the Creative Commons Attribution 4.0 License.
the Creative Commons Attribution 4.0 License.
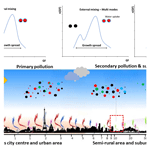
External particle mixing influences hygroscopicity in a sub-urban area
Birgit Wehner
Silvia Henning
Jean-Eudes Petit
Pauline Fombelle
Olivier Favez
Hartmut Herrmann
Mira Pöhlker
Hygroscopicity strongly influences aerosol properties and multiphase chemistry, which is essential in several atmospheric processes. Although CCN (cloud condensation nuclei) properties are commonly measured, sub-saturated hygroscopicity measurements remain rare. During the ACROSS campaign (Atmospheric ChemistRy Of the Suburban foreSt, conducted in Paris in summer 2022), particles' hygroscopic growth rates at 90 % relative humidity (RH) and chemical composition were measured at the sub-urban site using a Hygroscopicity Tandem Differential Mobility Analyser (HTDMA, scanning at 100, 150, 200, and 250 nm) and an Aerodyne High-Resolution Time-of-Flight Aerosol Mass Spectrometer (HR-ToF-AMS). Growth factor probability density functions (GF-PDFs) revealed two distinct modes, namely hydrophobic and hygroscopic, suggesting a combination of internal and external particle mixing, with the split at GF 1.2. The prevalence of the hygroscopic mode increased with particle size, with mean hygroscopicity (κ) values of 0.23 and 0.38 for 100 and 200 nm particles, respectively. Using the Zdanovskii–Stokes–Robinson (ZSR) mixing rule, the agreement between measured and chemically derived hygroscopicity was approximately 51% for 100 nm particles, which declined for 200 and 250 nm. These emphasise the large effect of external particle mixing and its influence on predicting hygroscopicity. The ZSR approach proves to be unreliable in predicting the wide growth distribution of externally mixed particles. In this measurement, 80 %–90 % of the particles were externally mixed and influenced by fresh emission, which affected the hygroscopicity prediction by a factor of 2. A cluster analysis based on backward trajectories and meteorological conditions gives valuable insights into the chemical composition and variations in the hygroscopicity of different air masses.
- Article
(4720 KB) - Full-text XML
-
Supplement
(2019 KB) - BibTeX
- EndNote
The atmospheric aerosol significantly impacts the Earth's radiation budget and climate on regional and global scales, exerting direct and indirect effects (IPCC, 2013; Rosenfeld et al., 2014; Li et al., 2016). Aerosols affect cloud formation through their hygroscopic properties and according to how they are incorporated into climate model simulations. Understanding these interactions is important for precise climate predictions. Hygroscopicity is the ability of particles to absorb water from the environment and is one of the essential physiochemical properties of aerosols. The hygroscopic growth of aerosols in the sub-saturate regime, where the ambient relative humidity is below 100 %, is crucial for discerning their interactions with water vapour in the atmosphere, influencing their size, shape, composition, and involvement in atmospheric processes. Aerosols absorb water and grow in size, impacting their optical properties and ability to form cloud droplets, influencing both direct and indirect radiative forcing (IPCC, 2007, 2021). These aerosol particles engage with solar radiation through absorption and scattering, contributing to positive or negative radiative forcing (Haywood and Boucher, 2000).
The hygroscopic growth of aerosols can be measured or estimated through direct and indirect techniques (Hegg et al., 2007; Achtert et al., 2009). The hygroscopic tandem differential mobility analyser (HTDMA) is used as a real-time direct measurement technique for fine-mode aerosols. It gives the growth factor (GF) of particles at a given dry particle diameter and relative humidity (RH). The hygroscopic parameter κ, a simplified model parameter representing the composition's dependence on the solution water activity, can be calculated from the measured GF (Petters and Kreidenweis, 2007). κ values for highly hygroscopic aerosols, such as sea salts and sulfates, range between 0.5 and 1.4, while non-hygroscopic aerosols, like soot, have values close to zero (Gysel et al., 2007; Bezantakos et al., 2013). A common approach to conducting long-term measurements of aerosol hygroscopicity is to use cloud condensation nuclei (CCN) counters (Paramonov et al., 2015; Schmale et al., 2018), which are quite popular but are used under supersaturated regimes. HTDMAs are employed for sub-saturated regimes. A limited number of long-term studies using the HTDMA technique have been published (Kammermann et al., 2010; Fors et al., 2011). These available studies give insights into the connection between particle hygroscopic growth, chemical composition, and the processes involved in their formation and transformation. HTDMA measurements can improve knowledge on water uptake and RH effects under non-cloud conditions for aerosols, and this can also be useful in improving the prediction of light absorption and scattering, which directly affect Earth's energy budget and climate. Hygroscopicity primarily depends on the aerosol size and chemical composition (Gysel et al., 2007; Gunthe et al., 2009).
The output of aerosol mass spectrometry (AMS) measurements gives detailed information on organic molecular fragments (Kanakidou et al., 2005) and the chemical composition of aerosol particles. Organic compounds constitute a substantial but variable fraction of atmospheric aerosols. In contrast to inorganic species, which exhibit well-characterised hygroscopic growth, our understanding of the water uptake of the organic aerosol fraction (which contributes 30 %–70 % of fine particles) is limited (Zhang et al., 2007; Hallquist et al., 2009). Note that AMS instruments can only detect particle material that can be volatilised at temperatures up to 600 °C (DeCarlo et al., 2006). Disregarding the non-detectable refractory fraction, which includes widely abundant elemental carbon and sea salt, may lead to a 25 %–40 % underprediction or overprediction of the hygroscopic growth factor in closure studies (Gysel et al., 2007; Wu et al., 2013b). The Aethalometer AE33 or MAAP instrument can measure undetected black carbon and can be incorporated in closure. A common practice in predicting hygroscopic growth involves utilising known hygroscopic growth factors of specific pure chemical species while assuming the Zdanovskii–Stokes–Robinson (ZSR) mixing rule, as proposed by Stokes and Robinson (1966) and Zdanovskii (1948). This rule has demonstrated its applicability mainly for the internally mixed particles when compared to directly measured growth factors of various atmospheric aerosols (Cubison et al., 2008; Petters et al., 2009; Wu et al., 2013b; Pöhlker et al., 2023). The climate-relevant characteristics of atmospheric aerosol particles are heavily influenced by their hygroscopicity and mixing state (Kaufman et al., 2002; McFiggans et al., 2006).
The hygroscopicity and aerosol mixing state are vital in comprehending aerosol interactions with the environment and various atmospheric processes. Ambient aerosol is commonly considered to be a heterogeneous mixture of particles and gases with diverse chemical compositions and sizes. It refers to an internal mixture of aerosol when the particles of the same size have a similar chemical composition (Spitieri et al., 2023). In contrast, an external mixture occurs when particles of the same size have distinctly different chemical compositions. Urban and sub-urban environments typically exhibit external mixtures from fresh local emissions and hygroscopic background aerosols, while marine environments tend to have highly hygroscopic aerosols (Massling et al., 2007; Swietlicki et al., 2008; Enroth et al., 2018; Wang et al., 2018).
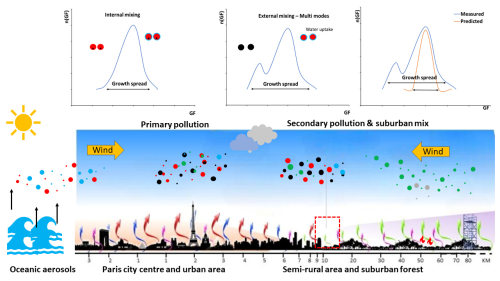
Figure 1Illustrative diagram showing the general behaviour of hygroscopicity and particle mixing, with the measurement site of the ACROSS 2022 campaign at LSCE-SIRTA shown in a dotted red box. Background pictures were sourced from the ACROSS campaign (ACROSS campaign, 2025a).
The hygroscopic properties of aerosols change continuously over the lifetime of the particles. This study provides size-resolved hygroscopicity data, which have not often been extensively measured. It aims to understand the ambient aerosol hygroscopic properties, along with the chemical composition; 1 month of measurements during the ACROSS ((Atmospheric ChemistRy Of the Suburban foreSt) campaign provides insights into microphysical parameters, including time- and size-resolved HTDMA data under sub-saturated conditions in a sub-urban environment, shown in Fig. 1. The upward arrows define the processes by which primary pollutants, such as oxides of nitrogen and volatile organic compounds (in red, pink, and blue colours), are emitted into the atmosphere, leading to their oxidation and ultimate removal while, at the same time, producing secondary species, such as ozone and organic aerosols (in green colours) over sub-urban to forest regions. The hygroscopicity of ambient aerosol was investigated in the particle size range between 100 to 250 nm, providing information about the hygroscopicity and the degree of the mixing state for selected particle sizes. The standard deviation or sigma (σ) of the growth factor derived from the HTDMA can explain the degree of the mixing of particles and the growth spread (Sjogren et al., 2008; Spitieri et al., 2023); the large σ tends to have multiple modes in the GF distribution and wide growth spread, which is associated with external mixing and vice versa for internally mixed particles shown in the top figure in the illustrative diagram. Atmospheric measurements were meticulously recorded during the field experiment at the site. Based on these data, our study provides a characterisation of particle physicochemical properties associated with mixing state and hygroscopic growth. The κ will inform the relationship between the measured and the chemically derived hygroscopicity. It can improve our understanding of aerosol growth in sub-saturated regimes and their role in the atmosphere, influencing their direct impact on the aerosol microstructure and optical properties.
2.1 Sampling site
Measurements used in this study were performed at the SIRTA observatory (Site Instrumental de Recherche par Télédétection Atmosphérique, http://sirta.ipsl.fr, last access: 24 September 2024), located approximately 23 km southwest of the city centre of Paris (Haeffelin et al., 2005) on the Saclay plateau (48.708° N, 2.148° E; 150 m a.s.l.). This “supersite” is surrounded by sub-urban facilities, forests, agricultural fields, and roads connecting Paris and has provided long-term, in situ observations of the atmospheric aerosol's chemical, optical, and physical properties since 2011 (Petit et al., 2015). It is part of the European Research Infrastructure for the observation of Aerosol, Clouds, and Trace gases, known as ACTRIS (Laj et al., 2024). Atmospheric composition measurements performed at SIRTA are considered to be representative of background conditions for the Paris region.
Regarding seasonal features, the summer periods frequently experience pollution episodes mainly related to forest fire and mobile sources (road traffic), and agricultural emissions at a regional scale, together with the transport of polluted air masses, are associated with mesoscale high-pressure systems (Petit et al., 2014). Standard meteorological parameters were recorded at the station, including temperature, humidity, wind speed, and wind direction. The meteorological sensors were positioned on a meteorological mast at a height of 10 m above ground level.
