the Creative Commons Attribution 4.0 License.
the Creative Commons Attribution 4.0 License.
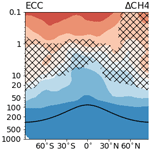
Chemistry–climate feedback of atmospheric methane in a methane-emission-flux-driven chemistry–climate model
Franziska Winterstein
Patrick Jöckel
Michael Ponater
Mariano Mertens
Martin Dameris
The chemical sink of atmospheric methane (CH4) depends on the temperature and on the chemical composition. Here, we assess the feedback on atmospheric CH4 induced by changes in the chemical sink in a warming climate using a CH4-emission-flux-driven set-up of the chemistry–climate model EMAC (ECHAM/MESSy Atmospheric Chemistry), in which the chemical feedback of CH4 mixing ratios can evolve explicitly. We perform idealized perturbation simulations driven either by increased carbon dioxide (CO2) mixing ratios or by increased CH4 emission fluxes. The CH4 emission flux perturbation leads to a large increase of CH4 mixing ratios. Remarkably, the factor by which the CH4 mixing ratio increases is larger than the increase factor of the emission flux, because the atmospheric lifetime of CH4 is extended.
In contrast, the individual effect of the global surface air temperature (GSAT) increase is to shorten the CH4 lifetime, which results in a significant reduction of CH4 mixing ratios in our set-up. The corresponding radiative feedback is estimated at −0.041 and −0.089 W m−2 K−1 for the CO2 and CH4 perturbation, respectively. The explicit response of CH4 mixing ratios leads to secondary feedbacks on the hydroxyl radical (OH) and ozone (O3). Firstly, the OH response includes the CH4–OH feedback, which enhances the CH4 lifetime change, and, secondly, the formation of tropospheric O3 is reduced. Our CH4 perturbation induces the same response of GSAT per effective radiative forcing (ERF) as the CO2 perturbation, which supports the applicability of the ERF framework for CH4.
- Article
(6754 KB) - Full-text XML
-
Supplement
(3771 KB) - BibTeX
- EndNote
Methane (CH4) is, after carbon dioxide (CO2), the second most important anthropogenic greenhouse gas (GHG). Compared to CO2, CH4 has a larger radiative efficiency (Forster et al., 2021) and a shorter atmospheric lifetime of about 10 years (e.g. Prather et al., 2012; Stevenson et al., 2020). Therefore, reducing atmospheric CH4 mixing ratios is considered an important measure to mitigate climate change on a decadal timescale (Saunois et al., 2016; Collins et al., 2018; Ocko et al., 2021; Staniaszek et al., 2022). The relatively short atmospheric lifetime of CH4 is a consequence of the fact that CH4 is a chemically active species. According to Saunois et al. (2020), the most important sink of atmospheric CH4 is oxidation by the hydroxyl radical (OH). Thus, understanding the chemical mechanisms underlying CH4 oxidation is crucial when assessing its climate impact and mitigation options.
Besides its direct radiative effect, indirect contributions from ozone (O3) and stratospheric water vapour (H2O) enhance the effective radiative forcing (ERF) of CH4 (Shindell et al., 2005, 2009; Stevenson et al., 2013; Winterstein et al., 2019; Thornhill et al., 2021b; O'Connor et al., 2022). In addition to its climate impact, tropospheric O3 poses harmful effects on human health (Nuvolone et al., 2018) and on vegetation (Ashmore, 2005). Therefore, mitigation options involving CH4 emission reductions have beneficial effects on air quality (Shindell et al., 2012; Staniaszek et al., 2022) and plant productivity (Sitch et al., 2007). In addition to the effects on O3 and H2O, CH4 oxidation reduces OH, which feeds back onto its own atmospheric lifetime (e.g. Winterstein et al., 2019) and affects the rate of formation of secondary aerosols, leading to a shift in the aerosol-size distribution. The latter, in turn, influences aerosol–radiation interactions and aerosol–cloud interactions and is considered another indirect contribution to the ERF of CH4 (Kurtén et al., 2011; O'Connor et al., 2022).
Next to its importance for indirect contributions to the ERF, CH4 oxidation largely constrains the atmospheric lifetime of CH4 and, thus, together with the magnitude of the emissions, its direct radiative effect. The atmospheric lifetime of CH4 is not constant but depends on the temperature and on the chemical background, which determines the abundance of its sink reactants, especially OH. OH is influenced by a multitude of factors (e.g. Voulgarakis et al., 2013; Stevenson et al., 2020). Among others, meteorological factors such as humidity and temperature influence the abundance of OH. Hence, climate feedbacks of the chemical sink of CH4 and thereby its lifetime are to be expected. More precisely, the CH4 lifetime is projected to shorten as a result of tropospheric warming (Voulgarakis et al., 2013; Stecher et al., 2021; Heimann et al., 2020; Thornhill et al., 2021a). To date, only a limited number of studies have assessed the corresponding response of CH4 mixing ratios directly (Heimann et al., 2020). Even though efforts are ongoing to employ chemistry–climate models (CCMs) in a CH4-emission-flux-driven model set-up (Shindell et al., 2013; He et al., 2020; Folberth et al., 2022), it is still common practice to prescribe CH4 mixing ratios at the lower boundary. For instance, the latter method was pursued in the Aerosol Chemistry Model Intercomparison Project (AerChemMIP; Collins et al., 2017), which was endorsed in the Coupled Model Intercomparison Project 6 (CMIP6; Eyring et al., 2016), which, in turn, built the basis for the last report of the Intergovernmental Panel on Climate Change (IPCC, 2021).
When CH4 mixing ratios are prescribed at the surface, the CH4 adjustment to changes in the chemical sink is suppressed in the troposphere and can be only derived offline from the atmospheric lifetime change (Dietmüller et al., 2014; Heinze et al., 2019; Thornhill et al., 2021a). This offline method, however, suppresses indirect feedbacks induced by the CH4 response. Firstly, when CH4 mixing ratios are free to evolve, the resulting CH4 response alters the atmospheric CH4 lifetime, which in turn leads to further changes in the CH4 mixing ratios. The derivation of the CH4 response from the lifetime change usually accounts for this effect by including a constant CH4–OH feedback factor f (Heinze et al., 2019; Thornhill et al., 2021a) so that the equilibrium CH4 mixing ratio [CH4]eq is estimated as (e.g. Stevenson et al., 2020)
where [CH4]ref is the reference CH4 mixing ratio and τref and τexp are the reference and perturbed atmospheric lifetimes of CH4, respectively. Estimates of f are in the range of 1.19 to 1.55 (Fiore et al., 2009; Voulgarakis et al., 2013; Stevenson et al., 2013; Thornhill et al., 2021b; Stevenson et al., 2020; Sand et al., 2023). Holmes (2018) found that f can vary geographically and seasonally and that it strengthens with an increasing CH4 burden. Secondly, the subsequent CH4 response affects other chemical constituents such as O3. This effect is also sometimes accounted for by scaling the sensitivity of O3 towards CH4 perturbations with the expected CH4 response (Fiore et al., 2009; Thornhill et al., 2021b). However, previous studies that assessed the climate feedback of O3 and the corresponding implication for the global surface air temperature (GSAT) response did not, or only rudimentarily, account for the interaction between changes in O3 and CH4 (Dietmüller et al., 2014; Nowack et al., 2015; Marsh et al., 2016; Li and Newman, 2023). As for O3 alone, the latter studies indicate that its feedback reduces the resulting GSAT response, or in other words that it is a negative feedback. The magnitude of this feedback, however, is highly model dependent.
In this study, we use a CH4-emission-flux-driven set-up of the CCM ECHAM/MESSy Atmospheric Chemistry (EMAC; Jöckel et al., 2016) to explicitly simulate the response of atmospheric CH4 mixing ratios resulting from changes in the chemical sink and in CH4 emissions. We perform idealized perturbation simulations with either increased CO2 mixing ratios (with CO2 being an inert GHG) or increased CH4 emission fluxes. The CH4 perturbation affects the chemical composition directly, whereas the CO2 perturbation influences the chemical composition only indirectly, e.g. through the temperature change. From the simulation results we assess the change in CH4 mixing ratios and its implications for OH and O3. In addition to the CH4 feedback, GSAT changes induce other processes that influence O3. For instance, temperature changes affect chemical reaction rates, emissions of precursor species from natural sources, circulation, or the abundance of H2O (e.g. Stevenson et al., 2006; Chiodo et al., 2018; Abalos et al., 2020; Griffiths et al., 2021; Zanis et al., 2022). Therefore, we apply an attribution method for O3 (TAGGING; Grewe et al., 2017; Rieger et al., 2018) to identify and quantify the importance of individual source categories that influence tropospheric O3 under tropospheric warming.
Our analysis is based on the ERF conceptual framework (Shine et al., 2003; Hansen et al., 2005; Ramaswamy et al., 2018; Forster et al., 2021), which means that the so-called fast and (slow) climate responses are assessed separately. The fast response represents the part of the full response that develops, on short timescales, independently of the corresponding GSAT change, whereas the climate response represents the isolated effect of the GSAT change. There is no formal timescale that separates the fast and slow responses, but they are distinguished conceptually by the dependence on the GSAT response, which is coupled to the (slow) response of the ocean. It is noteworthy that the CH4 adjustment that follows the increase of CH4 emission fluxes evolves on the timescale of decades, whereas typical physical (rapid) adjustments evolve on the timescale of weeks or months (e.g. Smith et al., 2018). We derive ERF and the fast response from simulations with prescribed sea surface temperatures (SSTs) and sea ice concentrations (SICs) as recommended by Forster et al. (2016). The climate response is assessed as the difference between the response in a simulation coupled to a mixed layer ocean model and the respective fast response. Analogously, we assess (rapid radiative) adjustments as the radiative effects corresponding to changes in the fast response and (slow climate) feedbacks as the radiative effects corresponding to changes in the climate response.
The paper is structured as follows. Section 2 explains the simulation set-up (Sect. 2.1.), as well as the TAGGING method to attribute O3 to individual source categories (Sect. 2.2.), and the method to derive the radiative effects corresponding to composition changes in individual species (Sect. 2.3.). Additionally, Sect. 2.3. introduces the theoretical framework for radiative forcing and climate sensitivity. Section 3 presents the simulation results. In Sect. 3.1. and 3.2., we present composition changes in the CO2 and CH4 perturbation simulations, respectively. In Sect. 3.3., the corresponding radiative effects are addressed. We conclude with a general discussion and summary of our findings in Sect. 4. Parts of the paper are based on the PhD thesis of the first author (Stecher, 2024).
2.1 Model description and simulation strategy
We use the modular CCM ECHAM/MESSy Atmospheric Chemistry (EMAC; Jöckel et al., 2016) in version 2.55.2. All simulations are performed at a resolution of T42L90MA, i.e. at a triangular (T) truncation at wave number 42 of the spectral dynamical core, corresponding to a quadratic Gaussian grid of approximately 2.8° × 2.8° resolution in latitude and longitude and 90 vertical levels, with the uppermost level centred around 0.01 hPa. The simulations are performed as time slices, which means that the same boundary conditions are repeated cyclically for each simulation year. The boundary conditions, i.e. prescribed emissions and mixing ratios of well-mixed GHGs, represent the year 2010. The quasi-biennial oscillation (QBO) is nudged following the method of Giorgetta and Bengtsson (1999) as described by Jöckel et al. (2016), which introduces some interannual variability. The simulation set-up builds on previous studies assessing the impact of enhanced CH4 mixing ratios with EMAC (Winterstein et al., 2019; Stecher et al., 2021), with the important advance that for the present study EMAC is used in a CH4-emission-flux-driven set-up, which means that CH4 emission fluxes instead of CH4 mixing ratios are prescribed at the lower boundary. The CH4 emission fluxes are prescribed as offline emission fluxes; i.e. there are no feedbacks on CH4 emission fluxes from natural sources such as wetlands or permafrost, which are expected to change in a changing climate (e.g. O'Connor et al., 2010; Dean et al., 2018).
The applied CH4 emission inventory is an inverse optimized inventory for the EMAC model (Frank, 2018). For the two reference simulations (see Table 1 and text below), monthly mean emissions of the year 2010 are repeated cyclically and scaled by a globally constant factor of 1.08, which corresponds to total annual mean emissions of 625.3 Tg (CH4) a−1. The scaling was applied to bring the simulated CH4 surface mixing ratios closer to observations. As the tropospheric mean CH4 lifetime is about 10 years (e.g. Prather et al., 2012; Stevenson et al., 2020), the CH4 mixing ratios of the year 2010 result not only from CH4 emissions of the year 2010, but also from emissions of the years before. Therefore, it cannot be expected that the cyclic repetition of CH4 emissions of the year 2010 results in CH4 mixing ratios that represent the year 2010 exactly. Applying the scaling, the resulting global mean CH4 surface mixing ratio is 1.82 ppmv for both reference simulations. This is in close agreement with observational estimates for the years 2010 and 2012 of 1.80 and 1.81 ppmv by the National Oceanic and Atmospheric Administration Earth System Research Laboratories (NOAA/ESRL) (Lan et al., 2022) and estimates of 1.81 and 1.82 ppmv by the WMO World Data Centre for Greenhouse Gases (WMO, 2022). The estimates of NOAA/ESRL tend to be lower, as only unpolluted marine surface sites contribute to the global estimate. The chemical sink reactions of CH4 with OH, excited oxygen (O(1D)) and chlorine (Cl), and CH4 photolysis are interactively accounted for by the MESSy submodels Module Efficiently Calculating the Chemistry of the Atmosphere (MECCA; Sander et al., 2019) and JVAL (Sander et al., 2014). Oxidation by OH dominates the tropospheric CH4 sink, so that the reaction with Cl accounts for only 0.23 % of the total chemical tropospheric CH4 loss (see Table S1 in the Supplement). Therefore, we focus on CH4 lifetime changes with respect to oxidation by OH. In addition to the sink reactions of CH4, the chemical mechanism covers the basic chemistry of O3, OH, hydroperoxyl (HO2), nitrogen oxides, alkanes and alkenes up to four C atoms, and isoprene (C5H8). Further, halogen chemistry of bromine and chlorine species is included. Alkynes, aromatics, and mercury are not considered. In total, the used mechanism covers 265 gas-phase, 82 photolysis, and 12 heterogeneous reactions of 160 species. The chemical feedback on H2O modifies the prognostic specific humidity, and vice versa. The soil sink of CH4 is included by the submodel DDEP (Kerkweg et al., 2006a), which uses a prescribed deposition rate (Spahni et al., 2011; Curry, 2007) that is scaled to the actual CH4 mixing ratio in the corresponding grid box. On average, the global soil sink is 27.6 Tg (CH4) a−1 for both reference simulations.
Table 1Overview of performed simulations. REF indicates that the respective reference is used, which is 388.4 ppmv for the global mean surface mixing ratio of CO2 and 625.3 Tg (CH4) a−1 for the CH4 surface emissions. The prescribed multi-year monthly mean climatology of SSTs and SIC is based on an observation-based estimate of the years 2000 to 2009 from the Met Office Hadley Centre (Rayner et al., 2003).
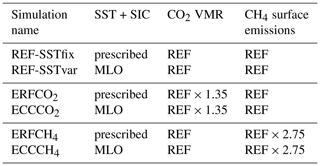
Precursor emissions of O3, in particular nitrogen oxides NO and NO2 (NOx), non-methane hydrocarbons (NMHCs), and carbon monoxide (CO), are treated as described by Jöckel et al. (2016). Anthropogenic emissions of these species are prescribed from the MACCity inventory (Lamarque et al., 2010; Granier et al., 2011; Diehl et al., 2012), whereby the (mostly monthly resolved) emission fluxes of the year 2010 are repeated cyclically. In addition, climatologies of biogenic emissions of NMHCs and CO are prescribed from the Global Emissions InitiAtive (GEIA). Natural emissions of NOx from lightning, emissions of NOx and C5H8 from biogenic sources, and the exchange of chemical species between the atmosphere and ocean are parameterized. For lightning NOx the parameterization of Grewe et al. (2001) is used in the MESSy submodel LNOX (Tost et al., 2007). The 20-year mean global emissions from lightning NOx are 5.2 Tg (N) a−1 for both reference simulations (see Table 3). Interactive biogenic emissions of soil NOx and C5H8 are calculated by the submodel ONEMIS (Kerkweg et al., 2006b). On average, biogenic NOx emissions are 6 Tg (N) a−1, and biogenic C5H8 emissions are about 307 Tg (C) a−1 for both reference simulations (see Table 3). The atmosphere–ocean exchange of the chemical species C5H8, dimethyl sulfide (DMS), and methanol (CH3OH) is parameterized using the submodel AIRSEA (Pozzer et al., 2006). The mixing ratios of CO2, nitrous oxide (N2O), and ozone-depleting substances (ODSs) are prescribed at the lower boundary using monthly mean values of the year 2010 (Meinshausen et al., 2011; Carpenter et al., 2018). For the radiation, a CFC-11 equivalent is calculated lumping additional radiatively active ODSs via radiative efficiencies following the approach by Meinshausen et al. (2017). For the short-lived halocarbons CHCl2Br, CHClBr2, and CH2ClBr, as well as CH2Br2 and CHBr3, surface emissions are prescribed from Warwick et al. (2006) and Liang et al. (2010), respectively.
