the Creative Commons Attribution 4.0 License.
the Creative Commons Attribution 4.0 License.
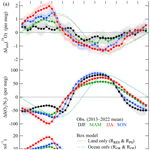
Diurnal, seasonal, and interannual variations in δ(18O) of atmospheric O2 and its application to evaluate natural and anthropogenic changes in oxygen, carbon, and water cycles
Satoshi Sugawara
Atsushi Okazaki
Variations in the δ(18O) of atmospheric O2, δatm(18O), are an indicator of biological and water processes associated with the Dole–Morita effect (DME). The DME and its variations have been observed in ice cores for paleoclimate studies; however, variations in present-day δatm(18O) have never been detected so far. Here, we present diurnal, seasonal, and interannual variations of δatm(18O) based on observations at a surface site in central Japan. The average diurnal δatm(18O) cycle reached a minimum during the daytime, and its amplitude was larger in summer than in winter. We found that use of δatm(18O) enabled separation of variations of atmospheric δ() into contributions from biological activities and fossil fuel combustion. The average seasonal δatm(18O) cycle reached at a minimum in summer, and the peak-to-peak amplitude was about 2 per meg (1 per meg is 0.001 ‰). A box model that incorporated biological and water processes reproduced the general characteristics of the observed diurnal and seasonal cycles. A slight but significant secular increase in δatm(18O) by (0.22 ± 0.14) per meg a−1 occurred during 2013–2022. Secular changes in δatm(18O) were also simulated by using the box model considering long-term changes in terrestrial gross primary production (GPP), photorespiration, and δ(18O) of leaf water (δLW(18O)). We calculated changes in δLW(18O) using a state-of-the-art, three-dimensional model, MIROC5-iso. The observed secular increase in δatm(18O) was reproduced by the box model that incorporated the isotopic effects associated with the DME from Bender et al. (1994), while the simulated δatm(18O) showed a secular decrease when the model incorporated the isotopic effects from Luz and Barkan (2011). Therefore, long-term observations of δatm(18O) and better understanding of the DME are indispensable for an application of δatm(18O) to constrain long-term changes in global GPP and photorespiration.
- Article
(5074 KB) - Full-text XML
- BibTeX
- EndNote
The ratio of atmospheric O2, δatm(18O), is about 24 ‰ higher than that of ocean water (per definition 0 ‰ according to Vienna Standard Mean Ocean Water – VSMOW) due to various processes in the global oxygen and water cycle (e.g., Craig, 1961; Barkan and Luz, 2005). The enrichment of δatm(18O) is well known as the Dole–Morita effect (DME) (Dole, 1935; Morita, 1935). The DME is determined from the balance between enrichment of δatm(18O) due to discrimination against 18O during terrestrial and marine respiratory O2 consumption and the terrestrial and marine photosynthetic O2 flux, for which the δ(18O) is close to that of ocean water. Bender et al. (1994) (hereafter referred to as “B94”) reported that the isotopic effects of dark respiration, photorespiration, and the Mehler reaction associated with terrestrial respiration are 18 ‰, 21.2 ‰, and 15.1 ‰, respectively, and the terrestrial photosynthetic O2 flux is also affected by discrimination against 18O during evapotranspiration (4.4 ‰) (see Table 1 of B94 for a summary of the isotopic effects related to the DME). Luz and Barkan (2011) (hereafter referred to as “L&B11”) also reported the isotopic effects of dark respiration, photorespiration, and evapotranspiration to be 15.8 ‰, 22 ‰, and 6.5 ‰, respectively. The DME is a useful tool for examining Earth system models because it integrates land and ocean biological and climatic components (e.g., Bender et al., 1994; Luz et al., 1999; Angert et al., 2001, 2003; Hoffmann et al., 2004; Barkan and Luz, 2005; Severinghaus et al., 2009; Luz and Barkan, 2011). Some paleoclimate studies have focused on the temporal changes in δatm(18O). B94 have reported that the DME is on average lower by 0.05 ‰ than that of air during the past 130 000 years, and the standard deviation of the DME from the average was ±0.2 ‰. They suggested that the DME was nearly unchanged between glacial maxima and interglacial periods, and the variability is small and may be due to variations of the relative rates of primary production on the land and in the ocean. Severinghaus et al. (2009) reported the δatm(18O) in the Siple Dome ice core, Antarctica, and have found that its variations over the past 60 kyr are related to Heinrich and Dansgaard–Oeschger events. They have suggested that the DME is primarily governed by the strength of the Asian and North African monsoons and have confirmed that widespread changes in low-latitude terrestrial rainfall accompany abrupt climate changes.
Table 1Budgets and isotopic effects of the atmospheric O2 used in the box model. Isotopic values of the external sources and DME are described versus VSMOW. B94 and L&B11 denote Bender et al. (1994) and Luz and Barkan (2011), respectively.
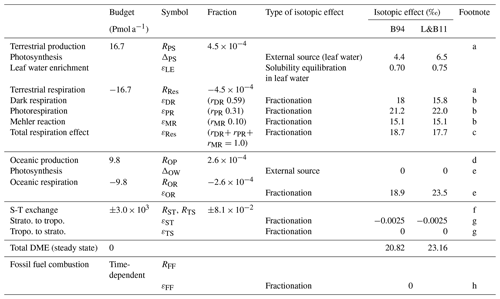
a 16.7 Pmol a−1 is the value by Hoffmann et al. (2004). R values were calculated by assuming that the total amount of atmospheric O2 is 3.706×104 Pmol. b The relative ratios of dark respiration, photorespiration, and Mehler reaction, as well as the isotopic effect of the Mehler reaction by B94. c , which becomes the same value by L&B11. d Calculated by using the ratio of 0.63:0.37 for the fraction of O2 production by L&B11. e L&B11 showed that the total oceanic DME is 23.5 ‰ and that fractionations of oceanic photosynthesis exist. We assume that the same fractionation occurs only by respiration to realize the same extent of oceanic DME. f Calculated by using the net mass flux of S-T exchange in Olsen et al. (2004). g The stratospheric diminution effect was calculated as the 18O discrimination in stratosphere–troposphere exchange. Because the S-T exchange flux is about 100 times larger than the total terrestrial and oceanic flux, this contribution to the DME becomes about −0.3 ‰ ( (RPS+ROP)), which is the same value by L&B11. h We assume that atmospheric oxygen is consumed without isotope effects in fossil fuel combustion considering high temperature during industrial combustion processes.
Hoffmann et al. (2004) developed a model of the DME by combining the results of three-dimensional (3D) models of carbon and oxygen cycles with results of atmospheric general circulation models with built-in water isotope diagnostics and have obtained an average DME of 22.4 ‰ to 23.3 ‰. However, they did not simulate temporal or spatial variations of δ18Oatm in the present atmosphere, which have not yet been detected. The diurnal cycle of the atmospheric ratio at forest sites is caused mainly by activities in the terrestrial biosphere, and the peak-to-peak amplitudes are roughly 100 per meg (1 per meg is 0.001 ‰) (e.g., Ishidoya et al., 2013a; Battle et al., 2019; Faassen et al., 2023). Diurnal variations of δ18Oatm associated with activities in the terrestrial biosphere are therefore expected to be very small. Keeling (1995) predicted that δatm(18O) should be lower in summer than in winter in both hemispheres by about 2 per meg by assuming a 100 per meg seasonal increase in the atmospheric ratio due to the input of terrestrial and oceanic photosynthetic O2, which has a δ(18O) that is lower than δatm(18O) by 20 ‰. Seibt et al. (2005), who calculated potential effects of human activity on the DME, estimated that global changes in the terrestrial biosphere may have led to a decrease in δatm(18O) on the order of 70 per meg over the last 150 years (−0.5 per meg a−1). They have estimated that of the total decrease is due to a decrease in photorespiration globally accompanied by a 100 µmol mol−1 increase in the fraction of atmospheric CO2 during those 150 years. Diurnal, seasonal, and secular changes in δatm(18O) in the present atmosphere will therefore be a new indicator of activities of the land and oceanic biospheres, although sufficiently precise measurements of δatm(18O) to validate the suggestions by Keeling (1995) and Seibt et al. (2005) have never been reported.
In this study, we present diurnal, seasonal, and secular changes in δatm(18O) observed at Tsukuba (TKB), Japan (36° N, 140° E). We then compare the observed changes in δatm(18O) at TKB with a one-box model that incorporates the biosphere and water processes associated with the DME. To evaluate the secular changes in water processes, we used an isotope-enabled version of the Model for Interdisciplinary Research on Climate (MIROC5-iso) (Okazaki and Yoshimura, 2019) and calculated the δ(18O) of leaf water, δLW(18O). We suggest some applications of δatm(18O): (1) separation of the diurnal δ() cycle into contributions from biological activities and fossil fuel combustion, (2) constraint of the seasonal δLW(18O) cycle, and (3) evaluation of recent secular changes in terrestrial gross primary production (GPP) and photorespiration.
2.1 Continuous atmospheric measurements of δatm(18O) and δ()
Air was sampled with a diaphragm pump from an air intake located on the roof of a laboratory building of the National Institute of Advanced Industrial Science and Technology (AIST) at TKB. The gas velocity exceeded 5 m s−1(4 mm i.d. and a flow rate of 4 L min−1) at the tip of the air intake, which was high enough to prevent thermally diffusive inlet fractionation (Sturm et al., 2006; Blaine et al., 2006). The sample air was introduced into a 1 L, stainless-steel buffer tank after water vapor in the air had been reduced by using an electric cooling unit at 2 °C. The gas was then exhausted from the buffer tank at a flow rate of about 4 L min−1. A small portion of this exhausted gas was introduced into a 3.2 mm (1/8 in.) o.d. stainless-steel tube, and any remaining water vapor was removed using a cold trap at −90 °C. Finally, the remaining sample air was vented through an outlet path at a rate of about 10 mL min−1, and a minuscule amount of it was transferred to the ion source (or waste line) of a mass spectrometer (Thermo Fisher Scientific Delta V) through a thin, insulated, fused-silica capillary. The reference air was always supplied from a high-pressure cylinder at a flow rate of about 4 mL min−1, and a minuscule amount of it was introduced into the ion source (or waste line) of the mass spectrometer through another fused-silica capillary. The standard air, which was supplied from a high-pressure cylinder at a flow rate of 4 mL min−1, was introduced like the reference air into the ion source (or waste line) of the mass spectrometer, but through the line for sample air. We analyzed the standard air about once every 2 months. Details of the continuous measurement system we used have been reported by Ishidoya and Murayama (2014).
We repeatedly conducted alternate analyses of the sample and reference air for continuous measurements of stable isotopic ratios of O2, N2, and Ar (δatm(18O), δatm(15N), and δatm(40Ar)) as well as the ratio and amount fraction of CO2. The time required to obtain a measured value was 62 s. However, the standard deviation of the δatm(18O) was about 20 per meg, which was much larger than the standard deviation required to detect the expected respective seasonal (2 per meg) and secular changes (−0.5 per meg a−1) in δatm(18O) calculated by Keeling (1995) and Seibt et al. (2005). We therefore averaged more than 1000 data points and used the averaged value as the observed δatm(18O). This averaging theoretically results in a standard error of the observed δatm(18O) of less than 0.6 per meg assuming no temporal drift during the averaging period. In this regard, we confirmed that the measured values of the δatm(18O) against reference air were stable enough for a period much longer than the averaging period. We therefore needed to calibrate with a primary and secondary air standard (described below) only once every 2 months. Figure 1 shows an example of the measured δatm(18O) of a standard air against reference air. We found the standard deviations of 200 and 400 averaged data points to be 1.4 and 0.4 per meg, respectively, which are consistent with the theoretically expected values of 1.3 and 1.0 per meg. In general, mass spectrometer behavior can change suddenly due to maintenance, such as a filament change or ion source tuning. To minimize the uncertainties associated with the changes in the conditions of the mass spectrometer, we used specific filaments for the measurements of air samples with the atmospheric level amount fraction of O2 supplied by the Thermo Fisher Scientific. This enabled us to carry out continuous measurements in the present study for 11 months without exchanging the filament (when we used the original filament supplied for the mass spectrometer, then we needed to exchange it every 3 months). After the exchange of the filament, several weeks are needed to stabilize the condition of the ion source of the mass spectrometer by flowing the sample and reference air, especially for the elemental ratios of , , and . Once the condition was stabilized, we did not tune the ion source throughout the period using the same filament. Furthermore, the mass spectrometer was dedicated only to measurements of δatm(18O) and related components, including those for flask samples (e.g., Ishidoya et al., 2021, 2022), and it was run day and night autonomously to keep the condition of the ion source.
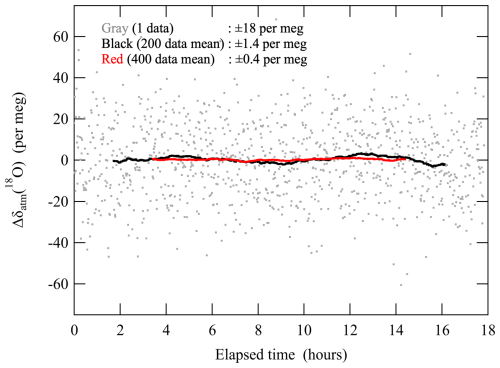
Figure 1Typical analytical results of the difference (Δ) of the δatm(18O) of standard air against a reference air. Data are shown as deviations from the average value throughout the analysis. Gray dots, black lines, and red lines denote raw data and averages of 200 and 400 data points (corresponding to about 62 s, 3.5 h, and 7 h), respectively.
