrease much with increasing wind. In this sector the above-mentioned dilution effect required stronger winds: the highest σsp was observed at WS ≈ 2.5 ± 0.5 m s−1 but almost as high values were still observed at WS ∼ 7 m s−1, above which σsp started decreasing. However, the frequency of winds from this sector WD was very low, only 1.3 % of whole period so it does not change the general picture of decreasing scattering with increasing wind.
High σsp (>480 Mm−1) was also observed at weak (WS < 2 m s−1) southwesterly (SW, WD ≈ 215 ± 10∘) winds. This is probably a mixture of large-scale, regional, and local emissions. The center of the city is in that direction and at low wind speeds pollutants are easily transported to SORPES without a strong dilution. However, the center of Nanjing is still too close to be the most important contributor to light-scattering particles, especially in case particles are formed by NPF and growth by condensation. A rough estimate of the transport distance needed to grow particles to sizes that scatter light efficiently can be calculated by using the information on the particle growth rate and wind speed. The time evolution of the average diurnal particle size distribution (Fig. 5) shows that new particles with Dp≈10 nm formed before noon grew to sizes that scatter light significantly, i.e., 100 nm in about 10 ± 1 h yielding an approximate growth rate (GR) of ≈ 9 ± 1 nm h−1, which agrees with the analysis of Qi et al. (2015). In 10 h at WS = 0.5 m s−1 the air mass would have drifted a distance of 18 km which is just the distance to the city center. At the weakest winds from the SW the contribution of the city to the amount of scattering particles may thus have been observed even if particles were formed by NPF. However, in a city a large fraction of aerosols are primary particles, especially BC emitted from vehicles in the size range of ∼ 60 ± 20 nm (e.g., Bond et al., 2013; Kulmala et al., 2016). At a GR of ≈ 9 nm h−1 it would take ∼ 5 ± 2 h for them to grow to sizes >100 nm. In these cases particles emitted from within Nanjing will also affect scattering but their SSA would be lower than the observed SSA. This was estimated by a core-shell Mie code (Wu and Wang, 1991) that yields SSA < 0.6 at λ=520 nm for BC particles with a core of 60 ± 20 nm coated with 40 nm of scattering material, for instance ammonium sulfate. At the weakest winds from the SW sector the observed SSA at λ=520 nm was > 0.9, clearly higher than it would be if particles were emitted as BC particles within Nanjing and coated by condensation, supporting the interpretation that sources of the high σsp during weak winds are not only within Nanjing.
When wind speed is higher, regional transport of pollutants plays an even more important role. The highest σsp in Fig. 6a is in the WNW sector at WD ≈ 285 ± 15∘ and WS ≈ 2.5 ± 0.5 m s−1. At this speed the air masses drift 90 ± 18 km in 10 h that was estimated to be the time to reach optically significant particle sizes since the formation by NPF. This is outside the urban area of Nanjing. In an urban environment, significant amounts of particles are also emitted as primary particles, mainly BC, so it should be estimated whether they could explain the observation. At WS = 2.5 m s−1 it would take approximately 2 h for air masses to be transported the 18 km from the center of the city so at the observed high growth rate of 9–10 nm h−1 particles would grow 18–20 nm. Fresh BC particles are typically in the size range of ∼ 60 ± 20 nm (Bond et al., 2013) so they would reach the size range of ∼ 80 ± 20 nm. This is still much too low to explain the high σsp in the WNW sector. Further, 60 ± 20 nm BC particles coated with 20 nm of scattering material would have a very low SSA: the core-shell Mie code (Wu and Wang, 1991) yields SSA < 0.3 at λ=520 nm, whereas the SSA at WD ≈ 285 ± 15∘ and WS ≈ 2.5 ± 0.5 m s−1 was high, > 0.95. These analyses suggest that the aerosol responsible for these highest values originated from outside the urban area of Nanjing regardless of whether they were formed by NPF or emitted as primary particles. An additional analysis (Supplement Sect. S7.2) shows that K+ concentration was slightly elevated in this sector suggesting biomass burning may have contributed some to the high σsp.
At low and moderate winds (WS < ∼ 3 m s−1) from east and southeast (WD range ∼ 75–165∘), σsp was also high, > 480 Mm−1, suggesting that the air masses from the YRD have a higher σsp than the average value. The SSA in this sector was also high, > 0.92, indicating aged aerosol which was in agreement with the high photochemical age of air masses, i.e., −log(NOx / NOy) > 0.28 (Fig. 5c).
The absorption coefficient had a different dependence on wind. The relationship between σap and WS is very clear: at weak wind, σap was obviously high and it decreased significantly as WS increased in all wind directions (Fig. 6a, b, S7). It was the highest at weak southwesterly winds (SW, WD ≈ 225 ± 15∘ and WS ≈ 0.8 ± 0.2 m s−1) but high σap values were observed especially at winds from the southern sector WD = 180 ± 30∘ at WS < 2.5 m s−1. The high σap values in the SSW sector (WD ≈ 200 ± 10∘) were observed approximately from the same direction as the above-mentioned σsp SW peak direction and can be attributed to emissions from the urban areas of Nanjing. This is also supported by the comparison with the polar contour plot of the photochemical age of air masses, i.e., −log(NOx / NOy) (Fig. 5c). It shows that photochemically fresh air with −log(NOx / NOy)≤0.12 was observed at SORPES with winds from this same direction. Also σap≥42 Mm−1 from the SSE sector (WD ≈ 165 ± 15∘) follows approximately the contour of −log(NOx / NOy)≤0.12 suggesting that the high σap from this sector was associated with photochemically fresh traffic emissions. An analysis using aerosol chemical composition and wind data (Supplement S2.3) suggests that the main cause for the high σap during winds from the SSE sector is the nighttime heavy traffic on country dirt roads to several small factories and mines located within about 3–10 km to the SE of SORPES
A careful inspection of the contour polar plots for σsp and σap (Fig. 5a, b) shows that in the WNW sector actually both of them have local maxima at the same WS–WD combinations. In these local maxima SSA is high, ∼ 0.95 or higher, suggesting that the aerosol is aged. On the other hand, photochemically fresh air with −log(NOx / NOy)≤0.2 was also observed with winds from the same WNW sector as the highly scattering aerosol. These are apparently controversial results since to grow particles to the size range that scatters light at high efficiency requires time. Wind blows from this direction mostly in winter (Fig. 5d). The high σsp was observed in winter pollution episodes during which SSA grew during the evolution of the episodes, as will be discussed below. There is a highway located to the west of SORPES so the lower-than-expected photochemical age can be explained by the flow of the aged and grown particles over the highway and mixing with fresh NOx emissions.
Another interesting wind direction is the NE sector (WD ≈ 45 ± 15∘). At all wind speeds from that sector the photochemical age is relatively low and the lowest (−log(NOx / NOy)≤0.12) at high wind speeds (WS ≈ 6 ± 1 m s−1). With this WS–WD combination both σsp and σap were low. This suggests that in this direction there is a NOx emitter that does not emit significant amounts of BC and that it is relatively close since particles have not grown to size ranges large enough to affect σsp significantly.
3.4.2 Lagrangian dispersion modeling
The 72 h retroplumes were calculated every 3 h, so there were eight retroplumes per day. To assess the source areas of high (low) σsp and σap the retroplumes of the 3 days with the highest (lowest) daily-averaged σsp and σap of each month were averaged, altogether 576 retroplumes. Since there are approximately 30 days per month, the three lowest daily averages represent approximately the lowest 10 % and the three highest daily averages the highest 10 % of the daily averages. The reasoning for this approach is that (1) diurnal cycles are dominated by cycles of PBLH and photochemistry and (2) seasonal variation hides obvious pollution episodes in cleaner months. The results are shown in Fig. 7. It shows that the potential source region of the highest 10 % of both σsp and σap are within a large area from eastern China, with the highest retroplume around Nanjing spread between the longitudes 115–123∘ E (∼ 700 km) and the latitudes 28–35∘ N (∼ 800 km) (Fig. 7a, b). The color represents the product of integrated particle concentration and residence time of the particles within a certain grid cell, as explained in Section 2.5. It can be interpreted that pollutants emitted in a grid cell with the value of 10−12 can affect the extensive AOPs at SORPES 100 times more than a grid cell with the value of 10−14 if the emission rate is the same and chemical reactions, removal, and other processes during the transport are ignored. For the lowest 10 % of both σsp and σap daily-average air masses mainly originated from the ocean in the east with a fast transport pathway (Fig. 7c, d). A comparison of Fig. 7a, b suggests a slightly different transport pattern for the highest 10 % of σsp and σap daily averages. For both σsp and σap, sub-regional air masses from the southeast contribute clearly to the highest 10 %, i.e., from the city cluster in the YRD region (Ding et al., 2013b), as well as air masses transported from various directions, indicating more local emissions for Nanjing and the adjacent cities. The most obvious difference is that high σsp is more clearly associated with air mass transport from the northwest, especially from regions north of 35∘ N, i.e., from the NCP and Shandong province. These are regions where the high emission of SO2 could have a large regional impact on scattering coefficient in the south as a high concentration of sulfate is formed.