Table 1 summarizes the performed simulations. The reference simulation with prescribed SSTs and SICs and interactive chemistry (REF-SSTfix) and the reference simulation with MLO and interactive chemistry (REF-SSTvar) serve as references for the experiment simulations and represent year 2010 conditions. REF-SSTfix is performed using prescribed SSTs and SICs, whereas for REF-SSTvar a mixed layer ocean (MLO) model is coupled (MESSy submodel MLOCEAN, Kunze et al., 2014; original ECHAM5 code by Roeckner et al., 1995, described in the ECHAM5 documentation, Chap. 6.3–6.5 in Roeckner et al., 2003). The prescribed multi-year monthly mean climatology of SSTs and SIC is an observation-based estimate of the years 2000 to 2009 from the Met Office Hadley Centre (Rayner et al., 2003). The same climatology was used by Winterstein et al. (2019). Appendix C of Stecher (2024) provides a comparison of the two reference simulations. Overall, the simulation REF-SSTvar reproduces the simulation REF-SSTfix well. The largest differences are in the Southern Hemisphere (SH) polar region, where the MLO model tends to underestimate the sea ice area of the prescribed climatology, which has been noted for a similar application of the MLOCEAN submodel as well (Stecher et al., 2021).
The CO2 perturbation simulation with prescribed SSTs and SICs and interactive chemistry (ERFCO2) and the CH4 perturbation simulation with prescribed SSTs and SICs and interactive chemistry (ERFCH4) are performed with the same prescribed climatology of SSTs and SICs as REF-SSTfix to assess the so-called fast response and to quantify the ERF and the adjustments following the fixed SST method (e.g. Forster et al., 2016). ERF is defined as the top-of-atmosphere (TOA) net radiative flux change between the experiment and the reference simulation. The perturbations of CO2 and CH4 are scaled to result in ERFs of similar magnitude, because for a most fair comparison of the climate sensitivity parameters of different perturbation agents, the respective forcings need to be at the same order of magnitude as the climate sensitivity, as it can be dependent on the magnitude of the forcing (e.g. Hansen et al., 2005; Dietmüller et al., 2014). The ERF is targeted to be large enough to cause statistically significant and interpretable feedbacks (Forster et al., 2016) and small enough to be reached with still realistically large perturbations of CO2 and CH4 (Dietmüller et al., 2014; Winterstein et al., 2019). Perturbations of 1.35 × CO2 mixing ratios and 2.75 × CH4 surface emission fluxes result in ERFs of 1.61 ± 0.16 and 1.72 ± 0.17 W m−2, respectively (see Table 4). The scaling of 1.35 × CO2 mixing ratios or 2.75 × CH4 surface emission fluxes is applied to all CO2 and CH4 perturbation experiments, respectively. The CO2 perturbation simulation with MLO and interactive chemistry (ECCCO2) and the CH4 perturbation simulation with MLO and interactive chemistry (ECCCH4) are so-called equilibrium climate change simulations. In these simulations the MLO model accounts for the response of SSTs and SICs. Therefore, the effect of GSAT-driven feedbacks is included in these simulations.
In the following, we assess the so-called fast response as the difference between ERFCO2 or ERFCH4 and REF-SSTfix, as well as the full response as the difference between ECCCO2 or ECCCH4 and REF-SSTvar. The difference between the full and the fast response is assessed as the climate response, which represents the isolated effect of the GSAT change. Similarly, adjustments and feedbacks are defined as the radiative effects corresponding to the fast response and the climate response, respectively. For the analysis, results of 20 simulated years after the spin-up period are used. The spin-up ensures that a quasi-equilibrium is reached and is, therefore, different for the individual simulations according to the simulation set-up. For ERFCH4 the longest spin-up period of 90 years was necessary; for ECCCO2 and ECCCH4 a period of 50 years was necessary, respectively; and for ERFCO2 a period of 25 years was necessary. Time series of the global mean surface CH4, the total atmospheric masses of CH4 and O3, the TOA radiation balance, and GSAT (for the MLO simulations) were monitored to decide whether an equilibrium is reached. In addition, we assessed the spin-up of the mass of CH4 of the simulation ERFCH4 in more detail. A curve fit was applied to the spin-up period to derive the atmospheric mass of CH4 in equilibrium. The mass of CH4 follows the exponential function of the form closely. The mass of CH4 in the last year of the spin-up, simulation year 90, is about 0.5 % smaller than the derived equilibrium estimate (parameter a) and therefore spun-up sufficiently well (see Fig. S14). The derived perturbation lifetime (parameter c) is 21.6 years. We note that the perturbation lifetime is larger than that of the CH4 emission reduction experiment by Staniaszek et al. (2022). As the perturbation lifetime increases with increasing CH4 burden (Holmes, 2018), this can be expected. In addition, model differences and the magnitude of the emission change might play a role.
In this study, the CH4 lifetime is calculated according to Jöckel et al. (2016) as
with being the mass of CH4 in kilograms (kg), being the temperature (T)-dependent reaction rate coefficient of the reaction in cm3 s−1, cair being the concentration of air in cm−3, and xOH being the mole fraction of OH in mol mol−1 in all grid boxes b∈B. B is the region for which the lifetime should be calculated, e.g. all grid boxes below the tropopause for the mean tropospheric lifetime. For the CH4 lifetime calculation a climatological tropopause, defined as tpclim=300–215 hPa ⋅cos 2(ϕ), with ϕ being the latitude in degrees north, is used as recommended by Lawrence et al. (2001). The reaction rate coefficient is calculated as in the applied kinetic equation system (submodel MECCA), i.e. as
2.2 TAGGING
The TAGGING method (Grewe et al., 2017; Rieger et al., 2018) quantifies the contributions of individual source categories to the mixing ratios of tagged tracers. Tagged tracers are O3, CO, reactive nitrogen compounds (NOy), peroxyacyl nitrate (PAN), NMHCs, OH, and HO2. For computational reasons NMHCs and NOy are considered with a family approach. For these species or families of species, the individual contributions of emission categories or source processes are calculated. In this study O3 production from the following categories is considered:
-
through photolysis of molecular oxygen (O2) in the stratosphere (O3 stratosphere);
-
from emissions of lightning NOx (O3 lightning);
-
from biogenic precursor emissions, mainly soil NOx and C5H8 (O3 biogenic);
-
from products of the CH4 decomposition (O3 CH4);
-
from products of the N2O decomposition (O3 N2O);
-
from biomass burning precursor emissions (O3 biomass burning); and
-
from anthropogenic precursor emissions (O3 anthropogenic).
The categories are the same as defined by Grewe et al. (2017), except for the category O3 anthropogenic, which, in our study, combines O3 production from all anthropogenic emissions, i.e. of the sectors industry, road traffic, shipping, and aviation.
The tagged tracers (i.e. the individual contributions) undergo the same processes as the corresponding total species. These are transport, emissions, dry and wet deposition, and chemical production and loss (see Grewe et al., 2017, for details). For the short-lived species OH and HO2 a steady state between chemical production and loss is assumed (Rieger et al., 2018). The chemical reaction rates are taken from the submodel MECCA. Effective production and loss are taken into account for O3, meaning that production and loss terms from a family, which includes all fast exchanges between O3 and other chemical species, are considered. The diagnostic tool ProdLoss (Grewe et al., 2017) is used to identify all reactions that contribute to effective O3 production and loss in the applied chemical mechanism. The reaction rates of effective O3 production and loss are then manually grouped into O3 production and loss rates, depending on which tagged species contributes to O3 production or loss.
2.3 Quantification of individual radiative effects
The assessment of radiative effects in this study follows the ERF framework. This means that (rapid radiative) adjustments, which are defined as the TOA net radiative flux change corresponding to changes in the fast response, i.e. independent from GSAT changes, are accounted for as part of the forcing. Thus, ERF is given as the sum of instantaneous radiative forcing (IRF), defined as the net radiative flux change at TOA excluding any adjustment, and the sum of all individual adjustments Ai (e.g. Smith et al., 2018):
Consistently, (slow climate) feedbacks are defined as the TOA net radiative flux change induced by atmospheric parameter changes that correspond to the gradually changing GSAT. These feedbacks act to reduce or enhance the associated GSAT change (ΔT) and determine the climate sensitivity parameter λ (units: K (W m−2)−1), which is the proportionality constant that relates the equilibrium change in GSAT to the ERF as
The feedback parameter α is the negative inverse of the climate sensitivity parameter λ (; units: W m−2 K−1). The feedback parameter quantifies the net radiative flux change at TOA for a given change in GSAT. Under the assumption of linearity it can be decomposed into the radiative contributions of individual processes affected by the change in GSAT, i.e. the individual feedback parameters αi, so that (e.g. Forster et al., 2021)
where N is the radiative flux change at TOA induced by the change in an individual variable of the Earth system xi.
We quantify adjustments and feedbacks corresponding to composition changes in CO2, CH4, O3, and stratospheric H2O following the method used by Winterstein et al. (2019) and Stecher et al. (2021). Additional simulations are performed with EMAC using the option for multiple diagnostic radiation calls (Dietmüller et al., 2016; Nützel et al., 2024). These simulations are performed (for the sake of saving computational resources) without interactive chemistry but with prescribed climatologies for the radiatively active trace gases CH4, CO2, O3, N2O, and the chlorofluorocarbons (CFCs) from the simulations REF-SSTfix or REF-SSTvar. SSTs and SICs are prescribed using the same observation-based climatology as used for REF-SSTfix (Rayner et al., 2003). Thus, the background climate of the simulations represents reference conditions. The additional simulations are run over 2 years each (plus 1-year spin-up).
In these simulations the first radiation call, P, provides the radiative heating rates that drive the base model, whereas the other radiation calls, D1, D2, etc., are purely diagnostic. The radiation call D1 serves as the reference for the perturbations and receives identical input as P, except for the specific humidity, for which a monthly mean climatology from the respective reference simulation (REF-SSTfix or REF-SSTvar) is used instead of the prognostic specific humidity from the base model. This is necessary because the radiation calls to calculate the radiative effect of H2O use monthly mean climatologies of the specific humidity from the sensitivity simulations (see below) and therefore need to be compared to a radiation call, which uses a monthly mean climatology of specific humidity instead of the prognostic, highly variable, specific humidity.
In the other radiation calls (D2, D3, etc.) either CO2, CH4, O3, or the specific humidity is replaced by a monthly mean climatology from the sensitivity simulation (either ERFCO2, ECCCO2, ERFCH4, or ECCCH4) to calculate the corresponding radiative effect. For example, to derive the radiative effect of the full response of CH4 of the CO2 perturbation, the reference CH4 climatology in D1 is replaced by the monthly mean CH4 climatology from ECCCO2 in D2. For O3 and the specific humidity, the radiative effects of changes in the troposphere and in the stratosphere are derived separately. The diagnostic radiation calls include the stratospheric temperature adjustment induced by the respective perturbation following the method of Stuber et al. (2001). Therefore, the radiative effect corresponding to CH4 (CO2) from the simulation ERFCH4 (ERFCO2) represents stratospheric-temperature-adjusted radiative forcing (SARF), i.e. the direct radiative effect of the perturbation including the associated stratospheric temperature adjustment. To define the region in which the stratospheric temperature adjustment is applied, as well as to separate tropospheric and stratospheric radiative effects of O3 and the specific humidity, the climatological tropopause tpclim is used consistently with the CH4 lifetime calculation (see Sect. 2.1).
There is one methodological difference compared to Winterstein et al. (2019) and Stecher et al. (2021). They used the climatological specified humidity directly in the first prognostic radiation call, which then served as the reference for the perturbed calls. However, here it was decided to use the prognostic specific humidity in the first radiation call as it is consistent with the model's background meteorology, e.g. the cloud cover. The influence on the calculated radiative effects was tested and found to be up to 1.02 % (or 0.004 W m−2), with the maximum deviation for the perturbations of specific humidity, which is negligible in comparison to other uncertainties.
3.1 Methane and ozone composition changes following 1.35 × CO2 perturbation
In this section, we present the simulation results of the 1.35 × CO2 perturbation. Figure 1 shows the annual zonal mean composition changes in CH4 mixing ratios in the simulations ERFCO2 (fast response) and ECCCO2 (full response), as well as their difference, which is interpreted as the climate response. The fast response is dominated by increasing CH4 mixing ratios in the upper stratosphere and mesosphere. In this region, the cooling to be expected from the CO2 increase (see Fig. S1 in the Supplement) leads to the prolongation of the CH4 lifetime. The stratospheric CH4 loss by reaction with OH, Cl, and O(1D) is reduced by about 2 % (see Table S1 in the Supplement). A similar effect has been noted by Dietmüller et al. (2014). In the fast response, tropospheric CH4 shows a slight increase below 2 %.
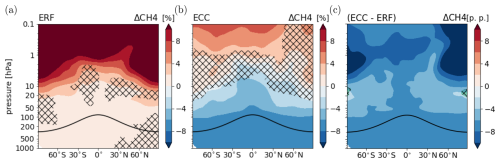
Figure 1CH4 response following the CO2 perturbation. Relative differences between the annual zonal mean CH4 mixing ratios of sensitivity simulations (a) ERFCO2 (fast response) and (b) ECCCO2 (full response) and their respective reference simulation in percent (%). (c) Climate response as the difference between the CH4 responses in panels (a) and (b) in percentage points (p.p.). Non-hatched areas are significant at the 95 % confidence level according to a Welch test based on annual mean values. The solid black line indicates the location of the climatological tropopause.
In contrast to the fast response, the full response shows a significant reduction of CH4 mixing ratios in the troposphere and lower stratosphere. As CH4 emission fluxes are prescribed in the simulation set-up and cannot respond to changes in meteorology or composition, any feedback on natural CH4 emissions (e.g. Dean et al., 2018) is suppressed. Therefore, the decrease of CH4 mixing ratios results from enhanced chemical decomposition of CH4, mainly by the oxidation with OH. The tropospheric CH4 lifetime with respect to oxidation by OH shortens by about 7 months (0.56 a or 7.4 %; see Table 2). This shortening is a combined result of the direct influence of the temperature on the reaction rate coefficient and of an enhanced abundance of OH. Tropospheric warming increases OH mixing ratios throughout the troposphere, with the maximum increase in the tropics (see Fig. S3 in the Supplement). Firstly, emissions from lightning NOx increase by about 0.3 Tg (N) a−1 in the climate response (see Table 3), which leads to enhanced production of OH. To estimate the effect on the CH4 lifetime, we use the multi-model mean sensitivity of the CH4 lifetime towards changing lightning NOx emissions of −4.8 % (Tg (N) a−1)−1 from Thornhill et al. (2021a), which suggest a shortening of the CH4 lifetime due to lightning NOx emissions by 1.4 %. Additionally, the increase in the tropospheric humidity associated with higher temperatures leads to enhanced production of OH.
Table 2Global annual mean values of tropospheric CH4 lifetime with respect to oxidation by OH, as well as CH4 surface volume mixing ratio for the performed simulations.
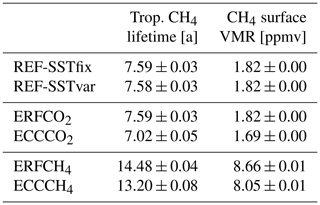
The values after the ± are the corresponding interannual standard deviations based on 20 annual mean values, which are listed to estimate the year-to-year variability.
Table 3Global annual mean emissions of NOx from lightning, NOx from biogenic sources, and C5H8 from biogenic sources.
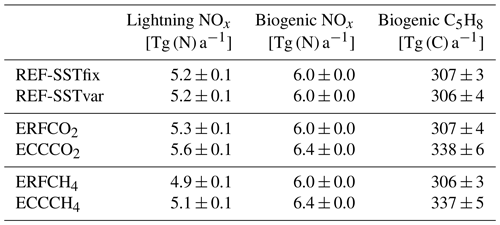
The values after the ± are the corresponding interannual standard deviations based on 20 annual mean values, which are listed to estimate the year-to-year variability. The model-calculated biogenic C5H8 emissions are scaled by a factor of 0.6 before being added to the atmospheric C5H8 tracer (see Jöckel et al., 2016). The values shown here include the scaling.
In addition to the reduction in the troposphere, the CH4 mixing ratios decrease also in the lower stratosphere as part of the full response. As the reaction partners of CH4, namely OH, Cl, and O(1D), do not show any significant response in the lower stratosphere (see Fig. S3 for OH) and the stratospheric CH4 loss does not change (see Table S1 in the Supplement), the decrease is likely a transport effect. Tropospheric air masses with reduced CH4 mixing ratios compared to the reference simulation enter the stratosphere by the upwelling branch of the Brewer–Dobson circulation. Tropical upwelling is enhanced in the climate response (see Fig. S13 in the Supplement). Dietmüller et al. (2014) noted an increase of CH4 mixing ratios throughout the stratosphere as a result of 2 × CO2 due to stratospheric cooling and thereby a slower CH4 oxidation. In addition, enhanced tropical upwelling transports CH4 enriched air in the stratosphere more efficiently. In our set-up, the reduction of tropospheric CH4, which is suppressed in the set-up of Dietmüller et al. (2014) as CH4 mixing ratios are prescribed at the lower boundary, dominates the latter two processes.
