the Creative Commons Attribution 4.0 License.
the Creative Commons Attribution 4.0 License.
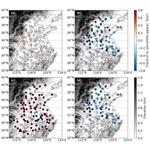
Opposing trends in the peak and low ozone concentrations in eastern China: anthropogenic and meteorological influences
Zhuang Wang
Chune Shi
Hao Zhang
Xianguang Ji
Yizhi Zhu
Congzi Xia
Xiaoyun Sun
Xinfeng Lin
Shaowei Yan
Suyao Wang
Yuan Zhou
Yujia Chen
Due to considerable reductions in nitrogen oxides (NOx), ozone trends and variations in eastern China remain inadequately understood. Long-term observations of ozone precursors were conducted to explore the factors influencing ozone trends in this region. Combined with satellite and surface measurements, we evaluated the trends in low (2nd percentile), typical (50th percentile), and peak (98th percentile) ozone concentrations in detail. Observations indicate a significant decrease in peak ozone concentrations (−0.5 % yr−1), alongside an increase in low ozone concentrations (0.3 % yr−1), across eastern China during May–September from 2017 to 2022. The decline in typical ozone concentrations is notably slower than that of peak ozone concentrations, which is approximately −0.02 ppb yr−1 (−0.0 % yr−1) during the same period. Anthropogenic emissions primarily drive trends in low and peak ozone concentrations in eastern China, though meteorological effects also play a role. Ozone formation sensitivity shifts from volatile organic compound (VOC)-limited or transitional regimes in the morning (08:00–11:00 local time, LT), when ozone concentrations rise sharply, to NOx-limited regimes around peak concentrations (∼ 14:00 LT). The reduction in NOx concentrations is identified as a key factor driving the decline in peak ozone concentrations, aiming to further reduce ozone exceedance days. Thus, controlling NOx concentrations emerges as crucial for mitigating peak ozone levels. Moreover, the increase in low ozone concentrations can also be attributed to both anthropogenic emissions and meteorological factors. Our findings underscore the beneficial impacts of NOx reduction on managing peak ozone levels. Regular changes in ozone formation sensitivity throughout the day should be considered when formulating effective ozone control policies.
- Article
(9392 KB) - Full-text XML
-
Supplement
(1917 KB) - BibTeX
- EndNote
In recent decades, China's rapid industrialization and urbanization have yielded substantial economic benefits but have also brought about serious environmental challenges (Li et al., 2018; Song et al., 2023; Z. Wang et al., 2023). Air pollutants, notably ozone (O3; in warm seasons) and fine particulate matter (PM2.5; in cold seasons), have been the primary targets of China's air quality improvement efforts (Zhang and Cao, 2015; Xing et al., 2024, 2022; Wang et al., 2020; Bauwens et al., 2022). To combat this severe air pollution, the Chinese government initiated the Clean Air Action Plan in 2013 (State Council of China, 2013), resulting in significant reductions in most air pollutant concentrations. The PM2.5 annual average concentrations were reduced by 30 % to 50 % from 2013 to 2018 (Zhai et al., 2019), while nitrogen oxide (NOx) and carbon monoxide (CO) emissions dropped by 21 % and 23 %, respectively, from 2013 to 2017 (Zheng et al., 2018). However, O3 concentrations showed a yearly increase of 3.3 ± 4.7 from 2015 to 2019 (Mousavinezhad et al., 2021). Subsequently, the second phase of the Clean Air Action Plan was launched in 2018 (State Council of China, 2018), focusing on additional controls for O3 emissions. Driven by both anthropogenic activities and meteorological patterns, the upward trend in O3 levels in eastern China persisted through at least 2019 (K. Li et al., 2020), making the study of O3 formation, sources, and trends in densely populated areas a topic of increasing global concern (Y. Wang et al., 2023b).
Ozone forms rapidly in polluted air through the photochemical oxidation of volatile organic compounds (VOCs) in the presence of NOx () (Li et al., 2022; Cooper et al., 2012). Ambient O3 concentrations are influenced by various factors, including precursor levels (Y. Wang et al., 2022; Ding et al., 2023), local meteorological conditions (Han et al., 2020), regional transport (Lang et al., 2021; Y. Wang et al., 2023a), and deposition (Wu et al., 2023). Elevated O3 levels can have significant impacts on human health, ecosystems, and climate change, leading to substantial economic losses (Guan et al., 2021; Gao et al., 2022). The formation sensitivity of O3 (VOC-limited, transition, and NOx-limited regimes) in a region depends on the relative abundance of VOCs and NOx and their competition for OH radicals, highlighting the importance of controlling both VOCs and NOx to manage O3 production and removal effectively (J. Ren et al., 2022). While NOx concentrations have significantly declined in eastern China since 2013 (Lin et al., 2019), anthropogenic VOC emissions continued to increase until 2019 (Zheng et al., 2018; Bauwens et al., 2022).
Numerous studies have investigated the drivers of the increasing O3 trend in China over the past decade, particularly from 2013 to 2017 (K. Li et al., 2020; Lu et al., 2020; Liu et al., 2023). Changes in meteorological conditions and anthropogenic emissions have been identified as the primary causes, with observations and models concluding that anthropogenic impacts dominate the increasing trend of summer O3 in China. For instance, K. Li et al. (2020) found that the increase in surface O3 in the North China Plain (NCP) from 2013 to 2019 was more influenced by changes in emissions (1.2 ppb yr−1) than meteorological changes (0.7 ppb yr−1). Additionally, the decrease in PM2.5, mainly achieved by reducing the scavenging of hydroxyl (HO2) radicals on the aerosol surface, has been found to be crucial for the increase in O3 (Li et al., 2018). However, the implications of the heterogeneous uptake of HO2 radicals remain debated. These studies have largely focused on a single O3 indicator, and due to the recent NOx reduction, the reasons for the variations in O3 trends in eastern China, particularly the low and peak O3 trends, are not fully understood. This poses a significant challenge for controlling O3 pollution and developing effective strategies for sustained air quality improvement.
This study aims to address two key issues by combining extensive ground-based and satellite observations (1) to reveal the surface low, typical, and peak O3 trends in eastern Chinese cities in recent years and (2) to explore the driving forces behind these trends. First, we report long-term records of surface O3 and related parameters observed at urban air quality monitoring sites and by satellites in eastern China, characterizing the trends of low, typical, and peak surface O3 concentrations during the warm season (May–September) from 2017 to 2022. Then, a multiple linear regression (MLR) model is used to evaluate the anthropogenic and meteorological contributions to the 98th and 2nd O3 percentile trends. Next, secondary formaldehyde (HCHO) and NO2 are employed to diagnose the diurnal variations in O3 formation sensitivity and investigate the reasons for peak O3 concentration trends in the context of current NOx reduction. Finally, we discuss the reasons for the potential increase in low O3 concentrations and the sensitivity of peak and low O3 trends during the study period.
2.1 Surface measurements
The densely populated areas in eastern China mainly include the NCP and the middle and lower Yangtze River Plain (MLYRP), which are both vast and economically developed. It is one of the most polluted areas in China (Mousavinezhad et al., 2021). In this study, O3 trends in 105 cities in eastern China were investigated, and the spatial distribution of 105 cities in eastern China is shown in Fig. 1a. Real-time hourly observed urban O3, NO2, and CO concentrations in 105 cities in eastern China from 2017 to 2022 were obtained from the open website of the Ministry of Ecology and Environment of China (MEE; https://www.mee.gov.cn; last access: 7 January 2024), with the archive at https://quotsoft.net/air/ (last access: 7 January 2024). As of 31 August 2018, MEE reported concentrations (in µg m−3) under standard conditions (273 K; 1013 hPa). The reference status changed to 298 K and 1013 hPa on 1 September 2018. To facilitate the analysis of the long-term series, the mass concentrations (µg m−3) of O3, NO2, and CO at each site were converted to volume mixing ratios (VMRs; ppb) to eliminate the effect of these changes on trend calculations. Data quality control methods and the calculation of daily maximum 8 h O3 (MDA8 O3) concentrations were implemented according to the statistical requirements of the Technical Regulations for Environmental Air Quality Evaluation (Trial) (HJ633–2013). According to the Technical Regulations of the China Ambient Air Quality Index (AQI) (HJ633–2012), a maximum daily 8 h average (MDA8) O3 concentration greater than 160 µg m−3 is defined as O3 exceedance days; otherwise, it is an O3 normal day. Note that all times mentioned in this study refer to local time.
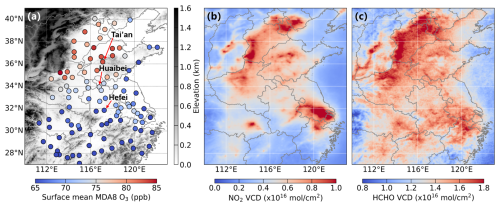
Figure 1Spatial distributions of O3 and its precursors in eastern China. (a) Spatial distributions of surface mean MDA8 O3 concentrations during May–September 2017–2022. The red arrow indicates the name of each city which is equipped with ground-based MAX-DOAS observations. Spatial distributions of tropospheric mean (b) NO2 and (c) HCHO VCDs during May–September 2018–2022.
2.2 MAX-DOAS measurements
There were 3 typical cities selected from the 105 cities in eastern China to be representative and to conduct multi-axis differential optical absorption spectroscopy (MAX-DOAS) observations, namely Hefei, Huaibei, and Tai'an. First, the three cities are located at similar longitudes, with large differences in latitude, transitioning sequentially from south to north (Fig. 1a). Second, the O3 concentrations of the three cities differed greatly, with Tai'an having a higher surface mean MDA8 O3 concentration (82.9 ppb), Hefei having a lower surface mean MDA8 O3 concentration (65.5 ppb), and Huaibei having an intermediate surface mean MDA8 O3 concentration (74.3 ppb). The three observation stations in Hefei (31.827° N, 117.233° E), Huaibei (33.962° N, 116.805° E), and Tai'an (36.205° N, 117.094° E) are all set up in urban areas, and the observation periods were from 22 December 2020 to 15 May 2023, 12 April 2019 to 27 May 2022, and 15 July 2021 to 15 May 2023, respectively.
The MAX-DOAS employed in this study consisted of a telescope, two spectrometers (UV: is 303–370 nm; VIS: 390–550 nm; temperature stabilized at ∼ 20° C), and a computer as the control and data acquisition unit. The telescope elevation angles were set to 1–6, 8, 10, 15, 30, and 90° and controlled by stepper motors. MAX-DOAS measures spectral information to retrieve aerosols and trace gas profiles. The system was operated only during the daytime (08:00–17:00 local time, LT), with a temporal resolution of 15 min and a spatial resolution of 100 m. Because this study focused on surface O3 formation sensitivity, only the lowest-level data of NO2 and HCHO VMRs (ppb) were used. Detailed information regarding the MAX-DOAS instrument, measurement procedures, data inversion algorithms, and data quality control can be found in previous studies (Liu et al., 2022b; Wang et al., 2020; Xing et al., 2022).
Ground-based MAX-DOAS and TROPOspheric Monitoring Instrument (TROPOMI) observations were compared to ensure reliability of the data used in this study. Because the satellite overpass was at approximately 13:30 LT, the mean MAX-DOAS results between 13:00 and 14:00 LT were used for comparison. TROPOMI observations were averaged over a range of 0.2° from the ground-based MAX-DOAS station. Comparisons of NO2 and HCHO tropospheric vertical column densities (VCDs) from the MAX-DOAS and TROPOMI observations are shown in Figs. S1 and S2 in the Supplement. In general, the MAX-DOAS and TROPOMI observations were in good agreement. The Pearson correlation coefficient of monthly averaged NO2 VCDs is 0.99 (P < 0.01), 0.96 (P < 0.01), and 0.96 (P < 0.01) in Hefei, Huaibei, and Tai'an, respectively, and the Pearson correlation coefficient of monthly averaged HCHO VCDs is 0.88 (P < 0.01), 0.77 (P < 0.01), and 0.68 (P < 0.01) in Hefei, Huaibei, and Tai'an, respectively. Generally, the NO2 and HCHO VCDs observed by TROPOMI were smaller than those observed by MAX-DOAS, and the difference may be caused by fitting errors, a priori model bias, cloud and aerosols, and spatiotemporal resolution (De Smedt et al., 2021; Dimitropoulou et al., 2020). In addition, the bottom NO2 concentrations observed by MAX-DOAS were also compared with urban surface NO2 concentrations measured by MEE (Fig. S3), and the results were also comparable to the comparisons reported in previous studies (Lin et al., 2022; Wang et al., 2020), with Pearson correlation coefficient of 0.74 (P < 0.01), 0.66 (P < 0.01), and 0.73 (P < 0.01) for Hefei, Huaibei, and Tai'an, respectively. Thus, the MAX-DOAS data are reliable. The differences between MAX-DOAS and MEE observations arise from these two components. First, there was a difference in the detection geometries, as the urban NO2 concentration observed by MAX-DOAS was the result of scanning along a certain direction, whereas the urban NO2 concentration observed by MEE was sampled in situ. Second, there were some differences in their locations, and the urban NO2 concentration of the MEE was the average of several in situ observation stations (10, 3, and 3 in Hefei, Huaibei, and Tai'an, respectively), whereas we only used one MAX-DOAS in each city.
