the Creative Commons Attribution 4.0 License.
the Creative Commons Attribution 4.0 License.
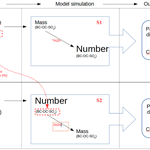
Advancing global aerosol simulations with size-segregated anthropogenic particle number emissions
Filippo Xausa
Pauli Paasonen
Risto Makkonen
Mikhail Arshinov
Aijun Ding
Hugo Denier Van Der Gon
Veli-Matti Kerminen
Markku Kulmala
Climate models are important tools that are used for generating climate change projections, in which aerosol–climate interactions are one of the main sources of uncertainties. In order to quantify aerosol–radiation and aerosol–cloud interactions, detailed input of anthropogenic aerosol number emissions is necessary. However, the anthropogenic aerosol number emissions are usually converted from the corresponding mass emissions in pre-compiled emission inventories through a very simplistic method depending uniquely on chemical composition, particle size and density, which are defined for a few, very wide main source sectors. In this work, the anthropogenic particle number emissions converted from the AeroCom mass in the ECHAM-HAM climate model were replaced with the recently formulated number emissions from the Greenhouse Gas and Air Pollution Interactions and Synergies (GAINS) model. In the GAINS model the emission number size distributions vary, for example, with respect to the fuel and technology. Special attention was paid to accumulation mode particles (particle diameter dp > 100 nm) because of (i) their capability of acting as cloud condensation nuclei (CCN), thus forming cloud droplets and affecting Earth's radiation budget, and (ii) their dominant role in forming the coagulation sink and thus limiting the concentration of sub-100 nm particles. In addition, the estimates of anthropogenic CCN formation, and thus the forcing from aerosol–climate interactions, are expected to be affected. Analysis of global particle number concentrations and size distributions reveals that GAINS implementation increases CCN concentration compared with AeroCom, with regional enhancement factors reaching values as high as 10. A comparison between modeled and observed concentrations shows that the increase in number concentration for accumulation mode particles agrees well with measurements, but it leads to a consistent underestimation of both nucleation mode and Aitken mode (dp < 100 nm) particle number concentrations. This suggests that revisions are needed in the new particle formation and growth schemes currently applied in global modeling frameworks.
- Article
(8072 KB) - Full-text XML
-
Supplement
(447 KB) - BibTeX
- EndNote
In recent years, the link between anthropogenic aerosol particles and climate change has been the subject of several studies (e.g., Baker et al., 2015; Zhang et al., 2016). Anthropogenic aerosol particles play an important role in the global climate system via aerosol–radiation and aerosol–cloud interactions by scattering and absorbing solar radiation and by acting as cloud condensation or ice nuclei, thereby changing many cloud properties (Boucher et al., 2013). The global and regional radiative effects of aerosol particles depend on the spatial and temporal distribution of the aerosol number size distribution and chemical composition (Lohmann and Feichter, 2005; Schulz et al., 2006; Forster et al., 2007; Stier et al., 2007).
While anthropogenic primary emissions introduce cloud condensation nuclei (CCN) directly into the atmosphere, a significant fraction of the global CCN population is likely to be formed through the condensation of organic and other low-volatility vapors onto ultrafine particles (particle diameter dp < 100 nm) in the atmosphere (Spracklen et al., 2008; Merikanto et al., 2009; Kerminen et al., 2012; Paasonen et al., 2013). Aerosol particles and their precursor vapors are emitted from both biogenic and anthropogenic sources, in addition to which they may also result from interactions between biogenic and anthropogenic emissions (Spracklen et al., 2011; Shilling et al., 2013). The increasing number concentration of accumulation mode particles decreases the formation and growth of smaller particles by increasing the sink for condensing vapor molecules, termed the condensation sink (CS; Kulmala et al., 2001), and by increasing the coagulation sink for small, freshly formed particles. Hence, the number concentration of accumulation mode particles from primary emissions affects secondary aerosol formation. The effects of these physical processes on future aerosol climate forcing requires the application of detailed aerosol microphysical schemes in global climate models. Furthermore, the global uncertainty in CCN is highly sensitive to the assumed emission size distribution (Lee et al., 2013).
The global aerosol climate model ECHAM-HAM (Stier et al., 2005; Zhang et al., 2012) is a useful tool that aims at increasing our understanding of aerosol–climate interactions. Past simulations performed with the ECHAM-HAM include an extensive analysis of particle nucleation (Makkonen et al., 2009; Kazil et al., 2010), aerosol properties (Roelofs et al., 2010) and emission inventory implementation (Zhang et al., 2012). Although the ECHAM-HAM has a detailed microphysics module for describing the aerosol size distribution (Vignati et al., 2004), previous studies have not included an exhaustive module for emitted particle number size distribution. Also, in other climate models, the mass-only aerosol input is a commonly applied setting (Jones et al., 2007; Shindell et al., 2007). Advances in primary emission size distribution have been hindered by global climate model limitations in both the structure of the aerosol microphysics and the availability of size-segregated emission inventories.
One of the emission inventories that has been widely used in ECHAM-HAM simulations, as well as in other Earth system models (Pozzoli et al., 2011; Makkonen et al., 2009, 2012; Tonttila et al., 2015), is the Aerosol Inter Comparison inventory, AeroCom (Dentener et al., 2006), developed for the purpose of conducting improved simulations of aerosol–climate interactions (Samset et al., 2014). However, the AeroCom emission inventory does not include a specific framework for particle number emissions. Hence, the input particle number emissions used in the simulations with AeroCom are estimated from the particle mass emissions by the ECHAM-HAM during the initialization routine. In more detail, the estimation of number emissions consists of a simplistic multiplication of the given AeroCom mass emissions by a mass-to-number conversion factor. Each conversion factor that is applied for building the lognormal distribution is calculated by assuming that the mass emissions for each main source sector are distributed to predefined modes according to predefined densities, geometric mean radii and standard deviations, as described by Vignati et al. (2004) and Stier et al. (2005). This simplistic mass-to-number conversion factor does not represent the relationship between the particle mass and number size distributions in a realistic way because such a framework does not take into account the variation in emitted particle number size distributions from different emitting sources. The AeroCom inventory includes anthropogenic activities, from which the mass-to-number converted emissions are split into half between the Aitken and accumulation modes and finally converted into lognormal modes. However, the recently developed inventories allow for global aerosol simulations with a more detailed aerosol emission size distribution (Paasonen et al., 2016) with the GAINS emission scenario model (Greenhouse Gas and Air Pollution Interactions and Synergies; Amann et al., 2011). The GAINS inventory is organized into more detailed anthropogenic sources than AeroCom, with different particle number emissions and size distributions related to different fuels and technologies.
In this work, we first develop a novel module for anthropogenic particle number emissions in Earth system models. Our experiment, performed with the ECHAM-HAM, consists of replacing the mass-to-number converted anthropogenic AeroCom aerosol emissions with number emissions from the GAINS model. In more detail, the implementation of the GAINS inventory is performed by using ECHAM-HAM default assumptions for the AeroCom inventory implementation. This study has a two objectives: first, it aims at improving the ECHAM-HAM capability for estimating particle number concentrations, with a special focus on accumulation mode particles, and second, it investigates the feasibility of using the GAINS model for global climate modeling studies by running the ECHAM-HAM with both AeroCom and GAINS inventories. We present a comparison between the novel GAINS implementation and the default implementation of AeroCom in the ECHAM-HAM, including modeled particle number concentrations and size distributions, as well as modeled CCN number concentrations. Finally, we compare the modeled number size distributions with observations in different environments around the world.
2.1 The ECHAM5.5-HAM2 climate model
We used the global aerosol climate model ECHAM5.5-HAM2 (Stier et al., 2005; Zhang et al., 2012) with the M7 microphysics module (Vignati et al., 2004). The M7 describes the aerosol number size distribution with seven lognormal modes, in which the Aitken, accumulation and coarse modes are present in both the soluble and insoluble phases, while the nucleation mode is present only as the soluble mode. The compounds modeled in our simulations are black carbon (BC), organic carbon (OC), sulfate (SO4), dust and sea salt. The emission module used in the ECHAM-HAM reads data for anthropogenic, biogenic, wildfire, volcanic, agricultural emissions, secondary organic aerosols (SOAs) and shipping sources. In our experiments, we modified only the part of the ECHAM-HAM source code that handles the anthropogenic emissions. The model has a horizontal Gaussian grid (192 × 96) with a grid box size of ∼ 200 × 200 km at the equator and a vertical resolution of 31 hybrid sigma layers.