Previous studies also found that tropospheric warming leads to increasing OH mixing ratios and correspondingly to the shortening of the CH4 lifetime (e.g. Voulgarakis et al., 2013; Dietmüller et al., 2014; Heimann et al., 2020; Stecher et al., 2021; Thornhill et al., 2021a). The novelty of our study is that the associated reduction of CH4 mixing ratios is simulated explicitly, which was assessed by only a small number of studies so far (Heimann et al., 2020). In our CO2 perturbation experiment, the CH4 lifetime change per unit change in GSAT is −0.51 a K−1 or −6.7 % K−1.
Voulgarakis et al. (2013) addressed the sensitivity of the CH4 lifetime towards climate change in the Atmospheric Chemistry and Climate Model Intercomparison Project (ACCMIP) model ensemble. In the respective sensitivity simulations the boundary conditions for SSTs, SICs, and CO2 were set to RCP8.5 conditions for the year 2030 or the year 2100, while all other boundary conditions were representative of the year 2000. They found sensitivities of the tropospheric CH4 lifetime of −0.31 ± 0.14 a K−1 (−3.2 ± 1.0 % K−1) and −0.34 ± 0.12 a K−1 (−3.4 ± 0.8 % K−1) for the year 2030 and the year 2100 experiments, respectively1. The CMIP6 AerChemMIP model ensemble as analysed by Thornhill et al. (2021a) suggests a sensitivity of the whole-atmosphere CH4 lifetime towards climate change of −0.6 ± 4.5 % K−1 assessed from abrupt 4 × pre-industrial CO2 experiments. The large intermodel spread results from one model that shows an extension of CH4 lifetime as a result to 4 × CO2. The three models showing a shortening of CH4 lifetime suggest a sensitivity of −3.2 ± 0.8 % K−1 in close agreement with Voulgarakis et al. (2013).
Our study indicates a larger sensitivity of the CH4 lifetime towards climate change compared to Voulgarakis et al. (2013) and Thornhill et al. (2021a). Possible reasons are the different magnitudes of the perturbations, differences in the simulation set-ups, or the explicit treatment of the CH4 feedback in our study. The similar estimates for the years 2030 and 2100, corresponding to 1.14 and 4.76 K change in GSAT, respectively2, by Voulgarakis et al. (2013) suggest that the sensitivity is not highly dependent on the magnitude of the perturbation. Furthermore, the set-ups of individual models in Voulgarakis et al. (2013) and Thornhill et al. (2021a) differ, e.g. with respect to the level of complexity of the chemical mechanism, whether interactive aerosol is used, or through the different treatment of natural O3 precursor emissions. Nevertheless, the present estimate is larger than the estimates of all individual models in Voulgarakis et al. (2013) and Thornhill et al. (2021a), except for two models which do not parameterize the effect of stratospheric O3 on photolysis rates below, which is taken into account here. In the simulation set-ups analysed by Voulgarakis et al. (2013) and Thornhill et al. (2021a) CH4 mixing ratios are prescribed at the lower boundary in all models, except for the GISS-E2-R model analysed by Voulgarakis et al. (2013). The lifetime response per unit temperature change derived from GISS-E2-R is comparably weak. GISS-E2-R calculates wetland emissions of CH4 online, presumably also for the climate sensitivity experiments, which makes it difficult to compare the CH4 lifetime response in this model to our study. Nevertheless, compared to the other models, the explicit treatment of the CH4 feedback in our set-up allows for a subsequent feedback on OH and correspondingly for a self-feedback on the CH4 lifetime, which can explain the enhanced sensitivity of the CH4 lifetime towards climate change.
If the CH4 mixing ratio cannot adapt to changes in its lifetime, the corresponding CH4 equilibrium mixing ratio can be estimated using Eq. (1). The feedback factor f in the equation accounts for the CH4–OH feedback. In our CH4-emission-driven simulation the CH4–OH feedback is implicitly included in the simulated response of OH and the CH4 lifetime, so that using Eq. (1) with f > 1 applies the CH4–OH feedback twice in this case. Equation (1) indicates a global mean CH4 equilibrium mixing ratio in the range of 1.61 to 1.66 ppmv for for the present changes in the CH4 lifetime. Thus, it suggests a larger reduction than simulated by the model, which adjusts to a global mean CH4 equilibrium mixing ratio of 1.69 ppmv (see Table 2). However, if the feedback factor is not applied (f = 1), Eq. (1) gives 1.68 ppmv, which is in close agreement with the simulated response of CH4 mixing ratios.
The corresponding response of O3 is shown in Fig. 2. The fast response is dominated by O3 increases of up to 8 % in the middle and upper stratosphere. In these regions, CO2-induced stratospheric cooling causes slower chemical O3 depletion (e.g. Rind et al., 1998; Rosenfield et al., 2002; Portmann and Solomon, 2007; Dietmüller et al., 2014; Chiodo et al., 2018). In the lowermost stratosphere, O3 mixing ratios decrease by up to 4 %. This decrease can be explained by the so-called reversed self-healing (Rosenfield et al., 2002; Portmann and Solomon, 2007), which describes the effect that increasing O3 above leads to a reduction of ultraviolet radiation that reaches the lower stratosphere and consequently to reduced photochemical production of O3. The effect of transport from the troposphere into the stratosphere is expected to play a minor role in the fast response, as the strength of tropical upwelling is largely determined by the response of SSTs (Garny et al., 2011; Butchart, 2014). The fast response of tropospheric O3 is smaller than 2 %.
The climate response of O3 is dominated by a reduction of up to 10 % in the lowermost tropical stratosphere (see Fig. 2c). Enhanced tropical upwelling transports tropospheric O3 poor air from the troposphere into the stratosphere more efficiently. This is a robust feature across CCMs (Dietmüller et al., 2014; Nowack et al., 2015; Marsh et al., 2016; Chiodo et al., 2018). In the troposphere, O3 mixing ratios decrease by up to 6 % in the tropics close to the surface and decrease slightly in the upper tropical troposphere.
The pattern of the full response of stratospheric O3 is qualitatively consistent with previous studies of O3 changes resulting from a CO2 perturbation (Dietmüller et al., 2014; Nowack et al., 2015; Marsh et al., 2016; Nowack et al., 2018; Chiodo et al., 2018; Thornhill et al., 2021a). However, the tropospheric response is different here. Most studies using various CCMs consistently show an increase of O3 in the tropical upper troposphere as part of the full response (Dietmüller et al., 2014; Nowack et al., 2015; Marsh et al., 2016; Nowack et al., 2018; Chiodo et al., 2018). In the studies by Dietmüller et al. (2014) and Nowack et al. (2015, 2018), and presumably also in the studies by Marsh et al. (2016) and Chiodo et al. (2018), CH4 mixing ratios are prescribed at the lower boundary. Consequently, the negative CH4 feedback as discussed above cannot evolve. This can lead to an overestimation of O3 produced from products of the CH4 oxidation and is consistent with the positive response of O3 in the upper tropical troposphere. In particular, the comparison with the study by Dietmüller et al. (2014) indicates an effect of the CH4 feedback on O3 because the EMAC model was used as well in that study.
Using the MESSy submodel TAGGING (Grewe et al., 2017; Rieger et al., 2018) the tropospheric O3 response is attributed to individual source categories representing different processes of O3 production. Consistent with the small fast response of total tropospheric O3 (Fig. 2a), the corresponding response of the individual categories is below 0.5 % of the total reference O3 for all categories, except for O3 stratosphere (see Fig. S5 in the Supplement). The response of the category O3 stratosphere confirms that less O3 is produced via photolysis in the lower tropical stratosphere. Additionally, it indicates enhanced transport from the stratosphere into the troposphere in middle and higher latitudes of the Northern Hemisphere (NH).
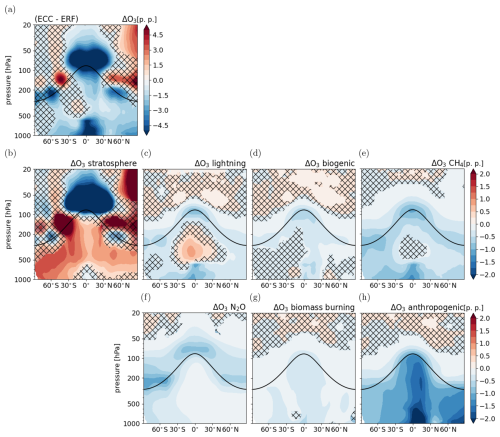
Figure 3Climate response of tropospheric O3 following the CO2 perturbation: (a) response of total O3 (same as Fig. 2c but with differently scaled colour levels to better compare with the response in the individual source categories), (b–h) response of O3 in individual categories relative to total reference O3 . Non-hatched areas are significant at the 95 % confidence level according to a Welch test based on annual mean values. The solid black line indicates the location of the climatological tropopause.
Figure 3 shows the climate response of individual O3 source categories presented as the difference between the fast and full response of each category in percentage points (p.p.):
The presentation relative to the total reference O3 allows the direct comparison of the responses of the individual categories to the relative response of total O3 as shown in Figs. 2c and 3a.
The climate response of the category O3 stratosphere shows significantly enhanced transport of stratospheric O3 into the troposphere in both hemispheres. In the extratropical middle troposphere, O3 mixing ratios increase by up to 1.5 % relative to the total reference O3 in the full response, which is the largest positive contribution to the total tropospheric O3 response. This supports current knowledge, as enhanced entry of stratospheric O3 under increasing GHG concentrations has been a robust feature in CCMs (Abalos et al., 2020). The category O3 stratosphere is also the largest contributor to the strong reduction in the lowermost stratosphere. The category O3 lightning shows a significant increase of up to 1.25 % relative to total reference O3 in the middle tropical troposphere. This is consistent with an increase of lightning NOx emissions by about 0.3 Tg (N) a−1 globally (see Table 3). Lightning NOx is emitted mainly in the upper tropical troposphere where convection is strongest (not shown). Biogenic emissions of NOx and C5H8 increase in the full response as well. Biogenic C5H8 emissions increase the most in the Amazon region and the Congo River basin, whereas biogenic NOx emissions increase over land in the tropics and mid-latitudes (not shown). However, the zonal mean climate response of O3 biogenic is mostly not significant due to competing effects of enhanced precursor emissions and of enhanced chemical loss with H2O. An enhanced sink of O3 via the reaction of O(1D) with H2O, which leads to effective O3 loss, is expected in a warmer and moister troposphere (e.g. Stevenson et al., 2006). The spatial distribution of the tropospheric O3 column shows mainly a reduction over the tropical ocean (see Fig. S7 in the Supplement), which is also reflected by the significant reduction between the Equator and 30° N in the zonal mean (Fig. 3d). Locally over regions with increasing precursor emissions, e.g. over the Amazon region and the Congo River basin, the tropospheric O3 column increases in the category O3 biogenic (see Fig. S7 in the Supplement). Anthropogenic and biomass burning emissions are prescribed and are therefore not affected by the CO2 increase. In these categories, decreasing O3 from enhanced loss or reduced O3 production efficiency is shown. The reduction of O3 anthropogenic is most pronounced over the tropical ocean, where a decline of O3 due to enhanced loss via H2O is expected (Stevenson et al., 2006; Zanis et al., 2022). The effect of the reduction of O3 biomass burning on total O3 is small, since also its absolute contribution is small. In addition to the enhanced sink, reduced O3 production per emitted NOx could play a role in the latter two categories as O3 precursor emissions from natural categories increase. The category O3 CH4 shows a reduction throughout the troposphere. This is consistent with the reduction of CH4 mixing ratios, as in the new equilibrium fewer products of the CH4 oxidation are available for O3 production, resulting in reduced O3 production in this category. Further, enhanced chemical loss can contribute to the reduction of this category. In the upper tropical troposphere the increase of lightning NOx emissions counteracts the effect of the CH4 decrease by providing enhanced levels of NOx, which react with the products of the CH4 oxidation more efficiently. The climate response of O3 CH4 is not significant in this region. The climate response in the category O3 N2O shows significant decreases in the lower stratosphere and troposphere. In the stratosphere, N2O mixing ratios increase (not shown) indicating less N2O decomposition (Dietmüller et al., 2014). Thereby, less nitrogen oxide (NO) is produced to form O3, which is consistent with the decrease of O3 formed from N2O decomposition.
3.2 Methane and ozone composition changes following 2.75 × CH4 emission flux perturbation
In this section, we present the simulation results of the 2.75 × CH4 emission flux perturbation. Figure 4 shows the zonal mean distribution of CH4 mixing ratios of the reference simulation REF-SSTfix and of the two simulations with CH4 emission fluxes increased by a globally constant factor of 2.75. As expected, CH4 mixing ratios increase everywhere in the fast response shown in Fig. 4b. Hereby, the increase factor of CH4 mixing ratios is even larger than the increase factor of the emission fluxes. Table 2 shows that an increase of CH4 emissions by a factor of 2.75 results in an increase of the global mean surface CH4 mixing ratio by a factor of 4.76. This is caused by a large extension of the tropospheric CH4 lifetime by about 7 years (see Table 2). The CH4 increase reduces the tropospheric OH mixing ratios by up to 60 % (see Fig. S3 in the Supplement), thereby extending the CH4 lifetime.
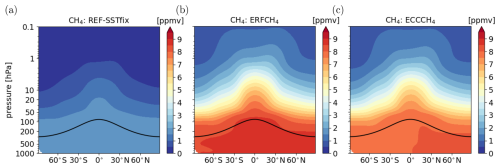
Figure 4Annual zonal mean distribution of CH4 mixing ratios in simulation (a) REF-SSTfix, (b) ERFCH4 (fast response), and (c) ECCCH4 (full response) in ppmv.
A similar effect was found by Winterstein et al. (2019), who analysed the fast response of 2 × and 5 × CH4 surface mixing ratios in a set-up with prescribed CH4 surface mixing ratios also using EMAC. The magnitude of the present CH4 perturbation is comparable to their 5 × CH4 experiment. In particular, to reach the prescribed CH4 surface mixing ratios a pseudo surface emission flux is diagnosed in their set-up. The increase factor of the pseudo flux that corresponds to an increase of 5 × CH4 is 2.75 (Stecher et al., 2021), exactly the increase factor of CH4 surface emissions used in our study. Thus, in our study the increase of emission fluxes results in a close to 5-fold increase of the CH4 surface mixing ratio. The global mean reference CH4 mixing ratio and the corresponding pseudo emission flux are slightly lower in Winterstein et al. (2019), namely about 1.8 ppmv and 567.7 × 1012 g CH4 a−1 (Tg (CH4) a−1). Additionally, the spatial distribution of their diagnosed pseudo flux is different and might be unrealistic. The latter two points explain why the increase factor of CH4 mixing ratios is not exactly the same in our study. Nevertheless, the results suggest that the relation between the increase of CH4 emissions and mixing ratios at the lower boundary is consistent if either the emissions or the mixing ratios are increased.
We derive the feedback factor f (see Eq. 1) from ERFCH4 using two approaches. Firstly, it is calculated from Eq. (12) by Holmes (2018) as . Secondly, it is derived from a curve fit of the function (Holmes, 2018) of the spin-up of the atmospheric mass of CH4 using the yearly mean CH4 lifetime with respect to OH oxidation for τ (see Fig. S14). Both approaches suggest f = 1.55. However, the derived f is not expected to be representative of CH4 perturbations of EMAC close to present-day conditions because f increases with increasing CH4 burden (Holmes, 2018). This might also explain why our estimate of f is at the upper end of previous estimates (Fiore et al., 2009; Voulgarakis et al., 2013; Stevenson et al., 2013; Thornhill et al., 2021b; Stevenson et al., 2020; Sand et al., 2023).
In the stratosphere, CH4 loss by OH is enhanced due to an increase of stratospheric HOx, whereas CH4 loss by Cl is reduced (see Table S1 in the Supplement). Overall, chemical stratospheric CH4 loss is increased by 17.5 %. However, the increase of CH4 mixing ratios is larger than the increase factor of surface emissions of 2.75 in the whole stratosphere as well.
In the full response, as shown in Fig. 4c, CH4 mixing ratios are lower in comparison to the fast response, as shown in Fig. 4b. Similar to the climate response following the CO2 perturbation, higher tropospheric temperatures lead to increased production of OH (see Fig. S3 in the Supplement). Additionally, the temperature-dependent reaction rate coefficient leads to a faster CH4 oxidation. The corresponding sensitivity of the CH4 lifetime per unit change in GSAT is −1.09 a K−1 or −7.6 % K−1 (relative to the lifetime in ERFCH4). Both the absolute and the relative sensitivity are larger compared to the CO2 perturbation experiment, which is possibly caused by the different CH4 conditions in the respective fast responses (ERFCO2 and ERFCH4).
