the Creative Commons Attribution 4.0 License.
the Creative Commons Attribution 4.0 License.
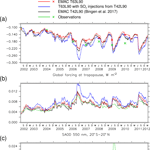
Stratospheric aerosol radiative forcing simulated by the chemistry climate model EMAC using Aerosol CCI satellite data
Christoph Brühl
Jennifer Schallock
Klaus Klingmüller
Charles Robert
Christine Bingen
Lieven Clarisse
Andreas Heckel
Peter North
Landon Rieger
This paper presents decadal simulations of stratospheric and tropospheric aerosol and its radiative effects by the chemistry general circulation model EMAC constrained with satellite observations in the framework of the ESA Aerosol CCI project such as GOMOS (Global Ozone Monitoring by Occultation of Stars) and (A)ATSR ((Advanced) Along Track Scanning Radiometer) on the ENVISAT (European Environmental Satellite), IASI (Infrared Atmospheric Sounding Interferometer) on MetOp (Meteorological Operational Satellite), and, additionally, OSIRIS (Optical Spectrograph and InfraRed Imaging System). In contrast to most other studies, the extinctions and optical depths from the model are compared to the observations at the original wavelengths of the satellite instruments covering the range from the UV (ultraviolet) to terrestrial IR (infrared). This avoids conversion artifacts and provides additional constraints for model aerosol and interpretation of the observations.
MIPAS (Michelson Interferometer for Passive Atmospheric Sounding) SO2 limb measurements are used to identify plumes of more than 200 volcanic eruptions. These three-dimensional SO2 plumes are added to the model SO2 at the eruption times. The interannual variability in aerosol extinction in the lower stratosphere, and of stratospheric aerosol radiative forcing at the tropopause, is dominated by the volcanoes. To explain the seasonal cycle of the GOMOS and OSIRIS observations, desert dust simulated by a new approach and transported to the lowermost stratosphere by the Asian summer monsoon and tropical convection turns out to be essential. This also applies to the radiative heating by aerosol in the lowermost stratosphere. The existence of wet dust aerosol in the lowermost stratosphere is indicated by the patterns of the wavelength dependence of extinction in observations and simulations. Additional comparison with (A)ATSR total aerosol optical depth at different wavelengths and IASI dust optical depth demonstrates that the model is able to represent stratospheric as well as tropospheric aerosol consistently.
- Article
(7163 KB) - Full-text XML
-
Supplement
(313 KB) - BibTeX
- EndNote
Climate effects of stratospheric aerosols can be important, as analyzed for example by Solomon et al. (2011), Santer et al. (2014) and Ridley et al. (2014). Stratospheric aerosol exerts a negative radiative forcing on the troposphere because enhanced scattering by the particles reduces solar radiation reaching the surface and the lower atmosphere. In addition, changes in diffuse light fraction have shown their potential to enhance photosynthesis (Gu et al., 2003). The aim of the present paper is to jointly use model simulations and satellite observations, taking into account the multiple spectral channels of the instruments to better understand the spatiotemporal evolution of the stratospheric aerosol burden and the contribution of the different aerosol types to the observed dynamical aerosol patterns at the different altitudes. Most earlier studies focus on the effects of major volcanic eruptions like Pinatubo (e.g., Aquila et al., 2012; English et al., 2013). For the post-Pinatubo period with only medium size eruptions Mills et al. (2016, 2017) present simulations with the chemistry climate model WACCM (Whole Atmosphere Community Model) with interactive aerosol, using estimates for volcanic injections mostly from nadir sounders. That and the present study contribute to the SPARC/SSIRC initiative (Stratosphere-troposphere Processes And their Role in Climate / Stratospheric Sulfur and Its Role in Climate, see for example Timmreck et al., 2018), aiming at a better understanding of the composition, microphysical and radiative properties characteristics of stratospheric aerosols and their impact on climate (Kremser et al., 2016). In this work, we rely on the multiple instrument satellite dataset provided in the Climate Change Initiative (CCI) of the European Space Agency (ESA) (Popp et al., 2016), which was developed as a tool for evaluation and improvement of the treatment of stratospheric and tropospheric aerosols in global chemistry climate models, like the EMAC (ECHAM5/MESSy Atmospheric Chemistry) model (Brühl et al., 2015). The datasets providing extinctions or total optical depth at wavelengths from the ultraviolet (UV) to terrestrial infrared (IR) are very useful to validate and optimize assumptions on the size distribution and on the composition of aerosol in the model, but also on aerosol sources. Some aspects of the stratospheric part of this study follow up Bingen et al. (2017). The ATSR and IASI datasets provide additional constraints on tropospheric aerosol, especially desert dust. We find in the present study that this latter aerosol compound can penetrate the tropopause via the Asian summer monsoon system and, to a smaller extent, via tropical convection.
The present paper is organized as follows: in Sect. 2, we briefly present the satellite datasets used to evaluate the model, and to check for consistency of observations at different wavelengths: GOMOS, IASI, (A)ATSR and OSIRIS. In Sect. 3 we describe the EMAC model and the various versions and resolutions used in our work, including the use of MIPAS SO2 for input. In Sect. 4, we study the impact of the main aerosol sources on the upper tropospheric and lower stratospheric aerosol burden. The influence of volcanic sources derived from satellite data, but also of dust and organic aerosols, is analyzed. We present examples of the constraints by satellite observations in different spectral regions on different aerosol types with respect to particle size and composition. We discuss the evolution of the optical depth and radiative forcing by stratospheric aerosols, including uncertainties introduced be horizontal model resolution. Finally, we show that the findings concerning the importance of dust for the lower stratosphere are consistent with observations and simulations of tropospheric aerosol. Conclusions are drawn in Sect. 5.
2.1 GOMOS (Global Ozone Monitoring by Occultation of Stars)
GOMOS is an instrument based on the stellar occultation technique (Bertaux et al., 2010) and provides atmospheric measurements in the UV-visible-IR range (248–690, 755–774 and 926–954 nm). The use of stellar occultation results in a high rate of occultation measurements, and, consequently, a very good spatial coverage compared to solar occultation. As a drawback, the signal-to-noise ratio of each measurement is much lower than in the solar case, and varies with the star characteristics (especially its magnitude and its temperature). The operational retrieval, IPF (Instrument Processing Facility), provides density profiles for trace gases such as ozone (O3), nitrogen dioxide (NO2) and nitrogen trioxide (NO3) (Kyrölä et al., 2010), as well as aerosol extinction. However, the extinction shows a poor quality for the reference wavelength at 500 nm. For this reason an alternative inverse algorithm called AerGOM was specifically developed to optimize the aerosol retrieval (Vanhellemont et al., 2016; Robert et al., 2016). AerGOM provides vertical profiles of the same gas species, and the total extinction coefficient for the nongaseous species and its spectral dependence, currently over the range 250–750 nm. The nature of the total extinction fraction for nongaseous species is then inferred using simple criteria based on the geolocation, associated temperature value and extinction value, and each point of the vertical extinction profile is attributed to aerosols, cirrus clouds, polar stratospheric clouds or meteoritic dust.