The δatm(18O) and δ() are reported in per meg:
Here, the subscripts “sa” and “st” indicate the sample air and the standard air, respectively. Because O2 constitutes 0.2093 mol mol−1 of air by volume (Aoki et al., 2019), a change of 4.8 per meg in δ() is equivalent to about a change of 1 µmol mol−1. In this study, the δatm(18O) and δ() of each air sample were determined against our primary standard air (cylinder no. CRC00045) using a mass spectrometer. Our standards were dried in ambient air or industrially purified-air-based CO2 in 48 L high-pressure aluminum cylinders. The standards were classified as either primary or secondary. Figure 2 shows the value of each analysis and the corresponding annual average of δatm(18O) of three secondary standards against the primary air standard. As shown in Fig. 2, variations of the annual average δatm(18O) of our three secondary standards were within ±0.8 to ±1.1 per meg (±0.9 per meg, on average) and nearly stable for 10 years with respect to the primary standard. We therefore allowed an uncertainty of ±0.9 per meg associated with the stability of the standard air for the annual average δatm(18O) in this study. This uncertainty corresponds to an uncertainty of ±0.13 () per meg a−1 for the 10-year-long secular trend.
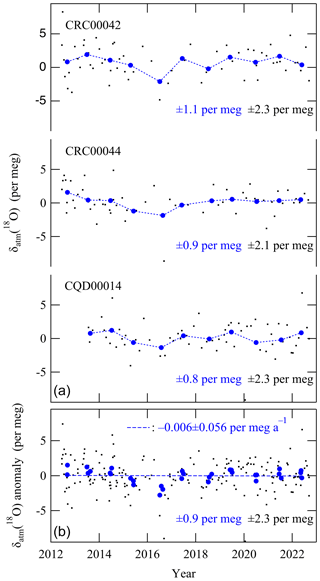
Figure 2(a) Each value (black dots) and the corresponding annual average (blue circles) of δatm(18O) of three secondary standards against the primary standard air. (b) Anomalies of δatm(18O) of the three secondary standards. The dashed blue line denotes the regression line fit to the data.
We have examined the influence of the amount fraction of CO2 in sample air on the δ() measured on a mass spectrometer in past studies (Ishidoya et al., 2003; Ishidoya and Murayama, 2014). In this study, we also experimentally examined the influences of the amount fraction of CO2 and the δ() on δatm(18O). Figure 3a shows typical examples of the relationships between the measured δatm(18O) of the air sample and the amount fraction of CO2. To obtain these relationships, a small amount of pure CO2 was added to the flow line of the continuous measurement system during the analysis of standard air, or 1 L flasks were analyzed before and after a small amount of pure CO2 was added to the flasks. The precision of the measurements of the flask air samples was about ±4 per meg. As seen in Fig. 3a, δatm(18O) increased linearly with increasing amount fractions of CO2. We therefore decided to correct the δatm(18O) values by using amount fractions of CO2 that were measured simultaneously. The mechanism of the positive correlation between δatm(18O) and CO2 is not clarified yet since there is no isobaric interference. In this regard, we found no significant influences of CO2 amount fraction on δatm(18O) for a different mass spectrometer: Finnigan MAT-252 (Ishidoya, 2003). This suggests that the influences should be examined carefully for each mass spectrometer. Figure 3b shows the relationships between the measured δatm(18O) values of the air samples and their δ(). To obtain these relationships, δatm(18O) and δ() were measured for 1 L flasks or 48 L cylinders before and after pure N2 was added to them. 1 L flasks were filled with the air in the cylinders for the analyses. It is apparent from Fig. 3b that we did not find a clearly increasing or decreasing trend of δatm(18O), at least when the δ() was decreasing by about −8000 per meg. We therefore decided that we would not correct the δatm(18O) values for the changes in the simultaneously measured δ(). It is noteworthy that we obtained a different result – an increase in δatm(18O) with a decrease in δ() – in our earlier flask studies in 2013. We have not yet clarified the cause(s), but we expect the results shown in Fig. 3b to be valid because we repeatedly obtained results that were consistent between flasks and cylinders.
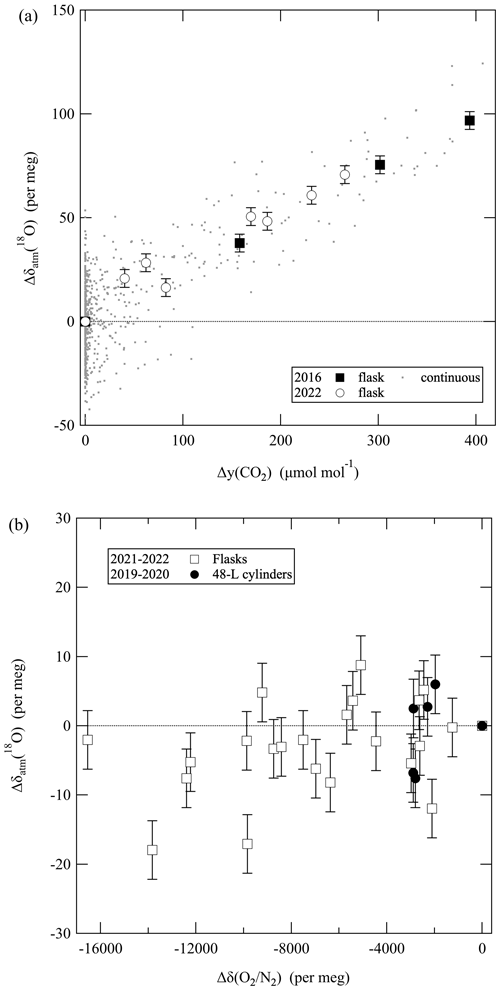
Figure 3(a) Changes (Δ) in the measured δatm(18O) of air samples as a function of the amount fraction of CO2. Δy(CO2) represents the difference between the amount fraction of CO2 of the air sample after and before adding pure CO2. y stands for the dry amount fraction of gas. (b) Changes in the measured δatm(18O) of the air sample as a function of its δ(). Δδ() represents the difference between the δ() of the air sample after and before adding pure N2. Error bars in (a) and (b) indicate uncertainties (±1σ) for the measurements of air samples in flasks.
2.2 Box model for simultaneous analysis of δatm(18O) and δ()
The box model used in this study is the same as that described by B94. However, the isotopic effects for the sink–source processes are updated by a more recent study of L&B11. Therefore, we performed calculations using the isotope effects from both studies and compared them, especially for the long-term variations of δatm(18O). Since there are a lot of symbols used in this study, we present a list of the symbols in the main text in Table A1 in Appendix A. Equation (3) is the mass balance equation for δatm(18O) (see Appendix B for derivation).
where rMR, rPR, and rDR are the relative ratios of the Mehler reaction, photorespiration, and dark respiration to the total O2 consumption associated with terrestrial respiration, and εMR, εPR, εDR, εLE, and εOR denote the isotope effects of the Mehler reaction, photorespiration, dark respiration, leaf water enrichment, and marine respiration, respectively. Values of O2 budgets and isotopic effects are summarized in Table 1. The isotopic enrichment of O2 produced by the terrestrial photosynthesis, ΔPS, is basically determined by the δLW(18O)(Gonfiantini et al., 1965; Dongmann et al., 1972; Farquhar et al., 1993), and we assumed ΔPS to be 4.4 ‰ or 6.5 ‰ for the steady state. There is still a large uncertainty in δLW(18O)(Farquhar et al., 1993; Bender et al., 1994; Hoffmann et al., 2004; Keeling 1995; West et al., 2008). In this study, we used the δLW(18O) calculated by a 3D model (see Sect. 3.2 and 3.3). We used values of the relative ratios for the respiration (rMR, rPR, and rDR) following B94. Specifically, respective values of rMR, rPR, and rDR are 0.1, 0.31, and 0.59 in B94. As a result, the total isotope effects of terrestrial respiration become 18.7 ‰ and 17.7 ‰ for B94 and L&B11, respectively. This difference is caused by the large difference of their dark respiration effects (εDR). The biggest difference between the two studies is the effect of the ocean, and the respective oceanic DMEs are 18.9 ‰ and 23.5 ‰ for B94 and L&B11. The oceanic DME for L&B11 is almost the same magnitude as the total terrestrial DMEs, which are 22.4 (4.4–0.70 + 18.7) ‰ and 23.5 (6.5–0.75 + 17.7) ‰ for B94 and L&B11, respectively. L&B11 also showed that photosynthetic enrichment in the ocean cannot be ignored, contrary to previous studies. However, they did not clearly separate the effects of oceanic photosynthesis and respiration. Therefore, we assumed ΔOW and εOR to be zero and 23.5 ‰, respectively, to set the total oceanic DME to be 23.5 ‰ as L&B11. Unlike the previous studies, the stratospheric effect was formulated as fractionations, εTS and εST, which denote the isotope effects of air exchange between the troposphere and stratosphere. This is because we have continued precise measurements of the isotopic ratios of O2 in the stratosphere, which could provide new insights into stratospheric processes, as described later. RRes, RPS, ROR, and ROP (the unit is a−1) represent the relative ratios of the annual fluxes of O2 from terrestrial respiration, terrestrial production, marine respiration, and marine production, respectively, to the total amount of O2 in the atmosphere ( Pmol). For example, if we assume that the terrestrial flux is 16.7 Pmol a−1, RPS will be 16.7/(3.706 × 104) = 4.5× 10−4 a−1, as shown in Table 1. RTS and RST denote the relative ratios of the annual fluxes of O2 between the troposphere and stratosphere, respectively. εFF and RFF denote the isotopic effects in fossil fuel combustion and the relative ratios of the annual O2 consumption by fossil fuel combustion, respectively. We assumed that atmospheric oxygen is consumed without isotope effects in fossil fuel combustion (εFF=0), taking into account that industrial combustion processes usually occur at high temperature. Therefore, we consider no contribution to DME from fossil fuel combustion in this study. In this regard, it is known that large oxygen isotope fractionation occurs in combustion processes such as biomass burning due to complex combustion processes (Schumacher et al., 2011). In such cases, it will be necessary to consider isotopic fractionation in the consumption of atmospheric oxygen associated with combustion. However, at present, little is known about the impact of this on DME. The box model also calculates the amount fraction of atmospheric O2, y(O2) by solving the following mass balance equation:
Here, y stands for the dry amount fraction of gas, as recommended by the IUPAC Green Book (Cohen et al., 2007). To compare with the observed results for δ(), the amount fraction of O2 calculated by the box model was converted to δ() assuming a normal atmosphere.
We assumed the value of terrestrial O2 production, PT, to be 16.7 Pmol a−1, which is the value reported by Hoffmann et al. (2004). The ratio of terrestrial and marine production was assumed to be 0.63:0.37 (Luz and Barkan, 2011). It is known that mass-independent isotopic fractionation of 17O and 18O between O3 and CO2 occurs in the stratosphere via photochemical processes (e.g., Gamo et al., 1989; Thiemens, 1999). B94 estimated the isotopic effect on atmospheric O2 by scaling the δ(18O) of CO2 and calculated that it would reduce δatm(18O) by 0.4 ‰, considering the turnover time between the troposphere and the stratosphere. L&B11 showed that the global Δ(17O) budget supports their result and have estimated the stratospheric isotope effect on δatm(18O) to be 0.3 ‰. In this study, the flux between the troposphere and stratosphere was set to 3000 Pmol a−1, which is calculated from the stratosphere–troposphere (S-T) mass flux (Olsen et al., 2004). This S-T O2 flux is approximately 100 times the flux from the biosphere (Luz et al., 1999). εST, which is the isotopic fractionation of O2 that returns from the stratosphere to the troposphere, is currently considered to be so small that it is impossible to actually detect it in the stratosphere. Note that εST represents the fractionation of stratosphere–troposphere exchange flux in this study, while εstrat in L&B11 represents the stratospheric effect on DME. As a rough estimate, considering that the value of Δ(17O) is −1.5 per meg with respect to the tropospheric value (Luz et al., 1999), εST is expected to be about −3 per meg based on the mass-independent effect (δ17O ≈δ18O). Here, εST was set to −2.5 per meg so that the diminution of δatm(18O) at equilibrium was −0.3 ‰. Because there are no isotopic effects during the transport of air from the troposphere to the stratosphere, εTS should be zero.
Based on the above discussion, the δ(18O) in the stratosphere should be −2.5 to −3 per meg lower than in the troposphere due to photochemical processes. As a matter of fact, the δ(18O) of stratospheric O2 has been observed with high precision by balloon experiments and is known to decrease significantly with increasing altitude because of gravitational separation (e.g., Ishidoya et al., 2013b; Sugawara et al., 2018). At an altitude of 35 km over Japan, δ(18O) is lower than the tropospheric value by approximately −100 per meg, which is anomalously lower (i.e., larger diminution) than that expected on the basis of photochemical diminution. The implication is that enrichment of approximately 5 per meg is permanently occurring in the troposphere because of gravitational separation in the stratosphere (Ishidoya et al., 2021). It is currently uncertain how gravitational separation affects the process by which isotopically light oxygen is transported to the troposphere through troposphere–stratosphere exchange. For example, changing εST from −2.5 to −5.0 per meg yields the δatm(18O) trend of approximately −0.2 per meg a−1 because the flux from the stratosphere is over 100 times greater than the surface biospheric flux. This uncertainty complicates the problem of interannual δatm(18O) change and suggests that gravitational separation may be involved in small fluctuations in the DME.
