the Creative Commons Attribution 4.0 License.
the Creative Commons Attribution 4.0 License.
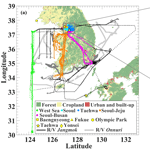
Evaluating high-resolution forecasts of atmospheric CO and CO2 from a global prediction system during KORUS-AQ field campaign
Avelino F. Arellano
Joshua P. DiGangi
Yonghoon Choi
Glenn S. Diskin
Anna Agustí-Panareda
Mark Parrington
Sebastien Massart
Benjamin Gaubert
Youngjae Lee
Danbi Kim
Jinsang Jung
Jinkyu Hong
Je-Woo Hong
Yugo Kanaya
Mindo Lee
Ryan M. Stauffer
Anne M. Thompson
James H. Flynn
Jung-Hun Woo
Accurate and consistent monitoring of anthropogenic combustion is imperative because of its significant health and environmental impacts, especially at city-to-regional scale. Here, we assess the performance of the Copernicus Atmosphere Monitoring Service (CAMS) global prediction system using measurements from aircraft, ground sites, and ships during the Korea-United States Air Quality (KORUS-AQ) field study in May to June 2016. Our evaluation focuses on CAMS CO and CO2 analyses as well as two higher-resolution forecasts (16 and 9 km horizontal resolution) to assess their capability in predicting combustion signatures over east Asia. Our results show a slight overestimation of CAMS CO2 with a mean bias against airborne CO2 measurements of 2.2, 0.7, and 0.3 ppmv for 16 and 9 km CO2 forecasts, and analyses, respectively. The positive CO2 mean bias in the 16 km forecast appears to be consistent across the vertical profile of the measurements. In contrast, we find a moderate underestimation of CAMS CO with an overall bias against airborne CO measurements of −19.2 (16 km), −16.7 (9 km), and −20.7 ppbv (analysis). This negative CO mean bias is mostly seen below 750 hPa for all three forecast/analysis configurations. Despite these biases, CAMS shows a remarkable agreement with observed enhancement ratios of CO with CO2 over the Seoul metropolitan area and over the West (Yellow) Sea, where east Asian outflows were sampled during the study period. More efficient combustion is observed over Seoul ( ppbv ppmv−1) compared to the West Sea ( ppbv ppmv−1). This “combustion signature contrast” is consistent with previous studies in these two regions. CAMS captured this difference in enhancement ratios (Seoul: 8–12 ppbv ppmv−1, the West Sea: ∼30 ppbv ppmv−1) regardless of forecast/analysis configurations. The correlation of CAMS CO bias with CO2 bias is relatively high over these two regions (Seoul: 0.64–0.90, the West Sea: ∼0.80) suggesting that the contrast captured by CAMS may be dominated by anthropogenic emission ratios used in CAMS. However, CAMS shows poorer performance in terms of capturing local-to-urban CO and CO2 variability. Along with measurements at ground sites over the Korean Peninsula, CAMS produces too high CO and CO2 concentrations at the surface with steeper vertical gradients (∼0.4 ppmv hPa−1 for CO2 and 3.5 ppbv hPa−1 for CO) in the morning samples than observed (∼0.25 ppmv hPa−1 for CO2 and 1.7 ppbv hPa−1 for CO), suggesting weaker boundary layer mixing in the model. Lastly, we find that the combination of CO analyses (i.e., improved initial condition) and use of finer resolution (9 km vs. 16 km) generally produces better forecasts.
- Article
(16524 KB) - Full-text XML
-
Supplement
(3706 KB) - BibTeX
- EndNote
Anthropogenic combustion significantly impacts air quality, climate, ecosystem, agriculture, and public health at local to global scales (Charlson et al., 1992; Doney et al., 2007; Feely et al., 2004; Heald et al., 2006; Maher et al., 2016). This is especially the case in megacities where human activities are most intense, accompanied by immense energy consumption, mainly in the form of fossil-fuel combustion, which directly leads to enhanced emissions of air pollutants, greenhouse gases, and waste energy. In particular, cities in the Asian region that are rapidly developing in recent decades are subject to more frequent severe pollution conditions (Yang, 2013; Guo et al., 2014; Ohara et al., 2007; Shindell et al., 2008, 2011). It is imperative therefore that we enhance our current capability to monitor, verify, and assess anthropogenic combustion and its impacts as the number of megacities across the globe is expected to rapidly grow in the following decades (United Nations, 2016). The Copernicus Atmosphere Monitoring Service (CAMS) has a state-of-the-art global and integrated prediction system that is currently being implemented to meet this need. The service is funded by the European Union and it builds upon a legacy of projects such as the Monitoring Atmospheric Composition and Climate (MACC) and Global and Regional Earth System Monitoring Using Satellite and In Situ Data (GEMS) (Hollingsworth et al., 2008).
Table 1Configuration of CAMS global atmospheric composition products valid during the period of the Korea-United States Air Quality (KORUS-AQ) field campaign (May to June 2016). The tracers evaluated in this paper are highlighted in boldface. Time availability is in number of days with respect to real time (n/a is used when this is not applicable).
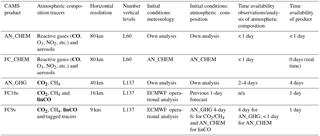
For nearly a decade, CAMS has been operationally producing daily global near-real-time forecasts and analyses of reactive trace gases, greenhouse gases, and aerosols including global reanalyses and estimation of emissions of these atmospheric constituents (Morcrette et al., 2009; Benedetti et al., 2009; Kaiser et al., 2012; Flemming et al., 2015, 2017; Massart et al., 2016; Agustí-Panareda et al., 2014, 2017). CAMS global forecasts and analyses are based on the Integrated Forecasting System (IFS) of the European Centre for Medium-Range Weather Forecasts (ECMWF), which is also used for numerical weather prediction (NWP). CAMS recently developed two forecasts at higher resolution, which have potential advantages compared to lower-resolution analysis and/or forecast, in terms of local-to-regional air quality (Table 1).
The Korea-United States Air Quality (KORUS-AQ) field measurement campaign offers a unique opportunity to assess the accuracy and consistency of the high-resolution forecast and analysis system of CAMS and its skill in simulating atmospheric CO2 from anthropogenic combustion. During May to June 2016, the KORUS-AQ field campaign collected comprehensive measurements of air quality (including CO2 and tracers of fossil-fuel combustion) over the South Korean peninsula and its surrounding waters. KORUS-AQ is an international collaboration between the US and South Korea to better understand the factors controlling air quality in the region across urban, rural, and coastal interfaces (Kim and Park, 2014, KORUS-AQ White Paper). This field campaign follows several NASA-led suborbital missions in the past focusing on air quality in the United States (e.g., DISCOVER-AQ, SEAC4RS) and pollution outflows from Asia (e.g., TRACE-P, INTEX-B, ARCTAS), and integrating the measurements from these campaigns to satellite retrievals and air quality models (Crawford and Pickering, 2014; Toon et al., 2016; Jacob et al., 2003, 2010; Singh et al., 2009). Local measurements over the West (Yellow) Sea, often representative of Chinese pollution outflow, and over the Seoul metropolitan area provide a rich dataset that is very useful in evaluating global prediction and analysis systems like CAMS at city-to-regional scale.
In this study, we evaluate CAMS forecast and analysis of fossil-fuel combustion signatures over the KORUS-AQ spatial and temporal domain. In particular, we use measurements of the main products of combustion (i.e., CO and CO2; Gamnitzer et al., 2006) from the NASA DC-8 aircraft, along with observations from five ground sites, two research ships, and four satellites to assess the capability of CAMS to monitor anthropogenic combustion. Although CAMS CO and CO2 forecasts and analyses have been evaluated previously (Agustí-Panareda et al., 2014, 2016, 2017; Claeyman et al., 2010; Massart et al., 2016; Flemming et al., 2009, 2015, 2017), this study is unique for the following reasons. (1) This study is a joint evaluation of CO and CO2 species, including their associated enhancement ratios which provide insight on CAMS representation of anthropogenic combustion processes. (2) A focus on megacities provides an important baseline investigation. This is especially the case in east Asia where there is still lack of detailed information and measurements to constrain emission inventories. (3) KORUS-AQ provides a unique opportunity to evaluate the new high-resolution global CAMS forecasts of CO and CO2 at local-to-regional scale. This paper begins with a brief description of CAMS and KORUS-AQ (Sect. 2), followed by an evaluation of CAMS with airborne measurements (Sect. 3) and with ground sites, ships, and satellites (Sect. 4). We provide a summary of our findings in Sect. 5.
2.1 CAMS CO and CO2 forecasts and analysis
CAMS has been providing global forecasts and analysis of atmospheric composition on a daily basis at ECMWF for nearly a decade with applications on air quality and monitoring of long-lived greenhouse gases. CAMS uses the IFS for NWP to assimilate a wealth of meteorological observations plus satellite products of atmospheric composition to produce atmospheric analysis of reactive gases (e.g., CO, O3, NO2, SO2), aerosols, and long-lived greenhouse gases (e.g., CO2, CH4) on the NWP model grid which are then used as initial conditions to forecast the atmospheric composition with a 5-day lead time. The IFS simulates transport of the chemical species (Flemming et al., 2009; Agustí-Panareda et al., 2017) and includes the online integration of modules for atmospheric chemistry (Flemming et al., 2015, 2017) and biogenic CO2 fluxes from terrestrial vegetation (Boussetta et al., 2013) to model atmospheric composition in conjunction with an assimilation system based on four-dimensional variational (4D-Var) data assimilation (Rabier et al., 2000; Inness et al., 2015). The CAMS global atmospheric analysis and prediction system runs at different resolutions and at a different lag times for the various atmospheric species depending on the use of chemistry in the model and the timeliness of the satellite retrievals used in the analysis. The system providing reactive trace gases and aerosols runs at approximately 80 km horizontal resolution with 60 vertical levels, and its analysis is available less than 1 day behind real time. While higher horizontal and vertical resolution are used for the analysis and forecasts of greenhouse gases, the analysis of CO2 and CH4 is available at around 40 km in the horizontal and 137 vertical levels. Currently, the forecasts of CO2 and CH4 have the same resolution as the operational weather forecast at ECMWF (137 levels with 9 km horizontal resolution) but previously their resolution was 16 km (from 2015 to 2016). A CO tracer with simplified chemistry based on a linear CO scheme (Massart et al., 2015) is also available in the high-resolution forecasts. However, the CO2 and CH4 analysis is only available 4 days behind real time as the satellite retrievals are not available closer to real time. Because of this, in the 16 km resolution forecast, CO2, CH4, and linear CO are free running, and only the meteorology is initialized with the meteorological operational analysis (see Agustí-Panareda et al., 2014 for further details on the free-running forecast configuration). Following a recent improvement in the timeliness of the satellite retrievals, the linear CO is initialized with CO analysis, while CO2 and CH4 are initialized with a 4-day forecast from the CO2 and CH4 40 km analysis in the 9 km forecasts. In order not to lose the small-scale features in the initialization process, a spectral filter is applied to only adjust the large scales in the initial conditions of the forecast (Sebastien Massart, personal communication, 2016). Table 1 (as well as Fig. S1 in the Supplement) provides a summary of the three CAMS configurations and five resulting CAMS products evaluated in this paper and Fig. S2 depicts the different vertical and horizontal resolutions used in the different CAMS configurations.
For this study, we focus on evaluating the three CO and CO2 forecasts and analysis products listed above, namely, CO2 and CO 16 km forecasts (FC16s), analyses (ANs) of CO2 (at 40 km) and CO (at 80 km), and relatively recent CAMS 9 km CO2 and CO forecast products (FC9s) which are initialized from their respective analysis. The FC9s are different from FC16s in terms of both resolution and initialization as described above (e.g., the FC16s are produced from a free-running simulation of CO2 and CO). The near-real-time ANs of CO and CO2 are also different from FC16s and FC9s as these ANs continuously assimilate satellite retrievals of CO total column from the Measurements Of Pollution In The Troposphere (MOPITT V5-TIR) and the Infrared Atmospheric Sounding Interferometer (IASI) (Inness et al., 2015), and column-averaged dry-air mole fractions of CO2 (XCO2) from the Greenhouse gases Observing Satellite (GOSAT) (Massart et al., 2016), in addition to the available meteorological data. Observations of both CO and CO2 are assimilated in 12 h assimilation windows. Inness et al. (2015) found that CO total column field, vertical distribution, and concentrations in the lower troposphere are improved by assimilating the CO total column from MOPITT. Assimilation of the GOSAT XCO2 led to improvements in mean absolute error and bias variability in XCO2 fields during the year 2013 (Massart et al., 2016). FC9s CO are initialized from MOPITT and IASI CO analysis at a previous time, which are then downscaled from 80 km to 9 km by a spectral filtering scheme. Due to observational and computing constraints, FC9s of CO2 are initialized and downscaled from a 96 h forecast of CO2 initialized by GOSAT analysis 4 days earlier.