From the AerGOM extinction, climate data records (CDRs) were developed in the framework of the ESA Aerosol CCI project for different quantities including the aerosol extinction and the related aerosol optical depth at several wavelengths (355, 440, 470, 550 and 750 nm; Bingen et al., 2017). Particular attention was paid to the grid choice, which should optimally render the information contained in the GOMOS measurement set. The most important conclusions of this optimization were that grid resolutions should be chosen to ensure a reasonable statistical sampling in most of the grid cells, and that it should optimally reflect the typical transport of volcanic plumes after an eruption reaching the upper troposphere or the lower stratosphere (UTLS). Therefore, the grid should represent, in a coherent way, the longitudinal and latitudinal air mass transport, and the time needed for this transport. Also, the temporal resolution should be short enough to enable the detection of volcanic signatures, taking into account the typical lifetime of the plume. In this respect, we could verify that time intervals of about 5 days are able to represent the signature of most of the eruptions injecting sulfuric gases in the UTLS, while such a signature is often diluted, underestimated or even disappears in the case of coarser grid cells. This is the case, for instance, for monthly zonal means, even though this representation is very commonly used in the field. The ability of the grid to reproduce the signature of volcanic plume in a satisfactory way is of particularly great importance when the CDRs are used to constrain climate models. More detail about the investigations of the optimal grid choice and all other aspects of the implementation of the CDRs can be found in (Bingen et al., 2017).
In their current version (version 3.0), these CDRs are defined on a grid with a resolution of 5∘ in latitude, 60∘ in longitude, 1 km in altitude and 5-day time period. The records cover the whole ENVISAT period (March 2002–April 2012) and include the total extinction of nongaseous species, but also the polar stratospheric cloud (PSC) fraction and the cloud-free aerosol fraction which is dominated by sulfate aerosols below an altitude of 32 km. It is important to mention that cloud detection is not yet optimal, and that cloud contamination of the aerosol fraction is possible in the UTLS region. This issue is still under investigation.
2.2 IASI (Infrared Atmospheric Sounding Interferometer)
The IASI dust dataset of the Université Libre de Bruxelles (ULB) was generated in the context of ESA CCI's project (Popp et al., 2016). It is based on a statistical regression technique and the use of a neural network trained on synthetic IASI data. A similar scheme has already been applied for the retrieval of NH3 (ammonia; Whitburn et al., 2016; Van Damme et al., 2017). As input variables it uses the IASI L2 pressure, humidity and temperature information, as well as spectral information and a CALIPSO (Cloud-Aerosol Lidar and Infrared Pathfinder Satellite Observation) derived dust altitude climatology. The main output variables are dust optical depth at 10 and 11 µm (and 550 nm). Initial results and validation performance are provided in Popp et al. (2016).
2.3 (A)ATSR ((Advanced) Along Track Scanning Radiometer)
The ATSR (SU) algorithm has been developed at Swansea University for estimation of atmospheric aerosol and surface reflectance for the ATSR-2, AATSR sensors and SLSTR (Sea and Land Surface Temperature Radiometer) on Sentinel-3. Over land, the algorithm employs a parameterized model of the surface angular anisotropy (North, 2002), and uses the dual-view capability of the instrument to allow aerosol property estimation without a priori assumptions on surface spectral reflectance. Over ocean, the algorithm uses a simple a priori model of ocean surface reflectance at both nadir and along-track view angles. A climatology (Kinne et al., 2006) is used to constrain chemical composition of the aerosol components at latitude–longitude grid, while the method retrieves aerosol size and optical thickness on a 10 km grid. Both optical thickness and size are retrieved as vertical column values. Size is not resolved vertically, but is represented by fraction of fine and coarse mode aerosol in total. The algorithm has been developed from initial prototype (Bevan et al., 2012) under the Aerosol CCI program, and results and validation performance for version 4.21 are provided in Popp et al. (2016). The version used here (v 4.3) differs from that summarized in Popp et al. (2016) by improvements in retrieval of coarse/fine mode fraction, and improved cloud screening over ocean in the region of dense plumes, resulting in approximately 10 % greater coverage, with small improvement in correlation against AERONET (AErosol RObotic NETwork) values. AERONET is recognized as a reference dataset for validation of satellite data products (Holben et al., 1998).
2.4 OSIRIS (Optical Spectrograph and InfraRed Imager) – an additional instrument
OSIRIS was launched on board the Odin satellite, and has provided vertical profiles of limb scattered radiance between 280 and 810 nm since 2001 (Llewellyn et al., 2004). The radiance profiles are inverted to provide aerosol extinction measurements at 750 nm at altitudes between 10 and 35 km with a vertical resolution of approximately 2 km (Bourassa et al., 2012). This technique provides high sampling rates with hundreds of measurements per day over the sunlit portion of the globe, enabling excellent spatial and temporal sampling of short-lived events. OSIRIS aerosol extinction retrievals agree well with coincident occultation measurements from Stratospheric Aerosol and Gas Experiments II and III during background periods but have known low biases above approximately 25 km, and will have some cloud contamination near and below the tropopause (Bourassa et al., 2012; Rieger et al., 2015). Additionally, seasonal biases are possible due to the orbital geometry and changes in aerosol optical properties such as after volcanic eruptions may also bias the retrievals. These effects are described in more detail by Rieger et al. (2014, 2018). This work uses the OSIRIS version 5.10 aerosol retrieval (Bourassa et al., 2018) averaged into daily, 5∘ latitude by 30∘ longitude bins for comparisons.
For the simulations of the radiative and chemical effects of stratospheric aerosol, the ECHAM5 (5th generation of European Centre Hamburg) general circulation model coupled to the Modular Earth Submodel System Atmospheric Chemistry (EMAC) was used (Brühl et al., 2015), updated to the version of Jöckel et al. (2010). In contrast to Jöckel et al. (2016) – who use stratospheric aerosol extinction climatologies derived from observations – our model setup aerosol and its optical properties are calculated from precursor gases and emissions. As dust reaching the UTLS region turned out to be sensitive to model resolution, we used different model resolutions: the T42 resolution (spectral, 2.75∘ in latitude and longitude) of the previous studies, T63 resolution (1.88∘), the standard resolution for the stratosphere used in this study and T106 resolution (1.1∘) for a 1-year sensitivity test. The vertical grid has 90 layers from the surface up to 0.01 hPa (80 km altitude, short L90) with finest resolution in the boundary layer and near the tropopause. For T106 only simulations with the low top model version with 31 levels up to 30 km altitude (L31), the setup used by Klingmüller et al. (2018), which is well tested regarding the representation of tropospheric aerosol, are discussed here in detail. In all simulations, except the T42L90 one of the previous studies, the meteorology below about the 100 hPa level is nudged to the reanalysis ERA-Interim (Jöckel et al., 2006). The simulations were performed for the ENVISAT time period from July 2002 to March 2012 to allow for the use of data from MIPAS for input, and GOMOS and ATSR for validation. The period from 1997 to 2002 using SAGE II (Stratospheric Aerosol and Gas Experiment) was simulated first to get consistent initial conditions.
The applied aerosol module GMXE (Pringle et al., 2010) accounts for seven modes using lognormal size distributions (nucleation mode, soluble and insoluble Aitken, accumulation and coarse modes). The boundary between accumulation mode and coarse mode, a model parameter, is set at a dry particle radius of 1.6 µm to avoid too fast sedimentation of a too large coarse mode fraction in case of major volcanic eruptions. For dust sensitivity studies in T106 which focus on the troposphere, a boundary of 1.0 µm is also used. The mode parameters are used for every aerosol type and listed for convenience in Table S1 of the Supplement. Optical properties for the types sulfate, dust, organic carbon and black carbon (OC and BC), sea salt, and aerosol water are calculated using Mie-theory-based lookup tables consistent with the selected size distribution widths of the modes. The resulting optical depths, single scattering albedos and asymmetry factors are used in radiative transfer calculations which (except for the T106 low top sensitivity studies) feedback to atmospheric dynamics. The contribution of stratospheric aerosol to (instantaneous) radiative forcing and heating is calculated online via multiple calls of the radiation module.
