the Creative Commons Attribution 4.0 License.
the Creative Commons Attribution 4.0 License.
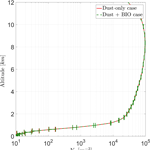
Simulating the influence of primary biological aerosol particles on clouds by heterogeneous ice nucleation
Matthias Hummel
Corinna Hoose
Bernhard Pummer
Caroline Schaupp
Janine Fröhlich-Nowoisky
Ottmar Möhler
Primary ice formation, which is an important process for mixed-phase clouds with an impact on their lifetime, radiative balance, and hence the climate, strongly depends on the availability of ice-nucleating particles (INPs). Supercooled droplets within these clouds remain liquid until an INP immersed in or colliding with the droplet reaches its activation temperature. Only a few aerosol particles are acting as INPs and the freezing efficiency varies among them. Thus, the fraction of supercooled water in the cloud depends on the specific properties and concentrations of the INPs. Primary biological aerosol particles (PBAPs) have been identified as very efficient INPs at high subzero temperatures, but their very low atmospheric concentrations make it difficult to quantify their impact on clouds.
Here we use the regional atmospheric model COSMO–ART to simulate the heterogeneous ice nucleation by PBAPs during a 1-week case study on a domain covering Europe. We focus on three highly ice-nucleation-active PBAP species, Pseudomonas syringae bacteria cells and spores from the fungi Cladosporium sp. and Mortierella alpina. PBAP emissions are parameterized in order to represent the entirety of bacteria and fungal spores in the atmosphere. Thus, only parts of the simulated PBAPs are assumed to act as INPs. The ice nucleation parameterizations are specific for the three selected species and are based on a deterministic approach. The PBAP concentrations simulated in this study are within the range of previously reported results from other modeling studies and atmospheric measurements. Two regimes of PBAP INP concentrations are identified: a temperature-limited and a PBAP-limited regime, which occur at temperatures above and below a maximal concentration at around −10 ∘C, respectively. In an ensemble of control and disturbed simulations, the change in the average ice crystal concentration by biological INPs is not statistically significant, suggesting that PBAPs have no significant influence on the average state of the cloud ice phase. However, if the cloud top temperature is below −15 ∘C, PBAP can influence the cloud ice phase and produce ice crystals in the absence of other INPs. Nevertheless, the number of produced ice crystals is very low and it has no influence on the modeled number of cloud droplets and hence the cloud structure.
- Article
(7980 KB) - Full-text XML
- BibTeX
- EndNote
The initial formation of ice crystals in mixed-phase clouds is catalyzed by ice-nucleating particles (INPs) at certain temperatures and relative humidities, depending on the properties of the aerosol particle acting as the INP (Hoose and Möhler, 2012). It is the current consensus that only a small proportion of all atmospheric aerosol particles can act as INPs. Among these are certain types of mineral dust and metallic and combustion particles (Murray et al., 2012). Some primary biological aerosol particles (PBAPs) are found to be ice nucleation active in the immersion freezing mode, i.e., initiating freezing from the inside of a supercooled liquid cloud droplet. When comparing the freezing onset temperatures, some ice-nucleation-active bacteria and fungi are among the most active INPs present in the Earth's atmosphere (Després et al., 2012; Hoose and Möhler, 2012; Murray et al., 2012; Morris et al., 2013).
PBAPs are a ubiquitous group of the Earth's atmospheric aerosols and are defined as solid and insoluble particles of biological origin (Després et al., 2012; Elbert et al., 2007; Fröhlich-Nowoisky et al., 2016). PBAPs include microorganisms (e.g., bacteria) as well as reproductive units from the biosphere (e.g., fungal spores, plant pollen) (Després et al., 2012). Single bacteria cells and agglomerates typically have the size of 0.25–3 and 3–8 µm in diameter, respectively, whereas actively wet discharged ascospores1 are usually 2–20 µm and actively wet discharged basidiospores1 are usually 1–10 µm in aerodynamic diameter (Elbert et al., 2007; Gregory, 1961).
Table 1Physical characteristics of the different PBAP types used for the model simulation of this study. a and b are the semiaxes of the prolate spheroid.

1 Hinds (1999), Lamanna et al. (1973). 2 Schaupp (2013). 3 Hummel et al. (2015). 4 Haga et al. (2013).
Ice-nucleation-active PBAPs potentially influence cloud microphysical properties by increasing ice formation at high subzero temperatures (DeMott and Prenni, 2010), and subsequently also impact cloud dynamics. It has been hypothesized that a more effective glaciation of cloud droplets decreases the cloud lifetime and the cloud albedo (termed glaciation indirect effect when referring to anthropogenic INPs (Boucher et al., 2013; Lohmann and Hoose, 2009). The presence of specific INPs in clouds can be observed indirectly by analyzing cloud and precipitation particle residuals (Cziczo et al., 2013). Biological particles have been observed ubiquitously in precipitation and snowpack at ground level (Christner et al., 2008; Lu et al., 2016; Stopelli et al., 2015), inside clouds by airborne measurements (DeLeon-Rodriguez et al., 2013; Pratt et al., 2009), and in cloud water collected at mountaintop stations (Joly et al., 2014). Furthermore, they have been shown to significantly contribute to INP concentrations measured in cloud-free air at ground level (Creamean et al., 2013; Huffman et al., 2013; Prenni et al., 2013).
Previous modeling studies suggest that primary biological INPs (PB-INPs) have a limited influence on clouds and precipitation on global average because the INP number concentrations of mineral dust and soot are found to be several orders of magnitude higher than the INP number concentration of PBAPs (Hoose et al., 2010b; Sesartic et al., 2012, 2013; Spracklen and Heald, 2014). However, these studies neglect constraints on the ice nucleation activity of nonbiological particles at high subzero temperatures ( ∘C), for which DeMott and Prenni (2010) suggest that PB-INPs are most important for ice nucleation in mixed-phase clouds. In this range, laboratory measurements of the ice nucleation activity of mineral dust and soot are scarce since nucleation rates are too low at high temperatures to be measured in the laboratory under representative conditions. However, the parameterizations used in previous model studies are extrapolated from measurements at lower temperatures. In contrast, most laboratory measurements of bacteria INPs are reported in this temperature range. Different parameterization approaches for these have been suggested and strongly impact model results (Sahyoun et al., 2016). Furthermore, in parameterizations based on field studies (Phillips et al., 2008), the attribution of the INP activity to different aerosol types is ambiguous. Secondary ice formation, e.g., from splinter production during ice-droplet collisions (Hallett and Mossop, 1974), has been suggested to be active at ∘C and could enhance the effect of biological ice nucleation. The model representation of these processes is uncertain. In regimes colder than −15 ∘C, mineral dust and other nonbiological INPs are also active and the relative abundance of PBAPs compared to inorganic particles is probably too small for PB-INPs to contribute significantly to ice formation. With the coarse horizontal and vertical resolution employed in global models, this narrow temperature window, in which PB-INPs are thought to be most effective, is poorly resolved. Also, local concentration maxima of biological particles close to source areas cannot be captured by global models.
In this study, we present mesoscale model simulations of PBAPs and their interactions with clouds with a grid spacing of 14 km. For this, a limited-area atmospheric model is extended by surface emissions, atmospheric dispersion, and heterogeneous ice nucleation for two different PBAPs (bacteria and fungal spores). Recent laboratory measurements of the ice-nucleating efficiency of different PBAPs are used to derive parameterizations for immersion freezing. The number concentrations of PB-INPs during a 1-week episode in July 2010 in Europe are analyzed and compared to background dust INP concentrations. Furthermore, the impact of PB-INPs on clouds is quantified in ensemble simulations with and without PBAPs acting as INPs.