The results from the retroplume calculation (Fig. 7) apparently seem inconsistent with the results from the wind rose analysis (Fig. 6). One of the explanations is that the wind rose analysis was based on hourly data but the retroplume calculations on daily averages. In other words, the wind rose results show more details about the change of aerosol coefficients according to changes in wind direction of local wind at high temporal resolution, but the latter shows more information about the history of air masses when they get transported long distances.
3.5 Analysis of a polluted winter period
The above-presented analyses were made by using the whole data set. Pollution episodes in SORPES are clearer in winter so a winter-time polluted period from 1 November 2013 to 28 February 2014 will be discussed in detail below. Tang et al. (2016) analyzed the sources contributing to submicron particulate matter in the haze episodes observed in the center of Nanjing in December 2013 and concluded that the high aerosol pollution was mainly due to regional transport. The analysis below complements that by Tang et al. (2016) since they did not present an analysis of the evolution of AOPs.
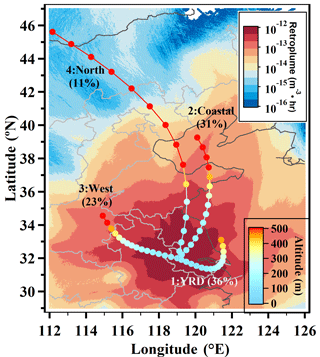
Figure 8 The average back-trajectories of the clusters obtained from the back-trajectory cluster analysis for the winter period 1 November 2013–28 February 2014. The duration of the trajectories is 48 h. The average 72 h retroplume for the same period is depicted with the background color.
The back-trajectories were clustered into four clusters as explained in Sect. 2.5. The average trajectories of the clusters obtained for the period are presented in Fig. 8. The clusters are given more descriptive names: cluster 1 is labeled YRD that constitutes 36 % of the trajectories and represents air from the east; cluster 2 is labeled Coastal, 31 % of the trajectories, representing air coming from the northeast partly over the sea at a low speed; cluster 3 is labeled West, 23 % of the trajectories, representing purely continental air from the west; cluster 4 is North, 11 % of the trajectories, representing fast-flowing air from the north. In addition to the average trajectories of each cluster, the average 72 h retroplume is also depicted in Fig. 7 as the background color. It is reasonable as it resembles the retroplumes for the highest 10 % daily averages of σsp and σap (Fig. 6a, b) for the whole period.
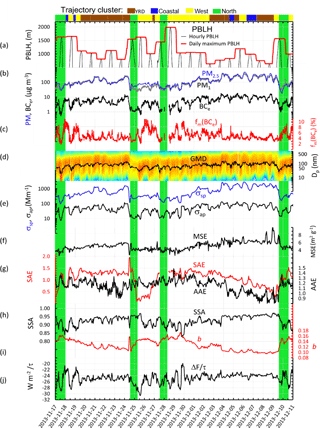
Figure 9Hourly-averaged aerosol optical properties, mass concentrations, and modeled boundary layer height during a polluted period 17 November 2013–11 December 2013. Top: time series of trajectory clusters shown with color coding, (a) PBLH: planetary boundary layer height, (b) PM2.5 and PM1: mass concentrations of particles smaller than 2.5 and 1 µm, BCe: equivalent black carbon concentration, (c) fm(BCe): mass fraction of BCe, (d) particle number size distribution and GMD: geometric mean diameter, (e) σsp: scattering coefficient at λ=525 nm, σap: absorption coefficient at λ=520 nm, (f) MSE: mass scattering efficiency at λ=525 nm, (g) SAE and AAE: scattering and absorption Ångström exponents, (h) SSA: single-scattering albedo at λ=520 nm, (i) b: backscatter fraction at λ=525 nm, and (j) ΔF∕τ: aerosol radiative forcing efficiency at λ=525 nm
The time series of several extensive and intensive aerosol properties in the period 17 November 2013–11 December 2013, a subset of the whole winter period analyzed, are presented together with the modeled PBLH in Fig. 9. In addition, the time series of the trajectory cluster classes are presented on the top line with colors. This is not the whole period for which the back-trajectory clusters were calculated because of the amount of details. The full time series of the winter period are shown in the Supplement (Fig. S11).
3.5.1 Extensive aerosol properties
It is possible to count approximately 14–16 distinguishable pollution episodes by using either the PM concentration or the scattering coefficient time series. The analyzed period was 120 days long so, on average, there was a pollution episode about every 8 ± 1 days. The definition of the start and end of a pollution episode is not unambiguous, so the above number should be treated cautiously. The concentrations of PM2.5 and PM1 tracked each other very closely suggesting that, within the uncertainties, most of the aerosol mass is secondary, pollution-related compounds, and that soil dust which in general is in the super-micron size range contributed very little to aerosol mass and scattering at SORPES.
The trajectory cluster time series, color coded on the top of Fig. 9, shows that when air masses were associated with the West or the YRD clusters, there were no big differences in concentrations. The Coastal trajectory cluster was often but not always associated with lower concentrations. Most of the episodes ended with trajectories associated with the North cluster (see Supplement Fig. S11). Meteorological analyses show that the trajectories associated with the North cluster brought air from the north, high above Beijing during cold fronts. There were also episodes during which there were trajectories belonging to many different clusters, YRD, West, and Coastal but there were no clear differences in concentrations until the clearing phase associated with the cluster North. During these episodes, polluted air arrived from all directions in line with wind roses that showed there was no strong dependence on wind direction. Instead, the concentrations kept rising.
Most episodes followed a similar pattern: during the evolution phase the PM concentrations grew day after day during several days at a rate of some tens of µg m−3 day−1 but the end of the episode was usually abrupt, air cleared within hours. The largest drop in the period occurred on 2–3 February when PM concentrations, σsp, and σap decreased by more than an order of magnitude within hours. The same cycle applied to all extensive parameters: PM and BCe concentrations, σsp and σap clearly increased more slowly during the growth phase of the episodes than decreased in the end (Fig. 9b, e). At the same time the daily maximum PBLH (Fig. 9a) decreased during most of the episodes from more than ∼ 1500 m to less than ∼ 700 m. This PBLH decrease is in agreement with the analysis of Petäjä et al. (2016) and Ding et al. (2016a) who showed that high PM and especially BC concentrations enhance the stability of a polluted boundary layer, which in turn decreases the boundary layer height and consequently cause a further increase in PM concentrations.
Even during the growing phase of the episodes there were obvious diurnal cycles of the AOPs. For instance, low PM concentrations, σsp, and σap during daytime and higher at night combined with a growing trend can be explained with the formation of a residual layer: when the PBLH decreases at night part of the aerosol remains above the PBL. The following day new pollutants get mixed with the pollutants remaining in the residual layer. This leads to a continuous accumulation of aerosols in the PBL and a slower, non-symmetric cycle. Part of the accumulation is due to BC, as is seen in the increasing BCe concentration even though the mass fraction of BCe (fm(BCe)=BCe/PM2.5) decreased from ∼ 10 to ∼ 3 % during the growth phase of the episodes (Fig. 9c). The particle number size distribution time series (Fig. 9d) shows that there are indications of NPF also during the polluted period. Even though these new particles grow quickly, it is shown above that their contribution to total scattering remains low during the day of NPF.