Stecher et al. (2021) analysed the climate response of the 2 × and 5 × CH4 surface mixing ratio experiments corresponding to Winterstein et al. (2019). The sensitivity of CH4 lifetime per unit change in GSAT corresponding to their 5 × CH4 surface mixing ratio perturbation is , which corresponds to a relative change of −5.9 % K−1 (relative to the lifetime in their fast response), and is thus less pronounced than the respective value of our study. The major difference between the simulation set-ups is that tropospheric CH4 mixing ratios cannot respond to the lifetime response in the set-up of Stecher et al. (2021). This suggests that in the present study the sensitivity of CH4 lifetime towards climate change is enhanced, because the CH4–OH feedback is included in the response of OH and, therefore, in the CH4 lifetime. The same is indicated by the results of the CO2 perturbation as discussed above.
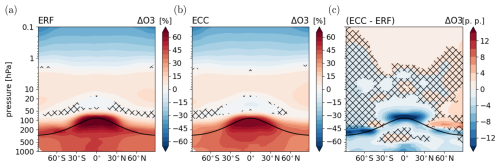
Figure 5O3 response following the CH4 perturbation (same as Fig. 2 but for the CH4 perturbation). Relative differences between the annual zonal mean O3 mixing ratios of sensitivity simulations (a) ERFCH4 (fast response) and (b) ECCCH4 (full response) and their respective reference simulation in percent (%). (c) Climate response as the difference between the O3 responses in panels (a) and (b) in percentage points (p.p.). Non-hatched areas are significant at the 95 % confidence level according to a Welch test based on annual mean values. The solid black line indicates the location of the climatological tropopause.
The response of O3 is shown in Fig. 5. In the fast response (Fig. 5a), O3 mixing ratios increase significantly throughout the troposphere, with a maximum increase of up to 60 % in the upper tropical troposphere. The CH4 perturbation leads to enhanced O3 formation by enhanced production of O3 precursor species through CH4 oxidation. In the stratosphere, radiatively induced cooling (a rapid adjustment) leads to O3 increases in the middle stratosphere. Above 1 hPa, O3 mixing ratios decrease due to enhanced catalytic depletion by odd hydrogen (HOx). HOx is increased by enhanced production of stratospheric H2O caused by CH4 oxidation (see Fig. S2 in the Supplement) and also by enhanced formation via the sink reaction of CH4 with O(1D). In the lower tropical stratosphere O3 decreases, which can be explained by the reversed self-healing effect (Rosenfield et al., 2002; Portmann and Solomon, 2007), which is also effective for the CO2 perturbation (see above). The fast response of O3 is consistent with the fast response evolving in the comparable 5 × CH4 surface mixing ratio experiment (Winterstein et al., 2019), as the same processes are effective, which are explained in more detail by Winterstein et al. (2019).
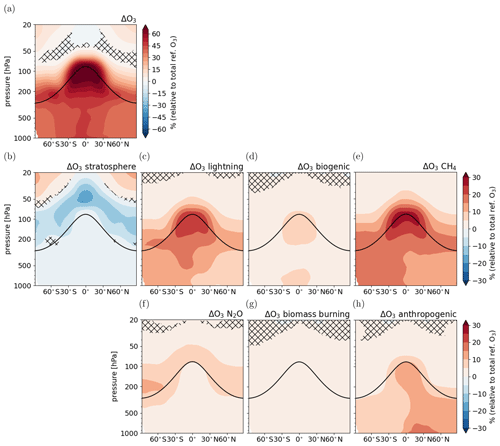
Figure 6Fast response of tropospheric O3 following the CH4 perturbation: (a) response of total O3 (same as Fig. 5a but data only shown up to 20 hPa to better compare with the response in the individual categories), (b–h) response of O3 in individual source categories relative to total reference O3 . Non-hatched areas are significant at the 95 % confidence level according to a Welch test based on annual mean values. The solid black line indicates the location of the climatological tropopause.
Figure 6 shows the fast response of individual O3 categories derived using the TAGGING submodel. Shown is the difference between ERFCH4 and REF-SSTfix of one category relative to the total reference O3:
allowing a direct comparison with the relative response of total O3. The O3 mixing ratios increase in all categories except for the category O3 stratosphere. This category shows reduced O3 production through photolysis of O2 in the lower stratosphere consistent with the reverse self-healing effect. The increase is strongest in the category O3 CH4, as the CH4 increase directly leads to the formation of O3. The increase in this category is most pronounced in the upper tropical troposphere and reaches up to 30 % relative to the total reference O3. The larger abundance of NMHCs and CO also affects O3 production of the other categories as their reaction with precursors from other categories, in particular NOx, leads to enhanced O3 production in the category O3 CH4 but also in the other categories. This effect is largest for the category O3 lightning, which shows O3 increases of up to 20 % relative to the total reference O3, even though emissions of lightning NOx decrease by 0.3 Tg (N) a−1 globally in the ERFCH4 simulation compared to REF-SSTfix (see Table 3). The CH4 perturbation leads to upper tropospheric/lower stratospheric warming peaking at around 100 hPa in the tropics (see Fig. S1 in the Supplement). The higher static stability leads to less convection and thereby to decreasing lightning NOx emissions. Upper troposphere/lower stratosphere warming following increased CH4 has been already noted elsewhere and is expected to be even more pronounced if shortwave (SW) absorption by CH4 is accounted for in the simulation set-up (Modak et al., 2018; Allen et al., 2023). Nevertheless, the enhanced abundance of precursors from CH4 oxidation leads to enhanced O3 production in this category. The category showing the third most pronounced increase is O3 anthropogenic. Here, the increase of up to 15 % relative to the total reference O3 is most pronounced in the lower NH, where the contribution of O3 anthropogenic to total O3 is largest.
The climate response of O3 shown as the difference between full and fast response (see Fig. 5c) represents the isolated effect of the GSAT response on O3. It shows a strong reduction of O3 mixing ratios in the lower tropical stratosphere, which is caused by enhanced tropical upwelling (consistent with the CO2 simulation). In the northern polar lower stratosphere, O3 mixing ratios are enhanced, pointing towards strengthened poleward and downward transport (i.e. strengthening of the Brewer–Dobson circulation; see Fig. S13 in the Supplement) of stratospheric air masses. In the southern polar tropopause region the rise of the tropopause in the climate response leads to a large O3 reduction. This process is also apparent in the NH, albeit less pronounced. Apart from that, the full response of O3 in the stratosphere is mainly caused by the fast response. The climate response of tropospheric O3 shows a reduction, except for the tropical middle troposphere, where the response shows a weak, not significant, increase in the zonal mean. In this region, enhanced emissions of lightning NOx lead to enhanced O3 formation (see also discussion of TAGGING results below). The climate response of O3 is strikingly similar to the climate response pattern resulting from the CO2 perturbation (see Fig. 2c), even though the fast response is different. The spatial distribution of the climate response of surface O3 is likewise similar for the CO2 and the CH4 perturbation (see Fig. S4).
The similarity of the climate response patterns of O3 resulting from CO2 and CH4 perturbations has been also noted by Stecher et al. (2021). However, the O3 climate response resulting from their 5 × CH4 mixing ratio increase shows a significant increase of O3 in the tropical middle troposphere. As already stated, the main difference between their set-up and ours is that the feedback on CH4 mixing ratios, and thereby also any secondary effects on O3, is suppressed. As for the CO2 perturbation, the reduction of CH4 mixing ratios feeds back on O3.
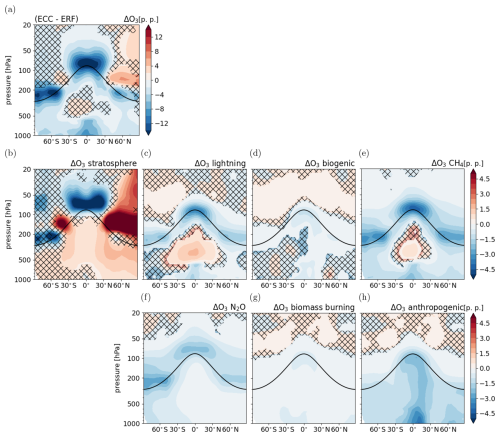
Figure 7Climate response of tropospheric O3 following the CH4 perturbation (same as Fig. 3 but for the CH4 perturbation): (a) response of total O3 (same as Fig. 5c but data only shown up to 20 hPa to better compare with the response in the individual categories), (b–h) response of O3 in individual source categories relative to total reference O3 . Non-hatched areas are significant at the 95 % confidence level according to a Welch test based on annual mean values. The solid black line indicates the location of the climatological tropopause.
Figure 7 shows the climate response of individual O3 source categories. It is calculated using Eq. (7) in accordance with the corresponding analysis of the CO2 perturbation. The patterns of the climate response of the individual categories are overall consistent with those of the CO2 perturbation.
The category O3 stratosphere increases in the troposphere, indicating enhanced transport of stratospheric O3 into the troposphere. The increase is significant everywhere, except for the extratropical SH and the lower tropical troposphere. This category contributes most strongly to the reduction of O3 in the lower tropical stratosphere. The category O3 lightning shows increases in the tropical middle troposphere resulting from an increase of the lightning NOx emissions by 0.2 Tg (N) a−1 in ECCCH4 compared to ERFCH4 (see Table 3) and decreases in the lower troposphere. Biogenic NOx emissions increase by 0.37 Tg (N) a−1 and biogenic C5H8 emissions increase by about 30 Tg (C) a−1 as a reaction to climate change (see Table 3). However, the zonal mean climate response of O3 in this category is mostly not significant and shows a decrease in the lower tropical and upper NH troposphere (see Fig. 7d). The tropospheric O3 columns in this category increase locally over the Amazon region and the Congo River basin, where biogenic emissions of C5H8 increase the most, and the tropospheric O3 columns decrease mostly over the tropical ocean (see Fig. S9 in the Supplement). As already mentioned, the sink of O3 via the reaction of O(1D) with H2O is expected to strengthen in a warmer and moister troposphere. Similar to the CO2 perturbation, the effects of increased O3 precursor emissions and the enhanced chemical sink due to a larger abundance of tropospheric H2O compete in this category. The category O3 CH4 decreases everywhere in the zonal mean, except for the tropical middle troposphere. The reduction is consistent with the reduction of CH4 mixing ratios in the climate response, which leads to a reduced formation of O3. In addition, the enhanced chemical sink leads to further reduction of O3. The increase in the tropical middle troposphere coincides with the maximum increase of O3 production from lightning NOx emissions, which indicates that enhanced NOx from lightning reacts with products of the CH4 oxidation, resulting in an increased O3 production in both categories. The corresponding response of the tropospheric O3 columns is not significant in the tropics, because of the counteracting responses in the lower and middle troposphere, but shows a significant decrease in the extratropics (see Fig. S9 in the Supplement). The categories with prescribed O3 precursor emissions, O3 biomass burning and O3 anthropogenic, show decreased O3 mixing ratios throughout the troposphere (see Fig. 7g and h), consistently with the climate response resulting from the CO2 perturbation. Additionally, reduced O3 production per emitted molecule NOx can play a role as O3 precursor emissions of natural categories increase.
3.3 Radiative effects and climate sensitivity
Table 4 shows results for the total SARF, ERF, ΔGSAT, and the associated climate sensitivity parameters λ, as well as individual radiative effects corresponding to the composition changes in CH4, O3, and stratospheric H2O. ERF includes physical and chemical adjustments, whereas SARF represents the radiative effect of the CO2 or CH4 composition change and the corresponding stratospheric temperature adjustment only.
Winterstein et al., 2019Stecher et al., 2021Table 4Estimates of total SARF, ERF, ΔGSAT, and the corresponding climate sensitivity parameters λ, as well as adjustments and feedbacks of individual composition changes in CH4, O3, and stratospheric H2O. ERF includes physical and chemical adjustments, whereas SARF represents the radiative effect of the CO2 or CH4 composition change and the corresponding stratospheric temperature adjustment only. The climate sensitivity parameters λSARF and λERF are calculated using SARF or ERF, respectively. Adjustments are calculated as the radiative flux changes in the fast response (in W m−2). Feedbacks are calculated as the difference of radiative flux changes between the full and the fast response divided by the corresponding change in global surface air temperature, ΔGSAT (in W m−2 K−1). The radiative effects of individual composition changes include the corresponding stratospheric temperature adjustment (Stuber et al., 2001). All radiative estimates are evaluated at TOA. In addition, the estimates of the 5 × CH4 volume mixing ratio experiments analysed by Winterstein et al. (2019) and Stecher et al. (2021) are shown in the third column.
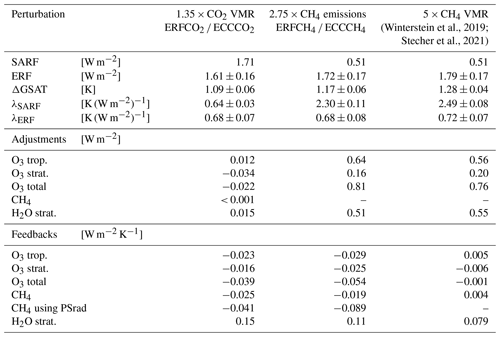
Values after the ± sign are 2 × the standard error of the mean calculated on the basis of 20 annual mean values, which approximate the corresponding 95 % confidence intervals. The standard errors for the climate sensitivity parameters are calculated from the standard error of the corresponding radiative forcing SERF and the standard error of ΔGSAT SEΔGSAT, as .
The method to derive stratospheric-temperature-adjusted radiative estimates does not account for interannual variability, which is why no uncertainty estimates are provided for the respective estimates.
For the CO2 perturbation, the estimate of ERF is smaller than SARF, but the difference is not significant due to the large statistical uncertainty associated with ERF (e.g, Forster et al., 2016). Along with this, the climate sensitivity parameter based on ERF, λERF, is larger compared to λSARF, but the difference is not statistically significant either. In contrast, for the CH4 perturbation, the estimate of ERF of 1.72 ± 0.17 W m−2 is considerably larger than SARF, which is estimated at 0.51 W m−2. The estimate of SARF reproduces the result of the comparable 5 × CH4 mixing ratios experiment with the EMAC model (Winterstein et al., 2019). However, the radiative effect of CH4 is known to be underestimated by the used radiative transfer scheme (Winterstein et al., 2019; Nützel et al., 2024). For instance, using the formula by Etminan et al. (2016)3 for the present CH4 perturbation a SARF of about 1.7 W m−2 is derived. The underestimation of the radiative effect of CH4 affects the estimate of ERF as well. Under the assumption that all other adjustments remain the same, only the direct contribution of CH4 is exchanged, which results in an ERF of about 2.9 W m−2. This considerably larger ERF suggests a correspondingly larger response of GSAT as well.
As illustrated by Table 4, chemical adjustments play a minor role for the CO2 perturbation. The fast response of stratospheric O3 induces a negative adjustment of −0.034 W m−2, whereas the fast response of tropospheric O3 induces a positive adjustment of 0.012 W m−2. The adjustment of tropospheric CH4 is negligible, whereas stratospheric CH4 induces a small positive adjustment due to its increase in the stratosphere and associated local radiative cooling. H2O increases in the lowermost stratosphere by up to 5 % (see Fig. S2 in the Supplement), which leads to a positive adjustment of 0.015 W m−2. The change in H2O in the lowermost stratosphere is largely driven by a change in the tropical cold point temperature (CPT) (see Fig. S10). For the CO2 perturbation, chemical production of H2O in the stratosphere is slightly reduced up to about 2 hPa. Thus, the adjustment of stratospheric H2O is unlikely to be chemically induced. To summarize, interactive chemistry dampens the ERF of the CO2 perturbation by about −1.3 %, mainly by the effect of stratospheric O3.
For the CH4 perturbation, chemical adjustments of O3 and stratospheric H2O are important contributions to the ERF. The adjustment of tropospheric O3 is 0.64 W m−2, and that of stratospheric O3 is 0.16 W m−2. In addition, the adjustment of stratospheric H2O is estimated at 0.51 W m−2. The CH4 perturbation leads to relative increases of H2O up to 250 % in the upper stratosphere and mesosphere (see Fig. S2 in the Supplement) because the increased abundance of CH4 leads to enhanced production of H2O by CH4 oxidation. Additionally, warming of the tropical cold point (see Fig. S10) leads to reduced dehydration of upwelling air parcels and thus to an increased abundance of H2O in the lower stratosphere. The zonal mean warming of the tropical cold point is 1.5 K and thereby more pronounced than in the respective CO2 experiment. The CH4 perturbation induces a direct radiative heating at the tropical cold point of up to 1 K (see Fig. S12 in the Supplement), and the response of stratospheric O3 leads to additional radiative heating of about 1.5 K in this region (see Fig. S12 in the Supplement). To summarize, the adjustments of O3 and stratospheric H2O enhance the ERF of the CH4 perturbation by 1.31 W m−2. The estimates of the adjustments of O3 and stratospheric H2O are consistent with the results of the comparable 5 × CH4 mixing ratios experiment (Winterstein et al., 2019, see also column 3 in Table 4).