2.3 Satellite observations
TROPOMI is an imaging spectrometer on board the European Space Agency's Copernicus Sentinel 5 Precursor satellite launched in October 2017, with a daily overpass of approximately 13:30 LT. TROPOMI has a spatial resolution of 3.6 × 7.2 km (before 6 August 2019) and 3.6 × 5.6 km (after 6 August 2019). A more detailed description of TROPOMI can be found in Veefkind et al. (2012). The TROPOMI NO2 (“S5P_OFFL_L2__NO2 …”) and HCHO (“S5P_OFFL_L2__HCHO …”) tropospheric VCD products during May–September 2018–2022 and TROPOMI O3 profiles (“S5P_OFFL_L2__O3__PR …”) during May–September 2022 were used in this study (downloaded from https://search.earthdata.nasa.gov/search; last access: 7 January 2024) (Van Geffen et al., 2020; De Smedt et al., 2018). The recommended quality control (QC; in the range of 0–1) filter was applied to exclude HCHO and O3 profile retrieval values with QC marks <0.5, and NO2 retrieval values with QC marks <0.75. In addition, the TROPOMI-observed HCHO VCDs and NO2 VCDs were regridded to 0.05 × 0.05° (approximately 5 × 5 km) in this study.
2.4 Stepwise multiple linear regression model
To quantify the importance of meteorological drivers, numerous previous studies have used stepwise MLR to derive the relationships between meteorological factors and observed surface O3 concentrations in China (Liu et al., 2023; K. Li et al., 2020; Li et al., 2018; Han et al., 2020; X. Zhang et al., 2023). These studies demonstrated the importance of stepwise MLR in quantifying the contribution of meteorological and anthropogenic components to O3 concentrations. We used the same stepwise MLR modeling approach as Zhai et al. (2019), Li et al. (2018), K. Li et al. (2020), Sun et al. (2022), and Liu et al. (2023). Stepwise regressions were performed, adding and removing terms based on their independent statistical significance to obtain the best model fit. Daily meteorological variables were obtained from the ERA5 reanalysis data (downloaded from https://cds.climate.copernicus.eu, last access: 7 January 2024) and included temperature (T; °C), surface relative humidity (RH; %), total cloud cover (TCC), total precipitation (TP; mm), mean sea level pressure (MSLP; hPa), wind speed of the U and V components (U; V; m s−1), boundary layer height (BLH; m), and vertical velocity at 850 hPa (V850; m s−1). Details of the meteorological parameters are presented in Table S1 in the Supplement.
First, we used the MLR model to remove the effects of meteorological variability from 2017 to 2022 according to 98th or 2nd O3 percentile trends. The meteorological anomalies Xk during May–September 2017–2022 were obtained by removing the 6-year means of the 50 d moving averages from the 10 d mean time series. The anomalies calculated in this process were deseasonalized but not detrended. This yields the meteorology-driven 98th or 2nd O3 percentile anomalies Ym(t),
where R is the regression constant, and βk is the regression coefficient. Second, to avoid overfitting, only the three most important meteorological parameters were selected based on their individual contributions to the regressed 98th or 2nd O3 percentiles, along with the requirement that they be statistically significant above the 95 % confidence level in the MLR model (Li et al., 2018). The fit results and selected meteorological variables varied by city but were regionally consistent (Tables S2 and S3). The 98th or 2nd O3 percentile anomalies Ya(t) were obtained by deseasonalizing, but not detrending, the 98th or 2nd O3 percentile time series in a similar manner to that for the meteorological variables (by removing the 6-year means of the 50 d moving averages). The residual anomaly Yr(t) after removing the meteorology-driven 98th or 2nd O3 percentile anomalies from the MLR model is given by
Finally, the residual is an anomalous component that cannot be explained by the MLR meteorological model and is referred to as meteorologically corrected data by Zhai et al. (2019). It consists of noise due to the limitations of the MLR model and other factors and can be mainly attributed to long-term trends in anthropogenic emission changes over a 6-year period. The trend in the regressed 98th or 2nd O3 percentile reflected the meteorological contribution, and the residual was then used to reflect the presumed anthropogenic contribution.
2.5 Regression model for source separation in primary and secondary HCHO
Tracer-driven linear regression models have been widely used to separate primary and secondary sources of HCHO (Lin et al., 2022; Sun et al., 2021; Hong et al., 2018; Liu et al., 2024; Bao et al., 2022; Heue et al., 2014; MacDonald et al., 2012). CO is emitted directly into the atmosphere through combustion processes (e.g., incomplete combustion in vehicle engines) and can be used as a tracer for primary HCHO emissions (Garcia et al., 2006). O3 reacts with NO emitted from automobiles to form NO2. Therefore, odd amounts of oxygen () are often used as tracers of photochemical processes in urban atmospheres (Wood et al., 2010). In this study, CO and Ox were selected as tracers to separate the primary and secondary sources of ambient HCHO, as expressed in Eq. (3) as follows:
where β0, β1, and β2 are regression coefficients and where [HCHO], [CO], and [Ox] are the ambient HCHO, CO, and Ox VMRs, respectively.
The relative contributions of the primary, secondary, and atmospheric background HCHO to the total ambient HCHO were calculated from the tracer VMRs and the corresponding regression coefficients as follows:
where Ppri denotes the contribution of primary sources of HCHO (e.g., vehicular and industrial emissions), Psec denotes the contribution of secondary sources of HCHO (photochemical oxidation), and Pbg denotes the background HCHO. According to previous studies in central and eastern China (Ma et al., 2016; Wang et al., 2015), the background level of HCHO was limited to 1 ppb. Therefore, the regression parameter β0 was fixed at 1 ppb in this study (Hong et al., 2018; Lin et al., 2022). We first perform hourly averaging of HCHO data from MAX-DOAS observations to match CO and Ox data from MEE observations. Primary and secondary HCHO will then be separated for all available HCHO data from May to September in the MAX-DOAS measurement period. The fitted parameters of the MLR for the measured and modeled HCHO are shown in Fig. S4; it is comparable to the comparisons reported in previous studies (Lin et al., 2022; Sun et al., 2021). As other factors (e.g., meteorological conditions) can also affect the atmospheric HCHO concentration, regression models do not allow us to obtain very consistent results.
3.1 Trends of O3 and its precursors in eastern China
Figure 1 presents the spatial distribution of the surface mean MDA8 O3 VMRs at all available urban sites (105 cities in total) in eastern China during May–September of 2017–2022. High MDA8 O3 VMRs were concentrated in the western cities of eastern China, with the highest values in Jiaozuo of up to 86.4 ppb. High O3 VMRs indicate intensive anthropogenic emissions of O3 precursors from these cities, most of which are located in the NCP, which is one of the most polluted areas in China (Li et al., 2018). Figure 1b and c show the average VCDs of NO2 and HCHO observed by TROPOMI during May–September 2018–2022, respectively. High NO2 and HCHO levels were clustered mainly in the NCP and MLYRP. In this study, the 2nd, 50th, and 98th percentiles of the hourly O3 and NO2 concentrations for cities in eastern China were calculated to determine long-term trends at low, typical, and peak concentration levels, respectively (Cooper et al., 2012; Li et al., 2022; Gaudel et al., 2020). Different percentiles may be related to different influences, such as background concentration levels, emission changes, climate change, and regional transport effects (Lefohn et al., 2010). Li et al. (2014) reported that concentrations below the 5th, between 25th and 75th, and above 95th percentile represent background, typical, and polluted concentrations, respectively. In principle, the lowest daily O3 concentrations usually occur before sunrise due to nighttime titration of NO, and the low percentile (2nd percentile) usually characterizes baseline or background conditions because increases in the low O3 percentile tend to be associated with increases in baseline or background O3 concentrations. Similar conclusions were also obtained from both models and observations (Jacob et al., 1999; Cynthia Lin et al., 2000). O3 pollution in eastern China generally occurs in the late afternoon on clear days in the warm season (W. Wang et al., 2022), when the ambient O3 concentration is highest, so the high percentile (98th) characterizes the conditions of the pollution events. The middle percentiles (25th, 50th, and 75th) usually follow the same trend as the mean values and therefore represent typical conditions (Cooper et al., 2012; Li et al., 2022). Using these indicators to investigate long-term changes in O3 and NO2 helps us gain a more detailed understanding through analyses.
As shown in Fig. 2, the 98th O3 percentile in eastern China showed a significant decreasing trend (about −0.39 ppb yr−1; −0.5 % yr−1) during May–September 2017–2022, and the corresponding O3 exceedance days (−1.1 % yr−1) and exceedance hours (−1.6 % yr−1) also dropped. This is in stark contrast to previous studies which have widely observed a rapid increase in average surface O3 concentrations in Chinese cities, while ignoring changes in their peak concentrations (Lu et al., 2020; Liu et al., 2023; Li et al., 2018, 2021; Chan et al., 2017). The decline rate of the 50th O3 percentile is considerably slower than that of the 98th O3 percentile during May–September 2017–2022, which is about −0.02 ppb yr−1 (−0.0 % yr−1). Unexpectedly, the 2nd O3 percentile in eastern China showed an increasing trend (about 0.06 ppb yr−1; 0.3 % yr−1) during May–September 2017–2022; the increase in 2nd O3 percentile concentrations may be due to the decrease in O3 titration from fresh NO emissions as NOx emissions decrease (Li et al., 2023). It can be confirmed in Fig. 2b that all three NO2 percentiles (2nd, 50th, and 98th) show a significant decreasing trend during May–September 2017–2022, with relative decreasing trends of −2.1 % yr−1, −1.8 % yr−1, and −1.8 % yr−1, respectively. Notably, NO2 declines more rapidly in 2020 and 2021, mainly because of the impact of the COVID-19 pandemic, which has been discussed in detail in previous studies (Liu et al., 2022a). Satellite observations also found a significant decrease in NO2 concentration in eastern China (Fig. 3a), with a decrease rate of about 0.09×1015 mol cm−2 yr−1 (−1.8 % yr−1). Since 2013, NOx emissions have decreased in China owing to a series of air pollution prevention and control policies (Lin et al., 2019; Wang et al., 2019).
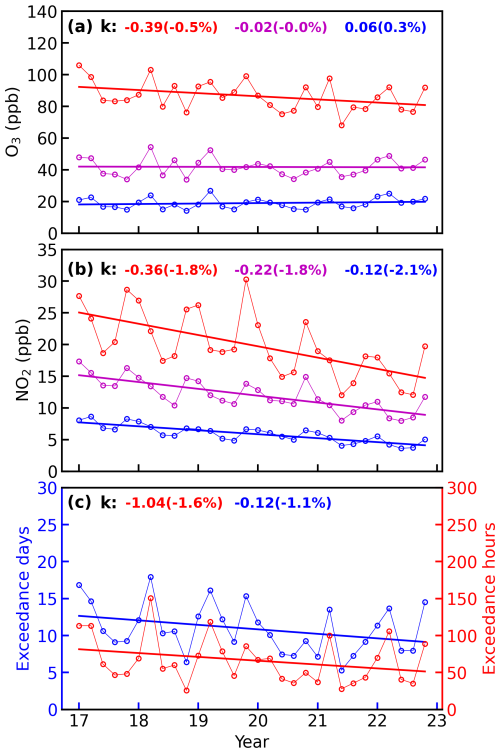
Figure 2Trends of surface (a) O3, (b) NO2, and (c) O3 exceedance days and O3 exceedance hours in eastern China during May–September 2017–2022. The solid red, magenta, and blue lines in panels (a) and (b) indicate the trends for the 98th, 50th, and 2nd percentiles, respectively. The labels in panels (a) and (b) represent the trends in O3 and NO2 for May–September 2017–2022 (units in ppb per year). The labels in panel (c) represent the trends in O3 exceedance days and O3 exceedance hours for May–September 2017–2022. The percentage change is indicated in brackets.
