the Creative Commons Attribution 4.0 License.
the Creative Commons Attribution 4.0 License.
Two decades of satellite observations of AOD over mainland China using ATSR-2, AATSR and MODIS/Terra: data set evaluation and large-scale patterns
Larisa Sogacheva
Edith Rodriguez
Konstantinos Kourtidis
Aristeidis K. Georgoulias
Georgia Alexandri
Vassilis Amiridis
Emmanouil Proestakis
Eleni Marinou
Ronald van der A
The retrieval of aerosol properties from satellite observations provides their spatial distribution over a wide area in cloud-free conditions. As such, they complement ground-based measurements by providing information over sparsely instrumented areas, albeit that significant differences may exist in both the type of information obtained and the temporal information from satellite and ground-based observations. In this paper, information from different types of satellite-based instruments is used to provide a 3-D climatology of aerosol properties over mainland China, i.e., vertical profiles of extinction coefficients from the Cloud-Aerosol Lidar with Orthogonal Polarization (CALIOP), a lidar flying aboard the Cloud-Aerosol Lidar and Infrared Pathfinder Satellite Observation (CALIPSO) satellite and the column-integrated extinction (aerosol optical depth – AOD) available from three radiometers: the European Space Agency (ESA)'s Along-Track Scanning Radiometer version 2 (ATSR-2), Advanced Along-Track Scanning Radiometer (AATSR) (together referred to as ATSR) and NASA's Moderate Resolution Imaging Spectroradiometer (MODIS) aboard the Terra satellite, together spanning the period 1995–2015. AOD data are retrieved from ATSR using the ATSR dual view (ADV) v2.31 algorithm, while for MODIS Collection 6 (C6) the AOD data set is used that was obtained from merging the AODs obtained from the dark target (DT) and deep blue (DB) algorithms, further referred to as the DTDB merged AOD product. These data sets are validated and differences are compared using Aerosol Robotic Network (AERONET) version 2 L2.0 AOD data as reference. The results show that, over China, ATSR slightly underestimates the AOD and MODIS slightly overestimates the AOD. Consequently, ATSR AOD is overall lower than that from MODIS, and the difference increases with increasing AOD. The comparison also shows that neither of the ATSR and MODIS AOD data sets is better than the other one everywhere. However, ATSR ADV has limitations over bright surfaces which the MODIS DB was designed for. To allow for comparison of MODIS C6 results with previous analyses where MODIS Collection 5.1 (C5.1) data were used, also the difference between the C6 and C5.1 merged DTDB data sets from MODIS/Terra over China is briefly discussed.
The AOD data sets show strong seasonal differences and the seasonal features vary with latitude and longitude across China. Two-decadal AOD time series, averaged over all of mainland China, are presented and briefly discussed. Using the 17 years of ATSR data as the basis and MODIS/Terra to follow the temporal evolution in recent years when the environmental satellite Envisat was lost requires a comparison of the data sets for the overlapping period to show their complementarity. ATSR precedes the MODIS time series between 1995 and 2000 and shows a distinct increase in the AOD over this period. The two data series show similar variations during the overlapping period between 2000 and 2011, with minima and maxima in the same years. MODIS extends this time series beyond the end of the Envisat period in 2012, showing decreasing AOD.
- Article
(14729 KB) -
Supplement
(104 KB) - BibTeX
- EndNote
An aerosol is a suspension of droplets and/or particles in a fluid (Seinfeld and Pandis, 1997). For atmospheric aerosols, the fluid is the air and the aerosols are generally referred to as particles. This convention will also be followed in this paper. The particles usually consist of solid and/or liquid material, or a mixture of these, depending on their origin and aggregation state, dissolved in liquid (usually water). These particles can be more or less hygroscopic, depending on their chemical composition, and water vapor is released or taken up depending further on the ambient relative humidity (RH), until they are in an equilibrium state. At very high RH, around 100 %, hygroscopic particles are activated to cloud condensation nuclei, grow into the cloud droplet size range and are no longer considered aerosol particles. When they are transported into a lower RH environment, the water evaporates, but the remaining aerosol particle may have a different chemical composition than the original one. The initial chemical composition of an aerosol particle depends on the original generation mechanism, i.e., on the sources such as biomass burning, dust lifted up from the surface or sea spray aerosol generated at the sea surface by the action of wind and waves. Alternatively, aerosol particles are generated from their precursor gases by nucleation under the influence of UV radiation or catalysts (e.g., Kulmala and Kerminen, 2008) after which these very small particles, with a radius of a few nanometers, may grow by condensation and coagulation. In this process, their chemical composition may change, and hence the atmospheric aerosol is a complex mixture of chemical components distributed over a wide range of sizes spanning 5–6 orders of magnitude from a few nanometers to tens of micrometers and a wide range of concentrations spanning about 10 orders of magnitude, depending on the particle size. Aerosol particles are important because of their effects on climate (e.g., Rosenfeld et al., 2008; Koren et al., 2014; Guo et al., 2016a), health (Pope et al., 2009; Anenberg et al., 2010), atmospheric chemistry, visibility (Sisler and Malm, 1994), cultural heritage, etc.
In this paper, we focus on satellite retrieval of aerosol properties, which requires that the particles are optically active; i.e., their size is of the same order of magnitude as the wavelength of the incident light. The radiometers which are commonly used for aerosol measurements from space, making use of the Earth-reflected solar radiation at the top of the atmosphere (TOA), are not sensitive to particles smaller than about 100 nm due to their low scattering efficiency at wavelengths in the UV–visible (UV–VIS) part of the electromagnetic spectrum (Sundström et al., 2015). Very large particles, larger than several tens of micrometers, occur in very low concentrations and therefore contribute little to the radiance measured at TOA. Hence, the particles observed from satellites, during clear-sky conditions, are in the size range of about 100 nm to several tens of micrometers and thus do not include newly formed particles (Sundström et al., 2015), unless proxies are used (Kulmala et al., 2011; Sundström et al., 2015). On the other hand, cloud droplets with sizes on the order of 10 µm do affect the thermal infrared (TIR) radiances, and this is used for cloud detection.
China, with its large variability of aerosol concentrations and a wide range of emissions of both different types of aerosol particles and precursor gases as well as different climate regions and meteorological conditions, offers unique opportunities to study aerosols. Many studies have been published on aerosols in relation to air quality in the eastern part of China, including satellite remote sensing, ground-based measurements, modeling and combinations thereof, which often focus on local or regional aspects (e.g., Song et al., 2009; S. Wang et al., 2011; Ma et al., 2016; Zou et al., 2017; Xue et al., 2017; Miao et al., 2017; Guo et al., 2017). Satellites offer the opportunity to obtain information, using the same instruments and methods, over a large area during a longer period of time. In addition, using a lidar such as the Cloud-Aerosol Lidar with Orthogonal Polarization (CALIOP) flying aboard the Cloud-Aerosol Lidar and Infrared Pathfinder Satellite Observation (CALIPSO) (Winker et al., 2007), complementary information can be obtained on the aerosol vertical structure (Winker et al., 2009), and hence a 3-D aerosol climatology can be developed (Winker et al., 2013). CALIOP also provides information on aerosol type (Omar et al., 2009).
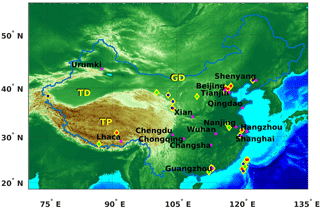
Figure 1The study area is mainland China, i.e., the area within the Chinese border, indicated in this elevation map by the blue line. Also indicated are some major cities (purple dots) and the locations of the Aerosol Robotic Network (AERONET) sites (yellow diamonds) used in this study for validation. The color of the dot inside each diamond gives an indication of the length of the data record at that site (red: years, green: months, blue: days). Areas mentioned in this paper are the Taklamakan Desert (TD), Gobi Desert (GD) and Tibetan Plateau (TP). Other areas are the Beijing–Tianjin–Hebei (BTH) area, the Yangtze River Delta (YRD) including Shanghai and Nanjing, the Pearl River Delta in the south including Guangzhou and the North China Plain (NCP) including BTH, YRD and the area in between.
The objective of the current study is to present the aerosol spatial and temporal distribution over mainland China, using two decades of satellite observations. The focus is on the use of the European Space Agency (ESA) Along-Track Scanning Radiometer (ATSR), i.e., on ATSR-2 which flew on the European Remote Sensing satellite ERS-2 and provided data from 1995 to 2003, and the Advanced ATSR (AATSR) which flew on the environmental satellite Envisat from 2002 to April 2012 when contact with the satellite was lost and its mission ended. Hence, a period of 17 years of ATSR data is available. With the focus on aerosols over land, the aerosol optical depth (AOD) at a wavelength of 550 nm (AOD550, from here on referred to as AOD, unless specified otherwise) retrieved using the ATSR dual view algorithm (ADV; Kolmonen et al., 2016; Sogacheva et al., 2017) was used. This data set is further extended to 2015 by using AOD data available from Moderate Resolution Imaging Spectroradiometer (MODIS) aboard the Terra satellite, Collection 6 (C6) (Levy et al., 2013). CALIOP data, available from 2007, were used to obtain information on the vertical distribution of the AOD (at 532 nm) over part of the study area. Thus, a 3-D aerosol climatology over China was obtained, and the time dimension was added by evaluating a two-decadal time series by combining ATSR and MODIS data spanning the period 1995–2015. The study encompasses the area between 18–54∘ N and 73–135∘ E (see Fig. 1), but the discussion focuses on AOD and vertical extinction profiles over China, i.e., the area over land within the Chinese border indicated by the blue line.
The ATSR dual view offers the opportunity to effectively separate the contributions of the surface and atmospheric reflections to the total reflection measured at TOA and thus retrieve aerosol properties independent of a surface correction, assuming that the ratio of the surface reflections in the forward and nadir views is independent of wavelength (Veefkind et al., 1998; Kolmonen et al., 2016). However, that approach may fail over very bright surfaces such as snow, ice or some desert areas. ATSR AOD data retrieved using the ADV algorithm have been successfully applied in many studies (e.g., Veefkind et al., 1998, 1999, 2000; Robles-Gonzalez et al., 2000, 2003, 2006, 2008; Schmid et al., 2003; Sundström et al., 2012; Holzer-Popp et al., 2013; Virtanen et al., 2014; de Leeuw et al., 2015; Rodriguez et al., 2015; Sogacheva et al., 2015, 2017; Popp et al., 2016). By combining the ATSR-2 and AATSR data sets, a unique time series of AOD over land, from June 1995 to April 2012, offers the opportunity to analyze the temporal variation of the AOD and possibly detect trends. However, the most recent changes in response to emission regulations in China (e.g., van der A et al., 2017) cannot be observed and the analysis of the ATSR time series remains inconclusive. Therefore, this time series has been extended with MODIS/Terra C6 AOD data obtained from the dark target (DT) and deep blue (DB) algorithms and merged into a single data set referred to as DTDB. The MODIS/Terra AOD data set was selected because of the proximity of the overpass times of the ERS-2/Envisat and Terra satellites over China within about 1 h. MODIS/Terra data are available from April 2000. Furthermore, the most recent MODIS C6 data have been selected because of updates described by Levy et al. (2013), with further specification for DB updates by Hsu et al. (2013) and expanded description and evaluation of DTDB by Sayer et al. (2014). To use these data sets together requires an evaluation of their similarities and differences across the study area, where it is possible to include an independent reference data set like the one provided by the Aerosol Robotic Network (AERONET; Holben et al., 1998), CARSNET (Che et al., 2015; Che. et al., 2016) or SONET (Li et al., 2017).
Earlier studies on the aerosol climatology and trends over China were made using ground-based remote sensing, i.e., sun photometers in CARSNET (Che et al., 2015), hand-held sun photometers in the Chinese Sun Hazemeter Network (CSHNET; Y. Wang et al., 2011) and solar radiation measurements (e.g., X. Xu et al., 2015), or satellite data, in particular MODIS (e.g., Li et al., 2003; Song et al., 2009; S. Wang et al., 2011; Luo et al., 2014; Tan et al., 2015; H. Xu et al., 2015; Ma et al., 2016; He et al., 2016) but also using multiple satellite data (Lin et al., 2010; Guo et al., 2011, 2016b; Dong et al., 2017; Zhao et al., 2017; Zhang et al., 2017). However, these studies used MODIS C5.1 AOD data and substantial differences exist between C6 and C5.1 (e.g., Levy et al., 2013; Sayer et al., 2014; Tao et al., 2015; Xiao et al., 2016). Furthermore, in addition to data sets over dark and brighter surfaces from the DT and DB algorithms, respectively, C6 also provides a merged DTDB data set based on criteria using the quality flags in each product (Sayer et al., 2013). The merged DTDB data set offers better coverage but at the expense of a slight decrease in accuracy (Tao et al., 2015). Direct and systematic comparisons between C5.1 and C6 AOD over China have been published for the individual DT and DB data but not for the merged DTDB AOD. This task is undertaken here to support the comparison of the results from the current study with those from previous work.