The IFS contains several components, including an atmospheric general circulation model, a land surface model, an ocean wave model, an ocean general circulation model, and perturbation models for the data assimilation and forecast (Persson, 2001). Model dynamics and numerical procedures, and physical processes are documented in IFS documentation Cy43r3 (ECMWF, 2017; https://www.ecmwf.int/search/elibrary/part?title=part&year=2017&secondary_title=IFS, last access: 15 May 2018). Detailed cloud and precipitation physics of the IFS benefits the calculation of wet deposition (Flemming et al., 2017). As for emissions and surface fluxes, CAMS uses the Global Fire Assimilation System (GFAS) for biomass burning fluxes of CO2 (Kaiser et al., 2012). CAMS uses the anthropogenic CO2 fluxes that are based on the annual mean of the Emission Database for Global Atmospheric Research version 4.2 (EDGARv4.2). As the most recent year available for EDGARv4.2 is 2008, estimated and climatological trends are used to extrapolate to the years after 2008. The land vegetation fluxes for CO2 are calculated online by the carbon module of the land surface model in IFS CTESSEL (Boussetta et al., 2013). A biogenic flux adjustment scheme (BFAS) is employed in CAMS to improve the continental budget of CO2 fluxes (Agustí-Panareda et al., 2014, 2016). Specifically, (1) BFAS computes the scaling factors for the model net ecosystem exchange (NEE) based on reference (NEE climatology from the optimized fluxes); (2) the scaling factors are used to adjust biogenic CO2 fluxes from the land surface model (i.e., flux bias correction); (3) the bias-corrected fluxes are then used to simulate the atmospheric CO2. According to Agustí-Panareda et al. (2016), in northern Asia, the employment of BFAS slightly decreases NEE in May and has negligible impacts on NEE in June. CO2 overestimation by CAMS over the Northern Hemisphere (NH) in winter and spring is enhanced by BFAS. For CO, CAMS uses anthropogenic and biogenic emissions that are based on the MACC/CityZEN EU projects (MACCity) (Granier et al., 2011), and a climatology of the Model of Emissions of Gases and Aerosols from Nature developed under the MACC (MEGAN-MACC) emission inventories (Sindelarova et al., 2014). GFAS is also used for fire emissions. ANs for CO use the online implemented chemical mechanism (C-IFS-CB05; Flemming et al., 2015) that is an extended version of the Carbon Bond mechanism 5 (CB05; Yarwood et al., 2005). Because hydroxyl radical (OH) is an important sink for CO, modeled OH is critical for the simulation of CO (Gaubert et al., 2016, 2017). In the ANs for CO, the global and NH means of air mass-weighted OH are and molecules cm−3 during May 2016, respectively (calculated following recommendations from Lawrence et al., 2001). The mean OH from the ANs for CO is consistent with previous studies (e.g., Lawrence et al., 2001; Lelieveld et al., 2016; Gaubert et al., 2016, 2017). A linear chemistry scheme (C-IFS-LINCO) is used in FC16s and FC9s for CO for computational expediency (Claeyman et al., 2010; Massart et al., 2015; Eskes et al., 2017). C-IFS-LINCO computes CO sources and sinks using the approach developed by Cariolle and Déqué (1986) and updated by Cariolle and Teyssèdre (2007), without direct use of modeled OH. C-IFS-LINCO is less computationally demanding than the full chemistry, permitting simulations at higher resolutions (Massart et al., 2015). Key aspects of the three CAMS configurations evaluated in this study are listed in Table 1.
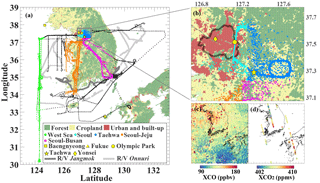
Figure 1Domain of the study and KORUS-AQ measurements used in this study. Panel (a) shows land cover of the domain (Broxton et al., 2014), DC-8 aircraft tracks, ship tracks, and location of ground sites. The airborne measurements are classified into five groups (the West (Yellow) Sea, Seoul, Taehwa, Seoul–Jeju jetway, and Seoul–Busan jetway), as marked in bright green, bright blue, mazarine blue, orange, and magenta. The ground sites are labeled with bright yellow markers. The Olympic Park and Yonsei sites are located in urban regions (Seoul) while the Baengnyeong and Fukue (Kanaya et al., 2016) sites are located in remote regions. The Taehwa (Kim et al., 2013) site is located in a forest near Seoul. Tracks of the two ships are marked in dark grey (R/V Jangmok) and light grey (R/V Onnuri). Also shown in panel (b) is the zoomed-in version of the grey box in panel (a). Panel (c) shows a composite MOPITT XCO retrievals during KORUS-AQ campaign while panel (d) shows OCO-2 XCO2 retrievals in the same time period.
2.1.1 CO and CO2 measurements during KORUS-AQ
KORUS-AQ is a comprehensive field campaign based on international collaboration between the US and South Korea (https://espo.nasa.gov/korus-aq, last access: 10 June 2018). The goal is to better understand the factors controlling air quality (AQ) in the region across urban, rural, and coastal interfaces. The field campaign was conducted over the South Korean peninsula and surrounding waters from May to June 2016. The South Korean peninsula and its surrounding waters are a desirable region to conduct the campaign because (1) South Korea's urban/rural sectors are distinct, which is advantageous for distinguishing anthropogenic and natural emissions; (2) South Korea is embedded in a rapidly changing region; (3) the region allows studies of local versus transboundary pollution; and (4) air quality monitoring and ground-based measurements are provided by South Korea. AQ measurements (including CO2) from aircraft, ships, and ground sites were obtained during this period. The campaign was designed to answer three scientific questions. (1) What are the challenges and opportunities for satellite observations of air quality? (2) What are the factors governing ozone photochemistry and aerosol evolution? (3) How well do models perform, and what improvements are needed to better represent atmospheric composition over South Korea and its connection to the larger global atmosphere (Kim and Park, 2014, KORUS-AQ White Paper)?
Figure 1 shows the study domain (30–39∘ N, 123–133∘ E) along with the tracks from DC-8 aircraft flights and research ship deployments. The locations of ground sites are also added in Fig. 1. Satellite retrievals from MOPITT CO and Orbiting Carbon Observatory-2 (OCO-2) CO2 are shown in Fig. 1 to provide spatial context and coverage of remote sensing measurements during the campaign. All the observational data used in this study are summarized in Table 2.
2.1.2 Airborne CO and CO2 measurements
We use measurements of CO2 and CO from the DC-8 aircraft. CO2 was measured by Atmospheric Vertical Observations of CO2 in the Earth's Troposphere (AVOCET) using a modified LI-COR model 6252 non-dispersive infrared spectrometer (NDIR). This instrument provides CO2 concentrations with high precision by sensing the difference in light absorption between the continuously flowing sample and reference gases (Vay et al., 2003, 2011; https://airbornescience.nasa.gov/instrument/AVOCET, last access: 10 June 2018). CO2 1 Hz 1σ precision and accuracy are ±0.1 ppm and ±0.25 ppm, respectively. CO was measured by the Differential Absorption CO Measurement (DACOM) instrument via infrared wavelength modulation spectroscopy. The system uses three tunable diode lasers providing 4.7, 4.5, and 3.3 µm radiation for accessing absorption lines of CO, N2O, and CH4. The time response for CO measurements is 1 s; the precision is < 1 % or 0.1 ppbv; the accuracy is 2 % (Warner et al., 2010; https://airbornescience.nasa.gov/instrument/DACOM, last access: 10 June 2018). Calibrations for both instruments were performed during flight at regular intervals using gas standards traceable to the WMO scale (CO2: x2012; CO: x2008) and certified by the National Oceanic and Atmospheric Administration (NOAA) Earth System Research Laboratory (ESRL). Details about the two instruments are listed in Table 2. Note that we use the 1 min (60 s) merged DC-8 data in this study. The data are available at the NASA Langley Research Center archive (https://www-air.larc.nasa.gov/missions/korus-aq/, last access: 10 June 2018).
There were 20 formal DC-8 science flights. Note that for time reference, the “date” in this paper refers to the day on which the flight started in UTC time instead of South Korean local time, unless the term “local time” is explicitly used. This “date” in UTC time is 1 day behind South Korean local time as all flights typically start at 08:00 LT. We also divide the flight measurements into five groups based on the land cover below the flight tracks and types of pollution sources with which they can be broadly associated. These groups are classified as the Seoul metropolitan area, Taehwa, the West (Yellow) Sea, Seoul–Jeju jetway, and Seoul–Busan jetway (please refer to Fig. 1 for an illustration of these flight groups). The Seoul metropolitan area represents air samples over the large city of Seoul which can have a dominant signature from anthropogenic combustion processes. On the other hand, Taehwa represents air samples over a forest area near Seoul, which can be influenced by both surface carbon fluxes from the local forest as well as anthropogenic emissions from Seoul. Measurements over the West Sea were designed to capture China pollution outflows. The flight tracks over the West Sea were typically zonal tracks forming a “wall” between China and South Korea (see Fig. 1). These flights are conducted only when a China outflow is expected to be present based on weather and AQ forecasts during the campaign. These measurements enable us to investigate combustion signatures from China and differentiate them from Seoul. The Seoul–Jeju jetway and Seoul–Busan jetway groups are two jetway flights on which the DC-8 aircraft frequently obtain measurements. The two jetways are both above the Korean Peninsula, connecting Seoul to Jeju and Busan, respectively. Flights in the Seoul–Busan jetway are designed to capture activities in forest, rural, and Busan urban regions. The flights in the Seoul–Jeju jetway, on the other hand, sample air over local power plants, transported air from the West Sea, and over nearby croplands. We will discuss our CAMS evaluation for each of these five groups in Sect. 3.
2.1.3 Ground-based CO and CO2 measurements
Observations from the following ground sites are used for comparison with CAMS CO and CO2: Baengnyeong, Fukue, Olympic Park, Taehwa, and Yonsei University (see Fig. 1 for the site locations). The sites in Baengnyeong and Taehwa are managed by the National Institute of Environmental Research (NIER). The Baengnyeong site is located on the sparsely populated Baengnyeong Island, Incheon, northwest of Seoul. The Fukue site belongs to the Japan Agency for Marine-Earth Science and Technology (JAMSTEC) and is located on the remote island of Fukue, Japan (Kanaya et al., 2016). The Olympic Park and Yonsei University sites belong to Korea Research Institute of Standards and Science and Yonsei University, respectively. Both sites are located within the Seoul metropolitan area. These five ground sites cover different environments, which allows us to differentiate between urban (Olympic Park and Yonsei University) and remote (Baengnyeong and Fukue) air quality conditions during the campaign. The sites in Baengnyeong, Fukue, and Olympic Park provide measurements of CO (in ppbv), while the site at Yonsei University provides measurements of CO2 (in ppmv). Only the site in Taehwa provides measurements of both CO (in ppbv) and CO2 (in mg m−3) (Kim et al., 2013). Locations of the five sites, and corresponding instruments and data intervals are provided in Table 2. Note that we use data from these sites taken during the KORUS-AQ campaign period to provide the ground context of our evaluation.
2.1.4 Ship observations
We use ship measurements of CO from R/Vs Jangmok and Onnuri. Both of them are research vessels owned by Korea Institute of Ocean Science and Technology. The ship deployments are part of the Korea-United States Ocean Color (KORUS-OC) field study coinciding with KORUS-AQ. KORUS-OC was led by NASA and the Korean Institute of Ocean Science and Technology, focusing on the ocean color, biology, and biogeochemistry as well as atmospheric composition in coastal waters adjacent to South Korea (https://www.asp.ucar.edu/sites/default/files/4_Emmons_07_27_2016.pdf, last access: 10 June 2018). The two ships sailed along the South Korean coast from 20 May to 5 June. Tracks of the two ships are shown in Fig. 1 in dark grey (Jangmok) and light grey (Onnuri). CO measurements on R/Vs Jangmok and Onnuri were taken from the Thermo 48i-TLE CO analyzer and Thermo 48C CO analyzer, respectively (http://www.kiost.ac.kr/kor.do, last access: 10 June 2018), and are provided every minute.
2.1.5 Satellite-derived CO and CO2 retrievals
We use four sets of satellite-derived measurements for comparison with CAMS CO and CO2. We use retrievals of CO2 column-averaged dry-air mole fraction (XCO2) from NASA OCO-2, version 7, level 2 (L2) full product with the standard quality flag and warn level ≤15 (Crisp et al., 2004; Boesch et al., 2011; Wunch et al., 2011a, b, 2017; Osterman et al., 2016; Mandrake et al., 2015; https://oco.jpl.nasa.gov/, last access: 10 June 2018), and from the Japan Aerospace Exploration Agency (JAXA) GOSAT, level 2 (L2), version 2 (Yokota et al., 2004, 2009; Morino et al., 2011; Crisp et al., 2012; http://global.jaxa.jp/projects/sat/gosat/, last access: 10 June 2018). Short-wavelength infrared observations measured by the Thermal And Near-infrared Sensor for carbon Observation (TANSO) aboard the GOSAT satellite are used to retrieve XCO2. OCO-2 also has three specific near-infrared (NIR) wavelength bands to retrieve XCO2 (https://oco.jpl.nasa.gov/). For CO, we use the NASA Terra MOPITT version 6, level 2, multispectral (thermal infrared/near infrared; TIR/NIR) total column retrievals (MOP02J, L2, V6) with the standard quality flag. Compared to thermal-infrared-only retrievals (TIR), these retrievals have an enhanced sensitivity to the lower tropospheric CO (Deeter et al., 2014; https://www2.acom.ucar.edu/mopitt, last access: 10 June 2018). In addition, we also use total column mole fractions of CO from IASI level 2 data with the standard quality flag (George et al., 2009; Clerbaux et al., 2009). IASI is aboard MetOp-A and B satellites and uses Fast Optimal Retrievals on Layers for IASI (FORLI) to retrieve CO distributions from the TIR spectra. We applied the associated averaging kernels from MOPITT and IASI to CAMS CO before comparison as these retrievals exhibit large sensitivities in the free troposphere. We also note that both IASI and MOPITT have significantly more observations than OCO-2 and GOSAT. As summarized in Table 2, the resolutions of OCO-2, GOSAT, MOPITT, and IASI are 2.25×1.29, 10.5×10.5, 22×22, and 12×12 km, respectively. The overpass times for the four satellites are also different. OCO-2 overpasses at 13:18–13:33 LT, GOSAT overpasses at around 13:00 LT. Overpass time is 10:30 LT for MOPITT, and 09:30 LT for IASI. Uncertainties have also been reported for these satellite products. OCO-2 XCO2 has uncertainties of 1–2 ppm (Boesch et al., 2011) while GOSAT XCO2 has retrieval errors of 2 ppm (Morino et al., 2011; Crisp et al., 2012). Deeter et al. (2014) reported 0.09×1018 molecules cm−2 for total column retrieval for MOPITT. De Wachter et al. (2012) reported uncertainties to be < 13 % for IASI FORLI.
