the Creative Commons Attribution 4.0 License.
the Creative Commons Attribution 4.0 License.
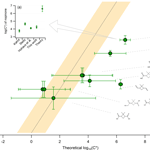
Gas-to-particle partitioning of major biogenic oxidation products: a study on freshly formed and aged biogenic SOA
Georgios I. Gkatzelis
Ralf Tillmann
Iulia Gensch
Markus Müller
Philipp Eichler
Kang-Ming Xu
Patrick Schlag
Sebastian H. Schmitt
Zhujun Yu
Robert Wegener
Martin Kaminski
Rupert Holzinger
Armin Wisthaler
Astrid Kiendler-Scharr
Secondary organic aerosols (SOAs) play a key role in climate change and air quality. Determining the fundamental parameters that distribute organic compounds between the phases is essential, as atmospheric lifetime and impacts change drastically between the gas and particle phase. In this work, gas-to-particle partitioning of major biogenic oxidation products was investigated using three different aerosol chemical characterization techniques. The aerosol collection module, the collection thermal desorption unit, and the chemical analysis of aerosols online are different aerosol sampling inlets connected to a proton-transfer reaction time-of-flight mass spectrometer (ACM-PTR-ToF-MS, TD-PTR-ToF-MS, and CHARON-PTR-ToF-MS, respectively, referred to hereafter as ACM, TD, and CHARON). These techniques were deployed at the atmosphere simulation chamber SAPHIR to perform experiments on the SOA formation and aging from different monoterpenes (β-pinene, limonene) and real plant emissions (Pinus sylvestris L.). The saturation mass concentration C* and thus the volatility of the individual ions was determined based on the simultaneous measurement of their signal in the gas and particle phase.
A method to identify and exclude ions affected by thermal dissociation during desorption and ionic dissociation in the ionization chamber of the proton-transfer reaction mass spectrometer (PTR-MS) was developed and tested for each technique. Narrow volatility distributions with organic compounds in the semi-volatile (SVOCs – semi-volatile organic compounds) to intermediate-volatility (IVOCs – intermediate-volatility organic compounds) regime were found for all systems studied. Despite significant differences in the aerosol collection and desorption methods of the proton-transfer-reaction (PTR)-based techniques, a comparison of the C* values obtained with different techniques was found to be in good agreement (within 1 order of magnitude) with deviations explained by the different operating conditions of the PTR-MS.
The C* of the identified organic compounds were mapped onto the two-dimensional volatility basis set (2D-VBS), and results showed a decrease in C* with increasing oxidation state. For all experiments conducted in this study, identified partitioning organic compounds accounted for 20–30 % of the total organic mass measured from an aerosol mass spectrometer (AMS). Further comparison between observations and theoretical calculations was performed for species found in our experiments that were also identified in previous publications. Theoretical calculations based on the molecular structure of the compounds showed, within the uncertainties ranges, good agreement with the experimental C* for most SVOCs, while IVOCs deviated by up to a factor of 300. These latter differences are discussed in relation to two main processes affecting these systems: (i) possible interferences by thermal and ionic fragmentation of higher molecular-weight compounds, produced by accretion and oligomerization reactions, that fragment in the m∕z range detected by the PTR-MS and (ii) kinetic influences in the distribution between the gas and particle phase with gas-phase condensation, diffusion in the particle phase, and irreversible uptake.
- Article
(1889 KB) - Full-text XML
-
Supplement
(787 KB) - BibTeX
- EndNote
Secondary organic aerosol (SOA), formed by chemical reactions in the atmosphere, constitutes a major fraction of organic aerosol (OA; Jimenez et al., 2009) and thus plays a key role in climate change and air quality. A detailed understanding of SOA formation and composition is critical to developing strategies for impact mitigation (Volkamer et al., 2006; de Gouw et al., 2008; Hallquist et al., 2009; Jimenez et al., 2009). Defining the fundamental parameters that distribute organic molecules between the gas and particle phases is essential, as atmospheric lifetime and impacts change drastically between phases. The saturation vapor pressure (Pankow, 1994) and the enthalpies of vaporization and sublimation are key thermodynamic properties describing the gas-to-particle partitioning of organic compounds. Since SOA consists predominantly of oxidized multifunctional compounds (McFiggans et al., 2010), it is expected to show low-saturation vapor pressures, thus increasing the detection challenges due to the low gas-phase concentrations that need to be probed (Bilde et al., 2015).
Advanced instrumentation for defining the saturation vapor pressure and thus the volatility of single component and complex organic aerosol systems has been developed in the past decades both for laboratory and field studies. Dicarboxylic acids represent a class of low-volatility compounds commonly found in atmospheric aerosol that are commercially available. These molecules have been extensively studied by various techniques (Bilde et al., 2015). The Knudsen effusion mass spectrometry (KEMS) (Booth et al., 2009) is a method where macroscopic crystalline samples effuse in a Knudsen cell and the change in the concentration in the gas phase is measured using a mass spectrometer and translated to saturation vapor pressure values based on calibrated standards. Single-particle methods using optical tweezers (Mitchem and Reid, 2008) and the electrodynamic balance (EDB) (Pope et al., 2010) infer saturation vapor pressure values from the evaporation or condensational growth of a single particle in a controlled environment. Thermal desorption mass spectrometry (TDMS) has extended the studies from laboratory to ambient complex polydisperse systems. Thermodenuders have been extensively used to quantify the volatility of the bulk OA (An et al., 2007; Huffman et al., 2008; Faulhaber et al., 2009; Gkatzelis et al., 2016; Louvaris et al., 2017; Isaacman-VanWertz et al., 2017) with the support of model calculations (Riipinen et al., 2010; Karnezi et al., 2014). However, the detector used in most of these studies is an aerosol mass spectrometer (AMS) (Canagaratna et al., 2007) that operates at high vaporizer temperatures (600 ∘C) and ionizes the analytes by electron impact (70 eV), thus introducing excessive thermal and ionic dissociation.
Recently, several different methods have been developed that represent a compromise between molecular-level information for a small fraction of the OA mass (Williams et al., 2006, 2014; Kreisberg et al., 2009; Hohaus et al., 2010; Zhang et al., 2014) and chemical formula identification using soft-ionization mass spectrometry to achieve a nearly full OA characterization (Lopez-Hilfiker et al., 2014; Isaacman-VanWertz et al., 2017; Stark et al., 2017; Gkatzelis et al., 2018). Volatility measurements are performed either by calibrating with standards of known saturation vapor pressure (Lopez-Hilfiker et al., 2014, 2015) or by the simultaneous measurement of the gas and particle phase of an ion (Hohaus et al., 2015; Isaacman-VanWertz et al., 2016; Stark et al., 2017).
In order to identify the OA on a molecular level, thermal desorption techniques have been coupled to gas chromatography (GC) methods. The thermal desorption aerosol gas chromatograph–mass spectrometer (2D-TAG) (Goldstein et al., 2008) and the volatility and polarity separator (VAPS) (Martinez et al., 2016) are similar techniques that provide volatility- and polarity-resolved OA information by using a two-dimensional gas chromatography (2D-GC) approach. Volatility is derived based on the two-dimensional chromatographic retention times relative to those of known standards, thus establishing a retention time correlation (RTC) to the vapor pressure. Simultaneous measurements of the gas- and particle-phase mass of organic molecules have also been recently developed using the thermal desorption aerosol gas chromatograph (TAG) system sampling alternately with and without a gas-phase denuder in front of the inlet (Zhao et al., 2013b) and the modified semi-volatile TAG (SV-TAG) that utilizes two TAG cells in parallel (Isaacman-VanWertz et al., 2016). Although the above GC methods provide chemical speciation and gas-to-particle partitioning at a molecular level, they can only do so for a small fraction of the OA mass (10–40 %) (Williams et al., 2006, 2016).
Measurements using instrumentation to provide molecular identification (e.g., SV-TAG) or instrumentation for the identification of ions (e.g., different chemical ionization mass spectrometers, CIMSs) can be combined to increase the understanding of the partitioning of some compounds classes. This was shown in a field intercomparison investigating gas-particle partitioning of oxygenated volatile organic compounds (OVOCs) during the Southern Oxidant and Aerosol Study (SOAS) (Thompson et al., 2017).
Newly developed thermal desorption inlets have allowed the near-simultaneous chemical characterization of gas- and particle-phase ambient compounds (Holzinger et al., 2010; Lopez-Hilfiker et al., 2014; Yatavelli et al., 2014; Eichler et al., 2015; Stark et al., 2017; Gkatzelis et al., 2018). When coupled to chemical ionization high-resolution time-of-flight mass spectrometers (HR-ToF-CIMS), these inlets can provide information on a very broad volatility range (Eichler et al., 2017). By simultaneous measurement of the gas- and particle-phase mass concentration when applicable, direct volatility calculations of individual species can be performed. Indirect ways of estimating the vapor pressure for this type of system has also been established based on the desorption temperature of calibrated known species or mixtures (Lopez-Hilfiker et al., 2016; Stark et al., 2017). Since the above mass spectrometric techniques can provide elemental formulas, methods to derive the vapor pressure by assuming a functional group composition have also been widely used (Pankow and Asher, 2008; Krechmer et al., 2015; Daumit et al., 2013; Li et al., 2016). A detailed comparison of the three different methods of estimating the vapor pressure for this type of techniques has been performed for field studies under forested areas (Stark et al., 2017). Results suggested that thermal decomposition pathways could bias the direct partitioning calculation based on the gas- and particle-phase concentrations as well as calculations based on the chemical formula of the species detected. A detailed understanding of the decomposition pathways is to be determined in future studies.
There are two major ways established in the last years to treat partitioning for practical applications to atmospheric aerosol. One is through a thermodynamic model containing an ensemble of specific molecules while the other is based on empirical calculations (Donahue et al., 2014). When using explicit methods, model systems are treated as fully as possible and thus individual vapor pressures and activity coefficients are calculated based on several thermodynamic schemes (Fredenslund et al., 1975; Clegg et al., 2001; Zuend et al., 2011). These calculations are strongly affected by the wide range of vapor pressure estimates from the different theoretical approaches (Camredon et al., 2010; Donahue et al., 2014), thus further indicating the need for future development in this field. By contrast, empirical methods tend to simulate gas-to-particle partitioning based on fits of partitioning data derived from chamber observations. Frameworks like the two-dimensional volatility basis set (2D-VBS) classify OA in terms of bulk chemical characteristics and volatility (Donahue et al., 2012, 2013). A variety of the above, newly developed techniques can be mapped onto the 2D-VBS and thus provide important experimental input to further develop and test both the empirical methods and the newly developed instrumentation.
Deviations between the theoretical and experimental vapor pressure estimates are systematically observed (Bilde et al., 2015). Recent measurements show a greater enrichment of semi-volatile organic compounds in the particle phase relative to the gas phase than calculations based on equilibrium vapor pressure would suggest (Zhao et al., 2013a; Hohaus et al., 2015; Isaacman-VanWertz et al., 2016). It is currently unclear whether this is due to (i) uncertainties in the theoretical estimates of vapor pressures, (ii) thermal decomposition pathways affecting the experimental partitioning determination, or (iii) the existence of uptake pathways to particles other than absorption, e.g., adsorption or reactive uptake. The wide range of theoretical vapor pressure estimates combined with the large gas-to-particle partitioning discrepancies of the above techniques (Thompson et al., 2017) encourage further studies in order to bridge the gap between theory and experiments.
In this study, the gas-to-particle partitioning of major biogenic SOA (BSOA) oxidation products was investigated. An intercomparison was performed using three different inlet techniques connected to soft-ionization mass spectrometry, the aerosol collection module (Hohaus et al., 2010), the chemical analysis of aerosols online (Eichler et al., 2015), and the collection thermal desorption unit (Holzinger et al., 2010). Volatility measurements were derived based on the mass concentration of individual species in the gas and particle phase, implemented in the 2D-VBS and compared to various explicit methods.
2.1 Facilities
The SAPHIR chamber is an atmospheric simulation chamber made of a double-walled Teflon (FEP) foil with a volume of 270 m3. It has a cylindrical shape and is housed in a steel frame. A shutter system mounted on the steel frame allows experiments to be conducted in the dark or, when opened, exposes the chamber to natural sunlight to initiate photochemistry. The pressure inside the chamber is kept at about 50 Pa overpressure compared to ambient pressures to ensure no diffusion from trace gases from the outside into the chamber. Additionally, the interspace of the double-walled Teflon film is continuously flushed with pure nitrogen (Linde, purity 99.9999 %). A continuous flow of ultra-clean air into the chamber compensates for any losses due to leakages and ensures stable pressure conditions.