In this study, the spatial distribution of AOD over China is presented and discussed as well as the vertical distribution of the aerosol extinction coefficients and AOD inferred from CALIOP data. Seasonal variations of AOD are presented and briefly discussed, as well as the two-decade time series (1995–2015) of AOD. In a second paper (Sogacheva et al., 2018), the seasonal and long-term variations for different regions in China will be presented.
Different data sources are used in this study, including satellite and ground-based observations. Satellite data sets include AOD retrieved from ATSR and MODIS/Terra, and vertical profiles obtained from CALIOP. Independent and accurate ground-based data sets are used as reference for validation and evaluation. The study encompasses the area shown in Fig. 1, with a focus on mainland China.
2.1 Satellite data sets
2.1.1 ATSR (ATSR-2 and AATSR)
ATSR is a dual view instrument (near nadir and 55∘ forward). The two views facilitate effective separation of surface and atmospheric contributions to the observed upwelling radiances, as applied over land in the ATSR ADV algorithm (Kolmonen et al., 2016). Multiple wavelengths (seven) from VIS to TIR facilitate effective cloud screening and allow for multi-wavelength retrieval of aerosol properties. ATSR has a conical scan mechanism with a swath of 512 km, resulting in daily global coverage of 5–6 days. Data are provided with a nominal resolution of 1 × 1 km2; sub-nadir and aerosol data are provided at a default spatial resolution of 10 × 10 km2 on a sinusoidal grid (L2) and at 1∘ × 1∘ (L3).
ATSR-2 flew aboard ESA's ERS-2 from 1995 to 2003. AATSR flew on ESA's environmental satellite Envisat and provided data from May 2002 to April 2012. Both satellites flew in a Sun-synchronous descending orbit with a daytime Equator crossing time of 10:30 LT (ERS-2) and 10:00 LT (Envisat). Together, these instruments provided 17 years of global aerosol data. This time series is planned to be continued with a similar instrument on Sentinel-3, i.e., the Sea and Land Surface Temperature Radiometer (SLSTR), launched in the spring of 2016. A detailed description of the AATSR data processing, using the ADV algorithm over land and the ATSR single view (ASV) algorithm over the ocean, is provided in Kolmonen et al. (2016).
The ATSR product used in this paper is the aerosol optical depth at a wavelength of 550 nm (hereafter referred to as AOD) over the study area for the full ATSR mission. The data were produced using ADV version 2.31 which includes cloud postprocessing as described in Sogacheva et al. (2017).
2.1.2 MODIS
The MODIS sensor (Salomonson et al., 1989) aboard NASA's Terra satellite has been flying in a near-polar Sun-synchronous circular orbit for more than 15 years (launched on 18 December 1999), observing the Earth–atmosphere system. MODIS/Terra has a daytime Equator crossing time at 10:30 LT (descending orbit), a viewing swath of 2330 km (cross track) and provides near-global coverage on a daily basis. One of the most successful products of MODIS, which has been used in numerous aerosol-related studies, is the aerosol optical depth at 550 nm (hereafter referred to as AOD).
MODIS AOD is retrieved using two separate algorithms, DT and DB. In fact, two different DT algorithms are utilized: one for retrieval over land (vegetated and dark-soiled) surfaces (Kaufman et al., 1997; Remer et al., 2005; Levy et al., 2010, 2013) and one for retrieval over water surfaces (Tanré et al., 1997; Remer et al., 2005; Levy et al., 2013). The DB algorithm (Hsu et al., 2004, 2013) was traditionally used over bright surfaces where DT cannot be used (e.g., deserts, arid and semi-arid areas). However, the enhanced DB algorithm, which is used in C6, is capable of returning aerosol measurements over all land types (Sayer et al., 2013, 2014). The C6 DT expected error is ±(0.05 + 0.15τAERONET) over land and +(0.04 + 0.1τAERONET), −(0.02 + 0.1τAERONET) over sea relative to the AERONET optical thickness (τAERONET) (Levy et al., 2013). The C6 DB expected error is ∼ ±0.03 + 0.2τMODIS) relative to the MODIS optical thickness (τMODIS) (Hsu et al., 2013; Sayer et al., 2015).
Several changes have been made in C6 compared to C5.1. Details about these updates in C6 DT and DB data can be found in a number of recent studies (e.g., Levy et al., 2013; Tao et al., 2015; Sayer et al., 2015; Georgoulias et al., 2016). Corrections for polarization, gain and response-versus-scan corrections and detrending for MODIS/Terra degradation have been included in DB but not in DT. Another important update in C6 is the inclusion of a merged (DT and DB) data set as described in Levy et al. (2013). This includes measurements from both algorithms, offers a better spatial coverage and can be used in quantitative scientific applications (Sayer et al., 2014). In this work, the merged C6 L3 MODIS/Terra (MOD08_M3) monthly 1∘ × 1∘ gridded AOD data set is used for the period March 2000–December 2015.
2.1.3 CALIOP
LIght Detection And Ranging (lidar) is a powerful remote sensing technique for obtaining information related to the vertical distribution of aerosols in the atmosphere (Liu et al., 2002). On a global scale, lidar data are acquired by CALIOP which is the primary instrument aboard the CALIPSO satellite (Winker et al., 2007). CALIPSO, developed as a collaboration project between NASA and the space agency of France (CNES) has provided altitude-resolved profiles of aerosols and clouds since June 2006. In addition to the total attenuated backscatter signal at two wavelengths (532 and 1064 nm), CALIOP is capable of acquiring polarization measurements at 532 nm. Since the particle depolarization ratio is considered as the fingerprint of desert dust particles (Ansmann et al., 2003; Liu et al., 2008), CALIOP is an ideal instrument for studies related to the three-dimensional distribution and transport of dust in the atmosphere (Amiridis et al., 2013; Proestakis et al., 2018).
CALIPSO joined the A-Train constellation of satellites in April 2006 (Winker et al., 2007). Being an integral part of the A-Train formation, CALIPSO is in a Sun-synchronous polar orbit, with a local Equator crossing time at 13:30 LT and an orbit repetition frequency of approximately 16 days. Aboard CALIPSO, the primary instrument is CALIOP, a dual-wavelength and dual-polarization elastic backscatter Nd:YAG lidar (Hunt et al., 2009). CALIOP transmits linearly polarized pulses at 532 and 1064 nm, while a telescope of 1 m diameter collects the backscatter signals. Based on the 532 and 1064 nm total backscatter signals and on the parallel and perpendicular polarization components of the 532 nm backscatter signal, CALIOP provides global and continuous information on the vertical distribution of aerosols and clouds (Winker et al., 2009). The product of CALIOP is provided in different levels of processing. Here, we use the L2 product, which provides height-resolved information of aerosol and cloud backscatter and linear depolarization ratio along the CALIPSO track. Based on a number of parameters, namely the magnitude of the attenuated backscatter, the cross-to-total ratio of the attenuated backscatter signals, the altitude of the detected layers and the surface characteristics along the CALIPSO orbit, the CALIPSO algorithm classifies the detected atmospheric feature types into subtypes (Omar et al., 2009). In the case of aerosols, the algorithm assigns aerosol-dependent lidar ratios (LRs) to the different subtypes in order to convert the L2 backscatter coefficient profiles into profiles of extinction coefficient (Young and Vaughan, 2009). In this paper, the ESA-LIVAS (“LIdar climatology of Vertical Aerosol Structure for space-based lidar simulation studies” project) database is used. LIVAS is developed based on CALIPSO v3 L2 Aerosol and Cloud Profile products towards a global multi-wavelength (355, 532, 1064, 1570 and 2050 nm) aerosol and cloud optical database on a uniform 1∘ × 1∘ grid resolution (Amiridis et al., 2015). Here, the CALIPSO-based ESA-LIVAS product (via http://lidar.space.noa.gr:8080/livas/) is used to provide the three-dimensional climatology of the aerosol distribution over China for the period January 2007–December 2015.
2.2 Ground-based reference data: AERONET
For the validation of satellite-retrieved aerosol products, AERONET sun photometer data (Holben et al., 1998) are most commonly used as an independent data source which are publicly available at the AERONET website (http://aeronet.gsfc.nasa.gov/). An extensive description of the AERONET sites, procedures and data provided is available from this website. Ground-based sun photometers provide accurate measurements of AOD (uncertainty ∼ 0.01–0.02, Eck et al., 1999) because they directly observe the attenuation of solar radiation without interference from land surface reflections. The parameter used in this study is version 2 L2.0 (cloud screened and quality assured; Smirnov et al., 2000) AOD at 550 nm, obtained from interpolation between AOD retrieved at 440 and 675 nm using the Ångström exponent. The locations of the AERONET sites used in this study are indicated in Fig. 1, and their coordinates and periods for which data are available are listed in Table S1 in the Supplement.
3.1 ATSR-retrieved AOD for 1995–2012 using ADV v2.31
ATSR-2-retrieved AOD data are available for the period June 1995–December 2003, with some gaps in 1995 and 1996, and also toward the end the data were not reliable. Hence, in this study, ATSR-2 data are only used until August 2002. AATSR data are available for the period May 2002–April 2012, but some data are missing in 2002, and therefore we use these data only from August 2002 on. The consistency between the ATSR-2 and AATSR data sets has been discussed in Popp et al. (2016). Over the ocean, significant differences are observed with ATSR-2 consistently somewhat higher, whereas over land there is no significant shift in AOD from ATSR-2 to AATSR. In the current study, we only use data over land. Years for which data are not continuously available for operational purposes are not shown in multi-year averaged maps or aggregates. Here, the term aggregate is used instead of average because of missing data for, e.g., cloudy situations, bright surfaces and other situations where a successful retrieval was not achieved. Furthermore, satellite data are biased toward clear-sky situations, and hence no information is available for cloudy or partly cloudy scenes. In addition, satellite observations offer a snapshot during the overpass at a certain time of the day and, in the case of ATSR with a limited swath width, data are available only every 3–5 days, depending on latitude. Lacking information on the AOD for other days, the data cannot represent a true average.
A map showing the spatial distribution of the ATSR-retrieved AOD over China, aggregated for the full years 2000–2011, is shown in Fig. 2. This period was selected to allow for comparison with MODIS/Terra. Differences with the aggregated AOD map for the ATSR full-mission period (1995–2012) are very small (not shown). It is clear that in such aggregate the absolute AOD value may not be representative for the actual value in a certain area because systematic temporal and year-to-year variations are hidden in the process. In the aggregation process, all pixels retrieved and quality controlled were used to provide monthly averages and these in turn were used to aggregate to full years. Temporal variations will be discussed below based on time series.
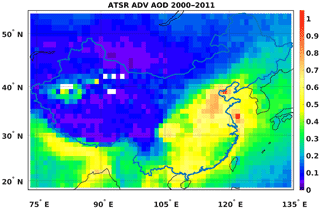
Figure 2Spatial distribution of the AOD over China, aggregated over the (full) years 2000–2011. The AOD has been retrieved from ATSR-2 and AATSR data, using the ADV v2.31 algorithm. The AOD scale is presented in the color bar to the right of the map. Areas for which no data are available are shown in white.
The map in Fig. 2 shows the commonly reported high AOD over southeast (SE) and southwest (SW) China with the highest values (on the order of 0.8) over the North China Plain (NCP) and the Sichuan province. Also, south of the Himalayas the AOD is high, with moderately high AOD over the area east of the Himalayas and SW China. Northwest (NW) of the NCP, the AOD is moderate with values around 0.3. In most other areas, the AOD is low with values of 0.15 and smaller. In the west, over the Taklamakan Desert, the multi-year aggregated AOD is high, due to the presence of wind-blown desert dust in the spring. However, the highest values are not observed over the bright desert surface where the ADV retrieval was not achieved and thus no data are available (white area). Along the Chinese coast, the AOD is high (on the order of 0.5), with overall smooth land–sea transitions, and it decreases toward the open ocean. Likely, the high AOD is due to a combination of transport from land and ship emissions along this very busy shipping route. A very high AOD area is observed at about 34∘ N, 122∘ E, which also occurs in the MODIS data (see below) as well as in Ozone Monitoring Instrument (OMI)-retrieved NO2 column data (Ding et al., 2017). Likely, ship emissions are also the reason for the AOD hotspot at 38∘ N, 119∘ E where NO2 concentrations are also high.
