the Creative Commons Attribution 4.0 License.
the Creative Commons Attribution 4.0 License.
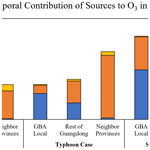
Spatiotemporal source apportionment of ozone pollution over the Greater Bay Area
Yiang Chen
Xingcheng Lu
Jimmy C. H. Fung
It has been found that ozone (O3) pollution episodic cases are prone to appear when the Greater Bay Area (GBA) is under the control of typhoons and subtropical high-pressure systems in summer. To prevent this pollution effectively and efficiently, it is essential to understand the contribution of O3 precursors emitted from different periods and areas under these unfavorable weather conditions. In this study, we further extended the Ozone Source Apportionment Technology (OSAT) from the Comprehensive Air Quality Model with Extensions (CAMx) model to include the function of tracking the emission periods of O3 precursors. Subsequently, the updated OSAT module was applied to investigate the spatiotemporal contribution of precursor emissions to the O3 concentration over the GBA in July and August 2016, when several O3 episodic cases appeared in this period. Overall, the emissions within the GBA, from other regions of Guangdong province (GDo), and from the neighboring provinces were the three major contributors, accounting for 23 %, 15 %, and 17 % of the monthly average O3 concentration, respectively. More than 70 % of the O3 on the current day was mainly formed from the pollutants emitted within 3 d, and the same day's emission contributed approximately 30 %. During the O3 episodes, when the typhoon approached, more pollutants emitted 2–3 d before from the GDo and adjacent provinces were transported to the GBA, leading to an increase in O3 concentrations within this region. Under the persistent influence of northerly wind, the pollutants originating from eastern China earlier than 2 d previously can also show a noticeable impact on the O3 over the GBA on the present day, accounting for approximately 12 %. On the other hand, the O3 pollution was primarily attributed to the local emission within 2 d when the GBA was mainly under the influence of the subtropical high-pressure systems. These results indicate the necessity for considering the influence of meteorological conditions in implementing the control measures. Meanwhile, analogous relationships between source area/time and receptor were derived by the zero-out method, supporting the validity of the updated OSAT module. Our approach and findings could offer more spatiotemporal information about the sources of O3 pollution, which could aid in the development of effective and timely control policies.
- Article
(8303 KB) - Full-text XML
-
Supplement
(3061 KB) - BibTeX
- EndNote
As one of the major air pollutants, ozone (O3) is a secondary pollutant formed by the photochemical reactions of nitrogen oxides (NOx) and volatile organic compounds (VOCs) in the presence of solar radiation. Surface O3 has detrimental effects on human health, such as causing respiratory and cardiovascular problems (Maji et al., 2019; Yin et al., 2017). It could also lead to the reduction in the crop yield and the damage of vegetation (Gong et al., 2021; Y. Wang et al., 2022). With the implementation of a series of control policies in China since 2013, the concentrations of other air pollutants, including particulate matter with aerodynamic diameters smaller than 2.5 µm (PM2.5), NOx, and sulfur dioxide (SO2), have gradually decreased. In contrast, due to the large reduction in the NOx emission and limited control of VOC emission in the early stage of the control period (Liu et al., 2023), the O3 concentration still continuously increased and has become the primary air pollutant across China. The Greater Bay Area (GBA), including nine cities in the Pearl River Delta (PRD), Hong Kong (HK), and Macau special administrative regions (SARs), is one of the most developed agglomerations in China and also faces the heavy O3 pollution problem. Based on the analysis of surface monitor observations, Cao et al. (2024) and Feng et al. (2023) revealed an overall upward trend in the maximum daily 8 h average (MDA8) O3 in the PRD region and HK, with an increase of 1.11 and 0.22 from 2013 to 2019 and from 2011 to 2022, respectively.