With these initial settings, we were able to reach a steady state after a 5000-year simulation, and we found that the equilibrium values of δatm(18O) were 20.82 ‰ and 23.16 ‰ for calculations following B94 and L&B11, respectively, which are almost same values reported by the two studies. Hereafter, the box model results are discussed based on the differences from these equilibrium values. The biospheric turnover time of O2 in the steady state was 1398 years, which is longer than the 1200 years estimated by B94. This may be a little too long, since the δatm(18O) variations reported by Severinghaus et al. (2009) based on ice core measurements showed a characteristic asymptotic decay curve after abrupt climate change events on a timescale of about ∼ 1000 years, implying that the turnover time of O2 in the atmosphere is about 1000 years. The biospheric turnover time is inversely proportional to the sum of the terrestrial and oceanic production of O2 incorporated into the box model, which is 26.5 (16.7 + 9.8) Pmol a−1 in this study (Table 1). This implies that total production of O2 for the initial value in our model is underestimated. In this regard, turnover time decreases to about 1000 years when we simulate a case in which the GPP is increased, as will be discussed later. In model calculations for the interpretation of long-term changes, we used the steady-state condition described above as the initial condition, and we performed some calculations by adding long-term changes to terrestrial GPP, photorespiration, and δLW(18O)(see details in Sect. 3.3).
The box model was suitable for simulations if we assumed that long-term and global changes occurred over time frames of hundreds to thousands of years. The box model naturally ignores atmospheric transport processes, and it is difficult to define the box atmosphere at local and regional spatial scales. There is hence a theoretical limit to the application of the box model to short-timescale phenomena. However, we tried to use this box model as a first step to understand the diurnal and seasonal changes in δatm(18O) recently observed by high-precision measurements. Because δ() was also observed at the same time during this study, the relationships between δatm(18O) and δ() provided information about the usefulness of the box model simulations. For the simulations of diurnal changes, the intensities of terrestrial O2 consumption and production were approximated by a simple function, which became a maximum at noon and zero during the night. We also carried out simulations of diurnal changes considering marine O2 consumption and production approximated by the similar simple function to examine sensitivities of the () ratio to the terrestrial and marine signals. Seasonal variations were also simulated by a simple sinusoidal function. We then tuned the magnitude of RRes (=RPS) so that the amplitude of the modeled δ() variation was close to the observed results. The box model did not incorporate the contributions of S-T O2 flux and fossil fuel combustion for the simulations of the diurnal and seasonal changes.
2.3 Numerical simulations of δLW(18O) using the 3D model MIROC5-iso
We simulated δLW(18O) using a stable-water-isotope-enabled general circulation model named MIROC5-iso (Okazaki and Yoshimura, 2017, 2019). MIROC5-iso is the fifth generation of the Model for Interdisciplinary Research on Climate (MIROC5; Watanabe et al., 2010). The stable water isotopes were implemented to the atmospheric and land surface components following Jouzel et al. (1987) and Yoshimura et al. (2006). MIROC5-iso calculates the isotopic ratio of atmospheric water vapor, precipitation, and reservoirs at ground level, including soil water and leaf water, with the equilibrium and kinetic fractionation at all phase transitions. The δLW(18O) is calculated by considering water conveyance driven by transpiration and diffusive isotopic movement (i.e., “back diffusion”) as follows:
Here, RLW is the isotopic ratio of the leaf water given by , where the subscripts “sample” and “standard” indicate the sample and the standard water, respectively, and the standard water is VSMOW. zL is the axis directed from the leaf base to tip, and VL and AL are the volume of leaf water and area of the leaf surface, respectively. ρ is the density of water, T is the transpiration flux, ILA is the leaf area index, D is the liquid diffusivity of an isotope, and τ is the crookedness of the leaf. The transpired water drawn up from the root zone layers is calculated by weighting the isotope ratio of soil water by root density. The transpiration fluxes of the water isotopes were calculated by the bulk method with the bulk exchange coefficient of Sellers et al. (1996), and the equilibrium and kinetic fractionations from liquid to gas at the stoma were considered.
In this study, MIROC5-iso was forced by observed sea surface temperature, sea ice concentration, observed greenhouse gases (carbon dioxide, methane, and chlorofluorocarbons), ozone, and changes in land use. The isotopic compositions of sea surface water and sea ice were kept constant and assumed to be 0 ‰ and 3 ‰ , respectively, as in Joussaume and Jouzel (1993). The model resolution was set to T42 (approximately 280 km at the Equator) with 40 vertical levels. After running MIROC5-iso for 100 years with the condition of 1871 CE for spin-up, we ran the model from 1871–2022 CE.
3.1 Diurnal variations of δatm(18O) and δ()
Figure 4a shows the average diurnal cycles of δatm(18O), δ(), the amount fraction of CO2, and δ() for each season observed at TKB during 2013–2022. δ() was defined in the same way as δ() but for the ratio. The error bands shown in Fig. 4a indicate year-to-year variations of the average diurnal cycles (± 1σ). In this study, we needed to remove any natural or artificial fractionation of 18O16O and 16O16O, other than the processes associated with the DME from the observed δatm(18O). For this purpose, we used the observed diurnal δ() cycle, which is potentially driven by the nighttime vertical temperature gradient (Adachi et al., 2006) and artificial inlet fractionation induced by radiative heating of an air intake (e.g., Blaine et al., 2006). The δ() underwent a slight diurnal cycle with a maximum in the early morning (Fig. 4a), and the difference between the maximum and minimum was about 4–6 per meg. It is difficult to specify the cause of the diurnal cycle, but it may result from natural variations due to a nighttime vertical temperature gradient at the inland TKB site because Adachi et al. (2006) reported much larger enrichment of δ() by 100 per meg at the center of a wide desert during the night. We therefore decided to correct the observed values for thermally diffusive fractionation following the method used by Ishidoya et al. (2014, 2022) and to use the corrected values for our discussion of diurnal variations. Specifically, we subtracted from the observed δatm(18O). The coefficient 1.5516.2 is the () ratio determined by laboratory experiments (Ishidoya et al., 2013b). In a similar manner, we also corrected the δ() for thermally diffusive fractionation by subtracting from the measured δ(). The coefficient 4.57/16.2 is the () ratio from the same laboratory experiments. The maximum corrections were 0.3 and 1.0 per meg for δatm(18O) and δ(), respectively. The correction for the amount fraction of CO2 was negligibly small.
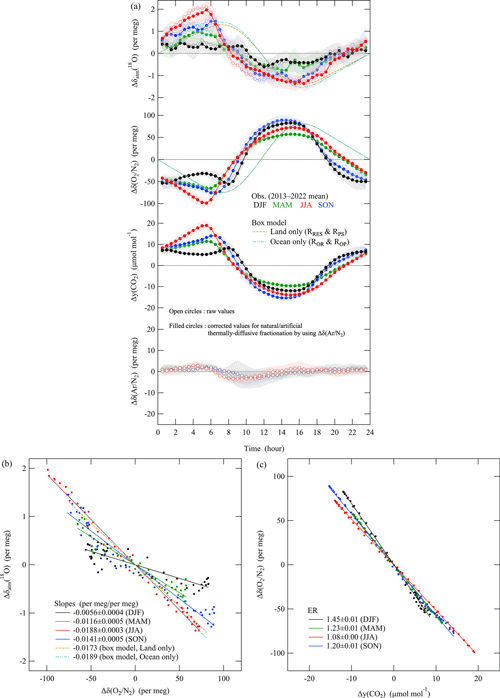
Figure 4(a) Plots of the average diurnal cycles of Δδatm(18O), Δδ(), Δy(CO2), and Δδ(Ar/N2) (open circles) observed at the TKB site during 2013–2022 for each season: December to February (black), March to May (green), June to August (red), and September to November (blue). Error bands indicate year-to-year variations during the observation periods (±1σ). Those of Δδatm(18O), Δδ(), and Δy(CO2), corrected for thermally diffusive fractionation, are also plotted (filled circles) (see text). The range of the vertical axis for Δδ() was adjusted arbitrarily to facilitate visual assessment of the variations of the observed Δδatm(18O) due to thermally diffusive fractionation. Average diurnal cycles of Δδatm(18O) and Δδ() that were simulated with a box model are also shown. The simulated values that considered only terrestrial and marine respiration and production are shown by the dashed ocher line and the dashed light blue two-dotted line, respectively (see text). Δ denotes deviations from the diurnal mean values. (b) Relationships between Δδatm(18O) and Δδ() for the data corrected for thermally diffusive fractionation in (a). Regression lines fitted to the observed and simulated data are also shown. (c) Same as in (b) but for the relationship between Δδ() and Δy(CO2).
δatm(18O) exhibited a clear diurnal cycle with a daytime minimum, especially in summer (Fig. 4a). δatm(18O) varied out of phase with δ(), and the ratio of the amplitude of the diurnal δatm(18O) cycles to those of δ() was substantially larger in summer than in winter. Figure 4a also shows the diurnal cycles of δatm(18O) and δ() simulated by the box model described in Sect. 2.2 that incorporated the isotopic effects from B94. The simulations were carried out under two conditions: one was the case when we ignored marine respiration and the production of O2 (ROR and ROP), and the other was the case when we ignored terrestrial respiration and the production of O2 (RRes and RPS). In both cases, the RRes and RPS (or ROR and ROP) in the model were adjusted to reproduce the observed seasonal δ () cycle subject to the constraint that the daily average RRes=RPS (or ROR=ROP). The initial value of the δatm(18O) relative to ocean water was then adjusted arbitrarily to establish a steady state for the simulated δatm(18O). The δ(18O) values relative to ocean water in the steady state were 22.0 ‰ and 18.9 ‰ for the case when we considered only terrestrial or only marine respiration and production, respectively. We found that the general characteristics of the diurnal cycles of δatm(18O) and δ() were reproduced by the simulated δatm(18O) for both cases when we considered only terrestrial or only marine processes (Fig. 4a).
To determine the cause(s) of the observed diurnal δatm(18O) cycles, we examined the relationships between the observed δatm(18O) and δ() (Fig. 4b) and those for δ() and the amount fraction of CO2 (Fig. 4c). The () ratios were −0.019 and −0.006 per meg (per meg)−1 in summer and winter, respectively. In Fig. 4b, we also plot the relationship between the simulated δatm(18O) and δ(). We found the simulated ratios to be –0.017 and −0.019 per meg (per meg)−1 when we considered only terrestrial or only marine respiration and production, respectively. These ratios were much closer to the ratio observed in summer than in winter. The O2 and CO2 exchange ratios (ERs, −Δy(O2)Δy(CO2)−1) calculated from the δ() and the amount fraction of CO2 shown in Fig. 4c were 1.08 and 1.45 in summer and winter, respectively. An oxidative ratio (OR, −Δy(O2)Δy(CO2)−1) of 1.05–1.1 is expected for terrestrial biosphere activities, and ratios of 1.17, 1.44, and 1.95 are expected for combustion of solid fuel, liquid fuel, and natural gas, respectively (Keeling, 1988; Severinghaus, 1995). The ER refers to the exchange between the atmosphere and organisms or ecosystems, whereas the OR reflects the stoichiometry of specific materials, in accord with Faassen et al. (2023) and Ishidoya et al. (2024). The ORs therefore suggested that the diurnal δ() cycle observed in summer could be attributed mainly to terrestrial biosphere activities, whereas that in winter was due to fossil fuel combustion. The observed wintertime ER of 1.45 was also consistent with the average OR of 1.52 ± 0.1 for fossil fuel consumption (hereafter referred to as ORFF) for the Kanto area, which includes TKB, of about 1.7×104 km2, calculated using the data on fossil fuel consumption reported by the Agency of Natural Resources and Energy (https://www.enecho.meti.go.jp/statistics/energy_consumption/ec002/results.html#headline2, last access: 28 March 2024, in Japanese) (Ishidoya et al., 2020). The implication is therefore that the isotopic discrimination of O2 during activities of the terrestrial biosphere was the main cause of the observed summertime diurnal δatm(18O) and δ() cycles, and the isotopic discrimination of O2 during fossil fuel combustion was very small or negligible.
The simulated diurnal cycle of δatm(18O) and the () ratio for the case when only terrestrial processes were considered were very similar to those for the case when only marine processes were considered. This similarity was due to the small difference between the isotopic discriminations of the terrestrial and marine processes (22.4–18.9=3.5 ‰). If we use the isotopic discriminations from L&B11, then the corresponding difference is much smaller (23.5–23.5=0 ‰). We could therefore estimate the variations of the observed δ() driven by the total activities of the terrestrial and marine biosphere (hereafter referred to as δBIO()) by dividing the observed variations in δatm(18O) by the ratio of the simulated δatm(18O) / δ() of about −0.017 to −0.019 per meg (per meg)−1. We could then estimate the variations of δ() driven by fossil fuel combustion (hereafter referred to as δFF()) by subtracting the δBIO() from the observed δ(). This method, hereafter referred to as the δatm(18O) method, enabled us to remove the impact on δ() of not only the activities of the terrestrial biosphere but also the contributions due to the air–sea O2 flux, which is driven mainly by activities in the marine biosphere (e.g., Nevison et al., 2012; Eddebbar et al., 2017), from the estimated δFF(). For an application of the δatm(18O) method, we assume there is no isotopic discriminations during fossil fuel combustion considering the seasonal differences in the () ratios in Fig. 4b. It would be generally reasonable since the combustion occurs at high temperature, which minimizes isotopic discriminations. However, Schumacher et al. (2011) reported isotopic discriminations on the order of up to 26 ‰ for the stable oxygen isotopic ratio of atmospheric CO2() derived from combustion of different kinds of materials. They suggested that natural combustion processes in the long term might enrich δatm(18O) and contribute to the DME. Therefore, isotopic discriminations of δatm(18O) due to combustion processes should be examined carefully in the future based on precise observations of δatm(18O).