The feedback parameters represent the radiative effects induced by changes in composition related to the climate response, i.e. to the isolated effect of GSAT changes. They are, as usual, given in W m−2 K−1, i.e. normalized by the corresponding GSAT response. For both perturbation types, CO2 and CH4, the feedbacks of CH4 and O3 are negative, which means that they dampen the resulting temperature change. The feedback parameter corresponding to the CH4 reduction in the CO2 perturbation experiment is −0.025 W m−2 K−1. As already mentioned, it is known that the direct radiative effect of CH4 is underestimated by the used radiative transfer scheme (Winterstein et al., 2019; Nützel et al., 2024). Therefore, we additionally calculate the feedback parameter for the same change in CH4 mixing ratios, but with the PSrad radiation scheme (Pincus and Stevens, 2013; Nützel et al., 2024), using otherwise the same methodology (see Sect. 2.3). With PSrad, the CH4 feedback is estimated at −0.041 W m−2 K−1, implying a more pronounced negative radiative feedback. Applying the formula of Etminan et al. (2016) for the change in CH4 mixing ratio diagnosed from the simulation suggests a radiative effect of −0.059 W m−2, which corresponds to a feedback parameter of −0.054 W m−2 K−1. Previous estimates of the CH4 feedback have been derived offline from the change in atmospheric CH4 lifetime and range from −0.014 ± 0.067 W m−2 K−1 (Thornhill et al., 2021a, if the estimates for changes in biogenic volatile organic compounds, lightning NOx, and meteorology are combined4) over −0.03 ± 0.01 W m−2 K−1 (Heinze et al., 2019) to −0.036 W m−2 K−1 (Dietmüller et al., 2014). Our estimate using the PSrad scheme is at the upper end of previous estimates but in the range of estimates from individual models analysed by Thornhill et al. (2021a).
For the CH4 perturbation, the feedback associated with the reduction of CH4 mixing ratios in the full response in comparison to the fast response is −0.019 W m−2 K−1. Using the PSrad radiation scheme with otherwise the same method, the feedback parameter is estimated at −0.089 W m−2 K−1 suggesting a clearly larger influence. The radiative feedback of CH4 corresponding to the 5 × CH4 mixing ratio experiment (Winterstein et al., 2019; Stecher et al., 2021) does not include the reduction of CH4 in the troposphere. The corresponding feedback parameter indicates a small positive feedback, caused by the larger mixing ratios of CH4 in the stratosphere in the full response compared to the fast response (Stecher et al., 2021).
The feedback parameters corresponding to O3 changes in the troposphere and stratosphere are both negative and add to total feedback parameters of −0.039 W m−2 K−1 for the CO2 perturbation and −0.054 W m−2 K−1 for the CH4 perturbation. Previous studies of the O3 feedback resulting from CO2 perturbations have assessed the full response in contrast to the climate response. The feedback parameter corresponding to the full response, i.e. including the adjustment, is −0.059 W m−2 K−1 in our study. Previous estimates range from −0.015 and −0.022 W m−2 K−1 (Dietmüller et al., 2014), −0.018 W m−2 K−1 (Marsh et al., 2016), −0.046 ± 0.018 W m−2 K−1 (Thornhill et al., 2021a), to −0.12 W m−2 K−1 (Nowack et al., 2015, if a corresponding GSAT response of 5.75 K is assumed). The feedback parameter of total O3 in the present study lies in the range of previous estimates but, notably, is more pronounced than the estimate by Dietmüller et al. (2014), who also used the EMAC model. Part of the difference can be explained by the different sign of the feedback of tropospheric O3. Dietmüller et al. (2014) found a positive feedback parameter of 0.008 to 0.009 W m−2 K−1 for tropospheric O3 compared to the negative feedback parameter in this study. The reduction of tropospheric CH4 mixing ratios leads to reduced O3 production and thereby modifies the response of O3 as discussed above. This indirect effect on O3 is a consequence of applying emission fluxes instead of a prescribed lower boundary mixing ratio for CH4. In addition, the negative feedback of stratospheric O3 is also more pronounced in this study, which might be explained by the different magnitude of the perturbations. Dietmüller et al. (2014) noted differences between their 2 × and 4 × CO2 experiments. Therefore, deviations for the 1.35 × CO2 in this study can be expected. In addition, the different vertical resolution of the simulation set-ups might affect the response of stratospheric O3. The results of the 5 × CH4 mixing ratio experiment by Stecher et al. (2021) do not indicate a significant feedback of total O3. This suggests that the reduction of CH4 mixing ratios in the full response drives the negative O3 feedback.
As explained above, the scaling factors of the CO2 and CH4 increase are chosen so that the resulting ERFs are comparable to allow an optimal comparison of the climate sensitivity of the two perturbation types, as the latter can depend on the magnitude of the radiative perturbation (e.g. Hansen et al., 2005; Dietmüller et al., 2014). The estimates of the climate sensitivity parameter based on ERF, λERF, are identical for the CO2 and CH4 perturbations of this study. In contrast, the climate sensitivity parameters based on SARF, λSARF, differ significantly between the CH4 and the CO2 perturbation. This finding confirms the results of previous studies that the climate sensitivity is in general less dependent on the type of perturbation for ERF (e.g. Richardson et al., 2019). The use of λERF as a climate sensitivity parameter to obtain an efficacy close to unity for the CH4 perturbation is more important in this study compared to Richardson et al. (2019), because of the effect of interactive chemistry, which leads to larger differences between SARF and ERF for the CH4 perturbation.
The estimate of λERF of 0.68 ± 0.08 K (W m−2)−1 is smaller than the corresponding estimate of the 5 × CH4 mixing ratio experiment of 0.72 ± 0.07 K (W m−2)−1 (Stecher et al., 2021). This suggests a reduction of the climate sensitivity parameter caused by the explicit simulation of the CH4 reduction which would be physically consistent with the negative feedbacks of O3 and CH4 in this study. The difference between the two estimates is, however, not statistically significant.
In this study, we assess the feedback of atmospheric CH4 resulting from changes in its chemical sink, which is mainly by oxidation with OH and which is influenced by temperature and the chemical composition of the atmosphere. We present results from numerical simulations with the CCM EMAC perturbed by either a 1.35 × CO2 mixing ratio or a 2.75 × CH4 emission flux increase. The scaling factors were chosen to reach a comparable ERF for both perturbation agents. EMAC is used in a CH4-emission-flux-driven set-up, which allows the atmospheric CH4 mixing ratio to adjust to changes in the chemical sink without constraints.
The increase of CH4 emissions by a globally constant factor of 2.75 corresponds to an increase of the global mean CH4 surface mixing ratio by a factor of 4.76. The larger increase of the CH4 mixing ratio compared to the emissions is caused by a strong reduction of tropospheric OH, which leads to the extension of the tropospheric CH4 lifetime. A similar effect was found by Winterstein et al. (2019), who analysed the response of 5 × CH4 mixing ratios using a comparable set-up of the EMAC model, but with prescribed CH4 surface mixing ratios. In particular, to reach the 5 × CH4 mixing ratio increase, a pseudo surface emission flux is calculated in their set-up. The increase factor of the pseudo flux that corresponds to an increase of 5 × CH4 is 2.75 (Stecher et al., 2021), exactly the scaling factor of CH4 surface emissions used in this study. To summarize, reduced chemical decomposition enhances the increase of the CH4 mixing ratios compared to the emissions. The relation between the increase of CH4 mixing ratios and CH4 emissions appears to be robust if either the mixing ratio or the emissions are increased.
We separately assess the so-called fast response in CO2 and CH4 perturbation simulations with prescribed SSTs and SICs, as well as the full response in simulations coupled to a MLO model. The CO2 perturbation affects the chemical composition only indirectly through temperature changes. In the fast response, radiatively induced cooling in the stratosphere causes slower chemical depletion and therefore leads to increasing mixing ratios of O3 and CH4. In particular, our results show that the well-known increase of upper and middle stratospheric O3 (e.g. Dietmüller et al., 2014; Nowack et al., 2015; Marsh et al., 2016; Chiodo et al., 2018) is part of the fast response and not related to the associated tropospheric warming. The CH4 emission increase directly influences the chemical composition in the troposphere and stratosphere. The fast response patterns of O3 and stratospheric water vapour are consistent with the changes following the 5 × CH4 mixing ratio increase (Winterstein et al., 2019) as expected from the comparable magnitude of CH4 mixing ratio increase.
Despite the different effect on the chemical composition in the fast response, the isolated effect of GSAT changes induced by either the CO2 or the CH4 increase is consistent. Tropospheric warming shortens the atmospheric lifetime of CH4. The corresponding reduction of CH4 mixing ratios is explicitly simulated by the used CH4-emission-flux-driven set-up. The explicit reduction of CH4 mixing ratios allows for secondary feedbacks on OH and O3.
Firstly, the CH4 lifetime response implicitly includes the CH4–OH feedback. The sensitivities of the CH4 lifetime per unit change in GSAT are −6.7 % K−1 for 1.35 × CO2 and −7.6 % K−1 for 2.75 × CH4, which is larger compared to previous CCM results using prescribed CH4 mixing ratios at the lower boundary (Voulgarakis et al., 2013; Thornhill et al., 2021a; Stecher et al., 2021). The results of the comparable CH4 increase experiment with prescribed CH4 surface mixing ratios (Stecher et al., 2021) provide a clear indication that the lifetime change per temperature change is larger in the CH4-emission-driven set-up. A comparable CO2 increase simulation using EMAC with prescribed CH4 surface mixing ratios is not available, but the comparison to the results of other CCMs (Voulgarakis et al., 2013; Thornhill et al., 2021a) indicates the same effect (see Sect. 3.1). Estimates of the CH4 lifetime change per temperature change from other CCMs driven by prescribed CH4 emission fluxes would be helpful to verify the influence of CH4 emission fluxes in comparison to prescribing CH4 at the lower boundary. Additionally, the multi-model differences of the CH4 lifetime change per unit change in GSAT are large (Voulgarakis et al., 2013; Thornhill et al., 2021a), and it would be valuable to identify reasons behind CCM differences in future studies.
Secondly, the reduction of CH4 mixing ratios results in reduced formation of O3 in the troposphere. This leads to substantial differences in the climate response of tropospheric O3 between this study and previous work using prescribed CH4 mixing ratios at the lower boundary (Dietmüller et al., 2014; Nowack et al., 2015; Marsh et al., 2016; Nowack et al., 2018; Chiodo et al., 2018; Stecher et al., 2021). The latter studies consistently show an increase of O3 mixing ratios in the tropical upper troposphere, whereas in this study the response is either insignificantly weak or indicates a reduction of O3 mixing ratios in this region. An attribution method is used to identify and quantify the processes that influence tropospheric O3 under climate change. Stronger stratosphere–troposphere exchange and larger natural emissions of O3 precursors lead to increases of tropospheric O3, whereas enhanced chemical loss caused by the increased tropospheric humidity and the reduction of CH4 mixing ratios lead to decreases. The contribution of the individual processes depends on the representation of the process of the model. For instance, the representation of O3 precursor emissions from natural sources is model dependent (e.g. Voulgarakis et al., 2013; Stevenson et al., 2020; Zanis et al., 2022). The climate response of lightning NOx emissions is uncertain so that even the sign of the projected change depends on the used parameterization (Finney et al., 2016, 2018; Zanis et al., 2022). This has implications for the climate response of tropospheric O3, for which changed lightning NOx emissions are found to be an important contribution. Most schemes used in CCMs to date project increasing lightning NOx emissions in response to tropospheric warming (Voulgarakis et al., 2013; Finney et al., 2016), which is in accordance with the results of this study, whereas a more sophisticated lightning NOx parameterization indicates a decrease of lightning NOx emissions (Finney et al., 2018). The spatial distribution of increases of biogenic C5H8 emissions over the Amazon region and the Congo River basin are in qualitative agreement with the climate response of biogenic emissions simulated by other CCMs (Zanis et al., 2022, see their Fig. S6). We expect the contribution of biogenic emission changes on O3 to be more important than diagnosed by the used version of the TAGGING method, as it underestimates the influence of C5H8 emissions on the diagnostic tracer O3 biogenic. The NMHC emissions are scaled by the number of C atoms in the molecule, i.e. five for C5H8, before they are added to the NMHC family tracer, which was not done in the case of the online calculated biogenic C5H8 emissions. We expect that this issue influences the quantitative response of the category O3 from biogenic sources and more precisely that changes in O3 biogenic caused by changes in C5H8 emissions are underestimated. However, we do not expect that the response patterns and general findings would change. Therefore, and due to the computational costs of the simulations, it was decided to not repeat the simulations. We also want to stress that this issue affects the diagnostic TAGGING results only but not the total O3 response. Furthermore, biogenic emissions of C5H8 depend on the underlying vegetation, which is expected to interact with changes in, for example, climate, atmospheric CO2 abundance, tropospheric O3, or land use change (e.g. Zhou et al., 2018; Vella et al., 2023), but such interactions are not included in the used set-up. In addition, droughts can inhibit the substrate supply and thereby reduce biogenic C5H8 emissions (e.g. Wang et al., 2022). A respective representation of the impact of droughts on C5H8 emissions is not included in the used model version.
We calculate the radiative effects that correspond to the composition changes in CH4, O3, and stratospheric H2O. Our results confirm that chemical adjustments of O3 and stratospheric H2O are important contributions to the ERF of the CH4 perturbation. Therefore, ERF is significantly larger than SARF, which represents the direct radiative effect of CH4 including the associated stratospheric temperature adjustment. The individual adjustments are in agreement with the estimates of the 5 × CH4 mixing ratio experiment (Winterstein et al., 2019).
Chemical adjustments play a minor role in the CO2 case. Here, stratospheric O3 induces a negative adjustment, which reduces the ERF by about −1.3 %. Previous studies defined the full response of O3 as its feedback (Dietmüller et al., 2014; Nowack et al., 2015; Marsh et al., 2016). Our results show that under the ERF framework a large part of the stratospheric O3 change is to be regarded as an adjustment. Further, our results do not indicate that ERF is significantly different from SARF for the CO2 perturbation, which is in accordance with simulation results, which account for physical adjustments only (Smith et al., 2018).
The scaling of the CO2 and the CH4 perturbations in this study is chosen so that the resulting ERFs are of similar magnitude. This allows an optimal comparison of the climate sensitivity parameters as these can depend on the magnitude of the perturbation (e.g. Hansen et al., 2005; Dietmüller et al., 2014). Our results suggest an efficacy of unity for the CH4 perturbation when the climate sensitivity parameters are based on ERF. The climate sensitivity parameters based on SARF differ significantly between the CO2 and the CH4 increase, because of the large effect of chemical adjustments for the CH4 perturbation. Thereby, our results support the finding that the climate sensitivity is in general less dependent on the type of perturbation for the ERF framework (e.g. Richardson et al., 2019). The multi-model mean efficacy based on ERF using the fixed SST method for a 3 × CH4 perturbation analysed by Richardson et al. (2019) is slightly less than 1, with a spread of individual models of 0.56–1.15. In their study, the efficacy based on SARF is slightly less than 1 as well. In our study, the efficacy based on SARF is significantly larger than 1, i.e. about 3.6, because of the effect of chemical adjustments, which is not included in the estimate of Richardson et al. (2019).
The feedbacks corresponding to tropospheric O3 changes in the full response are negative, in contrast to previous estimates derived with the EMAC model with prescribed CH4 mixing ratios at the lower boundary (Dietmüller et al., 2014; Stecher et al., 2021). As mentioned above, the explicit reduction of CH4 mixing ratios allows for secondary feedbacks on O3 to evolve and leads, more precisely, to reduced O3 formation, which is also reflected by the negative radiative feedback. Furthermore, the feedback parameter of tropospheric O3 derived from the multi-model mean analysed by Stevenson et al. (2006) does not account for the CH4 chemistry–climate feedback. The corresponding feedback parameter has been estimated at −0.007 ± 0.009 W m−2 K−1 by Heinze et al. (2019).
The reduction of CH4 mixing ratios in the climate response induces a negative feedback parameter, which is estimated at −0.025 W m−2 K−1 for the CO2 perturbation and at −0.019 W m−2 K−1 for the CH4 perturbation using the default radiative transfer scheme of EMAC. However, it is known that this radiative transfer scheme underestimates the direct radiative effect of CH4 (Winterstein et al., 2019; Nützel et al., 2024). Therefore, we derive the radiative feedback also with the PSrad radiation scheme (Pincus and Stevens, 2013; Nützel et al., 2024), which suggests clearly more pronounced feedback parameters of −0.041 W m−2 K−1 for the CO2 perturbation and of −0.089 W m−2 K−1 for the CH4 perturbation. The PSrad feedback parameters are in the range of previous individual model estimates derived from CH4 lifetime changes (Dietmüller et al., 2014; Heinze et al., 2019; Thornhill et al., 2021a).