2.1 Emission and dispersal of biological particles
The COSMO–ART (Consortium for Small-Scale Modeling–Aerosols and Reactive Trace Gases) regional atmospheric model system is based on the forecast model of the German weather service, combined with a two-way interaction module for simulating the spatial and temporal distribution of reactive gaseous and particulate components (Vogel et al., 2009). In this study, the treatment of two types of PBAPs has been included in the model. Bacteria and fungal spores are chosen because of their significant contributions to the PBAP mass and number (Després et al., 2012) and because highly ice-nucleation-active species have been identified within these groups (e.g., Maki et al., 1974 for Pseudomonas syringae; Fröhlich-Nowoisky et al., 2015 for Mortierella alpina). Pollen grains can also be simulated with COSMO–ART (Vogel et al., 2008; Zink et al., 2013). However, as their concentrations are significantly lower than bacteria and fungal spore concentrations, they are not considered any further in this work.
The physical properties of the simulated PBAPs are listed in Table 1. Bacteria and fungal spores are assumed to be monodisperse and spherical, except for the calculation of the sedimentation velocity, which assumes prolate spheroids (Hummel et al., 2015).
PBAPs are treated as inert tracers, neglecting any interactions with other aerosols or gases (coagulation or condensation), as well as fragmentation and bursting processes. The temporal development of the PBAP number concentration is calculated using the following prognostic equation:
with the number mixing ratio of PBAP . Here, Nf is the number concentration of PBAPs, N is the total number of particles and air molecules per volume of air, ρ is the air density, FT is the turbulent flux, FS is the sedimentation flux, λ is the washout coefficient, and FE is the vertical emission flux (Helbig et al., 2004; Vogel et al., 2008). FE is calculated separately for each type of PBAP by means of an emission parameterization: FE,B for bacteria and FE,FSP for fungal spores.
FE,B is the total emission flux of bacteria to the atmosphere. It consists of the sum of constant fluxes specified for particular ecosystems (Burrows et al., 2009). Each ecosystem-specific flux has been derived by adapting simulated bacteria concentrations to the observed near-surface bacteria number concentrations (Burrows et al., 2009). Therefore, the total bacteria emission flux is given by the sum of all individual emission fluxes multiplied by the tile fractions of the respective ecosystems (Hoose et al., 2010a). Descriptions of the ecosystem coverage used for the model simulation are given in Sect. 2.4.
The fungal spore emission is described by a flux FE,S depending on selected meteorological and ground parameters as derived from a previous model study with COSMO–ART (Hummel et al., 2015). In Hummel et al. (2015), the simulated fungal spore concentration has been adapted to local near-surface concentrations of measured fluorescent biological aerosol particles (FBAPs) in central and northern Europe. It is assumed that fungal spore concentrations may be best approximated by FBAP concentrations as the dominant FBAP size mode frequently coincides with typical fungal spore sizes (Huffman et al., 2012; Hummel et al., 2015; Pöschl et al., 2010). The FBAP emission flux has been fitted to measured FBAP concentrations with the assumption that the biological particles are evenly distributed throughout the boundary layer. As a result, the emission flux in m−2 s−1 is given by the following function:
where T is the surface temperature in K, qv the specific humidity in kilograms per kilogram, LAI the leaf area index in square meters per square meter, and b1=20.426 and are fit parameters (Hummel et al., 2015).
2.2 Parameterization of heterogeneous ice nucleation
Heterogeneous freezing of droplets containing an immersed PBAP is assumed to occur on specific ice-nucleation-active surface sites (INASs) at a characteristic temperature (Connolly et al., 2009; Pruppacher and Klett, 1997). For this approach, the surface density of ice-nucleation-active sites is labeled nS(T) and describes the number of surface sites that are ice nucleation active between 0 ∘C and T (Connolly et al., 2009). For monodisperse particles, the ratio fIN of PB-INPs to total PBAPs is then a function of nS(T) and the individual particle surface area AP calculated by
fIN(T) describes a functional form with a slope depending on nS(T) towards higher temperatures and that stays constant towards lower temperatures if fIN=1. The latter implies that every particle becomes activated as INP, which is not the case for some PB-INPs (Fig. 1b). Hence, fIN is modified by , where γ ≤ 1 describes the constant value when every potentially ice-nucleation-active PBAP is activated as an INP. Some PBAPs of the same species can remain non-activated, and γ can be estimated from the low-temperature tail of the laboratory results described here, where is approximately constant with T.
Taking this modification into account, a temperature-dependent INAS density is derived from different laboratory experiments. Pseudomonas syringae bacteria have been investigated at the AIDA cloud simulation chamber (Amato et al., 2015; Schaupp, 2013), data for Cladosporium sp. spores are derived with a flow cell with defined relative humidity and temperature (Iannone et al., 2011), and results for Mortierella alpina have been obtained by analyzing the washing water from the mycelium, which can contain spores (Fröhlich-Nowoisky et al., 2015). The measurements are also shown in Fig. 1. By least-square fitting, the parameters for the nS temperature spectrum can be derived:
including temperature T in K and parameters α and β and listed in Table 2. The parameterizations of nS(T) for different PBAPs and the consistent laboratory data from which they are derived are shown in Fig. 1a.
For INP descending from the species Mortierella alpina, we directly fit fIN, and no nS could be calculated because no size information was given with the measurements (Fröhlich-Nowoisky et al., 2015).
The upper temperature limit of the parameterization (Tmax, Table 2) is defined as the highest temperature at which ice nucleation with the same PBAP species as used for this study has been observed in any laboratory experiments, including others with a lower detection limit. Tmax may therefore be outside the temperature range covered by the laboratory data used for fitting the parameterization. An upper temperature limit is also given for Mortierella alpina but not used in the simulation as the slope of the parameterization is very steep close to Tmax and does not require a threshold.
Table 2Parameters for parameterizing the heterogeneous ice-nucleating ability of different PBAPs used in the model simulation of this study (Eqs. 3, 4, 5, 6).
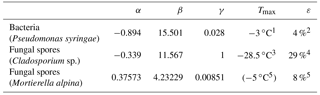
1 Amato et al. (2015). 2 Després et al. (2012). 3 Iannone et al. (2011). 4 Lang-Yona et al. (2012). 5 Fröhlich-Nowoisky et al. (2015).
The number concentration NbioIN of PB-INPs is calculated diagnostically by applying the parameterization as a function of T and PBAP number concentration Nf:
Here, ε is the ratio of potentially ice-nucleation-active PBAPs to all the PBAP species of the same type (here: bacteria or fungal spores). For example, potentially ice-nucleation-active bacteria are represented in this study by the species Pseudomonas syringae, which can have an abundance of up to 4 % of all bacteria in ambient air (Després et al., 2012). Therefore, as an upper estimate, ε is set to 0.04. Both types of ice nucleation fungal spores used here also represent only a subset of the total number of fungal spores in the atmosphere. Spores of the species Cladosporium are among the most abundant types of spores found in sampled air close to the ground (Iannone et al., 2011; Lang-Yona et al., 2012). A more recent study discovered Mortierella alpina to be particularly ice nucleation active at high temperatures (Fröhlich-Nowoisky et al., 2015). Here, their abundance in air was estimated from the frequency of CFUs (colonial forming units) found in the soil, which we assume to be fungal spores. Due to the different characteristics, both fungal spore types are included in the simulations. Conversely, most ice-nucleation-active bacteria show a “Pseudomonas-like” freezing behavior and are therefore included in the ε value selected here. It should be mentioned that estimations for ε have to be taken with extreme caution since (i) the microbial diversity is huge, with many species remaining undetectable, (ii) the activity of a species depends on strain, available nutrients, and growth conditions, which might be different for laboratory cultures, and (iii) only a few studies have been reproduced by more than one group with different methods.