In preparation for each experiment, the chamber is flushed with a high flow of up to 250 m3 h−1 for several hours using the ultra-clean air. The same high-flow rate is used to humidify the chamber before the start of each experiment. For humidification Milli-Q water is boiled and the steam is added to the airstream into the chamber. Two fans mounted inside the chamber generate well-mixed starting conditions and were turned off as soon as aerosol production was initiated to reduce aerosol losses in the chamber. Ozone is added using a silent discharge ozonizer. Standard instrumentation is continuously measuring the conditions inside the SAPHIR chamber. Instrumentation includes an ultrasonic anemometer (Metek USA-1, accuracy 0.3 K) to measure the air temperature, a frost point hygrometer (General Eastern model Hygro M4) to determine the humidity, and a chemiluminescence analyzer (ECO PHYSICS TR480) equipped with a photolytic converter (ECO PHYSICS PLC760) to measure NO and NO2. Ozone is measured by an UV absorption spectrometer (ANSYCO model O341M). Further details of the SAPHIR chamber are described in Rohrer et al. (2005).
Precursor compounds were added using two separate methods. The first method was to inject pure liquid compounds via a syringe in a heated inlet line, into the airstream with which the vapors were transported into the chamber. The second method was to use the plant chamber SAPHIR-PLUS (Hohaus et al., 2016) to transfer the emissions of six Pinus sylvestris L. (Scots pine) into the chamber. Injection flow from SAPHIR-PLUS was 6 m3 h−1, which, to a large extent, replaced the flow of clean air (8 m3 h−1) which is needed to replace air lost due to leakage and the withdrawal of analytical instrumentation. The environmental parameters of the plant chamber are fully controlled (e.g., temperature, soil relative humidity, photosynthetically active radiation). The average temperature inside the SAPHIR-PLUS chamber was 25 ∘C. Details on the SAPHIR-PLUS are provided in Hohaus et al. (2016).
2.2 Instrumentation
All instruments used in this study are described in detail in Gkatzelis et al. (2018) and only a brief overview is provided in the following. An Aerodyne high-resolution aerosol mass spectrometer (HR-AMS) (DeCarlo et al., 2006; Canagaratna et al., 2007) and a scanning mobility particle sizer (SMPS; TSI Classifier model 3080, TSI DMA 3081, TSI Water CPC 3786) were used to determine the aerosol chemical composition including the total organic mass concentration and the size distribution during the experiments, respectively. In order to determine the saturation mass concentrations (C*), parallel gas- and particle-phase measurements were performed. Particle-phase composition was measured using three different aerosol sampling techniques coupled to a proton-transfer reaction time-of-flight mass spectrometer (model PTR-TOF 8000; PTR-ToF-MS, Ionicon), the aerosol collection module (ACM-PTR-ToF-MS, referred to as “ACM” hereafter) (Hohaus et al., 2010), the chemical analysis of aerosols online (CHARON-PTR-ToF-MS, referred to as “CHARON” hereafter) (Eichler et al., 2015) and the collection thermal desorption unit (TD-PTR-ToF-MS, referred to as “TD” hereafter) (Holzinger et al., 2010). In the following, the most important characteristics and parameters are described briefly. The CHARON inlet combines a gas-phase denuder, an aerodynamic lens with an inertial sampler, and a thermodesorption unit which is coupled to a PTR-ToF-MS. The gas-phase denuder removes gas-phase analytes. Subsequently the aerosols are collimated by the aerodynamic lens and a particle-enriched sample flow is achieved by the inertial sampler. Afterwards the particles pass through a thermal desorption unit in which the particles are volatilized before being transferred to the gas-phase detector. The ACM has two sample air inlets. For the gas-phase inlet, air passes through a PTFE particle filter and is then directly introduced into the PTR-MS. For particle collection via the second sampling line air passes through an aerodynamic lens removing the gas phase and collimating particles onto a beam. The particles subsequently pass a vacuum chamber and are collected on a cooled sampling surface. Once collection is finished, particles are desorbed and transferred via a carrier gas (N2) to the PTR-ToF-MS detector. It is important to note that during the collection process the PTR-ToF-MS measures the gas phase in parallel allowing for a quasi-simultaneous characterization of gas and particle phase. The TD also employs a gas-phase denuder to remove gas-phase analytes before the aerosols are impacted using a Collection and Thermo-Desorption (CTD) cell. After collection particles are thermally desorbed and the components transferred to the PTR-ToF-MS. In the following, operational parameters are listed for all PTR-based instruments. CHARON is a real-time measurement (10 s integration time in the detector), while the ACM and TD have sampling times for this study of 120 and 240 min, respectively. The CHARON inlet was operated at low pressure (<1 atm) and at a constant temperature of 140 ∘C. The sampling in the ACM was under vacuum conditions and at sub-zero temperature (−5 ∘C). The sampling in the TD was at ambient temperature and at atmospheric pressure. CHARON used a gas-phase denuder to strip off gas-phase compounds while the AMS-type vacuum inlet system of the ACM ensured a removal of the gas phase. While the particle phase in CHARON was desorbed by passing particles through a thermodenuder, the particle phase in the ACM and TD was desorbed after collection from the collection surface using a temperature ramp reaching a final temperature of 250 and 350 ∘C, respectively. All three aerosol collection techniques utilize a PTR-ToF-MS as a detector. The operational conditions for each PTR-ToF-MS were different with regard to a different ratio of electric field strength (V cm−1) to buffer gas density (molecules cm−3) (E∕N). This can lead to different ionic fragmentation behavior. Therefore, the overlap of parent ions measured between the different instruments can be reduced. A detailed discussion about the E∕N effect has been investigated by Gkatzelis et al. (2018). Operational details for the different PTR-ToF-MS conditions are given also in Table S2. The PTR-ToF-MS of CHARON, ACM, and TD were operated at 100, 120, and 160 Td, respectively (1 Td V cm2 molecule−1). The drift tube conditions for the PTR-ToF-MS of CHARON, ACM, and TD were a temperature of 100 ∘C with a pressure of 2.30 mbar, 120 ∘C and a pressure of 2.40 mbar, and 120 ∘C and a pressure of 2.40 mbar, respectively. The limit of detection (LOD), depended on the different pre-concentration factors for each technique, which resulted in TD having the lowest LOD (10−3 ng m−3), followed by CHARON (1.4 ng m−3), while ACM showed the highest values (250 ng m−3). Finally, differences in sensitivity for each PTR-MS introduced minor deviations in this study and are discussed in detail in Gkatzelis et al. (2018). A characteristic time series of a major oxidation product from the β-pinene ozonolysis for the three different techniques can be found in Fig. S8.
Gas-phase organic compounds were detected by a standalone PTR-ToF-MS for CHARON and TD. The standalone PTR-ToF-MS was operated with an Td. The drift tube was kept at a temperature of 60 ∘C and a pressure of 2.30 mbar. The standalone PTR-MS was connected to SAPHIR via a 0.5 m perfluoroalkoxy alkane (PFA) line (inner diameter, i.d. 3.2 mm) and a 2 m polyether ether ketone (PEEK) line (i.d. 1 mm), heated to 60 ∘C with a flow of 0.6 L min−1, which resulted in an overall residence time of ∼0.6 s. The ACM was connected via a 4 m PFA line (i.d. 4 mm), at room temperature with a flow of 0.7 L min−1, resulting in a residence time of approximately 3 s. A PTFE particle filter (Merck Millipore) was additionally introduced to the PTR-MS line of the ACM to ensure complete particle-phase removal. Gas-phase compounds were then directed to the ACM-PTR-MS interface that was heated to 280 ∘C via a 5 cm coated stainless steel line (i.d. 0.8 mm) to the PTR-MS. The ACM design allowed for simultaneous gas-phase measurements with the same PTR-ToF-MS while sampling of the particle phase took place on the ACM collector. Comparison of gas- and particle-phase measurements was thus performed using the same detector avoiding any detector-related differences. It should be noted that TD and CHARON are also designed for simultaneous gas- and particle-phase measurements using the same PTR-MS, but in this study this feature was not operational.
2.3 Experimental conditions
Table 1Experimental conditions during each ozonolysis experiment. Two VOC injection periods were performed for the tree emissions experiment.

Before each experiment the SAPHIR chamber was flushed with clean air over night (total volume exchange was about 2000 m3) and humidified directly after the flushing process. Relative humidity (RH) in the chamber was about 55 % within a temperature range for all experiments between 295 and 310 K. After ensuring that all instruments had measured the background in the SAPHIR chamber, a single monoterpene (β-pinene or limonene), a monoterpene mixture (β-pinene and limonene), or the emissions of six Pinus sylvestris L. (Scots pine) were injected. The tree emissions were characterized using gas chromatography–mass spectrometry (GC-MS). The composition of the biogenic volatile organic compounds (BVOCs) consisted of 42 % δ3-carene, 38 % α-pinene, 5 % β-pinene, 4 % myrcene, 3 % terpinolene, and 8 % other monoterpenes. The details of all experiments are given in Table 1 and an experimental overview is provided in Fig. S1 in the Supplement. One hour after the injection of the volatile organic compounds (VOCs), ozone was introduced into the chamber to initiate ozonolysis with the subsequent formation of SOA. Experiments were done without the use of an OH scavenger. NOx concentrations during the experiments ranged between 10 and 60 pptv originating from a HONO background source (Rohrer et al., 2005). In all experiments, except for the experiment with limonene as a precursor, the roof of the SAPHIR chamber was opened 20 h after the start of the ozonolysis to initiate additional photochemistry with OH and the ageing of the SOA. For the limonene experiment instead of opening the roof, 30 ppbv of NO was added to the chamber. With the remaining ozone in the chamber, NO3 oxidation was initiated. In the tree emissions experiment the SAPHIR-PLUS chamber was recoupled to the SAPHIR chamber 11 h after the start of the ozonolysis, thus again injecting fresh BVOC emissions from the Scots pines. The experiment continued for an additional 6 h with the roof open allowing for further oxidation of the BVOCs and SOA by OH radicals. The duration of the experiments varied from 17 to 36 h, providing ample time to experimentally investigate the aging of the biogenic SOA.
2.4 Estimation of volatility distribution
In this work the volatility of different species was quantified based on their saturation mass concentration C* in units of µg m−3. Theoretical calculation of the saturation concentration was performed for known oxidation products of the monoterpenes studied based on their chemical structure as seen in Table S1 in the Supplement. Based on the absorption equilibrium partitioning formalism, the (subcooled liquid) saturation vapor pressure (pi,L) of a species was related to its C* based on Cappa and Jimenez (2010) as follows:
where MWOA is the molecular weight of the condensed organic phase (g mol−1), pi,L is the subcooled liquid saturation vapor pressure (Pa), ζi is the activity coefficient of species i in the OA phase, T is the chamber temperature (K) and R is the ideal gas constant (8.314 J mol−1 K−1). Here, the calculations were performed using a mean molecular weight of 180 g mol−1 (Prisle et al., 2010). In conformity with Donahue et al. (2014), the activity coefficients of all considered species partitioning into a mixed aerosol system containing similar compounds were assumed to be 1 throughout the study.
At present, there is a scarcity of reliable saturation vapor pressure data obtained through laboratory studies (Bilde et al., 2015). Therefore, pi,L is commonly estimated using empirical relationships derived from the Clausius–Clapeyron equation (e.g., Myrdal and Yalkowsky, 1997; Jenkin, 2004; Nannoolal et al., 2008). The required thermodynamic properties, such as the boiling temperature or the enthalpy of vaporization are predicted from the molecular structure of the investigated compounds (Mackay et al., 1982; Joback and Reid, 1987; Stein and Brown, 1994). Their explicit manual calculation using the existing functional group contribution methods is very laborious not only because of the high number of components but also because of the wide range of multifunctional organic compounds in the aerosol mixtures. Recently, a new web-based facility, UManSysProp (http://umansysprop.seaes.manchester.ac.uk, last access: 2 July 2018), was developed for automating predictions of, for example, pure component vapor pressures of organic molecules or activity coefficients for mixed liquid systems. For the group contribution approaches, only the molecular information must be uploaded in the form of SMILES (Simplified Molecular Input Line Entry System) strings (Toppings et al., 2016). At a defined temperature, there are several options for vapor pressure predictive techniques, providing the possibility to combine two different empirical representations of the Clausius–Clapeyron equation (Myrdal and Yalkowsky, 1997; Nannoolal et al., 2008 – further referred to as MY and NN, respectively) with three different prediction methods for thermodynamic properties of the investigated compound based on their molecular structure (Joback and Reid, 1987; Stein and Brown, 1994; Nannoolal et al., 2008 – the first item is further referred to as JR). Additionally, the EVAPORATION method (further referred to as EVAP) proposed by Compernolle et al. (2010) is available for the web-based calculations.
Here, we use the pi,L predicted online by UManSysProp facility, examining all seven estimation methods (Figs. S6 and S7). Only the results giving the lowest and highest vapor pressures for the studied compounds (the range of the estimates are indicated by the grey background color) are used in this study to compare measurements to the highest and lowest possible theoretical values.