The mineral dust emissions are calculated online using the emission scheme of Astitha et al. (2012) which builds on previous studies by Pérez et al. (2006), Spyrou et al. (2010), Laurent et al. (2008, 2010), Marticorena et al. (1997), Zender et al. (2003) and Tegen (2002). The emission scheme parameterizes saltation bombardment and aggregate disintegration by sand blasting, combining the surface friction velocity with descriptions of land cover type, clay fraction of the soil and vegetation cover. For an improved representation of dust at higher resolution, we adopted the updates presented by Klingmüller et al. (2018) in the T106L31 simulation.
Aerosol module parameters, for example the composition of sea salt, were optimized on the basis of the satellite data. We apply the chemical speciation of the sea salt emission flux used by Abdelkader et al. (2015) as listed in Table S2 of the Supplement. The sea salt composition affects the hygroscopic growth and thereby the AOD. The setting of Jöckel et al. (2016), dominated by Na and Cl ions, which we initially applied in our simulations produced very high AOD levels over the North Pacific which are not consistent with the satellite observations.
SO2 plumes (sulfur dioxide) from about 230 explosive volcanic eruptions into the stratosphere were derived from 3-dimensional data fields of MIPAS (Höpfner et al., 2015) and, in case of data gaps, of GOMOS on ENVISAT with a temporal resolution of 5 days, and added as volume mixing ratio to the simulated SO2 at the time of the eruption. Each identified volcanic eruption (with names from the Smithsonian volcanic database, http://www.volcano.si.edu, last access: 31 August 2018) is listed in an emission inventory published recently (Bingen et al., 2017), which provides an estimate of the altitude and the amount of SO2 injected into the atmosphere. The table and the 3-D fields of volcanic SO2 are available at https://doi.org/10.1594/WDCC/SSIRC_1. These data were derived from MIPAS within the uncertainty range but nearer the upper end for best results with the model resolution T42L90 and free running mode, which has some artifacts from the convection scheme and a dry bias at the tropical tropopause. For the nudged T63L90 simulation, the volcanic SO2 data of the inventory have to be downscaled by about a factor of 0.7 which is actually closer to the most likely MIPAS measurements. The actual values for each injection, which depend on the time span between the eruptions and on corrections for data gaps, are given in the Supplement (Table S3). Boundary conditions for background concentrations of SO2 from outgassing volcanoes into the troposphere are taken from the monthly climatology of Diehl et al. (2012) truncated at 200 hPa to avoid double counting in the stratosphere. The sulfur source gas OCS (carbonyl sulfide) is constrained by observed monthly zonal average surface volume mixing ratios (update of the data by Montzka et al., 2007). Marine DMS (dimethyl sulfide) as a natural sulfur source is also included in the model, using a module for exchange fluxes between seawater and atmosphere by Pozzer et al. (2006) and the Lana et al. (2011) climatology. For anthropogenic emissions of CO (carbon monoxide), NOx (nitrogen oxides), sulfur, OC and BC the DLR- MACCity emission inventory is used. Biomass burning is based on ACCMIP-MACCity and GFEDv2, OC-SOA (secondary organic aerosol) on AEROCOM_UMZ1. For details on these emission inventories selected for the Chemistry Climate Model Initiative (CCMI) see Jöckel et al. (2016).
4.1 Volcanic eruptions
Volcanic emissions have a large impact on the stratospheric aerosol burden. Even small and moderate eruptions contribute to the stratospheric aerosol load due to convective transport of SO2 and its gradual uplift to the upper troposphere and the lower stratosphere, and resulting accumulation of sulfate aerosol. Volcanic SO2 injections explain most of the interannual variability of stratospheric aerosol extinction (decadal logarithm) observed by GOMOS, as depicted in Fig. 1 at three wavelengths. For each wavelength (350 nm in Fig. 1a, b; 550 nm in Fig. 1c, d and 750 nm in Fig. 1e, f), the GOMOS time series (Fig. 1a, c, e) showing the altitude dependence in the tropics, is compared with the EMAC simulation in resolution T63L90 including the dust contribution (Fig. 1b, d, f; see Sect. 4.2 for more detail). Figure 1 shows, at all three wavelengths, that an enhancement of the extinction value is observed around 16–18 km, corresponding to the aerosol load resulting from a succession of volcanic eruptions during the whole period 2002–2012. The eruptions of Nabro in June 2011 and the successive eruptions of Soufriere Hills and Rabaul in 2006 have the largest effects on extinction in the lower stratosphere in the observations and the simulation. The best agreement between GOMOS and EMAC is found in the case of the extinction at 550 nm (Fig. 1c, d), where the quality of the GOMOS retrieval is the best. At 750 nm (Fig. 1e, f) also, GOMOS measurements agree well with EMAC for the aerosol layer (16–22 km) where measured extinction values exceed km−1. At lower altitudes (14–16 km), rather unstructured patterns of enhanced extinction are found by GOMOS, probably corresponding to cloud contamination. At 350 nm, where a decrease in the GOMOS quality is expected due to a loss in signal-to-noise ratio obtained in the UV spectral region while using cold stars, still the volcanic events stick out. More details over these aspects can be found in references (Robert et al., 2016; Bingen et al., 2017). Bingen et al. (2017) also present the latitude dependence of 550 nm aerosol extinction at 17 km altitude as observed by GOMOS and simulated by EMAC in the coarse resolution T42L90 in their Fig. 10.
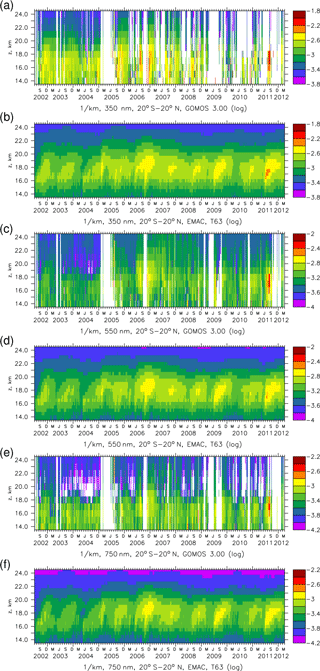
Figure 1GOMOS and EMAC extinctions (log) in the tropics as a function of altitude for different wavelengths: (a, b) UV 350 nm, (c, d) visible 550 nm and (e, f) near-infrared 750 nm; resolution T63L90.
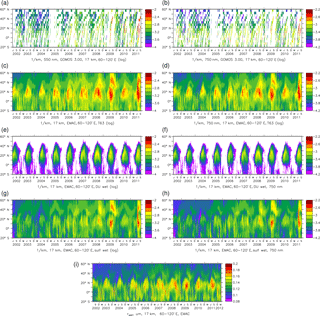
Figure 2Observed (a, b) and simulated (c, d, EMAC T63L90) extinction in the Asian sector (60–120∘ E, 20∘ S–60∘ N) for 550 nm (a, c) and 750 nm (b, d). Contribution of wet dust (e, f) and wet sulfate (g, h) to extinction for 550 nm (e, g) and 750 nm (f, h). (i) Median wet radius in accumulation mode (for effective radius multiply by 1.4).