Here, we evaluate CAMS forecasts and analysis of CO and CO2 with NASA DC-8 aircraft observations. We interpolate the 4-D fields of CAMS CO and CO2 model output to collocate with flight measurements in both space and time. The equivalent model data for all flights and for the three configurations (FC16s, FC9s, ANs) are made available in the same file format as the 1 min merged DC-8 dataset to facilitate model-to-observation comparison. We also estimate enhancement ratios of CO and CO2 from both airborne and model data and analyze their spatial and temporal variations across different flights. We present in the following subsections the summary statistics of our comparison of CAMS data with the DC-8 aircraft data.
3.1 Performance across all flights
Across all flight data, CAMS overestimates CO2, with mean biases of 2.2, 0.7, and 0.3 ppmv for FC16s, FC9s, and ANs, respectively. Agustí-Panareda et al. (2016) also suggested CO2 is overestimated by CAMS in the NH at the end of winter and throughout spring. In contrast, CAMS underestimates CO with mean biases for FC16s, FC9s, and ANs against the DC-8 aircraft data of −19.2, −16.7, and −20.7 ppbv, respectively. The mean bias is calculated as the average across all data of CAMS minus the DC-8 aircraft data. We also find that the overall pairwise correlation between the DC-8 aircraft data and CAMS is moderately high (CO2: 0.52–0.57, CO: 0.65–0.73) while the root mean square errors (RMSEs) in CAMS relative to the DC-8 aircraft data are about 7 ppmv for CO2 and 80 ppbv for CO. These statistics can be summarized using a Taylor diagram as shown in Figs. S3 and S4 of the Supplement. We also calculated the associated Taylor scores to summarize the skill of CAMS in capturing the observed CO2 or CO variations. The Taylor score (Taylor, 2001) is defined by
where is the ratio of σf (standard deviation of the model) and σr (standard deviation of observations), R is the correlation between model and observations, and R0 is the maximum potentially realizable correlation (equivalent to 0.9 in this study).
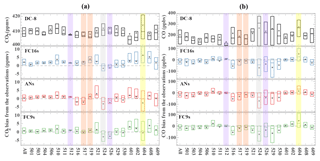
Figure 2Box plot for each individual flight. The flight date (MDD) for each box plot is indicated in the bottom x axis. Note that the dates here are in UTC time instead of South Korean time. Panel (a) is for CO2 and panel (b) is for CO. The first row corresponds to the box plot of the abundances measured by DC-8 aircraft. The second, third, and fourth rows correspond to the box plot of the bias of FC16s, ANs, and FC9s relative to the DC-8 aircraft data, respectively. The purple shade marks the flights with frontal passage, and orange shade marks the flights that may possibly be affected by biomass burning. The grey shade marks the flight measuring China outflow while yellow shade marks the flight surveying point emission sources.
We find that CAMS has relatively good skill regardless of configuration: for CO2, the skill scores are 0.82 (FC16s), 0.82 (FC9s), and 0.75 (ANs), while for CO, the skill scores are 0.85 (FC16s), 0.86 (FC9s), and 0.83 (ANs). However, it is important to note that these statistics can vary from flight to flight and the skill for CO2 is not necessarily related to that of CO. For instance, for the 10 May flight, where a southern peninsula outflow was expected, CAMS ANs show higher skill than those from FC9s in terms of both CO2 and CO, while the scores of FC16s are higher than those of FC9s in terms of CO (Fig. S5). Yet, for the 3 May flight, where a weak Chinese influence was expected, the scores of FC16s and FC9s are higher for CO2 than CO, while we find the opposite for the 2 June flight, where the DC-8 aircraft sampled local influences. Lastly, we note that the skill of CAMS during the 4 June flight is not high for either species. This flight was designed to measure local point sources with large variations at much finer scales.
3.2 Performance across individual flights
We present in Fig. 2 the summary statistics of CAMS against the DC-8 aircraft data for all 20 individual flights. This is shown in the second to fourth rows of Fig. 2 as box plots of the bias for FC16s, ANs, and FC9s, respectively. We also show the box plot of the airborne measurements of CO2 (first row, left column) and CO (first row, right column) for each flight as points of comparison. The overall mean, median, interquartile range (IQR), and standard deviation (σ) of the airborne measurements of CO2 mixing ratios (in ppmv) are 410.37, 408.25, 5.97, and 7.73, respectively. The overall mixing ratio, which varies within 1 to 2 %, is slightly higher than the monthly median observed in Mauna Loa (NOAA, https://www.esrl.noaa.gov/gmd/ccgg, last access: 10 June 2018) for May 2016 (408±1 ppmv). For the airborne measurements of CO mixing ratios (in ppbv), the corresponding statistics (mean: 204.59, median: 183.90, IQR: 127.97, σ: 101.74) show enhanced CO (and larger variance) than the background value observed in Mauna Loa (100±24 ppbv). In general, CAMS overestimates CO2 and underestimates CO for most flights. Differences also exist among the 20 flights in terms of both measured mixing ratios and model biases from the DC-8 aircraft. For flights with higher observed variances, CAMS biases and the corresponding variance of the biases tend to be also larger. This is related to variations in weather conditions during the campaign along with variations in sampling goals of the science flights. For example, parts of flight tracks on 3, 17, 24, 29, and 30 May were specifically designed to capture Chinese pollution outflow. In these days, the variances in CAMS biases for CO (but not CO2) are generally larger than the average, except for the flight tracks on 3 May when Chinese influences were expected to be weak. The colored shades in Fig. 2 indicate flights for “special conditions”. The grey and yellow shades indicate two special cases that we study in detail in later sections. In particular, DC-8 flew a “wall” over the West Sea on 24 May to investigate the transport of Chinese pollution. On 4 June, DC-8 flew near Seoul to measure pollution from local point sources (e.g., power plants). The other shades indicate that the flights were conducted during a frontal passage (purple) and that the flights may possibly be affected by fires in Siberia (orange). These flights were not further analyzed in this study since, for example, the 26 May flight (with frontal passage influence), and the 17 and 19 May flights (with possible fire influence) do not clearly stand out from the other flights (see Fig. 2).
3.3 Performance across flight groups
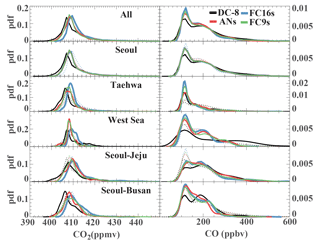
Figure 3Probability density functions (pdfs) of CO2 and CO for each flight group. Solid lines are pdfs for each group while the dashed lines are pdfs for all groups.
Here, we evaluate CAMS per flight group as described in Sect. 2.2.1. We show in Fig. 3 the probability density functions (pdfs) of CO and CO2 for the DC-8 aircraft data and CAMS per flight group. The pdfs of CAMS CO2 (which exhibits a longer tail to higher values) show a general offset to higher values relative to the DC-8 aircraft data (except for the West Sea). There is a systematic overestimation of CAMS CO2 against the DC-8 aircraft data. Accordingly, the “apparent local background” of CO2 (lower tails of the pdfs) is relatively higher in CAMS than the DC-8 aircraft data. In contrast, CO is underestimated in CAMS across all of the five groups. The pdfs of CO in CAMS show a bimodal distribution (except in Taehwa and the West Sea) indicative of two dominant AQ conditions sampled by DC-8 over this region. The shapes of the CO pdfs of CAMS largely differ from those of the DC-8 aircraft data (except in Taehwa). We see a higher frequency of occurrence of the two to three modes in the West Sea in CAMS that is not apparent in the DC-8 aircraft data, while the opposite is the case in Seoul–Busan. This suggests that the underestimation of CO in CAMS may not be systematic or may be caused by biases in CO background values. The pdf over the West Sea also shows that CAMS underestimates (or even misses) the more elevated CO observed by the DC-8 aircraft.
We further investigate the differences between CAMS and the DC-8 aircraft data by looking at the bias in the mean profiles. We show in Fig. 4 the mean profiles for all data and each individual group. We find that the overall bias in CAMS CO2 is systematic and close to uniform across all layers (FC16s: ∼2.2 ppmv, FC9s: ∼1 ppmv, and ANs: ∼0.8 ppmv). This overestimation is true for all flight groups except over the West Sea. On the other hand, for CO, the overall bias in CAMS is mostly evident in the lower troposphere (about −20 to 25 ppbv below 700 hPa). This underestimation is especially the case over the West Sea and is consistent with the pdfs in Fig. 3.
3.3.1 The Seoul metropolitan area and Taehwa
The airborne measurements over the Seoul metropolitan area were mostly during frequent aborted landing maneuvers (i.e., missed approaches) over the Seoul Air Base. More than 90 % of the measurements in this group were taken below 850 hPa. Figure 3 shows that the performance of FC16s, FC9s, and ANs is alike over Seoul for both CO and CO2, in contrast to the other four flight groups. Given that the measurements over Seoul are dominated by boundary layer (BL) and anthropogenic emissions in Seoul, the model performance over Seoul is most likely to be driven by local emissions. We show in Fig. 5 the mean vertical profiles over Seoul below 800 hPa. For CO2, FC9s profiles agree well with the observations. This is not the case for CO, where FC16s, FC9s, and ANs do not agree well with the DC-8 aircraft data, but with the bias in ANs being relatively smaller. However, the near-surface temporal variations (changes in the profile from morning to afternoon) observed by the DC-8 aircraft are captured by FC16s, FC9s, and ANs. It is worth noting that, over Seoul, there is an abrupt change in the profile at around 925 hPa for both CO and CO2 of the morning samples. Accordingly, CO is overestimated below 925 hPa and underestimated above 925 hPa. These vertical gradients below 925 hPa (i.e., change in mixing ratios divided by change in pressure) in the averaged profiles of the DC-8 aircraft data CO2 and CO are about 0.25 ppmv hPa−1 and 1.7 ppbv hPa−1, respectively. In contrast, the gradients of CO2 in CAMS are 0.50 ppmv hPa−1 for FC16s, 0.34 ppmv hPa−1 for FC9s, and 0.45 ppmv hPa−1 for ANs while the gradients of CO in CAMS are 4.2 ppbv hPa−1 for FC16s, 3.4 ppbv hPa−1 for FC9s, and 3.3 ppbv hPa−1 for ANs. It is evident that these gradients (CO and CO2) regardless of CAMS configuration are significantly steeper than observed. While in part this may be attributed to overestimation of emissions during rush hours (and nighttime) in Seoul along with model representativeness errors in the BL, we attribute this steep gradient to a possible weaker BL mixing in CAMS since there is an important contrast between near-surface CO (overestimation) and CO aloft (underestimation) which cannot be explained by emissions alone. This is not very apparent in CO2 since there is an overestimation of background CO2 superimposed on this difference. In addition, given the air traffic over the Seoul Air Base (where the DC-8 aircraft frequently conducted missed approaches), emissions from airplanes may also contribute to the model biases (Boschetti et al., 2015).
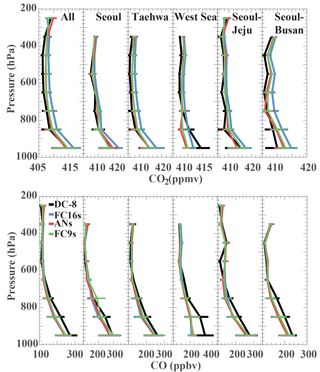
Figure 4Averaged vertical profiles of CO2 and CO mixing ratios from the DC-8 aircraft data and CAMS for each flight group. Horizontal bars correspond to the interquartile ranges (between 25th and 75th percentiles) of the layer bin.
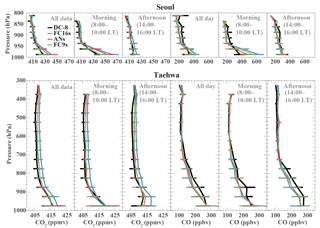
Figure 5Temporal variation of averaged vertical profiles of CO2 and CO mixing ratios from the DC-8 aircraft data and CAMS over the Seoul and Taehwa flight groups. The first, second, and third columns are averaged CO2 profiles for all day, morning (08:00–10:00 LT), and afternoon (14:00–16:00 LT), respectively. Horizontal bars correspond to interquartile ranges (between 25th and 75th percentiles) of the profiles. The fourth, fifth, and sixth columns are the same as the first three columns but for CO.