The formation of O3 is closely related to the sources of its precursors, and much effort has been devoted to investigating the source region and source category of O3 in the GBA using different methods (Liu et al., 2020). He et al. (2019) applied the positive matrix factorization (PMF) method to resolve the anthropogenic sources of VOCs. Combining a photochemical box model with the master chemical mechanism (PBM–MCM), they found that vehicular sources were the most significant source of the O3 formation, followed by biomass burning and solvent usage. Li et al. (2012) applied the Comprehensive Air Quality Model with Extensions (CAMx) and Ozone Source Apportionment Technology (OSAT) numerical model to track the source contribution to O3 in the GBA region and found that elevated local and regional contributions were dominant during the O3 episodes. W. Yang et al. (2019) applied the NAQPMS model with an online source apportionment module to explore the sources of O3 in different seasons in the PRD region. Their results showed that the vehicle sources were the largest contributor, followed by industry. Fang et al. (2021) used multi-modeling source apportionments to quantify the source impact on O3 in the PRD region. The on-road automobile and industrial process sources were found to be two major contribution sectors. Integrating satellite data and sensitivity model simulations, N. Wang et al. (2022) found that enhanced biogenic emission and cross-regional transport due to approaching typhoons were significant factors leading to ozone pollution in the PRD and Yangtze River Delta (YRD) regions. In addition to the source region and category, the emitting time of pollutants is also an important perspective that needs better understanding for effective and efficient control policymaking. Several studies have attempted to evaluate this temporal perspective (Xie et al., 2021; Ying et al., 2021). Xie et al. (2023) analyzed the age evolution of PM2.5 during a haze event in eastern China. The study showed that during the regional transport stage, more aged particles from the North China Plain (NCP) were transported to the downwind YRD region, leading to a sharp increase in the average age of different components of PM2.5 in the YRD. Y. Chen et al. (2022) investigated the temporal contributions of emissions to the concentration of PM2.5 in the PRD region and found that pollutants emitted 2 d earlier were trapped within the PRD region due to the weak wind during the episodic pollution. However, these studies mainly focused on the PM2.5, and the temporal contribution of sources to the O3 in the GBA region still remains unclear.
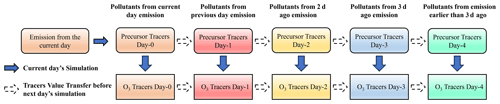
Figure 1Schematic diagram of temporal source apportionment (colors represent the pollutants released or formed by emissions on different days).
In addition to emission, meteorological conditions, another key factor that can affect the transportation, production, and destruction of O3 and its precursors, have also received much attention and have been extensively studied (Lu et al., 2019; T. Wang et al., 2017, 2022). The long-/short-term effects of meteorological changes on ozone concentrations have been investigated through various methods, such as statistical analysis of observations and numerical modeling (L. Yang et al., 2019; J. Xu et al., 2023; Zheng et al., 2023). Liu and Wang (2020) conducted sensitivity simulations using the Community Multiscale Air Quality (CMAQ) model to evaluate the contribution of variations in weather conditions to summer O3 levels from 2013–2017. Their results showed that the meteorological conditions were more conducive to ozone formation from 2014 to 2016 than in 2013, leading to an increase of more than 10 ppbv in MDA8 O3 in Guangzhou. Different objective and subjective classification technologies have been applied to summarize the impacts of unfavorable weather patterns on O3 pollution (Han et al., 2020; X. Chen et al., 2022; Cao et al., 2023). Gao et al. (2018) summarized the common synoptic patterns in the Guangdong province where O3 pollution always occurred and concluded that the subtropical high-pressure system and typhoons are two major patterns accounting for more than 60 % of cases in the PRD regions during 2014–2016. The major influencing factors and the dominant physical and chemical processes were also identified and analyzed (Gong et al., 2022; Zeren et al., 2022; Wu et al., 2023). Ouyang et al. (2022) analyzed the impact of a subtropical high and a typhoon on ozone pollution in the PRD region and found that low relative humidity, high boundary layer height, weak northerly surface wind, and strong downdrafts were the main meteorological factors contributing to the pollution. Deng et al. (2019) illustrated that the actinic flux was the important cause of the co-occurrence of high-ozone and aerosol pollution under the control of the typhoon periphery. Y. Li et al. (2022) also investigated the impact of peripheral circulation characteristics of typhoons and found that the chemical formation and vertical mixing effects were two major contributors to the enhancement of O3 levels, while the advection showed negative values. Qu et al. (2021) analyzed the typhoon-induced and non-typhoon O3 events in the PRD region and revealed that under the influence of typhoons, the contributions from the transport processes and sources outside the PRD increased. Usually, the ozone events are attributed to changes in meteorological conditions rather than sudden increases in emission intensity (Lin et al., 2019; Y. Xu et al., 2023). The changes in weather conditions will affect the time-sensitivity of emitted pollutants and lead to different types of O3 pollution, such as the long-range transport of aged pollutants or accumulation of local fresh pollutants. Hence, it is of great importance to clarify the impact of the pollutants from different source areas and emitting periods on the O3 pollution under different weather conditions in the GBA.