It must be kept in mind that these are 12-year aggregated values and strong deviations may occur in certain years or seasons.
3.2 MODIS/Terra 2000–2015: C6 merged DTDB AOD
The MODIS AOD used in this study is the MODIS/Terra C6 L3 merged DTDB AOD product for the period April 2000 to December 2015. Here, MODIS/Terra has been chosen because of the morning orbit with an Equator overpass (descending) time at 10:30 LT, i.e., close to the ATSR Equator overpass times (ATSR-2 at 10:30 LT; AATSR at 10:00 LT), which allows for comparison of the data over China within about 1 h. It is noted that the drift in the MODIS/Terra blue channel has been corrected from C5.1 to C6 (Levy et al., 2013).
The spatial distribution of the MODIS/Terra DTDB AOD for the period 2000–2011 is presented in Fig. 3. The overall AOD distribution is similar to that presented in Fig. 2 for ATSR but with some noticeable differences. The main features in Fig. 3 are the higher AOD provided by MODIS, as compared to ATSR, over almost the whole study area, as well as the much higher AOD over most of the Tibetan Plateau and the Taklamakan Desert. Obviously, the latter is due to the use of the DB algorithm over the bright areas in west China where DT provides hardly any AOD data (see also Tao et al., 2015), but also the ADV-retrieved AOD is very low (over the Tibetan Plateau) or not available due the bright surface. The AOD spatial pattern over SE and north China is similar for MODIS and ATSR, with MODIS AOD higher, and the same is observed over northern India. In contrast, the AOD retrieved by MODIS over Vietnam and Laos is lower than that retrieved using ATSR data. The differences between ATSR and MODIS will be further addressed in Sect. 4.
3.2.1 MODIS C5.1 vs. C6
Many studies on the AOD over China have been published, as mentioned in the introduction. These studies are relevant for comparison with the current study with regard to both the spatial resolution and the temporal behavior. In view of the rather recent production of the MODIS C6 data, most of the earlier studies used C5.1. Levy et al. (2013) made an initial comparison between C6 and C5.1 for 4 months of MODIS/Aqua AOD data showing that the C6–C5.1 AOD difference is smaller than 0.1. Levy et al. (2013) also compared MODIS/Terra aggregated 1∘ × 1∘ AOD for 1 month (July 2008) and noted an extra C6–C5 difference over land in the MODIS/Terra data. Their Fig. 22 shows that over China the Terra C6–C5 difference is larger than 0.1.
Sayer et al. (2014) did not specifically address the AOD over China although these authors noted that in C6 DB AOD tends to be lower than DT in the high-AOD region of China throughout the year. They also concluded that the merged product does not specifically outperform the DT or DB results. The Terra C6 3 km AOD product was validated by Xiao et al. (2016) using AERONET data from the DRAGON Asia campaign (2012–2013) (Holben et al., 2017) and over Beijing using hand-held sun photometers.
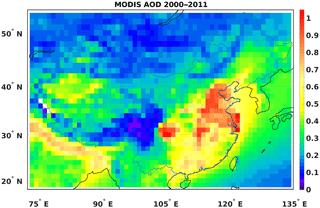
Figure 3Spatial distribution of the MODIS/Terra C6 merged DTDB AOD over China, aggregated for the full years 2000–2011.
Tao et al. (2015) evaluated MODIS/Aqua C6 AOD over different regions in China for both DB and DT products, but not for the merged DTDB AOD, using AERONET data as reference. One handicap is the sparsity of AERONET stations and their spatial distribution across China and another one is the length of operation of each station. Tao et al. considered five different regions, which are indicated as northern China, the Yangtze River Delta (YRD), southern China, northwestern China and scattered arid areas. It is noted that most AERONET stations are concentrated in the northern China and YRD regions. The validation results show the good performance of DB for the northern sites, whereas over all other sites DB underestimates the AOD. DT overestimates the AOD over almost all AERONET sites where sufficient quality retrievals are available to allow for proper evaluation. Seasonal mean DT AOD over eastern China may be 0.3–0.4 higher than DB. Tao et al. also note that DT misses haze periods with high AOD and further comment on the use of the normalized difference vegetation index (NDVI) in the merging procedure.
In view of the regional differences in the behavior of DB and DT, the regional quality of the merged product cannot be assessed a priori and depends on whether the DB or DT product has been selected, and when both are used, on their actual values in the merging process. The merged DTDB AOD from both Terra and Aqua were evaluated by Zhang et al. (2016) for two CSHNET (Xin et al., 2007) sites near Beijing, i.e., the city of Beijing and a rural mountain site west of Beijing (Beijing Forest). The results show the good performance of the merged DTDB AOD product although it is not better than any of the individual DT or DB products in all cases. At the rural site, DTDB performs similar to DT but better than DB, as compared to the CSHNET AOD, with a slight underestimation of the daily product over the rural site. Over the urban site, the DB product performs somewhat better than DTDB, with a slight overestimation of the latter.
In this study, a C5.1 merged DTDB AOD product has been produced over China following the procedure described in Levy et al. (2013). The difference between the C6 and C5.1 merged products is presented in Fig. 4. Figure 4 clearly shows the higher C6 AOD over most of eastern China as well as the Tibetan Plateau with the largest differences, up to 0.2, over the northeast (NE) of the NCP and Sichuan province and the western part of the Tibetan Plateau. On the other hand, C6 is lower over the lower part of western China and in particular the Taklamakan Desert where local differences are observed of −0.25. These differences need to be taken into account when comparing the results from using C6, as used in the current paper, with those in earlier papers using C5.1 data.
3.3 CALIOP 2007–2015: the three-dimensional distribution of aerosols
In addition to the presented and discussed horizontal variability of ATSR and MODIS columnar properties, CALIOP observations are synergistically used in this study, in order to provide information on the vertical distribution of aerosols over China. The vertical distribution of aerosols in the atmosphere greatly affects aerosol–cloud interaction (DeMott et al., 2009; Hatch et al., 2008) and is critical to estimations of the aerosol direct and indirect radiative forcing on climate (Haywood and Boucher, 2000), to human health and degradation of air quality (Goudie, 2014).
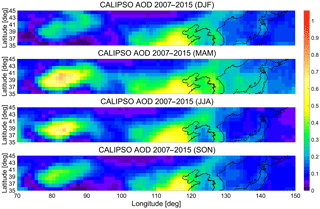
Figure 5Seasonal maps of AOD at 532 nm derived from CALIOP over the area 35–45∘ N, 70–150∘ E, including the Taklamakan/Gobi deserts and the Beijing–Tianjin–Hebei area, derived from 9 years of CALIPSO overpasses (2007–2015): winter (DJF), spring (MAM), summer (JJA) and autumn (SON).
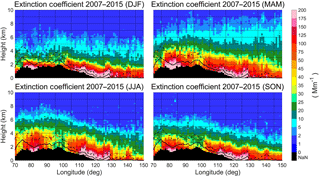
Figure 6Vertical distribution of the climatological extinction coefficient profiles at 532 nm over the area 35–45∘ N, 70–150∘ E, including the Taklamakan Desert and the Beijing–Tianjin–Hebei area, derived from 9 years of CALIOP measurements (2007–2015): winter (DJF), spring (MAM), summer (JJA) and autumn (SON). The solid and broken lines indicate the mean and maximum elevation of the aerosol extinction above the surface.
The horizontal variability of the CALIOP-derived AOD at 532 nm is shown in Fig. 5 for the domain 35–45∘ N and 70–150∘ E, for winter (DJF), spring (MAM), summer (JJA) and autumn (SON), where the data for each season have been averaged over the years 2007–2015. The vertical distribution of aerosols over the same domain (35–45∘ N, 55–155∘ E) and for the same seasons is presented through the climatological extinction coefficient profiles at 532 nm (Fig. 6). Through the combination of the vertical dimension (Fig. 6) with the horizontal AOD distribution (Fig. 5) the full 3-D overview of atmospheric aerosols over this domain is provided. Similar figures for other areas, encompassing SE Asia (5–55∘ N, 65–155∘ E) are provided in Proestakis et al. (2018).
The domain shown in Fig. 5 encompasses the Taklamakan and Gobi deserts and the densely populated Beijing–Tianjin–Hebei (BTH) area. Over this domain, similar patterns of AOD are observed throughout the year, with their intensity varying strongly with the seasons, especially over the dust sources. The Taklamakan/Gobi deserts and the BTH area are clearly mapped through the high AOD values. Large mean AOD values, of the order of 0.3–0.8, are observed over the arid region of the Taklamakan Desert/Tarim Basin and the BTH area, while the semi-arid Gobi Desert yields significantly lower mean AOD values, of the order of 0.1–0.3. Over the Taklamakan Desert, the highest AOD values, of the order of 0.5–0.8, are observed during MAM and JJA, while during the period between September and February AOD is much lower (∼ 0.3). The seasonal variation over the Gobi Desert is similar to that over the Taklamakan Desert, but AOD values are substantially lower, even at its maximum activity (< 0.3). The observed seasonality and variability of the AOD over the Taklamakan/Gobi deserts and the high AOD values observed during MAM over the Taklamakan Desert are strongly related to the activation mechanisms of the dust sources (Prospero et al., 2002), the local topography of the Tarim Basin (Yumimoto et al., 2009) and the cyclonic systems developed over Mongolia (Sun et al., 2001). Downwind from the Taklamakan and Gobi dust sources, the anthropogenic activity in the densely populated and highly industrialized BTH area results in a consistently high average AOD which is present throughout the year. Similar AOD features are observed between all four seasons with larger AOD values, of the order of 0.7, during JJA and lower AOD values, of the order of 0.5, during SON.
The CALIOP-derived 9-year averaged vertical distribution of the climatological extinction coefficients over the same domain as discussed above is shown in Fig. 6 for each of the four seasons in the period 2007–2015. Over the Taklamakan Desert and the BTH area, similar climatological extinction coefficient features are observed close to the surface, with values as high as 200 Mm−1 persistently present throughout the year. Over the vast semi-arid Gobi Desert, though, the near-surface climatological extinction coefficient values are significantly lower (∼ 50 Mm−1). The extinction coefficient values are altitude dependent and distinctly decrease with height. Over BTH and eastwards (110–130∘ E), high extinction coefficient values (∼ 200 Mm−1) are in general suppressed below 1 (above sea level), while over the Taklamakan Desert (77–86∘ E) high extinction coefficient values of the order of 200 Mm−1 are observed as high as 3 . The eastward long-range transport of dust aerosols generated from the Taklamakan and Gobi deserts is also evident. Over the BTH area, high dust-related extinction coefficient values (∼ 125 Mm−1, gradually decreasing with height) are observed at altitudes higher than 3 . This suggestion is supported by the areas of high extinction values (Taklamakan, about 200 Mm−1; Gobi, about 75 Mm−1) located to the west of BTH. Dust extinction coefficient profiles (Proestakis et al., 2018) suggest that part of the transported aerosol is dust, with a large contribution of locally produced aerosols over BTH. Over the whole region, the extinction decreases gradually with height above the surface up to an altitude of about 8 km, where the extinction coefficient has decreased to ∼ 10 Mm−1. However, the height of the layer gradually decreases toward the east, following the surface elevation to some degree, but also with gradually increasing layer depth. The synergy of the horizontal (Fig. 5) and vertical (Fig. 6) distributions allows for the simultaneous study of the emission sources (Taklamakan/Gobi deserts, BTH area), the aerosol load and the corresponding injection height of dust aerosols in the atmosphere.
The data overview presented above shows the differences between the AOD derived from ATSR, MODIS and CALIOP data. To evaluate the quality of the ATSR- and MODIS-retrieved AOD, these products are compared with reference AOD data available from AERONET sites in the study area (see Fig. 1 and Table S1 in the Supplement for locations). For this comparison, collocated data are used; i.e., satellite data within a circle with a radius of 0.125∘ around the AERONET site are averaged and compared with averaged AERONET data measured within ±1 h of the satellite overpass time.
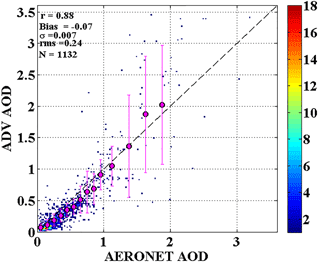
Figure 7Density scatterplot of ATSR-retrieved AOD, using ADV v2.31, over China for the years 2002–2012 vs. AOD from AERONET stations in mainland China (see Fig. 1 and Table S1 in the Supplement). The filled circles are the averaged ATSR AOD binned in 0.1 AERONET AOD intervals (0.2 for AERONET AOD > 1.0) and the vertical lines on each circle represent the 1σ SD of the fits. Statistics in the upper left corner indicate correlation coefficient r, bias, SD, root mean square (rms) error and number of data points (N). The color bar on the right indicates the number of data points.