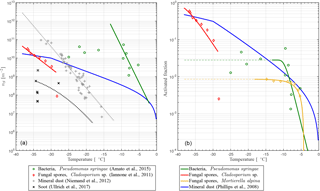
Figure 1(a) INAS density nS and (b) ice-active fraction fIN versus temperature T for PB-INPs (Pseudomonas syringae bacteria, Cladosporium sp., and Mortierella alpina fungal spores; rN of Table 1 used here) as well as mineral dust (Phillips et al., 2008) and soot (Ullrich et al., 2016) used in this study.
2.3 Cloud microphysics
A two-moment cloud microphysics scheme is used for calculating mass and number densities of cloud and precipitation particles separately (Seifert and Beheng, 2006). The size distribution of liquid drops is split into cloud droplets and raindrops at a defined mass threshold. Warm-phase processes refer to the formation and growth of cloud droplets and raindrops by nucleation, condensation, and collection of smaller droplets as well as to disruption by collisional breakup into fragments and evaporation at subsaturated regions (Seifert and Beheng, 2006). Within this chain of processes, nucleation of cloud droplets is represented by an aerosol activation parameterization depending on size distribution and chemical mixing state of aerosol particles, which can potentially act as cloud condensation nuclei (CCN) (Nenes and Seinfeld, 2003). PBAPs are not included in the CCN scheme because of their low number concentrations.
Within the processes including the ice phase, the scheme for nucleation of cloud ice has been modified in order to take the contribution of PB-INPs into account (Sect. 2.3). Hence, the number concentration of PB-INPs is added to the total INP number concentration before calculating the ice crystal concentration.
In the original scheme, the number concentration of cloud ice crystals with a prescribed minimum mass is given by a maximum supersaturation ratio that is calculated per grid box. Heterogeneous freezing considers the size distribution and the composition of a polydisperse aerosol population (Barahona and Nenes, 2009) and uses a parameterization by Phillips et al. (2008) for mineral dust and soot INPs. Insoluble organics as described by Phillips et al. (2009) potentially represent at least partly active ice-nucleating bacteria but are not included in the present model setup. Towards warmer temperatures, no upper threshold for heterogeneous freezing of mineral dust is defined in Phillips et al. (2008). In order to account for potential ice-nucleation-active site contributions from embedded organic compounds in the mineral dust parameterization, an upper temperature threshold of −15 ∘C is used for non-PBAP ice nucleation (DeMott and Prenni, 2010).
2.4 Model setup
The COSMO–ART mesoscale model system is driven by initial and boundary data for meteorological conditions. They are updated every 6 h and result from interpolation of the coarse-grid operational atmospheric model analysis of the ECMWF (European Centre for Medium-Range Weather Forecasts). No initial and boundary concentrations are predefined for aerosols or gases. Therefore, all gaseous species are set to climatological, homogeneously distributed initial concentrations. Emission rates for chemical compounds included in the ART module are updated hourly. They are provided by EMPA (Swiss Federal Laboratories for Materials Science and Technology) based on the TNO/MACC (Monitoring Atmospheric Composition and Climate) inventory (Kuenen et al., 2011). The treatment of emissions for COSMO–ART is described in Knote et al. (2011). Homogeneously distributed mass densities for each aerosol are used as initial conditions, together with initial size distributions. Primary particle emissions are parameterized based on meteorological and surface conditions. Land use data and constant surface properties are derived from the GLC2000 database (Bartholomé and Belward, 2005). All parameters are post-processed to the rotated spherical coordinate system of COSMO–ART (Doms and Schättler, 2002). For the purpose of this paper, the model domain covers most parts of western Europe from mainland Portugal to northern Finland, the longitudinal extension being 2849 km and the latitudinal extension being 3803 km with a horizontal spacing of 0.125∘ (14 km) on a rotated grid. A 1-week case study in July 2010 (22 to 29 July 2010) is simulated by the model. The time of year is chosen to cover the maximal concentrations for fungal spores and bacteria within their annual cycle, as they are expected to have the largest influence on cloud properties. In the vertical direction the model reaches up to an altitude of about 24 km distributed over 40 terrain-following levels. The time stepping of the Runge–Kutta dynamical core is set to 30 s. Model results are written to output files with a time resolution of 1 h.
Small changes in the present meteorological base state may influence the effects of PB-INPs on cloud properties. Therefore, an ensemble of five independent model runs has been created by shifting large-scale atmospheric fields in each horizontal direction (Sasse and Schädler, 2014; Schlüter and Schädler, 2010). In the present model setup, the domain is shifted by two grid boxes (28 km) in each direction. Two different cases are considered for all five ensemble members, a control run without PB-INPs (“DST”) and a modified case including all types of PB-INP as described in Sect. 2.3 (“BIO”). Influences of PB-INPs on cloud properties are given by differences of ensemble means between both cases (BIO − DST). Before calculating the ensemble means for each case, domain mean values (horizontally and temporally) after the model spin-up are calculated for each model run. The ensemble means are then used to compare both cases to each other. Error bars represent the standard deviation of the ensemble members.
In the DST case, mineral dust and soot are available INPs for heterogeneous freezing. The setup requires prescribed concentrations for mineral dust because no desert, as a requirement for mineral dust emission, is covered by the model domain. Therefore, mineral dust is set to a constant and homogeneously distributed number concentration of 100 L−1, adapted to typical atmospheric background concentrations for mineral dust in Europe, when no dust is transported from the Sahara desert towards Europe (Klein et al., 2010; Stanelle et al., 2010). A recent study about seasonal variations in desert dust confirms this value for a summertime background concentration over Europe (Hande et al., 2015). Soot concentrations are included in the COSMO–ART simulation by prescribed emission rates (Vogel et al., 2009).
3.1 PBAP concentrations
The horizontally distributed bacteria and fungal spore number concentrations at the lowest model layer (∼10 m above ground) are shown in Figs. 2 and 3 for the selected case study to illustrate the order of magnitude of aerosol concentrations that might serve as INPs. Bacteria concentrations (Fig. 2) over land are of the order of ∼ 10 L−1 (6 L−1 and much lower (< 1 L−1) over the ocean). As bacteria emissions are not defined for sea surfaces, land-emitted bacteria can only be transported there and decrease with distance from the shore due to sedimentation. The emission of bacteria in this study is constant with time; therefore, the number and distribution of bacteria concentrations are only dependent on transport and removal processes. At surface level, the simulated fungal spore concentrations (Fig. 3) are always higher than the bacteria concentrations. Typical fungal spore concentrations over land are between ∼ 10 and 100 L−1, which is in agreement with the range given in other studies (Després et al., 2012). In this study, fungal spore concentrations increase towards southern latitudes of the model domain because the time-dependent emission parameterization also depends on temperature and specific humidity, which are usually higher towards southern latitudes (on the Northern Hemisphere). The highest fungal spore concentrations occur in the Italian Po Valley, where it is wet and warm and there is a high amount of vegetated or agricultural area. Horizontal distribution of bacteria and fungal spores in the lowest, surface-most model layer strongly depends on present meteorological conditions, i.e., wind direction and intensity, advection, and emission drivers.