2.2 Hygroscopic growth measurements and data inversion
A custom-built humidified tandem differential mobility analyser (HTDMA) by TROPOS was used to measure the hygroscopic growth factor distributions of ambient aerosol particles (Massling et al., 2007; Wu et al., 2013b). The polydisperse aerosol was first dried by passing through a Nafion aerosol dryer and brought to charge equilibrium by an 85Kr bipolar neutraliser, shown in Fig. S1 in the Supplement. The selected dry diameters D0 for this campaign were located at specific narrow size fractions centred around 100, 150, 200, and 250 nm in a differential mobility analyser (DMA-1) and were exposed to a relative humidity (RH) of 90±3 % (Bezantakos et al., 2013; Wu et al., 2013b). Both DMAs were operated with a sheath flow rate of 5 L min−1 and a sample flow rate of ∼1 L min−1. It is to be noted that the variance of RH may have a measurable impact on GF, particularly with low-soluble compounds and their sensitivity to RH in this range. This could contribute to GF value uncertainties, especially in organics or less hygroscopic dominant species. Although the RH calibration was maintained carefully, this uncertainty is acknowledged to be a potential source of error in our measurements.
The hygroscopic growth factor (GF) determined by the HTDMA is the ratio of the particle mobility diameter Dp (RH) at a given RH to its dry diameter D0, as shown in Eq. (1):
The TDMAinv method developed by Gysel et al. (2009) was used to invert the data. During the entire duration of the field measurements, the GF of 100 nm pure ammonium sulfate particles was measured once every 4 h at 90 % RH to ensure high-quality data and thus to validate the accuracy and performance of the HTDMA in terms of RH (Gysel et al., 2002). Correspondingly, the relative uncertainty of around ±3 % for GFs of ammonium sulfate measurements is shown in Fig. S2. The residence time of particles at RH of 90 % before entering the DMA-2 is estimated to be approximately 2.5 s in the TROPOS-HTDMA system (Wu et al., 2013b). The short residence time could bring an additional bias into the measurements for the particles dominated by organics (Peng and Chan, 2001; Chan and Chan, 2005; Sjogren et al., 2007; Duplissy et al., 2009).
The GF standard deviation or sigma (σ) of a GF-PDF was determined according to Eq. (C.6) in Gysel et al. (2009). To assess the variability in growth factors and to characterise the mixing state, the sigma is employed as a metric for the spread of GF (σGF), as outlined by Sjogren et al. (2008). In the present study, the inverted data are categorised into two cases, representative of aerosol mixing states, as illustrated in Fig. S7. Specifically, σGF≤0.08 signifies an internally mixed aerosol (Fig. S7a), while σGF≥0.10 characterises an externally mixed aerosol with two distinct modes (Fig. S7b). This categorisation is used to identify the modes in GF-PDF for all scans for all diameters over time. The σGF not only describes the spread of single mode but also gives details of a broader distribution for bimodal GF spread. A brief explanation is provided in the Supplement.
2.3 Particle number size distributions (PNSDs)
The particle number size distributions (PNSDs) of ambient aerosol in its dry state were measured using a TROPOS-style MPSS (mobility particle size spectrometer), operated concurrently with the HTDMA and AMS at the SIRTA-LSCE station. It operated with a 5 min time resolution and an aerosol-to-sheath flow ratio of 1/5 L min−1, covering a particle size range from 10 to 850 nm. Both aerosol and sheath flows were dehydrated to a relative humidity lower than 40 % using a Nafion dryer. The uncertainty of this instrument's total particle number concentration was determined to be ±15 %.
2.4 Chemical composition and black-carbon measurement
The Aerodyne High-Resolution Time-of-Flight Aerosol Mass Spectrometer (HR-ToF-AMS, referred to as AMS) was typically operated with a time resolution of 2 min. The AMS, owing to the vaporiser's 600 °C surface temperature (DeCarlo et al., 2006), exclusively analyses the non-refractory chemical composition of particles, making it unable to detect soot, crustal material, and sea salt. Consequently, based on the transmission efficiency of the aerodynamic lens and the identified compounds, the AMS furnishes the chemical composition of the sub-micrometre non-refractory aerosol fraction at NR-PM1 (Canagaratna et al., 2007). Utilising the approach developed by Aiken et al. (2008) and improved by Canagaratna et al. (2015), high-resolution organic particle mass spectra were employed to determine the elemental composition and the oxygen-to-carbon (O : C) atomic ratio. The uncertainty of the chemical composition within the AMS varies for different chemical species, and it shows a reduced using calibration collection efficiency (CE) for the system. For these measurements, the ion CE was , and, considering this, the uncertainty for the chemical composition was roughly estimated to be 10 %–15 %.
The concentration of equivalent black carbon (eBC) was measured using a multi-wavelength aethalometer (AE33 model, Magee Scientific, 1 min time resolution, wavelength λ=880 nm) from Weingartner et al. (2003). For ambient measurements, uncertainties in BC concentrations using the AE33 can range from 5 % to 15 % (Drinovec et al., 2015). The uncertainty during this measurement was roughly 5 %. The PNSD data from MPSS are also used for data quality checks with AMS and BC measurements. The mass closure between both measurements agrees with the correlation (r=0.93), with a slope, as shown in Fig. S13.
2.5 The ZSR mixing rule
The hygroscopicity parameter (κ) can be computed using the hygroscopic growth factor (GF) measured through an HTDMA, based on Stokes and Robinson (1966) and outlined by Gysel et al. (2007).
Dpdry and GF are the initial dry particle diameter and hygroscopic growth factor at 90 % RH measured by the HTDMA. is the droplet surface tension (assumed to be that of pure water, N m−2), Mw is the molecular weight of water, ρw is the density of liquid water, R is the universal gas constant, and T is the absolute temperature.
Alternatively, κchem can be predicted using a simple mixing rule based on chemical volume fractions (Vfi), as proposed by Petters and Kreidenweis (2007):
Here, κi and Vfi are the hygroscopicity parameter and volume fraction for the individual (dry) component in the mixture, with i being the number of components in the mixture. We derive Vfi from the particle chemical composition measured by the AMS and AE33. The calculation of the volume fraction is described in detail in Sect. 2.6. Throughout subsequent discussions, κmeasured and κchem denote the kappa values derived from the HTDMA and AMS, as well as AE33 measurements. The composition in this study indicates the presence of both hygroscopic and hydrophobic compounds, which may affect the particle's overall hygroscopicity. The approach we used to predict hygroscopicity follows the Zdanovskii–Stokes–Robinson (ZSR) mixing rule. To some extent, we indirectly incorporate solubility and chemical composition information in κ calculations, particularly for particles with significant fractions of organics (more than 50 %). Hence, in ZSR-based κ predictions, the values of κi used in this study (see Table 1) are based on literature-measured values, with some water activity calculation. Changing κi in calculations does not change κchem prediction significantly as less soluble compounds like organics generally show only slight hygroscopic growth but a much better activity as a CCN than indicated by the hygroscopic growth, suggesting highly non-ideal behaviour for aerosol water contents at relative humidities of less than 98 % (Petters et al., 2009; Wex et al., 2009). The individual values of κi reflect literature-measured and theoretical predictions as cited in Table 1. In other words, ammonium nitrate, ammonium sulfate, and organics are based on empirical measurements. Considering the uncertainties in the HTDMA and AMS measurements, the uncertainty between κmeasured and κchem of 10 %–15 % (leading to an uncertainty of ±0.06 for κ) is roughly estimated, as shown in the Supplement.
2.6 Hygroscopicity–chemical composition closure
The Aerosol Mass Spectrometer (AMS) provides the particle mass concentrations, encompassing sulfate (SO4), nitrate (NO3), ammonium (NH4), and chloride (Cl) ions, as well as the concentration of organic compounds. A simplified ion-pairing scheme converts ion mass concentrations to the mass concentrations of their corresponding inorganic salts (Gysel et al., 2007).
2.7 Air mass trajectory cluster analysis
To determine air mass origin during specific pollution episodes, 72 h back trajectories were calculated on an hourly basis using the HYSPLIT Trajectory with GDAS meteorological field data (SplitR package, 2024) by Stein et al. (2015). Back trajectories were set to end at SIRTA z5 coordinates (48.70° N, 2.14° E) at 100 m above ground level (a.g.l.). The clustering analysis provides insights into the influence of different source regions and atmospheric processes on local air quality. It improves our understanding of aerosol transport mechanisms and their impact on the aerosol hygroscopic properties. The trajectory cluster analysis was performed using the openair package from R (Rstudio, version 4.4.1), which uses the angle method to get the cluster (Carslaw and Ropkins, 2012). The angle method of clustering is reliable and is beneficial for analysing the directional aspects of air mass transport, providing a better understanding of trajectory differences and enhancing the accuracy of clustering in trajectory analysis.
The optimal number of clusters was determined using the k-means elbow method, identifying five distinct clusters shown in Fig. S3. Subsequent analysis classified hygroscopicity growth and other parameters based on these clusters. The clusters were designated as C2, primarily influenced by continental air masses; C1, influenced by marine and continental air masses; and C3, C4, and C5, influenced by marine air masses traversing over land, as explained in Sect. 3.2.
3.1 Hygroscopicity and chemical composition overview
Figure 3 comprehensively represents the growth factor probability density function (GF-PDF) and chemical composition throughout the measurement campaign. The sub-micrometre bulk particle chemical composition was derived from AMS and AE33 measurements. As shown in Figure S5, the meteorological conditions for the initial period correspond to heatwave-1 and local PM emissions, followed by a later period characterised by cloudy and rainy conditions, leading to a reduction in concentration. During measurements, the temperature reached 40 °C from 17 to 19 June, referring to heatwave-1, whereas wind speed was 1 to 5 ms−1. The chemical composition during the entire measurement period indicates that organic matter accounted for approximately 60 % of the total, with sulfate emerging as the second-largest contributor. The OM : OC (organic mass-to-organic carbon) ratio experiences a decline coinciding with an eBC rise, indicating the influence of black carbon in the composition, as illustrated in Fig. S4, primarily associated with a nearby burning-plume event also seen in the mass fraction during 28 June. Figure 3a portrays the temporal evolution of AMS species and eBC. Elevated sulfate peaks observed on 4 and 11 July were attributed to transport events, either from industrial emissions in Rouen (northern side of Paris) or shipping emissions in the Channel–Le Havre region, influenced by northwestern winds. Throughout the campaign, transported air masses from the west, predominantly marine aerosols, were more influential, contributing to coarse aerosols such as sea spray and marine sulfates, as discussed in Sect. 3.2. The nitrate concentration was negligible during this measurement, and so ammonium nitrate loss due to evaporation may not apply here. The coarse-mode aerosol influence is also depicted in Fig. S4, showing the volume distribution from MPSS during the initial heatwave-1 period, which also coincides with a high PM mass being dominant, with larger particles in terms of volume. The GF-PDF illustrates a conspicuous size dependency and temporal variability in the hygroscopic growth of particles.