4.1 ATSR
AATSR AOD data sets over China produced by three different algorithms, including ADV, have been validated by Che et al. (2016) using as reference the AOD data from selected AERONET and CARSNET (Che et al., 2015) sites for the years 2007, 2008 and 2010. The results show that the AATSR-retrieved AOD is underestimated by a factor which increases with increasing AOD. However, Che et al. (2016) likely used an older version of the ADV-retrieved data set than the one produced by v2.31 which is used in the current work. The v2.31 AOD is substantially different from the earlier version as shown in Sogacheva et al. (2017), especially for high AOD regions. (Che et al. (2016) do not mention which ADV version was used, but at their submission date the latest updates described in Sogacheva et al., 2017 had not been implemented). Sogacheva et al. (2017) present a validation of the full-mission AATSR AOD over China vs. AERONET AOD. Figure 7 shows a density scatterplot of the ATSR-retrieved AOD vs. AERONET AOD for the sites listed in Table S1 in the Supplement. Comparison of the AOD scatterplots in Che et al. (2016) (their Fig. 2a) with those in Fig. 7 shows the better performance of the newer ADV version v2.31 over China although a small underestimation of less than 0.1 remains for AOD up to ∼ 0.5, increasing somewhat with increasing AOD between 0.5 and 0.9. For AOD larger than 1.4, ADV v2.31 overestimates with respect to the AERONET reference AOD and, in view of the low number of valid data points and their large scatter, the use of these data is not recommended.
4.2 MODIS/Terra C6 merged DTDB AOD
MODIS AOD over China has been validated vs. AERONET and CARSNET AOD. However, as discussed in Sect. 3.2, only few publications address the validation of the MODIS C6 data suite and most of these consider only the Aqua DT and DB data set. The validation of the MODIS/Terra C6 merged DTDB L2 AOD product is shown in the density scatterplot of Fig. 8, where MODIS/Terra AOD has been plotted vs. AERONET AOD using the available AERONET sites listed in Table S1 in the Supplement. The MODIS AOD has been binned in AERONET bins with a width of 0.1 showing the good agreement between MODIS and AERONET data for AOD up to 1.8 but with a slight overestimation on the order of 0.1 for AOD up to 0.5 and somewhat more for higher AOD.
4.3 Intercomparison of AATSR and MODIS/Terra C6 merged DTDB AOD
Having established that both the ADV and the MODIS/Terra C6 merged DTDB AOD data sets compare well with the AERONET reference AOD data, we can address the differences observed in the AOD maps in Figs. 2 and 3, with MODIS AOD overall higher than that from ATSR. The difference map in Fig. 9 shows the actual differences between the two data sets (ATSR-MODIS) aggregated over the overlapping years (2000–2011) which are largest over brighter areas, such as the Taklamakan Desert and the Tibetan Plateau, where MODIS DTDB is governed by the DB data, which underestimate the AOD with respect to AERONET (Tao et al., 2015, 2017), whereas ADV and especially DT provide few successful retrievals. However, also over areas with very high AOD, such as the Sichuan province, NCP and YRD, the differences are large. In contrast, along the mountains from the NW to the SW of China and around the Sichuan province, the AATSR and MODIS AOD values are in very good agreement, within ±0.05, i.e., the estimated retrieval uncertainty over land (for MODIS). In other areas, the AOD difference is about 0.1, as may be expected from the validation presented above, i.e., showing that overall MODIS slightly overestimates and AATSR slightly underestimates with respect to AERONET which adds up to the AOD difference of about 0.1. It should be noted here that, as pointed out by a reviewer (A. Sayer, private communication, 2017), the MODIS team has been working on a new version, Collection 6.1, which has significantly lower AOD over, e.g., the Tibetan Plateau, while over east China the differences between C6 and C6.1 are very small. (At the time of writing of the current paper, C6.1 was not available.)
The surprising finding is thus the high AOD difference over SE China, i.e., mostly over the low elevation part of China (Liu et al., 2003) classified as forests and cropland (Bai et al., 2014), i.e., dark surfaces where retrieval algorithms are expected to perform best. This is also the area where most of the AERONET sun photometers are located, with clusters in the BTH area and the YRD. Apparently, the slight under- and overestimations by ATSR and MODIS, respectively, are not due to the surface correction in the retrieval algorithms and likely caused by the aerosol types used.
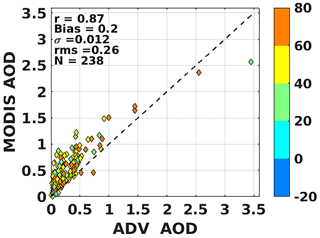
Figure 10MODIS/Terra C6 merged DTDB AOD vs. AATSR ADV v2.31 AOD, for collocated AATSR-MODIS-AERONET data, as described in the text of Sect. 4.3. The colors (see scale on the right) indicate the difference between the MODIS/Terra and ATSR overpass times in minutes.
Figure 10 shows a scatterplot of MODIS AOD vs. AATSR AOD, for collocated AATSR-MODIS-AERONET data; i.e., only AOD data are shown for AERONET sites where both MODIS and AATSR have achieved a successful retrieval and the overpasses were within ±1 h while also AERONET data were available, for the period 2002–2012. The color code indicates the difference in the exact overpass time. Figure 10 shows that the difference in exact overpass time, varying between 20 and 80 min, does not lead to a systematic effect on the MODIS/AATSR AOD differences. Hence, the somewhat later MODIS/Terra overpass, which could influence the AOD as a result of a developing atmospheric boundary layer during the morning hours and associated temperature and relative humidity difference, does not explain the higher MODIS AOD.
4.4 CALIOP
The comparison of the CALIOP data over the region 35–45∘ N over China with ATSR and MODIS data in Figs. 2 and 3 (or better in Fig. 11, which shows the seasonal variations of the AOD retrieved from ATSR and MODIS data), shows similar patterns with high AOD over the Taklamakan Desert and the BTH area (differences between ATSR and MODIS were discussed in Sect. 4.3). However, differences are also observed, i.e., the underestimation of CALIOP AOD compared to AOD retrieved by MODIS/Terra. As mentioned above, the CALIOP AODs refer to the years 2007–2015, while ATSR and MODIS/Terra AODs refer to the years 2000–2011. Hence, a direct comparison of the spatial distributions of the AOD should not be made since substantial year-to-year variations may occur, depending on meteorological and synoptic conditions. Furthermore, CALIPSO overpasses happen in the afternoon, whereas Envisat and Terra overpasses happen in the morning. The differences between the ATSR, MODIS/Terra and CALIOP AOD are likely due to the highly non-uniform data sample and to the fundamentally different algorithms and operation of the sensors. Following the literature, the CALIOP aerosol extinction coefficients are slightly underestimated as compared with EARLINET lidars (Papagiannopoulos et al., 2016). Tian et al. (2017) obtained similar results from comparison with lidar measurements in SACOL (China). A comparison of the CALIOP AOD climatological product against spatially and temporally co-located AERONET observations is discussed in Amiridis et al. (2015). In their Fig. 15, the absolute bias of the means between the CALIOP optimized product (named LIVAS) and AERONET reveals biases within ±0.1 in terms of AOD.
The spatial variation presented in the previous chapter is strongly influenced by emissions and meteorological factors which obviously vary seasonally due to both natural processes and human activities. As a result, strong seasonal variations are observed in the AOD distributions as shown in Fig. 11 where the ATSR- and MODIS-retrieved AOD values over the study area are shown for winter (DJF), spring (MAM), summer (JJA) and autumn (SON). As mentioned above, ATSR retrieval is often not successful over bright surfaces and has hardly any coverage over the Taklamakan and Gobi deserts and the Tibetan Plateau and also in the north during the winter. Due to the use of the merged DTDB AOD, MODIS has better coverage over bright surfaces and thus higher AOD over the Tibetan Plateau and the Taklamakan and Gobi deserts as well as in the north of China during the winter season. Seasonal AOD maps from CALIOP for the region 35–45∘ N over China were shown in Fig. 5 and the vertical climatological extinction coefficient profiles for the same region were presented in Fig. 6. CALIOP, ATSR and MODIS/Terra are used synergistically for the analysis of the regional and seasonal variations of the AOD over China. The horizontal variability of CALIOP AOD (Fig. 5) shows features similar to the AOD distributions provided by ATSR and MODIS/Terra (Fig. 11), i.e., high AOD values over the Taklamakan Desert and over the BTH area, with lower values over the Gobi Desert. However, differences also exist, as discussed in Sect. 3.3.
The AOD spatial distribution over China differs between seasons. The highest AOD is observed in the summertime over the NCP including the BTH area, with AOD of the order of 0.9 and somewhat lower values (about 0.6) in the spring and autumn, and minima in the winter. This seasonal behavior is in contrast to that of near-surface aerosol concentrations, indicated, for instance, by PM2.5, i.e., the mass concentration of dry particles with in situ diameters smaller than 2.5 µm, which peak in the winter and reach a minimum in the summer (e.g., Wang et al., 2015). These differences can be explained by seasonal variations in synoptic patterns (Xia et al., 2007; Miao et al., 2017) and associated boundary layer height and relative humidity, transport of aerosols, emission of primary aerosols and aerosol precursors contributing to chemical processes leading to secondary formation of new aerosol particles and thus higher concentrations (e.g., Tang et al., 2016). In particular, the seasonal emissions of dust aerosol (spring) and biomass burning aerosol (season varies with region) have a strong influence. Song et al. (2009) also noted that the temporal correlation between the monthly values of the PM10 concentrations and MODIS AOD exhibit regional seasonality contrasts over China, with correlation coefficients > 0.6 near the southeast coast and −0.6 or lower in the north-central regions. They attribute this contrast to differences in the aerosol size distributions and support their argument by MODIS data on the distribution of the Ångström exponent and fine-mode fraction.
Relatively high AOD is observed over the Sichuan province and Chongqing in all seasons, with values of about 0.8 and somewhat lower in the autumn. Also, over the Pearl River Delta (PRD), the AOD is relatively high throughout the year with values on the order of 0.4–0.6 in winter, summer and autumn and a maximum in the spring of about 0.8. In the Shanghai/Nanjing region in the YRD and the area to the SW of YRD toward PRD, the AOD is relatively high in the spring, summer and autumn, with values of about 0.5–0.6 and lower in the winter (around 0.3). South of the YRD, along the coast, an area is observed with more moderate AOD of about 0.3 throughout the whole year, which however stretches inland further in the winter then in the summer and autumn and is smallest in the spring. Over sea, along the SE coast of China, the AOD is on the order of 0.5 in the area north of the YRD, while further south it varies between about 0.3 and 0.5. Also the extent of the elevated AOD area varies with the season and is largest in the spring when it reaches east beyond Taiwan. It is noted that the BTH, PRD and YRD regions host three major urban clusters that constitute huge spatial sources of anthropogenic aerosols (Kourtidis et al., 2015). This brief discussion clearly illustrates the seasonal variation of the AOD, as well as the regional differences in the seasonal variation as earlier shown by, e.g., Song et al. (2009), Luo et al. (2014) and Li et al. (2017).
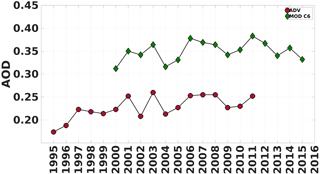
Figure 12Time series of ATSR- and MODIS-retrieved AOD over China for two decades: 1995–2015. Note that data are missing in the beginning of the ATSR-2 observation period in 1995, and also MODIS/Terra data are available starting in April 2000; AATSR data start in May 2002. Therefore, the ATSR-2 1995 data point includes data for June–December and for 1996 July–December. ATSR-2 was used from 1995 to July 2002; for August 2002 to 2011 AATSR data were used. AATSR for 2012 is not shown since only 3 months of data are available, mainly in the winter (January–February–March) with low coverage. The MODIS 2000 data point includes data from April to December.
The combined ATSR-2 and AATSR AOD data set provides a continuous record of 17 years from 1995 to 2012. The ATSR time series, for yearly averaged AOD over mainland China as defined in Fig. 1, is presented in Fig. 12. The first two data points, for 1995 and 1996, miss the winter and spring months and may therefore be not fully representative. This time series shows a strong initial increase in the AOD by 50 %, from about 0.18 in 1995 to 0.27–0.28 in 2001 and 2003 but with a dip in 2002. It is noted that 2002 is the transition between ATSR-2 and AATSR, and the data point presented in Fig. 12 is the average of 7 months (January–July) of ATSR-2 AOD and 5 months (August–December) of AATSR AOD.