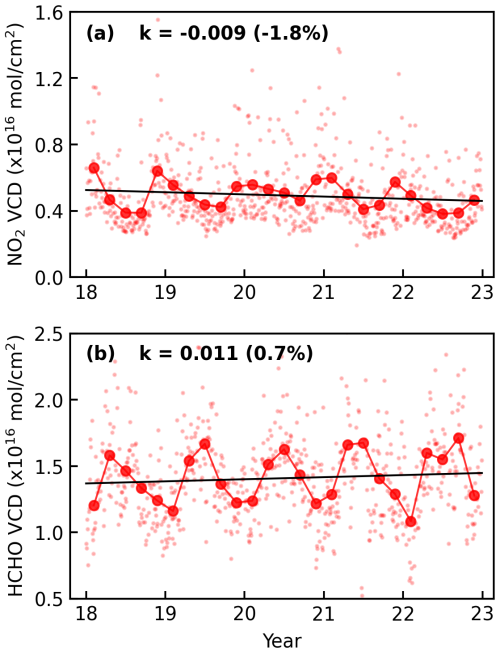
Figure 3Trends of TROPOMI observed in (a) NO2 VCDs and (b) HCHO VCD averaged over eastern China during May–September 2018–2022. The labels at the top of each panel represent the trends in NO2 VCD and HCHO VCD, respectively. The percentage change is indicated in parentheses. The light red dots in panels (a) and (b) represent the daily values, and the solid red dots are monthly mean values.
We further examined the trends in the 98th and 2nd O3 percentiles across cities in eastern China from May to September 2017–2022 (Fig. 4). Generally, the trend in low O3 concentrations in most cities showed an increase, with the 2nd O3 percentile ranging from −0.2 to 0.5 ppb yr−1 (−1.6 % yr−1 to 5.6 % yr−1). Conversely, peak O3 concentrations exhibited a decreasing trend, with the 98th O3 percentile ranging from −1.1 to 0.2 ppb yr−1 (−1.0 % yr−1 to 0.3 % yr−1). The trend in typical O3 concentrations in eastern China from May to September 2017–2022 ranging from −0.4 to 0.3 ppb yr−1 (−0.8 % yr−1 to 0.8 % yr−1), with about one-third of the cities increasing and two-thirds decreasing. The decline in peak O3 concentrations and the rise in low O3 concentrations were notably more pronounced in the NCP compared to the MLYRP. The highest increase in low O3 concentrations was observed in Handan at 0.5 ppb yr−1, while the lowest decrease in peak O3 concentrations was seen in Baoding at 1.1 ppb yr−1. Additionally, we investigated the relationship between the trend in mean NO2 concentrations and the trend in the 98th O3 percentiles from May to September 2017–2022, revealing a significant positive correlation with a Pearson correlation coefficient of R = 0.42 (P < 0.01; Fig. 5). In contrast, the trend in the 98th NO2 percentile during the same period showed a significant negative correlation with the trend in the 2nd O3 percentiles, with a Pearson correlation coefficient of R = −0.41 (P < 0.01). Therefore, the substantial reduction in NOx emissions may have had divergent impacts on low and peak O3 levels, contributing to the observed decrease in low O3 concentrations and increase in peak O3 concentrations across urban areas in eastern China.
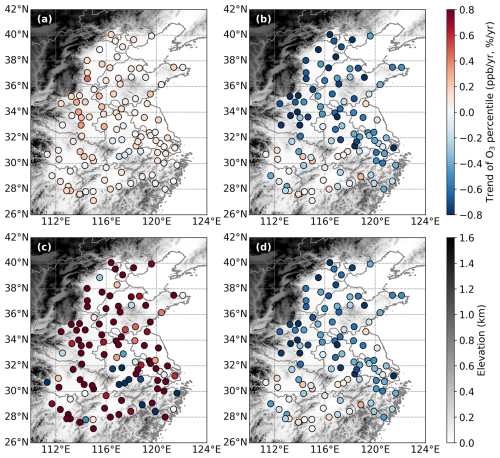
Figure 4Trends of peak and low O3 concentrations in eastern China. Trend of (a) 2 % and (b) 98 % O3 percentiles (units in ppb per year), with percentage variations in the (c) 2 % and (d) 98 % O3 percentile (units in % per year).
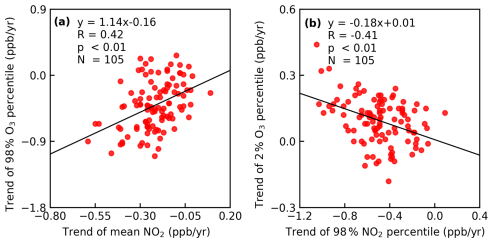
Figure 5Scatterplots showing the relationships between the (a) trend of mean NO2 concentrations and the trend of the 98th O3 percentiles, as well as the (b) trend of the 98th NO2 percentiles and trend of the 2nd O3 percentiles in each city in eastern China during May–September 2017–2022. The correlation coefficients are shown in the top left of each panel. N is for the number of cities.
Due to the absence of long-term ground-based VOCs observations, satellite-observed HCHO VCDs were utilized to indicate VOC variations in eastern China from May to September 2018–2022. HCHO is a transient product reflecting the oxidation of various VOCs, serving as a proxy for VOC emissions, as used in previous studies (Zheng et al., 2018; Zhang et al., 2019). The TROPOMI satellite observations showed a significant upward trend in HCHO VCDs in eastern China during May–September from 2018 to 2022 of approximately 0.11×1015 mol cm−2 yr−1 (0.7 % yr−1) (Fig. 3b). Generally, HCHO VCD exhibits seasonal variability (Li et al., 2021), with higher temperatures and sufficient radiation enhancing photochemical reactions of VOCs and biogenic VOC emissions, thereby promoting HCHO formation (J. Ren et al., 2022). In China, anthropogenic VOC emissions continued to increase until 2019 (Zheng et al., 2018), after which measures were implemented to control them. However, biogenic VOC emissions have been rising in recent years, particularly during extreme heat events such as those experienced in the MLYRP in 2022, leading to a significant increase in biogenic VOC emissions (Zhang et al., 2022; T. Zhang et al., 2023). Importantly, reductions in anthropogenic VOCs have not been sufficient to offset the overall increasing trend in VOC emissions across eastern China (L. Li et al., 2020).
3.2 Anthropogenic and meteorological contributions to O3 trends
The MLR model was applied to assess the significance of the anthropogenic and meteorological components on the 98th and 2nd O3 percentile trends. It is worth noting that the aim of this section is not to accurately assess the contribution of each meteorological factor and precursor concentration to O3 trends but to explore trends in the 98th and 2nd O3 percentile concentrations due to variations in meteorological conditions and anthropogenic emissions in eastern China and to determine the magnitude of the 98th and 2nd O3 percentile trends. Based on the daily output of the MLR model, the monthly mean meteorological and anthropogenic components of the 98th and 2nd O3 percentiles from May to September 2017–2022 were calculated, and the relative contributions of the meteorological and anthropogenic components to the 98th and 2nd O3 percentile trends were quantified. Based on the MLR model, the three key meteorological factors with the most significant effects on the 98th and 2nd O3 percentiles were selected (Li et al., 2018; K. Li et al., 2020). The meteorological components of the 98th and 2nd O3 percentile concentrations were then simulated using the MLR model driven by the three selected key meteorological factors, and the coefficients of each factor and the Pearson correlation coefficients (R) of the fitted MLR model were obtained. The R for each city in eastern China was in the range of 0.16 to 0.77 for the 2nd O3 percentiles (Table S2) and 0.24 to 0.84 for the 98th O3 percentiles (Table S3), respectively. RH and T were the most important factors affecting the 98th and 2nd O3 percentile concentrations, followed by TCC, BLH, U, V, and V850 (Tables S2 and S3). These results are consistent with the current understanding of the meteorological effects of O3 (Weng et al., 2022; K. Li et al., 2020; Ding et al., 2023).
Figure 6 shows the trends in the monthly mean observed 98th and 2nd O3 percentile concentrations, meteorological 98th and 2nd O3 percentile components in the MLR simulations, and the residual anthropogenic 98th and 2nd O3 percentile components during May–September 2017–2022. The trend of the monthly mean 98th O3 percentile meteorological component obtained by the MLR model from May to September 2017–2022 was considerably smaller than that of the anthropogenic component, with almost no trend between 2017 and 2022 (0.005 ppb yr−1). Meteorological influences explained only 3 % (0.005 ppb yr−1) of the observed 98th O3 percentile trend during May–September 2017–2022, with the remaining −103 % (−0.183 ppb yr−1) determined by anthropogenic influences. However, if we count the monthly mean 98th O3 percentiles trends from May–September 2017–2021, we find a more significant downward trend in 98th O3 percentiles (−0.363 ppb yr−1), with a contribution of −0.119 ppb yr−1 (−33 %) from the meteorological component and −0.244 ppb yr−1 (−67 %) from the anthropogenic component (Table 1). The differences in the O3 trend statistics for 2017–2022 and 2017–2021 are mainly due to the meteorological conditions in 2022, which will be analyzed in detail in Sect. 4. Separating the observed 2017–2022 98th O3 percentile trends by month shows that the seasonal May–September trend of −0.178 ppb yr−1 over eastern China (Table 1) is driven by June, July, and September. The observed trends were −0.020 ppb yr−1 for May, −4.437 ppb yr−1 for June, −1.745 ppb yr−1 for July, −0.687 ppb yr−1 for August, and 1.999 ppb yr−1 for September. This month-to-month difference was driven mainly by meteorology. As derived from the MLR model, the meteorologically driven 98th O3 percentile trends for July and September were −1.100 and 1.136 ppb yr−1, respectively, which are larger than those of the anthropogenic trend (−0.645 ppb yr−1 for July and 0.863 ppb yr−1 for September).
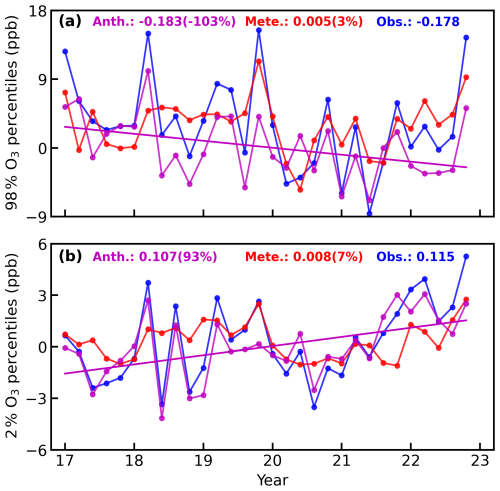
Figure 6Trends of observed (blue lines), meteorological (red lines), and anthropogenic (magenta lines) (a) 98th and (b) 2nd O3 percentile components in eastern China during May–September 2017–2022. The labels at the top of each panel represent the trend in the observed, meteorological, and anthropogenic components.
Table 1Observed (Obs.), meteorologically (Mete.), and anthropogenically (Anth.) driven trends of the 2 % and 98 % O3 percentiles in eastern China from 2017 to 2022 and from 2017 to 2021.