3.5.2 Evolution of intensive aerosol properties
The intensive aerosol properties, i.e., those that do not depend on the amount of particles, clearly evolved during the pollution episode cycle. First, the effective particle size grew, which is depicted as the time series of the geometric mean diameter (GMD; Fig. 9d). There was an obvious diurnal cycle with the GMD as well. The growth leads to many changes in the intensive AOPs. The mass scattering efficiency MSE = σsp m−1 (Fig. 9f) grew during the extended pollution episodes from about 4 to ∼ 6 m2 g−1, which is in the range presented by Hand and Malm (2007). In other words, the unit mass of aerosol scattered light more efficiently at the end of the episode than at the beginning. An obvious explanation is that this is due to the growth of both particle diameter and the scattering efficiency (Qs) even though the changing refractive index also plays a role in this. The Ångström exponents of scattering and absorption (SAE and AAE, respectively; Fig. 9g) as well as the backscatter fraction b (Fig. 9i) decreased as the particles grew. The decrease in AAE during the growing phase of the episodes are explainable by a growing shell on a BC core as has been modeled by Gyawali et al. (2009) and Lack and Cappa (2010). At the same time, SSA (Fig. 9h) increased, which can be explained by condensation of light-scattering material and thus increasing the thickness of a shell surrounding a BC core.
Contrary to the strong evolution of the other AOPs during the growth phase of the pollution episodes, the radiative forcing efficiency (RFE = ΔF∕τ; Fig. 9j) did not vary strongly. This is interesting since intuitively it could be thought that the higher SSA grows the lower is the RFE, in other words the more the particles cool the atmosphere. That RFE remained fairly stable is due to the growth of the particles: larger particles scatter light upwards less efficiently than small ones, which to some extent compensates the higher SSA as will be shown below. A similar phenomenon was observed by Garland et al. (2008) during an intensive campaign in Guangzhou in southeastern China in July 2006.
3.6 Relationships of AOPs
Above, the evolution of AOPs during the polluted winter period were discussed, here the whole 2 year data set will be used for an analysis of the relationships of AOPs. First some of them are compared with the effective mean diameters obtained from the DMPS data, next some dependencies of AOPs on each other and the photochemical age are discussed, and in the end there is an analysis of the radiative forcing efficiency.
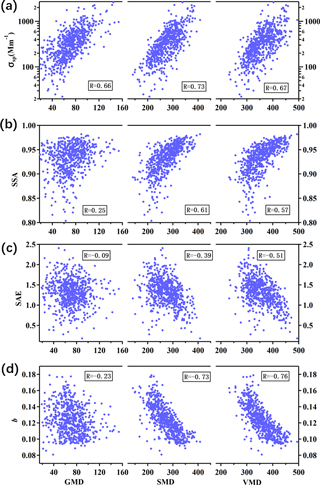
Figure 10Relationships between (a) scattering coefficient at λ=525 nm, (b) SSA at λ=525 nm, (c) Ångström exponent of scattering (SAE), and (d) backscatter fraction at λ=525 nm and the effective mean diameters GMD, SMD, and VMD. The correlation coefficients are those obtained from linear regressions.
3.6.1 Relationships between optical properties and particle size
The scatter plots (Fig. 10) show that all the analyzed AOPs clearly depend on SMD and VMD but not so obviously on GMD. σsp was generally higher the larger the weighted diameters were (Fig. 10a). This is the intuitively most logical relationship of all those presented in Fig. 10 since σsp of a size distribution is calculated from Eq. (13) that includes the surface area of a spherical particle. When particles grow their surface area grows and they scatter more light.
The observed darkest aerosol had SSA < 0.85, which is not even close to that of pure fresh BC. Then GMD was in the range of 30–80 nm, SMD at 250 nm, and VMD at 300–350 nm. These can be compared with BC size distributions observed elsewhere. Schwarz et al. (2008) measured BC size distributions with a single-particle soot photometer (SP2) and found that the mass median diameter (MMD) and geometric standard deviation of these distributions were 170 and 1.71 nm, respectively, in an urban air, and 210 nm and 1.55, respectively, in continental background air. These values yield number mean BC diameters of 72 and 118 nm for urban and continental background air, respectively. Our GMD was in the same range but VMD was clearly larger. It can therefore be deduced that even the darkest aerosol we observed was not fresh BC. The cases with very high SSA and GMD < 40 were very probably associated with NPF events. The SSA growing with growing weighted mean diameters is plausibly explainable by a larger scattering shell on an absorbing core.
SAE is a parameter that is often used as a qualitative indicator of dominating particle size so that large values indicate a large contribution of small particles and small values a large contribution of large particles. For SMD and VMD this is indeed so in our data (Fig. 10c). GMD, on the other hand, cannot be predicted at any uncertainty with SAE. All these are consistent with the relationships observed in a completely different environment, the boreal forest at SMEAR II, Finland (Virkkula et al., 2011). All the same conclusions apply to the relationship between the weighted mean diameters and backscatter fraction, b, which is an even better indicator of the dominating particle size (Fig. 10d). This is logical since it is well known that forward scattering increases with growing particle size. Both b and SAE provide information on the particle size distribution but they are sensitive to somewhat different particle size ranges (e.g., Andrews et al., 2011; Collaud Coen et al., 2007). Collaud Coen et al. (2007) presented a detailed model analysis of both of these AOPs and showed that b is most sensitive to small accumulation mode particles, i.e., particles in the size range < 400 nm whereas SAE is more sensitive to particles in the size range 500–800 nm. Delene and Ogren (2002) showed the importance of fine-to-coarse scattering ratio (ratio of scattering in the PM1 and PM10 size ranges).
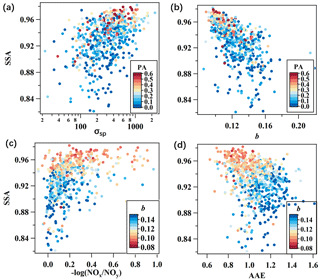
Figure 11Relationships of single-scattering albedo SSA at λ=525 with (a) scattering coefficient, (b) backscatter fraction, (c) photochemical age, and (d) Ångström exponent of absorption. In (a) and (b) the data points are color coded with the photochemical age (PA) and in (c) and (d) with backscatter fraction.
The relationships of SSA are analyzed further with the scatter plots in Fig. 11. SSA was in general higher than σsp was but the range of SSA was very large, ∼ 0.82–0.98 when σsp was in the range < 400 Mm−1 (Fig. 11a). SSA was always high, > 0.94 in the most polluted air masses when σsp was > 1000 Mm−1. The color coding with the photochemical age further shows that the most polluted air masses were mainly aged with PA > 0.4. There are, however, some data points with PA < 0.2 indicating relatively fresh air even at σsp≈1000 Mm−1. These points have a lower SSA, down to ∼ 0.90. It is very unlikely that scattering coefficients as high as 1000 Mm−1 are due to nearby emissions so the data suggests that in these cases the aerosol consists of an external mixture of long-range-transported strongly scattering particles and fresh, possibly traffic-related, BC particles that have not yet been coated with a thick shell. The comparison of SSA with the backscatter fraction b (Fig. 11b) shows that the lowest SSA was mainly observed when b was in the range ∼ 0.12–0.16 and the highest SSA at b < 0.10, which indicates large particles as discussed above. The highest backscatter fractions were observed in fresh air masses (PA < 0.2) and lowest in the most aged air masses. However, there were again some points with both a low PA and b suggesting that there was an external mixture of long-range-transported and fresh aerosols. The scatter plot of SSA vs. PA (Fig. 11c) shows very clearly that the darkest aerosol (SSA < 0.9) was in the freshest air masses. But it also shows that in some of the fresh air masses with PA < 0.1, SSA was very high, > 0.96, suggesting that there were also such NOx emissions that were not associated with BC emissions. This is in line with the analysis presented above in Sect. 3.4.1.
The comparison of SSA with AAE (Fig. 11d) shows that AAE was in the range ∼ 0.9–1.2 which is approximately that estimated from traffic emissions (e.g., Zotter et al., 2017). Lower AAE was observed with high SSA and low backscatter fractions, i.e., when particle size distributions were dominated by large particles. In the analysis of the polluted winter period it was stated that the decrease in AAE during the growing phase of the episodes could be explained by a growing shell on a BC core (e.g., Gyawali et al., 2009; Lack and Cappa, 2010). A similar relationship is observed in the scatter plot of the whole data set.