Figure 5 shows the δBIO() and δFF() estimated by the δatm(18O) method for each season. The largest amplitudes of the diurnal δBIO() and δFF() cycles were in summer and winter, respectively. For comparison, we separated the contributions of terrestrial biosphere activities and fossil fuel combustion to the observed δ() based on the observed ER and amount fraction of CO2 (hereafter referred to as the “ER method”). For this purpose, (1) we assumed that the diurnal cycle of the amount fraction of CO2 was driven by terrestrial biosphere activities and fossil fuel combustion, (2) we ignored the contribution of the air–sea O2 flux to δ(), and (3) we assumed the OR for activities in the terrestrial biosphere (ORB) to be 1.1 (Severinghaus, 1995), which has been widely used in past studies (e.g., Manning and Keeling, 2006; Tohjima et al., 2019), and the ORFF to be 1.4, 1.5, 1.6, or 1.7 considering mixed combustion of solid fuel, liquid fuel, and natural gas. It is noted that some recent studies have used the ORB of 1.05 rather than 1.1 (e.g., Morgan et al., 2021).
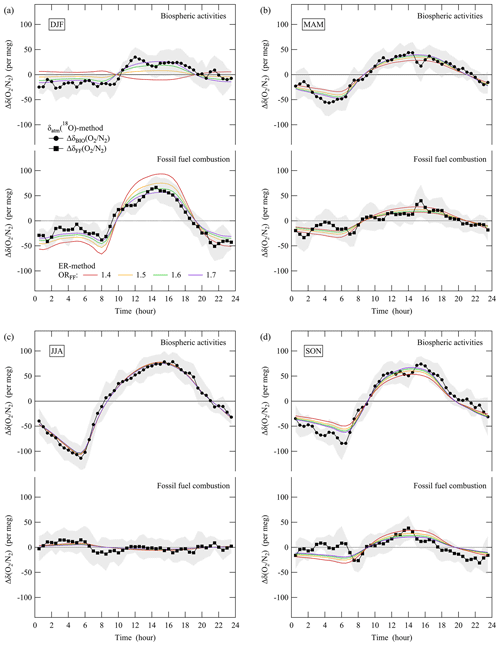
Figure 5(a) Plots of the average diurnal cycles of ΔδBIO() and ΔδFF() for each season estimated by the δatm(18O) method. Δδ() values driven by activities in the terrestrial biosphere and fossil fuel combustion estimated by the ER method are also shown. See the text for details of the δatm(18O) and ER methods. Δ denotes deviations from the diurnal mean values. Error bands for ΔδBIO() are derived from Δδatm(18O) in Fig. 4a. Error bands for ΔδFF() are assumed to be the same as those for ΔδBIO().
The equations for the ER method can be written as
Here, Δy(CO2, B) and Δy(CO2, FF) are changes in the amount fraction of CO2 driven by terrestrial biosphere activities and fossil fuel combustion, respectively. Δy(CO2) is the observed average diurnal cycle of the amount fraction of CO2 for each season shown in Fig. 4a. αB, αF, and αobs are the ORB, ORFF, and observed ER for each season shown in Fig. 4c. Once Δy(CO2, B) and Δy(CO2, FF) are calculated by solving Eqs. (5) and (6), they can be converted to Δδ() by using ORB and ORFF, respectively.
Figure 5 shows the δ() driven by terrestrial biosphere activities and fossil fuel combustion estimated by the ER method. The results agreed well with the δBIO() and δFF() for all seasons, especially when we chose the ORFF to be 1.6 or 1.7, which are higher and lower than those expected from liquid fuel and natural gas fuel combustion, respectively. The implication is therefore that the diurnal δFF() cycles at TKB were driven by car traffic (liquid fuels) and household gas consumption. It is noteworthy that propane (CH3CH2CH3), for which the ORFF is 1.67 assuming complete combustion, should also be considered a household gas consumed in the TKB area.
To determine whether variations of δatm(18O) on hourly to daily time frames were observable, we plotted typical examples in Fig. 6 of rolling averages calculated over 300 cycles (5 h) and 1100 cycles (19 h) of mass spectrometric measurements of δatm(18O) and δ(). The summertime graphs (Fig. 6a) clearly show that the δatm(18O) varied in antiphase with δ() on timescales of both 5 and 19 h. The ratios of () were −0.017 per meg (per meg)−1 for data averaged over both 5 and 19 h. This result agreed with that obtained from the summertime average diurnal cycle (vide supra). In contrast, there was no clear correlation between variations of δatm(18O) and δ() in winter (Fig. 6b). We could distinguish some short-term δatm(18O) variations (Fig. 6b), but the causes were unclear. The variations may be partly due to activities in the biosphere because the δatm(18O) in winter showed small but substantial diurnal cycles of δatm(18O) and δBIO() (Figs. 4a and 5a). These characteristics suggest that we could apply the δatm(18O) method to resolve temporal variations of δBIO() and δFF() separately on time frames of several hours to day to day. Similar separation has been carried out for CO2 based on the simultaneous analysis of the Δ(14C) and amount fraction of CO2(e.g., Graven et al., 2018; Basu et al., 2016, 2020) or based on the simultaneous analysis of δ() and the amount fraction of CO2 by assuming an average ORFF based on a statistical assessment (e.g., Minejima et al., 2012; Sugawara et al., 2021; Pickers et al., 2022). The δatm(18O) method may have some advantages compared with methods used in previous studies because we could apply it without assuming any ORFF with a temporal resolution of 5 h or perhaps even shorter.
3.2 Seasonal cycles of δatm(18O) and δ()
Figure 7a shows the monthly mean values of δatm(18O) and δ() at TKB during 2013–2022. To reduce local effects of fossil fuel combustion around TKB, we extracted the successive maxima of δ() for 4320 cycles (3 d) of mass spectrometric measurements to calculate the monthly mean values of δ() plotted in Fig. 7a. In contrast, all data were used to calculate the monthly mean values of δatm(18O) to reduce their standard errors because fossil fuel combustion did not change δatm(18O) significantly, as discussed in Sect. 3.1. We removed anomalous δatm(18O) data from the plot during 4 months when the mass spectrometer was producing unreliable results. Therefore, Fig. 7a shows 116 and 120 data points for δatm(18O) and δ(), respectively. Some seasonal and interannual variations are apparent in Fig. 7a, not only for δ(), which has been reported in many past studies (Keeling and Manning, 2014), but also for δatm(18O). We examined the observed average seasonal cycle and secular trend of δatm(18O), and in the following paragraphs we discuss the implications thereof for the oxygen, carbon, and water cycles.
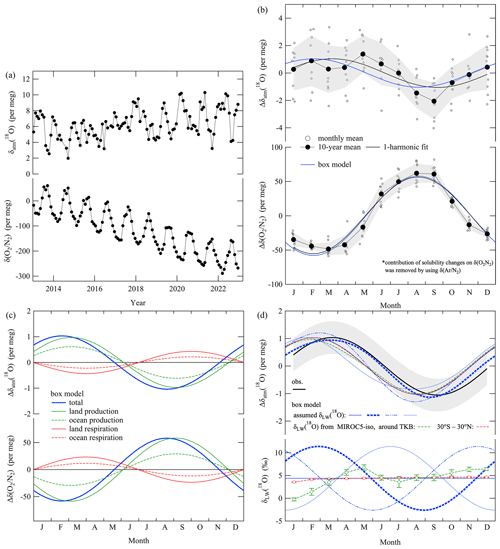
Figure 7(a) Monthly mean values of δatm(18O) and δ() observed at TKB for the period 2013–2022. Local effects of fossil fuel combustion around TKB were excluded from δ(). See the text for details. (b) Detrended monthly mean values (open black circles) and their 10-year average (filled black circles) of Δδatm(18O) at TKB for the period 2013–2022. Those of Δδ(), extracted by removing the contributions of solubility change by using the average seasonal δ() cycle at TKB (see text), are also shown. Average seasonal cycles of Δδatm(18O) and Δδ() obtained by applying one-harmonic best-fit curves to the data (solid black lines) and those simulated by the box model (solid blue lines) are also shown. We assumed a constant δLW(18O) of 4.4 ‰ for the simulation. Δ denotes deviations from the annual mean values. (c) Same average seasonal cycles of Δδatm(18O) and Δδ() simulated by the box model in (b) (solid blue lines), the contributions of terrestrial and ocean production (solid and dashed green lines, respectively), and terrestrial and ocean respiration (solid and dashed red lines, respectively). (d) The same best-fit curve for δatm(18O) as in (b). Error bands indicate average deviations from the 10-year average of Δδatm(18O) (±1σ). Same average seasonal cycle of Δδatm(18O) simulated by the box model as in (b) and corresponding δLW(18O) values (solid blue lines). Additional simulations of average seasonal Δδatm(18O) cycles and the incorporated seasonally varying δLW(18O) values for sensitivity tests (thick dashed blue line, dashed blue two-dot line, and dotted line), as well as those incorporating the average monthly δLW(18O) around TKB (36° N, 140° E) (green open circles) and at lower latitude (30° S–30° N) (red open circles) during 2013–2022 calculated by MIROC5-iso, are also shown (dashed green and red lines, respectively).
Figure 7b shows the average seasonal cycles of δatm(18O) and δ() at TKB during 2013–2022. The δ() values in this figure are the values after contributions from the solubility changes in the ocean were removed. For this purpose, we used the seasonal δ() cycle, which is driven mainly by the air–sea heat flux at the surface (e.g., Keeling et al., 2004; Ishidoya et al., 2021; Morgan et al., 2021). Specifically, the average seasonal cycle of δ() at TKB (Ishidoya et al., 2021), multiplied by a coefficient of 0.9 derived from differences in the solubilities of O2 and Ar (Weiss, 1970), was subtracted from the average seasonal cycle of δ(). We applied this correction so that we could discuss the variations of δ() associated with only the DME. The peak-to-peak amplitude of the corrected seasonal δ() cycle was smaller than that of the uncorrected seasonal δ() cycle by about 7 per meg. It is apparent in Fig. 7b that the δatm(18O) varied seasonally, roughly in antiphase with the seasonal cycle of δ(). The minimum of the seasonal δatm(18O) cycle appeared in late summer to early autumn, and the peak-to-peak amplitude was 2.1±0.6 per meg. The maximum of the seasonal δ() cycle occurred in summer, and its peak-to-peak amplitude was 112±10 per meg. The uncertainties for the amplitudes of δatm(18O)(δ()) were evaluated as a standard deviation of the 10-year average monthly mean values from the best-fit curve shown in Fig. 7b. Keeling (1995) expected δatm(18O) to be lower in summer than in winter by 2 per meg based on the assumption that the 100 per meg seasonal increase in δ() was driven by the input of photosynthetic O2, the δ(18O) of which is about 20 ‰ lower than δatm(18O). This can be calculated as
Here, δatm_summer(18O) and δatm_winter(18O) are δatm(18O) in summer and winter, respectively, y(O2)winter is the atmospheric O2 amount fraction in winter, and Δy(O2) is an input of photosynthetic O2 to the atmosphere. If we assume δatm_winter(18O) and δLW(18O) are 0 ‰ and −20 ‰, respectively, y(O2)winter is 209 400 µmol mol−1, and Δy(O2) is 21 µmol mol−1, which corresponds to a 100 per meg seasonal increase in δ(), then we obtain δatm_summer(18O) of −2 per meg as in Keeling (1995). Although his estimation was relatively simple, it reproduced the general characteristics of the seasonal δatm(18O) and δ() cycles observed in the present study well. In Fig. 7b, we also plot the seasonal cycles of δatm(18O) and δ() simulated by our box model that incorporated the isotopic effects from B94. The RRes, RPS, ROR, and ROP values in the model were adjusted to reproduce the observed seasonal δ() cycle by imposing the constraints that the annual average and . We then adjusted the initial value of the δatm(18O) in the model arbitrarily to establish a steady state for the simulated δatm(18O). We set the (or ) ratio to be 2 for the simulation in Fig. 7b. As discussed in Sect. 3.1, changes in that ratio do not substantially change the simulated results of δatm(18O). Figure 7c shows the same simulated seasonal cycles of δatm(18O) and δ() in Fig. 7b and the respective contributions of terrestrial production, terrestrial respiration, ocean production, and ocean respiration. As seen from Fig. 7c, the seasonal δatm(18O) cycle is driven mainly by production rather than respiration, which is consistent with the estimation by Keeling (1995). In this context, seasonal cycles of have been reported by some past studies (e.g., Peylin et al., 1999; Cuntz et al., 2003; Murayama et al., 2010). Peylin et al. (1999) and Cuntz et al. (2003) used 3D atmospheric transport models to reproduce the observations, and they found that the main contributors are respiration and production for the respective seasonal cycles of and CO2 amount fraction. These characteristics are different from the seasonal cycles of δatm(18O) and δ() observed in this study, both of which are driven mainly by production (Fig. 7c).