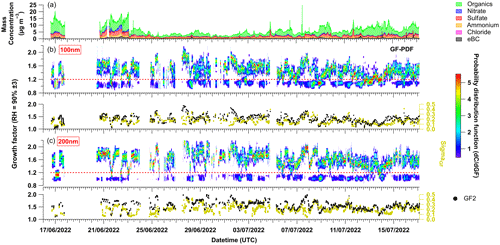
Figure 3Overview time series of chemical composition (a); size dependence of GF-PDF on GF2, which represents the mean growth factors of hygroscopic (GF >1.2) particles, with the dotted red line representing the cut-off between hydrophobic and hygroscopic modes for sizes of 100 and 200 nm, along with the sigma of the GF (σGF) (b–c).
In Fig. 3b–c, the time series includes two distinct diameter sizes, namely 100 and 200 nm, selected to represent particles of small and large sizes. Comparable analyses have been applied to other diameters, as detailed in the Supplement. The GF-PDFs show two distinct modes: the hydrophobic mode (GF <1.2) and hygroscopic mode (GF >1.2), also referred to as GF2 in Fig. 3b–c, signifying the prevalent mixing of aerosols. The colour scale represents the probability function (counts per GF), indicating the probability of a specific particle growth. A threshold value of GF 1.2 (dotted red line) is considered to be a cut-off line or differentiation between hydrophobic and hygroscopic particles for all sizes based on the mean of the observed GF-PDF distribution, where two modes are distinguished, representing a boundary where minimal water uptake occurs (Kim et al., 2020; Spitieri et al., 2023). This threshold GF coincides with the typical minimum in the observed GF-PDFs in this study, which also aligns with previous studies (Sjogren et al., 2008; Wu et al., 2013b). However, occasionally, the number fraction of the hygroscopic mode (F2 in Fig. S6) approaches unity for particle sizes exceeding 100 nm. The hygroscopic mode consistently predominates in the GF-PDF across all particle sizes. With increasing particle size, the prominence of the hygroscopic mode intensifies, accompanied by a decline in the hydrophobic fraction (F1 in Fig. S6). The hygroscopic-mode (F2) mean number fractions are 0.75, 0.82, 0.84, and 0.84 for 100, 150, 200, and 250 nm particle sizes, respectively. It was hypothesised that larger particles had undergone atmospheric ageing processes such as coagulation, condensation, chemical reaction, and cloud processing (Pöschl, 2005; Wu et al., 2013b) for longer than smaller particles. These ageing processes typically enhance the water solubility of particles (Pöschl, 2005; Jimenez et al., 2009a; Wu et al., 2013a). The σGF can be derived from data inversion of the HTDMA and can explain the degree of mixing of particles; the large σGF tends to have multiple modes in the GF distribution and a wide growth spread, which is associated with external mixing and vice versa for internally mixed particles (Sjogren et al., 2008; Spitieri et al., 2023). In the time series, the high GF is also associated with a large σGF, which is prominently seen for larger diameters of 200 nm. Larger σGF values are primarily associated with a heterogeneous mixture of particles with diverse hygroscopic properties. In some cases, such as on 29 June and 8 July, smaller particles grow as the organic mass fraction increases, but no significant change in GF is observed, as shown in PNSD in Fig. S4. This is hypothesised to be related to a new particle formation that needs further investigation as this is not the focus of this study. Correlating the volume fraction of chemical species with hygroscopicity will give a more general trend in terms of the contribution of each chemical component to the overall hygroscopic properties. It can quantify the variation of the water uptake capacity of aerosol.
3.1.1 Correlation between measured hygroscopicity and organic and inorganic volume fraction
The correlation between measured hygroscopicity (GF) and the organic volume fraction is illustrated in Fig. 4a, revealing a negative correlation between the two variables. The anti-correlation is evident as the hygroscopicity decreases with an increasing volume fraction of organics, which makes organics less hygroscopic, which also aligns with a previous study by Kamilli et al. (2014). The linear regression in Fig. 4a is evaluated as . Figure 4b shows the correlation of the volume fraction of the inorganic compounds with GF during the campaign. Here, inorganic compounds are the sum of all species from AMS measurements except organics, i.e. the sum of nitrate, sulfate, ammonium, and chloride. In contrast to the organic fraction, the inorganic compounds have a noticeable promoting effect on hygroscopic growth. The time series of the inorganic volume fraction and GF follow a similar pattern but with differing magnitudes, with a correlation of r=0.56. Here, the outliers are the highly hygroscopic events during the campaign period, which may influence the correlation.
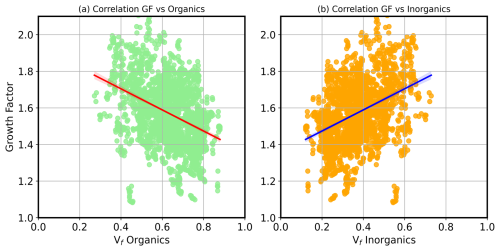
Figure 4Comparison between measured GF and volume fraction (Vf) of organics and inorganics for 200 nm.
The volume fractions (Vf) and their correlation with GF for different particle sizes help in understanding how the balance of organic and inorganic materials influences the overall hygroscopic behaviour. Vf shows their relevance for interpreting trends in measured GF and their application in κchem calculations, where they are explicitly used to represent the contribution of different chemical species. This linkage helps connect the measured hygroscopicity with chemical composition and highlights the importance of volume fractions in understanding the discrepancies between measured and predicted hygroscopicity. It can be further used in closure and trajectory cluster analysis.
3.2 Hygroscopic closure using PM1 bulk chemical composition and trajectory cluster analysis
In this section, hygroscopicity parameters are denoted as κmeasured and κchem. Corresponding κchem values are calculated using the ZSR mixing rule based on chemical composition. In principle, the values of both approaches agree quantitatively and are within the range of their uncertainty, and then closure is achieved (Gysel et al., 2007; Wu et al., 2013b).
κ is evaluated using Eqs. (2) and (4), representing measured and chemically derived values at 90 % RH. The variation of kappa over time is depicted in Fig. 5, primarily influenced by particle size and chemical composition. The κmeasured values for smaller particle sizes, specifically 100 and 150 nm, range between 0.24 and 0.29, as outlined in Table S1 in the Supplement. These values increase to 0.35 for larger sizes, particularly for accumulation mode particles of 200 nm. In Fig. 5, boxplots show the dataset overview and uncertainty, with the shaded region being the standard error on the line plot. It should be noted that the uncertainty involving instrument measurement and different corrections of the dataset (i.e. RH accuracy for the HTDMA or the volume fraction of different AMS species) was already explained in Sect. 2 and its subsections. The mean κ for each dataset, with the standard error as the uncertainty, is shown with the error bar in Fig. S12. It is hypothesised that the high hygroscopicity of accumulation mode particles is attributed to their comparatively larger surface area concentration and inherent water-absorbing capabilities (Liu et al., 2014).
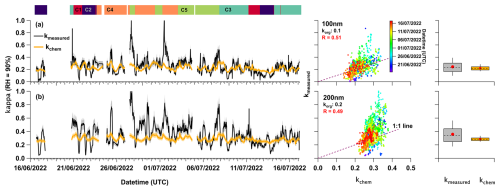
Figure 5(a–b) The time series and comparison of κmeasured and κchem with the correlation plot for 100 and 200 nm and clusters classification over time at the top. The boxplots represent the hygroscopic parameter kappa (κ) of the respective particle sizes for measured and chemically derived hygroscopicity, in which low and high whisker traces represent the 9th and 91st percentiles, respectively. The red marker indicates the average of the data, whereas the upper and lower sides of the boxes indicate the 75th and 25th percentiles of the data, respectively.
In most cases, κchem closely mirrors the trends observed in the direct κmeasured, barring a few outliers or instances of elevated hygroscopic peaks. These anomalies are occasionally linked to transported air masses from the northwest or southwest, potentially influenced by sea spray or highly hygroscopic salts non-detectable by the AMS and are further compounded by the lack of on-site measurements during the campaign. The notable hygroscopic peaks observed from late June to the first week of July are tentatively attributed to transported air masses carrying aerosols from the west to the southwest, as indicated by HYSPLIT Trajectory cluster analyses. We speculated that this is related to marine air masses with highly hygroscopic coarse aerosol particles like sea salt (NaCl) and sulfate salt or dimethyl sulfide (DMS). Marine air masses typically exhibit higher hygroscopicity than continental air masses (Huang et al., 2022).
The closure between measured and chemically derived hygroscopicity for particles of 100 nm, corresponding to the Aitken mode or smaller sizes, almost overlaps, except during high-peak events, correlating to approximately 51 %, as depicted in Fig. 5a. This congruence is not observed for larger sizes, which is speculated to arise from the uncertainty associated with the κchem value for externally mixed particles and the bimodal distribution of aerosols. The frequency of the bimodal distribution increases with particle size. Data segregation based on wind speed, direction, and high hygroscopicity did not make significant differences in correlation; the larger size correlations declined, as illustrated in Fig. S8. The κorg in chemically derived hygroscopicity is considered to be 0.1 and 0.2 for small and large particle sizes, respectively, as theoretically inspected through overlaps with measured hygroscopicity. κorg=0.2 for larger sizes of 200 and 250 nm is based on the slightly better correlation between κchem and κmeasured with the overlapping trend for time series. The κorg parameter represents the hygroscopicity of organic matter in aerosols. This parameter can vary (e.g. 0.1 to 0.2) based on factors like the type of organic compounds present. Some organic compounds may be more hygroscopic, corresponding to higher κorg values with a more oxygenated organic aerosol (OOA), while others may be less hygroscopic (Chang et al., 2010; Gunthe et al., 2011; Jimenez et al., 2009b; Petters and Kreidenweis, 2007). The nature of the organic matter and its interaction with water is based on factors like composition and environmental conditions, which are reflected in the differences between κorg values in ZSR calculations. The outliers in the κmeasured period reflect poor correlation, prompting trajectory analysis.