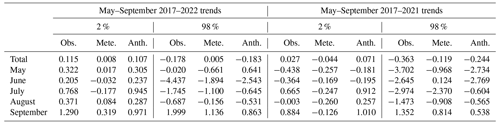
The trend of the mean 2nd O3 percentiles meteorological component (0.008 ppb yr−1, 7.0 %) was also smaller than that of the anthropogenic component (0.107 ppb yr−1, 93.0 %) from May–September 2017–2022. It is worth noting that the increasing trend of the 2nd O3 percentiles during May–September 2017–2021 was significantly smaller than that during May–September 2017–2022 (0.0270.115 ppb yr−1 vs. 0.115 ppb yr−1). The contribution of meteorological components to the 2nd O3 percentile trends during May–September 2017–2021 was −0.044 ppb yr−1 (−163.0 %), while the anthropogenic component trend of 2nd O3 percentiles was 0.071 ppb yr−1 (263.0 %) (Table 1). This indicates that meteorological factors are not conducive to the increasing trend of low O3 concentrations during May–September 2017–2021 and that the increasing trend of low O3 concentrations is mainly controlled by anthropogenic influences. In addition, the observed 2017–2022 2nd O3 percentile trend by month was 0.322 ppb yr−1 in May, 0.205 ppb yr−1 in June, 0.768 ppb yr−1 in July, 0.371 ppb yr−1 in August, and 1.290 ppb yr−1 in September. The observed 2017–2022 2nd O3 percentile trends by month were driven by July and September, while the sign of the 2nd O3 percentile trends, driven by meteorological and anthropogenic components, was reversed in July (−0.177 vs. 0.945). Therefore, although anthropogenic emissions are the main driver of opposing trends in peak and low O3 concentrations in eastern China, the effect of changes in meteorological components on the 2nd and 98th O3 percentile trends cannot be ignored.
3.3 Diurnal differences in surface O3 formation sensitivity
To elucidate the reasons for changes in peak, typical, and low O3 concentrations in the presence of emission reductions in O3 precursors (a substantial decrease in NOx emissions and a slight increase in VOC emissions), it is critical to accurately determine the O3 formation sensitivity to its precursors, especially at a high temporary resolution. Secondary HCHO and NO2 were selected as the representative VOCs and NOx, respectively (Xue et al., 2022; Hong et al., 2018, 2022; Lin et al., 2022; B. Ren et al., 2022). Surface NO2 and HCHO VMRs retrieved from ground-based MAX-DOAS observations were used to diagnose the surface-O3-formation-sensitive regime. The thresholds for the VOC-limited, transition, and NOx-limited regimes were determined based on the correlation between the O3 concentrations and the changes in the O3 precursor concentrations under different FNR (defined as the ratio of HCHO VMRs to NO2 VMRs; FNR = ). Here, we used FNRsec (defined as the ratio of secondary HCHO to NO2; FNRsec = ) as an indicator of O3 formation sensitivity.
Compared with conventional FNR, FNRsec eliminates the background and primary HCHO interference, improves the accuracy of diagnosing O3 formation sensitivity, and contributes to a better understanding of O3 formation sensitivity (Lin et al., 2022; Xue et al., 2022). Most of the FNRsec values (∼ 98 %) varied between 0.03 and 1.5 during the whole observations, and excessively low FNRsec values can be attributed to deficiencies in the HCHO source assignment in the MLR model. Therefore, FNRsec values greater than 1.5 (or less than 1.5 up to 0.03) were filtered out for quality control.
Three steps are involved in determining the FNRsec threshold. First, the surface hourly averaged secondary HCHO and NO2 VMRs during May–September based on MAX-DOAS observations were normalized by dividing their respective mean values because of the large differences in surface HCHO and NO2 concentrations (Su et al., 2017; B. Ren et al., 2022). The ratio of the hourly averaged O3 VMRs to the hourly averaged normalized NO2 VMRs () and the ratio of the hourly averaged O3 VMRs to the hourly averaged normalized secondary HCHO VMRs (SHCHO) were calculated. Finally, third-order polynomials were used to fit and SHCHO (Fig. 7).
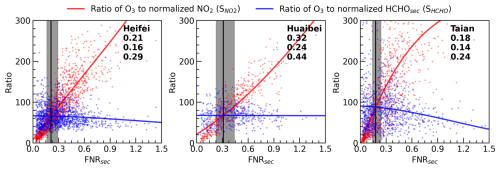
Figure 7Third-order fitting of ratios of O3 VMRs versus normalized NO2 VMRs and ratios of O3 VMRs versus normalized secondary HCHO VMRs in different FNRsec values in Hefei, Huaibei, and Tai'an during May–September, based on MAX-DOAS observations. The intersect at FNRsec is indicated by the solid black line. The vertical shadow indicates the relative difference between the ratios of O3 VMRs versus the normalized NO2 VMRs and ratios of O3 VMRs versus secondary HCHO VMRs within 25 % (transition regime). The labels at the top right of each panel represent the intersect FNRsec values and the thresholds for the NOx-limited regime (high) and VOC-limited regime (low) in Hefei, Huaibei, and Tai'an, respectively.
As shown in Fig. 7, the third-order fitting of increased almost linearly with the FNRsec values, similar to SHCHO. When is significantly larger than SHCHO, O3 formation is more sensitive to NOx, which is the NOx-limited regime, and vice versa. For example, in Hefei, and SHCHO intersected at FNRsec = 0.21. FNRsec values less than 0.16 and greater than 0.29 correspond to the VOC-limited regime and NOx-limited regime, respectively, where the relative difference between and SHCHO is more than 25 % (Lin et al., 2022), and the range of FNRsec values from 0.16 to 0.29 represents a transition regime. For Huaibei and Tai'an, the transition regime range was 0.24–0.44 and 0.14–0.24, respectively. The FNRsec threshold for O3 formation sensitivity varies in different cities, which may be due to differences in O3 precursor emissions.
Figure 8 shows the diurnal variations in O3 and its precursors at three stations (Hefei, Huaibei, and Tai'an) in eastern China, from south to north. O3 and NO2 VMRs showed diametrically opposite trends from 08:00 to 13:00 LT, with O3 concentrations rapidly increasing (about 8.2, 7.4, and 8.6 ppb h−1 in Hefei, Huaibei, and Tai'an, respectively) and NO2 concentrations gradually decreasing (about −0.90, −0.86, and −1.49 ppb h−1 in Hefei, Huaibei, and Tai'an, respectively). Ambient HCHO concentrations depend on the primary emissions and photo-oxidation of VOCs (Xue et al., 2022). We separated the primary and secondary sources of HCHO using the CO and Ox VMRs, and their diurnal variations are shown in Fig. 8d–f. The primary source contributed the most to the ambient HCHO concentration. Atmospheric primary HCHO concentrations are mainly derived from motor vehicle exhaust fumes, the petrochemical industry, solvent use, and combustion emissions (Ma et al., 2019). Hefei, Huaibei, and Tai'an are located in the NCP, which is the region with the highest primary emissions of air pollutants in China (Li et al., 2017). The rapid industrialization and urbanization in these developing cities has influenced the primary and secondary HCHO concentrations, and HCHO mainly stems from initial atmospheric pollutants (Lu et al., 2024). The HCHO from primary emissions was highest between 08:00 and 10:00 LT (about 2.63, 2.50, and 4.30 ppb in Hefei, Huaibei, and Tai'an, respectively) and then gradually decreased, reaching the lowest concentration around 15:00 LT with about 1.78, 1.54, and 1.82 ppb in Hefei, Huaibei, and Tai'an, respectively, gradually rising thereafter. High primary HCHO concentrations in the morning and evening may have been due to emissions from traffic (Hong et al., 2018; Zhang and Cao, 2015). Secondary HCHO concentrations were lowest in the morning, at about 0.58, 0.84, and 0.65 ppb in Hefei, Huaibei, and Tai'an, respectively; with the enhancement of photochemical reactions and the resumption of human activities, the secondary HCHO concentrations gradually increased from 08:00 to 12:00 LT, and the first peak usually occurred at 11:00–14:00 LT. The proportion of secondary HCHO VMRs in the total HCHO VMRs also increased rapidly, and the proportion of secondary HCHO VMRs in the total HCHO VMRs gradually stabilized after 12:00 LT, with about 24 %, 31 %, and 22 % in Hefei, Huaibei, and Tai'an, respectively. Similar diurnal variation trends were found in Shenyang (Xue et al., 2022), Nanjing (Hong et al., 2018), Guangzhou (Lin et al., 2022), Shenzhen (Zhang and Cao, 2015), Rome (Possanzini et al., 2002), and Toyama (Taguchi et al., 2020), etc. It is worth noting that the secondary HCHO VMRs in Hefei, Huaibei, and Tai'an increased significantly between 16:00 and 17:00 LT; however, the specific reasons remain to be further investigated. In general, NO2 concentrations were higher, and secondary HCHO concentrations were lower, in the early morning; with the enhancement of photochemical reactions (08:00–13:00 LT), NO2 concentrations decreased rapidly, and secondary HCHO concentrations increased gradually.
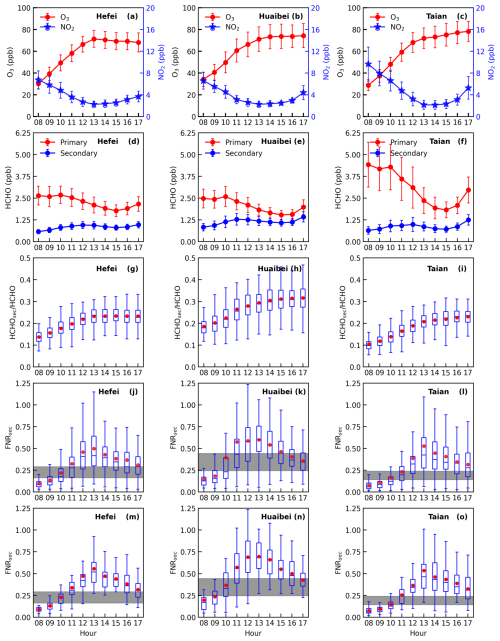
Figure 8Diurnal variation in surface (a–c) O3 and NO2 VMRs. (d–f) HCHO VMRs contributed by primary and secondary sources. (g–i) The ratio of secondary HCHO to total HCHO VMRs. (j–l) FNRsec during the whole observation. (m–o) FNRsec during O3 exceedance day in Hefei, Huaibei, and Tai'an during May–September, respectively. The vertical bars in panels (a)–(f) represent 1 standard deviation. The dot within the box indicates the mean value, and the positions of box plots represent the 5th, 25th, 50th, 75th, and 95th percentiles, respectively. The horizontal shadow in panels (j)–(o) represents the transition regime; the top of the shadow represents the NOx-limited regime, and the bottom of the shadow represents the VOC-limited regime.
The significant diurnal variation in O3 precursors contributes to the transition of O3 formation sensitivity, as indicated by the FNRsec, demonstrating a distinct single-peak pattern. As shown in Fig. 8j–l, FNRsec increases sharply from 08:00 to 13:00 LT, corresponding with considerable increases in O3 VMRs. In cities like Hefei, Huaibei, and Tai'an, O3 formation sensitivity starts in the VOC-limited regime (below the shaded area) at 08:00–09:00 LT, gradually transitioning to the transition regime by 10:00–11:00 LT. By 12:00–14:00 LT, O3 formation in Huaibei predominantly enters the NOx-limited regime before shifting back to the transition regime after 15:00 LT. Similarly, in Hefei and Tai'an, the NOx-limited regime prevails from 12:00 to 16:00 LT, shifting towards the transition regime after 17:00 LT. Overall, O3 formation sensitivity is VOC-limited or transition regime dominant during periods of rapid O3 concentration increases (08:00–12:00 LT), shifting to transition or NOx-limited regimes when O3 concentrations peak (12:00–14:00 LT). We further investigated the diurnal characteristics of FNRsec on O3 exceedance days (MDA8 O3 > 160 µg m−3). Compared to the entire observation period, FNRsec on O3 exceedance days exhibits a faster transition from 08:00 to 13:00 LT and prolonged persistence in the NOx-limited regime.
The diurnal variation in the O3 VMRs was very similar to that of FNRsec, particularly from 08:00 to 13:00 LT, and both increased sharply. The exponential function was applied to fit the relationship between the O3 concentration and FNRsec values from 08:00 to 13:00 LT in Hefei, Huaibei, and Tai'an (Fig. 9), and all three cities showed significant positive correlations, with Pearson correlation coefficients of 0.53 (P < 0.01), 0.40 (P < 0.01), and 0.52 (P < 0.01) in Hefei, Huaibei, and Tai'an, respectively. Moreover, the exponential fitting is better on the O3 exceedance days, and the correlation coefficients are higher, with 0.69 (P < 0.01), 0.59 (P < 0.01), and 0.61 (P < 0.01) in Hefei, Huaibei, and Tai'an, respectively. This indicates that the dependence of the O3 production rate on its precursors rapidly shifts with increasing O3 concentration, particularly on O3 exceedance days. These changes also suggest that the dependence of O3 on its precursors is extremely complex, and the precise control of O3 pollution requires the identification of O3 formation sensitivity mechanisms with high temporal resolution and targeted control of O3 precursor concentrations.