We found that the box model could reproduce the observed seasonal δatm(18O) cycles, although both the seasonal minimum and maximum of the simulated seasonal δatm(18O) cycle appeared slightly earlier (by about 1 month) than in the observed cycle represented by a one-harmonic, best-fit curve (Fig. 7b). A 1- to 2-month time shift between the observed and simulated seasonal cycles is also found in at various surface stations (Peylin et al., 1999; Cuntz et al., 2003), although the box model used in this study is much more primitive compared to the 3D models in past studies. To investigate the possible cause(s) of the phase difference, we carried out additional simulations that incorporated three different seasonally varying δLW(18O) values into the box model.
Figure 7d shows the simulated results along with the one-harmonic, best-fit curve to the observed data. It is apparent from this figure that the appearance of the seasonal minimum and maximum of δatm(18O) depended on the seasonal variations of δLW(18O). It is also apparent that the observed seasonal cycle of δatm(18O) was well reproduced by the simulation that incorporated the δLW(18O) represented by the thick dashed blue line. The scenario for the thick dashed blue line was determined based on some past studies that reported seasonal variations of δLW(18O) (e.g., Welp et al., 2008; Plavcová et al., 2018; Cernusak et al., 2022; Liu et al., 2023), and two other scenarios represented by a two-dot line and dotted lines were carried out as sensitivity tests to the phase difference in the seasonal δLW(18O) cycle. Welp et al. (2008) observed the time series of ecosystem water pools at a soybean canopy in Minnesota, USA, from 30 May to 27 September 2006 and found the most extreme enrichment of bulk δLW(18O) to be 20 ‰ above xylem water during the early part of the growing season (Fig. 1a in Welp et al., 2008). Plavcová et al. (2018) and Liu et al. (2023) also reported less enrichment of δLW(18O) toward the end of the vegetation season by about 10 ‰–20 ‰, and Cernusak et al. (2022) reported a strong negative correlation between the δLW(18O) and the relative humidity of air based on a recent global meta-analysis. These characteristics are roughly consistent with the δLW(18O) represented by the thick dashed blue line in Fig. 7d, which shows decreases in δLW(18O) toward the end of the vegetation season similar in magnitude to decreases reported in past studies. In this regard, Murayama et al. (2010) observed at a forest site in Japan and reported that monthly mean correlated positively with δ(18O) of precipitation (δprecip(18O)). Since variations in δLW(18O) are closely related to those in δprecip(18O), it is suggested that δLW(18O) is an important driver in modifying seasonal cycles for both δatm(18O) and .
We also carried out additional box model simulations that incorporated the average monthly δLW(18O) around TKB (36° N, 140° E) and at lower latitude (30° S–30° N) calculated by MIROC5-iso for the period 2013–2022. The results are plotted in Fig. 7d, and both the monthly δLW(18O) and simulated seasonal δatm(18O) cycles fall within the range of those represented by the blue two-dot lines and dotted lines in the figure discussed above. Another factor in changing the simulated seasonal δatm(18O) cycle is the choice of the isotopic effects from B94 or L&B11 (Table 1). If we use the isotopic effects from L&B11 and ignore RRes and RPS(i.e., we consider marine respiration and production only), then the seasonal amplitude of the simulated δatm(18O) increases by 20 % compared with that simulated by using the isotopic effects from B94. This is due to the difference in the isotopic effects of ocean respiration, which are 18.9 ‰ and 23.5 ‰ for B94 and L&B11, respectively. Such a difference will become apparent in the Southern Hemisphere, where the seasonal δ() cycle is driven mainly by the air–sea O2 flux (e.g., Keeling and Manning, 2014). Therefore, spatiotemporal variations in the seasonal δatm(18O) cycle will be useful to constrain not only spatiotemporal variations of δLW(18O) but also the isotopic effects of ocean respiration.
3.3 Secular trend in δatm(18O)
Figure 8 shows temporal changes in the annual average δatm(18O) observed at TKB. The error band denotes the ±0.9 per meg of the long-term stability of δatm(18O) in our standard air (Fig. 2). It is apparent in Fig. 8a that the δatm(18O) underwent a slight secular increase of (0.22±0.14) per meg a−1 throughout the observation period. This rate was calculated from the difference between the 2013 and 2022 annual average δatm(18O), and the uncertainty around the long-term stability was taken into account. The observed secular increasing trend was quite different from the secular decrease in the DME expected by Seibt et al. (2005), which was on the order of 70 per meg over the last 150 years (−0.5 per meg a−1). They calculated the secular change by assuming anthropogenic changes in the terrestrial oxygen cycle from pre- to post-industrial times: (1) a replacement of 3 % of terrestrial respiratory O2 release by biomass burning, (2) a 5 % decrease in global terrestrial GPP, (3) a decrease in global photorespiration due to the increase in the amount fraction of atmospheric CO2 by 100 µmol mol−1, (4) a 10 % decrease in stomatal conductance resulting from CO2 increases and a partial offset of photorespiratory decreases, and (5) a 5 % decrease in the O2-flux-weighted 18O enrichment of foliage water due to higher contributions of 18O-depleted northern midlatitude biomes. The fact that the observation period of 10 years in the present study was much shorter than the 150 years discussed in Seibt et al. (2005) makes it difficult to discuss the significance of the difference between the secular trends in the two studies. It would nevertheless be of interest to see if the observed secular trend could be reproduced using our box model. In that case we could explore the applicability of the precise observations of the δatm(18O).
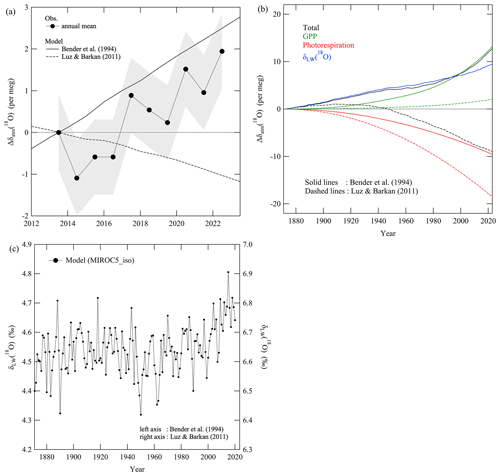
Figure 8(a) Annual average values of Δδatm(18O) observed at TKB (filled circles). Δδatm(18O) simulated by the box model for the period 2012–2022, assuming the isotopic effects reported by Bender et al. (1994) (solid line) and Luz and Barkan (2011) (dashed line). Δ denotes deviations from the observed value in 2013. See the text for details. (b) Same Δδatm(18O) simulated by the box model but for data during 1871–2022 and the respective contributions of the changes in GPP (green line), photorespiration (red line), and δLW(18O) (blue line) to the simulated δatm(18O). Solid and dashed lines denote the simulated data assuming the isotopic effects reported by Bender et al. (1994) and Luz and Barkan (2011), respectively. The solid and dashed blue lines almost overlap. Δ denotes deviations from the simulated value in 1871. (c) Annual average values of the global average δLW(18O) calculated by MIROC5-iso. The values in 1871 were arbitrarily adjusted to 4.4 (left axis) or 6.5 ‰ (right axis), respectively, for the DME at steady state by Bender et al. (1994) or Luz and Barkan (2011).
To explore that possibility, we carried out calculations in which we assumed that there were long-term changes in (1) GPP, (2) photorespiration, and (3) δLW(18O). Note that we considered long-term changes in only terrestrial fluxes for the GPP and photorespiration. Changes in marine photosynthetic and respiratory O2 fluxes should be included in more detailed future studies.
We first assumed that the global terrestrial GPP increases in proportion to the global average CO2 amount fraction. As the global average CO2 amount fraction, we used data from the Scripps CO2 Program (https://scrippsco2.ucsd.edu/data/atmospheric_co2/icecore_ merged_products.html, last access: 21 August 2024) based on ice core data and direct observations before and after 1959, respectively (Keeling et al., 2001; Rubino et al., 2019). We assume the initial terrestrial production of O2 in 1871 to be 16.7 Pmol a−1(Table 1), which corresponds to 107 Pg a−1(C equivalents) of global terrestrial GPP considering the rDR of 0.59 and the ORB of 1.1. Then, the GPP increased secularly with increasing CO2 amount fraction, and it was 141 Pg a−1(C equivalents) in 2006. Although this is somewhat larger than the average GPP of 125 Pg a−1(C equivalents) for the period 1992–2020 reported by Bi et al. (2022), it falls within a range of the global GPP estimates from various models summarized in Fig. 10 of Zheng et al. (2020). A similar increase in global GPP during the 20th century has also been reported by Campbell et al. (2017) based on long-term atmospheric carbonyl sulfide (COS) records derived from ice core, firn, and ambient air samples.
Second, we assumed an increase of 120 µmol mol−1 in the amount fraction of atmospheric CO2 during the 150 years from pre-industrial times to the present. This increase caused a decrease in global average photorespiration based on Farquhar et al. (1980):
where ϕ is the ratio of photorespiration to carboxylation (or total carbon fixation, the amount of which corresponds to the sum of GPP, photorespiration, and the Mehler reaction), and () is the ratio of the maximum oxygenation velocity to the maximum carboxylation velocity of RuP2 carboxylase–oxygenase (we used 0.21 for this ratio from Eq. 16 in Farquhar et al., 1980). y(O2) and y(CO2) are amount factions of O2 and CO2, respectively, in equilibrium with their dissolved amount fractions in the chloroplast stroma. We used atmospheric y(O2) and atmospheric y(CO2) multiplied by 0.7 following B94. () is the ratio of the Michaelis–Menten constants for carboxylation and oxygenation, respectively (we adopted 460 330 for this ratio from Table 1 in Farquhar et al., 1980). 10−3 is a coefficient to compare the calculated ϕ values with those in Farquhar et al. (1980) directly since they used units of millibars (mbar) and microbars (µbar) for y(O2) and y(CO2), respectively. We calculated ϕ to be 0.31 and 0.22 for CO2 amount fractions of 280 and 400 µmol mol−1, respectively.
We then calculated changes in δLW(18O) with MIROC5-iso for the period 1871–2022. We considered the water cycle to be in steady state before 1871, and we assumed the global average δLW(18O) in 1871 to be 4.4 ‰ or 6.5 ‰ based on previous studies for the DME in steady state by B94 or L&B11, respectively. In this connection, Hoffmann et al. (2004) reported an intermediate δLW(18O) of 5 ‰–6 ‰. It should be noted that the original δLW(18O) calculated by MIROC5-iso for 1871 was about −0.7 ‰, so we arbitrarily shifted all δLW(18O) values calculated by MIROC5-iso by 5.1 ‰ or 7.2 ‰. Clarifying the cause(s) of the low δLW(18O) calculated by MIROC5-iso will be a future task. Figure 8c shows the global average δLW(18O); δLW(18O) underwent a significant secular increase throughout the period. The increase was especially clear after the 1980s, which is the time when there was an increase in the δprecip(18O) simulated by MIROC5-iso (not shown). Rozanski et al. (1992) reported that δprecip(18O) increases with increasing surface air temperature by 0.6 ‰ K−1, and the global average surface air temperature has increased by about 1 K from 1980 to the present. The increase in surface air temperature has therefore caused at least part of the simulated secular increase in δLW(18O) since 1980. Previous studies have also reported that a lower (larger) relative humidity near the plant stomata enhances (diminishes) δLW(18O) (Eq. 2 of Hoffmann et al., 2004). Also, Byrne and O'Gorman (2018) reported that the relative humidity over land from 40° S to 40° N has decreased secularly since 1980. It is therefore possible that the decrease in relative humidity also contributed to the simulated secular increase in δLW(18O) since 1980. It should be noted that Welp et al. (2011) suggested that increases with increasing δprecip(18O) and δLW(18O) through the redistribution of moisture and rainfall in the tropics during an El Niño, which leads to substantial interannual variations in during 1977–2009 obtained from the Scripps Institution of Oceanography global flask network. Therefore, it will be important in future studies to examine not only the secular trend discussed in this study but also interannual variations in δLW(18O) and δatm(18O).
Figure 8a and b show δatm(18O) simulated by the box model that incorporated the abovementioned long-term changes in GPP, photorespiration, and δLW(18O). The observed and simulated secular trends in δatm(18O) agreed well with each other under the conditions assuming the isotopic effects from B94. On the other hand, the simulated δatm(18O) assuming the isotopic effects from L&B11 decreased secularly, contrary to the observed secular increase. Figure 8b shows the respective contributions of the changes in GPP, photorespiration, and δLW(18O) to the simulated δatm(18O). The simulated δatm(18O) showed secular increases with increasing GPP during 1871–2023, and the increase is much larger in the simulation assuming the isotopic effects from B94 than that from L&B11. The simulated δatm(18O) showed secular increases with increasing δLW(18O) for both the cases assuming isotopic effects from B94 and L&B11. This pattern differed from the results simulated by Seibt et al. (2005), who reported a secular decrease in δatm(18O) based on assumed secular decreases in GPP and δLW(18O) during the last 150 years. It is noted that the contributions of the changes in δLW(18O) to the simulated δatm(18O) increased with time monotonously, while a clear increase in δLW(18O) was found after the 1980s (Fig. 8b–c). This is due to the choice of the initial δLW(18O) in 1871; we set it to be 4.4 ‰ or 6.5 ‰ (the values for steady state by B94 or L&B11). As seen from Fig. 8c, the average δLW(18O) during 1872–1980 was higher than the initial values, which made the monotonous increase in δatm(18O) driven by the δLW(18O) changes. In contrast, both the present study and that of Seibt et al. (2005) found a secular decrease in the simulated δatm(18O) with a decreasing ratio of photorespiration to carboxylation (ϕ).