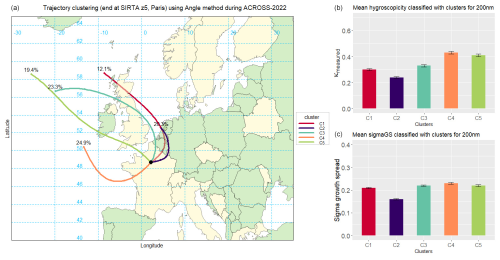
Figure 6(a) Trajectory cluster for the campaign period. (b) Mean bar plot of κmeasured and σGF spread, classified with clusters for 200 nm.
Trajectory cluster analysis was conducted to investigate the high-hygroscopicity events where discrepancies between κchem and κmeasured values were most pronounced. As depicted in Fig. 6a, five distinct trajectory clusters were identified: C1, C2, C3, C4, and C5. All clusters except C2 were generally influenced by marine air masses containing coarse particles, such as sea salt and dimethyl sulfide (DMS). Cluster C1, contributing 12.1 % of the trajectories, passed over land–sea regions, suggesting that these air masses spent significant time over continental areas, thereby introducing local pollutants mixed with coarse marine particles to the measurement site. Cluster C2, which contributed 20.3 % of the air mass, remained over the continent throughout its trajectory, originating from northwestern France, Belgium, and the Netherlands. This air mass passed over industrial regions, carrying local pollutants.
Clusters C3, C4, and C5 contributed 67.6 % of the trajectories, predominantly from marine regions. Specifically, C4 trajectories came from the southwest, with the air mass traversing southern France, likely transporting a mix of biogenic and oceanic aerosols to the site. Trajectories in C5 spent minimal time over the continent, predominantly carrying marine air masses and coarse particles. The parameters were classified according to the identified clusters to gain further insights into the variations in hygroscopicity and σGF, as illustrated in Fig. S9. These time series plots, presented as stacked bar charts, clearly show that high hygroscopic values were associated with C4 and C5 trajectories, which were influenced by coarse marine particles. Correspondingly, the σGF was also high for these trajectories, as seen in the mean values from the bar plot in Fig. 6b for 200 nm.
Notably, during a high-GF event on 27 June 2022, the air mass was primarily from C5, and a significant difference was observed between κchem and κmeasured. This discrepancy is likely to be due to the limitations of the AMS in measuring coarse or sea salt particles. Additionally, the lack of sea spray information in the chemical composition and the ZSR mixing rule prevented accurate prediction of hygroscopicity by κchem. Furthermore, the σGF during this event was 0.3, indicating the presence of multiple modes and externally mixed particles, which the ZSR mixing rule cannot account for.
Towards the end of the campaign, from 11 to 17 July 2022, the air masses were predominantly influenced by C1, C2, and C3, with a more substantial influence from local air masses, pollutants, and eBC. Since eBC is hydrophobic and has a low GF, the agreement between κchem and κmeasured during this period was close to the 1:1 line in Fig. 5b. In the ZSR mixing model, eBC is assumed to have a kappa value of zero, and the κmeasured during this period was around 0.4, indicating minimal influence from coarse particles on the predictions. The σGF for 100 nm particles during this time was less than 0.2, as shown in Fig. S9. This partially explains the stronger correlation between κchem and κmeasured for smaller diameters (e.g. 100 nm) compared to larger particle diameters (e.g. 200–250 nm). The presence of multiple modes and externally mixed particles changes the characteristics of aerosol and influences the prediction of hygroscopicity. To show the influence of the mixing state on hygroscopicity, the following analysis is described in Sect. 3.3.
3.3 Variability in GF-PDFs and particle mixing state influence
3.3.1 GF-PDF analysis from the ACROSS campaign
As depicted in Fig. 7, the mean GF-PDFs were computed by averaging individual GF-PDFs for each particle size with D0 of 100, 150, 200, and 250 nm over the entire campaign period. The mean GF-PDFs offer insights into the growth distribution, but they may not provide a clear depiction of the mixing state within these size fractions. However, the σGF, explained in earlier sections and seen as the widespread distribution of a GF of 1–2.2 on the x axis in Fig. 7, can give insights into the mixing state.
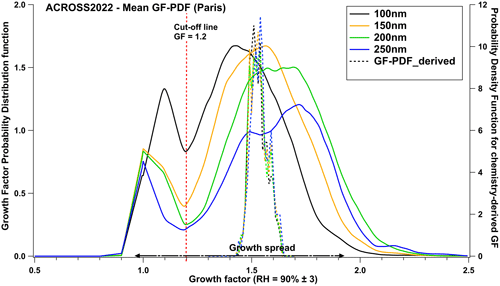
Figure 7The mean GF-PDFs for different diameters and the vertical red line represent the selected cut-off between the hydrophobic mode (GF <1.2) and the hygroscopic mode (GF >1.2).
The predicted GF-PDF is calculated using the corrected growth, as shown Eq. (2), used in the paper by Sjogren et al. (2008) and also mentioned in the Supplement. First, the calculated κchem from the ZSR mixing rule was used, and then the corresponding corrected growth at 90 % was calculated using the equation. After converting κchem to GFchem, the frequency distribution and the probability density function were calculated. This frequency distribution and probability function are calculated using the Microsoft Excel data analysis tool. The predicted GF-PDF is represented as dashed lines and the measured GF-PDF is represented as solid lines in Fig. 7. The mean measured GF-PDF shows a bimodal to multi-modal distribution, with a wide growth spread exhibiting the dominance of external mixing during the measurement period. In contrast, predicted GF-PDF derived from κchem presents a narrower growth spread and a mono-modal distribution, with a GF of 1.4–1.7. Whereas the chemical composition from the AMS is bulk without coarse particle information, it incorporates ZSR-based bulk aerosol hygroscopicity predictions that provide mean growth values exclusively and do not facilitate the identification of distinct growth modes, instead treating the ensemble as a perfect internal mixture. The measured GF-PDF incorporates all aerosol populations, giving a size-resolved GF and growth distribution. This can also influence the closure agreement. Furthermore, during clean conditions, slight variations in the average bulk inorganic ion mass fraction at a given diameter may lead to significant changes in the external mixing of the particle population. While this exerts a minor influence on the mean growth value, it significantly impacts the associated GF-PDF (Healy et al., 2014). A similar approach was performed when the correlation between κchem and κmeasured was better in the later part of the campaign from 13 to 17 July. However, no significant change was seen in GF-PDFs, except for a slightly narrower growth spread of the measured GF-PDF. To understand and get more insights into the mixing state and hygroscopicity of different diameters during the fresh emission, the diurnal pattern was compared with eBC properties. A similar comparison was also performed with data available on the measurement sites for other gases.
Figure 8 compares the diurnal patterns of κmeasured, σGF, and eBC properties. The discrimination of black carbon (eBC) into biomass burning (eBC_bb) and traffic fractions, employing the so-called aethalometer model (Drinovec et al., 2015; Sandradewi et al., 2008; Srivastava et al., 2019), revealed distinct behaviours about particle size. The eBC traffic exhibited a morning rush hour peak around 06:00 CET (Zhang et al., 2019), coinciding with a spike in σGF, suggesting an external mixing of particles with eBC. Furthermore, σGF follows the eBC traffic diurnal variation trend for 100 nm. In contrast, the κmeasured exhibits an opposing trend, aligning with the non-hygroscopic nature of eBC and its contribution to the particle mixture morphology. No significant trend relation was observed among the three parameters for accumulation mode particles of 200 nm. The contribution of the hygroscopic mode to total hygroscopicity exceeded that of 100 nm particles. Larger particles, typically more aged than their smaller counterparts, with higher hygroscopic growth (Cubison et al., 2006), are more closely associated with atmospheric processing during long-range transport (Kalivitis et al., 2015; Spitieri et al., 2023). This could further explain the 51 % agreement in closure for 100 nm particles, where mixing and eBC tend to be more homogeneous and limited to a bimodal distribution, unlike multiple modes for larger sizes.
3.3.2 Comparison with other measurement data
Another investigation was performed on a different dataset to assess further and to validate the closure study conducted during the ACROSS campaign. The data from the measurement campaign, which took place in Thuringia, central Germany, span from September to October 2010 and are part of the Hill Cap Cloud Thuringia (HCCT) at the Schmücke research site. The results and description of this study have been previously published by Wu et al. (2013b). Their closure study reported a better correlation between predicted and measured hygroscopicity, yielding correlation coefficients of 0.66 and 0.44 for larger and smaller particles, respectively. The analysis of similarly measured and predicted GF-PDF is presented in Fig. S10. Notably, the mean measured GF-PDFs exhibit a narrower growth spread and distinct modes, which overlap with predicted GF-PDFs. The comparable growth spread for measured and predicted suggests that aerosol particles during the HCCT campaign were aged and represented a well-distinguished mixture of compounds. Take note that Schmücke is a pristine and mountainous region with little variation in aerosol population, except for transportation events, and the measurement period was during winter, which will further support the better correlation of closure. As a consequence of our study, the influence of the mixing state in the prediction of hygroscopicity is seen. Further similar analysis should be done for different datasets to get insights into the influence of particulate mixing and to provide a threshold value for which the κchem derived using the classical ZSR mixing rule cannot be used. Due to the lack or unavailability of another dataset from Paris for comparison, the threshold value for κchem cannot be improved.
The mean GF-PDF and chemical composition during the ACROSS campaign suggest that the air mass was a mixture of complex compounds and externally mixed particles. Occasionally, aerosol source emissions are in close proximity. The external mixing is critical and has a complex composition of atmospheric aerosols. The role of the aerosol mixing state in determining climate-relevant properties such as CCN activity and aerosol optical characteristics (Riemer et al., 2019; Wang et al., 2020) needs advanced measurement techniques and modelling approaches, which may be computationally time-consuming and expensive. Using GF-PDFs to analyse aerosol mixing states adds a new perspective in terms of understanding how internal and external mixtures impact hygroscopic predictions. This complexity could contribute to the limited agreement in the closure study. Most studies referred to use the CCN measurements to derive κ, but mixing information is lacking in these measurements, whereas external mixing using growth spread can improve the understanding of hygroscopicity. The internally and externally mixed particles exhibit different water-uptake behaviours, and it is important to consider this when predicting aerosol hygroscopicity; it is suggested that using multiple complementary measurement techniques can provide a more comprehensive understanding of aerosol water uptake properties (Razafindrambinina et al., 2022). Our findings highlight the importance of external mixing, and an approach to growth spread in hygroscopicity can improve the modelling parameters. The inadequacy of the ZSR mixing rule is evident in polluted and mixed aerosol environments, challenging the classical method's applicability in hygroscopicity comparison and prediction. One must consider many assumptions to make it work. Similar studies should evaluate more deeply the knowledge on externally mixed particles and chemically derived hygroscopicity based on the classical ZSR mixing rule in different polluted environments and from different sources. This highlights the limitations of the ZSR mixing rule in predicting hygroscopic growth for externally mixed aerosols, which are common in urban to sub-urban areas affected by fresh emissions and which originate from various sources, including long-range transport and secondary organic aerosols. This approach gives a spectrum of size-resolved aerosol hygroscopic behaviour beyond bulk chemical composition. It can enhance the characterization of aerosol hygroscopicity in climate models as it directly influences the cloud microphysical processes and radiative forcing. This method can improve the accuracy of climate model simulations.