The experimental determination of the saturation mass concentration of the individual compounds was derived by applying the partitioning theory (Pankow, 1994) based on Donahue et al. (2006) as
where OA is the total organic mass (µg m−3) determined from AMS and Gi and Pi are the gas- and particle-phase mass concentration (µg m−3) of compound i, respectively, measured from PTR-based techniques. Assuming typical vaporization enthalpies (e.g., ΔHVAP=127 kJ mol−1) (Epstein et al., 2010), C* and therefore partitioning are strongly dependent on temperature with changes of ±15 ∘C resulting in a C* change by a factor of 10. During the campaign the average chamber temperatures and their standard deviations were 20±4, 17±4, 19±5, and 30±5 ∘C for the β-pinene, limonene, mixture, and trees experiment, respectively. The uncertainty added from these variations (<10 ∘C) was further examined with a focus on the β-pinene oxidation products (Fig. S7). The difference in volatility due to variations ranged from 0.3 to 0.6 log(C*) depending on the chemical structure of the compound. Nevertheless, these variations can be considered small and not strongly affecting the conclusions of this work. Therefore, for consistency with other studies, a reference temperature of 298 K was used throughout all C* calculations. This was recently proposed by Stark et al. (2017) to derive the average C* for the Biohydro-atmosphere interactions of Energy, Aerosols, Carbon H2O, Organics & Nitrogen Rocky Mountain Biogenic Aerosol Study (BEACHON-ROMBAS) and SOAS field campaigns making the assumption that deviations due to temperature changes (18±7 and 25±3 ∘C, respectively) were within the uncertainties of the measurements. Calculation of the average C* for each experiment was performed based on the time resolution of each instrument (Sect. 2.2). When the signal in the particle phase was close to the detection limit, introducing a high uncertainty, the calculation of C* was not performed.
3.1 Compound selection: assessment of ionic and thermal decomposition
For all PTR-based techniques the molecular formula (CxHyOzNa)H+ was attributed to each detected signal derived from the exact molecular mass which was determined by the time of flight mass spectrometer (ToF-MS). Whether the detected ion was an original SOA compound or a fragment detected on this mass could be affected by two major processes: (i) thermal dissociation during desorption and (ii) ionic dissociation in the ionization region of the PTR-ToF-MS.
Thermal dissociation has been found to introduce a high degree of fragmentation for compounds that contain multiple functional groups, including peroxide groups which are thermally labile (Lopez-Hilfiker et al., 2015). For organic alcohols and acids, thermal desorption has been shown to lead to loss of carboxyl (-CO2), carbonyl (-CO), and water groups (-H2O) (Canagaratna et al., 2015). Accretion reactions and gas-phase autoxidation have been found to play a key role in extremely low-volatility organic compounds (ELVOCs) formation (Tobias and Ziemann, 1999, 2001; Ehn et al., 2014). Upon heating, such products will thermally decompose (Barsanti et al., 2017) and be detected in the lower molecular-weight range, thus directly affecting the partitioning estimation (Jang and Kamens, 2001; Stark et al., 2017). All instruments deployed in this study were subjected to possible thermal dissociation, with decarboxylation and dehydration reactions strongly dependent on the temperature, pressure, and the heat exposure time of the molecules during desorption. CHARON was operated at the lowest temperature of 140 ∘C, under a few millibars of pressure, and with the lowest heat exposure time, therefore minimizing the dehydration reactions. ACM and TD were operated at 1 bar and up to 250 and 350 ∘C, respectively, with longer heat exposure times.
Functional group loss has been found to additionally occur in the ionization region of the PTR-ToF-MS instruments. Gkatzelis et al. (2018) showed that for this study the ratio of the electric field strength (V cm−1) to buffer gas density (molecules cm−3) (E∕N) in the PTR-ToF-MS instruments played a key role in decomposition, not only due to water loss but also due to carbon–oxygen bond breakage of the detected molecules. Even though PTR-MS is considered a soft-ionization technique compared to, e.g., AMS, these decomposition pathways could still lead to a misidentification of the original chemical composition of the SOA species. For the ACM the ionic fragmentation for the gas- and particle-phase species was identical since both measurement were conducted using the same PTR-ToF-MS as a detector. CHARON and TD saturation mass concentration (C*) was determined by using the gas-phase mass concentration measurements derived from a separately deployed PTR-ToF-MS operated at different E∕N conditions (see Sect. 2.2). Ionic dissociation was thus different for the gas- compared to the particle-phase measurements, increasing the uncertainty of the volatility estimation for CHARON and TD when compared to ACM.
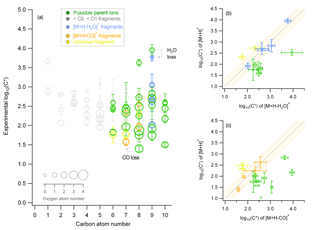
Figure 1Characteristic example of the fragment identification method from the β-pinene ozonolysis experiment for the ACM where (a) is the experimental saturation concentration (y axis) for all identified compounds with different carbon (x axis) and oxygen atom number (size of markers). Different colors indicate whether the compound represents a possible parent ion (green), a fragment with a carbon and oxygen atom number lower than 6 and 1, respectively (grey), or a fragment originating from the loss of water (blue) or CO (orange). This attribution results from panels (b) and (c), which show the correlation of the saturation concentration of identified ions to compounds with the same chemical formula subtracting water or CO . If the correlation is close to the 1:1 line, then the or compound is identified as a fragment and is given the respective color (blue or orange). If the or compound shows a higher volatility, it is considered as a possible parent ion (green). The orange background indicates the ±0.25 change in log(C*). Error bars correspond to the error of the average (±1σ).
A method to identify the ionic and thermal dissociation processes and their effect on the different techniques is presented in the following. This method was applied to the calculated average log(C*) of each ion, found both in the gas and particle phase, for each experiment for the individual instruments. A characteristic example of the β-pinene ozonolysis experiment (as shown in Fig. 1) for the ACM is used here to explain this method. Information on the carbon (x axis) and oxygen (size of the markers) atom number contained in the chemical formulas were used to differentiate between the different ions (Fig. 1a). Each marker indicates one ion; therefore, for the β-pinene experiment 72 ions were detected both in the gas and particle phase by the ACM. Their average saturation mass concentration log(C*) and therefore their volatility ranged from 101 to 104 µg m−3, an indication of semi-volatile and intermediate-volatile species in the SOA mass. Of these ions, 55 were identified as fragmentation products, accounting for 70 % of the partitioning ions, and only 25 % of these ions were used for further analysis.
Two major criteria were applied to differentiate between a possible parent ion (green markers) and a fragment. The first criterion was if the carbon and oxygen atom number were lower than 6 and 1, respectively. This criterion was chosen based on Donahue et al. (2006), who have shown that organic aerosols are expected from ELVOC to semi-volatile organic compounds (SVOCs) and intermediate-volatility organic compounds (IVOCs) with saturation concentrations ranging from −5 to 4. This volatility regime consists of species with carbon and oxygen atom numbers equal to or larger than 6 and 1, respectively (Donahue et al., 2011, 2012). Ions found in the particle phase with lower carbon and oxygen numbers were thus considered fragmentation products (grey markers) and were not used in the analysis. The second criterion focused on the dependence of the volatility on the number of oxygen and carbon atoms that constitute an organic molecule. As the oxygen and carbon atom number and thus the functionality of the molecule increased, the saturation mass concentration was expected to decrease (Pankow and Barsanti, 2009). If the volatility of an identified ion was identical to (within an uncertainty of log(C*) ±0.25) or higher than the volatility of ions with the same chemical formula subtracting a functional group the latter were considered highly affected by either ionic or thermal dissociation and were excluded from further analysis. Characteristic examples of this analysis are shown in Fig. 1b and c. The y axis corresponded to identified ions while the x axis corresponded to ions with the same chemical formula subtracting water (-H2O) (Fig. 1b) or a carbonyl group (-CO) (Fig. 1c). When the ions and were found to have identical saturation concentrations, ions were excluded (blue and orange markers in Fig. 1b and c, respectively). ions that showed lower volatility when compared to ions were considered fragments of unknown decomposition pathways (i.e., unknown parent ion composition) and were excluded as well (yellow markers). Only when ions showed higher volatility values than were they considered to be possible parent ions not strongly affected by thermal or ionic dissociation (green markers) and were further analyzed. The same comparison was not only performed for () and () functional group loss but was extended to (), (), () plus (), and () plus (). Checks were also performed for loss of the () functional group for the limonene NO3 oxidation experiment, but due to the high-E∕N operating conditions of all PTR-ToF-MS systems, no organic nitrates were identified (Duncianu et al., 2017).
It should be noted that PTR-MS provides information regarding the chemical formula of an ion and thus disregards the potential impact of the chemical structure. The functionality effect (e.g., stronger hydrogen bonding of an alcohol in a polar particle) can lead to a misidentification of potential parent ions as a fragment using the method described above due to the fact that a lower volatility is determined compared to an expected volatility based on the chemical formula. Nevertheless, although this method will potentially exclude parent ions, it will still also discard any possible fragments. Correlation analysis based on the time series of the different compounds could further improve the parent ion identification. However due to the low time resolution in this work, a time series analysis is not applicable. Another implication relies on the fact that ions could result from the decomposition of accretion reaction products or oligomers, consequently leading to an overestimation of their particulate-phase concentrations. This effect is not constrained by this method and is further addressed in Sect. 3.4. Furthermore, although this method can efficiently eliminate possible fragments, it does not provide proof that these fragments originate from the suggested fragmentation pathways. An overview of the fragmentation identification results of this method for each instrument and experiment are provided in Fig. S2. Percentages are derived based on the total number of fragment ions and how they are distributed (%) to the different fragmentation pathways. For all PTR-based techniques, 40 to 60 % of the partitioning ions were detected below the carbon and oxygen atom number threshold of C6 and O1, respectively. From the remaining species, ions affected by water (-H2O) loss were around 5–10 %, while carboxyl group (-CO2) fragmentation was identified for less than 10 % of the partitioning ions. Loss of (-CO), (-H2O2), (-H2O) plus (-CO), and (-H2O) plus (-CO2) functional groups affected less than 5 % of the ions for all experiments and instruments studied. Ions of unknown decomposition pathways represented ≤10 %, with TD showing the highest values. ACM showed increased contributions of lower molecular-weight ions, compared to TD and CHARON for the limonene and mixture experiments (max. 65 %). In total, the fraction of ions identified as parent compounds partitioning in the gas and particle phase that were chosen for further analysis in the next sections ranged between 20 and 40 % of the overall ions found in both phases, for each experiment and instrument studied.
The high contribution of lower-MW ions found both in the gas and particle phase for all PTR-based techniques further supported the idea that ionic and thermal dissociation played a key role in carbon–oxygen bond breakage. The higher E∕N values of ACM and TD compared to CHARON resulted in higher fragmentation and thus a higher contribution of the lower-MW partitioning ions (Gkatzelis et al., 2018). Although ACM was operated at lower-E∕N conditions compared to TD, the contribution of lower-MW ions was higher. The reason for this discrepancy was due to the higher limit of detection of the ACM (Sect. 2.2) compared to TD and CHARON. Ions of low concentrations in the higher-MW range that could be detected from CHARON and TD were below the detection limits of the ACM and were therefore not identified. For the remaining higher-MW species, the water (-H2O) loss was the dominant fragmentation pathway for all techniques. Although the PTR-based techniques were operated at a different temperature, desorption residence times, and pressure conditions, they showed a similar percentage of ions affected by water loss. This is an indication that for all techniques dehydration occurred mostly due to ionic fragmentation in the ionization region of the PTR-MS and not due to thermally initiated reactions for the partitioning ions studied. TD showed a higher contribution of fragments of unknown decomposition pathways when compared to ACM and CHARON due to the highest difference in E∕N operating conditions in the particle phase (160 Td) compared to the gas phase (120 Td), with the latter measured by a separately deployed PTR-ToF-MS. The higher ionic dissociation in the particle phase increased the concentration of lower-MW ions and decreased that of higher-MW ions. This had a direct effect on the calculation of the volatility based on Eq. (2). When this effect was strong enough, fragment ions showed higher concentrations in the particle phase and thus lower volatility when compared to possible parent ions . Based on this method, these ions were excluded as fragments of unknown fragmentation pathways and showed an expected higher contribution for systems like the TD. Fragment loss of (-CO2), (-CO), (-H2O2), (-H2O) plus (-CO), and (-H2O) plus (-CO2) accounted for 10 % or less, suggesting that these pathways were not dominating the partitioning ions studied. The interference of accretion reaction products or oligomers which could be detected at a lower m∕z due to decomposition processes are not accounted for in the previously described method. The possible effect of such an interference is further discussed in Sect. 3.4.
3.2 Volatility distribution coverage
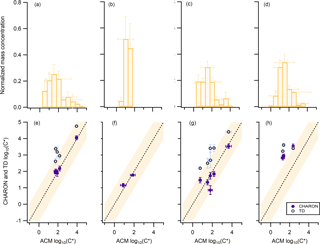
Figure 2Oxidation experiments using (a, e) β-pinene, (b, f) limonene, (c, g) a mixture of β-pinene and limonene, and (d, h) real tree emissions from Pinus sylvestris L. (Scots pine) as a precursor. Panels (a, b, c, d) correspond to the normalized average mass concentration from ACM, CHARON, and TD, distributed to the different volatility bins with a volatility resolution of 0.5 µg m−3. Panels (e, f, g, h) correspond to the average volatility of overlapping compounds seen from CHARON and ACM (purple circles) or TD and ACM (blue circles). The dash line represents the 1:1 line. The orange background color indicates the ±1 µg m−3 deviation from the 1:1 line. Error bars correspond to the ±1σ of the average throughout each experiment.