This initial increase is followed by a strong decrease in 2004 after which the AOD seems to increase to a plateau of about 0.27 in 2006–2008. In 2009 and 2010, the AOD is lower by about 0.03–0.04 and then increases again to about 0.27 in 2011. The interruption of the ATSR time series after 2011 is unfortunate because this year could be a tipping point where the AOD starts to decrease, as shown by Zhao et al. (2017) for east and central China. Therefore, to visualize the evolution of AOD over all of China beyond the ATSR era, the MODIS/Terra time series has been added in Fig. 12. It is noted that the first MODIS data point for 2000 may not be fully representative since the first 3 months are missing.
Clearly, as described above, there are differences between ATSR and MODIS/Terra AOD and the MODIS/Terra yearly averaged AOD is 0.1–0.2 higher than that of ATSR. However, as Fig. 12 shows, the two curves show similar features with minima and maxima in the same years. The offset between the two data sets is similar for most of the period 2003–2011. Hence, in spite of this offset, the temporal behavior of the AOD is well represented by both data sets, for the overlapping period. The clear advantage of using both data sets is that ATSR provides additional information on the pre-EOS (Earth Observing System) period, showing the initial increase of the AOD before 2000. MODIS/Terra complements the time series for the post-Envisat period, showing a decrease after 2011.
The observed AOD variations may be in response to the enforcement of policies to reduce emissions, but they may also be caused by meteorological influences or a combination of these and other factors. The initial growth between 1995 and 2001 reflects urbanization and economic growth (e.g., Hao and Wang, 2005) and was also reported by Guo et al. (2011) using TOMS-retrieved AOD and by Hsu et al. (2012) using SeaWiFS. The initial decline after 2003 may be due to emission control measures such as those implemented in Beijing and reducing atmospheric concentrations of PM10, SO2 and NO2 (Hao and Wang, 2005). The AOD minimum in 2002 is observed in both the ATSR and MODIS/Terra data in Fig. 12, but the Multi-angle Imaging Spectroradiometer (MISR) AOD over the NCP presented by Liu et al. (2013) shows a clear maximum. Liu et al. (2013) relate the variability in the AOD over the NCP to large-scale periodic climate variability modulated by the El Niño–Southern Oscillation (ENSO) with a period of 3–4 years.
Several authors identify a pivot point around 2006–2008 (Kang et al., 2016; Zhang et al., 2017; Zhao et al., 2017) after which the AOD fluctuates before the decrease sets in from 2011 (Zhao et al., 2017). Using a different analysis method, Zhang et al. (2017) suggest a decrease starting from about 2006–2008. It is noted that these studies were made for east or SE China with much higher AOD, as shown in Figs. 2 and 3, than those in Fig. 12 which are averages over all of China. The data in Fig. 12 show a decrease in the MODIS data for the period 2006–2009, as observed by Zhang et al. (2017), but the ATSR AOD does not show a clear decrease in that period. The decrease in both data sets in 2008–2009 could be the result of the economic recession as suggested by, e.g., Lin et al. (2010), He et al. (2016) and Zhao et al. (2017). The data in Fig. 12 do however suggest the onset of a decrease in 2011, confirming the conclusion by Zhao et al. (2017). This behavior with pivot points in 2006 and 2011 is in line with the reduction of emissions of aerosol precursor gases, such as SO2 and NO2 (van der A et al., 2017), possibly together with large-scale climate variability as discussed above. The increase/decrease of anthropogenic particles is expected to increase/decrease the water uptake from the aerosols and hence the recorded AODs (Pozzer et al., 2015).
Satellite data have been used to provide a 3-D aerosol climatology over mainland China for two decades (1995–2015), describing the spatial variation of the column-integrated extinction, or AOD, by combining ATSR-2, AATSR (both using the most recent data set produced by the ADV v2.31 algorithm) and MODIS/Terra C6 merged DTDB AOD data. The vertical dimension has been provided by the CALIOP extinction measurements since 2007 and the temporal variation has been provided by the time series of the yearly AOD. Inspection of the AOD data from different sources shows strong differences due to the failure of retrieval algorithms to deal with very bright surfaces, except for the MODIS DB algorithm which was designed for this purpose. However, DB and DT are complementary because DT performs better than DB over dark surfaces and the merged DTDB provides better coverage. Although the ATSR dual view algorithm was designed to eliminate the effect of surface reflectance on the radiances measured at TOA, it does not work well over very bright surfaces (Flowerdew and Haigh, 1995; Kolmonen et al., 2016). The differences between the ATSR- and MODIS/Terra-retrieved AODs have been addressed in detail by validation and evaluation of the individual AOD data sets vs. a reference data set provided by AERONET. This study presents the first extensive validation of MODIS/Terra C6 merged DTDB and ATSR v2.31 AOD data over China. The results show that both data sets are of high quality. ATSR slightly underestimates and MODIS/Terra C6 slightly overestimates AOD, with respect to AERONET AOD, resulting in an overall higher MODIS than ATSR AOD and the difference increases as AOD is larger. We have no explanation for this behavior and a more detailed study on the differences between the MODIS and ATSR algorithms is beyond the scope of the present study. Likely, they are due to the choice of the aerosol models and/or cloud screening, while also calibration issues may influence the results. The data in Fig. 10 are collocated MODIS/Terra, ATSR and AERONET data, and it is unlikely that the results from these instruments together, with different cloud-screening criteria, are cloud contaminated. MODIS, with its wider swath than ATSR, provides a much larger data sample and has global coverage on a nearly daily basis. Hence, MODIS might also provide a statistically better sample but the metrics shown in Figs. 7 and 8 are similar, except that ATSR underestimates by 0.07 and MODIS overestimates by a similar amount. It is noted that differences in the AOD value such as shown here between ATSR and MODIS are also observed between the two MODIS instruments (with a 4 h difference in overpass time which may influence the results) and between MODIS and MISR (Zhao et al., 2017; Zhang et al., 2017). MISR flies on Terra and has a swath width which is only a little smaller than that of ATSR. The comparison of the ATSR and MODIS/Terra AOD data sets over China with AERONET does not show a clear advantage for one of these, and statistically the validation results are similar in spite of differences in AOD values. This conclusion applies to areas where both ATSR and MODIS provide quality data, and hence over the bright surfaces in western China, such as the Taklamakan Desert, the ATSR data cannot be used. Further development is needed to account for the surface effects on the ATSR TOA radiance. In conclusion, the MODIS/Terra and ATSR AOD values are different, but there is no clear preference, in regard to data quality, for one or the other. In view of the slight overestimation of MODIS and the slight underestimation of ATSR, the complimentary use of the AOD retrieved from these instruments may provide added value for, e.g., data assimilation in chemical transport models.
The spatial distribution and the temporal behavior of the ATSR and MODIS AOD data sets show similar features, with similar covariation in time and space. The spatial AOD distribution is a 20-year climatology which updates and extends earlier climatologies derived from MODIS C5.1 data, for different periods, usually starting in about 2000 (Terra; e.g., Guo et al., 2016b; Luo et al., 2014) or 2002 (Aqua; e.g., He et al., 2016). The 3-D climatology by Guo et al. (2016b) focuses on the frequency of occurrence of aerosols over China for 2006–2014. MODIS C5.1 publications on AOD over China usually address certain aspects including, e.g., regional studies over SE China or over regions such as BTH (e.g., Li et al., 2007; Tang et al., 2016), YRD (e.g., Li et al., 2015; Kang et al., 2016) or PRD (e.g., Bilal et al., 2017), differences between DT- and DB-retrieved AOD over certain regions or validation. However, none of them present an overview for all of China, or even all of eastern China, and addresses differences between different regions. CSHNET (Y. Wang et al., 2011) or CARSNET (Che et al., 2015) do provide data all over China; however, these are point measurements with low coverage over rural areas. Satellite data fill these gaps but with lower temporal resolution. As briefly discussed in Sect. 5, some distinct differences in the AOD seasonal behavior can be observed over different parts of China, i.e., from south to north and from east to west.
The AOD time series presented in Sect. 6 are for all of China, including both the relatively clean western China and the relatively polluted SE and SW China. Clearly, this is not a good representation of differences of emission abatement policy for either aerosols (e.g., Zhao et al., 2017) or precursor gases (e.g., van der A et al., 2017) with a complicated effect on AOD (Lin et al., 2010) and their effects on different parts of industrialized China. In addition, the evolution of the AOD is not only determined by policy and economic development but also by the evolution of living standards and migration of people in China, such as urbanization and development of agriculture, which may be different across the country. Furthermore, different sources influence the aerosol content in different parts of China and in different seasons, i.e., dust emission in the west in the spring, biomass burning in the summer/autumn seasons in eastern China and the emissions of VOCs from vegetated areas (e.g., Tan et al., 2015). The effects of these emissions are augmented by the meteorological conditions which also vary by region and season (e.g., Ding and Murakami, 1994; Domros and Peng, 1988; Song et al., 2011; Jiang et al., 2015) and large-scale periodic climate variability (e.g., Liu et al., 2013).
Two decades of satellite-derived aerosol optical properties provide an extended aerosol climatology over China (1995–2015), using the most recent retrieval results. The analysis of the data from different sensors shows the following:
-
The MODIS/Terra C6 merged DTDB AOD over China is distinctly higher than that retrieved from ATSR using the ADV v2.31 algorithm and the difference increases with increasing AOD.
-
Validation of both data sets over China shows that both MODIS/Terra C6 DTDB and ATSR ADV v2.31 AOD compare well with AERONET reference data but MODIS slightly overestimates and ATSR slightly underestimates with respect to the AERONET AOD.
-
AOD time series for ATSR and MODIS AOD show similar features and, although with a substantial offset, they provide complimentary information in regard to the AOD increase in the late 1990s (pre-EOS) and the apparent decrease after the end of the Envisat mission in April 2012.
-
Seasonal variations in the AOD are evident and vary for different parts of China.
The regional variation of seasonality and long-term behavior of the AOD over China will be discussed in Sogacheva et al. (2018).
The ATSR data used in this paper are publicly available (after registration, a password will be issued) at http://www.icare.univ-lille1.fr/. MODIS data are publicly available at https://ladsweb.modaps.eosdis.nasa.gov/ and CALIOP data are available via the LIVAS NetCDF database (after obtaining log-in credentials) at ftp://lidar.space.noa.gr. A technical description of the LIVAS database is available under http://lidar.space.noa.gr:8080/livas/. A brief description of the product can be found in the recently published article in ACP: (http://www.atmos-chem-phys.net/15/7127/2015/acp-15-7127-2015.html). AERONET data are available at AERONET: https://aeronet.gsfc.nasa.gov/.
The supplement related to this article is available online at: https://doi.org/10.5194/acp-18-1573-2018-supplement.
Work presented in this contribution was undertaken as part of the MarcoPolo
project supported by the EU, FP7 SPACE grant agreement no. 606953 and as part
of the Globemission project ESA-ESRIN Data Users Element (DUE), project
AO/1-6721/11/I-NB, and contributes to the ESA/MOST DRAGON4 program. The ATSR
algorithm (ADV/ASV) used in this work is improved with support from ESA as
part of the Climate Change Initiative (CCI) project Aerosol_cci (ESA-ESRIN
projects AO/1-6207/09/I-LG and ESRIN/400010987 4/14/1-NB). Further support
was received from the Centre of Excellence in Atmospheric Science funded by
the Finnish Academy of Sciences Excellence (project no. 272041). Emmanouil
Proestakis acknowledges the Stavros Niarchos Foundation for its support. Many
thanks are expressed to NASA Goddard Space Flight Center (GSFC) Level 1 and
Atmosphere Archive and Distribution System (LAADS)
(http://ladsweb.nascom.nasa.gov) for making available the L3
MODIS/Terra C5.1 and C6 aerosol data. We thank the reviewers of this paper
for their valuable comments which helped improve the
manuscript.
Edited by: Stelios
Kazadzis
Reviewed by: Andrew Sayer and two anonymous referees
Amiridis, V., Wandinger, U., Marinou, E., Giannakaki, E., Tsekeri, A., Basart, S., Kazadzis, S., Gkikas, A., Taylor, M., Baldasano, J., and Ansmann, A.: Optimizing CALIPSO Saharan dust retrievals, Atmos. Chem. Phys., 13, 12089–12106, https://doi.org/10.5194/acp-13-12089-2013, 2013.