4.2 Dust and organics from the troposphere in the UTLS)
Extinction in the lowermost stratosphere and upper troposphere is to a large fraction due to desert dust and organic carbon aerosol. These contributions were strongly underestimated in Brühl et al. (2015) due to a crude parameterization in the used model version based on Jöckel et al. (2006), but overestimated in Bingen et al. (2017). Both simulations were performed in the relatively coarse resolution T42L90. Dust reaching the UTLS is sensitive to model resolution, mostly via the convection parameterization (Tiedtke, 1989). In Fig. 1 the simulated extinction at resolution T63L90 fits well to the GOMOS observations which appear to have a seasonal contribution from the Asian summer monsoon. For more detailed analysis, Fig. 2 shows observed and simulated extinction in the Asian sector at 17 km in the visible and the near-IR. The largest extinction values are indeed found at the location and time of the Asian summer monsoon at the altitude of outflow. This feature is clearest in years not perturbed by medium strength volcanic eruptions, for example 2010. For a clear separation, the contributions of wet dust and wet sulfate to extinction are displayed separately (Fig. 2e–h). The wet dust particles in the monsoon region have a larger median wet radius than the volcanic sulfate particles (e.g., from Sarychev in 2009, Fig. 2i) which is consistent with a relatively larger extinction in the infrared compared to the visible in the monsoon region in observations and simulations. Figure 2a–d demonstrates that dust is essential to reproduce the observations. Total extinction without wet dust in T63L90 is shown in the Supplement. Comparing Fig. S1b with Fig. 2g shows a small contribution of organics from biomass burning in northern spring (for volume mixing ratios see Fig. S2). Figure S1 also contains results from the T42L90 simulation of Bingen et al. (2017), showing that for this resolution the contribution of wet dust to extinction has to be downscaled (i.e., divided) by a factor of 2 to get agreement (Fig. S1d, factor of 3 if only dry dust is considered).
Observations by IASI and ATSR indicate a maximum in dust aerosol optical depth (DAOD) in early Northern Hemisphere summer over the Asian deserts located in the inflow regions of the monsoon (see Sect. 4.4). A similar feature is found in the simulations by EMAC. This supports our findings that desert dust is also important for the UTLS.
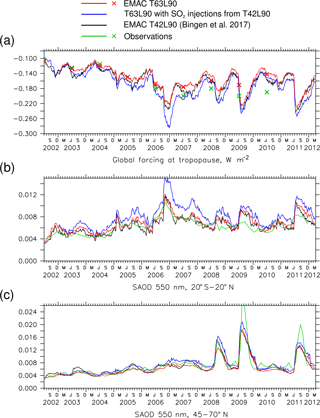
Figure 3(a) Stratospheric aerosol radiative forcing, (b, c) stratospheric AOD for tropics and midlatitudes. Red lines and crosses: EMAC, resolution T63L90, current version; black: EMAC T42L90 (Bingen et al., 2017); blue: T63L90 without downscaling the SO2 injections for T42L90; green: from observations (crosses annual mean for forcing; Solomon et al., 2011; SAGE II, CALIPSO, OSIRIS).
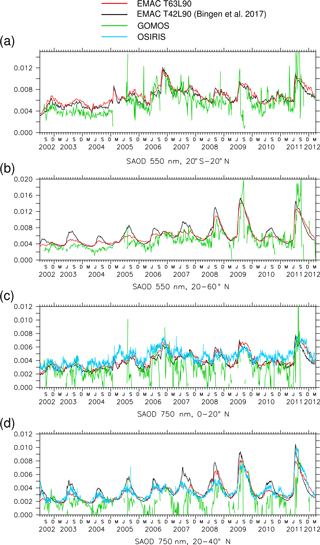
Figure 4(a, b) Stratospheric AOD at 550 nm observed by GOMOS (green) and simulated by EMAC in resolutions T42L90 (black) and T63L90 (red). (c, d) Stratospheric AOD at 750 nm in the northern tropics and subtropics (SAOD above 15 km), additionally with OSIRIS observations (light blue).
4.3 Stratospheric aerosol radiative forcing, stratospheric aerosol optical depth and radiative heating
Desert dust transported to the UTLS mostly via the Asian summer monsoon contributes significantly to the seasonal cycle of total stratospheric aerosol optical depth (SAOD) in satellite observations and the EMAC simulations shown in Fig. 3b for the tropics (vertical integral of extinction above about 16 km) and in Fig. 3c for midlatitudes (above about 14 km). Global radiative forcing at the tropopause is depicted in Fig. 3a. The figure contains in black results from the T42L90 simulation of Bingen et al. (2017) and in blue the T63L90 simulation with the high volcanic sulfur input derived for the coarse resolution. Green lines and symbols show estimates derived from satellite observations (SAGE II, OSIRIS and CALIPSO; Solomon et al., 2011; Santer et al., 2014; Bourassa et al., 2012; Glantz et al., 2014). Red shows results of the current model version in T63L90 with the Astitha et al. (2012) dust scheme and corrected SO2 input (see Sect. 3 and Supplement). Concerning global radiative forcing, the volcanoes are the dominating effect with up to 0.13 W m−2 for Rabaul and Nabro compared to the volcanically quiet period in 2002. Here the use of the SO2 inventory for T42L90 in the T63L90 simulation (blue) causes an overestimate of up to 50 % in 2006 and 2007 due to accumulation effects of eruptions following in short sequence. This is visible in the overestimate of tropical SAOD depicted by the blue curve in Fig. 3b.
Especially in northern midlatitude summer SAOD in T42L90 appears to be high because at that resolution the convective transport of dust to the UTLS in the Asian monsoon region is overestimated (Fig. 3c). This is clearly seen in Fig. 4 which shows in black the T42L90 simulation, in green the observations of 550 and 750 nm SAOD by GOMOS, and in light blue (Fig. 4c, d only) by OSIRIS in different latitude bands, including the monsoon region. For the narrow latitude bands in Fig. 4c and d, inclusion of OSIRIS data is important because GOMOS coverage is often too low. Nevertheless, for a lot of features the two satellite datasets agree well. Using the higher resolution T63L90, for which the convection parameterization was developed, the agreement with the satellite observations is much better (Figs. 3 and 4, red) than with T42L90, especially at midlatitudes and in the subtropics. In the subtropics (Fig. 4d), the simulation with low resolution (black) always overestimates the monsoon peaks in August compared to the ones seen in the observations. Comparing the model results with OSIRIS in the northern tropics (Fig. 4c) indicates that some volcanic events are still missing in the inventory, for example in spring 2007 and 2010. This would also explain the differences in radiative forcing (indicated by crosses in Fig. 3a) in these years.
The simulated aerosol radiative heating, derived from radiation calls with and without aerosol, reflects the medium volcanic eruptions with the largest effects near 18 km (Fig. 5). There, desert dust causes additional heating at the time of the Asian summer monsoon. In the UTLS, below, every year in September, a clear signal from biomass burning organic aerosol – its volume mixing ratio is shown in Fig. S2 of the Supplement – is visible. Above, around 22 km, the dust below in Northern Hemisphere summer causes a reduction of absorption of terrestrial radiation by ozone.