The mass concentrations of only the species identified as parent ions for ACM, CHARON, and TD were distributed to different volatility bins ranging from log(C*) of −1 to 5 with a 0.5-bin volatility resolution. The normalized volatility distribution (NVD) for each experiment accounting for all PTR-based techniques is shown in Fig. 2a, b, c, and d. Normalization was performed by dividing each volatility bin by the sum of the PTR-based technique mass concentration measured at each experiment. The detected biogenic SOA partitioning species showed log(C*) values from 0 to 4, an indication that mainly SVOCs and IVOCs were predominantly measured simultaneously in the gas and the particle phase. The limonene NO3 oxidation experiment had the lowest NVD starting from a log(C*) of 0.5, with a narrow spread up to 2. For the β-pinene and β-pinene/limonene mixture experiments the NVD moved towards more volatile species ranging from 0.5 to 4. When comparing the single compound experiment of β-pinene to the mixture, the latter showed a NVD shifted to lower saturation concentrations. Partitioning species detected from all the PTR-based techniques were further compared as seen in Fig. 2e, f, g, and h. ACM and CHARON showed same volatility values for all experiments with only the trees experiment resulting in higher deviations from the one-to-one line. TD presented higher log(C*) when compared to CHARON and ACM, suggesting the examined species were underestimated in the particle phase. A total of 5, 2, 6, and 4 ions were observed to partition with all three techniques for the β-pinene, limonene, β-pinene/limonene mixture and tree emissions experiment, respectively, after applying the parent ion identification method of Sect. 3.1.
The calculation of the log(C*) in this study relied on the ratio between the gas- and particle-phase signal of an ion (Eq. 2). Detection limits of both of these limited the measurable range of this ratio. This explains the narrow volatility distributions available with all PTR-based techniques, as has been previously reported by Stark et al. (2017). Combining the capabilities of these instruments and the above approach to calculate the volatility provided insights into a defined range of SVOCs and IVOCs. Within this volatility range the differences observed when using different precursors agree with bulk volatility measurement findings that limonene SOA is less volatile than β-pinene SOA (Lee et al., 2011). When focusing on the species measured differences of ACM and CHARON to TD could be explained by the higher-E∕N conditions of TD that have previously been discussed (Sect. 3.1). Since TD was more prone to particle-phase fragmentation compared to the gas phase, these higher-MW compounds showed lower concentrations and thus indicated higher volatility. This effect was negligible for ACM, which was using the same PTR-MS for gas- and particle-phase measurements, and lower for CHARON operated at lower-E∕N conditions. The agreement of ACM and CHARON for all experiments except the trees experiment further indicated that both techniques measured the same species in good agreement and within the uncertainties of these calculations. As the complexity of the system increased, this agreement deviated from the one-to-one line. Gkatzelis et al. (2018) reported that for the single-precursor and mixture experiments, ions were detected with C6 to C12 carbon atoms from all techniques. By contrast, during the tree emissions experiment CHARON was the only instrument to detect ions in the C13 to C20 range. These ions were not detected from ACM or TD, which were operated at higher-E∕N conditions and were more prone to ionic and thermal dissociation. Fragmentation of these higher-carbon atom ions could affect the volatility calculation of lower-MW species still detected by ACM and TD and thus explain the deviations seen for the tree emissions experiment.
The total number of species seen from all techniques was low due to the parent ion identification method applied in the previous section. An overview of the overlapping compounds is provided in Fig. S3. When all detected ions were taken into account, more than 50 ions were seen from all techniques at each experiment. After narrowing our focus on the partitioning ions and excluding the lower-MW fragments, the overlapping compounds dropped to ∼15 ions. Each technique was affected differently by ionic and thermal dissociation. By applying the above method to each technique, different ions were excluded for each instrument, thus leading to only a few species seen from all three techniques and considered to be parent ions.
3.3 Experimentally derived saturation concentration implemented in the 2D-VBS
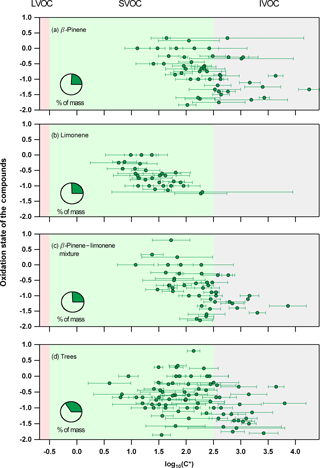
Figure 3The average experimental saturation concentration for detected ions (from ACM, CHARON, or TD) that act as parent ions identified using the described selection criteria during the (a) β-pinene, (b) limonene, (c) mixture of β-pinene and limonene, and (d) the real tree emissions experiments. Error bars indicate the ±1σ of the experimental average. Pie charts show the percent of mass (green) measured when adding all presented ions compared to the total organic mass obtained from the AMS.
Species identified as parent ions from each technique were combined and further analyzed with a focus on their average saturation concentration as seen in Fig. 3. For parent ions measured from more than one instrument, the average of all techniques was used to determine the overall experimental C* of the ion, with the error bars indicating the error of this average. The 2D-VBS (Donahue et al., 2011; Murphy et al., 2012) framework was used to implement the results for each experiment, with background colors corresponding to the different volatility classes, ranging from IVOCs (grey) to SVOCs (green) and LVOCs (red). It should be noted that the oxidation state (OSC) was not derived by bulk measurements, for example, using the AMS, but by using the OSC of the individual species based on their carbon, hydrogen, and oxygen atom number (Kroll, 2011). In total 48, 31, 46, and 79 ions were identified as parent ions for the β-pinene, limonene, β-pinene/limonene mixture, and tree emissions oxidation experiment, respectively. The saturation concentration showed a decrease for species with higher OS and oxygen atom number. For the limonene experiment, lower saturation concentration values for compounds defined by the same oxidation state were found when compared to the β-pinene, mixture, or tree emissions experiment. Overall, parent ions corresponded to 20–30 % of the overall organic mass measured from an AMS for all systems studied.
The observed volatility decrease with increasing OS and oxygen atom number is in good agreement with previous findings (Jimenez et al., 2009; Kroll, 2011). Lower-volatility values for limonene species with the same OS when compared to the β-pinene, mixture, or the tree emissions experiment suggested that species originating from different precursors and oxidation pathways with differences in their functionality and molecular structure affected their gas-to-particle partitioning. It should be noted that the lower volatility of limonene could be partly explained by the absence of TD measurement in this experiment and thus the absence of TD C* values when averaging the experimental results from all PTR-based techniques. Since TD was affected the strongest by ionic dissociation (highest E∕N), the C* values were biased to higher volatilities when compared to ACM and CHARON, with particle-phase measurements (Pi in Eq. 2) fragmenting more compared to the gas phase (Gi from dedicated gas-phase PTR operated at lower E∕N). Results when averaging all experiments and excluding the TD data are shown in Fig. S4. Although the limonene experiment would still show lower volatilities compared to the β-pinene and mixture experiments, this trend would be less strong suggesting that the absence of TD during the limonene experiment did lower the overall average volatility calculation presented in Fig. 3. The increased number of species detected during the tree emissions experiment occurred due to the higher complexity of this system, with more than one precursor oxidized to form SOA. In total, the PTR-based techniques showed that 20–30 % of the overall BSOA mass consisted of ions with volatilities within the SVOC to IVOC range further showing the importance of understanding the gas-to-particle partitioning and thermodynamic properties of compounds formed in such systems.
At this point, it should be noted that losses of gas-phase compounds through the lines, from the SAPHIR to the PTR-MS, could also affect the log(C*) calculation by changing the ratio of the gas to the particle phase. Gas-phase measurements were performed using a standalone PTR-MS for TD and CHARON while for the ACM both gas- and particle-phase measurements were obtained using the same PTR-MS of ACM. The two PTR-MS differed in inlet length, temperature, and material, with ACM-PTR-MS introducing higher residence times and thus a longer exposure of the gas-phase compounds to the line walls (see Sect. 2.2). If significant losses of gas-phase compounds in the ACM-PTR-MS compared to the standalone PTR-MS line occurred, the gas-phase concentration would be underestimated and therefore also the log(C*) derived from the ACM measurements. To test if the dissimilarities between the different PTR-MS inlet lines were biasing the results of the ACM, recalculation of the log(C*) was performed by using Eq. (2) and applying the ACM particle-phase concentration (Pi) but changing the gas-phase concentration (Gi) to measurements from the standalone PTR-MS. This calculation was performed for all ions identified as parent ions for the ACM when using the parent ion identification method. An overview of the correlation of the log(C*) using the two different gas-phase datasets is shown in Fig. S5. For all experiments and for most of the compounds, agreement within the uncertainty of the measurements was found. For the tree emissions oxidation experiment the fraction of compounds deviating from the one-to-one line was higher. The spread in the data around the one-to-one line can be explained by the fact that though both PTR-MS were the same type of model, differences in, for example, the TOF interface and the drift tube existed. These differences affected the fragmentation and resolution of the PTR-MS (Gkatzelis et al., 2018) and could therefore explain the deviations observed. Moreover, the tree emissions experiment showed the highest complexity in comparison with the single-precursor oxidation experiments, with detected ions that had up to 20 carbon atoms in the particles. These higher molecular-weight ions fragmented differently when passing through the differing ToF interfaces and thus resulted in the observed higher deviation. However the differences are within the experimental uncertainties and therefore no significant bias due to potential inlet line interference could be determined.
3.4 Experimentally derived saturation concentration compared to explicit methods
In order to derive further information from the experimentally determined parent ions, a comparison with previous publications was performed for the major oxidation products from (a) the β-pinene ozonolysis (Yu et al., 1999; Jenkin, 2004; Chen and Griffin, 2005; Steitz, 2010; Kahnt, 2012; Hohaus et al., 2015), (b) limonene ozonolysis and NO3 oxidation (Chen and Griffin, 2005; Leungsakul et al., 2005a, b; Jaoui et al., 2006; Kundu et al., 2012), and (c) tree emissions ozonolysis, with α-pinene and Δ3-carene being the major reactants (Yu et al., 1999; Chen and Griffin, 2005; Praplan et al., 2015). By attributing a chemical structure to the ions identified by the PTR-MS, detected parent ions that overlapped with compounds from previous publications were further examined based on their structural information. An overview of the overlapping compounds and their suggested structures are given in Table S1. Uncertainties introduced by assigning a chemical structure to an ion of a given chemical formula are further discussed in this section.
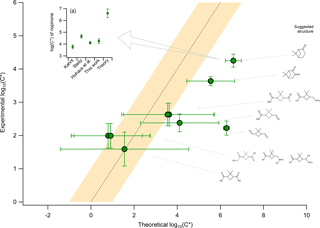
Figure 4The experimental average saturation concentration obtained from all PTR-based techniques (y axis) compared to the theoretical calculation of the saturation concentration (x axis). Theoretical calculations were performed by assuming a chemical structure for the experimentally observed ions. The chemical structure was attributed based on known oxidation products of the β-pinene ozonolysis experiment and is shown on the right side of the figure. Error bars on the y axis indicate the ±1σ error of the average based on the experimental results from ACM, TD, and CHARON. The error bars for the x axis act as indicators of the minimum and maximum range of nine different theoretical approaches, with the position of the marker indicating the average of these minimum and maximum values. More details on the theoretical calculations are provided in Sect. 2.4. Panel (a) provides experimentally determined values of the saturation concentration for nopinone based on Hohaus et al. (2015) and Kahnt (2012), together with the results of the experimental and theoretical approaches from this study.
A detailed analysis of the β-pinene ozonolysis experiment was performed as seen in Fig. 4. Experimental calculation of the saturation concentration was performed based on the average C* values throughout the experiment when taking into account all PTR-based techniques, with the error bars indicating the ±1σ of this averaging. The theoretical calculations by UManSysProp facility showed that the combinations of the boiling temperature (TB) prediction using NN with the pi,L empirical expressions using MY yielded the maximum C* values while TB by JR with pi,L by NN yielded the minimum C* values (Figs. S6 and S7). More details regarding the theoretical calculations are provided in the Supplement. The methods with the smallest and largest C* values for the given compounds were chosen to represent the upper and lower limits of the possible theoretical values when comparing them to the observed ones. These limits are expressed in Fig. 4 by the error bars on the x axis, with the marker points corresponding to their average. In total, 10 compounds were identified from previous publications to overlap with experimentally detected parent ions for the β-pinene ozonolysis experiment. For most of these compounds, theoretical and experimental values agreed within the uncertainties. No significant discrepancies were found for compounds in the SVOC volatility range. However, compounds in the IVOC range were underestimated from the experimental approaches when compared to theory. A characteristic IVOC first-generation product from the β-pinene ozonolysis is nopinone, which has been previously experimentally studied with a focus on the gas-to-particle partitioning (Steitz, 2010; Kahnt, 2012; Hohaus et al., 2015). A comparison of this work with previous studies was performed, as can be seen in Fig. 4a. The results showed agreement of C* within ±100.5 between the experimental approaches while the theory showed differences of 103 in the C* estimation. This comparison was extended to oxonopinone, being the second underestimated IVOC first-generation product, where again this study (log(C*) ) was in good agreement with Hohaus et al. (2015) (log(C*) ) using GC-MS but the same sampling technique.