Amiridis, V., Marinou, E., Tsekeri, A., Wandinger, U., Schwarz, A., Giannakaki, E., Mamouri, R., Kokkalis, P., Binietoglou, I., Solomos, S., Herekakis, T., Kazadzis, S., Gerasopoulos, E., Proestakis, E., Kottas, M., Balis, D., Papayannis, A., Kontoes, C., Kourtidis, K., Papagiannopoulos, N., Mona, L., Pappalardo, G., Le Rille, O., and Ansmann, A.: LIVAS: a 3-D multi-wavelength aerosol/cloud database based on CALIPSO and EARLINET, Atmos. Chem. Phys., 15, 7127–7153, https://doi.org/10.5194/acp-15-7127-2015, 2015.
Anenberg, S. C., Horowitz, L. W., Tong, D. Q., and West, J. J.: An estimate of the global burden of anthropogenic ozone and fine particulate matter on premature human mortality using atmospheric modeling, Environ. Health Persp., 11, 1189–1195, 2010.
Ansmann, A., Bösenberg, J., Chaikovsky, A., Comeron, A., Eckhardt, S., Eixmann, R., Freudenthaler, V., Ginoux, P., Komguem, L., Linne, H., Lopez Marquez, M. A., Matthias, V., Mattis, I., Mitev, V., Muller, D., Music, S., Nickovic, S., Pelon, J., Sauvage, L., Sobolewsky, P., Srivastava, M. K., Stohl, A., Torres, O., Vaughan, G., Wandinger, U., and Wiegner, M.: Long-range transport of Saharan dust to northern Europe: the 11–16 October 2001 outbreak observed with EARLINET, J. Geophys. Res., 108, 4783, https://doi.org/10.1029/2003JD003757, 2003.
Bai, Y., Feng, M., Jiang, V, Wang, J., Zhu, Y., and Liu, Y.: Assessing consistency of five global land cover data sets in China, Remote Sens.-Basel, 6, 8739–8759, https://doi.org/10.3390/rs6098739, 2014.
Bilal, M., Nichol, J. E., and Spak, S. N.: A new approach for estimation of fine particulate concentrations using satellite aerosol optical depth and binning of meteorological variables, Aerosol Air Qual. Res., 17, 356–367, 2017.
Che, H., Zhang, X.-Y., Xia, X., Goloub, P., Holben, B., Zhao, H., Wang, Y., Zhang, X.-C., Wang, H., Blarel, L., Damiri, B., Zhang, R., Deng, X., Ma, Y., Wang, T., Geng, F., Qi, B., Zhu, J., Yu, J., Chen, Q., and Shi, G.: Ground-based aerosol climatology of China: aerosol optical depths from the China Aerosol Remote Sensing Network (CARSNET) 2002–2013, Atmos. Chem. Phys., 15, 7619–7652, https://doi.org/10.5194/acp-15-7619-2015, 2015.
Che, Y., Xue, Y., Mei, L., Guang, J., She, L., Guo, J., Hu, Y., Xu, H., He, X., Di, A., and Fan, C.: Technical note: Intercomparison of three AATSR Level 2 (L2) AOD products over China, Atmos. Chem. Phys., 16, 9655–9674, https://doi.org/10.5194/acp-16-9655-2016, 2016.
de Leeuw, G., Holzer-Popp, T., Bevan, S., Davies, W., Descloitres, J., Grainger, R. G., Griesfeller, J., Heckel, A., Kinne, S., Klüser, L., Kolmonen, P., Litvinov, P., Martynenko, D., North, P. J. R., Ovigneur, B., Pascal, N., Poulsen, C., Ramon, D., Schulz, M., Siddans, R., Sogacheva, L., Tanré, D., Thomas, G. E., Virtanen, T. H., von Hoyningen Huene, W., Vountas, M., and Pinnock, S.: Evaluation of seven European aerosol optical depth retrieval algorithms for climate analysis, Remote Sens. Environ., 162, 295–315, https://doi.org/10.1016/j.rse.2013.04.023, 2015.
DeMott, P. J., Sassen, K., Poellot, M. R., Baumgardner, D., Rogers, D. C., Brooks, S. D., Prenni, A. J., and Kreidenweis, S. M.: African dust aerosols as atmospheric ice nuclei, Geophys. Res. Lett., 36, L07808, https://doi.org/10.1029/2009GL037639, 2009.
Ding, Y. H. and Murakami, M.: The Asian Monsoon, China Meteorological Press, Beijing, China, 1994.
Ding, J., van der A, R. J., Mijling, B., and Levelt, P. F.: Space-based NOx emission estimates over remote regions improved in DECSO, Atmos. Meas. Tech., 10, 925–938, https://doi.org/10.5194/amt-10-925-2017, 2017.
Domros, M. and Peng, G.: The Climate of China, Springer Verlag, Berlin, 1988.
Dong, Z., Li, Z., Yu, X., Cribb, M., Li, X., and Dai, J.: Opposite long-term trends in aerosols between low and high altitudes: a testimony to the aerosol–PBL feedback, Atmos. Chem. Phys., 17, 7997–8009, https://doi.org/10.5194/acp-17-7997-2017, 2017.
Eck, T. F., Holben, B. N., Reid, J. S., Dubovik, O., Smirnov, A., O'Neill, N. T., Slutsker, I., and Kinne, S.: Wavelength dependence of the optical depth of biomass burning, urban, and desert dust aerosols, J. Geophys. Res., 104, 31333–31349, https://doi.org/10.1029/1999JD900923, 1999.
Flowerdew, R. J. and Haigh, J. D.: An approximation to improve accuracy in the derivation of surface reflectances from multi-look satellite radiometers, Geophys. Res. Lett., 22, 1693–1696, 1995.
Georgoulias, A. K., Alexandri, G., Kourtidis, K. A., Lelieveld, J., Zanis, P., and Amiridis, V.: Differences between the MODIS Collection 6 and 5.1 aerosol datasets over the greater Mediterranean region, Atmos. Environ., 147, 310–319, https://doi.org/10.1016/j.atmosenv.2016.10.014, 2016.
Goudie, A. S.: Desert dust and human health disorders, Environ. Int., 63, 101–113, https://doi.org/10.1016/j.envint.2013.10.011, 2014.
Guo, J., Deng, M., Lee, S. S., Wang, F., Li, Z., Zhai, P., Liu, H., Lv, W., Yao, W., and Li, X.: Delaying precipitation and lightning by air pollution over the Pearl River Delta. Part I: Observational analyses, J. Geophys. Res.-Atmos., 121, 6472–6488, https://doi.org/10.1002/2015JD023257, 2016a.
Guo, J., Liu, H., Wang, F., Huang, J., Xia, F., Lou, M., Wu, Y., Jiang, J. H., Xie, T., Zhaxi, Y., and Yung, Y. L.: Three-dimensional structure of aerosol in China: a perspective from multi-satellite observations, Atmos. Res., 178–179, 580–589, 2016bs.
Guo, J., Xia, F., Zhang, Y., Liu, H., Li, J., Lou, M., He, J., Yan, Y., Wang, F., Min, M., and Zhai, P.: Impact of diurnal variability and meteorological factors on the PM2.5–AOD relationship: implications for PM2.5 remote sensing, Environ. Pollut., 221, 94–104, 2017.
Guo, J. P., Zhang, X.-Y., Wu, Y.-R., Zhaxi, Y., Che, H.-Z., La, B., Wang, W., and Li, X.-W.: Spatio-temporal variation trends of satellite-based aerosol optical depth in China during 1980–2008, Atmos. Environ., 45, 6802–6811, 2011.
Hao, J. and Wang, L.: Improving urban air quality in China: Beijing Case Study, JAPCA J. Air Waste Ma., 55, 1298–1305, https://doi.org/10.1080/10473289.2005.10464726, 2005.
Hatch, C. D., Gierlus, K. M., Schuttlefield, J. D., and Grassian, V. H.: Water adsorption and cloud condensation nuclei activity of calcite and calcite coated with model humic and fulvic acids, Atmos. Environ., 42, 5672–5684, https://doi.org/10.1016/j.atmosenv.2008.03.005, 2008.
Haywood, J. and Boucher, O.: Estimates of the direct and indirect radiative forcing due to tropospheric aerosols: a review, Rev. Geophys., 38, 513–543, https://doi.org/10.1029/1999RG000078, 2000.
He, Q., Ming, Z., and Huang, B.: Spatio-temporal variation and impact factors analysis of satellite based aerosol optical depth over China from 2002 to 2015, Atmos. Environ., 129, 79–90, 2016.
Holben, B. N., Eck, T. F., Slutsker, I., Tanré, D., Buis, J. P., Setzer, A., Vermote, E., Reagan, J. A., Kaufman, Y. J., Nakajima, T., Lavenu, F., Jankowiak, I., and Smirnov, A.: AERONET – a federated instrument network and data archive for aerosol characterization, Remote Sens. Environ., 66, 1–16, 1998.
Holben, B. N., Kim, J., Sano, I., Mukai, S., Eck, T. F., Giles, D. M., Schafer, J. S., Sinyuk, A., Slutsker, I., Smirnov, A., Sorokin, M., Anderson, B. E., Che, H., Choi, M., Crawford, J. H., Ferrare, R. A., Garay, M. J., Jeong, U., Kim, M., Kim, W., Knox, N., Li, Z., Lim, H. S., Liu, Y., Maring, H., Nakata, M., Pickering, K. E., Piketh, S., Redemann, J., Reid, J. S., Salinas, S., Seo, S., Tan, F., Tripathi, S. N., Toon, O. B., and Xiao, Q.: An overview of mesoscale aerosol processes, comparisons, and validation studies from DRAGON networks, Atmos. Chem. Phys., 18, 655–671, https://doi.org/10.5194/acp-18-655-2018, 2018.
Holzer-Popp, T., de Leeuw, G., Griesfeller, J., Martynenko, D., Klüser, L., Bevan, S., Davies, W., Ducos, F., Deuzé, J. L., Graigner, R. G., Heckel, A., von Hoyningen-Hüne, W., Kolmonen, P., Litvinov, P., North, P., Poulsen, C. A., Ramon, D., Siddans, R., Sogacheva, L., Tanre, D., Thomas, G. E., Vountas, M., Descloitres, J., Griesfeller, J., Kinne, S., Schulz, M., and Pinnock, S.: Aerosol retrieval experiments in the ESA Aerosol_cci project, Atmos. Meas. Tech., 6, 1919–1957, https://doi.org/10.5194/amt-6-1919-2013, 2013.
Hsu, N. C., Tsay, S. C., King, M. D., and Herman, J. R.: Aerosol properties over bright-reflecting source regions, IEEE T. Geosci. Remote, 42, 557–569, https://doi.org/10.1109/TGRS.2004.824067, 2004.
Hsu, N. C., Gautam, R., Sayer, A. M., Bettenhausen, C., Li, C., Jeong, M. J., Tsay, S.-C., and Holben, B. N.: Global and regional trends of aerosol optical depth over land and ocean using SeaWiFS measurements from 1997 to 2010, Atmos. Chem. Phys., 12, 8037–8053, https://doi.org/10.5194/acp-12-8037-2012, 2012.
Hsu, N. C., Jeong, M.-J., Bettenhausen, C., Sayer, A. M., Hansell, R., Seftor, C. S., Huang, J., and Tsay, S.-C.: Enhanced Deep Blue aerosol retrieval algorithm: the second generation, J. Geophys. Res.-Atmos., 118, 9296–9315, https://doi.org/10.1002/jgrd.50712, 2013.
Hunt, W. H., Winker, D. M., Vaughan, M. A., Powell, K. A., Lucker, P. L., and Weimer, C.: CALIPSO lidar description and performance assessment, J. Atmos. Ocean. Tech., 26, 1214–1228, 2009.
Jiang, Y., Yang, X.-Q., and Liu, X.: Seasonality in anthropogenic aerosol effects on East Asian climate simulated with CAM5, J. Geophys. Res.-Atmos., 120, 10837–10861, https://doi.org/10.1002/2015JD023451, 2015.
Kang, N., Kumar, K. R., Hu, K., Yu, X., and Yin, Y.: Long-term (2002–2014) evolution and trend in Collection 5.1 Level-2 aerosol products derived from the MODIS and MISR sensors over the Chinese Yangtze River Delta, Atmos. Res., 181, 29–43, 2016.
Kaufman, Y. J., Tanrè, D., Remer, L. A., Vermote, E. F., Chu, A., and Holben, B. N.: Operational remote sensing of tropospheric aerosol over land from EOS moderate resolution imaging spectroradiometer, J. Geophys. Res., 102, 17051, https://doi.org/10.1029/96JD03988, 1997.
Kolmonen, P., Sogacheva, L., Virtanen, T. H., de Leeuw, G., and Kulmala, M.: The ADV/ASV AATSR aerosol retrieval algorithm: current status and presentation of a full-mission AOD data set, Int. J Digit. Earth, 9, 545–561, https://doi.org/10.1080/17538947.2015.1111450, 2016.