4.4 Constraints from total aerosol optical depth in different spectral regions and for different aerosol subsets
The first comparisons are carried out for EMAC in T63L90, the standard resolution used in the previous sections. Here AOD refers to the troposphere and stratosphere. The DAOD (dust AOD) in terrestrial infrared is most sensitive to the coarse mode of tropospheric dust. Figure 6a, b shows that the model reproduces most of the IASI features. DAOD in the visible spectral region (Fig. 6c, d) is too high over central Asia, pointing to an overestimate of dust in the accumulation mode near the Taklamakan Desert. The patterns in the IR and visible spectral range are different despite considering the factor 2 often applied by the AEROCOM/AEROSAT (Aerosol Comparison between Observations and Models) community for conversion in the color scales of Fig. 6a, b and c, d. This holds for model and observations. The fine mode AOD fraction, which is dominated by the accumulation mode, is slightly overestimated over Europe and underestimated in the biomass burning regions in Africa (Fig. 6e, f). In the model this is sensitive to the way the extinction of aerosol water is attributed to the soluble aerosol species, especially sea salt. Absorbing AOD, i.e., AOD × (1−ω) with ω representing single scattering albedo, agrees surprisingly well (Fig. 6g, h). In the total AOD (Fig. 6i, j) there appears to be too much sea salt in the model, or still suboptimal parameters for the sea salt composition which controls water uptake (see Sect. 3).
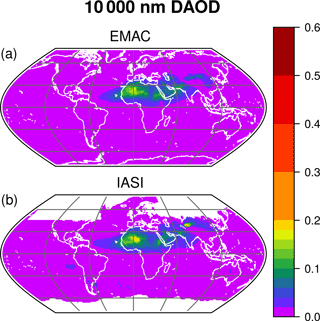
Figure 7Annual mean for 2011 of the DAOD at 10 µm wavelength observed by IASI (b, IASI ULB dataset version 8) and simulated by EMAC (a) at T106L31 resolution.
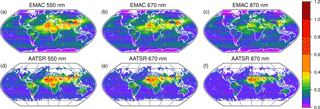
Figure 8Annual mean for 2011 of the AOD at (from left to right) 550, 670 and 870 nm wavelength observed by AATSR (d, e, f; SU-ATSR algorithm version 4.3) and simulated by EMAC (a, b, c) at T106L31 resolution.
Figure 7 compares the annual average for 2011 of the 10 µm DAOD observed by IASI and simulated by EMAC in the low top version with high horizontal resolution (T106L31, about 1.1∘). The satellite retrievals are taken from version 8 of the ULB dataset. The simulation uses the dust emission scheme of Klingmüller et al. (2018) which calculates the emissions online considering the meteorological conditions. To extract the DAOD from the total EMAC AOD at 10 µm, we apply a filter nullifying sea-salt-dominated AOD values. To identify the latter, we compare the AOD weighted with the volume of sea salt and dust.
The observed and modeled global DAOD distributions shown in Fig. 7 agree remarkably well. The pixel values of each map are strongly correlated with a correlation coefficient of 0.91. The overall AOD level is consistent as well, so that a similar variance in the pixel values is obtained for the observed (0.00038) and the modeled (0.00041) DAOD distribution. Interestingly, the DAOD from the older version 7 of the ULB dataset yields a pixel by pixel correlation coefficient of only 0.89 and a pixel value variance of only 0.00029. We conclude that the agreement of EMAC and IASI has improved with the update from version 7 to version 8 of the IASI ULB dataset.
The main disagreement of the two maps in Fig. 7 is the less pronounced maximum over the Taklamakan Desert in central Asia in the model result. This underestimation is related to the model surface friction velocity in mountainous regions like the surroundings of the Taklamakan Desert, which tends to be lower in simulations at higher horizontal resolution (e.g., T106) than at lower resolution (e.g., T63), possibly resulting in an underestimation of the dust emissions.
Figure 8 compares results from the T106L31 EMAC simulation for the annual average of the total AOD at visible and near-infrared wavelengths with AASTR retrievals using the ATSR (SU) algorithm version 4.3. Generally good agreement is obtained at 550 nm which is consistent with the good agreement between the 550 nm MODIS (Moderate-resolution Imaging Spectroradiometer) AOD and model results based on the same EMAC version (Klingmüller et al., 2018). As for the T63L90 simulation, the model yields higher sea-salt-related AOD levels over the oceans. In contrast, the model AOD over the Sahara is lower than the satellite retrieved values. This becomes even more evident at larger wavelengths (Fig. 8c, f): the model AOD over the Sahara, in contrast to most other regions, has a stronger wavelength dependence than the observed AOD, corresponding to a larger Ångström exponent. This discrepancy might be resolved by adjusting the dust particle size distribution in the model under the constraint of not sacrificing the good agreement of model and observed AOD at 550 nm and at 10 µm. This could involve modifying the parameters of the log-normal modes, i.e., their widths and boundaries, but also reassessing the parameterization of relevant processes such as emission, deposition, coagulation and hygroscopic growth, or even adding an extra mode for extremely coarse particles which can be relevant close to dust sources. Over South America, the biomass burning regions of Africa, and India and China the wavelength dependence of model and observed AOD is largely consistent.
Satellite data are not only important to constrain model parameters but they are also very important for model improvement. Comparing satellite data with model results at different wavelengths simultaneously provides additional information and is also valuable for the satellite community to check internal consistency, as in our case for GOMOS and OSIRIS.
Sophisticated modeling of dust and organic aerosol as well as a detailed volcano dataset are necessary to reproduce the seasonal cycle and the interannual variability in extinction in the lowermost stratosphere observed by GOMOS at different wavelengths. From the wavelength dependence in observations and simulations, aerosol in the UTLS with enhanced particle size due to water uptake can be identified as aged dust in the Asian monsoon region. Convective transport of dust into the UTLS is resolution dependent because of differences in convection top height and overshooting convection. A resolution of T63L90 (1.88∘ in longitude and latitude, 90 vertical layers) fits best to the observations. For the low resolution T42L90 (2.75∘), dust SAOD (and stratospheric mixing ratio) has to be downscaled by a factor of about 0.33; for higher resolutions (e.g., T106L90), upscaling is required. The resolution dependent differences in convection also modify the residence time of sulfur species in the lowermost stratosphere, and especially at low latitudes, at resolution T42L90, it appears to be too short.
The total AOD in the visible spectral range is very sensitive to aerosol water and the composition of sea salt. In the modal model, the bulk fraction has to be increased compared to ions to reduce artifacts of too much water uptake by sea salt. The satellite data helped to identify a preferred parameter set for the sea salt emission composition.
Our simulated dust total aerosol optical depth agrees with satellite data in the visible (ATSR SU) and the infrared (IASI ULB, version 8). The combined comparison at visible and infrared wavelengths provides strong constraints on the modeled particle size distribution. The direct comparison of observations and model reveals different structures in the extinction patterns at both spectral ranges. From this, we conclude that simply assuming a spatially constant factor of (about) 2 for conversion of DAOD from 10 µm to 550 nm, as commonly applied in the AEROCOM/AEROSAT community, is too crude.
Satellite datasets identifying volcanic SO2, including its vertical distribution or enhanced extinction by aged dust enable the model to get closer to observationally based estimates for radiative forcing, showing the interest of a close interaction between modeling and observation research teams.
The Aerosol CCI satellite data are available at ICARE, Lille. All model output of EMAC used here is stored at DKRZ, Hamburg, and available on request. This includes 5-day averages and 10-hourly values. Volcanic SO2 input data are available at https://doi.org/10.1594/WDCC/SSIRC_1 (Brühl, 2018).
The supplement related to this article is available online at: https://doi.org/10.5194/acp-18-12845-2018-supplement.
CBr wrote the paper and performed the stratospheric simulations, supported by JS. KK performed the tropospheric simulations and provided code for the stratospheric part. CBi and CR provided the GOMOS data and the corresponding text, LC the IASI data; PN and AH provided the ATSR data, and LR the OSIRIS data.
The authors declare that they have no conflict of interest.