To better understand the differences of the experimental to the theoretical approaches, the focus was placed on the potential sources of uncertainties within both calculations. For the theoretical approach, the more complex the molecules with increasing functional groups were, the higher the uncertainty of the saturation vapor pressure and thus the volatility was. This is depicted by the higher error bars when moving towards SVOCs. first-generation products like nopinone are not characterized by high complexity; thus, theory provided more reliable thermodynamic values, also reflected by the good agreement between all theoretical approaches (Figs. S6 and S7). The experimental calculation of the volatility performed by the PTR-based techniques could be affected by the (i) existence of isomers within a studied m∕z with different structural information and thus thermodynamic properties; (ii) thermal and ionic fragmentation of higher molecular-weight compounds, produced by accretion and oligomerization reactions, down to the m∕z detected by the instruments; and (iii) phase state of the bulk OA influencing the partitioning equilibrium timescales (τeq) of the individual compounds.
Mass spectrometric measurement approaches by definition provide molecular formulas; however, a given formula does not correspond to an individual compound. Isaacman-VanWertz et al. (2017) showed that during the α-pinene OH oxidation, molecules with larger carbon atom numbers (C8 to C10) corresponded to an increased number of unique isomers for each molecular formula. Differences in the functionality of these isomers may be critical for studies of their thermodynamic properties. To reduce biases in this work, the different isomers seen from previous publications were included in the theoretical calculations. For the β-pinene experiment, the isomers showed theoretical C* values within the estimated uncertainty, thus biasing this comparison to a minor extent. For formulas that corresponded to an individual compound, e.g., nopinone and oxonopinone, further comparison with previous publications was performed. The experimentally calculated C* was in good agreement with previous studies using a GC-MS to detect particle-phase nopinone (Kahnt, 2012; Hohaus et al., 2015). Since GC-MS techniques are capable of providing the exact molecular structure of nopinone, this further supported the identification of (C9H14O1)H+ and (C9H12O2)H+ as protonated nopinone and oxonopinone, respectively, in this study.
The treatment of the PTR dataset to exclude ions affected by thermal and ionic dissociation was described in detail in Sect. 3.1. However, higher-MW species (e.g., accretion reaction products or oligomers), of low volatility, which are not in the detection range of the PTR-ToF-MS, could decompose to lower-MW species during thermal breakdown (Barsanti et al., 2017) (Tillmann et al., 2010). These species could be identified as a parent ion when using the parent ion identification method (Sect. 3.1), consequently inducing an overestimation of their particulate-phase concentrations. This effect is not constrained in the method used and could potentially and selectively decrease the volatility of certain species. To explain the differences in the C* experimental vs. theoretical estimations for nopinone, the ratio from Eq. (2) should change by a factor of ∼300. This would suggest a particulate-phase mass concentration 300 times lower than the observed one in order to reach an agreement with the theoretical calculations. This fragmentation pathway should not only strongly affect the PTR-based techniques but also the previously mentioned GC-MS systems. The decomposition pathway would be narrowed to thermal dissociation during desorption, which is the only common pathway from all techniques. Finally, this thermal dissociation pathway needs to result in products with the exact chemical structure of nopinone.
When describing SOA formation, it is generally assumed that oxidation products rapidly adopt gas-to-particle equilibrium with the assumption of a homogeneously mixed condensed phase (Pankow, 1994; Odum et al., 1996). The nonideal behavior of a complex organic mixture could introduce mixing effects, changing the activity coefficients of the individual organic molecules and thus their gas-to-particle equilibrium. Isotopic labeling experiments have confirmed that SOA derived from different precursors will interact in a relatively ideal fashion, thus introducing minor deviations of the activity coefficient from unity (Dommen et al., 2009; Hildebrandt et al., 2011). Furthermore, Hohaus et al. (2015) showed that for the β-pinene ozonolysis oxidation products, the theoretically estimated activity coefficient values calculated by the thermodynamic group contribution model AIOMFAC (Zuend et al., 2011) were far from explaining the differences between theory and observations. These findings further suggest that in this work, gas-to-particle partitioning was not strongly affected by activity coefficient deviations and thus could not explain the obtained differences. By contrast, the phase state of the bulk OA strongly affects the partitioning equilibrium timescales (τeq), ranging from seconds in the case of liquid particles to hours or days for semisolid or glassy particles (Shiraiwa et al., 2011; Shiraiwa and Seinfeld, 2012). Biogenic SOA particles have been found to adopt an amorphous solid, most probably glassy state (Virtanen et al., 2010). The experimental conditions in this study (on average 55 % RH) suggest that a significant portion of the SOA can be in a semisolid or glassy state (Bateman et al., 2015). This amorphous (semi)solid state may influence the partitioning of semi-volatile compounds, hindering the lower-volatility species to leave the particles. Biogenic OA produced in this study would thus be directly affected by high partitioning equilibrium timescales leading to increased particulate-phase concentrations of more volatile compounds “trapped” within this glassy state of the OA. This would imply a direct decay of their volatility, thus explaining the observed lower C* values of the first-generation products.
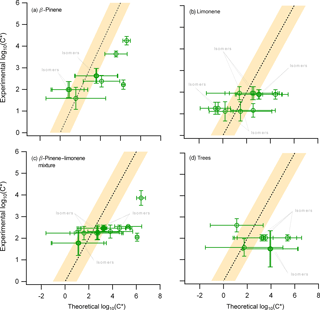
Figure 5The experimental average saturation concentration obtained from all PTR-based techniques (y axis) compared to the theoretical calculation of the saturation concentration (x axis) for the (i) β-pinene, (ii) limonene, (iii) mixture of β-pinene and limonene, and (iv) the real tree emissions experiments. Error bars on the y axis indicate the ±1σ error of the average based on the experimental results from ACM, TD, and CHARON. The error bars for the x axis act as indicators of the minimum and maximum range of nine different theoretical approaches, with the position of the marker indicating the average of these minimum and maximum values.
A comparison of the observed and calculated C* was performed for all experiments during this campaign as shown in Fig. 5. There were 11, 12, and 9 compounds observed in the limonene, terpene, and trees oxidation experiments, respectively, which have been described in previous publications. These compounds can be attributed to only five, eight, and four different molecular formulas (m∕z), suggesting an increased number of isomers found within these overlaps. The analysis yielded similar findings to those from the β-pinene experiment. The comparison between observations and theory showed relatively good agreement within the SVOC range for most of the compounds, while the C* for compounds expected to be in the IVOC range was experimentally underestimated, i.e., the measured particle-phase concentrations were higher than those explained by the equilibrium partitioning theory. When moving from single to multiple precursor experiments, e.g., from the ozonolysis of β-pinene to the ozonolysis and NO3 oxidation of limonene, the number of isomers increased rapidly, due to the higher complexity of the investigated systems. Certain isomers showed variations of up to 2 orders of magnitude in their estimated volatility values. On the other hand, due to an increased complexity of the systems, the limitations of the mass spectrometric techniques to define the molecular structure of the compounds might introduce large biases. However, despite these uncertainties, the theoretical volatility values were still found to be in fair agreement with the observations for all systems studied, suggesting that these deviations would still be within the already existing high uncertainties associated to the theoretical calculations.
There are two major effects that could be emphasized by presenting two case scenarios. In the first scenario the equilibrium partitioning theory correctly represents the studied systems. The experimental underestimation of the IVOC (and certain SVOC) volatility can thus only be explained by experimental uncertainties due to (i) the fragmentation of higher-MW compounds and oligomers to the detection range of the PTR-based technique, and/or (ii) the existence of isomers with high-volatility differences. However, as mentioned before, studies which performed a molecular identification of compounds (e.g., nopinone) show significantly different experimentally derived partitioning coefficient values when compared to theoretical calculations (Hohaus et al., 2015; Kahnt, 2012); therefore, isomers could not explain this discrepancy for all cases. In the second scenario, the assumption of equilibrium partitioning would be questioned due to the findings that BSOA forms a glassy phase state and thus gas-to-particle equilibrium might not be reached. This would imply that all theoretical calculations performed in this study and used in models to describe SOA formation would be developed under the wrong assumption, thus decreasing their reliability. This work provides clear evidence pointing towards these two effects but cannot provide a quantitative estimate to their individual contribution. Future studies combining the information provided by the PTR-based techniques with SOA phase-state measurements are essential. In order to bridge the gap between experimental data and theoretical volatility calculations, further development of instrumentation providing structural information at a molecular level is required. Techniques like the TAG (Williams et al., 2006; Isaacman et al., 2014; Zhang et al., 2014) coupled in parallel to the PTR-based techniques could provide further insight into different isomeric structures.
We have presented the first laboratory intercomparison of three in situ, near real-time measurement techniques of gas-to-particle partitioning with a focus on biogenic SOA formation and oxidation. These thermal desorption techniques are known to be affected by thermal dissociation during desorption and ionic dissociation during ionization in the drift tube of the PTR-MS (Gkatzelis et al., 2018). These fragmentation pathways could directly affect the gas-to-particle partitioning and thus the saturation mass concentration (C*) calculation. To reduce fragmentation biases, a method to identify and exclude ions affected by these decomposition pathways was developed and applied. Narrow volatility distributions were observed ranging from 0 to 4 with species in the semi-volatile (SVOCs) to intermediate-volatility (IVOCs) regime. The limonene oxidation experiment showed a lower-volatility distribution when compared to the β-pinene oxidation experiment, further supporting the idea that limonene SOAs are less volatile than β-pinene SOA (Lee et al., 2011). When comparing C* values obtained for species observed from all techniques, instruments showed good agreement within 1 decade, with deviations explained by the different operating conditions of the PTR-MS (Gkatzelis et al., 2018).
Determined species were mapped onto the 2D-VBS framework, and results showed a decrease in C* with increasing oxidation state and increasing oxygen atom number in accordance with previous findings (Jimenez et al., 2009; Kroll, 2011). These species accounted for 20–30 % of the total organic mass measured from an AMS. For species that overlapped with compounds from previous publications a comparison with theoretical calculations was performed based on their molecular structure. Accounting for the uncertainties of the measurements, results showed good agreement for SVOCs, while IVOCs introduced higher deviations. A detailed comparison of the partitioning values of nopinone, a first-generation product from the ozonolysis of β-pinene, with previous publications, was performed. Results showed agreement of C* within ±100.5 between all experimental approaches while theory showed differences of 103 in the C* estimation. These major differences are discussed in terms of possible uncertainties biasing the experimental values from (1) the existence of isomers within a studied m∕z; (2) thermal and ionic fragmentation of higher molecular-weight compounds, produced by accretion and oligomerization reactions, fragmenting to m∕zs detected by the instruments; (3) non-idealities of the organic mixtures; and (4) the phase state of the bulk OA affecting the partitioning equilibrium timescales (τeq) of the individual compounds. Results point to possible interferences by thermal and ionic fragmentation as well as kinetic influences in the distribution between the gas and particle phase, with diffusivity in the particle phase and irreversible uptake. These findings further encourage future work and parallel measurement of the phase state of the OA combined with compound-specific volatility determination from the PTR-based techniques.
The data of the experiments in the SAPHIR chamber used in this work are available on the EUROCHAMP data website: https://data.eurochamp.org/data-access/chamber-experiments/. The titles of the datasets and their unique identifiers on the website are as follows: “limonene+β-pinene+O3 – Aerosol study – particle formation” (69dd4354-95d-48aa-9def-2aa0b41092af); “limonene+O3 — Aerosol study – physical properties” (af247392-99fb-40a1-85f2-2b6843d8f4db); “β-pinene+O3 – Aerosol study – physical properties” (70d94847-c72e-400f-962c-7a571e43163d); “Plant emissions+O3 – Aerosol study – physical properties” (ae5cb780-3648-4d0b-95c2-718af8ed2f5f) (Tillmann, 2018).
The supplement related to this article is available online at: https://doi.org/10.5194/acp-18-12969-2018-supplement.
RT, RH, AW, and AKS designed the experiments. TH and RT operated the chambers. SHS, PS, PE, MM, KMX, GIG, RW, MK, AW, RH, RT, ZY, and TH conducted the data collection and evaluation for AMS, TD, CHARON, ACM, PTR, and GC-MS. IG conducted the theoretical calculations. GIG, TH, RT, and AKS did the data analysis. GIG did the data interpretation and prepared the paper with contributions from all coauthors.
The authors declare that they have no conflict of interest.