Aerosol hygroscopicity has been investigated for a peri-urban site in Paris using growth factor measurements by the HTDMA at a relative humidity of 90 %. Additionally, the ZSR mixing rule was used to predict hygroscopic growth factors based on the aerosol chemical composition measured by the AMS and AE33. The results indicate a pronounced size dependence of hygroscopic growth. During the measurement period, the median κ values of 100, 150, 200, and 250 nm particles derived from the HTDMA measurements were 0.24, 0.29, 0.35, and 0.36, respectively. Organics constitute approximately 60 % of the total chemical composition during the measurement period, with sulfate being the second-largest contributor at 20 %. The aerosol's mixing state is characterised based on the standard deviation or sigma (σ) of the inverted GF-PDF, distinguishing between internal (σGF<0.08) and external (σGF>0.10) mixing. The correlation between chemically derived and measured hygroscopicity is 51 % for smaller-sized particles, and the correlation declines for larger sizes. The temporal evolution of the predicted growth factor does not coincide well with the measured growth factor. Integrating chemical composition data with back trajectory clustering offers a novel approach to understanding how different air masses contribute to discrepancies between κmeasured and κchem values, particularly during highly hygroscopic events. Possible reasons for the limited agreement between measured and derived growth factors are as follows:
-
There are differences in GF-PDFs and growth spread.
-
Limitations of ZSR-based bulk aerosol hygroscopicity predictions provide mean GF values exclusively but do not distinguish GF modes.
-
The organic inputs of assigned compounds are not well-defined and are incorporated into the ZSR mixing rule computations with inaccuracies.
-
There are insufficient and ambiguous ion balance calculations and information on inorganic salts from marine air masses and size segregate chemical compositions. Size segregation can slightly improve the correlation.
However, it should be noted that the predicted GFs underestimate the measured GFs. These findings emphasise the need for caution when using such chemically derived hygroscopicity measurements in climate models, especially in regions with fresh aerosol emissions close to the source. The current results of combining hygroscopicity measurements with trajectory cluster analysis and κchem predictions represent a significant value in hygroscopicity prediction. Our findings align with previous studies highlighting the significant influence of the mixing state on aerosol hygroscopicity. Most referred studies use the CCN measurements to derive κ, but mixing information is lacking in these measurements. In contrast, external mixing using growth spread, especially in a sub-saturated regime, can improve the understanding of particle mixing in hygroscopicity prediction. The particle mixing significantly impacts global climate prediction, mainly based on local and regional models. Additionally, external mixing hygroscopicity is likely to impact aerosol-related health effects, such as lung deposition in a populated area where people typically live. These improve our understanding of aerosol growth in sub-saturated conditions and their broader impact on atmospheric processes, including aerosol microstructure and optical properties. The HTDMA data, however, can be further parameterised and utilised in machine learning to predict hygroscopicity using different models. The data can also be used as a proxy for predicting cloud condensation nuclei (CCN) or for κ predictions.
The trajectory clustering code and other processing toolkits can be made available upon request to the first author (deshmukh@tropos.de).
Level-2 datasets used in the present study from the ACROSS field campaign for the SIRTA site are available or will be made available soon through the AERIS data centre (https://across.aeris-data.fr/catalogue/, ACROSS campaign, 2025b). Also, data are available upon request to the author (deshmukh@tropos.de).
The supplement related to this article is available online at: https://doi.org/10.5194/acp-25-741-2025-supplement.
SD performed the formal analysis and wrote the original draft. SD and LP performed the investigation and data curation. JEP, LP, SD, and OF coordinated the ACROSS field campaign. SD, LP, PF, JEP, and OF contributed to the campaign setup, deployment and calibration and to the operation of the instrumentation, as well as to the data collection and analysis at the SIRTA site. SD, LP, MP, and SH provided the methodology and conceptualisation. MP and HH provided supervision and validation. LP, BW, JEP, and SH contributed to reviewing and editing of the paper. All the authors commented and contributed.
The contact author has declared that none of the authors has any competing interests.
Publisher’s note: Copernicus Publications remains neutral with regard to jurisdictional claims made in the text, published maps, institutional affiliations, or any other geographical representation in this paper. While Copernicus Publications makes every effort to include appropriate place names, the final responsibility lies with the authors.
This article is part of the special issue “Atmospheric Chemistry of the Suburban Forest – multiplatform observational campaign of the chemistry and physics of mixed urban and biogenic emissions”. It is not associated with a conference.
We acknowledge support from the SIRTA facility in conducting the measurements and for the collaboration in the ACROSS campaign. Support was received from other groups involved in the measurements, namely LSCE (Laboratoire des Sciences du Climat et de l'Environnement) and LAMP (Laboratoire de Météorologie Physique). We thank Etienne Brugere for supporting us in our measurements, as well as the members of the Institut National de l'Environnement Industriel et des Risques. The authors want to acknowledge support from TROPOS, the University of Leipzig, and PhD colleagues.
This research has been supported by the Deutsche Forschungsgemeinschaft (grant no. WE 2757/4-1). The ACROSS campaign has received funding from the French National Research Agency 475 (ANR) under the investment programme integrated into France 2030, with the reference no. ANR/17/MPGA/0002, and has been supported by the French national programme.
This paper was edited by Ann Fridlind and reviewed by three anonymous referees.
Achtert, P., Birmili, W., Nowak, A., Wehner, B., Wiedensohler, A., Takegawa, N., Kondo, Y., Miyazaki, Y., Hu, M., and Zhu, T.: Hygroscopic growth of tropospheric particle number size distributions over the North China Plain, J. Geophys. Res.-Atmos., 114, D00G07, https://doi.org/10.1029/2008JD010921, 2009.
ACROSS campaign: A description of the ACROSS project and its datasets, https://across.aeris-data.fr/description/, last access: 16 January 2025a.
ACROSS campaign: ACROSS field campaign data for the SIRTA site: AERIS data center, https://across.aeris-data.fr/catalogue, last access: 16 January 2025b.
Aiken, A. C., Decarlo, P. F., Kroll, J. H., Worsnop, D. R., Huffman, J. A., Docherty, K. S., Ulbrich, I. M., Mohr, C., Kimmel, J. R., Sueper, D., Sun, Y., Zhang, Q., Trimborn, A., Northway, M., Ziemann, P. J., Canagaratna, M. R., Onasch, T. B., Alfarra, M. R., Prevot, A. S. H., Dommen, J., Duplissy, J., Metzger, A., Baltensperger, U., and Jimenez, J. L.: O/C and OM/OC ratios of primary, secondary, and ambient organic aerosols with high-resolution time-of-flight aerosol mass spectrometry, Environ. Sci. Technol., 42, 4478–4485, https://doi.org/10.1021/es703009q, 2008.
Alfarra, M. R., Paulsen, D., Gysel, M., Garforth, A. A., Dommen, J., Prévôt, A. S. H., Worsnop, D. R., Baltensperger, U., and Coe, H.: A mass spectrometric study of secondary organic aerosols formed from the photooxidation of anthropogenic and biogenic precursors in a reaction chamber, Atmos. Chem. Phys., 6, 5279–5293, https://doi.org/10.5194/acp-6-5279-2006, 2006.
Bezantakos, S., Barmpounis, K., Giamarelou, M., Bossioli, E., Tombrou, M., Mihalopoulos, N., Eleftheriadis, K., Kalogiros, J., D. Allan, J., Bacak, A., Percival, C. J., Coe, H., and Biskos, G.: Chemical composition and hygroscopic properties of aerosol particles over the Aegean Sea, Atmos. Chem. Phys., 13, 11595–11608, https://doi.org/10.5194/acp-13-11595-2013, 2013.
Canagaratna, M. R., Jayne, J. T., Jimenez, J. L., Allan, J. D., Alfarra, M. R., Zhang, Q., Onasch, T. B., Drewnick, F., Coe, H., Middlebrook, A., Delia, A., Williams, L. R., Trimborn, A. M., Northway, M. J., DeCarlo, P. F., Kolb, C. E., Davidovits, P., and Worsnop, D. R.: Chemical and microphysical characterization of ambient aerosols with the aerodyne aerosol mass spectrometer, Mass. Spectrom. Rev., 26, 185–222, https://doi.org/10.1002/MAS.20115, 2007.
Canagaratna, M. R., Jimenez, J. L., Kroll, J. H., Chen, Q., Kessler, S. H., Massoli, P., Hildebrandt Ruiz, L., Fortner, E., Williams, L. R., Wilson, K. R., Surratt, J. D., Donahue, N. M., Jayne, J. T., and Worsnop, D. R.: Elemental ratio measurements of organic compounds using aerosol mass spectrometry: characterization, improved calibration, and implications, Atmos. Chem. Phys., 15, 253–272, https://doi.org/10.5194/acp-15-253-2015, 2015.
Carslaw, D. C. and Ropkins, K.: Openair – An r package for air quality data analysis, Environ. Model. Softw., 27–28, 52–61, https://doi.org/10.1016/J.ENVSOFT.2011.09.008, 2012.
Chan, M. N. and Chan, C. K.: Mass transfer effects in hygroscopic measurements of aerosol particles, Atmos. Chem. Phys., 5, 2703–2712, https://doi.org/10.5194/acp-5-2703-2005, 2005.
Chang, R. Y.-W., Slowik, J. G., Shantz, N. C., Vlasenko, A., Liggio, J., Sjostedt, S. J., Leaitch, W. R., and Abbatt, J. P. D.: The hygroscopicity parameter (κ) of ambient organic aerosol at a field site subject to biogenic and anthropogenic influences: relationship to degree of aerosol oxidation, Atmos. Chem. Phys., 10, 5047–5064, https://doi.org/10.5194/acp-10-5047-2010, 2010.
Cubison, M. J., Alfarra, M. R., Allan, J., Bower, K. N., Coe, H., McFiggans, G. B., Whitehead, J. D., Williams, P. I., Zhang, Q., Jimenez, J. L., Hopkins, J., and Lee, J.: The characterisation of pollution aerosol in a changing photochemical environment, Atmos. Chem. Phys., 6, 5573–5588, https://doi.org/10.5194/acp-6-5573-2006, 2006.