Further, the default radiation scheme used in EMAC so far does not account for absorption of CH4 in the solar SW spectrum. Recent studies have shown that accounting for SW absorption by CH4 influences adjustment and feedback processes of, for example, clouds (Smith et al., 2018; Modak et al., 2018; Allen et al., 2023, 2024). For an improved representation of the radiative effect of CH4 in future studies, the PSrad radiation scheme (Pincus and Stevens, 2013) is now available for use in online EMAC simulations (Nützel et al., 2024).
This study focuses on the role of CH4 for interactions between the gas-phase chemistry and climate change. However, further processes, which are not accounted for by the used simulation set-up, can also play a role. For instance, chemistry–aerosol–cloud coupling was identified to contribute to the ERF of CH4 perturbations (Kurtén et al., 2011; O'Connor et al., 2022) and might therefore also influence the corresponding climate response. In addition, natural emission sources of CH4, e.g. from wetlands or permafrost, have the potential to increase in a warming climate (e.g. O'Connor et al., 2010; Dean et al., 2018). For instance, the results of Thornhill et al. (2021a) suggest that the negative radiative effect corresponding to the shortening of the CH4 lifetime is offset by the positive radiative effect of CH4 emission increases from wetlands in response to a 4 × CO2 perturbation. The net effect of feedbacks on the gas-phase chemistry and on natural emissions influences the effect of associated secondary feedbacks, e.g. regarding the formation of O3.
To conclude, the atmospheric abundance CH4, and therefore its potential as a greenhouse gas, is linked to a number of complex interactions. This makes the assessment of the climate feedback of CH4 and its indirect effects a non-trivial undertaking, which requires comprehensive chemistry–climate simulations. The novelty of this study is that the feedback on CH4 mixing ratios to changes in its chemical sink, and thereby also associated secondary feedbacks on OH and O3, is accounted for explicitly, which is recommended to be adopted for further studies on chemical feedbacks.
The Modular Earth Submodel System (MESSy; https://doi.org/10.5281/zenodo.8360276, The MESSy Consortium, 2021) is continuously further developed and applied by a consortium of institutions. The usage of MESSy and access to the source code is licensed to all affiliates of institutions which are members of the MESSy Consortium. Institutions can become a member of the MESSy Consortium by signing the MESSy Memorandum of Understanding. More information can be found on the MESSy Consortium website (http://www.messy-interface.org, last access: 29 April 2025). The simulation results presented here are based on MESSy version 2.55.2 (https://doi.org/10.5281/zenodo.8360276, The MESSy Consortium, 2021). Furthermore the exact code version used to produce the simulation results is archived at the German Climate Computing Center (DKRZ) and can be made available to members of the MESSy community upon request. The simulation results are available at the DKRZ DOKU long-term archive under https://www.wdc-climate.de/ui/entry?acronym=DKRZ_LTA_1132_ds00001 (Stecher et al., 2024). The HadISST data (Rayner et al., 2003) are available from https://www.metoffice.gov.uk/hadobs/hadisst/data/download.html (Met Office, 2025). We used Climate Data Operators (CDO; https://code.mpimet.mpg.de/projects/cdo/, last access: 7 May 2025; Schulzweida, 2023) and netCDF Operators (NCO; Zender, 2008) for data processing. Further, we used Python, especially the packages Xarray (Hoyer and Hamman, 2017) and Matplotlib (Hunter, 2007), for data analysis and producing the figures.
The supplement related to this article is available online at https://doi.org/10.5194/acp-25-5133-2025-supplement.
FW and MP developed the concept of the study. LS performed the model simulations, analysed the data, and created the figures with significant contributions regarding the interpretation and evaluation of the model results from all coauthors. LS prepared the original draft, and all authors contributed to the writing and reviewing of the manuscript.
At least one of the (co-)authors is a member of the editorial board of Atmospheric Chemistry and Physics. The peer-review process was guided by an independent editor, and the authors also have no other competing interests to declare.
Publisher's note: Copernicus Publications remains neutral with regard to jurisdictional claims made in the text, published maps, institutional affiliations, or any other geographical representation in this paper. While Copernicus Publications makes every effort to include appropriate place names, the final responsibility lies with the authors.
This article is part of the special issue “The Modular Earth Submodel System (MESSy) (ACP/GMD inter-journal SI)”. It is not associated with a conference.
We thank Simone Dietmüller (DLR) for doing the internal review. Further, we thank Zosia Staniaszek and two anonymous referees for their helpful comments on the discussion paper. We furthermore thank all contributors of the project ESCiMo (Earth System Chemistry integrated Modelling), which provides the model configuration and initial conditions. This work used resources of the Deutsches Klimarechenzentrum (DKRZ) granted by its Scientific Steering Committee (WLA) under project MIMETIC (bd1132).
This research has been supported by the Deutsche Forschungsgemeinschaft (grant no. WI 5369/1-1) and the DLR space research program (project MABAK, Innovative Methoden zur Analyse und Bewertung von Veränderungen der Atmosphäre und des Klimasystems).
The article processing charges for this open-access publication were covered by the German Aerospace Center (DLR).
This paper was edited by Jason West and reviewed by two anonymous referees.
Abalos, M., Orbe, C., Kinnison, D. E., Plummer, D., Oman, L. D., Jöckel, P., Morgenstern, O., Garcia, R. R., Zeng, G., Stone, K. A., and Dameris, M.: Future trends in stratosphere-to-troposphere transport in CCMI models, Atmos. Chem. Phys., 20, 6883–6901, https://doi.org/10.5194/acp-20-6883-2020, 2020. a, b
Allen, R. J., Zhao, X., Randles, C. A., Kramer, R. J., Samset, B. H., and Smith, C. J.: Surface warming and wetting due to methane's long-wave radiative effects muted by short-wave absorption, Nat. Geosci., 16, 314–320, https://doi.org/10.1038/s41561-023-01144-z, 2023. a, b
Allen, R. J., Zhao, X., Randles, C. A., Kramer, R. J., Samset, B. H., and Smith, C. J.: Present-day methane shortwave absorption mutes surface warming relative to preindustrial conditions, Atmos. Chem. Phys., 24, 11207–11226, https://doi.org/10.5194/acp-24-11207-2024, 2024. a
Ashmore, M. R.: Assessing the future global impacts of ozone on vegetation, Plant Cell Environ., 28, 949–964, https://doi.org/10.1111/j.1365-3040.2005.01341.x, 2005. a
Butchart, N.: The Brewer-Dobson circulation, Rev. Geophys., 52, 157–184, https://doi.org/10.1002/2013RG000448, 2014. a
Carpenter, L. J., Daniel, J., Fleming, E., Hanaoka, T., Hu, J., Ravishankara, A. R., Ross, M. N., Tilmes, S., Wallington, T. J., and Wuebbles, D. J.: Scenarios and information for policymakers, Chap. 6 in Scientific Assessment of Ozone Depletion: 2018, Global Ozone Research and Monitoring Project–Report No. 58, World Meteorological Organization, Geneva, Switzerland, https://ozone.unep.org/sites/default/files/2019-05/SAP-2018-Assessment-report.pdf (last access: 29 April 2025)., 2018. a
Chiodo, G., Polvani, L. M., Marsh, D. R., Stenke, A., Ball, W., Rozanov, E., Muthers, S., and Tsigaridis, K.: The Response of the Ozone Layer to Quadrupled CO2 Concentrations, J. Climate, 31, 3893–3907, https://doi.org/10.1175/JCLI-D-17-0492.1, 2018. a, b, c, d, e, f, g, h
Collins, W. J., Lamarque, J.-F., Schulz, M., Boucher, O., Eyring, V., Hegglin, M. I., Maycock, A., Myhre, G., Prather, M., Shindell, D., and Smith, S. J.: AerChemMIP: quantifying the effects of chemistry and aerosols in CMIP6, Geosci. Model Dev., 10, 585–607, https://doi.org/10.5194/gmd-10-585-2017, 2017. a
Collins, W. J., Webber, C. P., Cox, P. M., Huntingford, C., Lowe, J., Sitch, S., Chadburn, S. E., Comyn-Platt, E., Harper, A. B., Hayman, G., and Powell, T.: Increased importance of methane reduction for a 1.5 degree target, Environ. Res. Lett., 13, 054003, https://doi.org/10.1088/1748-9326/aab89c, 2018. a
Curry, C. L.: Modeling the soil consumption of atmospheric methane at the global scale, Global Biogeochem. Cy., 21, GB4012, https://doi.org/10.1029/2006GB002818, 2007. a
Dean, J. F., Middelburg, J. J., Röckmann, T., Aerts, R., Blauw, L. G., Egger, M., Jetten, M. S. M., de Jong, A. E. E., Meisel, O. H., Rasigraf, O., Slomp, C. P., in't Zandt, M. H., and Dolman, A. J.: Methane Feedbacks to the Global Climate System in a Warmer World, Rev. Geophys., 56, 207–250, https://doi.org/10.1002/2017RG000559, 2018. a, b, c
Diehl, T., Heil, A., Chin, M., Pan, X., Streets, D., Schultz, M., and Kinne, S.: Anthropogenic, biomass burning, and volcanic emissions of black carbon, organic carbon, and SO2 from 1980 to 2010 for hindcast model experiments, Atmos. Chem. Phys. Discuss., 12, 24895–24954, https://doi.org/10.5194/acpd-12-24895-2012, 2012. a
Dietmüller, S., Ponater, M., and Sausen, R.: Interactive ozone induces a negative feedback in CO2-driven climate change simulations, J. Geophys. Res.-Atmos., 119, 1796–1805, https://doi.org/10.1002/2013JD020575, 2014. a, b, c, d, e, f, g, h, i, j, k, l, m, n, o, p, q, r, s, t, u, v, w, x, y, z, aa
Dietmüller, S., Jöckel, P., Tost, H., Kunze, M., Gellhorn, C., Brinkop, S., Frömming, C., Ponater, M., Steil, B., Lauer, A., and Hendricks, J.: A new radiation infrastructure for the Modular Earth Submodel System (MESSy, based on version 2.51), Geosci. Model Dev., 9, 2209–2222, https://doi.org/10.5194/gmd-9-2209-2016, 2016. a
Etminan, M., Myhre, G., Highwood, E. J., and Shine, K. P.: Radiative forcing of carbon dioxide, methane, and nitrous oxide: A significant revision of the methane radiative forcing, Geophys. Res. Lett., 43, 12614–12623, https://doi.org/10.1002/2016GL071930, 2016. a, b, c
Eyring, V., Bony, S., Meehl, G. A., Senior, C. A., Stevens, B., Stouffer, R. J., and Taylor, K. E.: Overview of the Coupled Model Intercomparison Project Phase 6 (CMIP6) experimental design and organization, Geosci. Model Dev., 9, 1937–1958, https://doi.org/10.5194/gmd-9-1937-2016, 2016. a
Finney, D. L., Doherty, R. M., Wild, O., Young, P. J., and Butler, A.: Response of lightning NOx emissions and ozone production to climate change: Insights from the Atmospheric Chemistry and Climate Model Intercomparison Project, Geophys. Res. Lett., 43, 5492–5500, https://doi.org/10.1002/2016GL068825, 2016. a, b
Finney, D. L., Doherty, R. M., Wild, O., Stevenson, D. S., MacKenzie, I. A., and Blyth, A. M.: A projected decrease in lightning under climate change, Nat. Clim. Change, 8, 210–213, https://doi.org/10.1038/s41558-018-0072-6, 2018. a, b
Fiore, A. M., Dentener, F. J., Wild, O., Cuvelier, C., Schultz, M. G., Hess, P., Textor, C., Schulz, M., Doherty, R. M., Horowitz, L. W., MacKenzie, I. A., Sanderson, M. G., Shindell, D. T., Stevenson, D. S., Szopa, S., Van Dingenen, R., Zeng, G., Atherton, C., Bergmann, D., Bey, I., Carmichael, G., Collins, W. J., Duncan, B. N., Faluvegi, G., Folberth, G., Gauss, M., Gong, S., Hauglustaine, D., Holloway, T., Isaksen, I. S. A., Jacob, D. J., Jonson, J. E., Kaminski, J. W., Keating, T. J., Lupu, A., Marmer, E., Montanaro, V., Park, R. J., Pitari, G., Pringle, K. J., Pyle, J. A., Schroeder, S., Vivanco, M. G., Wind, P., Wojcik, G., Wu, S., and Zuber, A.: Multimodel estimates of intercontinental source-receptor relationships for ozone pollution, J. Geophys. Res.-Atmos., 114, D04301, https://doi.org/10.1029/2008JD010816, 2009. a, b, c
Folberth, G. A., Staniaszek, Z., Archibald, A. T., Gedney, N., Griffiths, P. T., Jones, C. D., O'Connor, F. M., Parker, R. J., Sellar, A. A., and Wiltshire, A.: Description and Evaluation of an Emission-Driven and Fully Coupled Methane Cycle in UKESM1, J. Adv. Model. Earth Syst., 14, e2021MS002982, https://doi.org/10.1029/2021MS002982, 2022. a
Forster, P., Storelvmo, T., Armour, K., Collins, W., Dufresne, J.-L., Frame, D., Lunt, D., Mauritsen, T., Palmer, M., Watanabe, M., Wild, M., and Zhang, H.: The Earth's Energy Budget, Climate Feedbacks, and Climate Sensitivity. Climate Change 2021: The Physical Science Basis. Contribution of Working Group I to the Sixth Assessment Report of the Intergovernmental Panel on Climate Change, edited by: Masson-Delmotte, V., Zhai, P., Pirani, A., Connors, S. L., Péan, C., Berger, S., Caud, N., Chen, Y., Goldfarb, L., Gomis, M. I., Huang, M., Leitzell, K., Lonnoy, E., Matthews, J. B. R., Maycock, T. K., Waterfield, T., Yelekçi, O., Yu, R., and Zhou, B., Cambridge University Press, Cambridge, United Kingdom and New York, NY, USA, 423–552, https://doi.org/10.1017/9781009157896.009, 2021. a, b, c
Forster, P. M., Richardson, T., Maycock, A. C., Smith, C. J., Samset, B. H., Myhre, G., Andrews, T., Pincus, R., and Schulz, M.: Recommendations for diagnosing effective radiative forcing from climate models for CMIP6, J. Geophys. Res.-Atmos., 121, 12460–12475, https://doi.org/10.1002/2016JD025320, 2016. a, b, c, d
Frank, F.: Atmospheric methane and its isotopic composition in a changing climate: A modeling study, PhD thesis, Ludwig-Maximilians-Universität München, https://doi.org/10.5282/edoc.22578, 2018. a
Garny, H., Dameris, M., Randel, W., Bodeker, G. E., and Deckert, R.: Dynamically Forced Increase of Tropical Upwelling in the Lower Stratosphere, J. Atmos. Sci., 68, 1214–1233, https://doi.org/10.1175/2011JAS3701.1, 2011. a
Giorgetta, M. A. and Bengtsson, L.: Potential role of the quasi-biennial oscillation in the stratosphere-troposphere exchange as found in water vapor in general circulation model experiments, J. Geophys. Res.-Atmos., 104, 6003–6019, https://doi.org/10.1029/1998JD200112, 1999. a