2.1.1 Aerosol microphysics
The version of the ECHAM-HAM used in this work includes nucleation, condensation and coagulation modules. Previous studies have shown that the implementation of an activation-type nucleation improves particle number concentration estimations in modeling (Spracklen et al., 2010; Makkonen et al., 2012). In our experiment, we coupled a binary sulfuric acid–water nucleation scheme (Vehkamäki et al., 2002) with an activation–nucleation scheme described by Paasonen et al. (2010, Eq. 10), in which the nucleation rate (J) is a function of the activation coefficient and sulfuric acid concentration, expressed as
The settings of our simulations included a specific module for SOA formation. Here, we modeled the SOA formation with both kinetic condensation onto a Fuchs-corrected surface area (CS) and partitioning according to a preexisting organic mass (Riipinen et al., 2011; Jokinen et al., 2015). This SOA module includes three biogenic volatile organic compound (BVOC) tracers: isoprene, endocyclic monoterpenes and other monoterpenes, each having monthly resolutions for emissions. We did not use any nucleation scheme for organic vapors because the simple activation-type nucleation, while not accurate for individual sites, describes the nucleation in different environments reasonably well (Paasonen et al., 2010). The particle growth from nucleation size to the dp of 3 nm was calculated according to Kerminen and Kulmala (2002), considering both sulfuric acid and organic vapor condensation. More details can be found in Makkonen et al. (2012).
2.1.2 Natural emissions
BVOC emissions were implemented using the MEGAN2 (Guenther et al., 2006) model. MEGAN2 estimates biogenic emissions for about 150 compounds from different ecosystems, paying particular attention to monoterpenes. This framework takes into account several factors that influence BVOC emissions, including the leaf age, soil moisture and light environment. MEGAN2 was run off-line and its output data were used for the ECHAM-HAM input initialization.
All non-anthropogenic emissions, such as volcanic emissions, dimethyl-sulfide (DMS; Kloster et al., 2006) emitted by the sea and dust, were taken from AeroCom in both simulations. All emission data, excluding SOA precursors, DMS emissions and wildfire, were input as annual averages. As a result, the seasonality in concentrations of anthropogenic compounds is mostly due to the nudged meteorology.
2.1.3 Anthropogenic emissions
The first simulation was performed with the ECHAM-HAM default implementation of anthropogenic emissions from the AeroCom inventory for the year 2000. The AeroCom emissions taken by the ECHAM-HAM are provided by mass as kg m−2 s−1 with a chemical differentiation that includes BC, OC and SO4 and a bi-level vertical distribution (2-zL) that consists of two surface layers: a lower level below 100 m above the sea level for emissions from transportation and domestic combustion and a higher level for industrial activities whose emissions reach altitudes higher than 100 m. While BC does not require preprocessing during the simulation, input emissions of OC and SO4 undergo a further conversion during the initialization routine: OC mass is converted into primary organic matter (POM) mass with a multiplying factor of 1.4 (Turpin et al., 2000; Kupiainen and Klimont, 2007), and emissions containing sulfur (S) are input as both sulfur dioxide (SO2) and SO4. The primary SO4 particle fraction is estimated as 2.5 % of gaseous SO2, as described by Dentener et al. (2006). The masses of BC and POM are uniquely treated as Aitken mode particles (dp = 10–100 nm). The mass of SO4 is divided between the Aitken mode, accumulation mode (dp = 100–1000 nm) and coarse mode (dp > 1 µm) through a rough estimation: the lower surface-level SO4 is split equally between the Aitken mode and accumulation mode, whereas the higher surface-level SO4 is split equally between the accumulation mode and coarse mode. The mass is then converted by the model into a particle number size distribution. The mass-to-number flux factors, expressed as m2n in Fig. 1, are embedded in the emission-reading routine. The number of particles is calculated through the generic function
where M is the mass of given emissions and m is the average mass estimated for a single particle. The particle mass m in Eq. (2) is extended in the model according to the Hatch–Choate conversion equations (Hinds, 1982), in which the density, count median radius and standard deviation are predefined for each chemical compound and size mode, as described by Stier et al. (2005). The emission count median radius is fixed at 30 and 75 nm for the Aitken mode and accumulation mode, respectively, and the standard deviation is set to 1.59 for all the modes except the coarse mode, for which it is 2.0. The species density is set to 1841 kg m−3 for SO4 (input in the model as H2SO4) and 2000 kg m−3 for BC and OC. Altogether, these parameters differentiate between the species according to their chemistry and solubility. The number flux conversion is therefore expressed as
where ρ is the density of a determined chemical compound i and the expression in brackets is the mean radius of a particle with certain solubility j and size mode k. The quantity cmr is the predefined count median radius as it is expressed in the model code, while cmr2ram is a conversion factor that multiplies cmr in order to estimate the radius of average mass. The cmr2ram factor depends uniquely on the standard deviation of the lognormal particle number distribution.
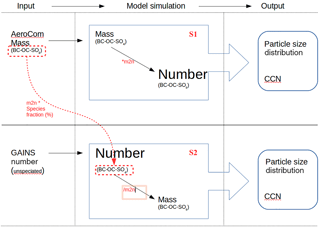
Figure 1Framework describing the off-line steps to implement GAINS mass and number anthropogenic emissions in the ECHAM-HAM. The AeroCom mass-to-number (m2n) conversion factors and the chemical species fractions (%) of AeroCom number emissions were used to speciate GAINS number emissions. A specific m2n factor was used for each species for either mass-to-number (*m2n) or number-to-mass (/m2n) conversion.
2.2 Emission scenario model GAINS
The GAINS model is an integrated assessment model developed at IIASA (International Institute for Applied Systems Analysis) in Laxenburg, Austria (Amann et al., 2011). In order to calculate the emissions related to specific anthropogenic source sectors, it combines the information of the annual level of the anthropogenic activities, amounts of different fuels consumed for combustion activities, shares of different emission abatement technologies and emission factors for different activity–fuel–technology combinations.
The GAINS scenarios include information on the annual activity levels and shares of emission control technologies for nearly 170 regions, i.e., countries or parts or groups of countries, at 5-year intervals from 1990 to 2050. The activity levels are based on national and international statistics, the latter being available from the International Energy Agency (IEA), the Organisation for Economic Co-operation and Development (OECD), the United Nations (UN), the Food and Agriculture Organization of the United Nations (FAO), and Eurostat, and the shares of control technologies are derived from national and international information on the related legislation, discussion with national experts and scientific publications. The emission factors for all combinations of source sectors, fuels and technologies are determined from the scientific publications or measurement databases. For a detailed description of sources and methods to derive underlying particulate matter emissions, see Klimont et al. (2017).
The particle number emission factors with the related number size distributions were recently implemented to GAINS (Paasonen et al., 2016). This implementation allowed for a detailed assessment of particle number emissions, with more than 1000 measures controlling emissions in each of the close to 170 regions, in an internally consistent manner with emissions of other air pollutants and greenhouse gases. The GAINS particle number emissions are known to be subject to uncertainties, especially in terms of nucleation mode emissions, but the major particle number sources, such as road transport and residential combustion, are reasonably well represented down to the control technology level. The determination of emission factors for particle number emissions and particle size distributions is based on the European particle number emission inventory developed by TNO (Netherland Organisation for Applied Scientific Research) (Denier van der Gon et al., 2009, 2010).
In this study, we applied the gridded particle number emissions for the year 2010 (Paasonen et al., 2016), in which the activity measures and emission abatement technology shares are based on the ECLIPSE version 5 inventory (Klimont et al., 2017) developed within the EU FP7 ECLIPSE project (Stohl et al., 2015). The gridded data and their brief characterization are freely available from the IIASA website: http://www.iiasa.ac.at/web/home/research/researchPrograms/air/PN.html (last access: 10 July 2018).
2.3 GAINS implementation in M7
In the second simulation, the sub-module that converts the input mass to the number flux described in Eqs. (2)–(3) was switched off and we implemented the recently developed 2010 GAINS anthropogenic emissions (Paasonen et al., 2016; see also Sect. 2.1.2). The emission sectors considered for our experiment included the energy production, flares, industrial combustion and processes, transportation, waste combustion, and domestic or commercial combustion. A detailed description of the sectors and emission factors is presented in Paasonen et al. (2016).