This article is part of the special issue “The Modular Earth Submodel System (MESSy) (ACP/GMD inter-journal SI)”. It is not associated with a conference.
This study was funded by the Aerosol CCI project, phase II, of the ESA Climate Change
Initiative, as a user option, and by the EU FP7 project STRATOCLIM.
Supporting work for the development of GOMOS datasets was performed in the
framework of a Marie Curie Career Integration Grant within the 7th European
Community Framework Programme under grant agreement no. 293560. The satellite
data, except OSIRIS, were provided via the Aerosol CCI database at ICARE,
Lille, France; the model simulations were performed at DKRZ, Hamburg,
Germany, where the results are also stored.
The article processing charges for this open-access
publication were covered by the Max Planck Society.
Edited by: Farahnaz Khosrawi
Reviewed by: two anonymous referees
Abdelkader, M., Metzger, S., Mamouri, R. E., Astitha, M., Barrie, L., Levin, Z., and Lelieveld, J.: Dust–air pollution dynamics over the eastern Mediterranean, Atmos. Chem. Phys., 15, 9173–9189, https://doi.org/10.5194/acp-15-9173-2015, 2015. a
Aquila, V., Oman, L. D., Stolarski, R. S., Colarco, P. R., and Newman, P. A.: Dispersion of the volcanic sulfate cloud from a Mount Pinatubolike eruption, J. Geophys. Res., 117, D06216, https://doi.org/10.1029/2011JD016968, 2012. a
Astitha, M., Lelieveld, J., Abdel Kader, M., Pozzer, A., and de Meij, A.: Parameterization of dust emissions in the global atmospheric chemistry-climate model EMAC: impact of nudging and soil properties, Atmos. Chem. Phys., 12, 11057–11083, https://doi.org/10.5194/acp-12-11057-2012, 2012. a, b
Bertaux, J. L., Kyrölä, E., Fussen, D., Hauchecorne, A., Dalaudier, F., Sofieva, V., Tamminen, J., Vanhellemont, F., Fanton d'Andon, O., Barrot, G., Mangin, A., Blanot, L., Lebrun, J. C., Pérot, K., Fehr, T., Saavedra, L., Leppelmeier, G. W., and Fraisse, R.: Global ozone monitoring by occultation of stars: an overview of GOMOS measurements on ENVISAT, Atmos. Chem. Phys., 10, 12091–12148, https://doi.org/10.5194/acp-10-12091-2010, 2010. a
Bevan, S., North, P., Los, S., and Grey, W.: A global dataset of atmospheric aerosol optical depth and surface reflectance from AATSR, Remote Sens. Environ., 116, 199–210, 2012. a
Bingen, C., Robert, C. E., Stebel, K., Brühl, C., Schallock, J., Vanhellemont, F., Mateshvili, N., Höpfner, M., Trickl, T., Barnes, J. E., Jumelet, J., Vernier, J.-P., Popp, T., de Leeuw, G., and Pinnock, S.: Stratospheric aerosol data records for the climate change initiative: Development, validation and application to chemistry-climate modelling, Remote Sens. Environ., 203, 296–321, 2017. a, b, c, d, e, f, g, h, i, j
Bourassa, A. E., Rieger, L. A., Lloyd, N. D., and Degenstein, D. A.: Odin-OSIRIS stratospheric aerosol data product and SAGE III intercomparison, Atmos. Chem. Phys., 12, 605–614, https://doi.org/10.5194/acp-12-605-2012, 2012. a, b, c
Bourassa, A. E., Roth, C. Z., Zawada, D. J., Rieger, L. A., McLinden, C. A., and Degenstein, D. A.: Drift-corrected Odin-OSIRIS ozone product: algorithm and updated stratospheric ozone trends, Atmos. Meas. Tech., 11, 489–498, https://doi.org/10.5194/amt-11-489-2018, 2018. a
Brühl, C.: Volcanic SO2 data derived from limb viewing satellites for the lower stratosphere from 1998 to 2012, and from nadir viewing satellites for the troposphere. World Data Center for Climate (WDCC) at DKRZ, https://doi.org/10.1594/WDCC/SSIRC_1, 2018. a
Brühl, C., Lelieveld, J., Tost, H., Höpfner, M., and Glatthor, N.: Stratospheric sulphur and its implications for radiative forcing simulated by the chemistry climate model EMAC, J. Geophys. Res.-Atmos. 120, 2103–2118, https://doi.org/10.1002/2014JD022430, 2015. a, b, c
Diehl, T., Heil, A., Chin, M., Pan, X., Streets, D., Schultz, M., and Kinne, S.: Anthropogenic, biomass burning, and volcanic emissions of black carbon, organic carbon, and SO2 from 1980 to 2010 for hindcast model experiments, Atmos. Chem. Phys. Discuss., 12, 24895–24954, https://doi.org/10.5194/acpd-12-24895-2012, 2012. a
English, J. M., Toon, O. B., and Mills, M. J.: Microphysical simulations of large volcanic eruptions: Pinatubo and Toba, J. Geophys. Res.-Atmos, 118, 1880–1895, https://doi.org/10.1002/jgrd.50196, 2013. a
Glantz, P., Bourassa, A., Herber, A., Iversen, T., Karlsson, J., and Kirkevåg, A.: Remote sensing of aerosols in the Arctic for an evaluation of evaluation of global climate model simulations, J. Geophys. Res.-Atmos., 119, 8169–8188, https://doi.org/10.1002/2013JD021279, 2014. a
Gu, L. H., Baldocchi, D. D., Wofsy, S. C., Munger, J. W., Michalsky, J. J., Urbanski, S. P., and Boden, T. A.: Response of a deciduous forest to the mount Pinatubo eruption: Enhanced photosynthesis, Science, 299, 2035–2038, 2003. a
Holben, B. N., Eck, T. F., Slutsker, I., Tanré, D., Buis, J. P., Setzer, A., Vermote, E., Reagan, J. A., Kaufman, Y. J., Nakajima, T., Lavenu, F., Jankowiak, I., and Smirnov, A.: AERONET – A Federated Instrument Network and Data Archive for Aerosol Characterization, Remote Sens. Environ., 66, 1–16, 1998. a
Höpfner, M., Boone, C. D., Funke, B., Glatthor, N., Grabowski, U., Günther, A., Kellmann, S., Kiefer, M., Linden, A., Lossow, S., Pumphrey, H. C., Read, W. G., Roiger, A., Stiller, G., Schlager, H., von Clarmann, T., and Wissmüller, K.: Sulfur dioxide (SO2) from MIPAS in the upper troposphere and lower stratosphere 2002–2012, Atmos. Chem. Phys., 15, 7017–7037, https://doi.org/10.5194/acp-15-7017-2015, 2015. a
Jöckel, P., Tost, H., Pozzer, A., Brühl, C., Buchholz, J., Ganzeveld, L., Hoor, P., Kerkweg, A., Lawrence, M. G., Sander, R., Steil, B., Stiller, G., Tanarhte, M., Taraborrelli, D., van Aardenne, J., and Lelieveld, J.: The atmospheric chemistry general circulation model ECHAM5/MESSy1: consistent simulation of ozone from the surface to the mesosphere, Atmos. Chem. Phys., 6, 5067–5104, https://doi.org/10.5194/acp-6-5067-2006, 2006. a, b