This work was supported by the EC's 7th Framework Programme
under grant agreement number 287382 (Marie Curie Initial Training Network
PIMMS); by the Helmholtz President's Fund (Backfeed); and by the Dutch NOW
Earth and Life Science (ALW), project 824.14.002. The authors acknowledge
support by the SAPHIR team electronic and mechanical
workshops.
The article processing
charges for this open-access
publication were covered by a
Research
Centre of the Helmholtz Association.
Edited by: David Topping
Reviewed by: two anonymous referees
An, W. J., Pathak, R. K., Lee, B.-H., and Pandis, S. N.: Aerosol volatility measurement using an improved thermodenuder: Application to secondary organic aerosol, J. Aerosol Sci., 38, 305–314, https://doi.org/10.1016/j.jaerosci.2006.12.002, 2007.
Barsanti, K. C., Kroll, J. H., and Thornton, J. A.: Formation of low-volatility organic compounds in the atmosphere: Recent advancements and insights, J. Phys. Chem. Lett., 8, 1503–1511, https://doi.org/10.1021/acs.jpclett.6b02969, 2017.
Bateman, A. P., Gong, Z., Liu, P., Sato, B., Cirino, G., Zhang, Y., Artaxo, P., Bertram, A. K., Manzi, A. O., Rizzo, L. V., Souza, R. A. F., Zaveri, R. A., and Martin, S. T.: Sub-micrometre particulate matter is primarily in liquid form over amazon rainforest, Nat. Geosci., 9, 34–37, https://doi.org/10.1038/ngeo2599, 2015.
Bilde, M., Barsanti, K., Booth, M., Cappa, C. D., Donahue, N. M., Emanuelsson, E. U., McFiggans, G., Krieger, U. K., Marcolli, C., Topping, D., Ziemann, P., Barley, M., Clegg, S., Dennis-Smither, B., Hallquist, M., Hallquist, A. M., Khlystov, A., Kulmala, M., Mogensen, D., Percival, C. J., Pope, F., Reid, J. P., Ribeiro da Silva, M. A., Rosenoern, T., Salo, K., Soonsin, V. P., Yli-Juuti, T., Prisle, N. L., Pagels, J., Rarey, J., Zardini, A. A., and Riipinen, I.: Saturation vapor pressures and transition enthalpies of low-volatility organic molecules of atmospheric relevance: From dicarboxylic acids to complex mixtures, Chem. Rev., 115, 4115–4156, https://doi.org/10.1021/cr5005502, 2015.
Booth, A. M., Markus, T., McFiggans, G., Percival, C. J., Mcgillen, M. R., and Topping, D. O.: Design and construction of a simple Knudsen Effusion Mass Spectrometer (KEMS) system for vapour pressure measurements of low volatility organics, Atmos. Meas. Tech., 2, 355–361, https://doi.org/10.5194/amt-2-355-2009, 2009.
Camredon, M., Hamilton, J. F., Alam, M. S., Wyche, K. P., Carr, T., White, I. R., Monks, P. S., Rickard, A. R., and Bloss, W. J.: Distribution of gaseous and particulate organic composition during dark a-pinene ozonolysis, Atmos. Chem. Phys., 10, 2893–2917, https://doi.org/10.5194/acp-10-2893-2010, 2010.
Canagaratna, M. R., Jayne, J. T., Jimenez, J. L., Allan, J. D., Alfarra, M. R., Zhang, Q., Onasch, T. B., Drewnick, F., Coe, H., Middlebrook, A., Delia, A., Williams, L. R., Trimborn, A. M., Northway, M. J., DeCarlo, P. F., Kolb, C. E., Davidovits, P., and Worsnop, D. R.: Chemical and microphysical characterization of ambient aerosols with the aerodyne aerosol mass spectrometer, Mass. Spectrom. Rev., 26, 185–222, https://doi.org/10.1002/mas.20115, 2007.
Canagaratna, M. R., Jimenez, J. L., Kroll, J. H., Chen, Q., Kessler, S. H., Massoli, P., Hildebrandt Ruiz, L., Fortner, E., Williams, L. R., Wilson, K. R., Surratt, J. D., Donahue, N. M., Jayne, J. T., and Worsnop, D. R.: Elemental ratio measurements of organic compounds using aerosol mass spectrometry: characterization, improved calibration, and implications, Atmos. Chem. Phys., 15, 253–272, https://doi.org/10.5194/acp-15-253-2015, 2015.
Cappa, C. D. and Jimenez, J. L.: Quantitative estimates of the volatility of ambient organic aerosol, Atmos. Chem. Phys., 10, 5409–5424, https://doi.org/10.5194/acp-10-5409-2010, 2010.
Chen, J. and Griffin, R.: Modeling secondary organic aerosol formation from oxidation of -pinene, -pinene, and -limonene, Atmos. Environ., 39, 7731–7744, https://doi.org/10.1016/j.atmosenv.2005.05.049, 2005.
Clegg, S. L., Seinfeld, J. H., and Brimblecombe, P.: Thermodynamic modelling of aqueous aerosols containing electrolytes and dissolved organic compounds, J. Aerosol Sci., 32, 713–738, 2001.
Compernolle, S., Ceulemans, K., and Müller, J.-F.: Technical Note: Vapor pressure estimation methods applied to secondary organic aerosol constituents from a-pinene oxidation: an intercomparison study, Atmos. Chem. Phys., 10, 6271–6282, https://doi.org/10.5194/acp-10-6271-2010, 2010.
Daumit, K. E., Kessler, S. H., and Kroll, J. H.: Average chemical properties and potential formation pathways of highly oxidized organic aerosol, Faraday Discuss., 165, 181–202, https://doi.org/10.1039/C3FD00045A, 2013.
DeCarlo, P. F., Kimmel, J. R., Trimborn, A., Northway, M. J., Jayne, J. T., Aiken, A. C., Gonin, M., Fuhrer, K., Horvath, T., Docherty, K. S., Worsnop, D. R., and Jimenez, J. L.: Field-deployable, high-resolution, time-of-flight aerosol mass spectrometer, Anal. Chem., 78, 8281–8289, https://doi.org/10.1021/ac061249n, 2006.
de Gouw, J. A., Brock, C. A., Atlas, E. L., Bates, T. S., Fehsenfeld, F. C., Goldan, P. D., Holloway, J. S., Kuster, W. C., Lerner, B. M., Matthew, B. M., Middlebrook, A. M., Onasch, T. B., Peltier, R. E., Quinn, P. K., Senff, C. J., Stohl, A., Sullivan, A. P., Trainer, M., Warneke, C., Weber, R. J., and Williams, E. J.: Sources of particulate matter in the northeastern united states in summer: 1. Direct emissions and secondary formation of organic matter in urban plumes, J. Geophys. Res., 113, D8, https://doi.org/10.1029/2007jd009243, 2008.
Dommen, J., Hellén, H., Saurer, M., Jaeggi, M., Siegwolf, R., Metzger, A., Duplissy, J., Fierz, M., and Baltensperger, U.: Determination of the aerosol yield of isoprene in the presence of an organic seed with carbon isotope analysis, Environ. Sci. Technol., 43, 6697–6702, https://doi.org/10.1021/es9006959, 2009.
Donahue, N. M., Robinson, A. L., Stanier, C. O., and Pandis, S. N.: Coupled partitioning, dilution, and chemical aging of semivolatile organics, Environ. Sci. Technol., 40, 2635–2643, https://doi.org/10.1021/es052297c, 2006.
Donahue, N. M., Epstein, S. A., Pandis, S. N., and Robinson, A. L.: A two-dimensional volatility basis set: 1. organic-aerosol mixing thermodynamics, Atmos. Chem. Phys., 11, 3303–3318, https://doi.org/10.5194/acp-11-3303-2011, 2011.
Donahue, N. M., Kroll, J. H., Pandis, S. N., and Robinson, A. L.: A two-dimensional volatility basis set – Part 2: Diagnostics of organic-aerosol evolution, Atmos. Chem. Phys., 12, 615–634, https://doi.org/10.5194/acp-12-615-2012, 2012.
Donahue, N. M., Chuang, W., Epstein, S. A., Kroll, J. H., Worsnop, D. R., Robinson, A. L., Adams, P. J., and Pandis, S. N.: Why do organic aerosols exist? Understanding aerosol lifetimes using the two-dimensional volatility basis set, Environ. Chem., 10, 151–157, https://doi.org/10.1071/en13022, 2013.
Donahue, N. M., Robinson, A. L., Trump, E. R., Riipinen, I., and Kroll, J. H.: Volatility and aging of atmospheric organic aerosol, Top Curr. Chem., 339, 97–143, https://doi.org/10.1007/128_2012_355, 2014.
Duncianu, M., David, M., Kartigueyane, S., Cirtog, M., Doussin, J.-F., and Picquet-Varrault, B.: Measurement of alkyl and multifunctional organic nitrates by proton-transfer-reaction mass spectrometry, Atmos. Meas. Tech., 10, 1445–1463, https://doi.org/10.5194/amt-10-1445-2017, 2017.
Ehn, M., Thornton, J. A., Kleist, E., Sipila, M., Junninen, H., Pullinen, I., Springer, M., Rubach, F., Tillmann, R., Lee, B., Lopez-Hilfiker, F., Andres, S., Acir, I. H., Rissanen, M., Jokinen, T., Schobesberger, S., Kangasluoma, J., Kontkanen, J., Nieminen, T., Kurten, T., Nielsen, L. B., Jorgensen, S., Kjaergaard, H. G., Canagaratna, M., Maso, M. D., Berndt, T., Petaja, T., Wahner, A., Kerminen, V. M., Kulmala, M., Worsnop, D. R., Wildt, J., and Mentel, T. F.: A large source of low-volatility secondary organic aerosol, Nature, 506, 476–479, https://doi.org/10.1038/nature13032, 2014.
Eichler, P., Müller, M., D'Anna, B., and Wisthaler, A.: A novel inlet system for online chemical analysis of semi-volatile submicron particulate matter, Atmos. Meas. Tech., 8, 1353–1360, https://doi.org/10.5194/amt-8-1353-2015, 2015.
Eichler, P., Müller, M., Rohmann, C., Stengel, B., Orasche, J., Zimmermann, R., and Wisthaler, A.: Lubricating oil as a major constituent of ship exhaust particles, Environ. Sci. Tech. Let., 4, 54–58, https://doi.org/10.1021/acs.estlett.6b00488, 2017.
Epstein, S. A., Riipinen, I., and Donahue, N. M.: A semiempirical correlation between enthalpy of vaporization and saturation concentration for organic aerosol, Environ. Sci. Technol., 44, 743–748, https://doi.org/10.1021/es902497z, 2010.
Faulhaber, A. E., Thomas, B. M., Jimenez, J. L., Jayne, J. T., Worsnop, D. R., and Ziemann, P. J.: Characterization of a thermodenuder-particle beam mass spectrometer system for the study of organic aerosol volatility and composition, Atmos. Meas. Tech., 2, 15–31, https://doi.org/10.5194/amt-2-15-2009, 2009.
Fredenslund, A., Jones, R. L., and Prausnitz, J. M.: Group-contribution estimation of activity coefficients in nonideal liquid mixtures, AIChE J., 21, 1086–1099, 1975.
Gkatzelis, G. I., Papanastasiou, D. K., Florou, K., Kaltsonoudis, C., Louvaris, E., and Pandis, S. N.: Measurement of nonvolatile particle number size distribution, Atmos. Meas. Tech., 9, 103–114, https://doi.org/10.5194/amt-9-103-2016, 2016.
Gkatzelis, G. I., Tillmann, R., Hohaus, T., Müller, M., Eichler, P., Xu, K.-M., Schlag, P., Schmitt, S. H., Wegener, R., Kaminski, M., Holzinger, R., Wisthaler, A., and Kiendler-Scharr, A.: Comparison of three aerosol chemical characterization techniques utilizing PTR-ToF-MS: a study on freshly formed and aged biogenic SOA, Atmos. Meas. Tech., 11, 1481–1500, https://doi.org/10.5194/amt-11-1481-2018, 2018.
Goldstein, A. H., Worton, D. R., Williams, B. J., Hering, S. V., Kreisberg, N. M., Panić, O., and Górecki, T.: Thermal desorption comprehensive two-dimensional gas chromatography for in-situ measurements of organic aerosols, J. Chromatogr. A, 1186, 340–347, https://doi.org/10.1016/j.chroma.2007.09.094, 2008.
Hallquist, M., Wenger, J. C., Baltensperger, U., Rudich, Y., Simpson, D., Claeys, M., Dommen, J., Donahue, N. M., George, C., Goldstein, A. H., Hamilton, J. F., Herrmann, H., Hoffmann, T., Iinuma, Y., Jang, M., Jenkin, M. E., Jimenez, J. L., Kiendler-Scharr, A., Maenhaut, W., McFiggans, G., Mentel, Th. F., Monod, A., Prévôt, A. S. H., Seinfeld, J. H., Surratt, J. D., Szmigielski, R., and Wildt, J.: The formation, properties and impact of secondary organic aerosol: current and emerging issues, Atmos. Chem. Phys., 9, 5155–5236, https://doi.org/10.5194/acp-9-5155-2009, 2009.