Figure 4 shows a vertical profile of PBAP and INP number concentration. Before calculating the horizontal mean values, a vertical model layer interpolation over equal heights is performed to compensate for the layer deformation due to model topography. Additionally, all data points that are included in the averaged lines are shown in a scatter cloud. This highlights the wide range of PBAP concentrations that occur especially in the upper model layers. Due to strong removal processes, the background concentration is very low in the middle layer of the atmosphere, and thus higher concentrations are mainly given by uplifting events that are stronger than the average.
The vertically distributed average concentrations of bacteria and fungal spores are similar to each other and show a decrease by about 3 orders of magnitude between the surface level (∼ 10 L−1) and the tropopause layer at ∼ 10 km (Fig. 4). The figure additionally shows the vertical distribution of mineral dust INPs and PB-INPs, which will be described in detail in the following sections.
3.2 Diagnostic INP
As a first step, the potential influence of PB-INPs on clouds through the immersion freezing of cloud droplets is estimated by analyzing the diagnostic INP concentrations. The term “diagnostic” refers to simulations without feedback between PB-INPs and clouds (see Sect. 3.3). The concentration of diagnostic PB-INPs (NbioIN) at each grid point of the model domain results from the parameterization for ice nucleation by PBAPs applied to the temperature and concentration of PBAPs (Nf) in the current grid box (Eq. 6).
Influences of PB-INPs on cloud properties by immersion freezing require supercooled liquid cloud droplets. Therefore, all results of INP concentrations refer to in-cloud conditions. Horizontal averaged PBAP and INP concentrations, resulting in a vertical profile (Fig. 4), can represent their abundance at cloud altitudes and thus potential impacts on cloud ice crystal concentration.
Total PB-INPs show the highest number concentrations at around 5 km above the surface. This results from the highest activity of the most efficient types of PB-INPs, i.e., bacteria. Additionally, two small maxima at (i) 1.6 km and (ii) 9 km occur due to (ii) less active types of PB-INPs, i.e., Cladosporium fungal spores and (i) slightly more active types of PB-INPs with lower concentrations than the bacteria, i.e., Mortierella fungal spores. A detailed analysis is shown in the following. A decrease of PB-INPs above the topmost peak at 9 km towards higher model levels is caused by transition from heterogeneous to homogeneous ice nucleation. For comparison, the other INPs used in the model, mineral dust and soot, are also included in Fig. 4. While dust INPs reach their highest concentrations at 7.5 km, the concentrations above 4 km are already within 1 order of magnitude of the maximum value. Below a height of 4 km, the temperature criterion for heterogeneous freezing of mineral dust INPs is rarely met. Hence, below 3 km only PB-INPs are activated.
In the following part, diagnostic INPs are shown in 2-D histograms for a more detailed view on the distribution of their concentration. Figures 5 and 6 show the 2-D histograms of bacteria INPs and fungal spore INPs with their concentration versus grid box temperature. Note that the model level thickness decreases with height, which results in higher temperatures occurring more often. A 2-D histogram avoids calculating mean or median values, which would be dominated by large regions with many low INP concentrations. The color of each box represents the frequency of occurrence of INPs at a certain concentration and temperature.
Both histograms show that maximal INP concentrations clearly decrease towards lower temperatures but also slightly towards the upper temperature threshold. Thus, the highest INP concentrations of 10−3 L−1 occur at −9 ∘C in the case of bacteria INPs and at −5 ∘C in the case of fungal spore INPs. Additionally, the abundance of INP decreases towards lower temperatures, which causes a decrease in mean concentration (solid line) in Fig. 4. This effect interferes with a larger extent of the model levels at higher altitudes and hence fewer data points at low temperatures (less dense scatter cloud in Fig. 4). However, decreasing PB-INP concentrations at low temperatures are mainly caused by a lack of ice-nucleation-active PBAPs. Therefore, both bacteria INPs and fungal spore INPs, decrease by about 2 to 3 orders of magnitude between −10 ∘C and −35 ∘C. This temperature region can be called “PBAP limited”.
At temperatures above −10 ∘C, the frequency of occurrence of concentration values differs slightly between both types of PB-INPs. The maximum concentration of bacteria INPs decreases by about 2 orders of magnitude between −9 and −2 ∘C. At −2 ∘C, a sharp edge occurs because the parameterization for ice nucleation is limited to temperatures below −2 ∘C. But still L−1 of bacteria can act as INPs at this temperature threshold. Together with high bacteria concentrations, which appear frequently at this temperature, a sharp edge between high and very abundant bacteria INP concentrations and zero INPs occurs. In contrast, the concentrations of fungal spore INPs decrease to a lesser degree and in a narrow temperature range (between −5 and −2 ∘C). A different mathematical description of heterogeneous ice nucleation with a continuously decreasing slope diminishes the sharp edge at the maximum temperature threshold. For both types of PB-INPs analyzed here, bacteria INPs and fungal spore INPs, concentrations decrease towards their upper temperature threshold because the parameterization for heterogeneous ice nucleation decreases more strongly than its PBAP concentrations increase. This region can be described as “temperature limited” because both parameterizations for heterogeneous ice nucleation are highly temperature dependent.
3.3 Prognostic INP
So called “prognostic” INPs are able to effect cloud ice crystal number concentration (Ni) and mass mixing ratio within the model. Thus, many different cloud processes, i.e., riming, aggregation to snow, ice multiplication, or melting, can be affected. In addition to heterogeneous ice nucleation, other microphysical processes can increase Ni, i.e., homogeneous ice nucleation or secondary ice multiplication.
In order to quantify the total effect of PB-INPs on cloud properties, vertical profiles of the ensemble means are calculated and compared in the further analysis (Sect. 2.4).
The vertical profile of average prognostic in-cloud INP concentrations (NIN) in Fig. (7) shows two stages. Below 4 km only PB-INPs are active and thus only the case BIO gives prognostic INP concentrations here, which increase with height. Above 4 km, concentrations are higher by several orders of magnitude because other INPs, mainly mineral dust, are active too. Here, both cases are very similar to each other because PB-INPs only cause a minor change to these high INP concentrations. Error bars give the standard deviation of the ensemble members. As the pure PB-INPs below a height of 3 km are a few orders of magnitude lower than total INP concentrations at around 8 km, the differences between both cases (ΔNIN) reflect this wide range too. Values of ΔNIN are a few orders of magnitude lower below 3 km in height than above 4 km in height. The latter has a maximum around 100 m−3, which is even larger than the PB-INP concentration as an initial perturbation of the simulation. Taking the variations among the ensembles into account, high values of ΔNIN above 4 km are not necessarily caused by this perturbation.
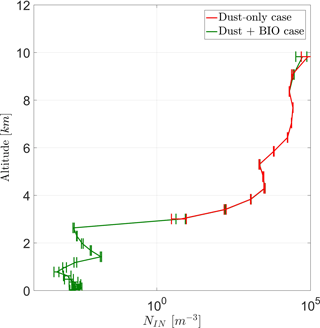
Figure 7Vertical profile for total prognostic in-cloud INPs (NIN) for a case with and without PB-INPs (cases BIO and DST).