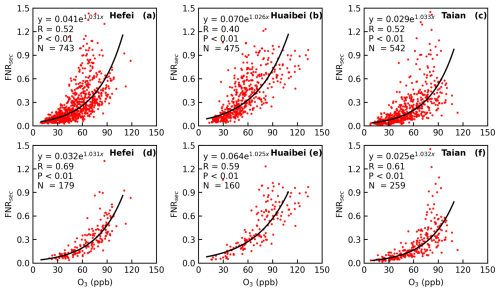
Figure 9The relationship between the O3 VMRs and FNRsec during (a–c) the whole observation, and (d–f) the O3 exceedance day from 08:00 to 13:00 LT in Hefei, Huaibei, and Tai'an, respectively. The black line represents the exponential fitting (). The fitting functions and correlation coefficients for exponential fit are shown at the top left of each panel. N is the number of samples.
Owing to the limitations of the observational data, the analysis of diurnal transitions in surface O3 formation sensitivity is limited to three cities in eastern China. Here, other cities in eastern China were further investigated using satellite observations, and we construct conventional FNR using TROPOMI-observed NO2 and HCHO VCDs from May to September 2018–2022. In order to avoid the misjudgment of O3 formation sensitivity caused by arbitrary selection of FNR thresholds, a third-order polynomial model was applied to investigate the empirical relationship between TROPOMI FNR and surface O3 VMRs, which has been widely used in other studies (J. Ren et al., 2022). Since the TROPOMI-observed surface O3 VMRs can be obtained after November 2021 in China, we only collected the relationship between TROPOMI FNR and surface O3 VMRs from May to September, 2022. The third-order polynomial fitting relationship between surface O3 VMRs and TROPOMI FNR is shown in Fig. 10a, assuming that the peak of the curve (with a slope of zero) marks the transition from the VOC-limited regime to the NOx-limited regime; the transition regime is defined as a range of slopes between −3 and +3 (J. Ren et al., 2022). Through the third-order polynomial model, the TROPOMI FNR threshold in eastern China was determined, which are FNR < 2.1 for a VOC-limited regime and FNR > 3.2 for a NOx-limited regime.
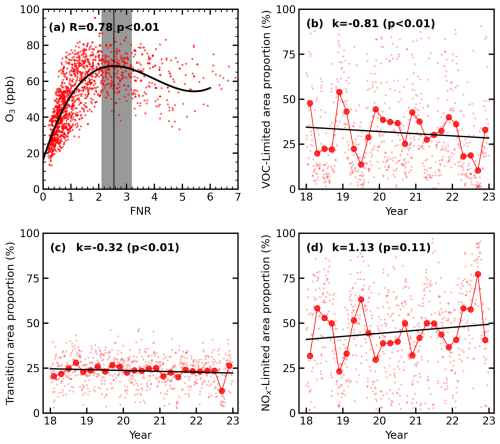
Figure 10(a) Variation in monthly mean O3 VMRs (∼ 13:30) with monthly mean TROPOMI FNR in eastern China during May–September 2022. The solid line represents third-order polynomial fitting. The vertical line represents the maximum value of the fitted curve, and the vertical shadow represents the range of the curve slope from −3 to +3 (transition regime). Trends of TROPOMI observed area proportion for the (b) VOC-limited regime, (c) transition regime, and (d) NOx-limited regime over eastern China during May–September 2018–2022. The light red dots in panels (b)–(d) represent the daily values, and the solid red dots are monthly mean values.
Figure 11 shows the occurrence probabilities of the VOC-limited regime, transition-limited regime, and NOx-limited regime spatial distributions derived from TROPOMI observations in eastern China during May–September 2018–2022. Since the TROPOMI satellite usually transits around 13:30, it can represent the spatial distribution of midday O3 formation sensitivity in eastern China. Apparently, the midday O3 formation sensitivity of most cities in eastern China is under a NOx-limited regime; only several cities in the northern part of the NCP and Yangtze River Delta are mainly controlled by VOC-limited regime. In addition, Fig. 10b–d show the trend of the area proportion of VOC-limited regime, transition regime, and NOx-limited regime in eastern China, where the area proportion of the VOC-limited regime and transition regime decreases at a rate of 0.62 % yr−1 and 0.18 % yr−1, respectively, while the NOx-limited regime area proportion increased at a rate of 0.80 % yr−1. More importantly, although there is a significant monthly variation in the area proportion of O3 formation sensitivity, it is usually below 50 % in May and September and below 25 % in June–August; that is, the NOx-limited regime dominates the midday O3 formation sensitivity in eastern China. Due to China's strict control of NOx emissions in recent years, the surface O3 formation sensitivity in many areas of China has shown a transition from the VOC-limited regime to the transition regime or NOx-limited regime.
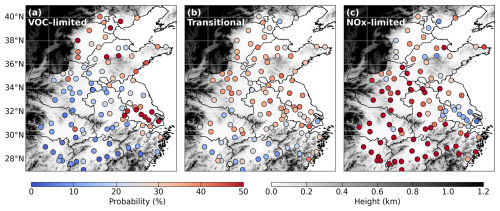
Figure 11Occurrence probabilities of the (a) VOC-limited regime, (b) transition-limited regime, and (c) NOx-limited regime spatial distributions in eastern China derived from TROPOMI observations during May–September 2018–2022.
In conclusion, significant diurnal transitions in surface O3 formation sensitivity primarily stem from fluctuations in O3 precursors. Early-morning conditions (08:00–09:00 LT) are mainly a VOC-limited regime; this shifts to a NOx-limited regime by midday (12:00–14:00 LT). In addition, the area proportion of VOC-limited regime was also declining, while the NOx-limited regime area proportion was increasing. Consequently, the substantial reduction in NOx emissions across eastern China has led to pronounced opposite trends in the low (increased) and peak (decreased) surface O3 concentrations, and the surface O3 formation sensitivity to VOCs is generally weakened year by year. Accordingly, the O3 improvements and benefits of VOC emission reductions may become weaker, while the O3 improvements and benefits of NOx emission reductions become larger. Furthermore, the long-distance transport of VOCs has a diminished impact on O3 concentrations due to chemical losses from OH radical oxidation during transport, highlighting NOx emission reductions as pivotal for intercity and even long-distance efforts to mitigate regional O3 pollution (Y. Wang et al., 2023a).
Previous analyses have shown that the trend of low O3 concentrations in eastern China is increasing, whereas the trend of peak O3 concentrations is decreasing. The opposite trend is mainly driven by anthropogenic emissions. Significant NOx emission reductions dominate the reduction in peak O3 concentrations in eastern China, owing to the diurnal transition in surface O3 formation sensitivity. The discussion focuses on the reasons for the differences in the O3 trend statistics for 2017–2022 and 2017–2021 and the reasons for the possible increase in nighttime O3.
As described in Sect. 3.2, the trends for the monthly mean observed, meteorological, and anthropogenic 98th O3 percentile concentrations during May–September 2017–2021 are −0.363 ppb yr−1, −0.119 ppb yr−1 (−33 %), and −0.244 ppb yr−1 (−67 %), respectively (Table 1), and the trends for the monthly mean observed, meteorological, and anthropogenic 2nd O3 percentile concentrations are 0.027 ppb yr−1, −0.044 ppb yr−1 (−163 %), 0.071 ppb yr−1 (263 %), respectively. However, the trends of monthly mean observed, meteorological, and anthropogenic 98th O3 percentiles during May–September 2017–2022 are −0.178 ppb yr−1, 0.005 ppb yr−1 (3 %), and −0.183 ppb yr−1 (−103 %), respectively, and the trends of the observed, meteorological, and anthropogenic 2nd O3 percentiles during May–September 2017–2022 are 0.115 ppb yr−1, 0.008 ppb yr−1 (7 %), and 0.107 ppb yr−1 (93 %), respectively. Although anthropogenic emissions dominated variations in O3 trends (May–September 2017–2022 and May–September 2017–2021), meteorological effects on O3 trends cannot be ignored, particularly in 2022. Shadowed by mid-latitude atmospheric circulation, tropical sea–air coupling, and local land–air feedback processes, a record-breaking super-heat-wave event occurred in most cities in eastern China in the summer of 2022, and some cities broke their highest temperature records (T. Zhang et al., 2023; Zhang et al., 2022). The most important meteorological variables in the MLR model were daily maximum temperature and RH (Tables S2 and S3). The temperature in eastern China showed that the monthly mean nighttime (daytime) temperature in June–August 2022 was 1.0° (1.1°), 0.8° (1.4°), and 2.2° (2.8°) higher than the monthly mean nighttime (daytime) temperature in June–August 2021, respectively (Fig. 12). The monthly mean nighttime (daytime) RH in eastern China in 2022 was 3.2 % (3.1 %), 1.9 % (4.5 %), and 9.4 % (11 %) lower than the monthly mean nighttime (daytime) RH in June–August 2021, respectively. Li et al. (2024) revealed that a sustained heat wave of extremely hot and dry summers in 2022 accelerated photochemical O3 production by increasing anthropogenic and biogenic emissions and exacerbated O3 accumulation by inhibiting dry deposition due to water-starved vegetation, resulting in an increase in O3 pollution by more than 30 % in urban areas. Our results also showed an increase in the meteorological components in the 98th and 2nd O3 percentiles in 2022 relative to the meteorological components in the 98th and 2nd O3 percentiles in 2021 (Fig. 6). Therefore, extremely hot and dry weather in 2022 will increase the peak and low O3 concentrations in eastern China, which is probably the main reason for the difference between the May and September 2017–2021 and May–September 2017–2022 meteorological component trends.
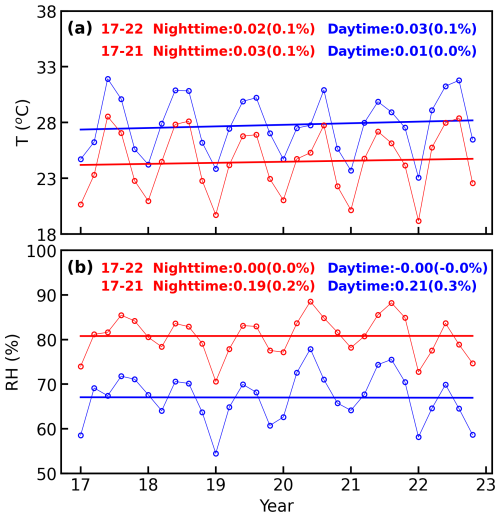
Figure 12Daytime (07:00–19:00 LT) and nighttime (19:00–07:00 LT) (a) T and (b) RH trends in ERA5 reanalysis data in eastern China during May–September 2017–2022 and May–September 2017–2021. The labels at the top right of each panel represent the trend of T and RH in eastern China during May–September 2017–2022 and May–September 2017–2021.
Overall, meteorological conditions affected O3 concentrations in three ways. The first is the effect on the photochemical reaction rates (Bloomer et al., 2009), which are affected by rising temperatures and increasing solar radiation intensity, leading to higher-O3 concentrations. Second, the effect on O3 precursors; high temperature promotes an increase in VOC emissions from land surface vegetation (Churkina et al., 2017), which further leads to an increase in the O3 concentration. However, favorable meteorological conditions, such as high wind speed and precipitation, can reduce the O3 precursor concentrations (Mousavinezhad et al., 2021), thereby reducing the O3 concentration. Finally, transmission and regional transport also affect the distribution of O3 in cities (Lang et al., 2021). However, the frequency of extreme weather events such as the super-heat-wave event in 2022 is increasing (Jin et al., 2021) in eastern China. In the context of the current warming climate, heat waves of extremely hot and dry conditions can elevate O3 concentrations by increasing photochemical rates and promoting natural emissions (e.g., soil emissions of NOx and vegetation emissions of VOCs), and meteorological components may have an increasing influence on O3 trends.