Koren, I., Dagan, G., and Altaratz, O.: From aerosol-limited to invigoration of warm convective clouds, Science, 344, 1143–1146, https://doi.org/10.1126/science.1252595, 2014.
Kourtidis, K., Stathopoulos, S., Georgoulias, A. K., Alexandri, G., and Rapsomanikis, S.: A study of the impact of synoptic weather conditions and water vapor on aerosol–cloud relationships over major urban clusters of China, Atmos. Chem. Phys., 15, 10955–10964, https://doi.org/10.5194/acp-15-10955-2015, 2015.
Kulmala, M. and Kerminen, V.-M.: On the formation and growth of atmospheric nanoparticles, Atmos. Res., 90, 132–150, 2008.
Kulmala, M., Arola, A., Nieminen, T., Riuttanen, L., Sogacheva, L., de Leeuw, G., Kerminen, V.-M., and Lehtinen, K. E. J.: The first estimates of global nucleation mode aerosol concentrations based on satellite measurements, Atmos. Chem. Phys., 11, 10791–10801, https://doi.org/10.5194/acp-11-10791-2011, 2011.
Levy, R. C., Remer, L. A., Kleidman, R. G., Mattoo, S., Ichoku, C., Kahn, R., and Eck, T. F.: Global evaluation of the Collection 5 MODIS dark-target aerosol products over land, Atmos. Chem. Phys., 10, 10399–10420, https://doi.org/10.5194/acp-10-10399-2010, 2010.
Levy, R. C., Mattoo, S., Munchak, L. A., Remer, L. A., Sayer, A. M., Patadia, F., and Hsu, N. C.: The Collection 6 MODIS aerosol products over land and ocean, Atmos. Meas. Tech., 6, 2989–3034, https://doi.org/10.5194/amt-6-2989-2013, 2013.
Li, C., Mao, J., Lau, K.-H. A., Chen, J.-C., Yuan, Z., Liu, X., Zhu, A., and Liu, G.: Characteristics of distribution and seasonal variation of aerosol optical depth in eastern China with MODIS products, Chinese Sci. Bull., 48, 2488–2495, 2003.
Li, L.-J, Wang, Y., Zhang, Q, Yu, T., Zhao, Y, and Jin, J.: Spatial distribution of aerosol pollution based on MODIS data over Beijing, China, J. Environ. Sci., 19, 955–960, 2007.
Li, S., Wang, T., Xie, M., Han, Y., and Zhuang, B.: Observed aerosol optical depth and angstrom exponent in urban area of Nanjing, China, Atmos. Environ., 123, 350–356, 2015.
Li, Z., Xu, H., Li, K., Li, D., Xie, Y., Li, L., Zhang, Y., Gu, X., Zhao, W., Tian, Q., Deng, R., Su, X., Huang, B., Qiao, Y., Cui, W., Hu, Y., Gong, C., Wang, Y., Wang, X., Wang, J., Du, W., Pan, Z., Li, Z., and Bu, D.: Comprehensive study of optical, physical, chemical and radiative properties of total columnar atmospheric aerosols over China: an overview of Sun-sky radiometer Observation NETwork (SONET) measurements, B. Am. Meteorol. Soc., https://doi.org/10.1175/BAMS-D-17-0133.1, in press, 2017.
Lin, J., Nielsen, C. P., Zhao, Y., Lei, Y., Liu, Y., and Mcelroy, B.: Recent changes in particulate air pollution over China observed from space and the ground: effectiveness of emission control, Environ. Sci. Technol., 44, 7771–7776, 2010.
Liu, D., Wang, Z., Liu, Z., Winker, D., and Trepte, C.: A height resolved global view of dust aerosols from the first year CALIPSO lidar measurements, J. Geophys. Res.-Atmos., 113, D16214, https://doi.org/10.1029/2007JD009776, 2008.
Liu, J. Y., Zhuang, D. F., Luo, D., and Xiao, X.:, Land-cover classification of China: integrated analysis of AVHRR imagery and geophysical data, Int. J. Remote Sens., 24, 2485–2500, https://doi.org/10.1080/01431160110115582, 2003.
Liu, Y., Liu, J., and Tao, S.: Interannual variability of summertime aerosol optical depth over East Asia during 2000–2011: a potential influence from El Niño Southern Oscillation, Environ. Res. Lett., 8, 1–8, https://doi.org/10.1088/1748-9326/8/4/044034, 2013.
Liu, Z., Sugimoto, N., and Murayama, T.: Extinction-to-backscatter ratio of Asian dust observed with high-spectral-resolution lidar and Raman Lidar, Appl. Optics, 41, 2760, https://doi.org/10.1364/AO.41.002760, 2002.
Luo, Y., Zheng, X., Zhao, T., and Chen, J.: A climatology of aerosol optical depth over China from recent 10 years of MODIS remote sensing data, Int. J. Climatol., 34, 863–870, 2014.
Ma, Z., Hu, X., Sayer, A. M., Levy, R., Zhang, Q., Xue, Y., Tong, S., Bi, J., Huang, L., and Liu, Y.: Satellite-based spatiotemporal trends in PM2.5 concentrations: China, 2004–2013, Environ. Health Persp., 124, 184–192, https://doi.org/10.1289/ehp.1409481, 2016.
Miao, Y., Guo, J., Liu, S., Liu, H., Li, Z., Zhang, W., and Zhai, P.: Classification of summertime synoptic patterns in Beijing and their associations with boundary layer structure affecting aerosol pollution, Atmos. Chem. Phys., 17, 3097–3110, https://doi.org/10.5194/acp-17-3097-2017, 2017.
Omar, A., Winker, D., Kittaka, C., Vaughan, M., Liu, Z., Hu, Y. X., Trepte, C., Rogers, R., Ferrare, R., Lee, K., Kuehn, R., and Hostetler, C.: The CALIPSO automated aerosol classification and Lidar ratio selection algorithm, J. Atmos. Ocean. Tech., 26, 1994–2014, https://doi.org/10.1175/2009jtecha1231.1, 2009.
Papagiannopoulos, N., Mona, L., Alados-Arboledas, L., Amiridis, V., Baars, H., Binietoglou, I., Bortoli, D., D'Amico, G., Giunta, A., Guerrero-Rascado, J. L., Schwarz, A., Pereira, S., Spinelli, N., Wandinger, U., Wang, X., and Pappalardo, G.: CALIPSO climatological products: evaluation and suggestions from EARLINET, Atmos. Chem. Phys., 16, 2341–2357, https://doi.org/10.5194/acp-16-2341-2016, 2016.
Pope, C. A., Ezzati, M., and Dockery, D. W.: Fine-particulate air pollution and life expectancy in the United States, New Engl. J. Med., 360, 376–386, 2009.
Popp, T., de Leeuw, G., Bingen, C., Brühl, C., Capelle, V., Chedin, A., Clarisse, L., Dubovik, O., Grainger, R., Griesfeller, J., Heckel, A., Kinne, S., Klüser, L., Kosmale, M., Kolmonen, P., Lelli, L., Litvinov, P., Mei, L., North, P., Pinnock, S., Povey, A., Robert, C., Schulz, M., Sogacheva, L., Stebel, K., Stein Zweers, D., Thomas, G., Tilstra, L. G., Vandenbussche, S., Veefkind, P., Vountas, M., and Xue, Y.: Development, production and evaluation of aerosol Climate Data Records from European satellite observations (Aerosol_cci), Remote Sens.-Basel, 8, 421, https://doi.org/10.3390/rs8050421, 2016.
Pozzer, A., de Meij, A., Yoon, J., Tost, H., Georgoulias, A. K., and Astitha, M.: AOD trends during 2001–2010 from observations and model simulations, Atmos. Chem. Phys., 15, 5521–5535, https://doi.org/10.5194/acp-15-5521-2015, 2015.
Proestakis, E., Amiridis, V., Marinou, E., Georgoulias, A. K., Solomos, S., Kazadzis, S., Chimot, J., Che, H., Alexandri, G., Binietoglou, I., Daskalopoulou, V., Kourtidis, K. A., de Leeuw, G., and van der A, R. J.: Nine-year spatial and temporal evolution of desert dust aerosols over South and East Asia as revealed by CALIOP, Atmos. Chem. Phys., 18, 1337–1362, https://doi.org/10.5194/acp-18-1337-2018, 2018.
Prospero, J. M., Ginoux, P., Torres, O., Nicholson, S. E., and Gill, T. E.: Environmental characterization of global sources of atmospheric soil dust identified with the Nimbus 7 Total Ozone Mapping Spectrometer (TOMS) absorbing aerosol product, Rev. Geophys., 40, 1002, https://doi.org/10.1029/2000RG000095, 2002.
Remer, L. A., Kaufman, Y. J., Tanré, D., Mattoo, S., Chu, D. A., Martins, J. V., Li, R.-R., Ichoku, C., Levy, R. C., Kleidman, R. G., Eck, T. F., Vermote, E., and Holben, B. N.: The MODIS aerosol algorithm, products, and validation, J. Atmos. Sci., 62, 947–973, https://doi.org/10.1175/JAS3385.1, 2005.
Robles-Gonzalez, C. and de Leeuw, G.: Aerosol properties over the SAFARI-2000 area retrieved from ATSR-2, J. Geophys. Res., 113, D05206, https://doi.org/10.1029/2007JD008636, 2008.
Robles-Gonzalez, C., Veefkind, J. P., and de Leeuw, G.: Mean aerosol optical depth over Europe in August 1997 derived from ATSR-2 data, Geophys. Res. Lett., 27, 955–959, 2000.
Robles González, C., Schaap, M., de Leeuw, G., Builtjes, P. J. H., and van Loon, M.: Spatial variation of aerosol properties over Europe derived from satellite observations and comparison with model calculations, Atmos. Chem. Phys., 3, 521–533, https://doi.org/10.5194/acp-3-521-2003, 2003.
Robles-Gonzalez, C., de Leeuw, G., Decae, R., Kusmierczyk-Michulec, J., and Stammes, P.: Aerosol properties over the Indian Ocean Experiment (INDOEX) campaign area retrieved from ATSR-2, J. Geophys. Res., 111, D15205, https://doi.org/10.1029/2005JD006184, 2006.
Rodríguez, E., Kolmonen, P., Virtanen, T. H., Sogacheva, L., Sundström, A.-M., and de Leeuw, G.: Indirect estimation of absorption properties for fine aerosol particles using AATSR observations: a case study of wildfires in Russia in 2010, Atmos. Meas. Tech., 8, 3075–3085, https://doi.org/10.5194/amt-8-3075-2015, 2015.
Rosenfeld, D., Lohmann, U., Raga, G. B., O'Dowd, C. D., Kulmala, M., Fuzzi, S., Reissell, A., and Andreae, M. O.: Flood or drought: how do aerosols affect precipitation?, Science, 321, 1309–1313, 2008.
Salomonson, V. V., Barnes, W. L., Maymon, P. W., Montgomery, H. E., and Ostrow, H.: MODIS: advanced facility instrument for studies of the Earth as a system, IEEE T. Geosci. Remote, 27, 145–153, 1989.
Sayer, A. M., Hsu, N. C., Bettenhausen, C., and Jeong, M. J.: Validation and uncertainty estimates for MODIS Collection 6 “deep Blue” aerosol data, J. Geophys. Res.-Atmos., 118, 7864–7872, https://doi.org/10.1002/jgrd.50600, 2013.
Sayer, A. M., Munchak, L. A., Hsu, N. C., Levy, R. C., Bettenhausen, C., and Jeong, M.-J.: MODIS Collection 6 aerosol products: comparison between Aqua's e-Deep Blue, Dark Target, and “merged” data sets, and usage recommendations, J. Geophys. Res.-Atmos., 119, 13965–13989, https://doi.org/10.1002/2014JD022453, 2014.
Sayer, A. M., Hsu, N. C., Bettenhausen, C., Jeong, M., Meister, G., and Al, S. E. T.: Effect of MODIS Terra radiometric calibration improvements on Collection 6 Deep Blue aerosol products: validation and Terra/Aqua consistency, J. Geophys. Res.-Atmos., 120, 12157–12174, https://doi.org/10.1002/2015JD023878, 2015.
Schmid, B., Redemann, J., Russell, P. B., Hobbs, P. V., Hlavka, D. L., McGill, M. J., Holben, B. N., Welton, E. J., Campbell, J. R., Torres, O., Kahn, R. A., Diner, D. J., Helmlinger, M. C., Chu, D. A., Robles Gonzalez, C., and de Leeuw, G.: Coordinated airborne, spaceborne, and ground-based measurements of massive, thick aerosol layers during the dry season in southern Africa, J. Geophys. Res., 108, 8496, https://doi.org/10.1029/2002JD002297, 2003.