The number size distribution inventory provided by GAINS is organized into nine size bins with a geometric diameter ranging from 3 to 1000 nm. However, in this study we implemented the GAINS inventory for the Aitken mode and accumulation mode only (dp = 10–1000 nm), so that the particle number implementation was consistent with the AeroCom simulation which lacked the nucleation mode conversion factor in the source code aerosol module. The conversion of GAINS emissions from sectional to modal size distribution was performed by splitting the total particle number concentration from the GAINS inventory between the Aitken and accumulation modes using the GAINS sectional particle diameter of 100 nm as the limit between these two modes. The rest of the modal parameters, i.e., the modal median radii and standard deviations, were taken as the default values of the ECHAM-HAM modal properties (Stier et al., 2005). This choice of implementation does not fully exploit all the information available in the GAINS size distribution because the default ECHAM-HAM emission module does not allow the emission diameter to vary on a per-grid-box basis. Although it would be possible to upgrade the ECHAM-HAM in this sense, it would be quite laborious and beyond the scope of our study. It should be noted that the ratio of Aitken to accumulation mode emissions can vary between grid cells in both AeroCom and GAINS. In AeroCom this variation is due to different mass-to-number conversion factors for different emission sectors, but in GAINS the size distributions are also different for different technologies and fuels within the emission sectors (e.g., different vehicle technologies, different domestic stove categories, diesel fuels with different sulfur contents, different coal types).
In the GAINS simulation we kept the AeroCom gas phase sulfur and coarse SO4 in order to identify the global impact of GAINS implementation on submicron particles. Furthermore, we used the same bi-level 2-zL scheme as for the SO4 vertical distribution in AeroCom: emissions from the transportation, agriculture fires, waste combustion and domestic combustion were put into the lower level (< 100 m a.s.l.), whereas the energy, flares, industry and power plant sectors of GAINS were implemented into the higher level (> 100 m a.s.l.).
GAINS provides the number emissions without chemical speciation and vertical distribution (see Table 1) and separately provides mass emissions of particle mass, particulate OC and BC, as well as gaseous pollutants, including SO2. However, distributing the different compounds between the different number size bins is a nontrivial task which, in order to be properly completed, requires an elaboration of the proper GAINS model, not only the implementation. For this reason, we decided to use the default ECHAM-HAM particle composition from AeroCom in this study and leave the implementation of the GAINS chemical composition for future studies. We followed a series of steps in order to partition the GAINS raw data into BC, POM and SO4 in a consistent format for the model. Table 1 and Fig. 1 visually illustrate the implementation framework. In more detail, we (I) off-line converted AeroCom mass into number using ECHAM-HAM factors, (II) estimated the chemical species fraction among the respective Aitken mode and accumulation mode in AeroCom numbers, (III) applied such fractions to the total Aitken mode and accumulation mode particle numbers in the GAINS to have the correspondent BC, OC and SO4 repartition, and finally, (IV) used the mass-to-number factors used in (I) to estimate the speciated GAINS mass. The above framework highlights that, while the mass-to-number conversion factors are unaltered for each specific mode, the mass taken from AeroCom and GAINS inventories by the ECHAM-HAM is different. Although the mass is not the focus of our study, this difference may have further implications in terms of simulating particle mass concentrations (see the Supplement for the total PM2.5 concentrations).
Shipping emissions are embedded in the AeroCom inventory but not included in GAINS. In our experiment, we masked out the AeroCom shipping emissions with a land–sea mask produced by applying Climate Data Operator (CDO) to the AeroCom. Hence, shipping emissions were not taken into consideration. Biomass burning emissions are included as mass-based emissions from the AeroCom inventory.
Table 1Input data provided from AeroCom and GAINS inventories for submicron particle emissions. The data are sorted according to their original structure in terms of mass, number, chemical species differentiation (BC, OC and SO4), bi-level vertical distribution (2-zL) and base year. ✓ and X indicate, respectively, whether the inventory contains certain information or not.
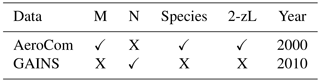
2.4 Simulation setup
Our experiment consisted of two 1-year simulations, using identical model settings but different inventories for anthropogenic sources: AeroCom and GAINS (see Sect. 2.3). The experiment run was set to start indicatively on 1 October 2009 and end on 31 December 2010 with a 3-month spin-up period and 1 h time resolution for the output. The modeled data for our analysis were collected from 1 January 2010 to 31 December 2010. The model was nudged against 2010 ECMWF ERA-Interim (Berrisford et al., 2011) observed meteorology in order to reduce noise in model estimations and to increase the statistical significance of the eventual anthropogenic aerosol perturbation signal (Kooperman et al., 2012).
2.5 Comparison with observation
Our study focused on particle number concentration and size distributions along with CCN concentrations at supersaturations of 0.2 % (CCN0.2) and 1.0 % (CCN1.0). We compared the modeled particle number concentrations and size distributions against observations collected from 11 sites around the world. A detailed description of the observation data is illustrated in Table 2. The modeled data extracted from all sites were averaged over the year and plotted against observations to investigate the overall model performance. In addition to the visual comparison between the modeled and observed concentrations, we calculated the relative bias, i.e., the ratio of modeled and measured concentrations, for each measurement site. For the sites where the ratio was smaller than 1, the bias was replaced with its multiplicative inverse. In this way we were able to calculate and compare the averages of the relative biases at different sites between the model runs.
The particle number concentration and mean particle radius of all the output data were used for plotting the number distributions of 6 of the 11 original sites, which were chosen to represent areas with a strong presence of anthropogenic emissions (Nanjing, São Paulo and Tomsk) as well as areas dominated by biogenic emissions (Hyytiälä, K-Puszta and Värriö). In both annual-average and number distribution comparisons, the modeled layer closest to Earth's surface was chosen for analysis. Modeled CCN concentrations were studied by comparing simulations with AeroCom emissions against those from GAINS emissions for both CCN0.2 and CCN1.0. CCN concentrations were extracted and averaged from the lowest three model layers in order to reduce background noise in mapping the global concentrations. Due to the coarse grid size and inhomogeneous sources around measurement sites, the evaluation against observations is not expected to yield one-to-one validation of aerosol concentrations (Schutgens et al., 2016).
Here we show the comparison between AeroCom and GAINS implementation before (emissions, Sect. 3.1) and after (atmospheric concentrations, Sect. 3.2 and 3.3) running the ECHAM-HAM model. Our experiment was performed with the same model settings in both simulations, and it was nudged against meteorology data. As a result, our analysis focused merely on the differences between the particle number emissions of the two inventories and their different effects on modeled particle concentrations. In the following sections, we will first show the difference between AeroCom and GAINS in terms of input emissions, after which we will compare the model-simulated particle number concentrations and size distributions with observational data. Finally, we will assess the effect of the GAINS implementation on global CCN concentrations.
3.1 Differences in particle number emissions
In this section, we present a preliminary assessment of input emissions to illustrate the main differences between the two inventories before starting the simulation. Table 3 shows global anthropogenic emissions and their ratios between GAINS and AeroCom for the whole domain. When the emissions were globally averaged (Rtot), GAINS showed higher total number emissions by a factor of 2.2. However, when looking at individual grid cells, the total particle number emission ratios between AeroCom and GAINS had a large spatial variability (Fig. 2), even though the median value of this ratio was very close to 1 (see Rgrid in Table 3). Figure 3 shows the spatial distribution of both emissions inventories. Globally, the Aitken-to-accumulation-mode particle emission ratio was about 2 orders of magnitude in AeroCom emissions, while being less than a factor of 4 in GAINS emission. The averaged emission ratios demonstrate that accumulation mode emissions play a critical role in the GAINS implementation, with both Rtot and Rgrid ratios increasing dramatically compared with AeroCom. The averaged Aitken mode particle emissions from GAINS did not show a similar increase, and the Rgrid median value was even lower than that in the AeroCom emissions. The Rtot and Rgrid ratios of Aitken mode emissions were 1.7 and 0.7, respectively. This difference shows that the Aitken mode particle emissions are quantitatively higher in GAINS than in AeroCom when their geographical distribution differences are not taken into account. However, when the inventories were compared by confronting each grid cell one by one, AeroCom emissions were higher than GAINS emissions in a prevalent area of the global domain.
Table 3Annual total anthropogenic particle number emissions (second and third columns) and respective global average ratios (fourth and fifth columns) computed for the whole domain. Rtot ratios are calculated by firstly averaging the emissions among the whole domain for each data set and secondly by dividing GAINS by AeroCom. This method aims at studying absolute differences in the global emissions with no regard to geographical distribution differences. In Rgrid we firstly divide the data sets to keep the information of data set differences for each grid cell and secondly compute the median of gridded ratios. Rgrid is weighted by surface area of the grid cell.