Figure 6b shows that the observed increase in the 2nd O3 percentile was mainly concentrated after 2020 with up to 0.44 ppb yr−1. The meteorological components did not change significantly in 2020 and 2021 but considerably increased in 2022, with a trend of 0.17 ppb yr−1 2020–2022. This rapid increase in the 2nd O3 percentile is mainly caused by anthropogenic emissions, with a trend of 0.27 ppb yr−1 for 2020–2022. Owing to the impact of the COVID-19 pandemic, the decrease in NOx concentrations was most significant in 2020–2022 (Fig. 2b), and the substantial reduction in NOx concentrations weakened the O3 titration of NO, resulting in an increase in nighttime O3 concentrations, which was also confirmed by the significant negative correlation between the trend of the 98th NO2 percentile and the trend of the 2nd O3 percentile during May–September 2017–2022 (Fig. 5b). A recent study showed that nighttime O3 depletion in China is mainly caused by the wet-scavenging effect and O3 titration from fresh NO emissions (Li et al., 2023). The wet-scavenging effect was similar to the effect of precipitation; the higher the ambient humidity, the more conducive it was to O3 depletion. The RH at night increased slowly in eastern China during May–September of 2017–2021 (Fig. 12b), and the nighttime RH in 2020 and 2021 was higher than that in other years. Moreover, a general wetting trend has been detected in eastern China during the summer in recent years (Hu et al., 2021), which is largely related to the increase in summer precipitation and decrease in summer potential evapotranspiration. RH had the most significant effect on the 2nd O3 percentile trends, according to MLR results (Table S2). Therefore, the meteorological component had an inhibitory effect on the increase in the 2nd O3 percentile trends during May–September 2017–2021. However, owing to the significant emission reduction in NOx concentrations, the titration of NOx was weakened, and the decrease in O3 depletion at night led to an increase in the overall 2nd O3 percentile trends.
Owing to the limitations of the observational data, the analysis of surface O3 precursors and O3 formation sensitivity is limited to three cities in eastern China. Although other cities in eastern China were further investigated using satellite observations, TROPOMI only provides observation results for column concentrations at approximately 13:30 LT each day, which did not allow us to obtain diurnal variations in the O3 formation sensitivity. Further observations must be extended to other cities to investigate the relationship between O3 and its precursors more comprehensively.
In conclusion, owing to the impact of the COVID-19 pandemic (significant decrease in NOx concentrations) and unfavorable meteorological conditions (high relative humidity) in 2020 and 2021 in eastern China, the 98th O3 percentile concentration in 2020 and 2021 was lower (compared to the 98th O3 percentile concentration in 2018 and 2019), while the 2nd O3 percentile concentration showed a rapid upward trend. In addition, the extremely hot and dry meteorological conditions in 2022 increased the 98th and 2nd O3 percentile concentrations, weakening the decreasing trend in peak O3 concentrations and increasing the upward trend at low O3 concentrations.
In this study, we investigated urban scale O3 trends in densely populated areas of eastern China using multiple data sources. Through long-term records of surface O3 and related parameters at 105 urban air quality monitoring sites in eastern China from May to September of 2017 to 2022, we found opposite trends for low and peak surface O3 concentrations. Statistical results showed that low O3 concentrations increased significantly (0.06 ppb yr−1, 0.3 % yr−1), while peak O3 concentrations decreased considerably (−0.39 ppb yr−1, −0.5 % yr−1). Anthropogenic emissions were the main driver of both trends, although meteorological effects also played a role.
Based on long-term MAX-DOAS observations in Hefei, Huaibei, and Tai'an, we found that surface O3 formation sensitivity in the early morning (08:00–09:00 LT) was mainly controlled by VOC concentrations, shifting to an NOx-limited regime by midday (12:00–14:00 LT). Moreover, O3 formation sensitivity is in the VOC-limited regime or transition regime during periods of sharp increases in O3 concentrations (08:00–11:00 LT) and is usually in the transition or NOx-limited regime when O3 concentration peaks (11:00–14:00 LT). Therefore, the decline in peak O3 concentrations was attributed to the significant reduction in NOx concentrations. To further suppress peak O3 concentrations and reduce the number of O3 exceedance days, controlling NOx emissions should not be neglected. The increase in low O3 concentrations can also be attributed to anthropogenic emissions and meteorological effects. Extremely hot and dry conditions can elevate O3 concentrations by increasing photochemical rates, promoting natural emissions and depressing nighttime O3 depletion by impairing the wet-scavenging effect. Additionally, a substantial reduction in NOx concentrations weakened O3 titration from fresh NO emissions and increased nighttime O3 concentrations.
Our results highlight the positive impact of the NOx reduction in controlling peak O3 levels. In response to the current severe O3 pollution in China, it is crucial to consider the regular transitions in O3 formation sensitivity throughout the day when formulating O3 prevention and control policies. When O3 concentrations are about to peak, a strict control of NOx emissions is necessary. This study provides novel insights into the spatiotemporal variability in O3 formation sensitivity in eastern China and will be further expanded at different altitude levels in our future studies.
Code related to this paper may be requested from the authors.
Measurement data in this study are available in the data repository maintained by Mendeley Data at https://doi.org/10.17632/zd9cb9dk75.1 (Wang, 2024). Real-time hourly observed urban O3, NO2, and CO concentrations in 105 cities in eastern China from 2017 to 2022 were obtained from https://quotsoft.net/air/ (Wang, 2025). TROPOMI data used in this study were obtained from https://search.earthdata.nasa.gov/search (NASA, 2025).
The supplement related to this article is available online at: https://doi.org/10.5194/acp-25-347-2025-supplement.
CL, CX, and YC conceived and supervised the study. ZW analyzed the data. ZW wrote the paper with input from CL, CX, and YC. CS and HZ reviewed and commented on the paper. All authors contributed to discussing the results and revising the draft.
The contact author has declared that none of the authors has any competing interests.
Publisher’s note: Copernicus Publications remains neutral with regard to jurisdictional claims made in the text, published maps, institutional affiliations, or any other geographical representation in this paper. While Copernicus Publications makes every effort to include appropriate place names, the final responsibility lies with the authors.
The authors extend their thanks to editor Jeffrey Geddes and the three reviewers for their time and expertise. We would like to express our gratitude to Fusheng Mou and SuWen Li of Huaibei Normal University for their assistance. We would like to thank the Ministry of Ecology and Environment of China for the O3, NO2, and CO data. We would like to thank the ERA5 data developers and TROPOMI data developers for providing free and open-source materials.
This research has been supported by grants from the Anhui Provincial Natural Science Foundation “Jianghuai Meteorological” Joint Fund (grant no. 2208085UQ04), the National Natural Science Foundation of China (grant nos. U21A2027 and 42207113), the Anhui Meteorological Bureau Special Programme for Innovation and Development (grant no. CXB202303), the East China Regional Meteorological Science and Technology Collaborative Innovation Fund (grant no. QYHZ202317), and the China Meteorological Administration “Application of quantum technology in meteorological detection” Youth Innovation Team Project (grant no. CMA2024QN11).
This paper was edited by Jeffrey Geddes and reviewed by three anonymous referees.
Bao, J., Li, H., Wu, Z., Zhang, X., Zhang, H., Li, Y., Qian, J., Chen, J., and Deng, L.: Atmospheric carbonyls in a heavy ozone pollution episode at a metropolis in Southwest China: Characteristics, health risk assessment, sources analysis, J. Environ. Sci.-China, 113, 40–54, https://doi.org/10.1016/j.jes.2021.05.029, 2022.
Bauwens, M., Verreyken, B., Stavrakou, T., Müller, J. F., and Smedt, I. D.: Spaceborne evidence for significant anthropogenic VOC trends in Asian cities over 2005–2019, Environ. Res. Lett., 17, 015008, https://doi.org/10.1088/1748-9326/ac46eb, 2022.
Bloomer, B. J., Stehr, J. W., Piety, C. A., Salawitch, R. J., and Dickerson, R. R.: Observed relationships of ozone air pollution with temperature and emissions, Geophys. Res. Lett., 36, L09803, https://doi.org/10.1029/2009gl037308, 2009.
Chan, K. L., Wang, S., Liu, C., Zhou, B., Wenig, M. O., and Saiz-Lopez, A.: On the summertime air quality and related photochemical processes in the megacity Shanghai, China, Sci. Total Environ., 580, 974–983, https://doi.org/10.1016/j.scitotenv.2016.12.052, 2017.
Chinese State Council: Action Plan on Air Pollution Prevention and Control, http://www.gov.cn/zwgk/2013-09/12/content_2486773.htm (last access: 1 January 2024), 2013 (in Chinese).
Chinese State Council: Three-Year Action Plan on Defending the Blue Sky, http://www.gov.cn/zhengce/content/2018-07/03/content_5303158.htm (last access: 1 January 2024), 2018 (in Chinese).
Churkina, G., Kuik, F., Bonn, B., Lauer, A., Grote, R., Tomiak, K., and Butler, T. M.: Effect of VOC Emissions from Vegetation on Air Quality in Berlin during a Heatwave, Environ. Sci. Technol., 51, 6120–6130, https://doi.org/10.1021/acs.est.6b06514, 2017.
Cooper, O. R., Gao, R. S., Tarasick, D., Leblanc, T., and Sweeney, C.: Long-term ozone trends at rural ozone monitoring sites across the United States, 1990–2010, J. Geophys. Res.-Atmos., 117, D22307, https://doi.org/10.1029/2012jd018261, 2012.
Cynthia Lin, C. Y., Jacob, D. J., Munger, J. W., and Fiore, A. M.: Increasing background ozone in surface air over the United States, Geophys. Res. Lett., 27, 3465–3468, 2000.
De Smedt, I., Theys, N., Yu, H., Danckaert, T., Lerot, C., Compernolle, S., Van Roozendael, M., Richter, A., Hilboll, A., Peters, E., Pedergnana, M., Loyola, D., Beirle, S., Wagner, T., Eskes, H., van Geffen, J., Boersma, K. F., and Veefkind, P.: Algorithm theoretical baseline for formaldehyde retrievals from S5P TROPOMI and from the QA4ECV project, Atmos. Meas. Tech., 11, 2395–2426, https://doi.org/10.5194/amt-11-2395-2018, 2018.
De Smedt, I., Pinardi, G., Vigouroux, C., Compernolle, S., Bais, A., Benavent, N., Boersma, F., Chan, K.-L., Donner, S., Eichmann, K.-U., Hedelt, P., Hendrick, F., Irie, H., Kumar, V., Lambert, J.-C., Langerock, B., Lerot, C., Liu, C., Loyola, D., Piters, A., Richter, A., Rivera Cárdenas, C., Romahn, F., Ryan, R. G., Sinha, V., Theys, N., Vlietinck, J., Wagner, T., Wang, T., Yu, H., and Van Roozendael, M.: Comparative assessment of TROPOMI and OMI formaldehyde observations and validation against MAX-DOAS network column measurements, Atmos. Chem. Phys., 21, 12561–12593, https://doi.org/10.5194/acp-21-12561-2021, 2021.
Dimitropoulou, E., Hendrick, F., Pinardi, G., Friedrich, M. M., Merlaud, A., Tack, F., De Longueville, H., Fayt, C., Hermans, C., Laffineur, Q., Fierens, F., and Van Roozendael, M.: Validation of TROPOMI tropospheric NO2 columns using dual-scan multi-axis differential optical absorption spectroscopy (MAX-DOAS) measurements in Uccle, Brussels, Atmos. Meas. Tech., 13, 5165–5191, https://doi.org/10.5194/amt-13-5165-2020, 2020.
Ding, J., Dai, Q., Fan, W., Lu, M., Zhang, Y., Han, S., and Feng, Y.: Impacts of meteorology and precursor emission change on O3 variation in Tianjin, China from 2015 to 2021, J. Environ. Sci.-China, 126, 506–516, https://doi.org/10.1016/j.jes.2022.03.010, 2023.
Gao, A., Wang, J., Poetzscher, J., Li, S., Gao, B., Wang, P., Luo, J., Fang, X., Li, J., Hu, J., Gao, J., and Zhang, H.: Coordinated health effects attributable to particulate matter and other pollutants exposures in the North China Plain, Environ. Res., 208, 112671, https://doi.org/10.1016/j.envres.2021.112671, 2022.
Garcia, A. R., Volkamer, R., Molina, L. T., Molina, M. J., Samuelson, J., Mellqvist, J., Galle, B., Herndon, S. C., and Kolb, C. E.: Separation of emitted and photochemical formaldehyde in Mexico City using a statistical analysis and a new pair of gas-phase tracers, Atmos. Chem. Phys., 6, 4545–4557, https://doi.org/10.5194/acp-6-4545-2006, 2006.