3.6.2 Analyses of the radiative forcing efficiency
The aerosol-related parameters affecting the radiative forcing efficiency RFE = ΔF∕τ, Eq. (9), are the single-scattering albedo and backscatter fraction. In this study, RFE is always negative, in other words aerosols cool the atmosphere. To avoid confusion, we define “increase” as “the magnitude of the effect is increasing; the sign of the effect is negative” throughout the text. A simple intuitive assumption is that the darker the aerosol, i.e., the lower the SSA is, the less it cools the atmosphere, which means the magnitude of RFE should be lower (the sign of the effect is negative). However, the scatter plot of the real data shows there was a very weak relationship between RFE and SSA alone (Fig. 12a). The relationship between RFE and b is somewhat clearer: the magnitude of RFE increases with increasing b (Fig. 12b). But the data are really scattered in a wide range, which is due to the fact that RFE depends on both SSA and b.
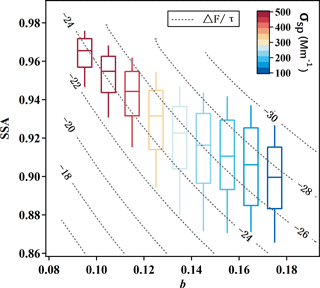
Figure 13Relationship of SSA with the backscatter fraction and the radiative forcing efficiency at λ=525 nm depicted with the RFE = ΔF∕τ isolines in W m−2.
The relationships between RFE, SSA, and b become clearer in a scatter plot of SSA vs. b, where the isolines of constant RFE as a function of both parameters are plotted (Fig. 13). Most data are between the RFE isolines −22 and −28 W m−2. The data do not exactly get clustered around any single RFE isoline. This was studied by classifying SSA into backscatter fraction bins with a width of Δb=0.01, calculating the percentiles of the cumulative distributions in each bin and presenting them in the box plots in Fig. 13. Interestingly, the highest b bin () has the lowest median SSA but also the most negative RFE, ∼ −28 W m−2, but the lowest b bin () has the highest SSA but the most negative RFE, ∼ −24 W m−2. This is systematic: decreasing b, i.e., growing particles resulted in a higher SSA and less negative RFE. This means that actually the magnitude of RFE was larger for the darker aerosol, which suggests they would cool the atmosphere more efficiently than the aerosol with the higher SSA. This is due to the size of the particles: small particles scatter light upwards more efficiently than the large ones, which to some extent compensates the darkness of them.
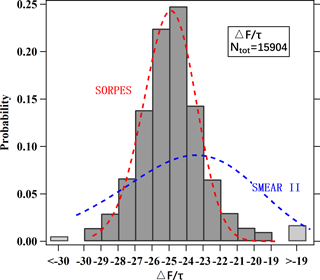
Figure 14Probability distribution of the radiative forcing efficiency at SORPES (red) and at SMEAR II (blue; Virkkula et al., 2011).
The probability distribution of RFE is presented in Fig. 13 and the percentiles of the cumulative distribution of RFE in Table 1. The median RFE = −25.0 W m−2 and the 10 to 90th percentile range from −27.2 to −22.7 W m−2. Again the values are compared with those calculated for the boreal forest site in Finland (Virkkula et al., 2011). At SMEAR II the median RFE = −23.4 W m−2, close but the magnitude is smaller than at SORPES and the 10 to 90th percentile range was from −28.8 to −16.9 W m−2, which is a clearly larger range of values. The main differences between these two sites are (i) that as the magnitude of RFE at SORPES is higher, i.e., the aerosol cools the atmosphere more efficiently and (ii) that the distribution of RFEs are more narrow than at the clean site. This may be due to the above-described relatively constant RFE during the evolution of the pollution episodes, which occurred frequently. This is also in line with the climatology shown by Andrews et al. (2011) according to which the cleanest sites had the widest RFE range.
Aerosol optical properties (AOPs) were measured at SORPES, a regional background station in Nanjing. In this study we have presented basic descriptive statistics, seasonal and diurnal variations, studies of transport, and relationships between the AOPs and some supporting data during the 2-year period from June 2013 to May 2015.
On average, aerosol was highly scattering with an average σsp=403 ± 314 and σap=26 ± 19 Mm−1 and single-scattering albedo 0.93 ± 0.03 for green light. A comparison showed that the SSA of aerosol is slightly higher in Nanjing than that published from most other sites in China and elsewhere. In the comparison, we also included other published data from other inland sites within the YRD region and also there the SSA appears to be higher than at most other sites. This suggests that the concentrations of the condensable vapors that make the aerosol grow may be higher within the source areas of aerosol influencing Nanjing than at the compared sites. However, the presented comparison has several sources of uncertainty: the data from the different sites did not cover equally long periods, the sampling protocols and instruments at the different sites were not similar and the data were processed with different algorithms. To get a reliable comparison of the aerosol at the different locations in China all methods should be harmonized and quality controlled.
The extensive AOPs had typical seasonal cycles with high scattering and absorption coefficients in winter and lower in summer: the averages were σsp=544 ± 422 and σap=36 ± 24 Mm−1 in winter and σsp=342 ± 281 and σap=20 ± 13 Mm−1 in summer. The intensive AOPs had no clear seasonal cycles; the variations in them were rather related to the evolution of pollution episodes. The diurnal cycles of the intensive AOPs were clear. The diurnal cycles of SAE and b suggest that particles were the largest just before the morning peak after which the optically dominating particle size decreased and b grew until afternoon or evening, depending on season. After the peak value, b decreased almost steadily during the night in agreement with the growing GMD, in principle very much like SAE. So the diurnal cycles of SAE and b were consistent with the cycle of the particle number size distribution. The differences in the amplitude of the variations and in the timing during the four seasons were very probably due to the variations in the solar radiation and the consequent gas-to-particle phase transition.
SAE is generally used as a qualitative indicator of dominating particle size with an inverse relationship between SAE and size. For surface mean diameter (SMD) and volume mean diameter (VMD) this was indeed so. The geometric mean diameter (GMD), on the other hand, did not correlate at all with SAE. An explanation for this is that the particle number size distributions are dominated by so small particle sizes that their contribution to light scattering is negligible. All these are consistent with the relationships observed in a completely different environment, the boreal forest at SMEAR II, Finland. All the same conclusions apply to the relationship between the weighted mean diameters and backscatter fraction, b which proved to be a slightly better indicator of the dominating particle size, there was even some – although weak – negative correlation between b and GMD.
The average AAE for the wavelength range 370–950 nm was 1.04 and the AAE range was 0.7–1.4. These AAE values can be explained with different amounts of non-absorbing coating on pure BC cores and different core sizes so the data does not suggest any significant contribution to absorption by brown carbon, which would result in a higher AAE. Note, however, that these values were calculated with a non-wavelength-dependent multiple-scattering correction factor Cref. Algorithms that assume wavelength-dependent Cref would yield higher AAE and would also lead to a conclusion of larger contribution by brown carbon. Comparison of the published AAEs is difficult since some authors have used wavelength-dependent Cref and some not. Since no unambiguous proof of this in either direction has been given, the uncertainty in AAE is high.
The source areas were studied by comparing the AOPs with the local wind, by backward Lagrangian dispersion modeling, and by a back-trajectory cluster analysis. High σspwas observed at all wind directions. By using the observed particle growth rates and local wind, it could be estimated that the center of Nanjing is too close to be the most important contributor to light-scattering particles if they were formed by NPF and subsequent growth within Nanjing. Primary particles, such as BC emitted from traffic, do have time to grow to optically significant sizes also from within Nanjing during weak winds. The comparison of σap with the local wind and with the photochemical age of air masses suggests that high concentrations of light-absorbing aerosol, mainly BC, originated from the urban areas of Nanjing and near-by traffic emissions. For the Lagrangian dispersion modeling, the retroplume analysis and the daily-averaged σsp and σap were used to eliminate the effect of diurnal variations, mainly due to the varying boundary layer height. The result of the retroplume analysis is that the sources of high σsp and σap are within a large area, it is not possible to pinpoint single sources for the high values with this method. The area that is the main contributor to the highest quartile is large. The distance from west to east between the longitudes 115 and 123∘ E at latitude 30∘ N is about 700 km and from south to north between latitudes 29 and 35∘ N about 800 km.