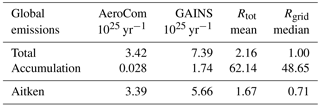
In the ECHAM-HAM, fossil fuel and biofuel are emitted into the Aitken insoluble mode and are converted into soluble particles after sulfate condensation. In GAINS, the particles estimated to contain BC are distributed into particle size bins at around 100 nm (Paasonen et al., 2016). The difference between the diameters of emissions from fossil fuel and biofuel combustion is the major reason behind the differences in accumulation mode emissions and concentrations.
3.2 Simulated particle number concentrations and size distributions
Here we present the core of our analysis, which includes an assessment of the modeled particle number concentrations against observations. Figure 4 shows the annually averaged modeled particle concentration in comparison with observations from 11 sites. Overall, both emission inventories showed a tendency to underestimate particle number concentrations in model simulations, especially for the locations with high observed particle number concentrations. The underestimation of the highest particle concentrations might be, at least partly, related to the spatial resolution of the ECHAM-HAM, due to which the typically high particle concentrations near urban or industrial areas will be distributed evenly into a large model grid cell (Stier et al., 2005). A comparison of the model results with the observational data shows that the GAINS implementation significantly improved the reproduction of observed concentrations in accumulation mode (dp > 100 nm), being closer to observations than AeroCom at all 11 sites. For the Aitken mode (dp = 10–100 nm), a similar improvement was not reached, as the observed concentrations were better reproduced with AeroCom than with GAINS at eight sites. The average relative bias described in Sect. 2.5 for the accumulation mode concentrations was 2.37 with GAINS emissions and 3.51 with AeroCom emissions. The average relative bias for the Aitken mode concentrations was 2.25 and 2.12 with GAINS and AeroCom emissions, respectively. It should be noted that the emissions from different emission sources and observations are not all from the same years. However, even though the GAINS emissions are for the year 2010 and AeroCom emissions for the year 2000 (and observations for the years indicated in Table 2), the differences in the modeled concentrations with GAINS and AeroCom at the most polluted sites, reaching factors of 2 and above, cannot be expected to originate in differences in emissions between 2000 and 2010.
Figure 5 shows the modeled particle number size distributions against observations at six measurement sites. The size distributions modeled with the GAINS emissions agreed relatively well with the measurements for the accumulation mode, whereas the nucleation and Aitken modes were underestimated in simulations with both emission inventories. GAINS underestimated the Aitken mode particle concentrations more heavily than AeroCom, by a factor of 2 to 3 in Hyytiälä, Värriö and K-Puszta, suggesting that the higher CS associated with higher accumulation mode particle emissions in GAINS had a significant impact on modeled ultrafine particle number concentrations. In addition, Hyytiälä and Värriö are regions in which BVOC emissions and clean air are the key influencing factors for new particle formation and particle growth (Ruuskanen et al., 2007; Corrigan et al., 2013; Liao et al., 2014). This was reflected in the model results: particle number size distributions in Hyytiälä and Värriö were quite similar between the two simulations based on different anthropogenic emission inventories. Contrary to this, Nanjing, São Paulo and Tomsk are areas with strong influences by anthropogenic emissions, so that in comparison with AeroCom, the simulations with GAINS emissions produced higher accumulation mode and Aitken mode particle number concentrations as well as better agreements with the observations in these regions. Nevertheless, the model was not able to reach the observed ultrafine particle concentration in either simulation in most areas, and the higher CS in GAINS significantly reduced particle number concentrations of the smallest particles in most regions. Some areas showed a dramatic reduction in simulated ultrafine particle number concentrations; e.g., in Nanjing the whole modeled nucleation mode was wiped out when using the GAINS emissions.
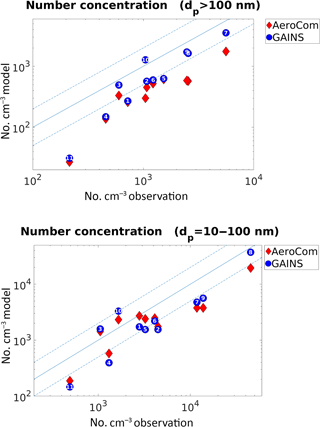
Figure 4Annually averaged number of particles compared to observational data. Measurement sites: 1: Botsalano; 2: Cabauw 3: Hohenpeißenberg; 4: Hyytiälä; 5: K-Puszta; 6: Melpitz; 7: Nanjing; 8: Po Valley; 9: São Paulo; 10: Tomsk FNV; 11: Värriö. Both plots include 1 : 1 and dashed 1 : 2, 2 : 1 lines.
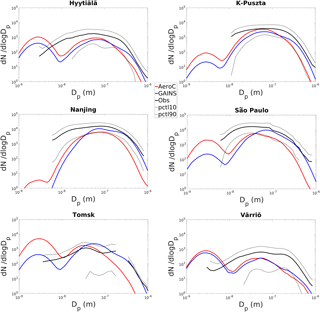
Figure 5Modeled particle number size distributions compared to observations at six measurement sites.
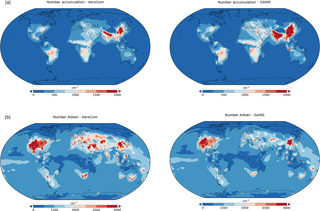
Figure 6Modeled annual particle number concentrations for accumulation mode (a) and Aitken mode (b) at surface level.
The differences in Aitken and accumulation mode emissions between GAINS and AeroCom implementations originate in three main differences. Firstly, the GAINS emission factors, especially in traffic and residential combustion sectors, are directly based on literature or databases of particle number emissions, whereas in AeroCom the number emissions are converted from mass emissions. This causes differences in the relative shares of different source sectors in the emission size distributions. Secondly, the original emission size distributions in GAINS contains one to three different modes, whereas in AeroCom the emissions are represented with only one mode. In many GAINS sources, e.g., road transport, the mode with a larger mean emission diameter contributes significantly to the emission of particles with dp > 100 nm, even though the total number emission is clearly dominated by a mode with a smaller mean diameter. Finally, as stated earlier, the GAINS emission size distributions are different for different technologies and fuels – in diesel-powered road transport also for different fuel sulfur contents. This increases the regional variability in the emissions.
The above results suggest that in the ECHAM-HAM the current nucleation and growth schemes may need further revisions. However, it is also likely that the anthropogenic emissions of nucleation mode particles, in particular, in GAINS are still severely underestimated for many source sectors (Paasonen et al., 2016). This is because many of the measurements on which the GAINS emission factors are based are not sensitive to nonsolid nucleation mode particles, such as those formed via nucleation of sulfur or organic vapors immediately after the combustion or at small downwind distances in plumes from different combustion sources (Stevens and Pierce, 2013). It should also be noted that our study does not include any sensitivity analysis based on the primary sulfate emissions parameterization (Luo and Yu, 2011). In addition, the lower modeled Aitken mode particle concentrations from GAINS emissions may, in some parts of the global domain, also be related to possible overestimations in the accumulation mode particle emissions in the GAINS model, which consequently affect the formation and growth of smaller particles. Nonetheless, all the model-versus-observation comparisons between the simulations clearly represent a consistent challenge for climate models in modeling ultrafine particle number size distributions.
Figure 6 shows absolute annual-average particle concentrations for the accumulation mode and Aitken mode with both AeroCom and GAINS emissions. While the regional distributions had similar patterns in both simulations, there were evident differences when looking at the two size modes. Accumulation mode particle concentrations were higher for the simulation with the GAINS emission in most regions, which is consistent with the input emissions assessment. The differences were particularly evident over the developing areas where anthropogenic activities represent the main source of atmospheric particles, especially in South America, central Africa, India, China and southeast Asia. As observed in Fig. 5, the high accumulation mode particle number concentrations in the simulation with the GAINS emission have a critical effect on Aitken mode particle concentrations at most sites. A peculiar pattern is observed in China, where the dominant presence of anthropogenic sources from GAINS led the model to predict high concentrations of ultrafine particles. The decrease in GAINS-derived Aitken mode particle number concentrations in areas where emissions were actually higher than the AeroCom emission implies that Aitken mode particles had been removed, or their secondary production was hindered, by the prominent increase in the CS caused by a higher number of emitted accumulation mode particles. It is important to note that while the accumulation mode particle concentration played a major role in increasing the CS (hence boosting the Aitken mode particle removal), the difference in the particle number concentrations of the Aitken mode might be also due to the lower Aitken mode emissions in GAINS (see Table 3). However, in this research it was not possible to quantify how much of this difference was actually due to the different Aitken mode particle number emissions.