In Taehwa, the differences between morning and afternoon samples are not as large compared to the Seoul metropolitan area. The CO2 profiles from ANs and FC9s are apparently closer to the DC-8 aircraft data than those from FC16s. However, this difference is not obvious for the CO profiles. Note that in the afternoon (14:00–16:00 LT), measured CO2 mixing ratio near the surface (at 975 hPa) becomes lower than the layer above, indicating a possible drawdown of CO2 by underlying vegetation in Taehwa. This change is captured by CAMS, especially in FC9s. We further find that compared with the Seoul metropolitan area, the observed vertical gradient of CO2 over Taehwa (∼0.03 ppmv hPa−1) below 925 hPa is smaller, which is relatively better captured by CAMS (0.02–0.12 ppmv hPa−1). This again implies the possible inefficient BL mixing in CAMS over the Seoul urban environment. CO over Taehwa is more likely to be due to regional transport, as Taehwa is not a strong CO source region. Thus, the vertical gradient of CO over Taehwa does not necessarily reflect the impact of BL mixing over Taehwa. We further compared the mixing layer (ML) height derived from the KORUS-AQ Airborne Differential Absorption Lidar – High Spectral Resolution Lidar (DIAL-HSRL) measurements of aerosol backscatter following the technique from Brooks (2003), and the BL heights from CAMS. We note that ML height is only approximately equal to BL height. We find that CAMS generally underestimates BL heights during KORUS-AQ (Fig. S6). The model underestimation of BL over the Seoul metropolitan area ( m) is stronger than that over Taehwa ( m) which is covered by forests instead of the urban environment. This is consistent with CAMS' relatively better capability of capturing vertical gradient of CO2 over Taehwa compared to that over Seoul, supporting our previous implication of the possible inefficient BL mixing in CAMS over the Seoul urban environment.
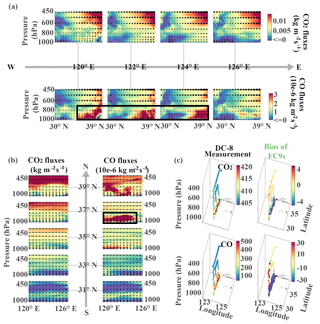
Figure 6Case study for the flight on 24 May (UTC time). (a) Vertical distributions (hereafter denoted as “sections”) of fluxes (kg m−2 s−1) at 09:00 UTC on 25 May (South Korean time) in meridional direction. Dots represent meridional winds going from west to east (i.e., from China to South Korea) and crosses represent meridional winds with the opposite direction. Sizes of the dots and crosses are proportional to the wind speed. “Sections” at the top are for CO2 fluxes and those at the bottom are for CO fluxes. (b) “Sections” of fluxes (kg m−2 s−1) at 09:00 UTC on 25 May (South Korean time) are in the zonal direction. Arrows represent meridional winds. “Sections” in panel (b) share the same color bar as panel (a). (c) The DC-8 aircraft measurements (left column) and bias of CAMS along the flight track over the West Sea (right column). The top row is for CO2 and the bottom row is for CO.
3.3.2 West (Yellow) Sea
As previously mentioned, the flights over the West (Yellow) Sea are focused on capturing pollution outflow from China. Both CO and CO2 in this flight group are underestimated by CAMS below 900 hPa (Fig. 4). It is the only group in which near-surface CO2 is underestimated by all the three CAMS configurations. In addition, the underestimation of CAMS CO over the West Sea is more significant than that over the other groups. We list two possible reasons for this unique model performance over the West Sea considering that the Chinese outflows constitute the dominant influence of CO and CO2 samples in this group. First, the transport of surface pollution from China to the West Sea is not well represented in CAMS. Second, emissions in China may not be as well quantified as in South Korea. During the 24 May flight, a strong outflow from China was expected, so the DC-8 aircraft flew an extended sampling “wall” over the West Sea to sample transport from China. We show in Fig. 6 some of the details of this flight. In particular, we show the vertical cross sections of meridional (Fig. 6a) and zonal (Fig. 6b) fluxes of CO and CO2 in CAMS FC9s. These fluxes are calculated as the product of meridional (from west to east) or zonal (from south to north) wind speed with simulated species density (i.e., in terms of units, ). The China outflow moving towards the West Sea and Seoul is well demonstrated in the fluxes of CO in Fig. 6a and b especially in the region marked by the black rectangles. This outflow is not apparent in the fluxes of CO2. This is because the variations in CO2 density are very low relative to CO2 background in contrast to CO variations. We also show in Fig. 6c the measurements from the DC-8 aircraft and the bias of FC9s over the West Sea on that day. As can be seen in Fig. 6, CAMS CO2 and CO are largely underestimated (CO2: 2–4 ppmv, CO: 86–88 ppbv) for this flight. This underestimation in both species is consistent with Fig. 4. Note that the underestimation of CO2 over the West Sea is not consistent with other flights and the overall results. This underestimation could be associated with an underestimation of anthropogenic emissions in China and/or transport from China to the West Sea. This is discussed in Sect. 3.4 in more detail. In summary, the transport pattern of China outflow (CO and CO2) to the West Sea is captured but the abundances of both CO and CO2 are underestimated by CAMS especially near the surface.
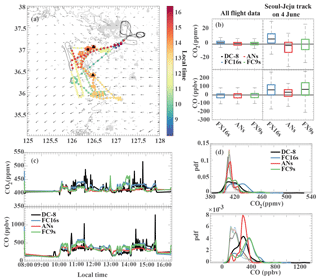
Figure 7Case study for the flight on 4 June (UTC time). (a) Flight track of DC-8 aircraft in the Seoul–Jeju jetway group for this day. The Daesan chemical facility is marked as a black pentagram and two power plants are marked as black triangles. Arrows correspond to 950 hPa wind field at 12:00 LT. (b) Box plot of CAMS bias from all the DC-8 aircraft measurements during the campaign (left) and from measurements on 4 June in the Seoul–Jeju jetway group (right). Top row is for CO2 and bottom row is for CO. (c) Time series of the DC-8 aircraft measurements and CAMS during the flight. (d) The pdfs of CO and CO2 for measurements on 4 June of the Seoul–Jeju jetway group (solid) and for all groups (dashed).
3.3.3 Seoul–Jeju and Seoul–Busan jetways
Measurements in the Seoul–Jeju and Seoul–Busan jetways are both above the South Korean peninsula, connecting Seoul to Jeju and Busan, respectively. While both flight groups share some common features, they are treated here as two distinct groups for the following reasons: (1) the Seoul–Jeju jetway is close to the west coast of South Korea, whereas the Seoul–Busan jetway sampled air southeast of Seoul and more inland; (2) there are more croplands, urban, and built-up areas along Seoul–Jeju jetway while there are more forested areas along Seoul–Busan jetway; (3) there are some important point sources along Seoul–Jeju jetway such as power plants and the Daesan chemical facility. In fact, the 4 June flight was designed to survey point sources west of Seoul and focused more to the Seoul–Jeju jetway. Details of the 4 June flight are summarized in Fig. 7. In contrast to the overall statistics across all flight groups, FC16s, FC9s, and ANs for this flight clearly overestimate CO near point sources. We also note that measurements for this flight are mostly taken below 900 hPa. As such, the spatial variations are larger near point sources than in other conditions. Nevertheless, these variations are not well captured by CAMS, especially by ANs. This may be due to its coarser grid representation (i.e., 40 km for CO2 and 80 km for CO). In addition, we find a difference in terms of mean bias in CO2 between CAMS FC9s and FC16s. This difference is not apparent in CO. This implies there might be large spatiotemporal errors existing in CO emission inventories in the region, since higher emission resolution does not result in an improvement. In this case, increasing the spatiotemporal resolution might even weaken the simulation results, whereas lower resolution usually agrees better with observations as it “diffuses” the error of the emissions.
3.4 Enhancement ratios of CO to CO2
We also evaluate the three CAMS configurations against the DC-8 aircraft data in terms of enhancement ratios of CO to CO2 (dCO∕dCO2) for all flights and for each flight group. We conduct a reduced major axis (RMA) regression to estimate the sensitivity of CO to CO2 (i.e., dCO∕dCO2) with the 1 min merges. We use RMA instead of ordinary least squares (OLS) regression as the two variables (CO and CO2) are both subject to error (Smith, 2009). The estimated regression slope in the RMA corresponds to the enhancement ratio of CO and CO2. This ratio can reflect the emission ratios of a particular area especially when using near-field data (Parrish et al., 2002). Despite its limitations (Yokelson et al., 2013), such analysis has been used in previous studies for surface CO and NOx (Parrish et al., 2002), emission factors for biomass burning (Wofsy et al., 1992; Lefer et al., 1994; van Leeuwen and van der Werf, 2011), flask samples of CO and CO2 in east Asia (Turnbull et al., 2011), airborne measurements of CO and CO2 during TRACE-P (Suntharalingam et al., 2004), surface CO and CO2 in rural Beijing (Wang et al., 2010), and more recently with satellite retrievals of CO (MOPITT) and CO2 (GOSAT) (Silva et al., 2013). We present our estimates of dCO∕dCO2 (with units of ppbv ppmv−1) from the DC-8 aircraft data and CAMS FC16s, FC9s, and ANs in Table 3. Overall, the observed dCO∕dCO2 during the KORUS-AQ campaign is ∼13 ppbv ppmv−1 (or ∼1.3 %). This is a relatively low value compared to reported ratios in more polluted megacities such as Beijing. The lowest dCO∕dCO2 among the five flight groups is observed over Seoul (∼9 ppbv ppmv−1). The observed dCO∕dCO2 for other groups within South Korea ranges from ∼10 ppbv ppmv−1 (Seoul–Jeju) to ∼16 ppbv ppmv−1 (Seoul–Busan and Taehwa). Taehwa is close to and sometimes downwind of Seoul but has higher observed dCO∕dCO2 than Seoul. We attribute this difference to biogenic CO sources and biospheric influence on CO2 over Taehwa. The highest dCO∕dCO2 (∼28 ppbv ppmv−1) is observed over the West Sea. This ratio is a sharp contrast to Seoul and other flight groups over South Korea. This indicates that the bulk combustion efficiency over Seoul is higher in Seoul than in the China pollution outflows over the West Sea. The ratio over the West Sea is very consistent with dCO∕dCO2 observed over China (upwind of the West Sea) during KORUS-AQ by ARIAs (20–100 ppbv ppmv−1. Such “combustion signature contrast” is consistent with previous studies in the region. During TRACE-P in 2001, the observed ratio over Japan was ∼12–17 and ∼50–100 ppbv ppmv−1 over northern China (Suntharalingam et al., 2004). Over Shangdianzi, China, and the Tae-Ahn Peninsula (TAP), South Korea, Turnbull et al. (2011) reported CO : CO2ff ratios (which are derived from measurements of CO and Δ14CO2 in flask samples taken during winter 2009/2010), of ∼47 and ∼44 ppbv ppmv−1, respectively. They also reported that the South Korean samples from TAP have CO : CO2ff of ∼13 ppbv ppmv−1. Wang et al. (2010) reported a change in observed dCO∕dCO2 near Beijing from 34–42 ppbv ppmv−1 in 2005–2007 to 22 ppbv ppmv−1 in 2008. Finally, dCO∕dCO2 values derived from satellite retrievals in 2010 indicate a similar contrast between Beijing/Tianjin (∼25–50 ppbv ppmv−1) and Seoul (∼7–9 ppbv ppmv−1). Despite the differences in the data sources (satellites, airborne measurements, flask samples) and time period, these dCO∕dCO2 values are consistent and all point to a “combustion signature contrast” between South Korea and China. We expect that this contrast may be decreasing over time as Chinese combustion activities become more efficient.
These observed ratios are remarkably consistent with dCO∕dCO2 from CAMS (see Table 3). The three CAMS configurations have dCO∕dCO2 over the Seoul metropolitan area of ∼8 to 12 ppbv ppmv−1 and over the West Sea of ∼31–32 ppbv ppmv−1. Our rough estimates of CO to CO2 emission ratios in CAMS over Seoul and China during KORUS-AQ also show marked similarity with CAMS enhancement ratios. The CO to CO2 emission ratio over China is about 28 (ppbv ppmv−1) and about 10 (ppbv ppmv−1) over South Korea. Our results suggest that CAMS emission ratios reflect this contrast and that the modeled dCO∕dCO2 is indicative of emissions of Seoul and China. To further understand the skill of CAMS in capturing this contrast, we compare the observed correlation between CO and CO2 and the correlation from CAMS FC16s, FC9s, and ANs. This corr(CO2,CO) is presented in the second row of Table 3. Over Seoul, the observed corr(CO2,CO) is moderately high (∼0.8), which is likely driven by common CO and CO2 sources (mostly local anthropogenic emissions from Seoul). This correlation is well captured by ANs and FC9s but not FC16s. We attribute this difference to a better initialization in ANs and FC9s due to assimilation. The observed corr(CO2,CO) over the West Sea is even higher (0.89), indicating that CO and CO2 come from common sources in China. However, this corr(CO2,CO) is not captured by any of the three configurations (0.25–0.42). A few factors may contribute to this low corr(CO2,CO) over the West Sea. First, the flight on 12 May is a noteworthy source of low corr(CO2,CO) in CAMS. We have shown in Fig. 2 that the major goal of this flight is to study AQ conditions during a frontal passage instead of sampling China outflows. Even though part of the track during 12 May is located in the West Sea, the AQ features of that day are evidently different from China outflow events. After excluding measurements during 12 May, the corr(CO2,CO) values in CAMS (FC16s: 0.51, FC9s: 0.43, and ANs: 0.29) are now higher albeit still lower than observed (0.9). Uncertainties in model transport can be a likely cause as the corr(CO2,CO) can be subject to transport errors even though dCO∕dCO2 may not necessarily be affected. Performance of CAMS over the Baengnyeong site (discussed in Sect. 4.1) also implies possible issues with transport of China pollution towards the West Sea. Furthermore, the difference in temporal representation of China emissions in CAMS may contribute to this mismatch in timing and hence result in low correlation. As mentioned in Sect. 2, CAMS uses prescribed monthly emission for CO while the diurnal cycle of CO2 fluxes is calculated online in CAMS. In fact, there is a strong diurnal cycle in the spatial correlations between CO emissions and CO2 fluxes in CAMS caused by diurnal cycles of the CO2 NEE (Fig. S8). The diurnal cycle of spatial correlations between CO emissions and CO2 fluxes over South Korea in CAMS peaks (∼0.7) in daytime when measurements over South Korea were made. On the other hand, during the nighttime, the correlations between CO emissions and CO2 fluxes in CAMS are relatively low over east China (< 0.4). This implies that the relatively low correlations between the CO and CO2 abundances over the West Sea in CAMS may reflect the effect of nighttime emissions from east China in CAMS. Lastly, the corr(CO2,CO) values in FC16s and FC9s are closer to observed corr(CO2,CO) than in ANs suggesting that resolution may also play a role. For the other three flight groups, the observed corr(CO2,CO) values are not as high as those over Seoul and the West Sea. This implies that CO2 and CO observed over these three flight groups may not come from common sources and/or have been mixed with the environment. CAMS corr(CO2,CO) values do not always agree with observed corr(CO2,CO). Overall, corr(CO2,CO) from FC16s is higher than observed while corr(CO2,CO) values from FC9s and ANs agree well with observed corr(CO2,CO). Again, this may be related to the fact that FC16s is generated from a free-running simulation (i.e., not initialized with analyses).