In this study, the CAMx–OSAT model was extended and used to track the temporal contribution of pollutants to the O3 pollution over the GBA under the impact of typhoons and subtropical high pressure during July and August in 2016, the two most important weather systems that influence O3 pollution over the GBA. The rest of this paper is organized as follows. The temporal source apportionment (TSA) method, the configuration of experiments, and the ozone episodes are introduced in Sect. 2. The spatiotemporal source apportionment results and zero-out simulation results are shown and discussed in Sect. 3. The major conclusions are summarized in Sect. 4.
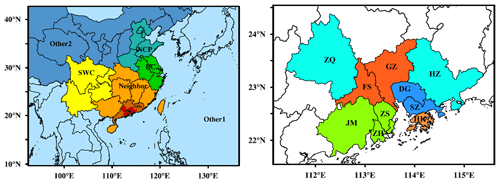
Figure 2The configuration of source areas in the source apportionment experiments (one color represents one source area). The GBA sources were divided into five source areas. Other 1 represents the ocean and other countries and regions. Other 2 represents the other areas within mainland China in the simulation domain. Publisher's remark: Please note that the above figure contains disputed territories.
2.1 Temporal source apportionment method
Previously, we have successfully implemented the PM2.5 temporal source apportionment method in the CAMx model and applied it to investigate the temporal influence of emissions on PM2.5 in the GBA (Y. Chen et al., 2022). Here, we further extend this method to track the temporal contribution of emissions to the precursors and the formation of O3. Similar to the OSAT method, the input data used in the TSA method developed in this work include the source area map and hourly emission data. The source area map assigns each model grid cell to one of the specific source regions. The hourly emission data are the same as the ones used in the normal CAMx model simulation without turning on the source apportionment module. The basic mechanism of the TSA method is to track the contribution of pollutants from different emitting periods using a set of tracers. In the TSA method (Fig. 1), the Precursor Tracer Day-x was used to track the precursors emitted from x d previously. The O3 Tracer Day-x was used to track the O3 formed from the precursors emitted from the corresponding x d previously (namely Precursor Tracer Day-x). The tracers in Day-x can be set into different finer periods (e.g., every 1, 6, or 24 h) as required. The total number of tracers will be decided according to the entire tracking period and the minimum tracking period per tracer. For instance, if the entire tracking period is 5 d, and the minimum tracking period per tracer is every 6 h, the total number of tracers will be 20. In each time step, the tracers go through all the processes, including emission, transport, diffusion, and chemical reactions, sequentially, as in the normal CAMx model simulation. Therefore, the precursors and O3 tracers that tracked different periods are calculated simultaneously. When the pollutants are emitted from the sources, they will be assigned to the Precursor Tracer Day-0, while the precursor tracers that tracked other periods and the O3 tracers remain unchanged. The data transfer between tracers (e.g., Day-1 to Day-2 and Day-0 to Day-1; dashed arrow in Fig. 1) will be conducted once after 1 d of simulation. As shown in Fig. 1, during each day's simulation, the contribution of the present day's emission is consistently tracked by the Day-0 tracers. After completing the current day's simulation and before starting the next day's simulation, each tracer Day-x value transfers to the corresponding tracer, Day-(x + 1), which represents 1 d earlier than Day-x, following the specified sequence. For example, beginning from the penultimate tracer, we take values from the Day-3 transfer and add them to Day-4, then add the values from the Day-2 transfer to Day-3, followed by Day-1 to Day-2, and, last, Day-0 to Day-1 (dashed arrow in Fig. 1). Here, the value in the Day-3 tracer will be added into the last tracer (Day-4) because the last tracer represents the total contribution of pollutants emitted earlier than 3 d previously. In the same way as the OSAT method, the TSA method also utilizes the photochemical indicator, namely the ratio of the production rate of hydrogen peroxide (H2O2) and nitric acid (HNO3), to determine the sensitivity of O3 formation. When the O3 formation is classified as NOx-limited (VOC-limited), the contributions are distributed to the NOx (VOC) sources emitted at different periods, based on the proportion of their emissions to the total NOx (VOC) emissions. More details of this method can be found in Y. Chen et al. (2022).