Jöckel, P., Kerkweg, A., Pozzer, A., Sander, R., Tost, H., Riede, H., Baumgaertner, A., Gromov, S., and Kern, B.: Development cycle 2 of the Modular Earth Submodel System (MESSy2), Geosci. Model Dev., 3, 717–752, https://doi.org/10.5194/gmd-3-717-2010, 2010. a
Jöckel, P., Tost, H., Pozzer, A., Kunze, M., Kirner, O., Brenninkmeijer, C. A. M., Brinkop, S., Cai, D. S., Dyroff, C., Eckstein, J., Frank, F., Garny, H., Gottschaldt, K.-D., Graf, P., Grewe, V., Kerkweg, A., Kern, B., Matthes, S., Mertens, M., Meul, S., Neumaier, M., Nützel, M., Oberländer-Hayn, S., Ruhnke, R., Runde, T., Sander, R., Scharffe, D., and Zahn, A.: Earth System Chemistry integrated Modelling (ESCiMo) with the Modular Earth Submodel System (MESSy) version 2.51, Geosci. Model Dev., 9, 1153–1200, https://doi.org/10.5194/gmd-9-1153-2016, 2016. a, b, c
Kinne, S., Schulz, M., Textor, C., Guibert, S., Balkanski, Y., Bauer, S. E., Berntsen, T., Berglen, T. F., Boucher, O., Chin, M., Collins, W., Dentener, F., Diehl, T., Easter, R., Feichter, J., Fillmore, D., Ghan, S., Ginoux, P., Gong, S., Grini, A., Hendricks, J., Herzog, M., Horowitz, L., Isaksen, I., Iversen, T., Kirkevåg, A., Kloster, S., Koch, D., Kristjansson, J. E., Krol, M., Lauer, A., Lamarque, J. F., Lesins, G., Liu, X., Lohmann, U., Montanaro, V., Myhre, G., Penner, J., Pitari, G., Reddy, S., Seland, O., Stier, P., Takemura, T., and Tie, X.: An AeroCom initial assessment – optical properties in aerosol component modules of global models, Atmos. Chem. Phys., 6, 1815–1834, https://doi.org/10.5194/acp-6-1815-2006, 2006. a
Klingmüller, K., Metzger, S., Abdelkader, M., Karydis, V. A., Stenchikov, G. L., Pozzer, A., and Lelieveld, J.: Revised mineral dust emissions in the atmospheric chemistry–climate model EMAC (MESSy 2.52 DU_Astitha1 KKDU2017 patch), Geosci. Model Dev., 11, 989–1008, https://doi.org/10.5194/gmd-11-989-2018, 2018. a, b, c, d
Kremser, S., Thomason, L. W., von Hobe, M., Hermann, M., Deshler, T., Timmreck, C., Toohey, M., Stenke, A., Schwarz, J. P., Weigel, R., Fueglistaler, S., Prata, F. J., Vernier, J.-P., Schlager, H., Barnes, J. E., Antuña-Marrero, J.-C., Fairlie, D., Palm, M., Mahieu, E., Notholt, J., Rex, M., Bingen, C., Vanhellemont, F. Bourassa, A., Plane, J. M. C., Klocke, D., Carn, S. A., Clarisse, L., Trickl, T., Neely, R., James, A. D., Rieger, L., Wilson, J. C., and Meland, B.: Stratospheric aerosol – Observations, processes, and impact on climate, Rev. Geophys., 54, 278–335, https://doi.org/10.1002/2015RG000511, 2016. a
Kyrölä, E., Tamminen, J., Sofieva, V., Bertaux, J. L., Hauchecorne, A., Dalaudier, F., Fussen, D., Vanhellemont, F., Fanton d'Andon, O., Barrot, G., Guirlet, M., Fehr, T., and Saavedra de Miguel, L.: GOMOS O3, NO2, and NO3 observations in 2002–2008, Atmos. Chem. Phys., 10, 7723–7738, https://doi.org/10.5194/acp-10-7723-2010, 2010. a
Lana, A., Bell, T. G., Simó, R., Vallina, S. M., Ballabrera-Poy, J., Kettle, A. J., Dachs, J., Bopp, L., Saltzman, E. S., Stefels, J., Johnson, J. E., and Liss, P. S.: An updated climatology of surface dimethlysulfide concentrations and emission fluxes in the global ocean, Global Biogeochem. Cy., 25, GB1004, https://doi.org/10.1029/2010GB003850, 2011. a
Laurent, B., Marticorena, B., Bergametti, G., Léon, J. F., and Mahowald, N. M.: Modeling mineral dust emissions from the Sahara desert using new surface properties and soil database, J. Geophys. Res.-Atmos., 113, D14218, https://doi.org/10.1029/2007JD009484, 2008. a
Laurent, B., Tegen, I., Heinold, B., Schepanski, K., Weinzierl, B., and Esselborn, M.: A model study of Saharan dust emissions and distributions during the SAMUM-1 campaign, J. Geophys. Res.-Atmos., 115, D21210, https://doi.org/10.1029/2009JD012995, 2010. a
Llewellyn, E. J., Lloyd, N. D., Degenstein, D. A., Gattinger, R. L., Petelina, S. V., Bourassa, A. E., Wiensz, J. T., Ivanov, E. V., McDade, I. C., Solheim, B. H., McConnell, J. C, Haley, C. S., von Savigny, C., Sioris, C. E., McLinden, C. A., Griffioen, E., Kaminski, J., Evans, W. F. J., Puckrin, E., Strong, K., Wehrle, V., Hum, R. H., Kendall, D. J. W., Matsushita, J., Murtagh, D. P., Brohede, S., Stegman, J., Witt, G., Barnes, G., Payne, W. F., Piché, L., Smith, K., Warshaw, G., Deslauniers, D.-L., Marchand, P., Richardson, E. H., King, R. A., Wevers, I., McCreath, W., Kyrölä, E., Oikarinen, L., Leppelmeier, G. W., Auvinen, H., Mégie, G., Hauchecorne, A., Lefèvre, F., de La Nöe, J., Ricaud, P., Frisk, U., Sjoberg, F., von Schéele, F., and Nordh, L.: The OSIRIS instrument on the Odin spacecraft, Can. J. Phys., 82, 411–422, 2004. a
Marticorena, B., Bergametti, G., Aumont, B., Callot, Y., N'Doumé, C., and Legrand, M.: Modeling the atmospheric dust cycle: 2. Simulation of Saharan dust sources, J. Geophys. Res.-Atmos., 102, 4387–4404, https://doi.org/10.1029/96JD02964, 1997. a
Mills, M. J., Schmidt, A., Easter, R., Solomon, S., Kinnison, D. E., Ghan, S. J., Neely III, R. R., Marsh, D. R., Conley, A., Bardeen, C. G., and Gettelman, A.: Global volcanic aerosol properties derived from emissions, 1990–2014, using CESM1(WACCM), J. Geophys. Res.-Atmos., 121, 2332–2348, https://doi.org/10.1002/2015JD024290, 2016. a
Mills, M. J., Richter, J. H., Tilmes, S., Kravitz, B., MacMartin, D. G., Glanville, A. A., and Kinnison, D. E.: Radiative and chemical response to interactive stratospheric sulfate aerosols in fully coupled CESM1(WACCM), J. Geophys. Res.-Atmos., 122, 13061–13078, https://doi.org/10.1002/2017JD027006, 2017. a
Montzka, S. A., Calvert, P., Hall, B. D., Elkins, J. W., Conway, T. J., Tans, P. P., and Sweeney, C.: On the global distribution, seasonality, and budget of atmospheric carbonyl sulfide and some similarities with CO2, J. Geophys. Res., 112, D09302, https://doi.org/10.1029/2006JD007665, 2007. a