Cubison, M. J., Ervens, B., Feingold, G., Docherty, K. S., Ulbrich, I. M., Shields, L., Prather, K., Hering, S., and Jimenez, J. L.: The influence of chemical composition and mixing state of Los Angeles urban aerosol on CCN number and cloud properties, Atmos. Chem. Phys., 8, 5649–5667, https://doi.org/10.5194/acp-8-5649-2008, 2008.
DeCarlo, P. F., Kimmel, J. R., Trimborn, A., Northway, M. J., Jayne, J. T., Aiken, A. C., Gonin, M., Fuhrer, K., Horvath, T., Docherty, K. S., Worsnop, D. R., and Jimenez, J. L.: Field-deployable, high-resolution, time-of-flight aerosol mass spectrometer, Anal. Chem., 78, 8281–8289, https://doi.org/10.1021/AC061249N, 2006.
Dinar, E., Mentel, T. F., and Rudich, Y.: The density of humic acids and humic like substances (HULIS) from fresh and aged wood burning and pollution aerosol particles, Atmos. Chem. Phys., 6, 5213–5224, https://doi.org/10.5194/acp-6-5213-2006, 2006.
Drinovec, L., Močnik, G., Zotter, P., Prévôt, A. S. H., Ruckstuhl, C., Coz, E., Rupakheti, M., Sciare, J., Müller, T., Wiedensohler, A., and Hansen, A. D. A.: The ”dual-spot” Aethalometer: an improved measurement of aerosol black carbon with real-time loading compensation, Atmos. Meas. Tech., 8, 1965–1979, https://doi.org/10.5194/amt-8-1965-2015, 2015.
Duplissy, J., Gysel, M., Sjogren, S., Meyer, N., Good, N., Kammermann, L., Michaud, V., Weigel, R., Martins dos Santos, S., Gruening, C., Villani, P., Laj, P., Sellegri, K., Metzger, A., McFiggans, G. B., Wehrle, G., Richter, R., Dommen, J., Ristovski, Z., Baltensperger, U., and Weingartner, E.: Intercomparison study of six HTDMAs: results and recommendations, Atmos. Meas. Tech., 2, 363–378, https://doi.org/10.5194/amt-2-363-2009, 2009.
Enroth, J., Mikkilä, J., Németh, Z., Kulmala, M., and Salma, I.: Wintertime hygroscopicity and volatility of ambient urban aerosol particles, Atmos. Chem. Phys., 18, 4533–4548, https://doi.org/10.5194/acp-18-4533-2018, 2018.
Fors, E. O., Swietlicki, E., Svenningsson, B., Kristensson, A., Frank, G. P., and Sporre, M.: Hygroscopic properties of the ambient aerosol in southern Sweden – a two year study, Atmos. Chem. Phys., 11, 8343–8361, https://doi.org/10.5194/acp-11-8343-2011, 2011.
Gunthe, S. S., King, S. M., Rose, D., Chen, Q., Roldin, P., Farmer, D. K., Jimenez, J. L., Artaxo, P., Andreae, M. O., Martin, S. T., and Pöschl, U.: Cloud condensation nuclei in pristine tropical rainforest air of Amazonia: size-resolved measurements and modeling of atmospheric aerosol composition and CCN activity, Atmos. Chem. Phys., 9, 7551–7575, https://doi.org/10.5194/acp-9-7551-2009, 2009.
Gunthe, S. S., Rose, D., Su, H., Garland, R. M., Achtert, P., Nowak, A., Wiedensohler, A., Kuwata, M., Takegawa, N., Kondo, Y., Hu, M., Shao, M., Zhu, T., Andreae, M. O., and Pöschl, U.: Cloud condensation nuclei (CCN) from fresh and aged air pollution in the megacity region of Beijing, Atmos. Chem. Phys., 11, 11023–11039, https://doi.org/10.5194/acp-11-11023-2011, 2011.
Gysel, M., Weingartner, E., and Baltensperger, U.: Hygroscopicity of aerosol particles at low temperatures. 2. Theoretical and experimental hygroscopic properties of laboratory generated aerosols, Environ. Sci. Technol., 36, 63–68, https://doi.org/10.1021/ES010055G, 2002.
Gysel, M., Crosier, J., Topping, D. O., Whitehead, J. D., Bower, K. N., Cubison, M. J., Williams, P. I., Flynn, M. J., McFiggans, G. B., and Coe, H.: Closure study between chemical composition and hygroscopic growth of aerosol particles during TORCH2, Atmos. Chem. Phys., 7, 6131–6144, https://doi.org/10.5194/acp-7-6131-2007, 2007.
Gysel, M., McFiggans, G. B., and Coe, H.: Inversion of tandem differential mobility analyser (TDMA) measurements, J. Aerosol Sci., 40, 134–151, https://doi.org/10.1016/j.jaerosci.2008.07.013, 2009.
Gysel, M., Laborde, M., Olfert, J. S., Subramanian, R., and Gröhn, A. J.: Effective density of Aquadag and fullerene soot black carbon reference materials used for SP2 calibration, Atmos. Meas. Tech., 4, 2851–2858, https://doi.org/10.5194/amt-4-2851-2011, 2011.
Haeffelin, M., Barthès, L., Bock, O., Boitel, C., Bony, S., Bouniol, D., Chepfer, H., Chiriaco, M., Cuesta, J., Delanoë, J., Drobinski, P., Dufresne, J.-L., Flamant, C., Grall, M., Hodzic, A., Hourdin, F., Lapouge, F., Lemaître, Y., Mathieu, A., Morille, Y., Naud, C., Noël, V., O'Hirok, W., Pelon, J., Pietras, C., Protat, A., Romand, B., Scialom, G., and Vautard, R.: SIRTA, a ground-based atmospheric observatory for cloud and aerosol research, Ann. Geophys., 23, 253–275, https://doi.org/10.5194/angeo-23-253-2005, 2005.
Hallquist, M., Wenger, J. C., Baltensperger, U., Rudich, Y., Simpson, D., Claeys, M., Dommen, J., Donahue, N. M., George, C., Goldstein, A. H., Hamilton, J. F., Herrmann, H., Hoffmann, T., Iinuma, Y., Jang, M., Jenkin, M. E., Jimenez, J. L., Kiendler-Scharr, A., Maenhaut, W., McFiggans, G., Mentel, Th. F., Monod, A., Prévôt, A. S. H., Seinfeld, J. H., Surratt, J. D., Szmigielski, R., and Wildt, J.: The formation, properties and impact of secondary organic aerosol: current and emerging issues, Atmos. Chem. Phys., 9, 5155–5236, https://doi.org/10.5194/acp-9-5155-2009, 2009.
Haywood, J. and Boucher, O.: Estimates of the direct and indirect radiative forcing due to tropospheric aerosols: A review, Rev. Geophys., 38, 513–543, https://doi.org/10.1029/1999RG000078, 2000.
Healy, R. M., Evans, G. J., Murphy, M., Jurányi, Z., Tritscher, T., Laborde, M., Weingartner, E., Gysel, M., Poulain, L., Kamilli, K. A., Wiedensohler, A., O'Connor, I. P., McGillicuddy, E., Sodeau, J. R., and Wenger, J. C.: Predicting hygroscopic growth using single particle chemical composition estimates, J. Geophys. Res., 119, 9567–9577, https://doi.org/10.1002/2014JD021888, 2014.
Hegg, D. A., Covert, D. S., Jonsson, H., and Covert, P. A.: An Instrument for Measuring Size-Resolved Aerosol Hygroscopicity at both Sub- and Super-Micron Sizes, Aerosol Sci. Technol., 41, 873–883, https://doi.org/10.1080/02786820701506955, 2007.
Huang, S., Wu, Z., Wang, Y., Poulain, L., Höpner, F., Merkel, M., Herrmann, H., and Wiedensohler, A.: Aerosol Hygroscopicity and its Link to Chemical Composition in a Remote Marine Environment Based on Three Transatlantic Measurements, Environ. Sci. Technol., 56, 9613–9622, https://doi.org/10.1021/acs.est.2c00785, 2022.
IPCC: AR4 Climate Change 2007, Synthesis Report, https://www.ipcc.ch/report/ar4/syr/ (last access: 31 August 2024), 2007.
IPCC: AR5 Climate Change 2013, The Physical Science Basis, https://www.ipcc.ch/report/ar5/wg1/ (last access: 31 August 2024), 2013.
IPCC: Climate Change 2021, The Physical Science Basis | Climate Change 2021, The Physical Science Basis, https://www.ipcc.ch/report/ar6/wg1/ (last access: 31 August 2024), 2021.
Jimenez, J. L., Canagaratna, M. R., Donahue, N. M., Prevot, A. S. H., Zhang, Q., Kroll, J. H., DeCarlo, P. F., Allan, J. D., Coe, H., Ng, N. L., Aiken, A. C., Docherty, K. S., Ulbrich, I. M., Grieshop, A. P., Robinson, A. L., Duplissy, J., Smith, J. D., Wilson, K. R., Lanz, V. A., Hueglin, C., Sun, Y. L., Tian, J., Laaksonen, A., Raatikainen, T., Rautiainen, J., Vaattovaara, P., Ehn, M., Kulmala, M., Tomlinson, J. M., Collins, D. R., Cubison, M. J., Dunlea, E. J., Huffman, J. A., Onasch, T. B., Alfarra, M. R., Williams, P. I., Bower, K., Kondo, Y., Schneider, J., Drewnick, F., Borrmann, S., Weimer, S., Demerjian, K., Salcedo, D., Cottrell, L., Griffin, R., Takami, A., Miyoshi, T., Hatakeyama, S., Shimono, A., Sun, J. Y., Zhang, Y. M., Dzepina, K., Kimmel, J. R., Sueper, D., Jayne, J. T., Herndon, S. C., Trimborn, A. M., Williams, L. R., Wood, E. C., Middlebrook, A. M., Kolb, C. E., Baltensperger, U., and Worsnop, D. R.: Evolution of organic aerosols in the atmosphere, Science, 326, 1525–1529, https://doi.org/10.1126/science.1180353, 2009a.