2.2 Model configuration and evaluation
The Weather Research and Forecasting (WRFv3.9) model was applied for a meteorological field simulation. The initial and boundary conditions for the WRF model were gained from the Final Operational Global Analysis data (FNL). CAMx v7.1 was used to simulate the spatiotemporal variation in the air pollutants. The initial and boundary conditions for the CAMx model were provided by the Model for Ozone and Related chemical Tracers, version 4 (MOZART-4). Regarding the emission, a highly resolved emission inventory provided by the Hong Kong Environmental Protection Department (HKEPD) was used for the GBA region, and the Multi-resolution Emission Inventory for China (MEIC; Li et al., 2017), developed by Tsinghua University, was applied for the area outside the GBA region. The biogenic emissions for the entire domain were calculated by the Model of Emissions of Gases and Aerosols from Nature (MEGAN version 3.1). The CB05 gas-phase chemistry, the ISORROPIA inorganic aerosol scheme, and the SOAP secondary organic aerosol scheme were used in the simulation. This model system has been applied to analyze the source of O3, NOx, and PM2.5 in the GBA region in previous studies (Lu et al., 2016; W. Chen et al., 2022; Y. Chen et al., 2022). Those interested in more configurations of this model system can refer to the work of Lu et al. (2016).
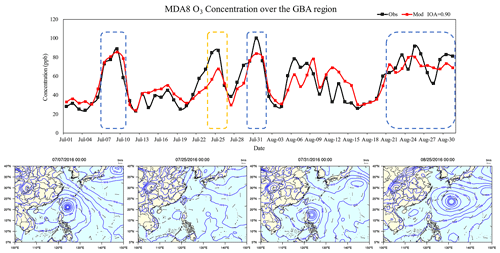
Figure 3The time series of the observed and simulated MDA8 O3 concentration over the GBA during July–August 2016 and the synoptic patterns during the O3 episodes. Blue box: typhoon case; yellow box: subtropical high-pressure case. The O3 observations were obtained from the CNEMC and the HKEPD. The synoptic patterns were plotted using ERA5 reanalysis data.
The three-nested simulation domain of the WRF–CAMx model is shown in Fig. S1 in the Supplement. The resolution of three domains was 27, 9, and 3 km, respectively. For the source apportionment experiments, the simulation domain was divided into 12 source regions, as shown in Fig. 2, including the North China Plain (NCP); eastern China (EC); southwestern China (SWC); other regions of inland China (Other 2); the ocean and other countries and regions (Other 1); neighboring provinces around Guangdong province (Neighbor); other regions within Guangdong province but outside the GBA (GDo); and different subregions within the GBA, namely Guangzhou and Foshan (GF), Shenzhen and Dongguan (SD), Hong Kong (HK), Zhuhai, Zhongshan, and Jiangmen (ZZJ), and Zhaoqing and Huizhou (GBAo). The cities within the GBA were separated into different subregions mainly based on administrated boundaries and their geographical location (in the same way as in the work of Y. Chen et al., 2022). The subregions mainly consist of neighboring cities. Zhaoqing and Huizhou, located in the northwestern and northeastern corners, respectively, were categorized into one group since they have a relatively lower emission density compared to other cities. Previous studies indicated that the air pollutants in Hong Kong were usually more influenced by long-range transport from regions outside the GBA, in contrast to the other cities in the GBA (Li et al., 2012; W. Chen et al., 2022; Y. Chen et al., 2022). Hence, Hong Kong is treated as a separate entity. The contribution of initial and domain 1 (27 km, D1) boundary conditions was also treated as two sources. In the following analysis, for the O3 concentrations in the target area over the GBA, the influence of pollutants emitted within the target area is treated as the local contribution, and the influence of pollutants originating from the other areas within the GBA region is treated as the regional contribution. The source tracking time period is 5 d. Day-0, Day-1, Day-2, and Day-3 represent the pollutants emitted within the present day, the previous day, 2 d previously, and 3 d previously, respectively. Day-4 represents the total contribution of pollutants emitted earlier than 3 d previously. The simulation period is July and August 2016, and the model spin-up was for 7 d to reduce the influence of the initial condition.
The performance of simulated hourly 2 m temperature, 10 m wind speed, and O3 concentration was evaluated and is shown in Table S1. Here, the statistical metrics, including mean bias (MB), normalized mean bias (NMB), index of agreement (IOA), and root mean square error (RMSE), were used for a model performance evaluation. The mathematical formulas for these metrics can be found in Table S6. The recommended values suggested by Emery and Tai (2001) and the US EPA (2007) were used as benchmarks and are shown in the parentheses in Table S1. The temperature is a little overestimated with a MB of 0.33, while the wind speed is underestimated with a MB of −0.45. The IOA is 0.82 and 0.70 for temperature and wind speed, respectively. The MBs and IOAs both fulfill the criteria. But the RMSE shows a result that is little higher than the value of the criteria. Regarding the O3, the IOA reaches 0.81. The small positive MB indicates that the model slightly overestimates the O3 concentration. The NMB is 0.13, which also meets the criteria. The time series comparison (Fig. S2) of the average O3 concentration in Guangzhou, Hong Kong, and Zhuhai illustrates that the model can catch and reproduce the variation trend of the O3 concentration in GBA well, although there are a few differences between the simulated and measured concentrations for some peaks, like during the period between 25 and 31 July in Guangzhou. Overall, the performance of the model simulation is comparable to the other studies in this region (Li et al., 2022; Yang and Zhao, 2023). Therefore, the simulation result is reasonable and can be further used for source analysis.