Granier, C., Bessagnet, B., Bond, T., D'Angiola, A., Denier van der Gon, H., Frost, G. J., Heil, A., Kaiser, J. W., Kinne, S., Klimont, Z., Kloster, S., Lamarque, J.-F., Liousse, C., Masui, T., Meleux, F., Mieville, A., Ohara, T., Raut, J.-C., Riahi, K., Schultz, M. G., Smith, S. J., Thompson, A., van Aardenne, J., van der Werf, G. R., and van Vuuren, D. P.: Evolution of anthropogenic and biomass burning emissions of air pollutants at global and regional scales during the 1980–2010 period, Climatic Change, 109, 163–190, https://doi.org/10.1007/s10584-011-0154-1, 2011. a
Grewe, V., Brunner, D., Dameris, M., Grenfell, J., Hein, R., Shindell, D., and Staehelin, J.: Origin and variability of upper tropospheric nitrogen oxides and ozone at northern mid-latitudes, Atmos. Environ., 35, 3421–3433, https://doi.org/10.1016/S1352-2310(01)00134-0, 2001. a
Grewe, V., Tsati, E., Mertens, M., Frömming, C., and Jöckel, P.: Contribution of emissions to concentrations: the TAGGING 1.0 submodel based on the Modular Earth Submodel System (MESSy 2.52), Geosci. Model Dev., 10, 2615–2633, https://doi.org/10.5194/gmd-10-2615-2017, 2017. a, b, c, d, e, f
Griffiths, P. T., Murray, L. T., Zeng, G., Shin, Y. M., Abraham, N. L., Archibald, A. T., Deushi, M., Emmons, L. K., Galbally, I. E., Hassler, B., Horowitz, L. W., Keeble, J., Liu, J., Moeini, O., Naik, V., O'Connor, F. M., Oshima, N., Tarasick, D., Tilmes, S., Turnock, S. T., Wild, O., Young, P. J., and Zanis, P.: Tropospheric ozone in CMIP6 simulations, Atmos. Chem. Phys., 21, 4187–4218, https://doi.org/10.5194/acp-21-4187-2021, 2021. a
Hansen, J., Sato, M., Ruedy, R., Nazarenko, L., Lacis, A., Schmidt, G. A., Russell, G., Aleinov, I., Bauer, M., Bauer, S., Bell, N., Cairns, B., Canuto, V., Chandler, M., Cheng, Y., Del Genio, A., Faluvegi, G., Fleming, E., Friend, A., Hall, T., Jackman, C., Kelley, M., Kiang, N., Koch, D., Lean, J., Lerner, J., Lo, K., Menon, S., Miller, R., Minnis, P., Novakov, T., Oinas, V., Perlwitz, J., Perlwitz, J., Rind, D., Romanou, A., Shindell, D., Stone, P., Sun, S., Tausnev, N., Thresher, D., Wielicki, B., Wong, T., Yao, M., and Zhang, S.: Efficacy of climate forcings, J. Geophys. Res.-Atmos., 110, D18104, https://doi.org/10.1029/2005JD005776, 2005. a, b, c, d
He, J., Naik, V., Horowitz, L. W., Dlugokencky, E., and Thoning, K.: Investigation of the global methane budget over 1980–2017 using GFDL-AM4.1, Atmos. Chem. Phys., 20, 805–827, https://doi.org/10.5194/acp-20-805-2020, 2020. a
Heimann, I., Griffiths, P. T., Warwick, N. J., Abraham, N. L., Archibald, A. T., and Pyle, J. A.: Methane Emissions in a Chemistry-Climate Model: Feedbacks and Climate Response, J. Adv. Model. Earth Syst., 12, e2019MS002019, https://doi.org/10.1029/2019MS002019, 2020. a, b, c, d
Heinze, C., Eyring, V., Friedlingstein, P., Jones, C., Balkanski, Y., Collins, W., Fichefet, T., Gao, S., Hall, A., Ivanova, D., Knorr, W., Knutti, R., Löw, A., Ponater, M., Schultz, M. G., Schulz, M., Siebesma, P., Teixeira, J., Tselioudis, G., and Vancoppenolle, M.: ESD Reviews: Climate feedbacks in the Earth system and prospects for their evaluation, Earth Syst. Dynam., 10, 379–452, https://doi.org/10.5194/esd-10-379-2019, 2019. a, b, c, d, e
Holmes, C. D.: Methane Feedback on Atmospheric Chemistry: Methods, Models, and Mechanisms, J. Adv. Model. Earth Syst., 10, 1087–1099, https://doi.org/10.1002/2017MS001196, 2018. a, b, c, d, e
Hoyer, S. and Hamman, J.: xarray: N-D labeled arrays and datasets in Python, Journal of Open Research Software, 5, 10, https://doi.org/10.5334/jors.148, 2017. a
Hunter, J. D.: Matplotlib: A 2D graphics environment, Comput. Sci. Eng., 9, 90–95, https://doi.org/10.1109/MCSE.2007.55, 2007. a
IPCC: Climate Change 2021: The Physical Science Basis. Contribution of Working Group I to the Sixth Assessment Report of the Intergovernmental Panel on Climate Change, edited by: Masson-Delmotte, V., Zhai, P., Pirani, A., Connors, S. L., Péan, C., Berger, S., Caud, N., Chen, Y., Goldfarb, L., Gomis, M. I., Huang, M., Leitzell, K., Lonnoy, E., Matthews, J. B. R., Maycock, T. K., Waterfield, T., Yelekçi, O., Yu, R., and Zhou, B., Cambridge University Press, Cambridge, United Kingdom and New York, NY, USA, https://doi.org/10.1017/9781009157896, 2021. a
Jöckel, P., Tost, H., Pozzer, A., Kunze, M., Kirner, O., Brenninkmeijer, C. A. M., Brinkop, S., Cai, D. S., Dyroff, C., Eckstein, J., Frank, F., Garny, H., Gottschaldt, K.-D., Graf, P., Grewe, V., Kerkweg, A., Kern, B., Matthes, S., Mertens, M., Meul, S., Neumaier, M., Nützel, M., Oberländer-Hayn, S., Ruhnke, R., Runde, T., Sander, R., Scharffe, D., and Zahn, A.: Earth System Chemistry integrated Modelling (ESCiMo) with the Modular Earth Submodel System (MESSy) version 2.51, Geosci. Model Dev., 9, 1153–1200, https://doi.org/10.5194/gmd-9-1153-2016, 2016. a, b, c, d, e, f
Kerkweg, A., Buchholz, J., Ganzeveld, L., Pozzer, A., Tost, H., and Jöckel, P.: Technical Note: An implementation of the dry removal processes DRY DEPosition and SEDImentation in the Modular Earth Submodel System (MESSy), Atmos. Chem. Phys., 6, 4617–4632, https://doi.org/10.5194/acp-6-4617-2006, 2006a. a
Kerkweg, A., Sander, R., Tost, H., and Jöckel, P.: Technical note: Implementation of prescribed (OFFLEM), calculated (ONLEM), and pseudo-emissions (TNUDGE) of chemical species in the Modular Earth Submodel System (MESSy), Atmos. Chem. Phys., 6, 3603–3609, https://doi.org/10.5194/acp-6-3603-2006, 2006b. a
Kunze, M., Godolt, M., Langematz, U., Grenfell, J., Hamann-Reinus, A., and Rauer, H.: Investigating the early Earth faint young Sun problem with a general circulation model, Planet. Space Sci., 98, 77–92, https://doi.org/10.1016/j.pss.2013.09.011, 2014. a
Kurtén, T., Zhou, L., Makkonen, R., Merikanto, J., Räisänen, P., Boy, M., Richards, N., Rap, A., Smolander, S., Sogachev, A., Guenther, A., Mann, G. W., Carslaw, K., and Kulmala, M.: Large methane releases lead to strong aerosol forcing and reduced cloudiness, Atmos. Chem. Phys., 11, 6961–6969, https://doi.org/10.5194/acp-11-6961-2011, 2011. a, b
Lamarque, J.-F., Bond, T. C., Eyring, V., Granier, C., Heil, A., Klimont, Z., Lee, D., Liousse, C., Mieville, A., Owen, B., Schultz, M. G., Shindell, D., Smith, S. J., Stehfest, E., Van Aardenne, J., Cooper, O. R., Kainuma, M., Mahowald, N., McConnell, J. R., Naik, V., Riahi, K., and van Vuuren, D. P.: Historical (1850–2000) gridded anthropogenic and biomass burning emissions of reactive gases and aerosols: methodology and application, Atmos. Chem. Phys., 10, 7017–7039, https://doi.org/10.5194/acp-10-7017-2010, 2010. a
Lan, X., Thoning, K., and Dlugokencky, E.: Trends in globally-averaged CH4, N2O, and SF6 determined from NOAA Global Monitoring Laboratory measurements, Version 2025-04, NOAA Global Monitoring Laboratory [data set], https://doi.org/10.15138/P8XG-AA10, 2022. a
Lawrence, M. G., Jöckel, P., and von Kuhlmann, R.: What does the global mean OH concentration tell us?, Atmos. Chem. Phys., 1, 37–49, https://doi.org/10.5194/acp-1-37-2001, 2001. a
Li, F. and Newman, P.: Prescribing stratospheric chemistry overestimates southern hemisphere climate change during austral spring in response to quadrupled CO2, Clim. Dynam., 61, 1105–1122, https://doi.org/10.1007/s00382-022-06588-4, 2023. a
Liang, Q., Stolarski, R. S., Kawa, S. R., Nielsen, J. E., Douglass, A. R., Rodriguez, J. M., Blake, D. R., Atlas, E. L., and Ott, L. E.: Finding the missing stratospheric Bry: a global modeling study of CHBr3 and CH2Br2, Atmos. Chem. Phys., 10, 2269–2286, https://doi.org/10.5194/acp-10-2269-2010, 2010. a
Marsh, D. R., Lamarque, J.-F., Conley, A. J., and Polvani, L. M.: Stratospheric ozone chemistry feedbacks are not critical for the determination of climate sensitivity in CESM1(WACCM), Geophys. Res. Lett., 43, 3928–3934, https://doi.org/10.1002/2016GL068344, 2016. a, b, c, d, e, f, g, h, i
Meinshausen, M., Smith, S. J., Calvin, K., Daniel, J. S., Kainuma, M. L. T., Lamarque, J.-F., Matsumoto, K., Montzka, S. A., Raper, S. C. B., Riahi, K., Thomson, A., Velders, G. J. M., and van Vuuren, D. P.: The RCP greenhouse gas concentrations and their extensions from 1765 to 2300, Climatic Change, 109, 1573–1480, https://doi.org/10.1007/s10584-011-0156-z, 2011. a
Meinshausen, M., Vogel, E., Nauels, A., Lorbacher, K., Meinshausen, N., Etheridge, D. M., Fraser, P. J., Montzka, S. A., Rayner, P. J., Trudinger, C. M., Krummel, P. B., Beyerle, U., Canadell, J. G., Daniel, J. S., Enting, I. G., Law, R. M., Lunder, C. R., O'Doherty, S., Prinn, R. G., Reimann, S., Rubino, M., Velders, G. J. M., Vollmer, M. K., Wang, R. H. J., and Weiss, R.: Historical greenhouse gas concentrations for climate modelling (CMIP6), Geosci. Model Dev., 10, 2057–2116, https://doi.org/10.5194/gmd-10-2057-2017, 2017. a
Met Office: HadISST1, Met Office [data set], https://www.metoffice.gov.uk/hadobs/hadisst/data/download.html (last access: 7 May 2025), 2025. a
Modak, A., Bala, G., Caldeira, K., and Cao, L.: Does shortwave absorption by methane influence its effectiveness?, Clim. Dynam., 51, 3653–3672, https://doi.org/10.1007/s00382-018-4102-x, 2018. a, b
Nowack, P. J., Luke Abraham, N., Maycock, A. C., Braesicke, P., Gregory, J. M., Joshi, M. M., Osprey, A., and Pyle, J. A.: A large ozone-circulation feedback and its implications for global warming assessments, Nat. Clim. Change, 5, 41–45, https://doi.org/10.1038/nclimate2451, 2015. a, b, c, d, e, f, g, h, i
Nowack, P. J., Abraham, N. L., Braesicke, P., and Pyle, J. A.: The Impact of Stratospheric Ozone Feedbacks on Climate Sensitivity Estimates, J. Geophys. Res.-Atmos., 123, 4630–4641, https://doi.org/10.1002/2017JD027943, 2018. a, b, c, d
Nützel, M., Stecher, L., Jöckel, P., Winterstein, F., Dameris, M., Ponater, M., Graf, P., and Kunze, M.: Updating the radiation infrastructure in MESSy (based on MESSy version 2.55), Geosci. Model Dev., 17, 5821–5849, https://doi.org/10.5194/gmd-17-5821-2024, 2024. a, b, c, d, e, f, g
Nuvolone, D., Petri, D., and Voller, F.: The effects of ozone on human health, Environ. Sci. Pollut. Res., 25, 8074–8088, https://doi.org/10.1007/s11356-017-9239-3, 2018. a
Ocko, I. B., Sun, T., Shindell, D., Oppenheimer, M., Hristov, A. N., Pacala, S. W., Mauzerall, D. L., Xu, Y., and Hamburg, S. P.: Acting rapidly to deploy readily available methane mitigation measures by sector can immediately slow global warming, Environ. Res. Lett., 16, 054042, https://doi.org/10.1088/1748-9326/abf9c8, 2021. a
O'Connor, F. M., Boucher, O., Gedney, N., Jones, C. D., Folberth, G. A., Coppell, R., Friedlingstein, P., Collins, W. J., Chappellaz, J., Ridley, J., and Johnson, C. E.: Possible role of wetlands, permafrost, and methane hydrates in the methane cycle under future climate change: A review, Rev. Geophys., 48, RG4005, https://doi.org/10.1029/2010RG000326, 2010. a, b
O'Connor, F. M., Johnson, B. T., Jamil, O., Andrews, T., Mulcahy, J. P., and Manners, J.: Apportionment of the Pre-Industrial to Present-Day Climate Forcing by Methane Using UKESM1: The Role of the Cloud Radiative Effect, J. Adv. Model. Earth Syst., 14, e2022MS002991, https://doi.org/10.1029/2022MS002991, 2022. a, b, c
Pincus, R. and Stevens, B.: Paths to accuracy for radiation parameterizations in atmospheric models, J. Adv. Model. Earth Syst., 5, 225–233, https://doi.org/10.1002/jame.20027, 2013. a, b, c
Portmann, R. W. and Solomon, S.: Indirect radiative forcing of the ozone layer during the 21st century, Geophys. Res. Lett., 34, L02813, https://doi.org/10.1029/2006GL028252, 2007. a, b, c
Pozzer, A., Jöckel, P., Sander, R., Williams, J., Ganzeveld, L., and Lelieveld, J.: Technical Note: The MESSy-submodel AIRSEA calculating the air-sea exchange of chemical species, Atmos. Chem. Phys., 6, 5435–5444, https://doi.org/10.5194/acp-6-5435-2006, 2006. a
Prather, M. J., Holmes, C. D., and Hsu, J.: Reactive greenhouse gas scenarios: Systematic exploration of uncertainties and the role of atmospheric chemistry, Geophys. Res. Lett., 39, L09803, https://doi.org/10.1029/2012GL051440, 2012. a, b
Ramaswamy, V., Collins, W., Haywood, J., Lean, J., Mahowald, N., Myhre, G., Naik, V., Shine, K. P., Soden, B., Stenchikov, G., and Storelvmo, T.: Radiative Forcing of Climate: The Historical Evolution of the Radiative Forcing Concept, the Forcing Agents and their Quantification, and Applications, Meteorol. Monog., 59, 14.1–14.101, https://doi.org/10.1175/AMSMONOGRAPHS-D-19-0001.1, 2018. a
Rayner, N. A., Parker, D. E., Horton, E. B., Folland, C. K., Alexander, L. V., Rowell, D. P., Kent, E. C., and Kaplan, A.: Global analyses of sea surface temperature, sea ice, and night marine air temperature since the late nineteenth century, J. Geophys. Res.-Atmos., 108, 4407, https://doi.org/10.1029/2002JD002670, 2003. a, b, c, d
Richardson, T. B., Forster, P. M., Smith, C. J., Maycock, A. C., Wood, T., Andrews, T., Boucher, O., Faluvegi, G., Fläschner, D., Hodnebrog, Ø., Kasoar, M., Kirkevåg, A., Lamarque, J.-F., Mülmenstädt, J., Myhre, G., Olivié, D., Portmann, R. W., Samset, B. H., Shawki, D., Shindell, D., Stier, P., Takemura, T., Voulgarakis, A., and Watson-Parris, D.: Efficacy of Climate Forcings in PDRMIP Models, J. Geophys. Res.-Atmos., 124, 12824–12844, https://doi.org/10.1029/2019JD030581, 2019. a, b, c, d, e
Rieger, V. S., Mertens, M., and Grewe, V.: An advanced method of contributing emissions to short-lived chemical species (OH and HO2): the TAGGING 1.1 submodel based on the Modular Earth Submodel System (MESSy 2.53), Geosci. Model Dev., 11, 2049–2066, https://doi.org/10.5194/gmd-11-2049-2018, 2018. a, b, c, d
Rind, D., Shindell, D., Lonergan, P., and Balachandran, N. K.: Climate Change and the Middle Atmosphere. Part III: The Doubled CO2 Climate Revisited, J. Climate, 11, 876–894, https://doi.org/10.1175/1520-0442(1998)011<0876:CCATMA>2.0.CO;2, 1998. a
Roeckner, E., Siebert, T., and Feichter, J.: Climatic response to anthropogenic sulfate forcing simulated with a general circulation model, in: Aerosol forcing of climate: Report of the Dahlem Workshop on Aerosol Forcing of Climate, Berlin, Germany, 24–29 April 1994, Wiley, Chichester, 349–362, https://hdl.handle.net/21.11116/0000-0009-0FE0-A (last access: 29 April 2025), 1995. a