Finally, we present the correlation between the biases of CAMS for the two species () (please see the third row of Table 3). This correlation provides another piece of information on whether the performance of CAMS in CO2 and CO is related. We find that values are high over Seoul and the West Sea, indicating that the performance of CAMS in CO and CO2 is related for the two groups. Over the West Sea, FC16s, FC9s, and ANs perform similarly. However, the values are lower in the other three groups relative to Seoul and the West Sea. In addition, our results show that ANs and FC9s usually have lower than FC16s, especially over Seoul. This implies that FC16s performance in CO2 and CO is more strongly related than FC9s and ANs performance, which could be associated again with the fact that FC16s come from a free-running simulation while FC9s and ANs are both initialized from analyses. The assimilation of CO and CO2 satellite retrievals may reduce the interdependence of CAMS CO2 and CO performance.
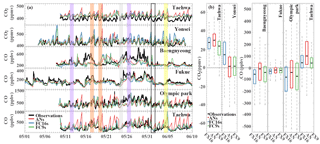
Figure 8Comparisons of CAMS against ground site measurements. Values of CAMS are averages across layers with pressure higher than 95 % of the surface pressure. (a) Time series of measured and CAMS CO2 from the Taehwa and Yonsei sites, and CO from the Baengnyeong, Fukue, Olympic Park, and Taehwa sites. Shades denote same events as they do in Fig. 2. (b) Box plot of CAMS bias for CO2 at the Taehwa and Yonsei site measurements, and for CO at the Baengnyeong, Fukue, Olympic Park, and Taehwa sites. Dates are in mm/dd format.
In this section, we evaluate CAMS FC16s and FC9s, and ANs against CO and/or CO2 measurements from five ground sites, two ships, and four satellites. Unlike the data from the DC-8 aircraft, data on CO2 or CO in these cases may not be jointly available. In particular, each ground site (except Taehwa) only measures one of the two species. The ships also provide measurements for CO only while the four sets of satellite retrievals of CO2 and CO are from four different instruments aboard four different satellites. Therefore, in this section, CO2 and CO are evaluated separately, and relationships between CO2 and CO inferred from some of these sites are only indicative of a larger pattern that we see in the DC-8 aircraft data.
4.1 Comparison with ground observations
Here, we focus our evaluation on CAMS performance in capturing surface conditions and diurnal cycle of CO2 and/or CO. Data from the following five ground sites are used in this study: Baengnyeong, Fukue, Olympic Park, Taehwa, and Yonsei University (Fig. 1 and Table 2). It can be seen in Fig. 8 that CO from Olympic Park and CO2 from Yonsei and Taehwa clearly show a diurnal cycle during KORUS-AQ. This feature is well captured by CAMS. CO at Taehwa, on the other hand, exhibits a very weak diurnal cycle that is not captured by CAMS. At this site, CO in CAMS (especially ANs) shows a strong diurnal cycle. Variations of CO in the remote sites of Baengnyeong and Fukue also appear to be irregular and episodic. Signatures of elevated CO can also be seen at these sites, some of which coincide with pollution transport from China sampled by the DC-8 aircraft. The mean diurnal cycle for these five ground sites can be found in Fig. S9.
While CAMS is able to get the observed timing of CO2, the modeled magnitudes of CO2 (and CO) at these sites from CAMS are too high (especially for the sites in and near Seoul). We took the average value across a few layers near the model surface in CAMS to provide a reasonable comparison at these sites. We use model vertical layers below 95 % of the model surface pressure (i.e., if surface pressure is 1000 hPa, we average the layers below 950 hPa) to account for potential weak BL mixing (especially near source regions). This feature in CAMS has been discussed in Sect. 3.3.1. Since this averaging may introduce errors in our comparison, we only evaluate CAMS in terms of relative patterns (diurnal cycle and spatial variability across sites). Note that CAMS CO along the ship tracks (to be discussed in the successive section) is also averaged across a few layers in the same way for consistency. We show in Fig. 8 the summary statistics of the bias in CAMS relative to ground observations. The box plots show that the variability of model bias in CO is in general smaller for remote sites and larger for the two sites in the Seoul metropolitan area. The bias in CAMS is also smaller in Fukue than in Baengnyeong, where a larger influence of pollution transport from China is observed but not well captured in CAMS. It is also worth mentioning that relative to other sites, CAMS significantly overestimates both CO and CO2 at Taehwa. This may be due to the proximity of Taehwa to Seoul. The model grid spacing may not be able to resolve well the subgrid-scale processes (emissions) and variations between Seoul and Taehwa. This overestimation is most apparent in CAMS ANs which has a coarser grid spacing (40 km for CO2 and 80 km for CO) than FC16s and FC9s. In the case of CO2 at Yonsei, we find lower bias in CAMS FC9s and ANs than FC16s suggesting improvements of CAMS due to better initialization.

Figure 9Comparisons of CAMS CO against ship measurements. Values of CAMS are averages across layers with pressure higher than 95 % of the surface pressure. (a) Bias of CAMS CO against ship measurements along the ship track. (b) Box plot of CAMS bias for CO compared with ship measurements.
We take advantage of the location of the sites in Olympic Park (CO) and Yonsei University (CO2) which are within the Seoul metropolitan area and the collocated measurements of CO and CO2 in Taehwa to investigate patterns of ground-based dCO∕dCO2 in Seoul and Taehwa. Here, we only discuss observed dCO∕dCO2 since the modeled dCO∕dCO2 at these ground sites may not be accurate given CAMS issues with vertical mixing near the surface and representativeness errors. Following similar analysis with the dCO∕dCO2 of the DC-8 aircraft data, regressions of CO to CO2 at these sites can represent emission ratios of CO to CO2 in the Seoul metropolitan area. Our estimate of dCO∕dCO2 from the Olympic Park and Yonsei sites is 11.32 ppbv ppmv−1. This is consistent with dCO∕dCO2 calculated from the DC-8 aircraft data which sampled air closely above these sites (∼9 ppbv ppmv−1). Our estimate of dCO∕dCO2 from the Taehwa site is 6.57 ppbv ppmv−1. This is different from our estimate of 15.3 ppbv ppmv−1 based on the DC-8 aircraft data. Unlike Seoul, 70 % of the airborne measurements over Taehwa are taken above 800 hPa. Over Taehwa, airborne dCO∕dCO2 varies with altitude from 8.92 ppbv ppmv−1 below 950 hPa, 10.28 ppbv ppmv−1 below 900 hPa, and 14.74 ppbv ppmv−1 above 400 hPa.
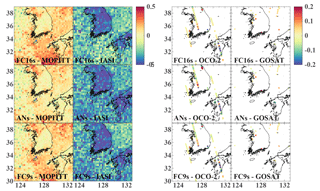
Figure 10Spatial distributions of CAMS bias against satellite retrievals. For XCO, the unit is 1018 molecules cm−2, while for XCO2, the unit is 1021 molecules cm−2.
4.2 Comparison with ship observations
Two research vessels (Jangmok and Onnuri) were deployed during KORUS-OC. The two ships traveled along the South Korean coast and measured CO from 20 May to 5 June (as marked in Fig. 1). Measurements of CO from ships and biases of CAMS FC16s, ANs, and FC9s are shown in Fig. 9. Note that CAMS values along ship tracks are also averaged across a few layers near the surface in the same way CAMS at ground sites was processed. CAMS at three (out of four) ground sites tend to underestimate CO, while CAMS overestimates CO relative to ship measurements. This seems to be inconsistent with our findings with airborne measurements (i.e., CO is underestimated by CAMS at the lowermost troposphere (Figs. 4 and 6). This is likely due to the differences in sampling between the airborne and ship measurements. Over sea, the DC-8 aircraft often sampled air from China outflow while the two ships continuously sampled air over the waters regardless of the presence of China outflows. The ship measurements reflect surface conditions over waters which may also be different from what is observed by the DC-8 aircraft along the vertical profile. This inconsistency is further discussed in the next section with satellite data.
4.3 Comparison with satellite retrievals
The total column dry-air mole fractions of CO2 and CO (XCO2 and XCO) derived from CAMS are compared here to XCO2 from OCO-2 and GOSAT, and XCO from MOPITT and IASI. It is worth noting that satellite retrievals may have associated bias and uncertainties, which are generally larger than those of ground and airborne measurements. Slight inconsistencies also exist between MOPITT XCO and IASI XCO (George et al., 2009, 2015). We show in Fig. 10 the spatial distribution of CAMS biases against these retrievals. We also summarize the statistics in Table 4. Overall, ANs tend to agree better with satellite observations than the forecasts. For CO, CAMS XCO tends to be higher than MOPITT but lower than IASI. In addition, CAMS XCO agrees better with MOPITT than IASI. For CO2, CAMS XCO2 tends to be higher than GOSAT but lower than OCO-2. FC16s, FC9s, and ANs differ from each other in terms of bias when compared to any of the four satellite retrievals although there is no clear difference in terms of RMSE. For XCO, when compared to MOPITT, ANs are better than the two forecasts in terms of bias, RMSE, and correlation. When compared to IASI, ANs are better in terms of correlation but not bias. For XCO2, ANs do not show improvements from the two forecasts when compared to both OCO-2 and GOSAT retrievals. For both XCO and XCO2, FC9s are not necessarily better than FC16s. In summary, ANs XCO shows better agreement with satellite retrievals but this is not the case for XCO2. Differences in the resolution and amount of satellite data of XCO and XCO2 could be two possible causes. The spatial and temporal resolutions of FC16s and FC9s are higher than those of ANs while ANs assimilate observational data from these satellite retrievals (except OCO-2). These two factors compete against each other. Because the amount of CO data (13 612 retrievals for MOPITT and 25 509 for IASI over our study domain during KORUS-AQ) is much larger than that of CO2 (42 for GOSAT over our domain during KORUS-AQ), there are more observational constraints for CO in CAMS resulting in better performance of ANs CO (Fig. 9 and Table 4). The opposite is the case for CO2. The model resolution dominates for CAMS CO2 performance especially with regards to capturing spatiotemporal variability. Scatter plots of CAMS XCO and XCO2 against satellite observations are also presented in Fig. S10 of the Supplement.
We note that CAMS overestimates XCO when compared with MOPITT XCO over the West Sea (Fig. 10). This appears to be contradictory to our conclusions in Sect. 3 and the similar inconsistency also exists when we compare CAMS CO with ship measurements (as mentioned in Sect. 4.2). To further explain this inconsistency, we compare CAMS FC9s with ship measurements and satellite XCO. Because the West Sea flight group in the DC-8 aircraft data forms a zonal “wall” and such measurements over the West Sea are only conducted when a China outflow is expected, we separate the days when China outflows are present. The following are the days during the campaign when China outflows were expected to occur and DC-8 flights measured walls over the West Sea: 3, 17, 24, 29, and 30 May. On 3, 17, 24, and 29 May, there are no MOPITT observations over the West Sea (Fig. S11). Therefore, the overall differences between CAMS FC9s and MOPITT observations are driven by the non-outflow days. On 30 May, however, there are MOPITT observations over the West Sea. Unlike the overall picture (Fig. 10), we find that CAMS actually underestimates the outflows over the West Sea on that day, which is consistent with our findings in Sect. 3. On 1 June (a non-China outflow day), comparison with ship measurements indicates that CAMS FC9s overestimate CO near the South Korean coast. It is also consistent with MOPITT XCO on 1 June (Fig. S11). This overestimation in CAMS FC9s is also captured in our comparison with Baengnyeong (highlighted by a black box in Fig. 9). We find similar overestimation using CAMS FC16s and ANs. Hence, during “normal” conditions, CAMS tend to overestimate CO over the West Sea, whereas during China outflow events, CAMS tends to underestimate CO. More elaborate analysis of source contributions during KORUS-AQ is beyond the scope of this study and can be found in Tang et al. (2018), who suggested that during China outflow events, the contribution from Chinese direct emissions to CO over the West Sea is largely enhanced and dominant.