3.3 Concentrations and sources of CCN
This section presents the impact of particle emissions on atmospheric CCN concentrations from annual and seasonal perspectives. It is important to note that the applied anthropogenic number emissions did not have seasonal variation, so the seasonal differences are entirely due to the variation in other emissions and mainly to the strong temperature dependence of biogenic SOA formation affecting the CCN concentration (Paasonen et al., 2013). Our results showed clear differences in the simulated CCN concentrations between the two primary emission inventories, and these differences depended strongly on the considered supersaturation (Table 4, Figs. 7 and 8).
At the 0.2 % supersaturation, the CCN concentrations were higher with the GAINS emissions compared with the AeroCom emissions in practically all the regions and during all seasons (Fig. 8). The annual-average CCN0.2 concentration ratio between the GAINS and AeroCom was 2 to 3 in most areas, with peaks of 4 to 10 in south America, central Africa and east Asia (Fig. 7). However, relatively high accumulation mode particle concentrations were observed in India, China and southeast Asia (see Fig. 6), and an increase in absolute CCN0.2 concentration due to anthropogenic emissions was also observed in eastern China and southeast Asia. Our analysis of the seasonality revealed that the difference between GAINS and AeroCom simulations in terms of CCN0.2 concentrations was the largest during the cold season in January, with boreal and arctic regions showing an increment of the GAINS ∕ AeroCom CCN0.2 ratio up to a factor of 7 to 10. The Southern Hemisphere also displayed notable differences in both South America and southeast Asia, with GAINS ∕ AeroCom CCN0.2 ratios of 3 to 10 during the warmest season.
At a supersaturation of 1.0 %, a significant fraction of Aitken mode particles is capable of acting as CCN. In contrast with the CCN0.2 concentrations, the simulated CCN1.0 concentrations with GAINS emissions were lower than with AeroCom emissions, with a GAINS ∕ AeroCom ratio of between 0.5 and 1 in most regions (Fig. 7). Our seasonality analysis showed that the simulation with the GAINS inventory produced higher CCN1.0 concentrations than AeroCom in Europe, India and East Asia during the winter. However, such a ratio was equal to 1 or below in most regions, except eastern Asia, during the warmer seasons. The substantially lower CCN1.0 concentrations with GAINS emissions arise from the relatively similar Aitken mode number emissions between GAINS and AeroCom but significantly larger CS from GAINS, causing a decrease in secondary ultrafine particle formation. However, in China and southeast Asia, the annual CCN1.0 concentration from GAINS was higher than from AeroCom by at least a factor of 2, suggesting that these regions may play a key role in contributing to the global anthropogenic emissions and increment of CCN.
It is important to remark that the substantial differences in CCN concentrations illustrated above are linked to the implementation of different inventories, and therefore the modeled estimations might be affected by uncertainties in the GAINS model as well. Furthermore, it may be questioned whether the ECHAM-HAM is actually able to estimate CCN concentrations with GAINS better than with AeroCom. This goes beyond the fundamental goal of this study, which is to address the feasibility of using GAINS emissions in global climate modeling. However, the modeled GAINS accumulation mode particle number concentrations agree with observation significantly better than AeroCom. This, based on the sensitivity analysis by Lee et al. (2013), suggests that the GAINS implementation is likely to estimate CCN concentrations better than AeroCom. In any case, further studies are needed to address the contribution of the GAINS model in improving modeled CCN concentration. Furthermore, it would be beneficial to investigate how the applied nucleation scheme, combined with the GAINS anthropogenic emissions, affects the estimation of CCN concentration to better identify the driving forces behind the uncertainties of modeling particle number size distributions with the global climate models.
The outcome of our experiment shows that the most significant differences between the GAINS and AeroCom emissions inventories are (i) the particle number emissions in the Aitken mode and accumulation mode and (ii) the geographical distribution of the particle number emissions over the global domain. The accumulation mode particle emissions from GAINS are significantly higher than AeroCom, by factors from 10 to 1000, thus potentially resulting in dramatic increases in climatically active primary particles and simultaneous decreases in secondary ultrafine particle formation due to higher values of CS and coagulation sink.
In comparison to AeroCom emissions, GAINS emissions produced much higher accumulation mode particle concentrations, but the consequently higher CS and coagulation sink led to lower Aitken mode concentrations with GAINS emissions than with AeroCom emissions. In comparison to observation data at 11 measurement sites, the modeled annually averaged concentrations with GAINS emissions performed better than with AeroCom emissions, in terms of bringing the modeled accumulation mode particle concentrations closer to observation at all 11 sites and Aitken mode particle concentrations closer to observation at 3 sites. However, a higher underestimation was observed in the simulation with GAINS emissions for particles with dp < 30 nm.
The underestimation of dp < 30 nm particle concentrations in the simulation with GAINS emissions highlighted the sensitivity of nucleation mode and Aitken mode particle concentrations to CS and coagulation sink. This underestimation is presumably partly caused by underestimations in emissions of nonsolid nucleation/Aitken mode particles in the GAINS model (Paasonen et al., 2016). As a next step, the modules for nucleation and subsequent growth and the sensitivity of the concentrations of sulfuric acid (the main precursor in the applied nucleation parameterization) to altered CS should be revisited.
It is important to note that the simulations performed in this study did not implement an up-to-date secondary organic aerosol (ELVOCS) nucleation scheme, nor a seasonal cycle of anthropogenic emissions, which may represent a further step to reduce the gap between the modeled and observed concentrations. Finally, given the high spatial variability in global emissions, more observation data and the establishment of new measurement stations in varying environments are urgently needed to better evaluate the model results.
The data of our analysis cannot be accessed freely because they are stored on a server which requires user credentials to go through its layer of security. Also, the data cannot be accessed easily as they are stored as terabytes of data. It is recommended that if an individual wants to access the data he/she should contact the first author directly.
The supplement related to this article is available online at: https://doi.org/10.5194/acp-18-10039-2018-supplement.
FX performed the model setup, the simulations and the analysis and drafted most of the manuscript. PP, RM and VMK elaborated on the idea for the experiment, supervised the model setup and the analysis, and drafted part of the manuscript. The other coauthors provided some of the measurement data and contributed to enhancing the quality of the text.
The authors declare that they have no conflict of interest.
This article is part of the special issue “Pan-Eurasian Experiment (PEEX)”. It is not associated with a conference.
This project was funded by the MAJ JA TOR NESSLING grant no. 201600369 and the Academy of Finland Center of Excellence (FCoE) grant no. 307331.
Particle number size distributions at Melpitz were provided by Wolfram Birmili, Kay Weinhold, André Sonntag, Birgit Wehner, Thomas Tuch and Alfred Wiedensohler (Leibniz Institute for Tropospheric Research, Leipzig, Germany).
Particle number size distributions at Hohenpeißenberg were provided by Harald Flentje and Björn Briel (German Weather Service, Hohenpeißenberg, Germany). Both measurements were supported by the German Federal Environment Ministry (BMU) grant UFOPLAN 370343200 (project duration 2008-2010). Both data sets can be publicly accessed through the German Ultrafine Aerosol Network (GUAN) at https://doi.org/10.5072/guan.
Particle number size distributions at Botsalano were provided by Ville Vakkari and Lauri Laakso (Finnish Meteorological Institute, Helsinki, Finland).
Particle number size distributions at São Paulo were provided by John Backman (Finnish Meteorological Institute, Helsinki, Finland).
Particle number size distributions at San Pietro Capofiume (Po Valley) were provided by Ari Laaksonen (Finnish Meteorological Institute, Helsinki, Finland).
We thank Chris Heyes and Zbigniew Klimont from the Air Quality and Greenhouse Gases program at IIASA and Kaarle Kupiainen from IIASA and the Finnish Environment Institute (SYKE) for their help and communication.
The EU FP7 BACCHUS project (grant no. 603445) and the Nordic Center of
Excellence eSTICC (Nordforsk grant no. 57001) are acknowledged for financial
support.
Edited by: Dominick Spracklen
Reviewed by: H. Gordon and one anonymous referee
Amann, M., Bertok, I., Borken-Kleefeld, J., Cofala, J., Heyes, C., Höglund-Isaksson, L., Klimont, Z., Nguyen, B., Posch, M., Rafaj, P., Sandler, R., Schöpp, W., Wagner, F., and Winiwarter, W.: Cost-effective control of air quality and greenhouse gases in Europe: Modeling and policy applications, Environ. Model. Softw., 26, 1489–1501, 2011.
Backman, J., Rizzo, L. V., Hakala, J., Nieminen, T., Manninen, H. E., Morais, F., Aalto, P. P., Siivola, E., Carbone, S., Hillamo, R., Artaxo, P., Virkkula, A., Petäjä, T., and Kulmala, M.: On the diurnal cycle of urban aerosols, black carbon and the occurrence of new particle formation events in springtime São Paulo, Brazil, Atmos. Chem. Phys., 12, 11733–11751, https://doi.org/10.5194/acp-12-11733-2012, 2012.