The contributions of ϕ and δLW(18O) almost canceled each other in the simulation assuming the isotopic effects from B94. As a result, the simulated δatm(18O) based on B94 increased secularly due mainly to the contribution of the secular increase in GPP. On the other hand, the contribution of GPP to the simulated δatm(18O) assuming the isotopic effects from L&B11 is much smaller. Moreover, the secular decrease in the simulated δatm(18O) due to the contribution of ϕ is larger for the simulation assuming the isotopic effects from L&B11 than that from B94. As a result, the simulated δatm(18O) based on L&B11 decreased secularly due mainly to the contribution of the secular decrease in ϕ. The substantial differences between the contributions of GPP for the simulations based on B94 and L&B11 are attributed to the differences in the terrestrial and oceanic DME in their studies. Specifically, the respective terrestrial and oceanic DMEs in steady state are 22.4 ‰ and 18.9 ‰ in B94, while they are 23.5 ‰ and 23.5 ‰ in L&B11 (Table 1). Therefore, the secular increase in terrestrial production incorporated into our simulations led to the secular increase in δatm(18O) for the isotopic effects based on B94. The substantial difference in the contributions of ϕ, found between the simulations based on B94 and L&B11, is attributed to the increase in rDR accompanied by a decrease in ϕ and the larger isotopic effect for dark respiration in B94 (18 ‰) than that in L&B11 (15.8 ‰). Therefore, we confirmed that secular trends in the simulated δatm(18O) are highly sensitive to the isotopic effects associated with the DME. This means that further studies are needed to determine the isotopic effects precisely in order to evaluate long-term changes in GPP and photorespiration based on δatm(18O). In this regard, some past studies evaluated the mechanisms responsible for the increase in global GPP. For example, Madani et al. (2020) reported an increase in GPP in northern latitudes caused by a reduction of cold-temperature constraints on plant growth. This scenario suggests that there has been an increase in negative carbon–climate feedback in high latitudes, whereas there has been a suggestion of an emerging positive climate feedback in the tropics, mainly due to an increase in the atmospheric vapor pressure deficit. They also pointed out that models have been struggling to determine how much additional CO2 is being taken up by plants as a result of increased amount fractions of atmospheric CO2. Therefore, an analysis based on a secular change in δatm(18O), which enables estimation of changes in the ratios of carboxylation to global GPP and photorespiration to global GPP, will facilitate better understanding of global CO2 fertilization processes.
We used the global average secular change in δLW(18O) simulated by MIROC5-iso in this analysis, and we found that it made a substantial contribution to the simulated δatm(18O). The implication is that the secular change in the water cycle must be accurate before the observed and simulated secular trends of δatm(18O) can be equated. In other words, δatm(18O) is a unique tracer for a comprehensive evaluation of global changes in the oxygen, carbon, and water cycles. For example, if the secular increase in the global average amount fraction of atmospheric CO2 stops without changes in the secular increasing trends of GPP, then the global average δatm(18O) will increase faster than the rate shown in Fig. 8a by assuming the isotopic effects from B94. A substantial secular decrease in the global average δatm(18O) may also be expected under pessimistic scenarios, such as substantial deforestation (secular decrease in GPP) and an increase in the average global amount fraction of atmospheric CO2. In both cases, the results are regulated by climate changes such as changes in surface air temperature and aridification that lead to secular changes in δLW(18O).
We recognize that secular changes in stratospheric gravitational separation may cause slight secular changes in the surface δatm(18O). Ishidoya et al. (2021) estimated this effect for atmospheric δ() at the surface to be 0.15 and −0.13 per meg a−1 when accompanied by a weakening or enhancement of the Brewer–Dobson circulation, respectively. These values correspond to 0.03 and −0.02 per meg a−1, respectively, for δatm(18O) if mass-dependent gravitational separation is assumed. The secular trend of δatm(18O) due to changes in stratospheric gravitational separation is negligible at present because the changes are much smaller than the uncertainty of the secular trend of the observed δatm(18O) at TKB ((0.22 ± 0.14) per meg a−1). If the observation period increases, the uncertainty of the secular trend will be smaller. Consideration of stratospheric gravitational separation changes may therefore be needed in the future.
We have carried out high-precision measurements of δatm(18O) at the TKB site since 2013. Clear variations of δatm(18O) with a daytime minimum were found for the average diurnal cycles throughout the observation period. The much larger amplitudes of the diurnal δatm(18O) cycles in summer than in winter suggest a substantial contribution of the activities in the terrestrial biosphere to the diurnal cycle. The amplitudes and phases of the diurnal δatm(18O) and δ() cycles simulated by a box model, which incorporated the terrestrial oxygen cycle, were roughly consistent with the observed diurnal cycles in summer. Seasonal changes in the ERs, calculated from the average diurnal cycles of the δ() and amount fractions of CO2, also indicated a larger contribution of the activities in the terrestrial biosphere in summer than in winter. We found that the 5 h and 19 h averaged δatm(18O) also varied in antiphase with δ() in summer. We found that the diurnal cycles of δBIO() and δFF(), estimated by the δatm(18O) method, agreed well with the diurnal δ() cycles driven by activities in the terrestrial biosphere and fossil fuel combustion estimated by the ER method.
The δatm(18O) varied seasonally in antiphase with δ() and was at a minimum in the summer. We found the peak-to-peak amplitude of the average seasonal δatm(18O) cycle to be about 2 per meg. These characteristics were generally reproduced by the box model, and the seasonal δatm(18O) cycle was driven mainly by an input of photosynthetic O2, the δ(18O) of which was about 20 ‰ lower than δatm(18O). The box model also suggested that the seasonal cycle of δatm(18O) was substantially affected by seasonally varying δLW(18O), which indicated the usefulness of δatm(18O) observations to constrain spatiotemporal variations of δLW(18O). There was a secular increase in δatm(18O) by (0.22 ± 0.14) per meg a−1 throughout the observation period. To interpret the secular trend, we used the box model that incorporated two kinds of isotopic effects associated with the DME from B94 and L&B11 to carry out simulations in which we considered the long-term changes in GPP, photorespiration, and δLW(18O). For the calculation of δLW(18O), we also used the 3D model MIROC5-iso. We found that all three components made substantial contributions to the simulated δatm(18O). When we assumed the isotopic effects from B94, the observed secular increase in δatm(18O) was reproduced by the simulation mainly due to the secular increase in GPP. On the other hand, the simulated δatm(18O) based on L&B11 decreased secularly due mainly to the contribution of the secular decrease in ϕ. The substantial differences between the secular δatm(18O) changes in the simulations based on B94 and L&B11 are attributed not only to the differences in the terrestrial and oceanic DME but also to the larger isotopic effect for dark respiration in B94. Therefore, further studies are needed to determine the isotopic effects for the DME precisely.
In conclusion, we confirmed that precise observations of the spatiotemporal variations of δatm(18O) will enable better understanding of the global cycles of O2, CO2, and water. However, no relevant observational results have previously been reported. Additional steps should therefore include observations of the δatm(18O) at some surface stations in both hemispheres using continuous measurement systems that are similar to the system used in the present study and a newly developed, precise measurement system for flask samples. Two- and three-dimensional models to calculate δatm(18O) should be developed to interpret the latitudinal differences of the observed δatm(18O) variations. There is also need for improvement of the three-dimensional model simulation of δLW(18O) because the original δLW(18O) calculated with MIROC5-iso was systematically lower than those reported by past studies. Such progress will better enable detection of the signal of climate changes associated with the DME.
There are a lot of symbols used in the present study, especially for the model simulations. For readers' convenience, names, definitions, and units of the symbols are summarized in Table A1.
Symbol | Definition | Unit |
δatm(18O) | ratio of atmospheric O2 | – |
δLW(18O) | ratio of leaf water | – |
ratio of atmospheric CO2 | – | |
δprecip(18O) | ratio of precipitation | – |
δatm_summer(18O) | δatm(18O) in summer | – |
δatm_winter(18O) | δatm(18O) in winter | – |
δ() | atmospheric ratio | |
δBIO() | variations of the δ() driven by the total activities of the terrestrial and marine biosphere | – |
δFF() | variations of δ() driven by fossil fuel combustion | – |
δ() | atmospheric ratio | – |
y(O2) | O2 amount fraction | µmol mol−1 |
y(O2)winter | atmospheric O2 amount fraction in winter | µmol mol−1 |
y(CO2) | CO2 amount fraction | µmol mol−1 |
Δy(CO2, B) | changes in the amount fraction of CO2 driven by terrestrial biosphere activities | µmol mol−1 |
Δy(CO2, FF) | changes in the amount fraction of CO2 driven by fossil fuel combustion | µmol mol−1 |
GPP | gross primary production | Pg a−1(C equivalents) |
PT | terrestrial O2 production | Pmol a−1 |
rMR | relative ratio for Mehler reaction | – |
rPR | relative ratio for photorespiration | – |
rDR | relative ratio for dark respiration | – |
ΔPS | isotopic effect of terrestrial photosynthesis | – |
ΔOW | isotopic effect of oceanic photosynthesis | – |
εMR | isotopic effect of Mehler reaction | – |
εPR | isotopic effect of photorespiration | – |
εDR | isotopic effect of dark respiration | – |
εLE | isotopic effect of leaf water enrichment | – |
εRes | isotopic effect of total respiration | – |
εOR | isotopic effect of marine respiration | – |
εTS | isotopic effect of air exchange from troposphere to stratosphere | – |
εST | isotopic effect of air exchange from stratosphere to troposphere | – |
εFF | isotopic effect in fossil fuel combustion | – |
RRes | relative ratios of the annual fluxes of O2 from terrestrial respiration | a−1 |
RPS | relative ratios of the annual fluxes of O2 from terrestrial production | a−1 |
ROR | relative ratios of the annual fluxes of O2 from marine respiration | a−1 |
ROP | relative ratios of the annual fluxes of O2 from marine production | a−1 |
RTS | relative ratios of the annual fluxes of O2 from troposphere to stratosphere | a−1 |
RST | relative ratios of the annual fluxes of O2 from stratosphere to troposphere | a−1 |
RFF | relative ratios of the annual O2 consumption by fossil fuel combustion | a−1 |
RLW | isotopic ratio of the leaf water (note that it is not δ) | – |
zL | axis directed from the leaf base to tip | m |
ρ | density of water | kg m−3 |
VL | volume of leaf water | m3 |
AL | area of leaf surface | m2 |
T | transpiration flux | kg m−2 s−1 |
ILA | leaf area index | – |
D | liquid diffusivity of an isotope | m2 s−1 |
τ | crookedness of a leaf | kg m−1 |
ER | O2 and CO2 exchange ratio between the atmosphere and organisms or ecosystems | – |
OR | oxidative ratio expected from the stoichiometry of specific materials | – |
ORFF or αF | OR for fossil fuel combustion | – |
ORB or αB | OR for activities in the terrestrial biosphere | – |
αobs | observed ER | – |
ϕ | ratio of photorespiration to carboxylation | – |
VO_max | maximum oxygenation velocity | µmol m−2 s−1 |
VC_max | maximum carboxylation velocity | µmol m−2 s−1 |
KC | Michaelis–Menten constants for carboxylation | µmol mol−1 |
KO | Michaelis–Menten constants for oxygenation | µmol mol−1 |
In the following formulas, capital letters represent values related to 16O16O and lowercase letters represent values related to 18O16O. Here we define the total amounts of atmospheric 16O16O and 18O16O as M and m, respectively. The DME is a result caused by mixed processes of the sinks and sources. The sinks oxidize by using atmospheric O2, which usually causes isotopic fractionation. On the other hand, the source adds O2 to the atmosphere with an isotopic ratio that is usually independent of atmospheric O2. Here we define the fluxes of sources as FSi and fSi and the fluxes of sink processes as FLj and fLj (i and j represent the types of sources and sinks, respectively). The source isotopic ratio, Δi, is expressed by the following equation:
Here, rstd is the ratio of the standard material. Sink fluxes can be expressed simply by using reaction rates Kj and kj as a first-order approximation:
Here, y(16O16O) and y(18O16O) represent amount fractions of atmospheric 16O16O and 18O16O, respectively. For the one-box model, the ratio of amount fractions is equal to that of the total amounts as follows:
The isotopic ratio of atmospheric O2 is defined as follows:
The enrichment factor, εj, is defined as follows:
The time derivative formula of the isotopic ratio of the atmospheric O2, δ, is
Here, the mass balance equations for 16O16O and 18O16O are
respectively. Substituting these into Eq. (B6) and rearranging using the source isotopic ratios, Δi, and the enrichment factor εj, we get
It can be approximated as follows:
and if we define the ratios of and as RSi and RLj, respectively, then we get
Equation (3) is obtained by applying Eq. (B10) to the various sources and sinks. Note that the sink ratio, RLj, is a negative value. Since εj>0, becomes a positive value, which results in enrichment for the atmosphere. As an exception, εST is given as the isotope effect during transport from the stratosphere to the troposphere, and εST<0. Conversely, the same stratospheric effect would be achieved if εTS was given as a positive value and εST was set to zero.