Seinfeld, J. H. and Pandis, S. N.: Atmospheric Chemistry and Physics: From Air Pollution to Climate Change, Wiley, New York, 1997.
Sisler, J. F. and Malm, W. C.: The relative importance of soluble aerosols to spatial and seasonal trends of impaired visibility in the United States, Atmos. Environ., 28, 851–862, 1994.
Smirnov, A., Holben, B. N., Eck, T. F., Dubovik, O., and Slutsker, I.: Cloud screening and quality control algorithms for the AERONET database, Remote Sens. Environ., 73, 337–349, https://doi.org/10.1016/S0034-4257(00)00109-7, 2000.
Sogacheva, L., Kolmonen, P., Virtanen, T. H., Rodriguez, E., Sundström, A.-M., and de Leeuw, G.: Determination of land surface reflectance using the AATSR dual-view capability, Atmos. Meas. Tech., 8, 891–906, https://doi.org/10.5194/amt-8-891-2015, 2015.
Sogacheva, L., Kolmonen, P., Virtanen, T. H., Rodriguez, E., Saponaro, G., and de Leeuw, G.: Post-processing to remove residual clouds from aerosol optical depth retrieved using the Advanced Along Track Scanning Radiometer, Atmos. Meas. Tech., 10, 491–505, https://doi.org/10.5194/amt-10-491-2017, 2017.
Sogacheva, L., de Leeuw, G., Rodriguez, E., Kolmonen, P., Kourtidis, K., Georgoulias, A. K., Alexandri, G., Amiridis, V., Proestakis, E., Marinou, E., Xue, Y., and van der A, R.: working title: Spatial and seasonal variations of aerosols over China from two decades of multi-satellite observations – Part I: ATSR (1995–2011) and MODIS C6.1 (2000–2017), in preparation for submission to Atmos. Chem. Phys., 2018.
Song, C.-K., Ho, C.-H., Park, R. J., Choi, Y.-S., Kim, J. Gong, D.-Y., and Lee, Y.-B.: Spatial and seasonal variations of surface PM10 concentration and MODIS Aerosol Optical Depth over China, Asia-Pac. J. Atmos. Sci., 45, 1, 33–43, 2009.
Song, Y., Achberger, C., and Linderholm, H. W.: Rain-season trends in precipitation and their effect in different climate regions of China during 1961–2008, Environ. Res. Lett., 6, 034025, https://doi.org/10.1088/1748-9326/6/3/034025, 2011.
Sun, J. M., Zhang, M. Y., and Liu, T. S.: Spatial and temporal characteristics of dust storms in China and its surrounding regions, 1960–1999: relations to source area and climate, J. Geophys. Res.-Atmos., 106, 10325–10333, https://doi.org/10.1029/2000JD900665, 2001.
Sundström, A.-M., Kolmonen, P., Sogacheva, L., and de Leeuw, G.: Aerosol retrievals over China with the AATSR Dual-View Algorithm, Remote Sens. Environ., 116, 189–198, 2012.
Sundström, A.-M., Nikandrova, A., Atlaskina, K., Nieminen, T., Vakkari, V., Laakso, L., Beukes, J. P., Arola, A., van Zyl, P. G., Josipovic, M., Venter, A. D., Jaars, K., Pienaar, J. J., Piketh, S., Wiedensohler, A., Chiloane, E. K., de Leeuw, G., and Kulmala, M.: Characterization of satellite-based proxies for estimating nucleation mode particles over South Africa, Atmos. Chem. Phys., 15, 4983–4996, https://doi.org/10.5194/acp-15-4983-2015, 2015.
Tan, C., Zhao, T., Xu, X., Liu, J., Zhang, L., and Tang, L.: Climatic analysis of satellite aerosol data on variations of submicron aerosols over East China, Atmos. Environ., 123, 392–398, 2015.
Tang, G., Zhang, J., Zhu, X., Song, T., Münkel, C., Hu, B., Schäfer, K., Liu, Z., Zhang, J., Wang, L., Xin, J., Suppan, P., and Wang, Y.: Mixing layer height and its implications for air pollution over Beijing, China, Atmos. Chem. Phys., 16, 2459–2475, https://doi.org/10.5194/acp-16-2459-2016, 2016.
Tanré, D., Kaufman, Y. J., Herman, M., and Mattoo, S.: Remote sensing of aerosol properties over oceans using the MODIS/EOS spectral radiances, J. Geophys. Res.-Atmos., 102, 16971–16988, https://doi.org/10.1029/96JD03437, 1997.
Tao, M., Chen, L., Wang, Z., Tao, J., Che, H., Wang, X., and Wang, Y.: Comparison and evaluation of the MODIS Collection 6 aerosol data in China, J. Geophys. Res.-Atmos., 120, 6992–7005, https://doi.org/10.1002/2015JD023360, 2015.
Tao, M., Chen, L., Wang, Z., Wang, J., Che, H., Xu, X., and Hou, C.: Evaluation of MODIS Deep Blue aerosol algorithm in desert region of East Asia: Ground validation and intercomparison, J. Geophys. Res.-Atmos., 122, 10357–10368, https://doi.org/10.1002/2017JD026976, 2017.
Tian, P., Cao, X., Zhang, L., Sun, N., Sun, L., Logan, T., Shi, J., Wang, Y., Ji, Y., Lin, Y., Huang, Z., Zhou, T., Shi, Y., and Zhang, R.: Aerosol vertical distribution and optical properties over China from long-term satellite and ground-based remote sensing, Atmos. Chem. Phys., 17, 2509–2523, https://doi.org/10.5194/acp-17-2509-2017, 2017.
van der A, R. J., Mijling, B., Ding, J., Koukouli, M. E., Liu, F., Li, Q., Mao, H., and Theys, N.: Cleaning up the air: effectiveness of air quality policy for SO2 and NOx emissions in China, Atmos. Chem. Phys., 17, 1775–1789, https://doi.org/10.5194/acp-17-1775-2017, 2017.
Veefkind, J. P., de Leeuw, G., and Durkee, P. A.: Retrieval of aerosol optical depth over land using two-angle view satellite radiometry during TARFOX, Geophys. Res. Letters., 25, 3135–3138, 1998.
Veefkind, J. P., de Leeuw, G., Durkee, P. A., Russell, P. B., Hobbs, P. V., and Livingston, J. M.: Aerosol optical depth retrieval using ATSR-2 and AVHRR data during TARFOX, J. Geophys. Res., 104, 2253–2260, 1999.
Veefkind, J. P., de Leeuw, G., Stammes, P., and Koelemeijer, R. B. A.: Regional distribution of aerosol over land derived from ATSR-2 and GOME, Remote Sens. Environ., 74, 377–386, 2000.
Virtanen, T. H., Kolmonen, P., Rodríguez, E., Sogacheva, L., Sundström, A.-M., and de Leeuw, G.: Ash plume top height estimation using AATSR, Atmos. Meas. Tech., 7, 2437–2456, https://doi.org/10.5194/amt-7-2437-2014, 2014.
Wang, S., Xing, J., Chatani, S., Hao, J., Klimont, Z., Cofala, J., and Amann, M.: Verification of anthropogenic emissions of China by satellite and ground observations, Atmos. Environ., 45, 6347–6358, 2011.
Wang, S., Li, G., Gong, Z., Du, L., Zhou, Q., Meng, X., Xie, S., and Zhou, L.: Spatial distribution, seasonal variation and regionalization of PM2.5 concentrations in China, Sci. China–Chem., 58, 1435–1443, https://doi.org/10.1007/s11426-015-5468-9, 2015.
Wang, Y., Xin, J., Li, Z., Wang, S., Wang, P., Hao, W. M., Nordgren, B. L., Chen, H., Wang, L., and Sun, Y.: Seasonal variations in aerosol optical properties over China, J. Geophys. Res., 116, D18209, https://doi.org/10.1029/2010JD015376, 2011.
Winker, D. M., Hunt, W. H., and McGill, M. J.: Initial performance assessment of CALIOP, Geophys. Res. Lett., 34, L19803, https://doi.org/10.1029/2007GL030135, 2007.
Winker, D. M., Vaughan, M. A., Omar, A. H., Hu, Y., Powell, K. A., Liu, Z., Hunt, W. H., and Young, S. A.: Overview of the CALIPSO Mission and CALIOP Data Processing Algorithms, J. Atmos. Ocean. Tech., 26, 2310–2323, https://doi.org/10.1175/2009JTECHA1281.1, 2009.
Winker, D. M., Tackett, J. L., Getzewich, B. J., Liu, Z., Vaughan, M. A., and Rogers, R. R.: The global 3-D distribution of tropospheric aerosols as characterized by CALIOP, Atmos. Chem. Phys., 13, 3345–3361, https://doi.org/10.5194/acp-13-3345-2013, 2013.
Xia, X., Chen, H., and Zhang, W.: Analysis of the dependence of column-integrated aerosol properties on long-range transport of air masses in Beijing, Atmos. Environ., 41, 7739–7750, 2007.
Xiao, Q., Zhang, H., Choi, M., Li, S., Kondragunta, S., Kim, J., Holben, B., Levy, R. C., and Liu, Y.: Evaluation of VIIRS, GOCI, and MODIS Collection 6 AOD retrievals against ground sunphotometer observations over East Asia, Atmos. Chem. Phys., 16, 1255–1269, https://doi.org/10.5194/acp-16-1255-2016, 2016.
Xin, J., Wang, Y., Li, Z., Wang, P., Hao, W. M., Nordgren, B. L., Wang, S., Liu, G., Wang, L., Wen, T., Sun, Y., and Hu, B.: Aerosol optical depth (AOD) and Angstrom exponent of aerosols observed by the Chinese Sun Hazemeter Network from August 2004 to September 2005, J. Geophys. Res., 2007, 27, 1703–1711.
Xu, H., Guo, J., Ceamanos, X., Roujean, J.-L., Min, M., and Carrer, D.: On the influence of the diurnal variations of aerosol content to estimate direct aerosol radiative forcing using MODIS data, Atmos. Environ., 119, 82–94, 2015.
Xu, X., Qiu, J., Xia, X., Sun, L., and Min, M.: Characteristics of atmospheric aerosol optical depth variation in China during 1993–2012, Atmos. Environ., 119, 82–94, 2015.
Xue, T., Zheng, Y., Geng, G., Zheng, B., Jiang, X., Zhang, Q., and He, K.: Fusing observational, satellite remote sensing and air quality model simulated data to estimate spatiotemporal variations of PM2.5 exposure in China, Remote Sens.-Basel, 9, 221, https://doi.org/10.3390/rs9030221, 2017.
Young, S. and Vaughan, M.: The retrieval of profiles of particulate extinction from Cloud Aerosol Lidar Infrared Pathfinder Satellite, Observations (CALIPSO) data: algorithm description, J. Atmos. Ocean. Tech., 26, 1105, https://doi.org/10.1175/2008JTECHA1221.1, 2009.
Yumimoto, K., Eguchi, K., Uno, I., Takemura, T., Liu, Z., Shimizu, A., and Sugimoto, N.: An elevated large-scale dust veil from the Taklimakan Desert: Intercontinental transport and three-dimensional structure as captured by CALIPSO and regional and global models, Atmos. Chem. Phys., 9, 8545–8558, https://doi.org/10.5194/acp-9-8545-2009, 2009.
Zhang, Q., Xin, J., Yin, Y., Wang, L., and Wang, Y.: The variations and trends of MODIS C5 and C6 products' errors in the recent decade over the background and urban areas of North China, Remote Sens.-Basel, 8, 754, https://doi.org/10.3390/rs8090754, 2016.
Zhang, J., Reid, J. S., Alfaro-Contreras, R., and Xian, P.: Has China been exporting less particulate air pollution over the past decade?, Geophys. Res. Lett., 44, 2941–2948, https://doi.org/10.1002/2017GL072617, 2017.
Zhao, B., Jiang, J. H., Gu, Y., Diner, D., Worden, J., Liou, K.-N., Su, H., Xing, J., Garay, M., and Huang, L.: Decadal-scale trends in regional aerosol particle properties and their linkage to emission changes, Environ. Res. Lett., 12, 054021, https://doi.org/10.1088/1748-9326/aa6cb2, 2017.
Zou, B., Chen, J., Zhai, L., Fang, X., and Zheng, Z.: Satellite based mapping of ground PM2.5 concentration using generalized additive modeling, Remote Sens.-Basel, 9, 1, https://doi.org/10.3390/rs9010001, 2017.