Roeckner, E., Bäuml, G., Bonaventura, L., Brokopf, R., Esch, M., Giorgetta, M., Hagemann, S., Kirchner, I., Kornblueh, L., Manzini, E., Rhodin, A., Schlese, U., Schulzweida, U., and Tompkins, A.: The atmospheric general circulation model ECHAM5. PART I: Model description, Report/Max-Planck-Institut für Meteorologie, 349, https://hdl.handle.net/11858/00-001M-0000-0012-0144-5 (last access: 29 April 2025), 2003. a
Rosenfield, J. E., Douglass, A. R., and Considine, D. B.: The impact of increasing carbon dioxide on ozone recovery, J. Geophys. Res.-Atmos., 107, ACH 7-1–ACH 7-9, https://doi.org/10.1029/2001JD000824, 2002. a, b, c
Sand, M., Skeie, R. B., Sandstad, M., Krishnan, S., Myhre, G., Bryant, H., Derwent, R., Hauglustaine, D., Paulot, F., Prather, M., and Stevenson, D.: A multi-model assessment of the Global Warming Potential of hydrogen, Communications Earth & Environment, 4, 203, https://doi.org/10.1038/s43247-023-00857-8, 2023. a, b
Sander, R., Jöckel, P., Kirner, O., Kunert, A. T., Landgraf, J., and Pozzer, A.: The photolysis module JVAL-14, compatible with the MESSy standard, and the JVal PreProcessor (JVPP), Geosci. Model Dev., 7, 2653–2662, https://doi.org/10.5194/gmd-7-2653-2014, 2014. a
Sander, R., Baumgaertner, A., Cabrera-Perez, D., Frank, F., Gromov, S., Grooß, J.-U., Harder, H., Huijnen, V., Jöckel, P., Karydis, V. A., Niemeyer, K. E., Pozzer, A., Riede, H., Schultz, M. G., Taraborrelli, D., and Tauer, S.: The community atmospheric chemistry box model CAABA/MECCA-4.0, Geosci. Model Dev., 12, 1365–1385, https://doi.org/10.5194/gmd-12-1365-2019, 2019. a
Saunois, M., Jackson, R. B., Bousquet, P., Poulter, B., and Canadell, J. G.: The growing role of methane in anthropogenic climate change, Environ. Res. Lett., 11, 120207, https://doi.org/10.1088/1748-9326/11/12/120207, 2016. a
Saunois, M., Stavert, A. R., Poulter, B., Bousquet, P., Canadell, J. G., Jackson, R. B., Raymond, P. A., Dlugokencky, E. J., Houweling, S., Patra, P. K., Ciais, P., Arora, V. K., Bastviken, D., Bergamaschi, P., Blake, D. R., Brailsford, G., Bruhwiler, L., Carlson, K. M., Carrol, M., Castaldi, S., Chandra, N., Crevoisier, C., Crill, P. M., Covey, K., Curry, C. L., Etiope, G., Frankenberg, C., Gedney, N., Hegglin, M. I., Höglund-Isaksson, L., Hugelius, G., Ishizawa, M., Ito, A., Janssens-Maenhout, G., Jensen, K. M., Joos, F., Kleinen, T., Krummel, P. B., Langenfelds, R. L., Laruelle, G. G., Liu, L., Machida, T., Maksyutov, S., McDonald, K. C., McNorton, J., Miller, P. A., Melton, J. R., Morino, I., Müller, J., Murguia-Flores, F., Naik, V., Niwa, Y., Noce, S., O'Doherty, S., Parker, R. J., Peng, C., Peng, S., Peters, G. P., Prigent, C., Prinn, R., Ramonet, M., Regnier, P., Riley, W. J., Rosentreter, J. A., Segers, A., Simpson, I. J., Shi, H., Smith, S. J., Steele, L. P., Thornton, B. F., Tian, H., Tohjima, Y., Tubiello, F. N., Tsuruta, A., Viovy, N., Voulgarakis, A., Weber, T. S., van Weele, M., van der Werf, G. R., Weiss, R. F., Worthy, D., Wunch, D., Yin, Y., Yoshida, Y., Zhang, W., Zhang, Z., Zhao, Y., Zheng, B., Zhu, Q., Zhu, Q., and Zhuang, Q.: The Global Methane Budget 2000–2017, Earth Syst. Sci. Data, 12, 1561–1623, https://doi.org/10.5194/essd-12-1561-2020, 2020. a
Schulzweida, U.: CDO User Guide (2.3.0), Zenodo, https://doi.org/10.5281/zenodo.10020800, 2023. a
Shindell, D., Kuylenstierna, J. C. I., Vignati, E., van Dingenen, R., Amann, M., Klimont, Z., Anenberg, S. C., Muller, N., Janssens-Maenhout, G., Raes, F., Schwartz, J., Faluvegi, G., Pozzoli, L., Kupiainen, K., Höglund-Isaksson, L., Emberson, L., Streets, D., Ramanathan, V., Hicks, K., Oanh, N. T. K., Milly, G., Williams, M., Demkine, V., and Fowler, D.: Simultaneously Mitigating Near-Term Climate Change and Improving Human Health and Food Security, Science, 335, 183–189, https://doi.org/10.1126/science.1210026, 2012. a
Shindell, D. T., Faluvegi, G., Bell, N., and Schmidt, G. A.: An emissions-based view of climate forcing by methane and tropospheric ozone, Geophys. Res. Lett., 32, L04803, https://doi.org/10.1029/2004GL021900, 2005. a
Shindell, D. T., Faluvegi, G., Koch, D. M., Schmidt, G. A., Unger, N., and Bauer, S. E.: Improved Attribution of Climate Forcing to Emissions, Science, 326, 716–718, https://doi.org/10.1126/science.1174760, 2009. a
Shindell, D. T., Pechony, O., Voulgarakis, A., Faluvegi, G., Nazarenko, L., Lamarque, J.-F., Bowman, K., Milly, G., Kovari, B., Ruedy, R., and Schmidt, G. A.: Interactive ozone and methane chemistry in GISS-E2 historical and future climate simulations, Atmos. Chem. Phys., 13, 2653–2689, https://doi.org/10.5194/acp-13-2653-2013, 2013. a
Shine, K. P., Cook, J., Highwood, E. J., and Joshi, M. M.: An alternative to radiative forcing for estimating the relative importance of climate change mechanisms, Geophys. Res. Lett., 30, 2047, https://doi.org/10.1029/2003GL018141, 2003. a
Sitch, S., Cox, P. M., Collins, W. J., and Huntingford, C.: Indirect radiative forcing of climate change through ozone effects on the land-carbon sink, Nature, 448, 791–794, https://doi.org/10.1038/nature06059, 2007. a
Smith, C. J., Kramer, R. J., Myhre, G., Forster, P. M., Soden, B., Andrews, T., Boucher, O., Faluvegi, G., Fläschner, D., Hodnebrog, Ø., Kasoar, M., Kharin, V., Kirkevåg, A., Lamarque, J.-F., Mülmenstädt, J., Olivié, D., Richardson, T., Samset, B. H., Shindell, D., Stier, P., Takemura, T., Voulgarakis, A., and Watson-Parris, D.: Understanding Rapid Adjustments to Diverse Forcing Agents, Geophys. Res. Lett., 45, 12023–12031, https://doi.org/10.1029/2018GL079826, 2018. a, b, c, d
Spahni, R., Wania, R., Neef, L., van Weele, M., Pison, I., Bousquet, P., Frankenberg, C., Foster, P. N., Joos, F., Prentice, I. C., and van Velthoven, P.: Constraining global methane emissions and uptake by ecosystems, Biogeosciences, 8, 1643–1665, https://doi.org/10.5194/bg-8-1643-2011, 2011. a
Staniaszek, Z., Griffiths, P. T., Folberth, G. A., O'Connor, F. M., Abraham, N. L., and Archibald, A. T.: The role of future anthropogenic methane emissions in air quality and climate, npj Clim. Atmos. Sci., 5, 21, https://doi.org/10.1038/s41612-022-00247-5, 2022. a, b, c
Stecher, L.: The role of methane for chemistry-climate interactions, Dissertation, Faculty of Physics, LMU München, https://doi.org/10.5282/edoc.33812, 2024. a, b
Stecher, L., Winterstein, F., Dameris, M., Jöckel, P., Ponater, M., and Kunze, M.: Slow feedbacks resulting from strongly enhanced atmospheric methane mixing ratios in a chemistry–climate model with mixed-layer ocean, Atmos. Chem. Phys., 21, 731–754, https://doi.org/10.5194/acp-21-731-2021, 2021. a, b, c, d, e, f, g, h, i, j, k, l, m, n, o, p, q, r, s, t, u
Stecher, L., Winterstein, F., Jöckel, P., Ponater, M., Mertens, M., and Dameris, M.: EMAC simulations of project IRFAM-ClimS with ECHAM5 radiation and interactive chemistry, DOKU at DKRZ [data set], https://www.wdc-climate.de/ui/entry?acronym=DKRZ_LTA_1132_ds00001 (last access: 29 April 2025), 2024. a
Stevenson, D. S., Dentener, F. J., Schultz, M. G., Ellingsen, K., van Noije, T. P. C., Wild, O., Zeng, G., Amann, M., Atherton, C. S., Bell, N., Bergmann, D. J., Bey, I., Butler, T., Cofala, J., Collins, W. J., Derwent, R. G., Doherty, R. M., Drevet, J., Eskes, H. J., Fiore, A. M., Gauss, M., Hauglustaine, D. A., Horowitz, L. W., Isaksen, I. S. A., Krol, M. C., Lamarque, J.-F., Lawrence, M. G., Montanaro, V., Müller, J.-F., Pitari, G., Prather, M. J., Pyle, J. A., Rast, S., Rodriguez, J. M., Sanderson, M. G., Savage, N. H., Shindell, D. T., Strahan, S. E., Sudo, K., and Szopa, S.: Multimodel ensemble simulations of present-day and near-future tropospheric ozone, J. Geophys. Res.-Atmos., 111, D08301, https://doi.org/10.1029/2005JD006338, 2006. a, b, c, d
Stevenson, D. S., Young, P. J., Naik, V., Lamarque, J.-F., Shindell, D. T., Voulgarakis, A., Skeie, R. B., Dalsoren, S. B., Myhre, G., Berntsen, T. K., Folberth, G. A., Rumbold, S. T., Collins, W. J., MacKenzie, I. A., Doherty, R. M., Zeng, G., van Noije, T. P. C., Strunk, A., Bergmann, D., Cameron-Smith, P., Plummer, D. A., Strode, S. A., Horowitz, L., Lee, Y. H., Szopa, S., Sudo, K., Nagashima, T., Josse, B., Cionni, I., Righi, M., Eyring, V., Conley, A., Bowman, K. W., Wild, O., and Archibald, A.: Tropospheric ozone changes, radiative forcing and attribution to emissions in the Atmospheric Chemistry and Climate Model Intercomparison Project (ACCMIP), Atmos. Chem. Phys., 13, 3063–3085, https://doi.org/10.5194/acp-13-3063-2013, 2013. a, b, c
Stevenson, D. S., Zhao, A., Naik, V., O'Connor, F. M., Tilmes, S., Zeng, G., Murray, L. T., Collins, W. J., Griffiths, P. T., Shim, S., Horowitz, L. W., Sentman, L. T., and Emmons, L.: Trends in global tropospheric hydroxyl radical and methane lifetime since 1850 from AerChemMIP, Atmos. Chem. Phys., 20, 12905–12920, https://doi.org/10.5194/acp-20-12905-2020, 2020. a, b, c, d, e, f, g
Stuber, N., Sausen, R., and Ponater, M.: Stratosphere adjusted radiative forcing calculations in a comprehensive climate model, Theor. Appl. Climatol., 68, 125–135, https://doi.org/10.1007/s007040170041, 2001. a, b
The MESSy Consortium: The Modular Earth Submodel System, Zenodo [code], https://doi.org/10.5281/zenodo.8360276, 2021. a, b
Thornhill, G., Collins, W., Olivié, D., Skeie, R. B., Archibald, A., Bauer, S., Checa-Garcia, R., Fiedler, S., Folberth, G., Gjermundsen, A., Horowitz, L., Lamarque, J.-F., Michou, M., Mulcahy, J., Nabat, P., Naik, V., O'Connor, F. M., Paulot, F., Schulz, M., Scott, C. E., Séférian, R., Smith, C., Takemura, T., Tilmes, S., Tsigaridis, K., and Weber, J.: Climate-driven chemistry and aerosol feedbacks in CMIP6 Earth system models, Atmos. Chem. Phys., 21, 1105–1126, https://doi.org/10.5194/acp-21-1105-2021, 2021a. a, b, c, d, e, f, g, h, i, j, k, l, m, n, o, p, q, r, s
Thornhill, G. D., Collins, W. J., Kramer, R. J., Olivié, D., Skeie, R. B., O'Connor, F. M., Abraham, N. L., Checa-Garcia, R., Bauer, S. E., Deushi, M., Emmons, L. K., Forster, P. M., Horowitz, L. W., Johnson, B., Keeble, J., Lamarque, J.-F., Michou, M., Mills, M. J., Mulcahy, J. P., Myhre, G., Nabat, P., Naik, V., Oshima, N., Schulz, M., Smith, C. J., Takemura, T., Tilmes, S., Wu, T., Zeng, G., and Zhang, J.: Effective radiative forcing from emissions of reactive gases and aerosols – a multi-model comparison, Atmos. Chem. Phys., 21, 853–874, https://doi.org/10.5194/acp-21-853-2021, 2021b. a, b, c, d
Tost, H., Jöckel, P., and Lelieveld, J.: Lightning and convection parameterisations – uncertainties in global modelling, Atmos. Chem. Phys., 7, 4553–4568, https://doi.org/10.5194/acp-7-4553-2007, 2007. a
Vella, R., Forrest, M., Lelieveld, J., and Tost, H.: Isoprene and monoterpene simulations using the chemistry–climate model EMAC (v2.55) with interactive vegetation from LPJ-GUESS (v4.0), Geosci. Model Dev., 16, 885–906, https://doi.org/10.5194/gmd-16-885-2023, 2023. a
Voulgarakis, A., Naik, V., Lamarque, J.-F., Shindell, D. T., Young, P. J., Prather, M. J., Wild, O., Field, R. D., Bergmann, D., Cameron-Smith, P., Cionni, I., Collins, W. J., Dalsøren, S. B., Doherty, R. M., Eyring, V., Faluvegi, G., Folberth, G. A., Horowitz, L. W., Josse, B., MacKenzie, I. A., Nagashima, T., Plummer, D. A., Righi, M., Rumbold, S. T., Stevenson, D. S., Strode, S. A., Sudo, K., Szopa, S., and Zeng, G.: Analysis of present day and future OH and methane lifetime in the ACCMIP simulations, Atmos. Chem. Phys., 13, 2563–2587, https://doi.org/10.5194/acp-13-2563-2013, 2013. a, b, c, d, e, f, g, h, i, j, k, l, m, n, o, p, q, r, s, t
Wang, H., Lu, X., Seco, R., Stavrakou, T., Karl, T., Jiang, X., Gu, L., and Guenther, A. B.: Modeling Isoprene Emission Response to Drought and Heatwaves Within MEGAN Using Evapotranspiration Data and by Coupling With the Community Land Model, J. Adv. Model. Earth Sy., 14, e2022MS003174, https://doi.org/10.1029/2022MS003174, 2022. a
Warwick, N. J., Pyle, J. A., Carver, G. D., Yang, X., Savage, N. H., O'Connor, F. M., and Cox, R. A.: Global modeling of biogenic bromocarbons, J. Geophys. Res.-Atmos., 111, D24305, https://doi.org/10.1029/2006JD007264, 2006. a
Winterstein, F., Tanalski, F., Jöckel, P., Dameris, M., and Ponater, M.: Implication of strongly increased atmospheric methane concentrations for chemistry–climate connections, Atmos. Chem. Phys., 19, 7151–7163, https://doi.org/10.5194/acp-19-7151-2019, 2019. a, b, c, d, e, f, g, h, i, j, k, l, m, n, o, p, q, r, s, t, u, v, w
WMO: WMO Greenhouse Gas Bulletin (GHG Bulletin) – No. 18: The State of Greenhouse Gases in the Atmosphere Based on Global Observations through 2021, WMO, https://library.wmo.int/idurl/4/58743 (last access: 29 April 2025), 2022. a
Zanis, P., Akritidis, D., Turnock, S., Naik, V., Szopa, S., Georgoulias, A. K., Bauer, S. E., Deushi, M., Horowitz, L. W., Keeble, J., Sager, P. L., O'Connor, F. M., Oshima, N., Tsigaridis, K., and van Noije, T.: Climate change penalty and benefit on surface ozone: a global perspective based on CMIP6 earth system models, Environ. Res. Lett., 17, 024014, https://doi.org/10.1088/1748-9326/ac4a34, 2022. a, b, c, d, e
Zender, C. S.: Analysis of Self-describing Gridded Geoscience Data with netCDF Operators (NCO), Environ. Modell. Softw., 23, 1338–1342, https://doi.org/10.1016/j.envsoft.2008.03.004, 2008. a
Zhou, S. S., Tai, A. P. K., Sun, S., Sadiq, M., Heald, C. L., and Geddes, J. A.: Coupling between surface ozone and leaf area index in a chemical transport model: strength of feedback and implications for ozone air quality and vegetation health, Atmos. Chem. Phys., 18, 14133–14148, https://doi.org/10.5194/acp-18-14133-2018, 2018. a
Relative estimates were calculated from estimates given in Tables 1 and 4 of Voulgarakis et al. (2013).
Multi-model mean changes in GSAT were calculated from the estimates given in Table 4 of Voulgarakis et al. (2013).
The CH4 mixing ratios of simulation ERFCH4 are outside of the range tested to derive the formula of Etminan et al. (2016), but the formula can still provide a rough estimate for the CH4 radiative effect.
If the model CESM2-WACCM, which projects a prolongation of CH4 lifetime with climate change, is excluded, the CH4 feedback is estimated at −0.053 ± 0.010 W m−2 K−1. The given uncertainties are standard deviations across models.