The δ value in a steady state (δss) can be obtained from the above formula as follows:
For example, if we simply consider only photosynthesis and respiration in terrestrial and oceanic biosphere and assume that and , respectively (here we use the same variables in Table 1), Eq. (B10) becomes the following equation:
In a steady state, Eq. (B12) becomes as follows:
If we substitute the relative ratios of the terrestrial and oceanic fluxes, and , respectively, into Eq. (B13), we get
This formula is often used for simple estimation of DME to separate the terrestrial and oceanic effects.
The observational data are available through Zenodo at https://doi.org/10.5281/zenodo.14221768 (Ishidoya, 2024).
SI designed the study, conducted measurements of δatm(18O), δ(), and CO2 amount fractions, and drafted the manuscript. SaS conducted the box model simulations. AO conducted the MIROC5-iso simulations. All authors approved the final manuscript.
The contact author has declared that none of the authors has any competing interests.
Publisher’s note: Copernicus Publications remains neutral with regard to jurisdictional claims made in the text, published maps, institutional affiliations, or any other geographical representation in this paper. While Copernicus Publications makes every effort to include appropriate place names, the final responsibility lies with the authors.
We thank Nobuyuki Aoki at the National Institute of Advanced Industrial Science and Technology (AIST) for supporting the experiments in Fig. 3b. We also thank Kei Yoshimura at the University of Tokyo for insightful discussions on the isotope-enabled land surface models.
This study was partly supported by JSPS KAKENHI (grant nos. 22H05006, 23H00513, 22H04938, and 22K14095) and the Global Environment Research Coordination System from the Ministry of the Environment, Japan (grant no. METI1953).
This paper was edited by Jan Kaiser and reviewed by Matthias Cuntz, Jeff Severinghaus, and one anonymous referee.
Adachi, Y., Kawamura, K., Armi, L., and Keeling, R. F.: Diffusive separation of the lower atmosphere, Science, 311, 1429, https://doi.org/10.1126/science.1121312, 2006.
Angert, A., Luz, B., and Yakir, D.: Fractionation of oxygen isotopes by respiration and diffusion in soils: Implications for the isotopic composition of atmospheric O2, Global Biogeochem. Cy., 15, 871–881, https://doi.org/10.1029/2000GB001371, 2001.
Angert, A., Barkan, E., Barnett, B., Brugnoli, E., Davidson, E. A., Fessenden, J., Maneepong, S., Panapitukkul, N., Randerson, J. T., Savage, K., Yakir, D., and Luz, B.: The contribution of soil respiration in tropical, temperate, and boreal forests to the 18O enrichment of atmospheric O2, Global Biogeochem. Cy., 17, 1089, https://doi.org/10.1029/2003GB002056, 2003.
Aoki, N., Ishidoya, S., Matsumoto, N., Watanabe, T., Shimosaka, T., and Murayama, S.: Preparation of primary standard mixtures for atmospheric oxygen measurements with less than 1 µmol mol−1 uncertainty for oxygen molar fractions, Atmos. Meas. Tech., 12, 2631–2646, https://doi.org/10.5194/amt-12-2631-2019, 2019.
Barkan, E. and Luz, B.: High precision measurements of 17O/16O and ratios in H2O, Rapid Commun. Mass Spectrom.,19, 3737–3742, 2005.
Basu, S., Miller, J. B., and Lehman, S.: Separation of biospheric and fossil fuel fluxes of CO2 by atmospheric inversion of CO2 and 14CO2 measurements: Observation System Simulations, Atmos. Chem. Phys., 16, 5665–5683, https://doi.org/10.5194/acp-16-5665-2016, 2016.
Basu, S., Lehman, S. J., Miller, J. B., Andrews, A. E., Sweeney, C., Gurney, K. R., Xu, X., Southon, J., and Tans, P. P.: Estimating US fossil fuel CO2 emissions from measurements of 14C in atmospheric CO2, P. Natl. Acad. Sci. USA, 117, 13300–13307, 2020.
Battle, M. O., Munger, J. W., Conley, M., Sofen, E., Perry, R., Hart, R., Davis, Z., Scheckman, J., Woogerd, J., Graeter, K., Seekins, S., David, S., and Carpenter, J.: Atmospheric measurements of the terrestrial O2: CO2 exchange ratio of a midlatitude forest, Atmos. Chem. Phys., 19, 8687–8701, https://doi.org/10.5194/acp-19-8687-2019, 2019.
Bender, M., Sowers, T., and Labeyrie, L.: The Dole effect and its variations during the last 130 000 years as measured in the Vostok ice core, Global Biogeochem. Cy., 8, 363–376, 1994.
Bi, W., He, W., Zhou, Y., Weimin, J., Liu, Y., Liu, Y., Zhang, X., Wei, X., and Cheng, N.: A global 0.05° dataset for gross primary production of sunlit and shaded vegetation canopies from 1992 to 2020, Sci. Data, 9, 213, https://doi.org/10.1038/s41597-022-01309-2, 2022.
Blaine, T. W., Keeling, R. F., and Paplawsky, W. J.: An improved inlet for precisely measuring the atmospheric Ar/N2 ratio, Atmos. Chem. Phys., 6, 1181–1184, https://doi.org/10.5194/acp-6-1181-2006, 2006.
Byrne, M. P. and O'Gorman, P. A.: Trends in continental temperature and humidity directly linked to ocean warming, P. Natl. Acad. Sci. USA, 115, 4863–4868, 2018.
Campbell, J. E., Berry, J. A., Seibt, U., Smith, S. J., Montzka, S. A., Launois, T. Belviso, S. Bopp, L., and Laine, M.: Large historical growth in global terrestrial gross primary production, Nature, 544, 84–87, https://doi.org/10.1038/nature22030, 2017.
Cernusak, L. A., Barbeta, A., Bush, R., Eichstaedt R., Ferrio, J., Flanagan, L., Gessler, A., Martín-Gómez, P., Hirl, R., Kahmen, A., Keitel., C., Lai, C., Munksgaard, N., Nelson, D., Ogée J., Roden, J., Schnyder, H., Voelker, S., Wang L., Stuart-Williams, H., Wingate, L., Yu, W., Zhao, L., and Cuntz, M.: Do 2H and 18O in leaf water reflect environmental drivers differently?, New Phytol., 235, 41–51, https://doi.org/10.1111/nph.18113, 2022.
Cohen, E. R., Cvitas, T., Frey, J. G., Holmstrom, B., Kuchitsu, K., Marquardt, R., Mills, I., Pavese, F., Quack, M., Stohner, J., Strauss, H., Takami, M., and Thor, A. J.: IUPAC Green Book: 3rd edn., RSC Publishing, ISBN 0854044337, ISBN-13 9780854044337, 2007.
Cuntz, M., Ciais, P., Hoffmann, G., Allison, C. E., Francey, R. J., Knorr, W., Tans, P. P., White, J. W. C., and Levin, I.: A comprehensive global three-dimensional model of δ18O in atmospheric CO2: 2. Mapping the atmospheric signal, J. Geophys. Res., 108, 4528, https://doi.org/10.1029/2002JD003154, 2003.
Craig, H.: Standard for reporting concentrations of deuterium and oxygen-18 in natural water, Science, 133, 1833–1834, 1961.
Dole, M.: The relative atomic weight of oxygen in water and in air, J. Am. Chem. Soc., 57, 2731, https://doi.org/10.1021/ja01315a511, 1935.
Dongmann, G., Forstel, H., and Wagener, K.: 18O-rich oxygen from land photosynthesis, Nat. New Biol., 240, 127–128, 1972.
Eddebbar, Y. A., Long, M. C., Resplandy, L., Rödenbeck, C., Rodgers, K. B., Manizza, M., and Keeling, R. F.: Impacts of ENSO on air-sea oxygen exchange: Observations and mechanisms, Global Biogeochem. Cy., 31, 901–921, https://doi.org/10.1002/2017GB005630, 2017.
Faassen, K. A. P., Nguyen, L. N. T., Broekema, E. R., Kers, B. A. M., Mammarella, I., Vesala, T., Pickers, P. A., Manning, A. C., Vilà-Guerau de Arellano, J., Meijer, H. A. J., Peters, W., and Luijkx, I. T.: Diurnal variability of atmospheric O2, CO2, and their exchange ratio above a boreal forest in southern Finland, Atmos. Chem. Phys., 23, 851–876, https://doi.org/10.5194/acp-23-851-2023, 2023.
Farquhar, G. D., von Caemmerer, S., and Berry, J. A.: A biochemical model of photosynthetic CO2 assimilation in leaves of C3 species, Planta, 149, 78–90, 1980.
Farquhar, G. D., Lloyd, J., Taylor, J. A., Flanagan, L., Syvertsen, J. P., Hubick, K., Wong, S., and Ehleringer, J. R.: Vegetation effects on the isotope composition of oxygen in atmospheric CO2, Nature, 363, 439–443, https://doi.org/10.1038/363439a0, 1993.
Gamo, T., Tsutsumi, M., Sakai, H., Nakazawa, T., Tanaka, M., Honda, H., Kubo, H., and Itoh, T.: Carbon and oxygen isotopic ratios of carbon dioxide of a stratospheric profile over Japan, Tellus B, 41, 127–133, https://doi.org/10.1111/j.1600-0889.1989.tb00130.x, 1989.
Gonfiantini, R., Gratzui, S., and Tongiorgi, E.: Oxygen isotopic composition of water in leaves, in Use of Isotopes and Radiation in Soil-Plant Nutrition Studies, Tech. Rep. Ser. 206, 405–410 pp., IAEA, Vienna, 1965.
Graven, H., Fischer, M. L., Lueker T., Jeong, S., Guiderson, T. P., Keeling, R. F., Bambha R., Brophy, K., Callahan, W., and Cui, X.: Assessing fossil fuel CO2 emissions in California using atmospheric observations and models, Environ. Res. Lett., 13, 65007, https://doi.org/10.1088/1748-9326/aabd43, 2018.
Hoffmann, G., Suntz, M., Weber, C., Ciais, P., Friedlingstein, P., Heimann, M., Jouzel, J., Kaduk, J., Maier-Reimer, E., Seibt, U., and Six, K.: A model of the Earth's Dole effect, Global Biogeochem. Cy., 18, GB1008, https://doi.org/10.1029/2003GB002059, 2004.
Ishidoya, S.: Development of high precision measurement technique of the atmospheric O2/N2 ratio and its application to the global carbon cycle, Ph.D. thesis, Tohoku University, Sendai, 2003.
Ishidoya, S.: Dataset for “Diurnal, seasonal, and interannual variations in δ(18O) of atmospheric O2 and its application to evaluate natural/anthropogenic changes in oxygen, carbon, and water cycles” by Ishidoya et al., Zenodo [data set], https://doi.org/10.5281/zenodo.14221768, 2024.
Ishidoya, S. and Murayama, S.: Development of high precision continuous measuring system of the atmospheric O2/N2 and Ar/N2 ratios and its application to the observation in Tsukuba, Japan, Tellus B, 66, 22574, https://doi.org/10.3402/tellusb.v66.22574, 2014.
Ishidoya, S., Aoki, S. and Nakazawa, T.: High precision measurements of the atmospheric O2/N2 ratio on a mass spectrometer, J. Meteorol. Soc. Jpn. 81, 127–140, 2003.
Ishidoya, S., Murayama, S., Takamura, C., Kondo, H., Saigusa, N., Goto, D., Morimoto, S., Aoki, N., Aoki, S., and Nakazawa, T.: O2:CO2 exchange ratios observed in a cool temperate deciduous forest ecosystem of central Japan, Tellus B, 65, 21120, https://doi.org/10.3402/tellusb.v65i0.21120, 2013a.
Ishidoya, S., Sugawara, S., Morimoto, S., Aoki, S., Nakazawa, T., Honda, H., and Murayama, S.: Gravitational separation in the stratosphere – a new indicator of atmospheric circulation, Atmos. Chem. Phys., 13, 8787–8796, https://doi.org/10.5194/acp-13-8787-2013, 2013b.
Ishidoya, S., Tsuboi, K., Matsueda, H., Murayama, S., Taguchi, S., Sawa, Y., Niwa, Y., Saito, K., Tsuji, K., Nishi, H., Y. Baba, Y., Takatsuji, S., Dehara, K., and Fujiwara, H.: New atmospheric O2/N2 ratio measurements over the western North Pacific using a cargo aircraft C-130H, SOLA, 10, 23–28, https://doi.org/10.2151/sola.2014-006, 2014.
Ishidoya, S., Sugawara, H., Terao, Y., Kaneyasu, N., Aoki, N., Tsuboi, K., and Kondo, H.: O2: CO2 exchange ratio for net turbulent flux observed in an urban area of Tokyo, Japan, and its application to an evaluation of anthropogenic CO2 emissions, Atmos. Chem. Phys., 20, 5293–5308, https://doi.org/10.5194/acp-20-5293-2020, 2020.
Ishidoya, S., Sugawara, S., Tohjima, Y., Goto, D., Ishijima, K., Niwa, Y., Aoki, N., and Murayama, S.: Secular change in atmospheric Ar/N2 and its implications for ocean heat uptake and Brewer–Dobson circulation, Atmos. Chem. Phys., 21, 1357–1373, https://doi.org/10.5194/acp-21-1357-2021, 2021.