Gaudel, A., Cooper, O. R., Chang, K.-L., Bourgeois, I., Ziemke, J. R., Strode, S. A., Oman, L. D., Sellitto, P., Nédélec, P., and Blot, R.: Aircraft observations since the 1990s reveal increases of tropospheric ozone at multiple locations across the Northern Hemisphere, Sci. Adv., 6, eaba8272, https://doi.org/10.1126/sciadv.aba8272, 2020.
Guan, Y., Xiao, Y., Rong, B., Zhang, N., and Chu, C.: Long-term health impacts attributable to PM2.5 and ozone pollution in China's most polluted region during 2015–2020, J. Clean. Prod., 321, 128970, https://doi.org/10.1016/j.jclepro.2021.128970, 2021.
Han, H., Liu, J., Shu, L., Wang, T., and Yuan, H.: Local and synoptic meteorological influences on daily variability in summertime surface ozone in eastern China, Atmos. Chem. Phys., 20, 203–222, https://doi.org/10.5194/acp-20-203-2020, 2020.
Heue, K.-P., Riede, H., Walter, D., Brenninkmeijer, C. A. M., Wagner, T., Frieß, U., Platt, U., Zahn, A., Stratmann, G., and Ziereis, H.: CARIBIC DOAS observations of nitrous acid and formaldehyde in a large convective cloud, Atmos. Chem. Phys., 14, 6621–6642, https://doi.org/10.5194/acp-14-6621-2014, 2014.
Hong, Q., Liu, C., Chan, K. L., Hu, Q., Xie, Z., Liu, H., Si, F., and Liu, J.: Ship-based MAX-DOAS measurements of tropospheric NO2, SO2, and HCHO distribution along the Yangtze River, Atmos. Chem. Phys., 18, 5931–5951, https://doi.org/10.5194/acp-18-5931-2018, 2018.
Hong, Q., Zhu, L., Xing, C., Hu, Q., Lin, H., Zhang, C., Zhao, C., Liu, T., Su, W., and Liu, C.: Inferring vertical variability and diurnal evolution of O3 formation sensitivity based on the vertical distribution of summertime HCHO and NO2 in Guangzhou, China, Sci. Total Environ., 827, 154045, https://doi.org/10.1016/j.scitotenv.2022.154045, 2022.
Hu, W., She, D., Xia, J., He, B., and Hu, C.: Dominant patterns of dryness/wetness variability in the Huang-Huai-Hai River Basin and its relationship with multiscale climate oscillations, Atmos. Res., 247, 105148, https://doi.org/10.1016/j.atmosres.2020.105148, 2021.
Jacob, D. J., Logan, J. A., and Murti, P. P.: Effect of rising Asian emissions on surface ozone in the United States, Geophys. Res. Lett., 26, 2175–2178, 1999.
Jin, H., Chen, X., Zhong, R., and Duan, K.: Frequency analysis of extreme precipitation in different regions of the Huaihe River Basin, Int. J. Climatol., 42, 3517–3536, https://doi.org/10.1002/joc.7430, 2021.
Lang, J., Liang, X., Li, S., Zhou, Y., Chen, D., Zhang, Y., and Xu, L.: Understanding the impact of vehicular emissions on air pollution from the perspective of regional transport: A case study of the Beijing-Tianjin-Hebei region in China, Sci. Total Environ., 785, 147304, https://doi.org/10.1016/j.scitotenv.2021.147304, 2021.
Lefohn, A. S., Hazucha, M. J., Shadwick, D., and Adams, W. C.: An alternative form and level of the human health ozone standard, Inhal. Toxicol., 22, 999–1011, 2010.
Li, J., Lu, K., Lv, W., Li, J., Zhong, L., Ou, Y., Chen, D., Huang, X., and Zhang, Y.: Fast increasing of surface ozone concentrations in Pearl River Delta characterized by a regional air quality monitoring network during 2006–2011, J. Environ. Sci.-China, 26, 23–36, https://doi.org/10.1016/s1001-0742(13)60377-0, 2014.
Li, K., Jacob, D. J., Liao, H., Shen, L., Zhang, Q., and Bates, K. H.: Anthropogenic drivers of 2013–2017 trends in summer surface ozone in China, P. Natl. Acad. Sci. USA, 116, 422–427, https://doi.org/10.1073/pnas.1812168116, 2018.
Li, K., Jacob, D. J., Shen, L., Lu, X., De Smedt, I., and Liao, H.: Increases in surface ozone pollution in China from 2013 to 2019: anthropogenic and meteorological influences, Atmos. Chem. Phys., 20, 11423–11433, https://doi.org/10.5194/acp-20-11423-2020, 2020.
Li, K., Jacob, D. J., Liao, H., Qiu, Y., Shen, L., Zhai, S., Bates, K. H., Sulprizio, M. P., Song, S., Lu, X., Zhang, Q., Zheng, B., Zhang, Y., Zhang, J., Lee, H. C., and Kuk, S. K.: Ozone pollution in the North China Plain spreading into the late-winter haze season, P. Natl. Acad. Sci. USA, 118, e2015797118, https://doi.org/10.1073/pnas.2015797118, 2021.
Li, L., Yang, W., Xie, S., and Wu, Y.: Estimations and uncertainty of biogenic volatile organic compound emission inventory in China for 2008–2018, Sci. Total Environ., 733, 139301, https://doi.org/10.1016/j.scitotenv.2020.139301, 2020.
Li, M., Liu, H., Geng, G., Hong, C., Liu, F., Song, Y., Tong, D., Zheng, B., Cui, H., Man, H., Zhang, Q., and He, K.: Anthropogenic emission inventories in China: a review, Natl. Sci. Rev., 4, 834–866, https://doi.org/10.1093/nsr/nwx150, 2017.
Li, M., Huang, X., Yan, D., Lai, S., Zhang, Z., Zhu, L., Lu, Y., Jiang, X., Wang, N., Wang, T., Song, Y., and Ding, A.: Coping with the concurrent heatwaves and ozone extremes in China under a warming climate, Sci. Bull., 69, 2938–2947, https://doi.org/10.1016/j.scib.2024.05.034, 2024.
Li, X.-B., Yuan, B., Parrish, D. D., Chen, D., Song, Y., Yang, S., Liu, Z., and Shao, M.: Long-term trend of ozone in southern China reveals future mitigation strategy for air pollution, Atmos. Environ., 269, 118869, https://doi.org/10.1016/j.atmosenv.2021.118869, 2022.
Li, X., Ren, J., Huang, R., Chen, L., Li, Y., Qiao, X., Cheng, Y., Zhao, B., Yin, D., Gao, D., Sun, Y., and Zhang, F.: The Aggravation of Summertime Nocturnal Ozone Pollution in China and Its Potential Impact on the Trend of Nitrate Aerosols, Geophys. Res. Lett., 50, e2023GL103242, https://doi.org/10.1029/2023gl103242, 2023.
Lin, H., Xing, C., Hong, Q., Liu, C., Ji, X., Liu, T., Lin, J., Lu, C., Tan, W., Li, Q., and Liu, H.: Diagnosis of Ozone Formation Sensitivities in Different Height Layers via MAX-DOAS Observations in Guangzhou, J. Geophys. Res.-Atmos., 127, e2022JD036803, https://doi.org/10.1029/2022jd036803, 2022.
Lin, N., Wang, Y., Zhang, Y., and Yang, K.: A large decline of tropospheric NO2 in China observed from space by SNPP OMPS, Sci. Total Environ., 675, 337–342, https://doi.org/10.1016/j.scitotenv.2019.04.090, 2019.
Liu, C., Hu, Q., Zhang, C., Xia, C., Yin, H., Su, W., Wang, X., Xu, Y., and Zhang, Z.: First Chinese ultraviolet–visible hyperspectral satellite instrument implicating global air quality during the COVID-19 pandemic in early 2020, Light: Science & Applications, 11, 28, https://doi.org/10.1038/s41377-022-00722-x, 2022a.
Liu, C., Xing, C., Hu, Q., Li, Q., Liu, H., Hong, Q., Tan, W., Ji, X., Lin, H., Lu, C., Lin, J., Liu, H., Wei, S., Chen, J., Yang, K., Wang, S., Liu, T., and Chen, Y.: Ground-Based Hyperspectral Stereoscopic Remote Sensing Network: A Promising Strategy to Learn Coordinated Control of O3 and PM2.5 over China, Engineering, 19, 71–83, https://doi.org/10.1016/j.eng.2021.02.019, 2022b.
Liu, J., Wang, S., Zhang, Y., Yan, Y., Zhu, J., Zhang, S., Wang, T., Tan, Y., and Zhou, B.: Investigation of formaldehyde sources and its relative emission intensity in shipping channel environment, J. Environ. Sci.-China, 142, 142–154, https://doi.org/10.1016/j.jes.2023.06.020, 2024.
Liu, Y., Geng, G., Cheng, J., Liu, Y., Xiao, Q., Liu, L., Shi, Q., Tong, D., He, K., and Zhang, Q.: Drivers of Increasing Ozone during the Two Phases of Clean Air Actions in China 2013–2020, Environ. Sci. Technol., 57, 8954–8964, https://doi.org/10.1021/acs.est.3c00054, 2023.
Lu, C., Li, Q., Xing, C., Hu, Q., Tan, W., Lin, J., Zhang, Z., Tang, Z., Cheng, J., Chen, A., and Liu, C.: Identification of O3 Sensitivity to Secondary HCHO and NO2 Measured by MAX-DOAS in Four Cities in China, Remote Sens., 16, 662, https://doi.org/10.3390/rs16040662, 2024.
Lu, X., Zhang, L., Wang, X., Gao, M., Li, K., Zhang, Y., Yue, X., and Zhang, Y.: Rapid Increases in Warm-Season Surface Ozone and Resulting Health Impact in China Since 2013, Environ. Sci. Tech. Let., 7, 240–247, https://doi.org/10.1021/acs.estlett.0c00171, 2020.
Ma, Y., Diao, Y., Zhang, B., Wang, W., Ren, X., Yang, D., Wang, M., Shi, X., and Zheng, J.: Detection of formaldehyde emissions from an industrial zone in the Yangtze River Delta region of China using a proton transfer reaction ion-drift chemical ionization mass spectrometer, Atmos. Meas. Tech., 9, 6101–6116, https://doi.org/10.5194/amt-9-6101-2016, 2016.
Ma, Z., Liu, C., Zhang, C., Liu, P., Ye, C., Xue, C., Zhao, D., Sun, J., Du, Y., Chai, F., and Mu, Y.: The levels, sources and reactivity of volatile organic compounds in a typical urban area of Northeast China, J. Environ. Sci.-China, 79, 121–134, https://doi.org/10.1016/j.jes.2018.11.015, 2019.
MacDonald, S. M., Oetjen, H., Mahajan, A. S., Whalley, L. K., Edwards, P. M., Heard, D. E., Jones, C. E., and Plane, J. M. C.: DOAS measurements of formaldehyde and glyoxal above a south-east Asian tropical rainforest, Atmos. Chem. Phys., 12, 5949–5962, https://doi.org/10.5194/acp-12-5949-2012, 2012.
Mousavinezhad, S., Choi, Y., Pouyaei, A., Ghahremanloo, M., and Nelson, D. L.: A comprehensive investigation of surface ozone pollution in China, 2015–2019: Separating the contributions from meteorology and precursor emissions, Atmos. Res., 257, 105599, https://doi.org/10.1016/j.atmosres.2021.105599, 2021.
NASA: Earthdata Search, https://search.earthdata.nasa.gov/search, last access: 5 January 2025.
Possanzini, M., Di Palo, V., and Cecinato, A.: Sources and photodecomposition of formaldehyde and acetaldehyde in Rome ambient air, Atmos. Environ., 36, 3195–3201, 2002.
Ren, B., Xie, P., Xu, J., Li, A., Qin, M., Hu, R., Zhang, T., Fan, G., Tian, X., Zhu, W., Hu, Z., Huang, Y., Li, X., Meng, F., Zhang, G., Tong, J., Ren, H., Zheng, J., Zhang, Z., and Lv, Y.: Vertical characteristics of NO2 and HCHO, and the ozone formation regimes in Hefei, China, Sci. Total Environ., 823, 153425, https://doi.org/10.1016/j.scitotenv.2022.153425, 2022.