In pollution episodes, the daily maximum PBLH decreased in agreement with the analysis of Petäjä et al. (2016) and Ding et al. (2016a) who showed that high PM and especially BC concentrations enhance the stability of a polluted boundary layer, which in turn decreases the boundary layer height and consequently cause a further increase in PM concentrations. In these episodes, PM concentrations, σsp, and σap typically increased gradually and remained high during several days but decreased faster, sometimes even by more than an order of magnitude within some hours and remained at the lower level for a shorter period than at the higher level. Most of the episodes ended with trajectories associated with the trajectory cluster that brought air from the north, high above Beijing during cold fronts. During the growth phase of the pollution episodes the intensive aerosol optical properties also evolved clearly. The mass scattering efficiency (MSE) of PM2.5 grew during the extended pollution episodes from ∼ 4 to ∼ 6 m2 g−1. In other words, the unit mass of aerosol scattered light more efficiently at the end of the episode than at the beginning. The mass fraction of BCe decreased from ∼ 10 to ∼ 3 % during the growth phase of the episodes. The growth of the particles also clearly lead to the decrease in the Ångström exponents of scattering and absorption (SAE and AAE), the backscatter fraction b, and to the growth of SSA. This further led to a decrease in the magnitude of the radiative forcing efficiency (RFE). In other words, the darker aerosol – the aerosol that had a higher BC mass fraction – had a more negative RFE, i.e., they have the property of cooling the atmosphere more efficiently per unit optical depth than the aerosol with the higher SSA and a lower BC mass fraction. This counterintuitive result is due to the size of the particles: the up-scattered fraction of small, more fresh BC particles is higher than that of larger aged ones, which more than compensated for the darkness of them. The RFE probability distribution at SORPES was clearly more narrow than at a clean background site, which is in agreement with a published RFE climatology.
The GDAS data used in the HYSPLIT calculation can be acquired from ftp://arlftp.arlhq.noaa.gov/pub/archives/gdas1. Measurement data at SORPES, including aerosol data and relevant trace gases as well as meteorological data, are available upon request from the corresponding author before the SORPES database is opened publicly.
The supplement related to this article is available online at: https://doi.org/10.5194/acp-18-5265-2018-supplement.
The authors declare that they have no conflict of interest.
The research was supported by National Key Research & Development Program
of China (2016YFC0202000, 2016YFC0200500), National Science Foundation of
China (D0512/91544231, D0512/41422504), the Jiangsu Provincial Natural
Science Fund (no. BK20140021), and the Academy of Finland's Centre of Excellence
program (Centre of Excellence in Atmospheric Science – From Molecular and
Biological processes to The Global Climate, project no. 272041).
Edited by: Dominick Spracklen
Reviewed by: two anonymous referees
Andreae, M. O., Schmid, O., Yang, H., Chand, D., Yu, J. Z., Zeng, L.-M., and Zhang, Y.-H.: Optical properties and chemical composition of the atmospheric aerosol in urban Guangzhou, China, Atmos. Environ., 42, 6335–6350, 2008.
Andrews, E., Sheridan, P. J., Fiebig, M.,McComiskey, A., Ogren, J. A., Arnott, P., Covert, D., Elleman, R., Gasparini, R., Collins, D., Jonsson, H., Schmid, B., and Wang, J.: Comparison of methods for deriving aerosol asymmetry parameter, J. Geophys. Res., 111, D05S04, https://doi.org/10.1029/2004JD005734, 2006.
Andrews, E., Ogren, J. A., Bonasoni, P., Marinoni, A., Cuevas, E., Rodríguez, S., Sun, J. Y., Jaffe, D. A., Fischer, E. V., Baltensperger, U., and Weingartner, E.: Climatology of aerosol radiative properties in the free troposphere, Atmos. Res., 102, 365–393, 2011.
Arnott, W. P., Hamasha, K., Moosmüller, H., Sheridan, P. J., and Ogren, J. A.: Towards Aerosol Light-Absorption Measurements with a 7-Wavelength Aethalometer: Evaluation with a Photoacoustic Instrument and 3-Wavelength Nephelometer, Aerosol Sci. Tech., 39, 17–29, https://doi.org/10.1080/027868290901972, 2005.
Backman, J., Rizzo, L. V., Hakala, J., Nieminen, T., Manninen, H. E., Morais, F., Aalto, P. P., Siivola, E., Carbone, S., Hillamo, R., Artaxo, P., Virkkula, A., Petäjä, T., and Kulmala, M.: On the diurnal cycle of urban aerosols, black carbon and the occurrence of new particle formation events in springtime São Paulo, Brazil, Atmos. Chem. Phys., 12, 11733–11751, https://doi.org/10.5194/acp-12-11733-2012, 2012.
Baltensperger, U., Barrie, L., Fröhlich, C., Gras, J., Jäger, H., Jennings, S. G., Li, S.-M., Ogren, J. A., Wiedensohler, A., Wehrli, C., and Wilson, J.: WMO/GAW Aerosol Measurement Procedures, Guidelines and Recommendations, WMO/GAW No. 153, 67 pp., 2003.
Bond, T. C. and Bergstrom, R. W.: Light Absorption by Carbonaceous Particles: An Investigative Review, Aerosol Sci. Tech., 40, 27–67, 2006.
Bond, T. C., Doherty, S. J., Fahey, D. W., Forster, P. M., Berntsen, T., DeAngelo, B. J., Flanner, M. G., Ghan, S., Kärcher, B., Koch, D., Kinne, S., Kondo, Y., Quinn, P. K., Sarofim, M. C., Schultz, M. G., Schulz, M., Venkataraman, C., Zhang, H., Zhang, S., Bellouin, N., Guttikunda, S. K., Hopke, P. K., Jacobson, M. Z., Kaiser, J. W., Klimont, Z., Lohmann, U., Schwarz, J. P., Shindell, D., Storelvmo, T., Warren, S. G., and Zender, C. S.: Bounding the role of black carbon in the climate system: A scientific assessment, J. Geophys. Res. Atmos., 118, 1–173, https://doi.org/10.1002/jgrd.50171, 2013.
Cheng, Y. F., Wiedensohler, A., Eichler, H., Su, H., Gnauk, T., Brüggemann, E., Herrmann, H., Heintzenberg, J., Slanina, J., and Tuch, T.: Aerosol optical properties and related chemical apportionment at Xinken in Pearl River Delta of China, Atmos. Environ., 42, 6351–6372, https://doi.org/10.1016/j.atmosenv.2008.02.034, 2008.
Cheng, T., Xu, C., Duan, J., Wang, Y., Leng, C., Tao, J., Che, H., He, Q., Wu, Y., Zhang, R., Li, X., Chen, J., Kong, L., and Yu, X.: Seasonal variation and difference of aerosol optical properties in columnar and surface atmospheres over Shanghai, Atmos. Environ., 123, 315–326, https://doi.org/10.1016/j.atmosenv.2015.05.029, 2015.
Collaud Coen, M., Weingartner, E., Nyeki, S., Cozic, J., Henning, S., Verheggen, B., Gehrig, R., and Baltensperger, U.: Long-term trend analysis of aerosolvariables at the high-alpine site Jungfraujoch, J. Geophys. Res. 112,D13213. https://doi.org/10.1029/2006JD007995, 2007.
Collaud Coen, M., Weingartner, E., Apituley, A., Ceburnis, D., Fierz-Schmidhauser, R., Flentje, H., Henzing, J. S., Jennings, S. G., Moerman, M., Petzold, A., Schmid, O., and Baltensperger, U.: Minimizing light absorption measurement artifacts of the Aethalometer: evaluation of five correction algorithms, Atmos. Meas. Tech., 3, 457–474, https://doi.org/10.5194/amt-3-457-2010, 2010.