Baker, L. H., Collins, W. J., Olivié, D. J. L., Cherian, R., Hodnebrog, Ø., Myhre, G., and Quaas, J.: Climate responses to anthropogenic emissions of short-lived climate pollutants, Atmos. Chem. Phys., 15, 8201–8216, https://doi.org/10.5194/acp-15-8201-2015, 2015.
Berrisford, P., Kållberg, P., Kobayashi, S., Dee, D., Uppala, S., Simmons, A. J., Poli, P., and Sato, H.: Atmospheric conservation properties in ERA-Interim, Q. J. Roy. Meteor. Soc., 137, 1381–1399, 2011.
Birmili, W., Weinhold, K., Rasch, F., Sonntag, A., Sun, J., Merkel, M., Wiedensohler, A., Bastian, S., Schladitz, A., Löschau, G., Cyrys, J., Pitz, M., Gu, J., Kusch, T., Flentje, H., Quass, U., Kaminski, H., Kuhlbusch, T. A. J., Meinhardt, F., Schwerin, A., Bath, O., Ries, L., Gerwig, H., Wirtz, K., and Fiebig, M.: Long-term observations of tropospheric particle number size distributions and equivalent black carbon mass concentrations in the German Ultrafine Aerosol Network (GUAN), Earth Syst. Sci. Data, 8, 355–382, https://doi.org/10.5194/essd-8-355-2016, 2016.
Boucher, O., Randall, D., Artaxo, P., Bretherton, C., Feingold, G., Forster, P., Kerminen, V.-M., Kondo, Y., Liao, H., Lohmann, U., Rasch, P., Satheesh, S. K., Sherwood, S., Stevens, B., and Zhang, X. Y.: Clouds and aerosols, in: Climate Change 2013: The Physical Science Basis. Contribution of Working Group I to the Fifth Assessment Report of the Intergovernmental Panel on Climate Change, edited by: Stocker, T. F., Qin, D., Plattner, G.-K., Tignor, M., Allen, S. K., Doschung, J., Nauels, A., Xia, Y., Bex, V., and Midgley, P. M., Cambridge University Press, 571–657, https://doi.org/10.1017/CBO9781107415324.016, 2013.
Corrigan, A. L., Russell, L. M., Takahama, S., Äijälä, M., Ehn, M., Junninen, H., Rinne, J., Petäjä, T., Kulmala, M., Vogel, A. L., Hoffmann, T., Ebben, C. J., Geiger, F. M., Chhabra, P., Seinfeld, J. H., Worsnop, D. R., Song, W., Auld, J., and Williams, J.: Biogenic and biomass burning organic aerosol in a boreal forest at Hyytiälä, Finland, during HUMPPA-COPEC 2010, Atmos. Chem. Phys., 13, 12233–12256, https://doi.org/10.5194/acp-13-12233-2013, 2013.
Dal Maso, M., Sogacheva, L., Anisimov, M. P., Arshinov, M., Baklanov, A., Belan, B., Khodzher, T. V., Obolkin, V. A., Staroverova, A., Vlasov, A., Zagaynov, V. A., Lushnikov, A., Lyubovtseva, Y. S., Riipinen, I., Kerminen, V.-M., and Kulmala, M.: Aerosol particle formation events at two Siberian stations inside the boreal forest, Boreal Environ. Res., 13, 81–92, 2008.
Denier van der Gon, H., Visschedijk, A., Johansson, C., Hedberg Larsson, E., Harrison, R. M., and Beddows, D.: Size-resolved Pan European Anthropogenic Particle Number Inventory, EUCAARI Deliverable report D141, 2009.
Denier van der Gon, H., Visschedijk, A., Johansson, C., Ntziachristos, L., and Harrison, R. M.: Size-resolved Pan-European Anthropogenic Particle Number Inventory, paper presented at International Aerosol conference (oral), 29 August–3 September 2010, Helsinki, Finland, 2010.
Dentener, F., Kinne, S., Bond, T., Boucher, O., Cofala, J., Generoso, S., Ginoux, P., Gong, S., Hoelzemann, J. J., Ito, A., Marelli, L., Penner, J. E., Putaud, J.-P., Textor, C., Schulz, M., van der Werf, G. R., and Wilson, J.: Emissions of primary aerosol and precursor gases in the years 2000 and 1750 prescribed data-sets for AeroCom, Atmos. Chem. Phys., 6, 4321–4344, https://doi.org/10.5194/acp-6-4321-2006, 2006.
Forster, P., Ramaswamy, V., Artaxo, P., Berntsen, T., Betts, R., Fahey, D.W., Haywood, J., Lean, J., Lowe, D. C., Myhre, G., Nganga, J., Prinn, R., Raga, G., Schulz, M., Van Dorland, R., and Miller, H. L.: Changes in Atmospheric Constituents and in Radiative Forcing, Chapter 2, Cambridge University Press, UK, 2007.
Guenther, A., Karl, T., Harley, P., Wiedinmyer, C., Palmer, P. I., and Geron, C.: Estimates of global terrestrial isoprene emissions using MEGAN (Model of Emissions of Gases and Aerosols from Nature), Atmos. Chem. Phys., 6, 3181–3210, https://doi.org/10.5194/acp-6-3181-2006, 2006.
Hamed, A., Joutsensaari, J., Mikkonen, S., Sogacheva, L., Dal Maso, M., Kulmala, M., Cavalli, F., Fuzzi, S., Facchini, M. C., Decesari, S., Mircea, M., Lehtinen, K. E. J., and Laaksonen, A.: Nucleation and growth of new particles in Po Valley, Italy, Atmos. Chem. Phys., 7, 355–376, https://doi.org/10.5194/acp-7-355-2007, 2007.
Hari, P., Kulmala, M., Pohja, T., Lahti, T., Siivola, E., Palva, L., Aalto, P., Hämeri, K., Vesala, T., Luoma, S., and Pulliainen, E.: Air pollution in Eastern Lapland: challenge for an environmental measurement station, Silva Fenn., 28, 29–39, 1994.
Hari, P. and Kulmala, M.: Station for Measuring Ecosystem-Atmosphere Relations (SMEAR II), Boreal Environ. Res., 10, 315–322, 2005.
Herrmann, E., Ding, A. J., Kerminen, V.-M., Petäjä, T., Yang, X. Q., Sun, J. N., Qi, X. M., Manninen, H., Hakala, J., Nieminen, T., Aalto, P. P., Kulmala, M., and Fu, C. B.: Aerosols and nucleation in eastern China: first insights from the new SORPES-NJU station, Atmos. Chem. Phys., 14, 2169–2183, https://doi.org/10.5194/acp-14-2169-2014, 2014.
Hinds, W. C.: Aerosol Technology, Wiley, New York, p. 85, 1982.
Jokinen, T., Berndt, T., Makkonen, R., Kerminen, V., Junninen, H., Paasonen, P., Stratmann, F., Herrmann, H., Guenther, A. B., Worsnop, D. R., Kulmala, M., Ehn, M., and Sipilä, M.: Production of extremely low volatile organic compounds from biogenic emissions: Measured yields and atmospheric implications, P. Natl. Acad. Sci. USA, 112, 7123–7128, 2015.
Jones, A., Haywood, J. M., and Boucher, O.: Aerosol forcing, climate response and climate sensitivity in the Hadley Centre climate model, J. Geophys. Res., 112, D20211, https://doi.org/10.1029/2007JD008688, 2007.
Kazil, J., Stier, P., Zhang, K., Quaas, J., Kinne, S., O'Donnell, D., Rast, S., Esch, M., Ferrachat, S., Lohmann, U., and Feichter, J.: Aerosol nucleation and its role for clouds and Earth's radiative forcing in the aerosol-climate model ECHAM5-HAM, Atmos. Chem. Phys., 10, 10733–10752, https://doi.org/10.5194/acp-10-10733-2010, 2010.
Kerminen, V.-M. and Kulmala, M.: Analytical formulae connecting the “real” and the “apparent” nucleation rate and the nuclei number concentration for atmospheric nucleation events, J. Aerosol Sci., 33, 609–622, 2002.
Kerminen, V.-M., Paramonov, M., Anttila, T., Riipinen, I., Fountoukis, C., Korhonen, H., Asmi, E., Laakso, L., Lihavainen, H., Swietlicki, E., Svenningsson, B., Asmi, A., Pandis, S. N., Kulmala, M., and Petäjä, T.: Cloud condensation nuclei production associated with atmospheric nucleation: a synthesis based on existing literature and new results, Atmos. Chem. Phys., 12, 12037–12059, https://doi.org/10.5194/acp-12-12037-2012, 2012.