North, P.: Estimation of aerosol opacity and land surface bidirectional reflectance from ATSR-2 dual-angle imagery: Operational method and validation, J. Geophys. Res., 107, 4149, https://doi.org/10.1029/2000JD000207, 2002. a
Pérez, C., Nickovic, S., Baldasano, J. M., Sicard, M., Rocadenbosch, F., and Cachorro, V. E.: A long Saharan dust event over the western Mediterranean: Lidar, Sun photometer observations, and regional dust modeling, J. Geophys. Res.-Atmos., 111, D15214, https://doi.org/10.1029/2005JD006579, 2006. a
Popp, T., de Leeuw, G., Bingen, C., Brühl, C., Capelle, V., Chedin, A., Clarisse, L., Dubovik, O., Grainger, R., Griesfeller, J., Heckel, A., Kinne, S., Klüser, L., Kosmale, M., Kolmonen, P., Lelli, L., Litvinov, P., Mei, L., North, P., Pinnock, S., Povey, A., Robert, C., Schulz, M., Sogacheva, L., Stebel, K., Stein-Zweers, D., Thomas, G., Tilstra, L. G., Vandenbussche, S., Veefkind, P., Vountas, M., and Xue, Y.: Development, production and evaluation of aerosol climate data records from European satellite observations (Aerosol_cci), Remote Sens., 8, 421, https://doi.org/10.3390/rs8050421, 2016. a, b, c, d, e
Pozzer, A., Jöckel, P., Sander, R., Williams, J., Ganzeveld, L., and Lelieveld, J.: Technical Note: The MESSy-submodel AIRSEA calculating the air-sea exchange of chemical species, Atmos. Chem. Phys., 6, 5435–5444, https://doi.org/10.5194/acp-6-5435-2006, 2006. a
Pringle, K. J., Tost, H., Message, S., Steil, B., Giannadaki, D., Nenes, A., Fountoukis, C., Stier, P., Vignati, E., and Lelieveld, J.: Description and evaluation of GMXe: a new aerosol submodel for global simulations (v1), Geosci. Model Dev., 3, 391–412, https://doi.org/10.5194/gmd-3-391-2010, 2010. a
Ridley, D. A., Solomon, S., Barnes, J.E ., Burlakov, V. D., Deshler, T., Dolgii, S. I., Herber, A. B., Nagai, T., Neely III, R. R., Nevzorov, A. V., Ritter, C., Sakai, T., Santer, B. D., Sato, M., Schmidt, A., Uchino, O., and Vernier, J. P.: Total volcanic stratospheric aerosol optical depths and implications for global climate change, Geophys. Res. Lett., 41, 7763–7769, https://doi.org/10.1002/2014GL061541, 2014. a
Rieger, L. A., Bourassa, A. E., and Degenstein, D. A.: Stratospheric aerosol particle size information in Odin-OSIRIS limb scatter spectra, Atmos. Meas. Tech., 7, 507–522, https://doi.org/10.5194/amt-7-507-2014, 2014. a
Rieger, L. A., Bourassa, A. E., and Degenstein, D. A.: Merging the OSIRIS and SAGE II stratospheric aerosol records, J. Geophys. Res.-Atmos., 120, 8890–8904, https://doi.org/10.1002/2015JD023133, 2015. a
Rieger, L. A., Malinina, E. P., Rozanov, A. V., Burrows, J. P., Bourassa, A. E., and Degenstein, D. A.: A study of the approaches used to retrieve aerosol extinction, as applied to limb observations made by OSIRIS and SCIAMACHY, Atmos. Meas. Tech., 11, 3433–3445, https://doi.org/10.5194/amt-11-3433-2018, 2018. a
Robert, C. É., Bingen, C., Vanhellemont, F., Mateshvili, N., Dekemper, E., Tétard, C., Fussen, D., Bourassa, A., and Zehner, C.: AerGOM, an improved algorithm for stratospheric aerosol extinction retrieval from GOMOS observations – Part 2: Intercomparisons, Atmos. Meas. Tech., 9, 4701–4718, https://doi.org/10.5194/amt-9-4701-2016, 2016. a, b
Santer, B. D., Bonfils, C., Painter, J. F., Zelinka, M. D., Mears, C., Solomon, S., Schmidt, G. A., Fyfe, J. C., Cole, J. N. S., Nazarenko, L., Taylor, K. E., and Wentz, F. J.: Volcanic contribution to decadal changes in tropospheric temperature, Nat. Geosci., 7, 185–189, 2014. a, b
Solomon, S., Daniel, J. S., Neely III, R. R., Vernier, J. P., Dutton, E. G., and Thomason, L. W.: The persistently variable “background” stratospheric aerosol layer and global climate change, Science, 333, 866–870, 2011. a, b, c
Spyrou, C., Mitsakou, C., Kallos, G., Louka, P., and Vlastou, G.: An improved limited area model for describing the dust cycle in the atmosphere, J. Geophys. Res.-Atmos., 115, D17211, https://doi.org/10.1029/2009JD013682, 2010. a
Tegen, I.: Impact of vegetation and preferential source areas on global dust aerosol: Results from a model study, J. Geophys. Res., 107, 4576, https://doi.org/10.1029/2001JD000963, 2002. a
Tiedtke, M.: A comprehensive mass flux scheme for cumulus parameterization in large-scale models, Mon. Weather Rev., 117, 1779–1800, 1989. a
Timmreck, C., Mann, G. W., Aquila, V., Hommel, R., Lee, L. A., Schmidt, A., Brühl, C., Carn, S., Chin, M., Dhomse, S. S., Diehl, T., English, J. M., Mills, M. J., Neely, R., Sheng, J., Toohey, M., and Weisenstein, D.: The Interactive Stratospheric Aerosol Model Intercomparison Project (ISA-MIP): motivation and experimental design, Geosci. Model Dev., 11, 2581–2608, https://doi.org/10.5194/gmd-11-2581-2018, 2018. a
Van Damme, M., Whitburn, S., Clarisse, L., Clerbaux, C., Hurtmans, D., and Coheur, P.-F.: Version 2 of the IASI NH3 neural network retrieval algorithm: near-real-time and reanalysed datasets, Atmos. Meas. Tech., 10, 4905–4914, https://doi.org/10.5194/amt-10-4905-2017, 2017. a
Vanhellemont, F., Mateshvili, N., Blanot, L., Robert, C. É., Bingen, C., Sofieva, V., Dalaudier, F., Tétard, C., Fussen, D., Dekemper, E., Kyrölä, E., Laine, M., Tamminen, J., and Zehner, C.: AerGOM, an improved algorithm for stratospheric aerosol extinction retrieval from GOMOS observations – Part 1: Algorithm description, Atmos. Meas. Tech., 9, 4687–4700, https://doi.org/10.5194/amt-9-4687-2016, 2016. a
Whitburn, S., Van Damme, M., Clarisse, L., Bauduin, S., Heald, C. L., Hadji-Lazaro, J., Hurtmans, D., Zondlo, M. A., Clerbaux, C., and Coheur, P.-F.: A flexible and robust neural network IASI-NH3 retrieval algorithm, J. Geophys. Res.-Atmos., 121, 6581–6599, https://doi.org/10.1002/2016JD024828, 2016. a
Zender, C. S., Bian, H., and Newman, D.: Mineral Dust Entrainment and Deposition (DEAD) model: Description and 1990s dust climatology, J. Geophys. Res.-Atmos., 108, 4416, https://doi.org/10.1029/2002JD002775, 2003. a