Cui, F., Chen, M.,. Ma, Y., Zheng, J., Yao, L., and Zhou, Y.: Optical properties and chemical apportionment of summertime PM2.5 in the suburb of Nanjing, J. Atmos. Chem. 73, 119–135, https://doi.org/10.1007/s10874-015-9313-5, 2016.
Delene, D. J. and Ogren, J. A.: Variability of aerosol optical properties at four North American surface monitoring sites, J. Atmos. Sci. 59, 1135–1150, 2002.
Ding, A. J., Fu, C. B., Yang, X. Q., Sun, J. N., Petäjä, T., Kerminen, V.-M., Wang, T., Xie, Y., Herrmann, E., Zheng, L. F., Nie, W., Liu, Q., Wei, X. L., and Kulmala, M.: Intense atmospheric pollution modifies weather: a case of mixed biomass burning with fossil fuel combustion pollution in eastern China, Atmos. Chem. Phys., 13, 10545–10554, https://doi.org/10.5194/acp-13-10545-2013, 2013a.
Ding, A. J., Fu, C. B., Yang, X. Q., Sun, J. N., Zheng, L. F., Xie, Y. N., Herrmann, E., Nie, W., Petäjä, T., Kerminen, V.-M., and Kulmala, M.: Ozone and fine particle in the western Yangtze River Delta: an overview of 1 yr data at the SORPES station, Atmos. Chem. Phys., 13, 5813–5830, https://doi.org/10.5194/acp-13-5813-2013, 2013b.
Ding, A. J., Wang, T., and Fu, C. B.: Transport characteristics and origins of carbon monoxide and ozone in Hong Kong, South China, J. Geophys. Res., 118, 9475–9488, https://doi.org/10.1002/jgrd.50714, 2013c.
Ding, A. J., Huang, X., Nie, W., Sun, J. N., Kerminen, V. M., Petäjä, T., Su, H., Cheng, Y. F., Yang, X.-Q., Wang, M.H., Chi, X. G.,Wang, J. P., Virkkula, A., Guo, W. D., Yuan, J., Wang, S. Y., Zhang, R. J., Wu,Y.F., Song, Y., Zhu, T., Zilitinkevich, S., Kulmala, M., and Fu., C. B. Enhanced haze pollution by black carbon in megacities in China, Geophys. Res. Lett., 43, https://doi.org/10.1002/2016GL067745, 2016a.
Ding,A., Nie, W., Huang, X., Chi, X., Sun, J., Kerminen, V.-M., Xu, Z., Guo, W., Petäjä, T., Yang, X., Kulmala, M., and Fu, C. B.: Long-term observation of air pollution-weather/climate interactions at the SORPES station: a review and outlook, Front. Environ. Sci. Eng., 10, https://doi.org/10.1007/s11783-016-0877-3, 2016b.
Draxler, R. and Hess, G. An Overview of the HYSPLIT 4 modelling system for trajectories, dispersion and deposition, Aust. Meteorol. Mag., 47, 295–308, 1998.
Garland, R. M., Yang, H., Schmid, O., Rose, D., Nowak, A., Achtert, P., Wiedensohler, A., Takegawa, N., Kita, K., Miyazaki, Y., Kondo, Y., Hu, M., Shao, M., Zeng, L. M., Zhang, Y. H., Andreae, M. O., and Pöschl, U.: Aerosol optical properties in a rural environment near the mega-city Guangzhou, China: implications for regional air pollution, radiative forcing and remote sensing, Atmos. Chem. Phys., 8, 5161–5186, https://doi.org/10.5194/acp-8-5161-2008, 2008.
Garland, R. M., Schmid, O., Nowak, A., Achtert, P., Wiedensohler, A., Gunthe, S. S., Takegawa, N., Kita, K., Kondo, Y., Hu, M., Shao, M., Zeng, L. M., Zhu, T., Andreae, M. O., and Pöschl, U.: Aerosol optical properties observed during Campaign of Air Quality Research in Beijing 2006 (CAREBeijing-2006): Characteristic differences between the inflow and outflow of Beijing city air, J. Geophys. Res., 114, https://doi.org/10.1029/2008jd010780, 2009.
Gyawali, M., Arnott, W. P., Lewis, K., and Moosmüller, H.: In situ aerosol optics in Reno, NV, USA during and after the summer 2008 California wildfires and the influence of absorbing and non-absorbing organic coatings on spectral light absorption, Atmos. Chem. Phys., 9, 8007-8015, https://doi.org/10.5194/acp-9-8007-2009, 2009.
Hand, J. L. and Malm, W. C.: Review of aerosol mass scattering efficiencies from groundbased measurements since 1990, J. Geophys. Res., 112, D16203, https://doi.org/10.1029/2007JD008484, 2007.
Haywood, J. M. and Shine, K. P.: The effect of anthropogenic sulfate and soot aerosol on the clear sky planetary radiation budget, Geophys. Res. Lett., 22, 603–606, 1995.
He, X., Li, C. C., Lau, A. K. H., Deng, Z. Z., Mao, J. T., Wang, M. H., and Liu, X. Y.: An intensive study of aerosol optical properties in Beijing urban area, Atmos. Chem. Phys., 9, 8903–8915, https://doi.org/10.5194/acp-9-8903-2009, 2009.
Hong, S.-Y., Noh, Y., and Dudhia, J.: A new vertical diffusion package with an explicit treatment of entrainment processes, Mon. Weather. Rev., 134, 2318–2341, 2006.
Horvath, H. H., Catalan, L., and Trier, A.: A study of the aerosol of Santiago de Chile. III : Light absorption measurements, Atmos. Environ., 31, 3737–3744, 1997.
Horvath, H., Kasahara, M., Tohno, S., Olmo, F. J., Lyamani, H., Alados-Arboledas, L., Quirantes A., and Cachorro, V.: Relationship between fraction of backscattered light and asymmetry parameter, J. Aerosol Sci., 91, 43–53, 2016.
Huang, R. J., Zhang, Y., Bozzetti, C., Ho, K. F., Cao, J. J., Han, Y., Daellenbach, K. R., Slowik, J. G., Platt, S. M., Canonaco, F., Zotter, P., Wolf, R., Pieber, S. M., Bruns, E. A., Crippa, M., Ciarelli, G., Piazzalunga, A., Schwikowski, M., Abbaszade, G., Schnelle-Kreis, J., Zimmermann, R., An, Z., Szidat, S., Baltensperger, U., El Haddad, I., and Prevot, A. S.: High secondary aerosol contribution to particulate pollution during haze events in China, Nature, 514, 218–222, https://doi.org/10.1038/nature13774, 2014.
IPCC, Climate Change 2013: The Physical Science Basis. Contribution of Working Group I to the Fifth Assessment Report of the Intergovernmental Panel on Climate Change, edited by: Stocker, T., Dahe, Q., and Plattner, G.-K., Cambridge University Press, Cambridge, UK and New York, NY, USA, 2013.
Kim, D.-H., Sohn, B.-J., Nakajima, T., Takamura, T., Takemura, T., Choi, B.-C., and Yoon, S.-C.: Aerosol opterical properties over east Asia determined from ground-based sky radiation measurements, J. Geophys. Res., 109, D02209, https://doi.org/10.1029/2003JD003387,2004.
Kirchstetter, T. W. and Thatcher, T. L.: Contribution of organic carbon to wood smoke particulate matter absorption of solar radiation, Atmos. Chem. Phys., 12, 6067–6072, https://doi.org/10.5194/acp-12-6067-2012, 2012.
Kleinman, L. I., Springston, S. R., Daum, P. H., Lee, Y.-N., Nunnermacker, L. J., Senum, G. I., Wang, J., Weinstein-Lloyd, J., Alexander, M. L., Hubbe, J., Ortega, J., Canagaratna, M. R., and Jayne, J.: The time evolution of aerosol composition over the Mexico City plateau, Atmos. Chem. Phys., 8, 1559–1575, https://doi.org/10.5194/acp-8-1559-2008, 2008.
Kulmala, M., Luoma, K., Virkkula, A., Petäjä, T., Paasonen, P., Kerminen, V.-M., Nie, W., Qi, X., Shen, Y., Chi, X., and Ding, A.: On mode segregated aerosol particle number concentration load: contributions of primary and secondary particles in Hyytiälä and Nanjing, Boreal Env. Res., 21, 319–331, 2016.