We use measurements from the NASA DC-8 aircraft, five ground sites (Baengnyeong, Fukue, Olympic Park, Taehwa, and Yonsei University), and two R/Vs (Jangmok and Onnuri) during the KORUS-AQ field campaign, along with four sets of satellite retrievals (MOPITT XCO, IASI XCO, OCO-2 XCO2, and GOSAT XCO2) to evaluate the capability of a high-resolution global modeling system (CAMS) in simulating anthropogenic combustion. Specifically, we evaluate the performance of CAMS FC16s, FC9s, and ANs of CO2, CO, and their relationships. Our assessment of the overall performance of CAMS against the DC-8 aircraft data show that (1) the nominal background CO2 in CAMS is slightly overestimated (bias is 2.2 ppmv for FC16s, 0.7 ppmv for FC9s, and 0.3 ppmv for ANs), which is further improved by CO2 analysis. On the other hand, CO is generally underestimated by CAMS (bias is −19.2 ppbv for FC16s, −16.7 ppbv for FC9s, and −20.7 ppbv for ANs); and (2) among the three forecasts/analysis configurations, FC9s are more accurate and consistent overall than FC16s and ANs because of the finer model resolution and improved initialization. While ANs are coarser in resolution, they generally perform better than FC16s as the impact of initialization surpasses the impact of resolution (Fig. S3). We also classify the airborne measurements into five groups based on land cover below the flight tracks and associated pollution sources. While CO2, CO, and their relationships vary across these five groups, CAMS performs well in terms of simulating regional pattern of anthropogenic combustion. This is because (1) CAMS simulations of both species have relatively low bias; and (2) CAMS reproduces dCO∕dCO2 observed by the DC-8 aircraft. Both CAMS and the DC-8 aircraft data show more efficient combustion (low dCO∕dCO2) over Seoul than over the West Sea which is representative of Chinese outflows. Our case study on the 24 May flight over the West Sea indicates that the Chinese outflow is captured by CAMS. However, the modeled CO and CO2 concentrations are significantly underestimated (by −2 to −4 ppmv for CO2 and −86 to −88 ppbv for CO) especially within the lowermost troposphere. This suggests that, although CAMS emission ratios are relatively consistent with dCO∕dCO2, the absolute magnitudes of China emissions are still underestimated. CAMS also shows poorer performance at local-to-urban scales as exemplified by our case study on the 4 June flight where larger variations near point sources were not represented in CAMS. Our comparisons with measurements from ground sites and two ships indicate that (1) the diurnal cycles of CO and CO2 are stronger over urban environments and such periodic features are reasonably captured by CAMS; (2) vertical mixing near sources (such as Seoul) is too weak in CAMS and needs to be improved; and (3) in some cases, FC9s do not show improvements from FC16s (such as over Seoul and the point sources during the 4 June flight), implying large spatiotemporal errors in emission inventories. In these cases, increasing the spatiotemporal resolution might even weaken the simulation results, whereas lower resolution usually agrees better with observations as it “diffuses” the error of the emissions. We also compared XCO and XCO2 derived from CAMS to satellite retrievals from four instruments (MOPITT CO, IASI CO, OCO-2 CO2, and GOSAT CO2). We find that ANs XCO shows better agreement with satellite retrievals compared to the forecasts, while ANs CO2 is no better than the forecasts. We attribute this contrast to significant differences in the number of XCO and XCO2 satellite data potentially available for assimilation.
We recognize the following limitations of this work. (1) The temporal distributions of airborne measurements are not completely independent from their spatial distributions. For example, most of the measurements in the West Sea group are conducted before noon, whereas measurements in Seoul–Busan jetway are concentrated in the afternoon. (2) CAMS is only evaluated over the South Korean peninsula and surrounding waters during the campaign (1 May to 10 June). More work is needed to determine if our findings are valid over other regions. For example, Agustí-Panareda et al. (2014) reported the overall overestimation of CO2 in spring over the whole NH and it is enhanced by biogenic flux correction. (3) Inconsistencies exist even among different satellite products (George et al., 2009, 2015), thus limiting our comparisons with CAMS to relative differences; and (4) our comparisons of CAMS with ground and ship measurements are only qualitative and indicative as CAMS surface concentrations are significantly higher than surface observations and not comparable.
Finally, this study has important implications on the design and implementation of current and future prediction systems for atmospheric composition and air quality. Although CAMS captured the regional combustion signatures, it still has difficulty representing the variability at local-to-urban scales even at finer resolution. This suggests the need for improvements in both observational constraints and model representation of relevant processes (e.g., emissions and BL mixing).
CAMS 16 km forecasts and analyses are available online at the website of ECMWF (http://apps.ecmwf.int/datasets/data/cams-nrealtime/levtype=sfc/; last access: 1 June 2018). CAMS 9 km forecasts are available upon request. Observational data from KORUS-AQ will be open to public soon (https://www-air.larc.nasa.gov/cgi-bin/ArcView/korusaq; last access: 5 June 2018). All the satellite data used in this study are available online. MOPITT CO and OCO-2 CO2 can be downloaded after NASA Earthdata login (https://reverb.echo.nasa.gov/reverb/; last access: 5 May 2016). IASI CO was downloaded from Jussieu's website for this work, and now can be found at EUMETSAT's website (https://navigator.eumetsat.int/product/EO:EUM:DAT:METOP:IASSND02; last access: 11 July 2018). GOSAT CO2 data after 2014 are available at NIES's website (http://www.gosat.nies.go.jp/en/; last access: 29 March 2017).
The supplement related to this article is available online at: https://doi.org/10.5194/acp-18-11007-2018-supplement.
WT and AFA designed the study. WT analyzed the data with help from AFA and BG. JPD, YC, and GSD conducted airborne measurements. AAP, MP, and SM provided CAMS data, interpretation, and/or analysis. YL, DK, JJ, JH, JWH, YK, ML, RMS, AMT, and JHF provided ground and ship observations. WT wrote the paper with contributions from all other co-authors. JHW provided emission data.
The authors declare that they have no conflict of interest.
This work is supported by NASA KORUS-AQ
(NNX16AE16G and NNX16AD96G). We thank the KORUS-AQ team for observational
data, the CAMS global production team for the model products of CO and
CO2, MOPITT, IASI, OCO-2, and GOSAT data teams for satellite data. IASI
CO is provided by LATMOS/CNRS and ULB. We acknowledge NASA and the OCO-2
project for OCO-2 CO2 data. We thank the DIAL-HSRL team for the mixed
layer heights product. The authors thank Cenlin He and Kazuyuki Miyazaki for helpful comments on improving the paper. NCAR is sponsored by
the National Science Foundation. Yugo Kanaya was supported by the
Environment Research and Technology Development Fund (2-1505 and 2-1803) of
the Ministry of the Environment, Japan. The authors thank the anonymous
reviewers for their comments and suggestions. The CAMS data were generated
using Copernicus Atmosphere Monitoring Service Information (2016).
Edited by: Christoph Gerbig
Reviewed by: two anonymous referees
Agustí-Panareda, A., Massart, S., Chevallier, F., Boussetta, S., Balsamo, G., Beljaars, A., Ciais, P., Deutscher, N. M., Engelen, R., Jones, L., Kivi, R., Paris, J.-D., Peuch, V.-H., Sherlock, V., Vermeulen, A. T., Wennberg, P. O., and Wunch, D.: Forecasting global atmospheric CO2, Atmos. Chem. Phys., 14, 11959–11983, https://doi.org/10.5194/acp-14-11959-2014, 2014.
Agustí-Panareda, A., Massart, S., Chevallier, F., Balsamo, G., Boussetta, S., Dutra, E., and Beljaars, A.: A biogenic CO2 flux adjustment scheme for the mitigation of large-scale biases in global atmospheric CO2 analyses and forecasts, Atmos. Chem. Phys., 16, 10399–10418, https://doi.org/10.5194/acp-16-10399-2016, 2016.
Agustí-Panareda, A., Diamantakis, M., Bayona, V., Klappenbach, F., and Butz, A.: Improving the inter-hemispheric gradient of total column atmospheric CO2 and CH4 in simulations with the ECMWF semi-Lagrangian atmospheric global model, Geosci. Model Dev., 10, 1–18, https://doi.org/10.5194/gmd-10-1-2017, 2017.
Benedetti, A., Morcrette, J. J., Boucher, O., Dethof, A., Engelen, R. J., Fisher, M., Flentje, H., Huneeus, N., Jones, L., Kaiser, J. W., and Kinne, S.: Aerosol analysis and forecast in the European centre for medium-range weather forecasts integrated forecast system: 2. Data assimilation, J. Geophys. Res.-Atmos., 114, D13205, https://doi.org/10.1029/2008JD011115, 2009.
Boesch, H., Baker, D., Connor, B., Crisp, D., and Miller, C.: Global characterization of CO2 column retrievals from shortwave-infrared satellite observations of the Orbiting Carbon Observatory-2 mission, Remote Sensing, 3, 270–304, 2011.
Boschetti, F., Chen, H., Thouret, V., Nedelec, P., Janssens-Maenhout, G., and Gerbig, C.: On the representation of IAGOS/MOZAIC vertical profiles in chemical transport models: contribution of different error sources in the example of carbon monoxide, Tellus B, 67, 28292, https://doi.org/10.3402/tellusb.v67.28292, 2015.
Boussetta, S., Balsamo, G., Beljaars, A., Panareda, A. A., Calvet, J. C., Jacobs, C., Hurk, B., Viterbo, P., Lafont, S., Dutra, E., and Jarlan, L.: Natural land carbon dioxide exchanges in the ECMWF Integrated Forecasting System: Implementation and offline validation, J. Geophys. Res.-Atmos., 118, 5923–5946, 2013.
Brooks, I. M.: Finding boundary layer top: Application of wavelet covariance transform to Lidar backscatter profiles, J. Atmos. Ocean. Tech., 20, 1092–1105, 2003.
Broxton, P. D., Zeng, X., Sulla-Menashe, D., and Troch, P. A.: A global land cover climatology using MODIS data, J. Appl. Meteorol. Clim., 53, 1593–1605, 2014.
Cariolle, D. and Déqué, M.: Southern Hemisphere medium-scale waves and total ozone disturbances in a spectral general circulation model, J. Geophys. Res.-Atmos., 91, 10825–10846, 1986.
Cariolle, D. and Teyssèdre, H.: A revised linear ozone photochemistry parameterization for use in transport and general circulation models: multi-annual simulations, Atmos. Chem. Phys., 7, 2183–2196, https://doi.org/10.5194/acp-7-2183-2007, 2007.
Charlson, R. J., Schwartz, S. E., Hales, J. M., Cess, R. D., Coakley, J. J., Hansen, J. E., and Hofmann, D. J.: Climate forcing by anthropogenic aerosols, Science, 255, 423–430, 1992.
Claeyman, M., Attié, J.-L., El Amraoui, L., Cariolle, D., Peuch, V.-H., Teyssèdre, H., Josse, B., Ricaud, P., Massart, S., Piacentini, A., Cammas, J.-P., Livesey, N. J., Pumphrey, H. C., and Edwards, D. P.: A linear CO chemistry parameterization in a chemistry-transport model: evaluation and application to data assimilation, Atmos. Chem. Phys., 10, 6097–6115, https://doi.org/10.5194/acp-10-6097-2010, 2010.
Clerbaux, C., Boynard, A., Clarisse, L., George, M., Hadji-Lazaro, J., Herbin, H., Hurtmans, D., Pommier, M., Razavi, A., Turquety, S., Wespes, C., and Coheur, P.-F.: Monitoring of atmospheric composition using the thermal infrared IASI/MetOp sounder, Atmos. Chem. Phys., 9, 6041–6054, https://doi.org/10.5194/acp-9-6041-2009, 2009.
Crawford, J. H. and Pickering, K. E.: DISCOVER-AQ: Advancing strategies for air quality observations in the next decade, Environ. Manage., 4–7, EM: The Magazine for Environmental Managers, Air & Waste Management Association, Pittsburgh, PA, available at: https://discover-aq.larc.nasa.gov/pdf/EM0914-60pFNL(L)-Copyright-1.pdf (last access: 11 July 2018), 2014.
Crisp, D., Atlas, R. M., Breon, F. M., Brown, L. R., Burrows, J. P., Ciais, P., Connor, B. J., Doney, S. C., Fung, I. Y., Jacob, D. J., and Miller, C. E.: The orbiting carbon observatory (OCO) mission, Adv. Space Res., 34, 700–709, 2004.
Crisp, D., Fisher, B. M., O'Dell, C., Frankenberg, C., Basilio, R., Bösch, H., Brown, L. R., Castano, R., Connor, B., Deutscher, N. M., Eldering, A., Griffith, D., Gunson, M., Kuze, A., Mandrake, L., McDuffie, J., Messerschmidt, J., Miller, C. E., Morino, I., Natraj, V., Notholt, J., O'Brien, D. M., Oyafuso, F., Polonsky, I., Robinson, J., Salawitch, R., Sherlock, V., Smyth, M., Suto, H., Taylor, T. E., Thompson, D. R., Wennberg, P. O., Wunch, D., and Yung, Y. L.: The ACOS CO2 retrieval algorithm – Part II: Global X data characterization, Atmos. Meas. Tech., 5, 687–707, https://doi.org/10.5194/amt-5-687-2012, 2012.