Hildebrandt, L., Henry, K. M., Kroll, J. H., Worsnop, D. R., Pandis, S. N., and Donahue, N. M.: Evaluating the mixing of organic aerosol components using high-resolution aerosol mass spectrometry, Environ. Sci. Technol., 45, 6329–6335, https://doi.org/10.1021/es200825g, 2011.
Hohaus, T., Trimborn, D., Kiendler-Scharr, A., Gensch, I., Laumer, W., Kammer, B., Andres, S., Boudries, H., Smith, K. A., Worsnop, D. R., and Jayne, J. T.: A new aerosol collector for quasi on-line analysis of particulate organic matter: the Aerosol Collection Module (ACM) and first applications with a GC/MS-FID, Atmos. Meas. Tech., 3, 1423–1436, https://doi.org/10.5194/amt-3-1423-2010, 2010.
Hohaus, T., Gensch, I., Kimmel, J. R., Worsnop, D. R., and Kiendler-Scharr, A.: Experimental determination of the partitioning coefficient of β-pinene oxidation products in soas, Phys. Chem. Chem. Phys., 17, 14796–14804, https://doi.org/10.1039/C5CP01608H, 2015.
Hohaus, T., Kuhn, U., Andres, S., Kaminski, M., Rohrer, F., Tillmann, R., Wahner, A., Wegener, R., Yu, Z., and Kiendler-Scharr, A.: A new plant chamber facility, PLUS, coupled to the atmosphere simulation chamber SAPHIR, Atmos. Meas. Tech., 9, 1247–1259, https://doi.org/10.5194/amt-9-1247-2016, 2016.
Holzinger, R., Williams, J., Herrmann, F., Lelieveld, J., Donahue, N. M., and Röckmann, T.: Aerosol analysis using a Thermal-Desorption Proton-Transfer-Reaction Mass Spectrometer (TD-PTR-MS): a new approach to study processing of organic aerosols, Atmos. Chem. Phys., 10, 2257–2267, https://doi.org/10.5194/acp-10-2257-2010, 2010.
Huffman, J. A., Ziemann, P. J., Jayne, J. T., Worsnop, D. R., and Jimenez, J. L.: Development and characterization of a fast-stepping/scanning thermodenuder for chemically-resolved aerosol volatility measurements, Aerosol Sci. Tech., 42, 395–407, https://doi.org/10.1080/02786820802104981, 2008.
Isaacman, G., Kreisberg, N. M., Yee, L. D., Worton, D. R., Chan, A. W. H., Moss, J. A., Hering, S. V., and Goldstein, A. H.: Online derivatization for hourly measurements of gas- and particle-phase semi-volatile oxygenated organic compounds by thermal desorption aerosol gas chromatography (SV-TAG), Atmos. Meas. Tech., 7, 4417–4429, https://doi.org/10.5194/amt-7-4417-2014, 2014.
Isaacman-VanWertz, G., Yee, L. D., Kreisberg, N. M., Wernis, R., Moss, J. A., Hering, S. V., de Sa, S. S., Martin, S. T., Alexander, M. L., Palm, B. B., Hu, W., Campuzano-Jost, P., Day, D. A., Jimenez, J. L., Riva, M., Surratt, J. D., Viegas, J., Manzi, A., Edgerton, E., Baumann, K., Souza, R., Artaxo, P., and Goldstein, A. H.: Ambient gas-particle partitioning of tracers for biogenic oxidation, Environ. Sci. Technol., 50, 9952–9962, https://doi.org/10.1021/acs.est.6b01674, 2016.
Isaacman-VanWertz, G., Massoli, P., O'Brien, R. E., Nowak, J. B., Canagaratna, M. R., Jayne, J. T., Worsnop, D. R., Su, L., Knopf, D. A., Misztal, P. K., Arata, C., Goldstein, A. H., and Kroll, J. H.: Using advanced mass spectrometry techniques to fully characterize atmospheric organic carbon: Current capabilities and remaining gaps, Faraday Discuss., 200, 579–598, https://doi.org/10.1039/C7FD00021A, 2017.
Jang, M. and Kamens, R. M.: Atmospheric secondary aerosol formation by heterogeneous reactions of aldehydes in the presence of a sulfuric acid aerosol catalyst, Environ. Sci. Technol., 35, 4758–4766, https://doi.org/10.1021/es010790s, 2001.
Jaoui, M., Corse, E., Kleindienst, T. E., Offenberg, J. H., Lewandowski, M., and Edney, E. O.: Analysis of secondary organic aerosol compounds from the photooxidation of d-limonene in the presence of nox and their detection in ambient PM2.5, Environ. Sci. Technol., 40, 3819–3828, https://doi.org/10.1021/es052566z, 2006.
Jenkin, M. E.: Modelling the formation and composition of secondary organic aerosol from α- and β-pinene ozonolysis using MCM v3, Atmos. Chem. Phys., 4, 1741–1757, https://doi.org/10.5194/acp-4-1741-2004, 2004.
Jimenez, J. L., Canagaratna, M. R., Donahue, N. M., Prevot, A. S. H., Zhang, Q., Kroll, J. H., DeCarlo, P. F., Allan, J. D., Coe, H., Ng, N. L., Aiken, A. C., Docherty, K. S., Ulbrich, I. M., Grieshop, A. P., Robinson, A. L., Duplissy, J., Smith, J. D., Wilson, K. R., Lanz, V. A., Hueglin, C., Sun, Y. L., Tian, J., Laaksonen, A., Raatikainen, T., Rautiainen, J., Vaattovaara, P., Ehn, M., Kulmala, M., Tomlinson, J. M., Collins, D. R., Cubison, M. J., Dunlea, J., Huffman, J. A., Onasch, T. B., Alfarra, M. R., Williams, P. I., Bower, K., Kondo, Y., Schneider, J., Drewnick, F., Borrmann, S., Weimer, S., Demerjian, K., Salcedo, D., Cottrell, L., Griffin, R., Takami, A., Miyoshi, T., Hatakeyama, S., Shimono, A., Sun, J. Y., Zhang, Y. M., Dzepina, K., Kimmel, J. R., Sueper, D., Jayne, J. T., Herndon, S. C., Trimborn, A. M., Williams, L. R., Wood, E. C., Middlebrook, A. M., Kolb, C. E., Baltensperger, U., and Worsnop, D. R.: Evolution of organic aerosols in the atmosphere, Science, 326, 1525–1529, https://doi.org/10.1126/science.1180353, 2009.
Joback, K. G. and Reid, R. C.: Estimation of pure-component properties from group contributions, Chem. Eng. Commun., 57, 233–243, https://doi.org/10.1080/00986448708960487, 1987.
Kahnt, A.: Semivolatile compounds from atmospheric monoterpene oxidation PhD, Fakultät für Chemie und Mineralogie, Universität Leipzig, Leipzig, Germany, 205 pp., 2012.
Karnezi, E., Riipinen, I., and Pandis, S. N.: Measuring the atmospheric organic aerosol volatility distribution: a theoretical analysis, Atmos. Meas. Tech., 7, 2953–2965, https://doi.org/10.5194/amt-7-2953-2014, 2014.
Krechmer, J. E., Coggon, M. M., Massoli, P., Nguyen, T. B., Crounse, J. D., Hu, W., Day, D. A., Tyndall, G. S., Henze, D. K., Rivera-Rios, J. C., Nowak, J. B., Kimmel, J. R., Mauldin, R. L., 3rd, Stark, H., Jayne, J. T., Sipila, M., Junninen, H., Clair, J. M., Zhang, X., Feiner, P. A., Zhang, L., Miller, D. O., Brune, W. H., Keutsch, F. N., Wennberg, P. O., Seinfeld, J. H., Worsnop, D. R., Jimenez, J. L., and Canagaratna, M. R.: Formation of low volatility organic compounds and secondary organic aerosol from isoprene hydroxyhydroperoxide low-no oxidation, Environ. Sci. Technol., 49, 10330–10339, https://doi.org/10.1021/acs.est.5b02031, 2015.
Kreisberg, N. M., Hering, S. V., Williams, B. J., Worton, D. R., and Goldstein, A. H.: Quantification of hourly speciated organic compounds in atmospheric aerosols, measured by an in-situ thermal desorption aerosol gas chromatograph (tag), Aerosol Sci. Tech., 43, 38–52, https://doi.org/10.1080/02786820802459583, 2009.
Kroll, J. H.: Carbon oxidation state as a metric for describing the chemistry of atmospheric organic aerosol, Nat. Chem., 3, 133–139, https://doi.org/10.1038/nchem.948, 2011.
Kundu, S., Fisseha, R., Putman, A. L., Rahn, T. A., and Mazzoleni, L. R.: High molecular weight SOA formation during limonene ozonolysis: insights from ultrahigh-resolution FT-ICR mass spectrometry characterization, Atmos. Chem. Phys., 12, 5523–5536, https://doi.org/10.5194/acp-12-5523-2012, 2012.
Lee, B.-H., Pierce, J. R., Engelhart, G. J., and Pandis, S. N.: Volatility of secondary organic aerosol from the ozonolysis of monoterpenes, Atmos. Environ., 45, 2443–2452, https://doi.org/10.1016/j.atmosenv.2011.02.004, 2011.
Leungsakul, S., Jaoui, M., and Kamens, R. M.: Kinetic mechanism for predicting secondary organic aerosol formation from the reaction of d-limonene with ozone, Environ. Sci. Technol., 39, 9583–9594, https://doi.org/10.1021/es0492687, 2005a.
Leungsakul, S., Jeffries, H. E., and Kamens, R. M.: A kinetic mechanism for predicting secondary aerosol formation from the reactions of d-limonene in the presence of oxides of nitrogen and natural sunlight, Atmos. Environ., 39, 7063–7082, https://doi.org/10.1016/j.atmosenv.2005.08.024, 2005b.
Li, Y., Pöschl, U., and Shiraiwa, M.: Molecular corridors and parameterizations of volatility in the chemical evolution of organic aerosols, Atmos. Chem. Phys., 16, 3327–3344, https://doi.org/10.5194/acp-16-3327-2016, 2016.
Lopez-Hilfiker, F. D., Mohr, C., Ehn, M., Rubach, F., Kleist, E., Wildt, J., Mentel, Th. F., Lutz, A., Hallquist, M., Worsnop, D., and Thornton, J. A.: A novel method for online analysis of gas and particle composition: description and evaluation of a Filter Inlet for Gases and AEROsols (FIGAERO), Atmos. Meas. Tech., 7, 983–1001, https://doi.org/10.5194/amt-7-983-2014, 2014.
Lopez-Hilfiker, F. D., Mohr, C., Ehn, M., Rubach, F., Kleist, E., Wildt, J., Mentel, Th. F., Carrasquillo, A. J., Daumit, K. E., Hunter, J. F., Kroll, J. H., Worsnop, D. R., and Thornton, J. A.: Phase partitioning and volatility of secondary organic aerosol components formed from α-pinene ozonolysis and OH oxidation: the importance of accretion products and other low volatility compounds, Atmos. Chem. Phys., 15, 7765–7776, https://doi.org/10.5194/acp-15-7765-2015, 2015.
Lopez-Hilfiker, F. D., Mohr, C., D'Ambro, E. L., Lutz, A., Riedel, T. P., Gaston, C. J., Iyer, S., Zhang, Z., Gold, A., Surratt, J. D., Lee, B. H., Kurten, T., Hu, W. W., Jimenez, J., Hallquist, M., and Thornton, J. A.: Molecular composition and volatility of organic aerosol in the southeastern U.S.: Implications for iepox derived soa, Environ. Sci. Technol., 50, 2200–2209, https://doi.org/10.1021/acs.est.5b04769, 2016.
Louvaris, E. E., Florou, K., Karnezi, E., Papanastasiou, D. K., Gkatzelis, G. I., and Pandis, S. N.: Volatility of source apportioned wintertime organic aerosol in the city of athens, Atmos. Environ., 158, 138–147, https://doi.org/10.1016/j.atmosenv.2017.03.042, 2017.
Mackay, D., Bobra, A., Chan, D. W., and Shiu, W. Y.: Vapor-pressure correlations for low-volatility environmental chemicals, Environ. Sci. Technol., 16, 645–649, https://doi.org/10.1021/es00104a004, 1982.
Martinez, R. E., Williams, B. J., Zhang, Y., Hagan, D., Walker, M., Kreisberg, N. M., Hering, S. V., Hohaus, T., Jayne, J. T., and Worsnop, D. R.: Development of a volatility and polarity separator (vaps) for volatility- and polarity-resolved organic aerosol measurement, Aerosol Sci. Tech., 50, 255–271, https://doi.org/10.1080/02786826.2016.1147645, 2016.
McFiggans, G., Topping, D. O., and Barley, M. H.: The sensitivity of secondary organic aerosol component partitioning to the predictions of component properties – Part 1: A systematic evaluation of some available estimation techniques, Atmos. Chem. Phys., 10, 10255–10272, https://doi.org/10.5194/acp-10-10255-2010, 2010.