Lack, D. A. and Cappa, C. D.: Impact of brown and clear carbon on light absorption enhancement, single scatter albedo and absorption wavelength dependence of black carbon, Atmos. Chem. Phys., 10, 4207–4220, https://doi.org/10.5194/acp-10-4207-2010, 2010.
Lyamani, H., Olmo, F. J., and Alados-Arboledas, L.: Physical and optical properties of aerosols over an urban location in Spain: seasonal and diurnal variability, Atmos. Chem. Phys., 10, 239–254, https://doi.org/10.5194/acp-10-239-2010, 2010.
Ma, N., Zhao, C. S., Nowak, A., Müller, T., Pfeifer, S., Cheng, Y. F., Deng, Z. Z., Liu, P. F., Xu, W. Y., Ran, L., Yan, P., Göbel, T., Hallbauer, E., Mildenberger, K., Henning, S., Yu, J., Chen, L. L., Zhou, X. J., Stratmann, F., and Wiedensohler, A.: Aerosol optical properties in the North China Plain during HaChi campaign: an in-situ optical closure study, Atmos. Chem. Phys., 11, 5959–5973, https://doi.org/10.5194/acp-11-5959-2011, 2011.
Menon, S., Hansen, J., Nazarenko, L., and Luo, Y.: Climate effects of black carbon aerosols in China and India, Science, 297, 2250–2253, 2002.
Müller, T., Laborde, M., Kassell, G., and Wiedensohler, A.: Design and performance of a three-wavelength LED-based total scatter and backscatter integrating nephelometer, Atmos. Meas. Tech., 4, 1291–1303, https://doi.org/10.5194/amt-4-1291-2011, 2011.
Olszyna, K. J., Bailey, E. M., Simonaitis, R., and Meagher, J. F.: O3 and NOy relationships at a rural site, J. Geophys. Res., 99, 14557–14563, 1994.
Petäjä, T., Järvi, L., Kerminen, V.-M., Ding, A. J., Sun, J. N., Nie, W., Kujansuu, J., Virkkula, A., Yang, X., Fu, C. B., Zilitinkevich, S., and Kulmala, M.: Enhanced air pollution via aerosol-boundary layer feedback in China, Sci. Rep., 6, 18998, https://doi.org/10.1038/srep18998, 2016.
Petzold, A., Ogren, J. A., Fiebig, M., Laj, P., Li, S.-M., Baltensperger, U., Holzer-Popp, T., Kinne, S., Pappalardo, G., Sugimoto, N., Wehrli, C., Wiedensohler, A., and Zhang, X.-Y.: Recommendations for reporting “black carbon” measurements, Atmos. Chem. Phys., 13, 8365–8379, https://doi.org/10.5194/acp-13-8365-2013, 2013.
Pitz, M., Cyrys, J., Karg, E., Wiedensohler, A., Wichmann, H. E., and Heinrich, J.: Variability of apparent particle density of an urban aerosol, Environ. Sci. Tech., 37, 4336–4342, 2003.
Qi, X. M., Ding, A. J., Nie, W., Petäjä, T., Kerminen, V.-M., Herrmann, E., Xie, Y. N., Zheng, L. F., Manninen, H., Aalto, P., Sun, J. N., Xu, Z. N., Chi, X. G., Huang, X., Boy, M., Virkkula, A., Yang, X.-Q., Fu, C. B., and Kulmala, M.: Aerosol size distribution and new particle formation in the western Yangtze River Delta of China: 2 years of measurements at the SORPES station, Atmos. Chem. Phys., 15, 12445–12464, https://doi.org/10.5194/acp-15-12445-2015, 2015.
Ramanathan, V., Ramana, M. V., Roberts, G., Kim, D., Corrigan, C., Chung, C. and Winker, D.: Warming trends in Asia amplified by brown cloud solar absorption, Nature, 448, 575–578, 2007.
Rizzo, L. V., Artaxo, P., Müller, T., Wiedensohler, A., Paixão, M., Cirino, G. G., Arana, A., Swietlicki, E., Roldin, P., Fors, E. O., Wiedemann, K. T., Leal, L. S. M., and Kulmala, M.: Long term measurements of aerosol optical properties at a primary forest site in Amazonia, Atmos. Chem. Phys., 13, 2391–2413, https://doi.org/10.5194/acp-13-2391-2013, 2013.
Saarikoski, S., Mäkelä, T., Hillamo, R., Aalto, P., Kerminen, V.-M., and Kulmala, M.: Physico-chemical characterization and mass closure of size-segregated atmospheric aerosols in Hyytiälä, Finland, Boreal Environ. Res., 10, 385–400, 2005.
Sheridan, P. J. and Ogren, J. A.: Observations of the vertical and regional variability of aerosol optical properties over central and eastern North America, J. Geophys. Res., 104, 16793–16805, 1999.
Schmid, O., Artaxo, P., Arnott, W. P., Chand, D., Gatti, L. V., Frank, G. P., Hoffer, A., Schnaiter, M., and Andreae, M. O.: Spectral light absorption by ambient aerosols influenced by biomass burning in the Amazon Basin. I: Comparison and field calibration of absorption measurement techniques, Atmos. Chem. Phys., 6, 3443–3462, https://doi.org/10.5194/acp-6-3443-2006, 2006.
Schnaiter, M., Horvath, H., Möhler, O., Naumann, K. H., Saathoff, H., and Schöck, O. W.: UV-VIS-NIR spectral optical properties of soot and soot-containing aerosols, J. Aerosol Sci., 34, 1421–1444, https://doi.org/10.1016/s0021-8502(03)00361-6, 2003.
Schuster, G. L., Dubovik, O., and Holben, B. N.: Angstrom Exponent and Bimodal Aerosol Size Distributions, J. Geophys. Res., 111, D07207, https://doi.org/10.1029/2005JD006328, 2006.
Schwarz, J. P., Gao, R. S., Spackman, J. R., Watts, L. A., Thomson, D. S., Fahey, D. W., Ryerson, T. B., Peischl, J., Holloway, J. S., Trainer, M., Frost, G. J., Baynard, T., Lack, D. A., de Gouw, J. A., Warneke, C., and Del Negro, L. A.: Measurement of the mixing state, mass, and optical size of individual black carbon particles in urban and biomass burning emissions. Geophys. Res. Lett. 35, L13810, https://doi.org/10.1029/2008GL033968, 2008.
Sherman, J. P., Sheridan, P. J., Ogren, J. A., Andrews, E., Hageman, D., Schmeisser, L., Jefferson, A., and Sharma, S.: A multi-year study of lower tropospheric aerosol variability and systematic relationships from four North American regions, Atmos. Chem. Phys., 15, 12487–12517, https://doi.org/10.5194/acp-15-12487-2015, 2015.
Stein, A. F., Draxler, R. R., Rolph, G. D., Stunder, B. J. B., Cohen, M. D., and Ngan, F.: NOAA's HYSPLIT atmospheric transport and dispersion modeling system, B. Am. Meteorol. Soc., 96, 2059–2077, 2015.
Tang, I.: Chemical and size effects of hygroscopic aerosols on light scattering aerosols, J. Geophys. Res., 101, 19245–19250, 1996.
Tang, L., Yu, H., Ding, A., Zhang, Y., Qin, W., Wang, Z., Chen, W., Hua, Y., and Yang, X.: Regional contribution to PM1 pollution during winter haze in Yangtze River Delta, China, Sci. Tot. Environ., 541, 161–166, 2016.
Tiwari, S., Pandithurai, G., Attri, S. D., Srivastava, A. K., Soni, V. K., Bisht, D. S., Anil Kumar, V., and Srivastava, M. K.: Aerosol optical properties and their relationship with meteorological parameters during wintertime in Delhi, India, Atmos. Res., 153, 465–479, https://doi.org/10.1016/j.atmosres.2014.10.003, 2015.
Virkkula, A., Mäkelä, T., Hillamo, R., Yli-Tuomi, T., Hirsikko, A., Hämeri, K., and Koponen, I. K.: A Simple Procedure for Correcting Loading Effects of Aethalometer Data, J. Air Waste Manage. Assoc., 57, 1214–1222, https://doi.org/10.3155/1047-3289.57.10.1214, 2007.