With the current model setup, vertical profiles of average ice crystals (Ni) for both cases show a maximum concentration at an altitude of ∼ 8 km (Fig. 8) of about 1×105 m−3. Above 9 km, Ni is reduced with height, as fewer ice clouds are present and the tropopause is reached. Between 1 and 9 km, Ni steadily increases with increasing height. Below a height of 1 km, Ni is very low because melting reduces its number in many regions of the model domain. Except this low area, the standard deviation of the ensemble members is below 5 %.
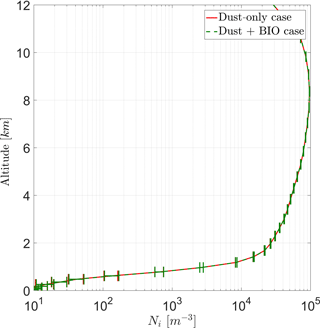
Figure 8Vertical profile of ice crystal number concentration (Ni) averaged over the entire domain for both cases (DST and BIO).
As both cases (DST and BIO) are almost equal to each other on average, the difference in Ni between both cases (BIO − DST) is given in Fig. 9. Positive values indicate that INP concentrations are higher in case BIO. It shows that mean Ni is almost always enhanced due to PB-INPs, but not significantly. Although ΔNi is mainly positive, wide error ranges suggest that differences could also be caused by ensemble variations. Nevertheless, the largest differences in the ice crystal concentration (ΔNi) are around 100 m−3 and thus similar to differences in INPs, but both are well below the error bars.
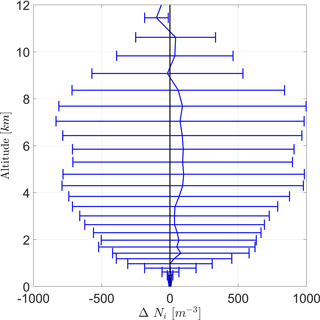
Figure 9Vertical profile of the difference of the ice crystal number concentration (ΔNi) between the cases BIO and DST with the same configuration for averaging as Fig. 8.
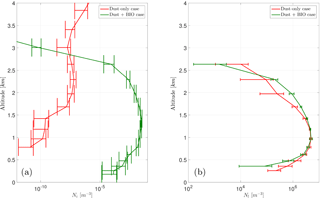
Figure 10Vertical profile of the ice crystal number concentration (a, Ni) and the cloud droplet number (b, Nl) for the cases DST and BIO for an exemplary section over Iceland.
Although PB-INPs on average over the model domain have no significant effect on the ice crystal concentration, they can have some effect under certain conditions, like shallow mixed-phase clouds (i.e., altostratus) with a cloud-top temperature above −15 ∘C. In the selected case shown here, an area over Iceland, the cloud mainly consists of liquid droplets with almost no ice crystals. When focusing on these conditions together with reasonably large PBAP concentrations, PB-INPs can create some ice nucleation and thus increase the vertical profile of Ni (Fig. 10). However, the number of ice crystals is still very low and the structure of the cloud is probably unchanged, as shown by the vertical profile of Nc which is remains without changes for case “BIO” compared to case ”CTL”. Hence, PB-INP can influence the cloud ice phase, but in a very limited manner.
PBAPs have been implemented in the regional model COSMO–ART by means of individual emission and freezing parameterizations to study their role as INPs for mixed-phase clouds. Compared to previous studies on a global scale (Hoose et al., 2010b), a regional model can utilize its higher resolution to observe potentially large temporal and spatial variations in PBAP concentration, which might be able to show a potential influence on the cloud ice phase more clearly.
For the simulation of PBAP concentrations, validated and previously tested emission parameterizations from the literature have been used (Burrows et al., 2009; Hummel et al., 2015). Still, the emission fluxes and atmospheric concentrations hold substantial uncertainties due to unknown or unresolved drivers in the PBAP release mechanism. In the case of bacteria, the emission function is evaluated on a global scale (Burrows et al., 2009), which might not be completely suitable to capture variations from regional-scale simulations. Our simulations predict higher near-surface concentrations for fungal spores than for bacteria. Within the range of uncertainty, the average near-surface concentrations of ∼ 10 L−1 shown here are of the same order of magnitude as results from previous modeling studies (Després et al., 2012; Hoose et al., 2010b). PBAP concentrations decrease rapidly with height and are only present in much smaller amounts, on average 2 orders of magnitude lower, within mixed-phase clouds than near the surface. This reduction is mainly due to an effective washout process and sedimentation taking place for bacteria and fungal spores. Also, the spread in number concentrations increases with higher altitude, which makes it difficult to identify a domain-average influence of PB-INPs on clouds. However, not much is known about the interstitial and cloud-borne concentrations of PBAPs or biological components. Evidence for biological components in cloud water is the presence of bacteria cells in rainwater samples, focusing on Pseudomonas sp. The investigations by Lu et al. (2016) show that Pseudomonas sp. is rarely present within rainwater samples collected over eastern China during summer. Similar to findings in this study, Lu et al. (2016) show that the frequency of bacterial INPs within the cumulative ice nucleation spectrum is very low. However, filtration and heat treatment experiments by Lu et al. (2016) clearly proved the existence of bacterial INPs. Further investigations in this research field would be very valuable for further studies about PB-INPs.
PBAPs have diverse properties. So far only a small fraction is discovered to be ice nucleation active and can therefore act as PB-INPs. The share of PB-INPs within the total PBAPs is one of the largest uncertainties (represented by ε) of this study. It is also suggested that PB-INPs can break up or burst and spread their ice-nucleation-active sites among many fragments (Diehl et al., 2002; Pummer et al., 2012, 2013). These processes have only been investigated on the microscale, so their contribution on the global scale is currently impossible to be even roughly estimated. Additionally, the temperature threshold that defines the highest temperature at which a certain PB-INP is ice nucleation active has been implemented into the deterministic parameterization used here. At this threshold, high PB-INP concentrations have the largest frequency of occurrence and a shift of the threshold might be relevant for influencing the INP concentrations. However, we do not expect that the results of this study, in particular the impact of PB-INPs on the ice crystal concentration, change substantially due to a change in the temperature threshold. The threshold is often only defined by a few data points within the measurements that are underlying this study, so it would be interesting for future studies to also measure the freezing behavior of PB-INPs at high temperatures.
To distinguish significant variations in the cloud properties from model variations when comparing both cases (BIO and DST), an ensemble of simulations is performed. Compared to ice crystals, the number concentration of PB-INPs is much lower and therefore PB-INPs are not able to significantly affect the average state of the ice phase. Even at lower parts of a cloud, with 0 ∘C and where no nonbiological INPs are allowed to be active, ice crystals from upper layers of the cloud frequently perturb the lower layers due to sedimentation, and the changes in ice crystal concentrations in the simulations with PB-INPs are smaller than the standard deviation in the ensemble. However, this study shows that PB-INPs can create few ice crystals if a cloud with ∘C is not perturbed by falling ice crystals, so it only contains liquid droplets. Even in this situation, the cloud properties do not change substantially. Hence, and most frequently within this simulation, PB-INPs have no effect on the ice crystal concentration.
The simulation output used to generate the figures of this paper is freely available for download in the institutional repository KITopenData (Hummel and Hoose, 2018).
MH and CH designed the study. MH selected the model setup, defined and implemented the parameterizations, and ran the simulations. MH and CH analyzed the simulation output. CS and OM provided laboratory ice nucleation data for bacteria and BP and JFN for the fungus M. alpina. MH wrote the paper, and all other authors edited the paper.
The authors declare that they have no conflict of interest.