Jimenez, J. L., Canagaratna, M. R., Donahue, N. M., Prevot, A. S. H., Zhang, Q., Kroll, J. H., DeCarlo, P. F., Allan, J. D., Coe, H., Ng, N. L., Aiken, A. C., Docherty, K. S., Ulbrich, I. M., Grieshop, A. P., Robinson, A. L., Duplissy, J., Smith, J. D., Wilson, K. R., Lanz, V. A., Hueglin, C., Sun, Y. L., Tian, J., Laaksonen, A., Raatikainen, T., Rautiainen, J., Vaattovaara, P., Ehn, M., Kulmala, M., Tomlinson, J. M., Collins, D. R., Cubison, M. J., Dunlea, E. J., Huffman, J. A., Onasch, T. B., Alfarra, M. R., Williams, P. I., Bower, K., Kondo, Y., Schneider, J., Drewnick, F., Borrmann, S., Weimer, S., Demerjian, K., Salcedo, D., Cottrell, L., Griffin, R., Takami, A., Miyoshi, T., Hatakeyama, S., Shimono, A., Sun, J. Y., Zhang, Y. M., Dzepina, K., Kimmel, J. R., Sueper, D., Jayne, J. T., Herndon, S. C., Trimborn, A. M., Williams, L. R., Wood, E. C., Middlebrook, A. M., Kolb, C. E., Baltensperger, U., and Worsnop, D. R.: Evolution of organic aerosols in the atmosphere, Science, 326, 1525–1529, https://doi.org/10.1126/SCIENCE.1180353, 2009b.
Kalivitis, N., Kerminen, V.-M., Kouvarakis, G., Stavroulas, I., Bougiatioti, A., Nenes, A., Manninen, H. E., Petäjä, T., Kulmala, M., and Mihalopoulos, N.: Atmospheric new particle formation as a source of CCN in the eastern Mediterranean marine boundary layer, Atmos. Chem. Phys., 15, 9203–9215, https://doi.org/10.5194/acp-15-9203-2015, 2015.
Kamilli, K. A., Poulain, L., Held, A., Nowak, A., Birmili, W., and Wiedensohler, A.: Hygroscopic properties of the Paris urban aerosol in relation to its chemical composition, Atmos. Chem. Phys., 14, 737–749, https://doi.org/10.5194/acp-14-737-2014, 2014.
Kammermann, L., Gysel, M., Weingartner, E., and Baltensperger, U.: 13-month climatology of the aerosol hygroscopicity at the free tropospheric site Jungfraujoch (3580 m a.s.l.), Atmos. Chem. Phys., 10, 10717–10732, https://doi.org/10.5194/acp-10-10717-2010, 2010.
Kanakidou, M., Seinfeld, J. H., Pandis, S. N., Barnes, I., Dentener, F. J., Facchini, M. C., Van Dingenen, R., Ervens, B., Nenes, A., Nielsen, C. J., Swietlicki, E., Putaud, J. P., Balkanski, Y., Fuzzi, S., Horth, J., Moortgat, G. K., Winterhalter, R., Myhre, C. E. L., Tsigaridis, K., Vignati, E., Stephanou, E. G., and Wilson, J.: Organic aerosol and global climate modelling: a review, Atmos. Chem. Phys., 5, 1053–1123, https://doi.org/10.5194/acp-5-1053-2005, 2005.
Kaufman, Y. J., Tanré, D., and Boucher, O.: A satellite view of aerosols in the climate system, Nature, 419, 215–223, https://doi.org/10.1038/NATURE01091, 2002.
Kim, N., Yum, S. S., Park, M., Park, J. S., Shin, H. J., and Ahn, J. Y.: Hygroscopicity of urban aerosols and its link to size-resolved chemical composition during spring and summer in Seoul, Korea, Atmos. Chem. Phys., 20, 11245–11262, https://doi.org/10.5194/acp-20-11245-2020, 2020.
Kondo, Y., Sahu, L., Moteki, N., Khan, F., Takegawa, N., Liu, X., Koike, M., and Miyakawa, T.: Consistency and Traceability of Black Carbon Measurements Made by Laser-Induced Incandescence, Thermal-Optical Transmittance, and Filter-Based Photo-Absorption Techniques, Aerosol Sci. Technol., 45, 295–312, https://doi.org/10.1080/02786826.2010.533215, 2011.
Laj, P., Myhre, C. L., Riffault, V., Amiridis, V., Fuchs, H., Eleftheriadis, K., Petäjä, T., Salameh, T., Kivekäs, N., Juurola, E., Saponaro, G., Philippin, S., Cornacchia, C., Arboledas, L. A., Baars, H., Claude, A., De Mazière, M., Dils, B., Dufresne, M., Evangeliou, N., Favez, O., Fiebig, M., Haeffelin, M., Herrmann, H., Höhler, K., Illmann, N., Kreuter, A., Ludewig, E., Marinou, E., Möhler, O., Mona, L., Murberg, L. E., Nicolae, D., Novelli, A., O'Connor, E., Ohneiser, K., Altieri, R. M. P., Picquet-Varrault, B., van Pinxteren, D., Pospichal, B., Putaud, J. P., Reimann, S., Siomos, N., Stachlewska, I., Tillmann, R., Voudouri, K. A., Wandinger, U., Wiedensohler, A., Apituley, A., Comerón, A., Gysel-Beer, M., Mihalopoulos, N., Nikolova, N., Pietruczuk, A., Sauvage, S., Sciare, J., Skov, H., Svendby, T., Swietlicki, E., Tonev, D., Vaughan, G., Zdimal, V., Baltensperger, U., Doussin, J. F., Kulmala, M., Pappalardo, G., Sundet, S. S., and Vana, M.: Aerosol, Clouds and Trace Gases Research Infrastructure (ACTRIS): The European Research Infrastructure Supporting Atmospheric Science, B. Am. Meteor. Soc., 105, E1098–E1136, https://doi.org/10.1175/BAMS-D-23-0064.1, 2024.
Li, Z., Lau, W. K. M., Ramanathan, V., Wu, G., Ding, Y., Manoj, M. G., Liu, J., Qian, Y., Li, J., Zhou, T., Fan, J., Rosenfeld, D., Ming, Y., Wang, Y., Huang, J., Wang, B., Xu, X., Lee, S. S., Cribb, M., Zhang, F., Yang, X., Zhao, C., Takemura, T., Wang, K., Xia, X., Yin, Y., Zhang, H., Guo, J., Zhai, P. M., Sugimoto, N., Babu, S. S., and Brasseur, G. P.: Aerosol and monsoon climate interactions over Asia, Rev. Geophys., 54, 866–929, https://doi.org/10.1002/2015RG000500, 2016.
Liu, H. J., Zhao, C. S., Nekat, B., Ma, N., Wiedensohler, A., van Pinxteren, D., Spindler, G., Müller, K., and Herrmann, H.: Aerosol hygroscopicity derived from size-segregated chemical composition and its parameterization in the North China Plain, Atmos. Chem. Phys., 14, 2525–2539, https://doi.org/10.5194/acp-14-2525-2014, 2014.
Massling, A., Leinert, S., Wiedensohler, A., and Covert, D.: Hygroscopic growth of sub-micrometer and one-micrometer aerosol particles measured during ACE-Asia, Atmos. Chem. Phys., 7, 3249–3259, https://doi.org/10.5194/acp-7-3249-2007, 2007.
McFiggans, G., Artaxo, P., Baltensperger, U., Coe, H., Facchini, M. C., Feingold, G., Fuzzi, S., Gysel, M., Laaksonen, A., Lohmann, U., Mentel, T. F., Murphy, D. M., O'Dowd, C. D., Snider, J. R., and Weingartner, E.: The effect of physical and chemical aerosol properties on warm cloud droplet activation, Atmos. Chem. Phys., 6, 2593–2649, https://doi.org/10.5194/acp-6-2593-2006, 2006.
Paramonov, M., Kerminen, V.-M., Gysel, M., Aalto, P. P., Andreae, M. O., Asmi, E., Baltensperger, U., Bougiatioti, A., Brus, D., Frank, G. P., Good, N., Gunthe, S. S., Hao, L., Irwin, M., Jaatinen, A., Jurányi, Z., King, S. M., Kortelainen, A., Kristensson, A., Lihavainen, H., Kulmala, M., Lohmann, U., Martin, S. T., McFiggans, G., Mihalopoulos, N., Nenes, A., O'Dowd, C. D., Ovadnevaite, J., Petäjä, T., Pöschl, U., Roberts, G. C., Rose, D., Svenningsson, B., Swietlicki, E., Weingartner, E., Whitehead, J., Wiedensohler, A., Wittbom, C., and Sierau, B.: A synthesis of cloud condensation nuclei counter (CCNC) measurements within the EUCAARI network, Atmos. Chem. Phys., 15, 12211–12229, https://doi.org/10.5194/acp-15-12211-2015, 2015.
Park, K., Kittelson, D. B., Zachariah, M. R., and McMurry, P. H.: Measurement of inherent material density of nanoparticle agglomerates, J. Nanopart. Res., 6, 267–272, https://doi.org/10.1023/B:NANO.0000034657.71309.e6, 2004.
Peng, C. and Chan, C. K.: The water cycles of water-soluble organic salts of atmospheric importance, Atmos. Environ., 35, 1183–1192, https://doi.org/10.1016/S1352-2310(00)00426-X, 2001.
Petit, J.-E., Favez, O., Sciare, J., Canonaco, F., Croteau, P., Močnik, G., Jayne, J., Worsnop, D., and Leoz-Garziandia, E.: Submicron aerosol source apportionment of wintertime pollution in Paris, France by double positive matrix factorization (PMF2) using an aerosol chemical speciation monitor (ACSM) and a multi-wavelength Aethalometer, Atmos. Chem. Phys., 14, 13773–13787, https://doi.org/10.5194/acp-14-13773-2014, 2014.
Petit, J.-E., Favez, O., Sciare, J., Crenn, V., Sarda-Estève, R., Bonnaire, N., Močnik, G., Dupont, J.-C., Haeffelin, M., and Leoz-Garziandia, E.: Two years of near real-time chemical composition of submicron aerosols in the region of Paris using an Aerosol Chemical Speciation Monitor (ACSM) and a multi-wavelength Aethalometer, Atmos. Chem. Phys., 15, 2985–3005, https://doi.org/10.5194/acp-15-2985-2015, 2015.
Petters, M. D. and Kreidenweis, S. M.: A single parameter representation of hygroscopic growth and cloud condensation nucleus activity, Atmos. Chem. Phys., 7, 1961–1971, https://doi.org/10.5194/acp-7-1961-2007, 2007.
Petters, M. D., Wex, H., Carrico, C. M., Hallbauer, E., Massling, A., McMeeking, G. R., Poulain, L., Wu, Z., Kreidenweis, S. M., and Stratmann, F.: Towards closing the gap between hygroscopic growth and activation for secondary organic aerosol – Part 2: Theoretical approaches, Atmos. Chem. Phys., 9, 3999–4009, https://doi.org/10.5194/acp-9-3999-2009, 2009.