Kiss, G., Varga, B., Galambos, I., and Ganszky, I. Characterization of water-soluble organic matter isolated from atmospheric fine aerosol, J. Geophys. Res., 107, 8339–8347, https://doi.org/10.1029/2001JD000603, 2002.
Klimont, Z., Kupiainen, K., Heyes, C., Purohit, P., Cofala, J., Rafaj, P., Borken-Kleefeld, J., and Schöpp, W.: Global anthropogenic emissions of particulate matter including black carbon, Atmos. Chem. Phys., 17, 8681–8723, https://doi.org/10.5194/acp-17-8681-2017, 2017.
Kloster, S., Feichter, J., Maier-Reimer, E., Six, K. D., Stier, P., and Wetzel, P.: DMS cycle in the marine ocean-atmosphere system – a global model study, Biogeosciences, 3, 29–51, https://doi.org/10.5194/bg-3-29-2006, 2006.
Kooperman, G. J., Pritchard, M. S., Ghan, S. J., Wang, M., Somerville, R. C. J., and Russell, L. M.: Constraining the influence of natural variability to improve estimates of global aerosol indirect effects in a nudged version of the Community Atmosphere Model 5, J. Geophys. Res., 117, D23204, https://doi.org/10.1029/2012JD018588, 2012.
Kulmala, M., Dal Maso, M., Mäkelä, J., Pirjola, L., Väkevä, M., Aalto, P., Miikkulainen, P., Hämeri, K., and O'dowd, C.: On the formation, growth and composition of nucleation mode particles, Tellus B, 53, 479–490, https://doi.org/10.3402/tellusb.v53i4.16622, 2001.
Kupiainen, K. and Klimont, Z.: Primary emissions of fine carbonaceous particles in Europe, Atmos. Environ., 41, 2156–2170, 2007.
Laakso, L., Laakso, H., Aalto, P. P., Keronen, P., Petäjä, T., Nieminen, T., Pohja, T., Siivola, E., Kulmala, M., Kgabi, N., Molefe, M., Mabaso, D., Phalatse, D., Pienaar, K., and Kerminen, V.-M.: Basic characteristics of atmospheric particles, trace gases and meteorology in a relatively clean Southern African Savannah environment, Atmos. Chem. Phys., 8, 4823–4839, https://doi.org/10.5194/acp-8-4823-2008, 2008.
Lee, L. A., Pringle, K. J., Reddington, C. L., Mann, G. W., Stier, P., Spracklen, D. V., Pierce, J. R., and Carslaw, K. S.: The magnitude and causes of uncertainty in global model simulations of cloud condensation nuclei, Atmos. Chem. Phys., 13, 8879–8914, https://doi.org/10.5194/acp-13-8879-2013, 2013.
Liao, L., Kerminen, V.-M., Boy, M., Kulmala, M., and Dal Maso, M.: Temperature influence on the natural aerosol budget over boreal forests, Atmos. Chem. Phys., 14, 8295–8308, https://doi.org/10.5194/acp-14-8295-2014, 2014.
Lohmann, U. and Feichter, J.: Global indirect aerosol effects: a review, Atmos. Chem. Phys., 5, 715–737, https://doi.org/10.5194/acp-5-715-2005, 2005.
Luo, G. and Yu, F.: Sensitivity of global cloud condensation nuclei concentrations to primary sulfate emission parameterizations, Atmos. Chem. Phys., 11, 1949–1959, https://doi.org/10.5194/acp-11-1949-2011, 2011.
Makkonen, R., Asmi, A., Korhonen, H., Kokkola, H., Järvenoja, S., Räisänen, P., Lehtinen, K. E. J., Laaksonen, A., Kerminen, V.-M., Järvinen, H., Lohmann, U., Bennartz, R., Feichter, J., and Kulmala, M.: Sensitivity of aerosol concentrations and cloud properties to nucleation and secondary organic distribution in ECHAM5-HAM global circulation model, Atmos. Chem. Phys., 9, 1747–1766, https://doi.org/10.5194/acp-9-1747-2009, 2009.
Makkonen, R., Asmi, A., Kerminen, V.-M., Boy, M., Arneth, A., Guenther, A., and Kulmala, M.: BVOC-aerosol-climate interactions in the global aerosol-climate model ECHAM5.5-HAM2, Atmos. Chem. Phys., 12, 10077–10096, https://doi.org/10.5194/acp-12-10077-2012, 2012.
Merikanto, J., Spracklen, D. V., Mann, G. W., Pickering, S. J., and Carslaw, K. S.: Impact of nucleation on global CCN, Atmos. Chem. Phys., 9, 8601–8616, https://doi.org/10.5194/acp-9-8601-2009, 2009.
Paasonen, P., Nieminen, T., Asmi, E., Manninen, H. E., Petäjä, T., Plass-Dülmer, C., Flentje, H., Birmili, W., Wiedensohler, A., Hõrrak, U., Metzger, A., Hamed, A., Laaksonen, A., Facchini, M. C., Kerminen, V.-M., and Kulmala, M.: On the roles of sulphuric acid and lowvolatility organic vapours in the initial steps of atmospheric new particle formation, Atmos. Chem. Phys., 10, 11223–11242, https://doi.org/10.5194/acp-10-11223-2010, 2010.
Paasonen, P., Asmi, A., Petäjä, T., Kajos, M. K, Äijälä, M., Junninen, H., Holst, T., Abbatt, J. P. D., Arneth, A., Birmili, W., van der Gon, H. D., Hamed, A., Hoffer, A., Laakso, L., Laaksonen, A., Richard Leaitch, W., Plass-Dülmer, C., Pryor, S. C., Räisänen, P., Swietlicki, E., Wiedensohler, A., Worsnop, D. R., Kerminen, V.-M., and Kulmala, M.: Warming-induced increase in aerosol number concentration likely to moderate climate change, Nat. Geosci., 6, 438–442, 2013.
Paasonen, P., Kupiainen, K., Klimont, Z., Visschedijk, A., Denier van der Gon, H. A. C., and Amann, M.: Continental anthropogenic primary particle number emissions, Atmos. Chem. Phys., 16, 6823–6840, https://doi.org/10.5194/acp-16-6823-2016, 2016.
Pozzoli, L., Janssens-Maenhout, G., Diehl, T., Bey, I., Schultz, M. G., Feichter, J., Vignati, E., and Dentener, F.: Re-analysis of tropospheric sulfate aerosol and ozone for the period 1980–2005 using the aerosol-chemistry-climate model ECHAM5-HAMMOZ, Atmos. Chem. Phys., 11, 9563–9594, https://doi.org/10.5194/acp-11-9563-2011, 2011.
Riipinen, I., Pierce, J. R., Yli-Juuti, T., Nieminen, T., Häkkinen, S., Ehn, M., Junninen, H., Lehtipalo, K., Petäjä, T., Slowik, J., Chang, R., Shantz, N. C., Abbatt, J., Leaitch, W. R., Kerminen, V.-M., Worsnop, D. R., Pandis, S. N., Donahue, N. M., and Kulmala, M.: Organic condensation: a vital link connecting aerosol formation to cloud condensation nuclei (CCN) concentrations, Atmos. Chem. Phys., 11, 3865–3878, https://doi.org/10.5194/acp-11-3865-2011, 2011.
Roelofs, G.-J., ten Brink, H., Kiendler-Scharr, A., de Leeuw, G., Mensah, A., Minikin, A., and Otjes, R.: Evaluation of simulated aerosol properties with the aerosol-climate model ECHAM5-HAM using observations from the IMPACT field campaign, Atmos. Chem. Phys., 10, 7709–7722, https://doi.org/10.5194/acp-10-7709-2010, 2010.
Ruuskanen, T. M., Kaasik, M., Aalto, P. P., Hõrrak, U., Vana, M., Mårtensson, M., Yoon, Y. J., Keronen, P., Mordas, G., Ceburnis, D., Nilsson, E. D., O'Dowd, C., Noppel, M., Alliksaar, T., Ivask, J., Sofiev, M., Prank, M., and Kulmala, M.: Concentrations and fluxes of aerosol particles during the LAPBIAT measurement campaign at Värriö field station, Atmos. Chem. Phys., 7, 3683–3700, https://doi.org/10.5194/acp-7-3683-2007, 2007.