The authors wish to thank Marco Paukert and Max Bangert for their help and
support with the model and also Isabelle Steinke and Romy Fösig for
their valuable discussions. The first author wants to acknowledge Frank
Schwarz and Romy Fösig for technical assistance. We acknowledge support
from the Deutsche Forschungsgemeinschaft and the Open Access Publishing Fund of
the Karlsruhe Institute of Technology. This research was funded by the
Helmholtz Association through the Helmholtz Climate Initiative REKLIM and the
President's Initiative and Networking Fund and by DFG through project HO
4612/1-1 (FOR 1525 INUIT). The AIDA ice nucleation experiments were funded by
the German Science Foundation (DFG) through the project BIOCLOUDS
(MO 668/2-1). Janine Fröhlich-Nowoisky and Bernhard G. Pummer acknowledge
support from the Deutsche Forschungsgemeinschaft (DFG FR3641/1-2,
FOR 1525 INUIT).
The article processing charges for this open-access
publication were covered by a Research
Centre of the Helmholtz Association.
Edited by: David Topping
Reviewed by: one anonymous referee
Amato, P., Joly, M., Schaupp, C., Attard, E., Möhler, O., Morris, C. E., Brunet, Y., and Delort, A.-M.: Survival and ice nucleation activity of bacteria as aerosols in a cloud simulation chamber, Atmos. Chem. Phys., 15, 6455–6465, https://doi.org/10.5194/acp-15-6455-2015, 2015. a, b
Barahona, D. and Nenes, A.: Parameterizing the competition between homogeneous and heterogeneous freezing in ice cloud formation – polydisperse ice nuclei, Atmos. Chem. Phys., 9, 5933–5948, https://doi.org/10.5194/acp-9-5933-2009, 2009. a
Bartholomé, E. and Belward, A. S.: GLC2000: a new approach to global land cover mapping from Earth observation data, Int. J. Remote Sens., 26, 1959–1977, https://doi.org/10.1080/01431160412331291297, 2005. a
Boucher, O., Randall, D., Artaxo, P., Bretherton, C., Feingold, G., Forster, P., Kerminen, V.-M., Kondo, Y., Liao, H., Lohmann, U., Rasch, P., Satheesh, S., Sherwood, S., Stevens, B., and Zhang, X.: Clouds and Aerosols, book section 7, 571–658, Cambridge University Press, Cambridge, UK and New York, NY, USA, 2013. a
Burrows, S. M., Elbert, W., Lawrence, M. G., and Pöschl, U.: Bacteria in the global atmosphere – Part 1: Review and synthesis of literature data for different ecosystems, Atmos. Chem. Phys., 9, 9263–9280, https://doi.org/10.5194/acp-9-9263-2009, 2009. a, b, c, d
Christner, B. C., Cai, R., Morris, C. E., McCarter, K. S., Foreman, C. M., Skidmore, M. L., Montross, S. N., and Sands, D. C.: Geographic, seasonal, and precipitation chemistry influence on the abundance and activity of biological ice nucleators in rain and snow, P. Natl. Acad. Sci. USA, 105, 18854–18859, https://doi.org/10.1073/pnas.0809816105, 2008. a
Connolly, P. J., Möhler, O., Field, P. R., Saathoff, H., Burgess, R., Choularton, T., and Gallagher, M.: Studies of heterogeneous freezing by three different desert dust samples, Atmos. Chem. Phys., 9, 2805–2824, https://doi.org/10.5194/acp-9-2805-2009, 2009. a, b
Creamean, J. M., Suski, K. J., Rosenfeld, D., Cazorla, A., DeMott, P. J., Sullivan, R. C., White, A. B., Ralph, F. M., Minnis, P., Comstock, J. M., Tomlinson, J. M., and Prather, K. A.: Dust and Biological Aerosols from the Sahara and Asia Influence Precipitation in the Western U.S., Science, 339, 1572–1578, https://doi.org/10.1126/science.1227279, 2013. a
Cziczo, D. J., Froyd, K. D., Hoose, C., Jensen, E. J., Diao, M., Zondlo, M. A., Smith, J. B., Twohy, C. H., and Murphy, D. M.: Clarifying the Dominant Sources and Mechanisms of Cirrus Cloud Formation, Science, 340, 1320–1324, https://doi.org/10.1126/science.1234145, 2013. a
DeLeon-Rodriguez, N., Lathem, T. L., Rodriguez-R, L. M., Barazesh, J. M., Anderson, B. E., Beyersdorf, A. J., Ziemba, L. D., Bergin, M., Nenes, A., and Konstantinidis, K. T.: Microbiome of the upper troposphere: Species composition and prevalence, effects of tropical storms, and atmospheric implications, P. Natl. Acad. Sci. USA, 110, 2575–2580, https://doi.org/10.1073/pnas.1212089110, 2013. a
DeMott, P. J. and Prenni, A. J.: New Directions: Need for defining the numbers and sources of biological aerosols acting as ice nuclei, Atmos. Environ., 44, 1944–1945, 2010. a, b, c
Després, V. R., Huffman, J. A., Burrows, S. M., Hoose, C., Safatov, A. S., Buryak, G., Fröhlich-Nowoisky, J., Elbert, W., Andreae, M. O., Pöschl, U., and Jaenicke, R.: Primary biological aerosol particles in the atmosphere: a review, Tellus B, 64, 15598, https://doi.org/10.3402/tellusb.v64i0.15598, 2012. a, b, c, d, e, f, g, h
Diehl, K., Matthias-Maser, S., Jaenicke, R., and Mitra, S. K.: The ice nucleating ability of pollen: Part II. Laboratory studies in immersion and contact freezing modes, Atmos. Res., 61, 125–133, https://doi.org/10.1016/s0169-8095(01)00132-6, 2002. a
Doms, G. and Schättler, U.: A Description of the Nonhydrostatic Regional COSMO-Model, Deutscher Wetterdienst, Offenbach, Germany, 2002. a
Elbert, W., Taylor, P. E., Andreae, M. O., and Pöschl, U.: Contribution of fungi to primary biogenic aerosols in the atmosphere: wet and dry discharged spores, carbohydrates, and inorganic ions, Atmos. Chem. Phys., 7, 4569–4588, https://doi.org/10.5194/acp-7-4569-2007, 2007. a, b
Fröhlich-Nowoisky, J., Hill, T. C. J., Pummer, B. G., Yordanova, P., Franc, G. D., and Pöschl, U.: Ice nucleation activity in the widespread soil fungus Mortierella alpina, Biogeosciences, 12, 1057–1071, https://doi.org/10.5194/bg-12-1057-2015, 2015. a, b, c, d, e
Fröhlich-Nowoisky, J., Kampf, C. J., Weber, B., Huffman, J. A., Pöhlker, C., Andreae, M. O., Lang-Yona, N., Burrows, S. M., Gunthe, S. S., Elbert, W., Su, H., Hoor, P., Thines, E., Hoffmann, T., Després, V. R., and Pöschl, U.: Bioaerosols in the Earth system: Climate, health, and ecosystem interactions, Atmos. Res., 182, 346–376, https://doi.org/10.1016/j.atmosres.2016.07.018, 2016. a
Gregory, P. H.: The microbiology of the atmosphere, Leonard Hill, London, UK, 1–251, 1961. a