Mitchem, L. and Reid, J. P.: Optical manipulation and characterisation of aerosol particles using a single-beam gradient force optical trap, Chem. Soc. Rev., 37, 756–769, https://doi.org/10.1039/b609713h, 2008.
Murphy, B. N., Donahue, N. M., Fountoukis, C., Dall'Osto, M., O'Dowd, C., Kiendler-Scharr, A., and Pandis, S. N.: Functionalization and fragmentation during ambient organic aerosol aging: application of the 2-D volatility basis set to field studies, Atmos. Chem. Phys., 12, 10797–10816, https://doi.org/10.5194/acp-12-10797-2012, 2012.
Myrdal, P. B. and Yalkowsky, S. H.: Estimating pure component vapor pressures of complex organic molecules, Ind. Eng. Chem. Res., 36, 2494–2499, https://doi.org/10.1021/ie950242l, 1997.
Nannoolal, Y., Rarey, J., and Ramjugernath, D.: Estimation of pure component properties. Part 3. Estimation of the vapor pressure of non-electrolyte organic compounds via group contributions and group interaction, Fluid Phase Equilibr., 269, 117–133, https://doi.org/10.1016/j.fluid.2008.04.020, 2008.
Odum, J. R., Hoffmann, T., Bowman, F., Collins, D., Flagan, R. C., and Seinfeld, J. H.: Gas/particle partitioning and secondary organic aerosol yields, Environ. Sci. Technol., 30, 2580–2585, https://doi.org/10.1021/es950943+, 1996.
Pankow, J. F.: An absorption model of gas/particle partitioning of organic compounds in the atmosphere, Atmos. Environ., 28, 185–188, 1994.
Pankow, J. F. and Asher, W. E.: SIMPOL.1: a simple group contribution method for predicting vapor pressures and enthalpies of vaporization of multifunctional organic compounds, Atmos. Chem. Phys., 8, 2773–2796, https://doi.org/10.5194/acp-8-2773-2008, 2008.
Pankow, J. F. and Barsanti, K. C.: The carbon number-polarity grid: A means to manage the complexity of the mix of organic compounds when modeling atmospheric organic particulate matter, Atmos. Environ., 43, 2829–2835, https://doi.org/10.1016/j.atmosenv.2008.12.050, 2009.
Pope, F. D., Dennis-Smither, B. J., Grifgiths, P. T., Clegg, S. L., and Cox, R. A.: Studies of single aerosol particles containing malonic acid, glutaric acid, and their mixtures with sodium chloride. I. Hygroscopic growth, J. Phys. Chem., 114, 5335–5341, 2010.
Praplan, A. P., Schobesberger, S., Bianchi, F., Rissanen, M. P., Ehn, M., Jokinen, T., Junninen, H., Adamov, A., Amorim, A., Dommen, J., Duplissy, J., Hakala, J., Hansel, A., Heinritzi, M., Kangasluoma, J., Kirkby, J., Krapf, M., Kürten, A., Lehtipalo, K., Riccobono, F., Rondo, L., Sarnela, N., Simon, M., Tomé, A., Tröstl, J., Winkler, P. M., Williamson, C., Ye, P., Curtius, J., Baltensperger, U., Donahue, N. M., Kulmala, M., and Worsnop, D. R.: Elemental composition and clustering behaviour of α-pinene oxidation products for different oxidation conditions, Atmos. Chem. Phys., 15, 4145–4159, https://doi.org/10.5194/acp-15-4145-2015, 2015.
Prisle, N. L., Engelhart, G. J., Bilde, M., and Donahue, N. M.: Humidity influence on gas-particle phase partitioning of α-pinene + O3 secondary organic aerosol, Geophys. Res. Lett., 37, L01802, https://doi.org/10.1029/2009gl041402, 2010.
Riipinen, I., Pierce, J. R., Donahue, N. M., and Pandis, S. N.: Equilibration time scales of organic aerosol inside thermodenuders: Evaporation kinetics versus thermodynamics, Atmos. Environ., 44, 597–607, https://doi.org/10.1016/j.atmosenv.2009.11.022, 2010.
Rohrer, F., Bohn, B., Brauers, T., Brüning, D., Johnen, F.-J., Wahner, A., and Kleffmann, J.: Characterisation of the photolytic HONO-source in the atmosphere simulation chamber SAPHIR, Atmos. Chem. Phys., 5, 2189–2201, https://doi.org/10.5194/acp-5-2189-2005, 2005.
Shiraiwa, M. and Seinfeld, J. H.: Equilibration timescale of atmospheric secondary organic aerosol partitioning, Geophys. Res. Lett., 39, L24801, https://doi.org/10.1029/2012gl054008, 2012.
Shiraiwa, M., Ammann, M., Koop, T., and Pöschl, U.: Gas uptake and chemical aging of semisolid organic aerosol particles, P. Natl. Acad. Sci. USA, 108, 11003–11008, https://doi.org/10.1073/pnas.1103045108, 2011.
Stark, H., Yatavelli, R. L. N., Thompson, S. L., Kang, H., Krechmer, J. E., Kimmel, J. R., Palm, B. B., Hu, W., Hayes, P. L., Day, D. A., Campuzano-Jost, P., Canagaratna, M. R., Jayne, J. T., Worsnop, D. R., and Jimenez, J. L.: Impact of thermal decomposition on thermal desorption instruments: Advantage of thermogram analysis for quantifying volatility distributions of organic species, Environ. Sci. Technol., 51, 8491–8500, https://doi.org/10.1021/acs.est.7b00160, 2017.
Stein, S. E. and Brown, R. L.: Estimation of normal boiling points from group contributions, J. Chem. Inf. Comp. Sci., 34, 581–587, 1994.
Steitz, B.: Experimental determination of the partitioning coefficient of nopinone as a marker substance in organic aerosol, PhD, Institute of Energy and Climate: Troposphere (IEK-8), Wuppertal University, Forschungszentrum Jülich GmbH, 2010.
Thompson, S. L., Yatavelli, R. L. N., Stark, H., Kimmel, J. R., Krechmer, J. E., Day, D. A., Hu, W., Isaacman-VanWertz, G., Yee, L., Goldstein, A. H., Khan, M. A. H., Holzinger, R., Kreisberg, N., Lopez-Hilfiker, F. D., Mohr, C., Thornton, J. A., Jayne, J. T., Canagaratna, M., Worsnop, D. R., and Jimenez, J. L.: Field intercomparison of the gas/particle partitioning of oxygenated organics during the southern oxidant and aerosol study (soas) in 2013, Aerosol Sci. Tech., 51, 30–56, https://doi.org/10.1080/02786826.2016.1254719, 2017.
Tillmann, R.: PIMMS SAPHIR secondary organic aerosol formation study, IEK-8, Forschungszentrum Jülich, available at: https://data.eurochamp.org/data-access/chamber-experiments/, last access: 13 March 2018.
Tillmann, R., Hallquist, M., Jonsson, Å. M., Kiendler-Scharr, A., Saathoff, H., Iinuma, Y., and Mentel, Th. F.: Influence of relative humidity and temperature on the production of pinonaldehyde and OH radicals from the ozonolysis of a-pinene, Atmos. Chem. Phys., 10, 7057–7072, https://doi.org/10.5194/acp-10-7057-2010, 2010.
Tobias, H. J. and Ziemann, P. J.: Compound identification in organic aerosols using temperature-programmed thermal desorption particle beam mass spectrometry, Anal. Chem., 71, 3428–3435, https://doi.org/10.1021/ac990056f, 1999.
Tobias, H. J. and Ziemann, P. J.: Kinetics of the gas-phase reactions of alcohols, aldehydes, carboxylic acids, and water with the c13 stabilized criegee intermediate formed from ozonolysis of 1-tetradecene, J. Phys. Chem. A, 105, 6129–6135, https://doi.org/10.1021/jp004631r, 2001.
Topping, D., Barley, M., Bane, M. K., Higham, N., Aumont, B., Dingle, N., and McFiggans, G.: UManSysProp v1.0: an online and open-source facility for molecular property prediction and atmospheric aerosol calculations, Geosci. Model Dev., 9, 899–914, https://doi.org/10.5194/gmd-9-899-2016, 2016.
Virtanen, A., Joutsensaari, J., Koop, T., Kannosto, J., Yli-Pirila, P., Leskinen, J., Makela, J. M., Holopainen, J. K., Pöschl, U., Kulmala, M., Worsnop, D. R., and Laaksonen, A.: An amorphous solid state of biogenic secondary organic aerosol particles, Nature, 467, 824–827, https://doi.org/10.1038/nature09455, 2010.
Volkamer, R., Jimenez, J. L., San Martini, F., Dzepina, K., Zhang, Q., Salcedo, D., Molina, L. T., Worsnop, D. R., and Molina, M. J.: Secondary organic aerosol formation from anthropogenic air pollution: Rapid and higher than expected, Geophys. Res. Lett., 33, L17811, https://doi.org/10.1029/2006gl026899, 2006.
Williams, B. J., Goldstein, A. H., Kreisberg, N. M., and Hering, S. V.: An in-situ instrument for speciated organic composition of atmospheric aerosols: Thermal desorptionaerosolgc/ms-fid (tag), Aerosol Sci. Tech., 40, 627–638, https://doi.org/10.1080/02786820600754631, 2006.
Williams, B. J., Jayne, J. T., Lambe, A. T., Hohaus, T., Kimmel, J. R., Sueper, D., Brooks, W., Williams, L. R., Trimborn, A. M., Martinez, R. E., Hayes, P. L., Jimenez, J. L., Kreisberg, N. M., Hering, S. V., Worton, D. R., Goldstein, A. H., and Worsnop, D. R.: The first combined thermal desorption aerosol gas chromatograph–aerosol mass spectrometer (tag-ams), Aerosol Sci. Tech., 48, 358–370, https://doi.org/10.1080/02786826.2013.875114, 2014.
Williams, B. J., Zhang, Y., Zuo, X., Martinez, R. E., Walker, M. J., Kreisberg, N. M., Goldstein, A. H., Docherty, K. S., and Jimenez, J. L.: Organic and inorganic decomposition products from the thermal desorption of atmospheric particles, Atmos. Meas. Tech., 9, 1569–1586, https://doi.org/10.5194/amt-9-1569-2016, 2016.
Yatavelli, R. L. N., Stark, H., Thompson, S. L., Kimmel, J. R., Cubison, M. J., Day, D. A., Campuzano-Jost, P., Palm, B. B., Hodzic, A., Thornton, J. A., Jayne, J. T., Worsnop, D. R., and Jimenez, J. L.: Semicontinuous measurements of gas–particle partitioning of organic acids in a ponderosa pine forest using a MOVI-HRToF-CIMS, Atmos. Chem. Phys., 14, 1527–1546, https://doi.org/10.5194/acp-14-1527-2014, 2014.
Yu, J., Cocker, D. R., Griffin, R. J., Flagan, R. C., and Seinfeld, J. H.: Gas-phase ozone oxidation of monoterpenes: Gaseous and particulate products, J. Atmos. Chem., 34, 207–258, https://doi.org/10.1023/a:1006254930583, 1999.
Zhang, Y. P., Williams, B. J., Goldstein, A. H., Docherty, K., Ulbrich, I. M., and Jimenez, J. L.: A technique for rapid gas chromatography analysis applied to ambient organic aerosol measurements from the thermal desorption aerosol gas chromatograph (tag), Aerosol Sci. Tech., 48, 1166–1182, https://doi.org/10.1080/02786826.2014.967832, 2014.
Zhao, Y., Kreisberg, N. M., Worton, D. R., Isaacman, G., Weber, R. J., Liu, S., Day, D. A., Russell, L. M., Markovic, M. Z., VandenBoer, T. C., Murphy, J. G., Hering, S. V., and Goldstein, A. H.: Insights into secondary organic aerosol formation mechanisms from measured gas/particle partitioning of specific organic tracer compounds, Environ. Sci. Technol., 47, 3781–3787, https://doi.org/10.1021/es304587x, 2013a.
Zhao, Y., Kreisberg, N. M., Worton, D. R., Teng, A. P., Hering, S. V., and Goldstein, A. H.: Development of an in situthermal desorption gas chromatography instrument for quantifying atmospheric semi-volatile organic compounds, Aerosol Sci. Tech., 47, 258–266, https://doi.org/10.1080/02786826.2012.747673, 2013b.
Zuend, A., Marcolli, C., Booth, A. M., Lienhard, D. M., Soonsin, V., Krieger, U. K., Topping, D. O., McFiggans, G., Peter, T., and Seinfeld, J. H.: New and extended parameterization of the thermodynamic model AIOMFAC: calculation of activity coefficients for organic-inorganic mixtures containing carboxyl, hydroxyl, carbonyl, ether, ester, alkenyl, alkyl, and aromatic functional groups, Atmos. Chem. Phys., 11, 9155–9206, https://doi.org/10.5194/acp-11-9155-2011, 2011.