Ishidoya, S., Tsuboi, K., Niwa, Y., Matsueda, H., Murayama, S., Ishijima, K., and Saito, K.: Spatiotemporal variations of the δ(O2/N2), CO2 and δ(APO) in the troposphere over the western North Pacific, Atmos. Chem. Phys., 22, 6953–6970, https://doi.org/10.5194/acp-22-6953-2022, 2022.
Ishidoya, S., Tsuboi, K., Kondo, H., Ishijima, K., Aoki, N., Matsueda, H., and Saito, K.: Measurement report: Method for evaluating CO2 emissions from a cement plant using atmospheric δ() and CO2 measurements and its implication for future detection of CO2 capture signals, Atmos. Chem. Phys., 24, 1059–1077, https://doi.org/10.5194/acp-24-1059-2024, 2024.
Joussaume, S. and Jouzel, J.: Paleoclimatic tracers: An investigation using an atmospheric general circulation model under ice age conditions 2. Water isotopes, J. Geophys. Res., 98, 2807–2830, 1993.
Jouzel, J., Russell, G. L., Suozzo, R. J., Koster, R. D., White, J. W. C., and Broecker, W. S.: Simulations of the HDO and HO atmospheric cycles using the NASA GISS general circulation model: The seasonal cycle for present-day conditions, J. Geophys. Res., 92, 14739–14760, 1987.
Keeling, C. D., Piper, S. C., Bacastow, R. B., Wahlen, M., Whorf, T. P., Heimann, M., and Meijer, H. A.: Exchanges of atmospheric CO2 and 13CO2 with the terrestrial biosphere and oceans from 1978 to 2000, I. Global aspects, SIO Reference Series, No. 01-06, Scripps Institution of Oceanography, San Diego, 88 pp., https://scrippsco2.ucsd.edu/assets/publications/keeling_sio_ref_series_exchanges_of_co2_ref_no_01-06_2001.pdf (last access: 1 February 2025), 2001.
Keeling, R. and Manning, A.: Studies of Recent Changes in Atmospheric O2 Content, in: Treatise on Geochemistry, 2nd edn., Elsevier Inc., 5, 385–404, https://doi.org/10.1016/B978-0-08-095975-7.00420-4, 2014.
Keeling, R. F.: Development of an Interferometric Oxygen Analyzer for Precise Measurement of the Atmospheric O2 Mole Fraction, PhD thesis, Harvard University, Cambridge, 1988.
Keeling, R. F.: The atmospheric oxygen cycle: The oxygen isotopes of atmospheric CO2 and O2 and the N2/O2 ratio, Rev. Geophys., 33, 1253–1262, 1995.
Keeling, R. F., Blaine, T., Paplawsky, B., Katz, L., At- wood, C., and Brockwell, T.: Measurement of changes in atmospheric Ar/N2 ratio using a rapid-switching, single-capillary mass spectrometer system, Tellus B, 56, 322–338, https://doi.org/10.3402/tellusb.v56i4.16453, 2004.
Liu, J., Jiang, C., Wu, H., Guo, L., Zhang, H., and Zhao, Y.: Controls on leaf water hydrogen and oxygen isotopes: a local investigation across seasons and altitude, Hydrol. Earth Syst. Sci., 27, 599–612, https://doi.org/10.5194/hess-27-599-2023, 2023.
Luz, B. and Barkan, E.: The isotopic composition of atmospheric oxygen, Global Biogeochem. Cy., 25, GB3001, https://doi.org/10.1029/2010GB003883, 2011.
Luz, B., Barkan, E., Bender, M. L., Thiemens, M. H., and Boering, K. A.: Triple-isotope composition of atmospheric oxygen as a tracer of biosphere productivity, Nature, 400, 547–550, https://doi.org/10.1038/22987, 1999.
Madani, N., Parazoo, N. C., Kimball, J. S., Ballantyne, A. P., Reichle, R. H., Maneta, M., Saatchi, S., Palmer P. I., Liu, Z., and Tagesson, T.: Recent amplified global gross primary productivity due to temperature increase is offset by reduced productivity due to water constraints, AGU Advances, 2, e2020AV000180, https://doi.org/10.1029/2020AV000180, 2020.
Manning, A. C. and Keeling, R. F.: Global oceanic and terrestrial biospheric carbon sinks from the Scripps atmospheric oxygen flask sampling network, Tellus B, 58, 95–116, 2006.
Minejima, C., Kubo, M., Tohjima, Y., Yamagishi, H., Koyama, Y., Maksyutov, S., Kita, K., and Mukai, H.: Analysis of ΔOCO2 ratios for the pollution events observed at Hateruma Island, Japan, Atmos. Chem. Phys., 12, 2713–2723, https://doi.org/10.5194/acp-12-2713-2012, 2012.
Morita, N.: The increased density of air oxygen relative to water oxygen, J. Chem. Soc. Japan, 56, 1291, 1935.
Morgan, E. J., Manizza, M., Keeling, R. F., Resplandy, L., Mikaloff-Fletcher, S. E., Nevison, C. D., Jin, Y., Bent, J. D., Aumont, O., Doney, S. C., Dunne, J. P., John, J., Lima, I. D., Long, M. C., and Rodgers, K. B.: An atmospheric constraint on the seasonal air-sea exchange of oxygen and heat in the extratropics. J. Geophys. Res.-Oceans, 126, e2021JC017510. https://doi.org/10.1029/2021JC017510, 2021.
Murayama, S., Takamura, C., Yamamoto, S., Saigusa, N., Morimoto, S., Kondo, H., Nakazawa, T., Aoki, S., Usami, T., and M. Kondo, M.: Seasonal variations of atmospheric CO2, δ13C, and δ18O at a cool temperate deciduous forest in Japan: Influence of Asian monsoon, J. Geophys. Res., 115, D17304, https://doi.org/10.1029/2009JD013626, 2010.
Nevison, C. D., Keeling, R. F., Kahru, M., Manizza, M., Mitchell, B. G., and Cassar, N.: Estimating net community production in the Southern ocean based on atmospheric potential oxygen and satellite ocean color data, Global Biogeochem. Cy., 26, GB1020, https://doi.org/10.1029/2011GB004040, 2012.
Okazaki, A. and Yoshimura, K.: Development and evaluation of a system of proxy data assimilation for paleoclimate reconstruction, Clim. Past, 13, 379–393, https://doi.org/10.5194/cp-13-379-2017, 2017.
Okazaki, A. and Yoshimura, K.: Global evaluation of proxy system models for stable water isotopes with realistic atmospheric forcing, J. Geophys. Res.-Atmos., 124, 8972–8993. https://doi.org/10.1029/2018JD029463, 2019.
Olsen, M. A., Schoeberl, M. R., and Douglass, A. R.: Stratosphere-troposphere exchange of mass and ozone, J. Geophys. Res., 109, D24114, https://doi.org/10.1029/2004JD005186, 2004.
Peylin, P., Ciais, P., Denning, A. S., Tans, P. P., Berry, J. A., and White, J. W. C.: A 3-dimensional study of δ18O in atmospheric CO2: Contribution of different land ecosystems, Tellus B, 51, 642–667, 1999.
Pickers, P. A., Manning, A. C., Le Quéré, C., Forster, G. L., Luijkx, I. T., Gerbig, C., Fleming, L. S., and Sturges, W. T.: Novel quantification of regional fossil fuel CO2 reductions during COVID-19 lockdowns using atmospheric oxygen measurements, Sci. Adv., 8, eabl9250, https://doi.org/10.1126/sciadv.abl9250, 2022.
Plavcová, L., Hronková, M., Šimková, M., Květoň , J., Vráblová, M., Kubásek, and Šantrůček, J.: Seasonal variation of δ18O and δ2H in leaf water of Fagus sylvatica L. and related water compartments, J. Plant Physiol., 227, 56–65, 2018.
Rozanski, K., Araguás-Araguás, L., and Gonfiantini, R.: Relation between long-term trends of oxygen-18 isotope composition of precipitation and climate, Science, 258, 981–985, https://doi.org/10.1126/science.258.5084.981, 1992.
Rubino, M., Etheridge, D., Thornton, D., Allison, C., Francey, R., Langenfelds, R., Steele, P., Trudinger, C., Spencer, D., Curran, M., Van Ommen, T., and Smith, A.: Law Dome Ice Core 2000-Year CO2, CH4, N2O and δ13C-CO2. v2. CSIRO, Data Collection, https://doi.org/10.25919/5bfe29ff807fb, 2019.
Schumacher, M., Werner, R. A., Meijer, H. A. J., Jansen, H. G., Brand, W. A., Geilmann, H., and Neubert, R. E. M.: Oxygen isotopic signature of CO2 from combustion processes, Atmos. Chem. Phys., 11, 1473–1490, https://doi.org/10.5194/acp-11-1473-2011, 2011.
Seibt, U., Berry, J. A., Battle, M., and Severinghaus, J. P.: Exploring potential anthropogenic changes in the Dole-Morita effect, abstract of the 7th International CO2 Conference, https://gml.noaa.gov/icdc7/proceedings/abstracts/seibtFF351.pdf (last access: 1 February 2025), 2005.
Sellers, P., Randall, D. A., Collatz, G. J., Berry, J. A., Field, C. B., Dazlich, D. A., Zhang, C., Collelo, G. D., and Bounoua, L.: A Revised Land Surface Parameterization (SiB2) for Atmospheric GCMS. Part I: Model Formulation, J. Climate, 9, 676–705, 1996.
Severinghaus, J.: Studies of the terrestrial O2 and carbon cycles in sand dune gases and in biosphere 2, PhD thesis, Columbia University, New York, 1995.
Severinghaus, J. P., Beaudette, R., Headly, M. A., Taylor, K., and Brook, E. J.: Oxygen-18 of O2 records the impact of abrupt climate change on the terrestrial biosphere, Science, 324, 1432–1434, https://doi.org/10.1126/Science.1169473, 2009.
Sturm, P., Leuenberger, M., Valentino, F. L., Lehmann, B., and Ihly, B.: Measurements of CO2, its stable isotopes, O2/N2, and 222Rn at Bern, Switzerland, Atmos. Chem. Phys., 6, 1991–2004, https://doi.org/10.5194/acp-6-1991-2006, 2006.
Sugawara, H., Ishidoya, S., Terao, Y., Takane, Y., Kikegawa, Y., and Nakajima, K.: Anthropogenic CO2 emissions changes in an urban area of Tokyo, Japan, due to the COVID-19 pandemic: A case study during the state of emergency in April–May 2020, Geophys. Res. Lett., 48, e2021GL092600, https://doi.org/10.1029/2021GL092600, 2021.
Sugawara, S., Ishidoya, S., Aoki, S., Morimoto, S., Nakazawa, T., Toyoda, S., Inai, Y., Hasebe, F., Ikeda, C., Honda, H., Goto, D., and Putri, F. A.: Age and gravitational separation of the stratospheric air over Indonesia, Atmos. Chem. Phys., 18, 1819–1833, https://doi.org/10.5194/acp-18-1819-2018, 2018.
Thiemens, M. H.: Mass-independent isotope effects in planetary atmospheres and the early solar system, Science, 283, 341–345, https://doi.org/10.1126/science.283.5400.341, 1999.
Tohjima, Y., Mukai, H., Machida, T., Hoshina, Y., and Nakaoka, S.-I.: Global carbon budgets estimated from atmospheric O2/N2 and CO2 observations in the western Pacific region over a 15-year period, Atmos. Chem. Phys., 19, 9269–9285, https://doi.org/10.5194/acp-19-9269-2019, 2019.
Watanabe, M., Suzuki, T., O'ishi, R., Komura, Y., Watanabe, S., Emori, S., Takemura, T., Chikira, M., Ogura, T., Sekiguchi, M., Takata, K., Yamazaki, D., Yokohata, T., Nozawa, T., Hasumi, H., Tatebe, H., and Kimoto, M.: Improve climate simulated by MIROC5: Mean states, variability, and climate sensitivity, J. Climate, 23, 6312–6335, 2010.
Weiss, R. F.: The solubility of nitrogen, oxygen and argon in water and seawater, Deep-Sea Res., 17, 721–735, 1970.
Welp, L. R., Lee, X., Kim, K., Griffis, T. J., Billmark, K. A., and Baker, J. M.: δ18O of water vapour, evapotranspiration and the sites of leaf water evaporation in a soybean canopy. Plant. Cell Environ., 31, 1214–1228, 2008.
Welp, L. R., Keeling, R. F., Meijer, H. A. J., Bollenbacher, A. F., Piper, S. C., Yoshimura, K., Francey, R. J., Allison, C. E., and Wahlen, M.: Interannual variability in the oxygen isotopes of atmospheric CO2 driven by El Niño, Nature, 477, 579–582, https://doi.org/10.1038/nature10421, 2011.
West, J. B., Sobek, A., and Ehleringer, J. R.: A simplified GIS approach to modeling global leaf water isoscapes, PLoS ONE, 3, e2447, https://doi.org/10.1371/journal.pone.0002447, 2008.
Yoshimura, K., Miyazaki, S., Kanae, S., and Oki, T.: Iso-MATSIRO, a land surface model that incorporates stable water isotopes, Glob. Planet. Change, 51, 90–107, 2006.
Zheng, Y., Shen, R., Wang, Y., Li, X., Liu, S., Liang, S., Chen, J. M., Ju, W., Zhang, L., and Yuan, W.: Improved estimate of global gross primary production for reproducing its long-term variation, 1982–2017, Earth Syst. Sci. Data, 12, 2725–2746, https://doi.org/10.5194/essd-12-2725-2020, 2020.