Haga, D. I., Iannone, R., Wheeler, M. J., Mason, R., Polishchuk, E. A., Fetch, T., Van Der Kamp, B. J., McKendry, I. G., and Bertram, a. K.: Ice nucleation properties of rust and bunt fungal spores and their transport to high altitudes, where they can cause heterogeneous freezing, J. Geophys. Res.-Atmos., 118, 7260–7272, https://doi.org/10.1002/jgrd.50556, 2013. a
Hallett, J. and Mossop, S. C.: Production of secondary ice particles during the riming process, Nature, 249, 26–28, 1974. a
Hande, L. B., Engler, C., Hoose, C., and Tegen, I.: Seasonal variability of Saharan desert dust and ice nucleating particles over Europe, Atmos. Chem. Phys., 15, 4389-4397, https://doi.org/10.5194/acp-15-4389-2015, 2015. a
Helbig, N., Vogel, B., Vogel, H., and Fiedler, F.: Numerical modelling of pollen dispersion on the regional scale, Aerobiologia, 20, 3–19, https://doi.org/10.1023/b:aero.0000022984.51588.30, 2004. a
Hoose, C. and Möhler, O.: Heterogeneous ice nucleation on atmospheric aerosols: a review of results from laboratory experiments, Atmos. Chem. Phys., 12, 9817–9854, https://doi.org/10.5194/acp-12-9817-2012, 2012. a, b
Hoose, C., Kristjánsson, J. E., and Burrows, S. M.: How important is biological ice nucleation in clouds on a global scale?, Environ. Res. Lett., 5, 24009, http://stacks.iop.org/1748-9326/5/i=2/a=024009 (last access: 7 August 2018), 2010a. a
Hoose, C., Kristjánsson, J. E., Chen, J.-P., and Hazra, A.: A Classical-Theory-Based Parameterization of Heterogeneous Ice Nucleation by Mineral Dust, Soot, and Biological Particles in a Global Climate Model, J. Atmos. Sci., 67, 2483–2503, https://doi.org/10.1175/2010jas3425.1, 2010b. a, b, c
Huffman, J. A., Sinha, B., Garland, R. M., Snee-Pollmann, A., Gunthe, S. S., Artaxo, P., Martin, S. T., Andreae, M. O., and Pöschl, U.: Size distributions and temporal variations of biological aerosol particles in the Amazon rainforest characterized by microscopy and real-time UV-APS fluorescence techniques during AMAZE-08, Atmos. Chem. Phys., 12, 11997–12019, https://doi.org/10.5194/acp-12-11997-2012, 2012. a
Huffman, J. A., Prenni, A. J., DeMott, P. J., Pöhlker, C., Mason, R. H., Robinson, N. H., Fröhlich-Nowoisky, J., Tobo, Y., Després, V. R., Garcia, E., Gochis, D. J., Harris, E., Müller-Germann, I., Ruzene, C., Schmer, B., Sinha, B., Day, D. A., Andreae, M. O., Jimenez, J. L., Gallagher, M., Kreidenweis, S. M., Bertram, A. K., and Pöschl, U.: High concentrations of biological aerosol particles and ice nuclei during and after rain, Atmos. Chem. Phys., 13, 6151–6164, https://doi.org/10.5194/acp-13-6151-2013, 2013. a
Hummel, M., Hoose, C., Gallagher, M., Healy, D. A., Huffman, J. A., O'Connor, D., Pöschl, U., Pöhlker, C., Robinson, N. H., Schnaiter, M., Sodeau, J. R., Stengel, M., Toprak, E., and Vogel, H.: Regional-scale simulations of fungal spore aerosols using an emission parameterization adapted to local measurements of fluorescent biological aerosol particles, Atmos. Chem. Phys., 15, 6127–6146, https://doi.org/10.5194/acp-15-6127-2015, 2015. a, b, c, d, e, f, g
Hummel, M. and Hoose, C.: Simulating the influence of primary biological aerosol particles on clouds by heterogeneous ice nucleation, KITopenData, https://doi.org/10.5445/IR/1000086437, 2018. a
Iannone, R., Chernoff, D. I., Pringle, A., Martin, S. T., and Bertram, A. K.: The ice nucleation ability of one of the most abundant types of fungal spores found in the atmosphere, Atmos. Chem. Phys., 11, 1191–1201, https://doi.org/10.5194/acp-11-1191-2011, 2011. a, b, c
Joly, M., Amato, P., Deguillaume, L., Monier, M., Hoose, C., and Delort, A.-M.: Quantification of ice nuclei active at near 0 ∘C temperatures in low-altitude clouds at the Puy de Dôme atmospheric station, Atmos. Chem. Phys., 14, 8185–8195, https://doi.org/10.5194/acp-14-8185-2014, 2014. a
Klein, H., Nickovic, S., Haunold, W., Bundke, U., Nillius, B., Ebert, M., Weinbruch, S., Schuetz, L., Levin, Z., Barrie, L. A., and Bingemer, H.: Saharan dust and ice nuclei over Central Europe, Atmos. Chem. Phys., 10, 10211–10221, https://doi.org/10.5194/acp-10-10211-2010, 2010. a
Knote, C., Brunner, D., Vogel, H., Allan, J., Asmi, A., Äijälä, M., Carbone, S., van der Gon, H. D., Jimenez, J. L., Kiendler-Scharr, A., Mohr, C., Poulain, L., Prévôt, A. S. H., Swietlicki, E., and Vogel, B.: Towards an online-coupled chemistry-climate model: evaluation of trace gases and aerosols in COSMO-ART, Geosci. Model Dev., 4, 1077–1102, https://doi.org/10.5194/gmd-4-1077-2011, 2011. a
Kuenen, J., Denier van der Gon, H., Visschedijk, A., van der Brugh, H., and Gijlswijk, R.: MACC European emission inventory for the years 2003–2007, tech. rep., Utrecht, the Netherlands, 2011. a
Lang-Yona, N., Dannemiller, K., Yamamoto, N., Burshtein, N., Peccia, J., Yarden, O., and Rudich, Y.: Annual distribution of allergenic fungal spores in atmospheric particulate matter in the Eastern Mediterranean; a comparative study between ergosterol and quantitative PCR analysis, Atmos. Chem. Phys., 12, 2681–2690, https://doi.org/10.5194/acp-12-2681-2012, 2012. a, b
Lohmann, U. and Hoose, C.: Sensitivity studies of different aerosol indirect effects in mixed-phase clouds, Atmos. Chem. Phys., 9, 8917–8934, https://doi.org/10.5194/acp-9-8917-2009, 2009. a
Lu, Z., Du, P., Du, R., Liang, Z., Qin, S., Li, Z., and Wang, Y.: The Diversity and Role of Bacterial Ice Nuclei in Rainwater from Mountain Sites in China, Aerosol Air Qual. Res., 16, 640–652, https://doi.org/10.4209/aaqr.2015.05.0315, 2016. a, b, c, d
Maki, L. R., Galyan, E. L., Chang-Chien, M.-M., and Caldwell, D. R.: Ice Nucleation Induced by Pseudomonas syringae, Am. Soc. Microbiol., 28, 456–459, 1974. a
Morris, C. E., Sands, D. C., Glaux, C., Samsatly, J., Asaad, S., Moukahel, A. R., Gonçalves, F. L. T., and Bigg, E. K.: Urediospores of rust fungi are ice nucleation active at > −10 ∘C and harbor ice nucleation active bacteria, Atmos. Chem. Phys., 13, 4223–4233, https://doi.org/10.5194/acp-13-4223-2013, 2013. a