Ren, J., Guo, F., and Xie, S.: Diagnosing ozone–NOx–VOC sensitivity and revealing causes of ozone increases in China based on 2013–2021 satellite retrievals, Atmos. Chem. Phys., 22, 15035–15047, https://doi.org/10.5194/acp-22-15035-2022, 2022.
Song, Y., Xing, C., Liu, C., Lin, J., Wu, H., Liu, T., Lin, H., Zhang, C., Tan, W., Ji, X., Liu, H., and Li, Q.: Evaluation of transport processes over North China Plain and Yangtze River Delta using MAX-DOAS observations, Atmos. Chem. Phys., 23, 1803–1824, https://doi.org/10.5194/acp-23-1803-2023, 2023.
Su, W., Liu, C., Hu, Q., Fan, G., Xie, Z., Huang, X., Zhang, T., Chen, Z., Dong, Y., Ji, X., Liu, H., Wang, Z., and Liu, J.: Characterization of ozone in the lower troposphere during the 2016 G20 conference in Hangzhou, Sci. Rep., 7, 17368, https://doi.org/10.1038/s41598-017-17646-x, 2017.
Sun, X., Zhao, T., Bai, Y., Kong, S., Zheng, H., Hu, W., Ma, X., and Xiong, J.: Meteorology impact on PM2.5 change over a receptor region in the regional transport of air pollutants: observational study of recent emission reductions in central China, Atmos. Chem. Phys., 22, 3579–3593, https://doi.org/10.5194/acp-22-3579-2022, 2022.
Sun, Y., Yin, H., Liu, C., Zhang, L., Cheng, Y., Palm, M., Notholt, J., Lu, X., Vigouroux, C., Zheng, B., Wang, W., Jones, N., Shan, C., Qin, M., Tian, Y., Hu, Q., Meng, F., and Liu, J.: Mapping the drivers of formaldehyde (HCHO) variability from 2015 to 2019 over eastern China: insights from Fourier transform infrared observation and GEOS-Chem model simulation, Atmos. Chem. Phys., 21, 6365–6387, https://doi.org/10.5194/acp-21-6365-2021, 2021.
Taguchi, S., Hagiwara, M., Shibata, A., Fujinari, H., Matsumoto, S., Kuwata, M., Sazawa, K., Hata, N., and Kuramitz, H.: Investigation and modeling of diurnal variation in suburban ambient formaldehyde concentration, Environ. Sci. Pollut. R., 28, 13425–13438, https://doi.org/10.1007/s11356-020-11465-w, 2020.
van Geffen, J., Boersma, K. F., Eskes, H., Sneep, M., ter Linden, M., Zara, M., and Veefkind, J. P.: S5P TROPOMI NO2 slant column retrieval: method, stability, uncertainties and comparisons with OMI, Atmos. Meas. Tech., 13, 1315–1335, https://doi.org/10.5194/amt-13-1315-2020, 2020.
Veefkind, J. P., Aben, I., McMullan, K., Förster, H., de Vries, J., Otter, G., Claas, J., Eskes, H. J., de Haan, J. F., Kleipool, Q., van Weele, M., Hasekamp, O., Hoogeveen, R., Landgraf, J., Snel, R., Tol, P., Ingmann, P., Voors, R., Kruizinga, B., Vink, R., Visser, H., and Levelt, P. F.: TROPOMI on the ESA Sentinel-5 Precursor: A GMES mission for global observations of the atmospheric composition for climate, air quality and ozone layer applications, Remote Sens. Environ., 120, 70–83, https://doi.org/10.1016/j.rse.2011.09.027, 2012.
Wang, M., Chen, W., Shao, M., Lu, S., Zeng, L., and Hu, M.: Investigation of carbonyl compound sources at a rural site in the Yangtze River Delta region of China, J. Environ. Sci.-China, 28, 128–136, https://doi.org/10.1016/j.jes.2014.12.001, 2015.
Wang, N., Lyu, X., Deng, X., Huang, X., Jiang, F., and Ding, A.: Aggravating O3 pollution due to NOx emission control in eastern China, Sci. Total Environ., 677, 732–744, https://doi.org/10.1016/j.scitotenv.2019.04.388, 2019.
Wang, W., Parrish, D. D., Wang, S., Bao, F., Ni, R., Li, X., Yang, S., Wang, H., Cheng, Y., and Su, H.: Long-term trend of ozone pollution in China during 2014–2020: distinct seasonal and spatial characteristics and ozone sensitivity, Atmos. Chem. Phys., 22, 8935–8949, https://doi.org/10.5194/acp-22-8935-2022, 2022.
Wang, X.: Air Quality and Weather, https://quotsoft.net/air/, last access: 5 January 2025.
Wang, Y., Yang, X., Wu, K., Mei, H., De Smedt, I., Wang, S., Fan, J., Lyu, S., and He, C.: Long-term trends of ozone and precursors from 2013 to 2020 in a megacity (Chengdu), China: Evidence of changing emissions and chemistry, Atmos. Res., 278, 106309, https://doi.org/10.1016/j.atmosres.2022.106309, 2022.
Wang, Y., Jiang, S., Huang, L., Lu, G., Kasemsan, M., Yaluk, E. A., Liu, H., Liao, J., Bian, J., Zhang, K., Chen, H., and Li, L.: Differences between VOCs and NOx transport contributions, their impacts on O3, and implications for O3 pollution mitigation based on CMAQ simulation over the Yangtze River Delta, China, Sci. Total Environ., 872, 162118, https://doi.org/10.1016/j.scitotenv.2023.162118, 2023a.
Wang, Y., Zhao, Y., Liu, Y., Jiang, Y., Zheng, B., Xing, J., Liu, Y., Wang, S., and Nielsen, C. P.: Sustained emission reductions have restrained the ozone pollution over China, Nat. Geosci., 16, 967–974, https://doi.org/10.1038/s41561-023-01284-2, 2023b.
Wang, Z.: Opposing trends in the peak and low ozone concentrations in eastern China: Anthropogenic and meteorological influences, Version V1, Mendeley Data [data set], https://doi.org/10.17632/zd9cb9dk75.1, 2024.
Wang, Z., Liu, C., Xie, Z., Hu, Q., Andreae, M. O., Dong, Y., Zhao, C., Liu, T., Zhu, Y., Liu, H., Xing, C., Tan, W., Ji, X., Lin, J., and Liu, J.: Elevated dust layers inhibit dissipation of heavy anthropogenic surface air pollution, Atmos. Chem. Phys., 20, 14917–14932, https://doi.org/10.5194/acp-20-14917-2020, 2020.
Wang, Z., Shi, C., Zhang, H., Chen, Y., Chi, X., Xia, C., Wang, S., Zhu, Y., Zhang, K., Chen, X., Xing, C., and Liu, C.: Measurement report: Dust and anthropogenic aerosols' vertical distributions over northern China dense aerosols gathered at the top of the mixing layer, Atmos. Chem. Phys., 23, 14271–14292, https://doi.org/10.5194/acp-23-14271-2023, 2023.
Weng, X., Forster, G. L., and Nowack, P.: A machine learning approach to quantify meteorological drivers of ozone pollution in China from 2015 to 2019, Atmos. Chem. Phys., 22, 8385–8402, https://doi.org/10.5194/acp-22-8385-2022, 2022.
Wood, E. C., Canagaratna, M. R., Herndon, S. C., Onasch, T. B., Kolb, C. E., Worsnop, D. R., Kroll, J. H., Knighton, W. B., Seila, R., Zavala, M., Molina, L. T., DeCarlo, P. F., Jimenez, J. L., Weinheimer, A. J., Knapp, D. J., Jobson, B. T., Stutz, J., Kuster, W. C., and Williams, E. J.: Investigation of the correlation between odd oxygen and secondary organic aerosol in Mexico City and Houston, Atmos. Chem. Phys., 10, 8947–8968, https://doi.org/10.5194/acp-10-8947-2010, 2010.
Wu, Y., Chen, W., You, Y., Xie, Q., Jia, S., and Wang, X.: Quantitative impacts of vertical transport on the long-term trend of nocturnal ozone increase over the Pearl River Delta region during 2006–2019, Atmos. Chem. Phys., 23, 453–469, https://doi.org/10.5194/acp-23-453-2023, 2023.
Xing, C., Liu, C., Hong, Q., Liu, H., Wu, H., Lin, J., Song, Y., Chen, Y., Liu, T., Hu, Q., Tan, W., and Lin, H.: Vertical distributions and potential sources of wintertime atmospheric pollutants and the corresponding ozone production on the coast of Bohai Sea, J. Environ. Manage., 319, 115721, https://doi.org/10.1016/j.jenvman.2022.115721, 2022.
Xing, C., Liu, C., Lin, J., Tan, W., and Liu, T.: VOCs hyperspectral imaging: A new insight into evaluate emissions and the corresponding health risk from industries, J. Hazard. Mater., 461, 132573, https://doi.org/10.1016/j.jhazmat.2023.132573, 2024.
Xue, J., Zhao, T., Luo, Y., Miao, C., Su, P., Liu, F., Zhang, G., Qin, S., Song, Y., Bu, N., and Xing, C.: Identification of ozone sensitivity for NO2 and secondary HCHO based on MAX-DOAS measurements in northeast China, Environ. Int., 160, 107048, https://doi.org/10.1016/j.envint.2021.107048, 2022.
Zhai, S., Jacob, D. J., Wang, X., Shen, L., Li, K., Zhang, Y., Gui, K., Zhao, T., and Liao, H.: Fine particulate matter (PM2.5) trends in China, 2013–2018: separating contributions from anthropogenic emissions and meteorology, Atmos. Chem. Phys., 19, 11031–11041, https://doi.org/10.5194/acp-19-11031-2019, 2019.
Zhang, Q., Zheng, Y., Tong, D., Shao, M., Wang, S., Zhang, Y., Xu, X., Wang, J., He, H., Liu, W., Ding, Y., Lei, Y., Li, J., Wang, Z., Zhang, X., Wang, Y., Cheng, J., Liu, Y., Shi, Q., Yan, L., Geng, G., Hong, C., Li, M., Liu, F., Zheng, B., Cao, J., Ding, A., Gao, J., Fu, Q., Huo, J., Liu, B., Liu, Z., Yang, F., He, K., and Hao, J.: Drivers of improved PM2.5 air quality in China from 2013 to 2017, P. Natl. Acad. Sci. USA, 116, 24463–24469, https://doi.org/10.1073/pnas.1907956116, 2019.
Zhang, T., Tam, C.-Y., Lau, N.-C., Wang, J., Yang, S., Chen, J., Yu, W., Jiang, X., and Gao, P.: Influences of the boreal winter Arctic Oscillation on the peak-summer compound heat waves over the Yangtze–Huaihe River basin: the North Atlantic capacitor effect, Clim. Dynam., 59, 2331–2343, https://doi.org/10.1007/s00382-022-06212-5, 2022.
Zhang, T., Deng, Y., Chen, J., Yang, S., and Dai, Y.: An energetics tale of the 2022 mega-heatwave over central-eastern China, npj Clim. Atmos. Sci., 6, 162, https://doi.org/10.1038/s41612-023-00490-4, 2023.
Zhang, W., Pan, S., Cao, L., Cai, X., Zhang, K., Xu, Y., and Xu, W.: Changes in extreme climate events in eastern China during 1960–2013: A case study of the Huaihe River Basin, Quatern. Int., 380–381, 22–34, https://doi.org/10.1016/j.quaint.2014.12.038, 2015.
Zhang, X., Xu, W., Zhang, G., Lin, W., Zhao, H., Ren, S., Zhou, G., Chen, J., and Xu, X.: First long-term surface ozone variations at an agricultural site in the North China Plain: Evolution under changing meteorology and emissions, Sci. Total Environ., 860, 160520, https://doi.org/10.1016/j.scitotenv.2022.160520, 2023.
Zhang, Y.-L. and Cao, F.: Fine particulate matter (PM2.5) in China at a city level, Sci. Rep., 5, 14884, https://doi.org/10.1038/srep14884, 2015.
Zheng, B., Tong, D., Li, M., Liu, F., Hong, C., Geng, G., Li, H., Li, X., Peng, L., Qi, J., Yan, L., Zhang, Y., Zhao, H., Zheng, Y., He, K., and Zhang, Q.: Trends in China's anthropogenic emissions since 2010 as the consequence of clean air actions, Atmos. Chem. Phys., 18, 14095–14111, https://doi.org/10.5194/acp-18-14095-2018, 2018.