Virkkula, A., Backman, J., Aalto, P. P., Hulkkonen, M., Riuttanen, L., Nieminen, T., dal Maso, M., Sogacheva, L., de Leeuw, G., and Kulmala, M.: Seasonal cycle, size dependencies, and source analyses of aerosol optical properties at the SMEAR II measurement station in Hyytiälä, Finland, Atmos. Chem. Phys., 11, 4445–4468, https://doi.org/10.5194/acp-11-4445-2011, 2011.
Virkkula, A., Chi, X., Ding, A., Shen, Y., Nie, W., Qi, X., Zheng, L., Huang, X., Xie, Y., Wang, J., Petäjä, T., and Kulmala, M.: On the interpretation of the loading correction of the aethalometer, Atmos. Meas. Tech., 8, 4415–4427, https://doi.org/10.5194/amt-8-4415-2015, 2015.
Wang, J., Virkkula, A., Gao, Y., Lee, S., Shen, Y., Chi, X., Nie, W., Liu, Q., Xu, Z., Huang, X., Wang, T., Cui, L., and Ding, A.: Observations of aerosol optical properties at a coastal site in Hong Kong, South China, Atmos. Chem. Phys., 17, 2653–2671, https://doi.org/10.5194/acp-17-2653-2017, 2017.
Wang, X., Heald, C. L., Sedlacek, A. J., de Sá, S. S., Martin, S. T., Alexander, M. L., Watson, T. B., Aiken, A. C., Springston, S. R., and Artaxo, P.: Deriving brown carbon from multiwavelength absorption measurements: method and application to AERONET and Aethalometer observations, Atmos. Chem. Phys., 16, 12733–12752, https://doi.org/10.5194/acp-16-12733-2016, 2016.
Wang, Z., Huang, X., and Ding, A.: Dome effect of black carbon and its key influencing factors: a one-dimensional modelling study, Atmos. Chem. Phys., 18, 2821–2834, https://doi.org/10.5194/acp-18-2821-2018, 2018.
Weingartner, E., Saathoff, H., Schnaiter, M., Streit, N., Bitnar, B., and Baltensperger, U.: Absorption of light by soot particles: determination of the absorption coefficient by means of aethalometers, J. Aerosol Sci., 34, 1445–1463, https://doi.org/10.1016/s0021-8502(03)00359-8, 2003.
Wilcox, E. M., Thomas, R. M., Praveen, P. S., Piston, K., Bender, F. A.-M., and Ramanathan, V.: Black carbon solar absorption suppresses turbulence in the atmospheric boundary layer, P. Natl. Acad. Sci. USA, 113, 11794–11799, https://doi.org/10.1073/pnas.1525746113, 2016.
Wiscombe, W. J. and Grams, G. W. : The backscattered fraction in two-stream approximations. J. Atmos. Sci., 33, 2440–2451, 1976.
WMO: WMO/GAW Aerosol Measurement Procedures Guidelines and Recommendations 2 Edn., (WMO TD No. 1177) – GAW Report No. 227, World Meteorological Organization, Geneva, 2016.
Wu, D., Mao, J. T., Deng, X. J., Tie, X. X., Zhang, Y. H., Zeng, L. M., Li, F., Tan, H. B., Bi, X. Y., Huang, X. Y., Chen, J., and Deng, T.: Black carbon aerosols and their radiative properties in the Pearl River Delta region, Sci. China Ser. D., 52, 1152–1163, https://doi.org/10.1007/s11430-009-0115-y, 2009.
Wu, Y. F., Zhang, R. J., Pu, Y. F., Zhang, L. M., Ho, K. F., and Fu, C. B.: Aerosol optical properties observed at a semi-arid rural site in northeastern China, Aerosol Air Qual. Res., 12, 503–514, 2012.
Wu, Z. P. and Wang, Y. P.: Electromagnetic scattering for multilaered spheres: recursive algorithms, Radio Sci., 26, 1393–1401, 1991.
Xie, Y., Ding, A., Nie, W., Mao, H., Qi, X., Huang, X., Xu, Z., Kerminen, V.-M., Petäjä, T., Chi, X., Virkkula, A., Boy, M., Xue, L., Guo, J., Sun, J., Yang, X., Kulmala, M., and Fu, C.: Enhanced sulfate formation by nitrogen dioxide: Implications from in situ observations at the SORPES station, J. Geophys. Res.-Atmos., 120, 12679–12694, https://doi.org/10.1002/2015jd023607, 2015.
Xu, J., Bergin, M. H., Yu, X., Liu, G., Zhao, J. Z., Carrico, C., and Baumann, K.: Measurement of aerosol chemical, physical and radiative properties in the Yangtze delta region of China, Atmos. Environ., 36, 161–173, 2002.
Xu, J., Tao, J., Zhang, R., Cheng, T., Leng, C., Chen, J., Huang, G., Li, X., and Zhu, Z.: Measurements of surface aerosol optical properties in winter of Shanghai, Atmos. Res., 109–110, https://doi.org/10.1016/j.atmosres.2012.02.008, 2012.
Yan, P., Tang, J., Huang, J., Mao, J. T., Zhou, X. J., Liu, Q., Wang, Z. F., and Zhou, H. G.: The measurement of aerosol optical properties at a rural site in Northern China, Atmos. Chem. Phys., 8, 2229–2242, https://doi.org/10.5194/acp-8-2229-2008, 2008.
Yang, M., Howell, S. G., Zhuang, J., and Huebert, B. J.: Attribution of aerosol light absorption to black carbon, brown carbon, and dust in China – interpretations of atmospheric measurements during EAST-AIRE, Atmos. Chem. Phys., 9, 2035–2050, https://doi.org/10.5194/acp-9-2035-2009, 2009.
Yu, X., Ma, J., Raghavendra Kumar, K., Zhu, B., An, J., He, J., and Li, M.: Measurement and analysis of surface aerosol optical properties over urban Nanjing in the Chinese Yangtze River Delta, Sci. Tot. Environ., 542, 277–291, https://doi.org/10.1016/j.scitotenv.2015.10.079, 2016.
Zhang, R., Khalizov, A. F., Pagels, J., Zhang, D., Xue, H., and McMurry, P. H.: Variability in morphology, hygroscopicity, and optical properties of soot aerosols during atmospheric processing, P. Natl. Acad. Sci., 105, 10291–10296, 2008.
Zhang, L., Sun, J. Y., Shen, X. J., Zhang, Y. M., Che, H., Ma, Q. L., Zhang, Y. W., Zhang, X. Y., and Ogren, J. A.: Observations of relative humidity effects on aerosol light scattering in the Yangtze River Delta of China, Atmos. Chem. Phys., 15, 8439–8454, https://doi.org/10.5194/acp-15-8439-2015, 2015.
Zhuang, B. L., Wang, T. J., Li, S., Liu, J., Talbot, R., Mao, H. T., Yang, X. Q., Fu, C. B., Yin, C. Q., Zhu, J. L., Che, H. Z., and Zhang, X. Y.: Optical properties and radiative forcing of urban aerosols in Nanjing, China, Atmos. Environ., 83, 43–52, https://doi.org/10.1016/j.atmosenv.2013.10.052, 2014.
Zhuang, B., Wang, T., Liu, J., Li, S., Xie, M., Han, Y., Chen, P., Hu, Q., Yang, X.-Q., Fu, C., and Zhu, J.: The surface aerosol optical properties in the urban area of Nanjing, west Yangtze River Delta, China, Atmos. Chem. Phys., 17, 1143–1160, https://doi.org/10.5194/acp-17-1143-2017, 2017.
Zotter, P., Herich, H., Gysel, M., El-Haddad, I., Zhang, Y., Močnik, G., Hüglin, C., Baltensperger, U., Szidat, S., and Prévôt, A. S. H.: Evaluation of the absorption Ångström exponents for traffic and wood burning in the Aethalometer-based source apportionment using radiocarbon measurements of ambient aerosol, Atmos. Chem. Phys., 17, 4229–4249, https://doi.org/10.5194/acp-17-4229-2017, 2017.