Samset, B. H., Myhre, G., Herber, A., Kondo, Y., Li, S.-M., Moteki, N., Koike, M., Oshima, N., Schwarz, J. P., Balkanski, Y., Bauer, S. E., Bellouin, N., Berntsen, T. K., Bian, H., Chin, M., Diehl, T., Easter, R. C., Ghan, S. J., Iversen, T., Kirkevåg, A., Lamarque, J.-F., Lin, G., Liu, X., Penner, J. E., Schulz, M., Seland, Ø., Skeie, R. B., Stier, P., Takemura, T., Tsigaridis, K., and Zhang, K.: Modelled black carbon radiative forcing and atmospheric lifetime in AeroCom Phase II constrained by aircraft observations, Atmos. Chem. Phys., 14, 12465–12477, https://doi.org/10.5194/acp-14-12465-2014, 2014.
Schulz, M., Textor, C., Kinne, S., Balkanski, Y., Bauer, S., Berntsen, T., Berglen, T., Boucher, O., Dentener, F., Guibert, S., Isaksen, I. S. A., Iversen, T., Koch, D., Kirkevåg, A., Liu, X., Montanaro, V., Myhre, G., Penner, J. E., Pitari, G., Reddy, S., Seland, Ø., Stier, P., and Takemura, T.: Radiative forcing by aerosols as derived from the AeroCom present-day and pre-industrial simulations, Atmos. Chem. Phys., 6, 5225-5246, https://doi.org/10.5194/acp-6-5225-2006, 2006.
Schutgens, N. A. J., Gryspeerdt, E., Weigum, N., Tsyro, S., Goto, D., Schulz, M., and Stier, P.: Will a perfect model agree with perfect observations? The impact of spatial sampling, Atmos. Chem. Phys., 16, 6335–6353, https://doi.org/10.5194/acp-16-6335-2016, 2016.
Shilling, J. E., Zaveri, R. A., Fast, J. D., Kleinman, L., Alexander, M. L., Canagaratna, M. R., Fortner, E., Hubbe, J. M., Jayne, J. T., Sedlacek, A., Setyan, A., Springston, S., Worsnop, D. R., and Zhang, Q.: Enhanced SOA formation from mixed anthropogenic and biogenic emissions during the CARES campaign, Atmos. Chem. Phys., 13, 2091–2113, https://doi.org/10.5194/acp-13-2091-2013, 2013.
Shindell, D. T., Faluvegi, G., Bauer, S. E., Koch, D. M., Unger, N., Menon, S., Miller, R. L., Schmidt, G. A., and Streets, D. G.: Climate response to projected changes in short-lived species under an A1B scenario from 2000–2050 in the GISS climate model, J. Geophys. Res., 112, D20103, https://doi.org/10.1029/2007JD008753, 2007.
Spracklen, D. V., Karslav, K. S., Kerminen, V.-M., Sihto, S.-L., Riipinen, I., Merikanto, J., Mann, G. W., Chipperfield, M. P., Wiedensohler, A., Birmili, W., and Lihavainen, H.: Contribution of particle formation to global cloud condensation nuclei concentrations, Geophys. Res. Lett., 35, L06808, https://doi.org/10.1029/2007GL033038, 2008.
Spracklen, D. V., Carslaw, K. S., Merikanto, J., Mann, G. W., Reddington, C. L., Pickering, S., Ogren, J. A., Andrews, E., Baltensperger, U., Weingartner, E., Boy, M., Kulmala, M., Laakso, L., Lihavainen, H., Kivekäs, N., Komppula, M., Mihalopoulos, N., Kouvarakis, G., Jennings, S. G., O'Dowd, C., Birmili, W., Wiedensohler, A., Weller, R., Gras, J., Laj, P., Sellegri, K., Bonn, B., Krejci, R., Laaksonen, A., Hamed, A., Minikin, A., Harrison, R. M., Talbot, R., and Sun, J.: Explaining global surface aerosol number concentrations in terms of primary emissions and particle formation, Atmos. Chem. Phys., 10, 4775–4793, https://doi.org/10.5194/acp-10-4775-2010, 2010.
Spracklen, D. V., Jimenez, J. L., Carslaw, K. S., Worsnop, D. R., Evans, M. J., Mann, G. W., Zhang, Q., Canagaratna, M. R., Allan, J., Coe, H., McFiggans, G., Rap, A., and Forster, P.: Aerosol mass spectrometer constraint on the global secondary organic aerosol budget, Atmos. Chem. Phys., 11, 12109–12136, https://doi.org/10.5194/acp-11-12109-2011, 2011.
Stevens, R. G. and Pierce, J. R.: A parameterization of sub-grid particle formation in sulfur-rich plumes for global- and regional-scale models, Atmos. Chem. Phys., 13, 12117–12133, https://doi.org/10.5194/acp-13-12117-2013, 2013.
Stier, P., Feichter, J., Kinne, S., Kloster, S., Vignati, E., Wilson, J., Ganzeveld, L., Tegen, I., Werner, M., Balkanski, Y., Schulz, M., Boucher, O., Minikin, A., and Petzold, A.: The aerosol-climate model ECHAM5-HAM, Atmos. Chem. Phys., 5, 1125–1156, https://doi.org/10.5194/acp-5-1125-2005, 2005.
Stier, P., Seinfeld, J. H., Kinne, S., and Boucher, O.: Aerosol absorption and radiative forcing, Atmos. Chem. Phys., 7, 5237–5261, https://doi.org/10.5194/acp-7-5237-2007, 2007.
Stohl, A., Aamaas, B., Amann, M., Baker, L. H., Bellouin, N., Berntsen, T. K., Boucher, O., Cherian, R., Collins, W., Daskalakis, N., Dusinska, M., Eckhardt, S., Fuglestvedt, J. S., Harju, M., Heyes, C., Hodnebrog, Ø., Hao, J., Im, U., Kanakidou, M., Klimont, Z., Kupiainen, K., Law, K. S., Lund, M. T., Maas, R., MacIntosh, C. R., Myhre, G., Myriokefalitakis, S., Olivié, D., Quaas, J., Quennehen, B., Raut, J.-C., Rumbold, S. T., Samset, B. H., Schulz, M., Seland, Ø., Shine, K. P., Skeie, R. B., Wang, S., Yttri, K. E., and Zhu, T.: Evaluating the climate and air quality impacts of short-lived pollutants, Atmos. Chem. Phys., 15, 10529–10566, https://doi.org/10.5194/acp-15-10529-2015, 2015.
Tonttila, J., Järvinen, H., and Räisänen, P.: Explicit representation of subgrid variability in cloud microphysics yields weaker aerosol indirect effect in the ECHAM5-HAM2 climate model, Atmos. Chem. Phys., 15, 703–714, https://doi.org/10.5194/acp-15-703-2015, 2015.
Turpin, B. J., Saxena, P., and Andrews, E.: Measuring and simulating particulate organics in the atmosphere: problems and prospects, Atmos. Environ., 34, 2983–3013, 2000.
van Ulden, A. and Wieringa, J.: Atmospheric boundary layer research at Cabauw, Bound.-Lay. Meteorol., 78, 39–69, 1996.
Vehkamäki, H., Kulmala, M., Napari, I., Lehtinen, K. E. J., Timmreck, C., Noppel, M., and Laaksonen, A.: An improved parameterization for sulfuric acid–water nucleation rates for tropospheric and stratospheric conditions, J. Geophys. Res., 107, 4622, https://doi.org/10.1029/2002JD002184, 2002.
Vignati, E., Wilson, J., and Stier, P.: M7: An efficient size-resolved aerosol microphysics module for large-scale aerosol transport models, J. Geophys. Res., 109, D22202, https://doi.org/10.1029/2003JD004485, 2004.
Zhang, K., O'Donnell, D., Kazil, J., Stier, P., Kinne, S., Lohmann, U., Ferrachat, S., Croft, B., Quaas, J., Wan, H., Rast, S., and Feichter, J.: The global aerosol-climate model ECHAM-HAM, version 2: sensitivity to improvements in process representations, Atmos. Chem. Phys., 12, 8911–8949, https://doi.org/10.5194/acp-12-8911-2012, 2012.
Zhang, S., Wang, M., Ghan, S. J., Ding, A., Wang, H., Zhang, K., Neubauer, D., Lohmann, U., Ferrachat, S., Takeamura, T., Gettelman, A., Morrison, H., Lee, Y., Shindell, D. T., Partridge, D. G., Stier, P., Kipling, Z., and Fu, C.: On the characteristics of aerosol indirect effect based on dynamic regimes in global climate models, Atmos. Chem. Phys., 16, 2765–2783, https://doi.org/10.5194/acp-16-2765-2016, 2016.