Murray, B. J., O'Sullivan, D., Atkinson, J. D., and Webb, M. E.: Ice nucleation by particles immersed in supercooled cloud droplets, Chem. Soc. Rev., 41, 6519–6554, 2012. a, b
Nenes, A. and Seinfeld, J. H.: Parameterization of cloud droplet formation in global climate models, J. Geophys. Res., 108, 4415, https://doi.org/10.1029/2002jd002911, 2003. a
Phillips, V. T. J., DeMott, P. J., and Andronache, C.: An Empirical Parameterization of Heterogeneous Ice Nucleation for Multiple Chemical Species of Aerosol, J. Atmos. Sci., 65, 2757–2783, https://doi.org/10.1175/2007jas2546.1, 2008. a, b, c, d
Phillips, V. T. J., Andronache, C., Christner, B., Morris, C. E., Sands, D. C., Bansemer, A., Lauer, A., McNaughton, C., and Seman, C.: Potential impacts from biological aerosols on ensembles of continental clouds simulated numerically, Biogeosciences, 6, 987–1014, https://doi.org/10.5194/bg-6-987-2009, 2009. a
Pöschl, U., Martin, S. T., Sinha, B., Chen, Q., Gunthe, S. S., Huffman, J. A., Borrmann, S., Farmer, D. K., Garland, R. M., Helas, G., Jimenez, J. L., King, S. M., Manzi, A., Mikhailov, E., Pauliquevis, T., Petters, M. D., Prenni, A. J., Roldin, P., Rose, D., Schneider, J., Su, H., Zorn, S. R., Artaxo, P., and Andreae, M. O.: Rainforest Aerosols as Biogenic Nuclei of Clouds and Precipitation in the Amazon, Science, 329, 1513–1516, https://doi.org/10.1126/science.1191056, 2010. a
Pratt, K. A., DeMott, P. J., French, J. R., Wang, Z., Westphal, D. L., Heymsfield, A. J., Twohy, C. H., Prenni, A. J., and Prather, K. A.: In situ detection of biological particles in cloud ice-crystals, Nat. Geosci., 2, 398–401, 2009. a
Prenni, A. J., Tobo, Y., Garcia, E., DeMott, P. J., Huffman, J. A., McCluskey, C. S., Kreidenweis, S. M., Prenni, J. E., Pöhlker, C., and Pöschl, U.: The impact of rain on ice nuclei populations at a forested site in Colorado, Geophys. Res. Lett., 40, 227–231, https://doi.org/10.1029/2012gl053953, 2013. a
Pruppacher, H. R. and Klett, J. D.: Microphysics of clouds and precipitation, D. Reidel Pub. Co, Dordrecht, Holland and Boston, USA, available at: http://nla.gov.au/nla.cat-vn946461 (last access: 11 April 2014), 1997. a
Pummer, B. G., Bauer, H., Bernardi, J., Bleicher, S., and Grothe, H.: Suspendable macromolecules are responsible for ice nucleation activity of birch and conifer pollen, Atmos. Chem. Phys., 12, 2541–2550, https://doi.org/10.5194/acp-12-2541-2012, 2012. a
Pummer, B. G., Bauer, H., Bernardi, J., Chazallon, B., Facq, S., Lendl, B., Whitmore, K., and Grothe, H.: Chemistry and morphology of dried-up pollen suspension residues, J. Raman Spectrosc., 44, 1654–1658, https://doi.org/10.1002/jrs.4395, 2013. a
Sahyoun, M., Wex, H., Gosewinkel, U., Šantl-Temkiv, T., Nielsen, N. W., Finster, K., Sørensen, J. H., Stratmann, F., and Korsholm, U. S.: On the usage of classical nucleation theory in quantification of the impact of bacterial INP on weather and climate, Atmos. Environ., 139, 230–240, https://doi.org/10.1016/j.atmosenv.2016.05.034, 2016. a
Sasse, R. and Schädler, G.: Generation of regional climate ensembles using Atmospheric Forcing Shifting, Int. J. Climatol., 34, 2205–2217, https://doi.org/10.1002/joc.3831, 2014. a
Schaupp, C.: Untersuchungen zur Rolle von Bakterien und Pollen als Wolkenkondensations- und Eiskeime in troposphärischen Wolken, Ph.D. thesis, Heidelberg, Germany, 2013. a
Schlüter, I. and Schädler, G.: Sensitivity of Heavy Precipitation Forecasts to Small Modifications of Large-Scale Weather Patterns for the Elbe River, J. Hydrometeorol., 11, 770–780, https://doi.org/10.1175/2010jhm1186.1, 2010. a
Seifert, A. and Beheng, K. D.: A two-moment cloud microphysics parameterization for mixed-phase clouds. Part 1: Model description, Meteorol. Atmos. Phys., 92, 45–66, https://doi.org/10.1007/s00703-005-0112-4, 2006. a, b
Sesartic, A., Lohmann, U., and Storelvmo, T.: Bacteria in the ECHAM5-HAM global climate model, Atmos. Chem. Phys., 12, 8645–8661, https://doi.org/10.5194/acp-12-8645-2012, 2012. a
Sesartic, A., Lohmann, U., and Storelvmo, T.: Modelling the impact of fungal spore ice nuclei on clouds and precipitation, Environ. Res. Lett., 8, 14029, https://doi.org/10.1088/1748-9326/8/1/014029, 2013. a
Spracklen, D. V. and Heald, C. L.: The contribution of fungal spores and bacteria to regional and global aerosol number and ice nucleation immersion freezing rates, Atmos. Chem. Phys., 14, 9051–9059, https://doi.org/10.5194/acp-14-9051-2014, 2014. a
Stanelle, T., Vogel, B., Vogel, H., Bäumer, D., and Kottmeier, C.: Feedback between dust particles and atmospheric processes over West Africa during dust episodes in March 2006 and June 2007, Atmos. Chem. Phys., 10, 10771–10788, https://doi.org/10.5194/acp-10-10771-2010, 2010. a
Stopelli, E., Conen, F., Morris, C. E., Herrmann, E., Bukowiecki, N., and Alewell, C.: Ice nucleation active particles are efficiently removed by precipitating clouds, Sci. Rep., 5, 16433, https://doi.org/10.1038/srep16433, 2015. a
Ullrich, R., Hoose, C., Möhler, O., Niemand, M., Wagner, R., Höhler, K., Hiranuma, N., Saathoff, H., and Leisner, T.: A New Ice Nucleation Active Site Parameterization for Desert Dust and Soot, J. Atmos. Sci., 74, 699–717, https://doi.org/10.1175/JAS-D-16-0074.1, 2016. a
Vogel, B., Vogel, H., Bäumer, D., Bangert, M., Lundgren, K., Rinke, R., and Stanelle, T.: The comprehensive model system COSMO-ART – radiative impact of aerosol on the state of the atmosphere on the regional scale, Atmos. Chem. Phys., 9, 14483–14528, https://doi.org/10.5194/acpd-9-14483-2009, 2009. a, b
Vogel, H., Pauling, A., and Vogel, B.: Numerical simulation of birch pollen dispersion with an operational weather forecast system, Int. J. Biometeorol., 52, 805–814, https://doi.org/10.1007/s00484-008-0174-3, 2008. a, b
Zink, K., Pauling, A., Rotach, M. W., Vogel, H., Kaufmann, P., and Clot, B.: EMPOL 1.0: a new parameterization of pollen emission in numerical weather prediction models, Geosci. Model Dev., 6, 1961–1975, https://doi.org/10.5194/gmd-6-1961-2013, 2013. a
Spores of fungi from the phyla Ascomycota or Basidiomycota, respectively.