Deeter, M. N., Martínez-Alonso, S., Edwards, D. P., Emmons, L. K., Gille, J. C., Worden, H. M., Sweeney, C., Pittman, J. V., Daube, B. C., and Wofsy, S. C.: The MOPITT Version 6 product: algorithm enhancements and validation, Atmos. Meas. Tech., 7, 3623–3632, https://doi.org/10.5194/amt-7-3623-2014, 2014.
De Wachter, E., Barret, B., Le Flochmoën, E., Pavelin, E., Matricardi, M., Clerbaux, C., Hadji-Lazaro, J., George, M., Hurtmans, D., Coheur, P.-F., Nedelec, P., and Cammas, J. P.: Retrieval of MetOp-A/IASI CO profiles and validation with MOZAIC data, Atmos. Meas. Tech., 5, 2843–2857, https://doi.org/10.5194/amt-5-2843-2012, 2012.
Doney, S. C., Mahowald, N., Lima, I., Feely, R. A., Mackenzie, F. T., Lamarque, J. F., and Rasch, P. J.: Impact of anthropogenic atmospheric nitrogen and sulfur deposition on ocean acidification and the inorganic carbon system, P. Natl. Acad. Sci. USA, 104, 14580–14585, 2007.
ECMWF: IFS Documentation CY43R3, European Centre for Medium-Range Weather Forecasts, Reading, UK, 2017.
Eskes, H. J., Wagner, A., Schulz, M., Christophe, Y., Ramonet, M., Basart, S., Benedictow, A., Blechschmidt, A.-M., Chabrillat, S., Clark, H., Cuevas, E., Flentje, H., Hansen, K. M., Im, U., Kapsomenakis, J., Langerock, B., Petersen, K., Richter, A., Sudarchikova, N., Thouret, V., Warneke, T., and Zerefos, C.: Validation report of the CAMS near-real-time global atmospheric composition service: Period June–August 2017, Copernicus Atmosphere Monitoring Service (Cams) Report, available at: https://atmosphere.copernicus.eu/sites/default/files/repository/CAMS84_2015SC2_D84.1.1.9_2017JJA_v1_0.pdf (last access: 11 July 2018), 2017.
Feely, R. A., Sabine, C. L., Lee, K., Berelson, W., Kleypas, J., Fabry, V. J., and Millero, F. J.: Impact of anthropogenic CO2 on the CaCO3 system in the oceans, Science, 305, 362–366, 2004.
Flemming, J., Inness, A., Flentje, H., Huijnen, V., Moinat, P., Schultz, M. G., and Stein, O.: Coupling global chemistry transport models to ECMWF's integrated forecast system, Geosci. Model Dev., 2, 253–265, https://doi.org/10.5194/gmd-2-253-2009, 2009.
Flemming, J., Huijnen, V., Arteta, J., Bechtold, P., Beljaars, A., Blechschmidt, A.-M., Diamantakis, M., Engelen, R. J., Gaudel, A., Inness, A., Jones, L., Josse, B., Katragkou, E., Marecal, V., Peuch, V.-H., Richter, A., Schultz, M. G., Stein, O., and Tsikerdekis, A.: Tropospheric chemistry in the Integrated Forecasting System of ECMWF, Geosci. Model Dev., 8, 975–1003, https://doi.org/10.5194/gmd-8-975-2015, 2015.
Flemming, J., Benedetti, A., Inness, A., Engelen, R. J., Jones, L., Huijnen, V., Remy, S., Parrington, M., Suttie, M., Bozzo, A., Peuch, V.-H., Akritidis, D., and Katragkou, E.: The CAMS interim Reanalysis of Carbon Monoxide, Ozone and Aerosol for 2003–2015, Atmos. Chem. Phys., 17, 1945–1983, https://doi.org/10.5194/acp-17-1945-2017, 2017.
Gamnitzer, U., Karstens, U., Kromer, B., Neubert, R. E., Meijer, H. A., Schroeder, H., and Levin, I.: Carbon monoxide: A quantitative tracer for fossil fuel CO2?, J. Geophys. Res.-Atmos., 111, D22302, https://doi.org/10.1029/2005JD006966, 2006.
Gaubert, B., Arellano, A. F., Barré, J., Worden, H. M., Emmons, L. K., Tilmes, S., Buchholz, R. R., Vitt, F., Raeder, K., Collins, N., and Anderson, J. L.: Toward a chemical reanalysis in a coupled chemistry climate model: An evaluation of MOPITT CO assimilation and its impact on tropospheric composition, J. Geophys. Res.-Atmos., 121, 7310–7343, https://doi.org/10.1002/2016JD024863, 2016.
Gaubert, B., Worden, H. M., Arellano, A. F. J., Emmons, L. K., Tilmes, S., Barré, J., Martinez Alonso, S., Vitt, F., Anderson, J. L., Alkemade, F., and Houweling, S.: Chemical Feedback From Decreasing Carbon Monoxide Emissions, Geophys. Res. Lett., 44, 9985–9995, 2017.
George, M., Clerbaux, C., Hurtmans, D., Turquety, S., Coheur, P.-F., Pommier, M., Hadji-Lazaro, J., Edwards, D. P., Worden, H., Luo, M., Rinsland, C., and McMillan, W.: Carbon monoxide distributions from the IASI/METOP mission: evaluation with other space-borne remote sensors, Atmos. Chem. Phys., 9, 8317–8330, https://doi.org/10.5194/acp-9-8317-2009, 2009.
George, M., Clerbaux, C., Bouarar, I., Coheur, P.-F., Deeter, M. N., Edwards, D. P., Francis, G., Gille, J. C., Hadji-Lazaro, J., Hurtmans, D., Inness, A., Mao, D., and Worden, H. M.: An examination of the long-term CO records from MOPITT and IASI: comparison of retrieval methodology, Atmos. Meas. Tech., 8, 4313–4328, https://doi.org/10.5194/amt-8-4313-2015, 2015.
Granier, C., Bessagnet, B., Bond, T. C., D'Angiola, A., Denier van der Gon, H., Frost, G. J., Heil, A., Kaiser, J. W., Kinne, S., Klimont, Z., Kloster, S., Lamarque, J.-F., Liousse, C., Masui, T., Meleux, F., Mieville, A., Ohara, T., Raut, J.-C., Riahi, K., Schultz, M. G., Smith, S. J., Thompson, A., Aardenne, J., Werf, G. R., and Vuuren, D. P.: Evolution of anthropogenic and biomass burning emissions of air pollutants at global and regional scales during the 1980–2010 period, Clim. Change, 109, 163–190, https://doi.org/10.1007/s10584-011-0154-1, 2011.
Guo, S., Hu, M., Zamora, M. L., Peng, J., Shang, D., Zheng, J., Du, Z., Wu, Z., Shao, M., Zeng, L., and Molina, M. J.: Elucidating severe urban haze formation in China, P. Natl. Acad. Sci. USA, 111, 17373–17378, 2014.
Heald, C. L., Jacob, D. J., Park, R. J., Alexander, B., Fairlie, T. D., Yantosca, R. M., and Chu, D. A.: Transpacific transport of Asian anthropogenic aerosols and its impact on surface air quality in the United States, J. Geophys. Res.-Atmos., 111, D14310, https://doi.org/10.1029/2005JD006847, 2006.
Hollingsworth, A., Engelen, R. J., Benedetti, A., Dethof, A., Flemming, J., Kaiser, J. W., Morcrette, J. J., Simmons, A. J., Textor, C., Boucher, O., and Chevallier, F.: Toward a monitoring and forecasting system for atmospheric composition: The GEMS project, B. Am. Meteorol. Soc., 89, 1147–1164, 2008.
Inness, A., Blechschmidt, A.-M., Bouarar, I., Chabrillat, S., Crepulja, M., Engelen, R. J., Eskes, H., Flemming, J., Gaudel, A., Hendrick, F., Huijnen, V., Jones, L., Kapsomenakis, J., Katragkou, E., Keppens, A., Langerock, B., de Mazière, M., Melas, D., Parrington, M., Peuch, V. H., Razinger, M., Richter, A., Schultz, M. G., Suttie, M., Thouret, V., Vrekoussis, M., Wagner, A., and Zerefos, C.: Data assimilation of satellite-retrieved ozone, carbon monoxide and nitrogen dioxide with ECMWF's Composition-IFS, Atmos. Chem. Phys., 15, 5275–5303, https://doi.org/10.5194/acp-15-5275-2015, 2015.
Jacob, D. J., Crawford, J. H., Kleb, M. M., Connors, V. S., Bendura, R. J., Raper, J. L., Sachse, G. W., Gille, J. C., Emmons, L., and Heald, C. L.: Transport and Chemical Evolution over the Pacific (TRACE-P) aircraft mission: Design, execution, and first results, J. Geophys. Res.-Atmos., 108, 1–19, https://doi.org/10.1029/2002jd003276, 2003.
Jacob, D. J., Crawford, J. H., Maring, H., Clarke, A. D., Dibb, J. E., Emmons, L. K., Ferrare, R. A., Hostetler, C. A., Russell, P. B., Singh, H. B., Thompson, A. M., Shaw, G. E., McCauley, E., Pederson, J. R., and Fisher, J. A.: The Arctic Research of the Composition of the Troposphere from Aircraft and Satellites (ARCTAS) mission: design, execution, and first results, Atmos. Chem. Phys., 10, 5191–5212, https://doi.org/10.5194/acp-10-5191-2010, 2010.
Kaiser, J. W., Heil, A., Andreae, M. O., Benedetti, A., Chubarova, N., Jones, L., Morcrette, J.-J., Razinger, M., Schultz, M. G., Suttie, M., and van der Werf, G. R.: Biomass burning emissions estimated with a global fire assimilation system based on observed fire radiative power, Biogeosciences, 9, 527–554, https://doi.org/10.5194/bg-9-527-2012, 2012.
Kanaya, Y., Pan, X., Miyakawa, T., Komazaki, Y., Taketani, F., Uno, I., and Kondo, Y.: Long-term observations of black carbon mass concentrations at Fukue Island, western Japan, during 2009–2015: constraining wet removal rates and emission strengths from East Asia, Atmos. Chem. Phys., 16, 10689–10705, https://doi.org/10.5194/acp-16-10689-2016, 2016.
Kim, S. and Park, R.: KORUS-AQ: An International Cooperative Air Quality Field Study in Korea, the KORUS-AQ white paper, available at: https://espo.nasa.gov/sites/default/files/documents/NASA KORUS-AQ White Paper 5 Dec 2014.pdf (last access: 11 July 2018), 2014.
Kim, S. Y., Jiang, X., Lee, M., Turnipseed, A., Guenther, A., Kim, J. C., Lee, S. J., and Kim, S.: Impact of biogenic volatile organic compounds on ozone production at the Taehwa Research Forest near Seoul, South Korea, Atmos. Environ., 70, 447–453, 2013.
Lawrence, M. G., Jöckel, P., and von Kuhlmann, R.: What does the global mean OH concentration tell us?, Atmos. Chem. Phys., 1, 37–49, https://doi.org/10.5194/acp-1-37-2001, 2001.
Lefer, B. L., Talbot, R. W., Harriss, R. H., Bradshaw, J. D., Sandholm, S. T., Olson, J. O., Sachse, G. W., Collins, J., Shipham, M. A., Blake, D. R., and Klemm, K. I.: Enhancement of acidic gases in biomass burning impacted air masses over Canada, J. Geophys. Res.-Atmos., 99, 1721–1737, 1994.
Lelieveld, J., Gromov, S., Pozzer, A., and Taraborrelli, D.: Global tropospheric hydroxyl distribution, budget and reactivity, Atmos. Chem. Phys., 16, 12477–12493, https://doi.org/10.5194/acp-16-12477-2016, 2016.
Maher, B. A., Ahmed, I. A., Karloukovski, V., MacLaren, D. A., Foulds, P. G., Allsop, D., Mann, D. M., Torres-Jardón, R., and Calderon-Garciduenas, L.: Magnetite pollution nanoparticles in the human brain, P. Natl. Acad. Sci. USA, 113, 10797–10801, 2016.
Mandrake, L., O'Dell, C. W., Wunch, D., Wennberg, P. O., Fisher, B., Osterman, G. B., and Eldering, A.: Orbiting Carbon Observatory-2 (OCO-2) Warn Level, Bias Correction, and Lite File Product Description, Jet Propulsion Laboratory, Pasadena, CA, USA, 2015.
Massart, S., Flemming, J., Cariolle, D., and Jones,L.: High resolution CO tracer forecasts, MACC-III Deliverable D022.04, available at: http://www.gmes-atmosphere.eu/documents/macciii/deliverables/grg/MACCIII_GRG_DEL_D_22.4_LinCO.pdf (last access: 11 July 2018), 2015.
Massart, S., Agustí-Panareda, A., Heymann, J., Buchwitz, M., Chevallier, F., Reuter, M., Hilker, M., Burrows, J. P., Deutscher, N. M., Feist, D. G., Hase, F., Sussmann, R., Desmet, F., Dubey, M. K., Griffith, D. W. T., Kivi, R., Petri, C., Schneider, M., and Velazco, V. A.: Ability of the 4-D-Var analysis of the GOSAT BESD XCO2 retrievals to characterize atmospheric CO2 at large and synoptic scales, Atmos. Chem. Phys., 16, 1653–1671, https://doi.org/10.5194/acp-16-1653-2016, 2016.