Pöhlker, M. L., Pöhlker, C., Quaas, J., Mülmenstädt, J., Pozzer, A., Andreae, M. O., Artaxo, P., Block, K., Coe, H., Ervens, B., Gallimore, P., Gaston, C. J., Gunthe, S. S., Henning, S., Herrmann, H., Krüger, O. O., McFiggans, G., Poulain, L., Raj, S. S., Reyes-Villegas, E., Royer, H. M., Walter, D., Wang, Y., and Pöschl, U.: Global organic and inorganic aerosol hygroscopicity and its effect on radiative forcing, Nat. Commun., 14, 6139, https://doi.org/10.1038/s41467-023-41695-8, 2023.
Pöschl, U.: Atmospheric aerosols: Composition, transformation, climate and health effects, Angew. Chem. Int. Edit., 44, 7520–7541, https://doi.org/10.1002/anie.200501122, 2005.
Razafindrambinina, P. N., Malek, K. A., DiMonte, K., Dawson, J. N., Raymond, T. M., Dutcher, D. D., Freedman, M. A., and Asa-Awuku, A. A.: Effects of mixing state on water-uptake properties of ammonium sulfate – Organic mixtures, Aerosol Sci. Technol., 56, 1009–1021, https://doi.org/10.1080/02786826.2022.2114313, 2022.
Riemer, N., Ault, A. P., West, M., Craig, R. L., and Curtis, J. H.: Aerosol Mixing State: Measurements, Modeling, and Impacts, Rev. Geophys., 57, 187–249, https://doi.org/10.1029/2018RG000615, 2019.
Rosenfeld, D., Andreae, M. O., Asmi, A., Chin, M., De Leeuw, G., Donovan, D. P., Kahn, R., Kinne, S., Kivekäs, N., Kulmala, M., Lau, W., Schmidt, K. S., Suni, T., Wagner, T., Wild, M., and Quaas, J.: Global observations of aerosol-cloud-precipitation-climate interactions, Rev. Geophys., 52, 750–808, https://doi.org/10.1002/2013RG000441, 2014.
Sandradewi, J., Prévôt, A. S. H., Szidat, S., Perron, N., Alfarra, M. R., Lanz, V. A., Weingartner, E., and Baltensperger, U. R. S.: Using aerosol light abosrption measurements for the quantitative determination of wood burning and traffic emission contribution to particulate matter, Environ. Sci. Technol., 42, 3316–3323, https://doi.org/10.1021/es702253m, 2008.
Schmale, J., Henning, S., Decesari, S., Henzing, B., Keskinen, H., Sellegri, K., Ovadnevaite, J., Pöhlker, M. L., Brito, J., Bougiatioti, A., Kristensson, A., Kalivitis, N., Stavroulas, I., Carbone, S., Jefferson, A., Park, M., Schlag, P., Iwamoto, Y., Aalto, P., Äijälä, M., Bukowiecki, N., Ehn, M., Frank, G., Fröhlich, R., Frumau, A., Herrmann, E., Herrmann, H., Holzinger, R., Kos, G., Kulmala, M., Mihalopoulos, N., Nenes, A., O'Dowd, C., Petäjä, T., Picard, D., Pöhlker, C., Pöschl, U., Poulain, L., Prévôt, A. S. H., Swietlicki, E., Andreae, M. O., Artaxo, P., Wiedensohler, A., Ogren, J., Matsuki, A., Yum, S. S., Stratmann, F., Baltensperger, U., and Gysel, M.: Long-term cloud condensation nuclei number concentration, particle number size distribution and chemical composition measurements at regionally representative observatories, Atmos. Chem. Phys., 18, 2853–2881, https://doi.org/10.5194/acp-18-2853-2018, 2018.
Sjogren, S., Gysel, M., Weingartner, E., Baltensperger, U., Cubison, M. J., Coe, H., Zardini, A. A., Marcolli, C., Krieger, U. K., and Peter, T.: Hygroscopic growth and water uptake kinetics of two-phase aerosol particles consisting of ammonium sulfate, adipic and humic acid mixtures, J. Aerosol Sci., 38, 157–171, https://doi.org/10.1016/J.JAEROSCI.2006.11.005, 2007.
Sjogren, S., Gysel, M., Weingartner, E., Alfarra, M. R., Duplissy, J., Cozic, J., Crosier, J., Coe, H., and Baltensperger, U.: Hygroscopicity of the submicrometer aerosol at the high-alpine site Jungfraujoch, 3580 m a.s.l., Switzerland, Atmos. Chem. Phys., 8, 5715–5729, https://doi.org/10.5194/acp-8-5715-2008, 2008.
Spitieri, C., Gini, M., Gysel-Beer, M., and Eleftheriadis, K.: Annual cycle of hygroscopic properties and mixing state of the suburban aerosol in Athens, Greece, Atmos. Chem. Phys., 23, 235–249, https://doi.org/10.5194/acp-23-235-2023, 2023.
SplitR package: RDocumentation, https://www.rdocumentation.org/packages/SplitR/versions/0.3, last access: 24 September 2024.
Srivastava, D., Favez, O., Petit, J. E., Zhang, Y., Sofowote, U. M., Hopke, P. K., Bonnaire, N., Perraudin, E., Gros, V., Villenave, E., and Albinet, A.: Speciation of organic fractions does matter for aerosol source apportionment. Part 3: Combining off-line and on-line measurements, Sci. Total Environ., 690, 944–955, https://doi.org/10.1016/j.scitotenv.2019.06.378, 2019.
Stein, A. F., Draxler, R. R., Rolph, G. D., Stunder, B. J. B., Cohen, M. D., and Ngan, F.: Noaa's hysplit atmospheric transport and dispersion modeling system, B. Am. Meteor. Soc., 96, 2059–2077, https://doi.org/10.1175/BAMS-D-14-00110.1, 2015.
Stokes, R. H. and Robinson, R. A.: Interactions in aqueous nonelectrolyte solutions. I. Solute-solvent equilibria, J. Phys. Chem., 70, 2126–2131, https://doi.org/10.1021/j100879a010, 1966.
Swietlicki, E., Hansson, H. C., Hämeri, K., Svenningsson, B., Massling, A., Mcfiggans, G., Mcmurry, P. H., Petäjä, T., Tunved, P., Gysel, M., Topping, D., Weingartner, E., Baltensperger, U., Rissler, J., Wiedensohler, A., and Kulmala, M.: Hygroscopic properties of submicrometer atmospheric aerosol particles measured with H-TDMA instruments in various environments—a review, Tellus B, 60 B, 432–469, https://doi.org/10.1111/J.1600-0889.2008.00350.X, 2008.
Wang, X., Ye, X., Chen, J., Wang, X., Yang, X., Fu, T.-M., Zhu, L., and Liu, C.: Direct links between hygroscopicity and mixing state of ambient aerosols: estimating particle hygroscopicity from their single-particle mass spectra, Atmos. Chem. Phys., 20, 6273–6290, https://doi.org/10.5194/acp-20-6273-2020, 2020.
Wang, Y., Li, Z., Zhang, Y., Du, W., Zhang, F., Tan, H., Xu, H., Fan, T., Jin, X., Fan, X., Dong, Z., Wang, Q., and Sun, Y.: Characterization of aerosol hygroscopicity, mixing state, and CCN activity at a suburban site in the central North China Plain, Atmos. Chem. Phys., 18, 11739–11752, https://doi.org/10.5194/acp-18-11739-2018, 2018.
Weingartner, E., Saathoff, H., Schnaiter, M., Streit, N., Bitnar, B., and Baltensperger, U.: Absorption of light by soot particles: determination of the absorption coefficient by means of aethalometers, J. Aerosol Sci., 34, 1445–1463, https://doi.org/10.1016/S0021-8502(03)00359-8, 2003.
Wex, H., Petters, M. D., Carrico, C. M., Hallbauer, E., Massling, A., McMeeking, G. R., Poulain, L., Wu, Z., Kreidenweis, S. M., and Stratmann, F.: Towards closing the gap between hygroscopic growth and activation for secondary organic aerosol: Part 1 – Evidence from measurements, Atmos. Chem. Phys., 9, 3987–3997, https://doi.org/10.5194/acp-9-3987-2009, 2009.
Wu, Z., Birmili, W., Poulain, L., Wang, Z., Merkel, M., Fahlbusch, B., van Pinxteren, D., Herrmann, H., and Wiedensohler, A.: Particle hygroscopicity during atmospheric new particle formation events: implications for the chemical species contributing to particle growth, Atmos. Chem. Phys., 13, 6637–6646, https://doi.org/10.5194/acp-13-6637-2013, 2013a.
Wu, Z. J., Poulain, L., Henning, S., Dieckmann, K., Birmili, W., Merkel, M., van Pinxteren, D., Spindler, G., Müller, K., Stratmann, F., Herrmann, H., and Wiedensohler, A.: Relating particle hygroscopicity and CCN activity to chemical composition during the HCCT-2010 field campaign, Atmos. Chem. Phys., 13, 7983–7996, https://doi.org/10.5194/acp-13-7983-2013, 2013b.
Zdanovskii, B.: Novyi metod rascheta rastvorimostei elektrolitov v mnogokomponentnykh sistema, Zh. Fiz. Khim+, 22, 1478–1485, 1486–1495, 1948.
Zhang, Q., Jimenez, J. L., Canagaratna, M. R., Allan, J. D., Coe, H., Ulbrich, I., Alfarra, M. R., Takami, A., Middlebrook, A. M., Sun, Y. L., Dzepina, K., Dunlea, E., Docherty, K., DeCarlo, P. F., Salcedo, D., Onasch, T., Jayne, J. T., Miyoshi, T., Shimono, A., Hatakeyama, S., Takegawa, N., Kondo, Y., Schneider, J., Drewnick, F., Borrmann, S., Weimer, S., Demerjian, K., Williams, P., Bower, K., Bahreini, R., Cottrell, L., Griffin, R. J., Rautiainen, J., Sun, J. Y., Zhang, Y. M., and Worsnop, D. R.: Ubiquity and dominance of oxygenated species in organic aerosols in anthropogenically-influenced Northern Hemisphere midlatitudes, Geophys. Res. Lett., 34, L13801, https://doi.org/10.1029/2007GL029979, 2007.
Zhang, Y., Favez, O., Petit, J.-E., Canonaco, F., Truong, F., Bonnaire, N., Crenn, V., Amodeo, T., Prévôt, A. S. H., Sciare, J., Gros, V., and Albinet, A.: Six-year source apportionment of submicron organic aerosols from near-continuous highly time-resolved measurements at SIRTA (Paris area, France), Atmos. Chem. Phys., 19, 14755–14776, https://doi.org/10.5194/acp-19-14755-2019, 2019.