Morcrette, J. J., Boucher, O., Jones, L., Salmond, D., Bechtold, P., Beljaars, A., Benedetti, A., Bonet, A., Kaiser, J. W., Razinger, M., and Schulz, M.: Aerosol analysis and forecast in the European Centre for medium-range weather forecasts integrated forecast system: Forward modeling, J. Geophys. Res.-Atmos., 114, D06206, https://doi.org/10.1029/2008JD011235, 2009.
Morino, I., Uchino, O., Inoue, M., Yoshida, Y., Yokota, T., Wennberg, P. O., Toon, G. C., Wunch, D., Roehl, C. M., Notholt, J., Warneke, T., Messerschmidt, J., Griffith, D. W. T., Deutscher, N. M., Sherlock, V., Connor, B., Robinson, J., Sussmann, R., and Rettinger, M.: Preliminary validation of column-averaged volume mixing ratios of carbon dioxide and methane retrieved from GOSAT short-wavelength infrared spectra, Atmos. Meas. Tech., 4, 1061–1076, https://doi.org/10.5194/amt-4-1061-2011, 2011.
OCO-2 Science Team/Gunson, M., and Eldering, A.: OCO-2 Level 2 bias-corrected XCO2 and other select fields from the full-physics retrieval aggregated as daily files, Retrospective processing V7r, Greenbelt, MD, USA, Goddard Earth Sciences Data and Information Services Center (GES DISC),available at: https://disc.gsfc.nasa.gov/datacollection/OCO2_L2_Lite_FP_7r.html (last access: 1 July 2016), 2015.
Ohara, T., Akimoto, H., Kurokawa, J., Horii, N., Yamaji, K., Yan, X., and Hayasaka, T.: An Asian emission inventory of anthropogenic emission sources for the period 1980–2020, Atmos. Chem. Phys., 7, 4419–4444, https://doi.org/10.5194/acp-7-4419-2007, 2007.
Osterman, G. B., Eldering, A., Avis, C., Chafin, B., O'Dell, C. W., Frankenberg, C., Fisher, B. M., Mandrake, L., Wunch, D., Granat, R., and Crisp, D.: Orbiting Carbon Observatory-2 (OCO-2) data product user's guide, operational L1 and L2 data versions 7 and 7R, Jet Propulsion Laboratory, Pasadena, CA, USA, 2016.
Parrish, D. D., Trainer, M., Hereid, D., Williams, E. J., Olszyna, K. J., Harley, R. A., Meagher, J. F., and Fehsenfeld, F. C.: Decadal change in carbon monoxide to nitrogen oxide ratio in US vehicular emissions, J. Geophys. Res.-Atmos., 107, 4140, https://doi.org/10.1029/2001JD000720, 2002.
Persson, A.: User Guide to ECMWF forecast products, Meteorological Bulletin M3.2, ECMWF, available at: http://cedadocs.ceda.ac.uk/1218/1/ECMWF_user_guide_2001.pdf (last access: 11 July 2018), 2001.
Rabier, F., Jarvinen, H., Klinker, E., Mahfouf, J.-F., and Simmons, A.: The ECMWF operational implementation of four-dimensional variational assimilation. I: Experimental results with simplified physics, Q. J. Roy. Meteor. Soc., 126, 1143–1170, 2000.
Shindell, D., Lamarque, J.-F., Unger, N., Koch, D., Faluvegi, G., Bauer, S., Ammann, M., Cofala, J., and Teich, H.: Climate forcing and air quality change due to regional emissions reductions by economic sector, Atmos. Chem. Phys., 8, 7101–7113, https://doi.org/10.5194/acp-8-7101-2008, 2008.
Shindell, D., Faluvegi, G., Walsh, M., Anenberg, S. C., Van Dingenen, R., Muller, N. Z., Austin, J., Koch, D., and Milly, G.: Climate, health, agricultural and economic impacts of tighter vehicle-emission standards, Nature Climate Change, 1, 59–66, 2011.
Silva, S. J., Arellano, A. F., and Worden, H.: Toward anthropogenic combustion emission constraints from space-based analysis of urban CO2/CO sensitivity, Geophys. Res. Lett., 40, 4971–4976, https://doi.org/10.1002/grl.50954, 2013.
Sindelarova, K., Granier, C., Bouarar, I., Guenther, A., Tilmes, S., Stavrakou, T., Müller, J.-F., Kuhn, U., Stefani, P., and Knorr, W.: Global data set of biogenic VOC emissions calculated by the MEGAN model over the last 30 years, Atmos. Chem. Phys., 14, 9317–9341, https://doi.org/10.5194/acp-14-9317-2014, 2014.
Singh, H. B., Brune, W. H., Crawford, J. H., Flocke, F., and Jacob, D. J.: Chemistry and transport of pollution over the Gulf of Mexico and the Pacific: spring 2006 INTEX-B campaign overview and first results, Atmos. Chem. Phys., 9, 2301–2318, https://doi.org/10.5194/acp-9-2301-2009, 2009.
Smith, R. J.: Use and misuse of the reduced major axis for line-fitting, Am. J. Phys. Anthropol., 140, 476–486, 2009.
Suntharalingam, P., Jacob, D. J., Palmer, P. I., Logan, J. A., Yantosca, R. M., Xiao, Y. P., Evans, M. J., Streets, D. G., Vay, S. L., and Sachse, G. W.: Improved quantification of Chinese carbon fluxes using CO2/CO correlations in Asian outflow, J. Geophys. Res.-Atmos., 109, D18S18, https://doi.org/10.1029/2003jd004362, 2004.
Tang, W., Emmons, L. K., Arellano, A. F., Gaubert, B., Knote, C., Tilmes, S., Buchholz, R. R., Pfister, G. G., Diskin, G. S., Blake, D. R., Blake, N. J., Meinardi, S., DiGangi, J P., Choi,Y., Woo, J., He, C., Schroeder, J. R., Suh, I., Lee, H., Jo, H., Kanaya, Y., Jung, J., Lee, Y., and Kim, D.: Source Contribution to Carbon Monoxide during KORUS-AQ Using CAM-chem Tagged Tracers, J. Geophys. Res.-Atmos., under review, 2018.
Taylor, K. E.: Summarizing multiple aspects of model performance in a single diagram, J. Geophys. Res.-Atmos., 106, 7183–7192, 2001.
Toon, O. B., Maring, H., Dibb, J., Ferrare, R., Jacob, D. J., Jensen, E. J., Luo, Z. J., Mace, G. G., Pan, L. L., Pfister, L., and Rosenlof, K. H.: Planning, implementation, and scientific goals of the Studies of Emissions and Atmospheric Composition, Clouds and Climate Coupling by Regional Surveys (SEAC4RS) field mission. J. Geophys. Res.-Atmos., 121, 4967–5009, 2016.
Turnbull, J. C., Tans, P. P., Lehman, S. J., Baker, D., Conway, T. J., Chung, Y. S., Gregg, J., Miller, J. B., Southon, J. R., and Zhou, L. X.: Atmospheric observations of carbon monoxide and fossil fuel CO2 emissions from East Asia, J. Geophys. Res.-Atmos., 116, D24306, https://doi.org/10.1029/2011JD016691, 2011.
United Nations: Population Division: The World's Cities in 2016 – Data Booklet (ST/ESA/ SER.A/392), Department of Economic and Social Affairs, available at: http://www.un.org/en/development/desa/population/publications/pdf/urbanization/the_worlds_cities_in_2016_data_booklet.pdf (last access: 4 August 2017), 2016.
van Leeuwen, T. T. and van der Werf, G. R.: Spatial and temporal variability in the ratio of trace gases emitted from biomass burning, Atmos. Chem. Phys., 11, 3611–3629, https://doi.org/10.5194/acp-11-3611-2011, 2011
Vay, S. A., Woo, J. H., Anderson, B. E., Thornhill, K. L., Blake, D. R., Westberg, D. J., Kiley, C. M., Avery, M. A., Sachse, G. W., Streets, D. G., and Tsutsumi, Y.: Influence of regional-scale anthropogenic emissions on CO2 distributions over the western North Pacific, J. Geophys. Res.-Atmos., 108, 8801, https://doi.org/10.1029/2002JD003094, 2003.
Vay, S. A., Choi, Y., Vadrevu, K. P., Blake, D. R., Tyler, S. C., Wisthaler, A., Hecobian, A., Kondo, Y., Diskin, G. S., Sachse, G. W., and Woo, J. H.: Patterns of CO2 and radiocarbon across high northern latitudes during International Polar Year 2008, J. Geophys. Res.-Atmos., 116, D14301, https://doi.org/10.1029/2011jd015643, 2011.
Wang, Y., Munger, J. W., Xu, S., McElroy, M. B., Hao, J., Nielsen, C. P., and Ma, H.: CO2 and its correlation with CO at a rural site near Beijing: implications for combustion efficiency in China, Atmos. Chem. Phys., 10, 8881–8897, https://doi.org/10.5194/acp-10-8881-2010, 2010.
Warner, J. X., Wei, Z., Strow, L. L., Barnet, C. D., Sparling, L. C., Diskin, G., and Sachse, G.: Improved agreement of AIRS tropospheric carbon monoxide products with other EOS sensors using optimal estimation retrievals, Atmos. Chem. Phys., 10, 9521–9533, https://doi.org/10.5194/acp-10-9521-2010, 2010.
Wofsy, S. C., Sachse, G. W., Gregory, G. L., Blake, D. R., Bradshaw, J. D., Sandholm, S. T., Singh, H. B., Barrick, J. A., Harriss, R. C., Talbot, R. W., Shipham, M. A., Browell, E. V., Jacob, D. J., and Logan, J. A.: Atmospheric chemistry in the Arctic and Subarctic: Influence of natural fires, industrial emissions, and stratospheric inputs, J. Geophys. Res.-Atmos., 97, 16731–16746, 1992.
Wunch, D., Wennberg, P. O., Toon, G. C., Connor, B. J., Fisher, B., Osterman, G. B., Frankenberg, C., Mandrake, L., O'Dell, C., Ahonen, P., Biraud, S. C., Castano, R., Cressie, N., Crisp, D., Deutscher, N. M., Eldering, A., Fisher, M. L., Griffith, D. W. T., Gunson, M., Heikkinen, P., Keppel-Aleks, G., Kyrö, E., Lindenmaier, R., Macatangay, R., Mendonca, J., Messerschmidt, J., Miller, C. E., Morino, I., Notholt, J., Oyafuso, F. A., Rettinger, M., Robinson, J., Roehl, C. M., Salawitch, R. J., Sherlock, V., Strong, K., Sussmann, R., Tanaka, T., Thompson, D. R., Uchino, O., Warneke, T., and Wofsy, S. C.: A method for evaluating bias in global measurements of CO2 total columns from space, Atmos. Chem. Phys., 11, 12317–12337, https://doi.org/10.5194/acp-11-12317-2011, 2011a.
Wunch, D., Toon, G. C., Blavier, J. F. L., Washenfelder, R. A., Notholt, J., Connor, B. J., Griffith, D. W., Sherlock, V., and Wennberg, P. O.: The total carbon column observing network, Philos. T. Roy. Soc. A, 369, 2087–2112, 2011b.
Wunch, D., Wennberg, P. O., Osterman, G., Fisher, B., Naylor, B., Roehl, C. M., O'Dell, C., Mandrake, L., Viatte, C., Kiel, M., Griffith, D. W. T., Deutscher, N. M., Velazco, V. A., Notholt, J., Warneke, T., Petri, C., De Maziere, M., Sha, M. K., Sussmann, R., Rettinger, M., Pollard, D., Robinson, J., Morino, I., Uchino, O., Hase, F., Blumenstock, T., Feist, D. G., Arnold, S. G., Strong, K., Mendonca, J., Kivi, R., Heikkinen, P., Iraci, L., Podolske, J., Hillyard, P. W., Kawakami, S., Dubey, M. K., Parker, H. A., Sepulveda, E., García, O. E., Te, Y., Jeseck, P., Gunson, M. R., Crisp, D., and Eldering, A.: Comparisons of the Orbiting Carbon Observatory-2 (OCO-2) measurements with TCCON, Atmos. Meas. Tech., 10, 2209–2238, https://doi.org/10.5194/amt-10-2209-2017, 2017.
Yang, X. J.: China's rapid urbanization, Science, 342, 310–310, 2013.
Yarwood, G., Rao, S., Yocke, M., and Whitten, G.: Updates to the carbon bond chemical mechanism: CB05. Final report to the US EPA, EPA Report Number: RT-0400675, available at: http://www.camx.com (last access: 10 June 2018), 2005.
Yokelson, R. J., Andreae, M. O., and Akagi, S. K.: Pitfalls with the use of enhancement ratios or normalized excess mixing ratios measured in plumes to characterize pollution sources and aging, Atmos. Meas. Tech., 6, 2155–2158, https://doi.org/10.5194/amt-6-2155-2013, 2013.
Yokota, T., Oguma, H., Morino, I., and Inoue, G.: A nadir looking SWIR FTS to monitor CO2 column density for Japanese GOSAT project, Proc. Twenty-fourth Int. Sympo. on Space Technol. And Sci. (Selected Papers), 30 May–6 June 2004, Miyazaki, Japan, 887–889, 2004.
Yokota, T., Yoshida, Y., Eguchi, N., Ota, Y., Tanaka, T., Watanabe, H., and Maksyutov, S.: Global concentrations of CO2 and CH4 retrieved from GOSAT: First preliminary results, Sola, 5, 160–163, 2009.