Table 1Contribution of pollutants from different source areas to the average hourly O3 concentration over the GBA in different cases.
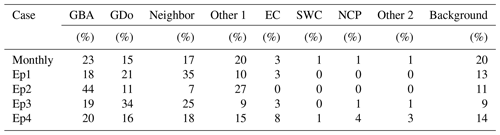
* Here, GDo represents areas outside the GBA but within Guangdong province. Neighbor represents the provinces around Guangdong province. Other 1 represents the ocean and other countries and regions. Other 2 represents other areas within mainland China in the simulation domain. Background represents the contribution of initial and boundary conditions.
2.3 Ozone episodes
There were several O3 episodes that occurred during the simulation period. Here, the maximum daily 8 h average (MDA8) O3 concentration over the GBA was calculated using the observation data from the surface monitoring stations (Fig. 3). The O3 observations were obtained from the China National Environmental Monitoring Centre (CNEMC) and the HKEPD. Here, pollution days were identified when the average MDA8 O3 observation concentrations over the GBA exceeded 80 ppb (W. Wang et al., 2022). To better capture the evolution of the O3 pollution, based on the characteristics of concentration variation, the days preceding and following the O3 pollution days were also included in the analysis, and the whole period was considered an O3 episode. The first O3 pollution occurred between the 7 and 10 July (Ep1). During this period, the GBA region was initially controlled by the subtropical high-pressure system. When the typhoon northwesterly moved from the Philippines Sea towards Taiwan, the GBA was located in the peripheral subsidence region. After the typhoon made landfall, the high-pressure situation in the GBA was relieved, and the O3 concentration decreased. There were another two O3 episodes between 24 July and 1 August. The GBA was mainly influenced by the subtropical high-pressure system during 24–26 July (Ep2), while the synoptic condition of the GBA between 30 July–1 August (Ep3) was similar to that of Ep1. During Ep3, another typhoon moved northwesterly from the Philippines Sea towards southern China and influenced the GBA region. It was found that this type of typhoon movement path was often accompanied by the occurrences of O3 pollution in the GBA (N. Wang et al., 2022). In late August, under the joint influence of the subtropical high-pressure system and the typhoon, the O3 over the GBA maintained a high concentration level between 21–31 August (Ep4). Unlike previous two typhoons, this typhoon moved southerly from the ocean of southern Japan and stayed near the ocean of northeastern Philippines. The typhoon moved northwards after 27 August, and northerly winds prevailed in the GBA. Hence, we conducted the simulation of the O3 concentration in the GBA during July–August 2016 and analyzed the spatiotemporal contributions of emissions in these episodic cases.
3.1 Source area contributions
The contribution of different source areas to the average hourly O3 concentration in the GBA region is shown in Table 1. Here, the contribution from the initial and boundary conditions was treated as the background contribution. Regarding the monthly average O3 concentration over the GBA region, the emission within the GBA can contribute about 23 %. The pollutants from other regions within Guangdong Province (GDo) and the neighboring provinces also had a large contribution, accounting for approximately 15 % and 17 %, respectively. Under the influence of prevailing southerly winds in the summertime, the contribution from the ocean and other countries and regions can also account for about 20 %. As some studies suggested that O3 originating from foreign countries is quite limited (Sahu et al., 2021), the main contributor of this source is likely to be marine ship emissions from the ocean. The pollutants from other source regions had a limited effect on the O3 in the GBA.
The monthly average source area contribution to the four subregions within the GBA region can be found in Table S2. Results show that the local emission had a significant influence on O3 in the GF and SD regions, accounting for 17 % of O3, but its impact was lower than 10 % on O3 in the ZZJ region and HK. The contribution of GBA regional emissions (contributed by other GBA tagged regions) had a relatively large impact on the monthly average O3 concentration in the GF region than the other subregions. This is because of the prevailing southerly wind in summer, which resulted in a greater influence of the pollutants within the GBA region on O3 in the GF area. The influences of pollutants from GDo and the neighboring provinces on different subregions ranged from 25 % to 31 %. As coastal regions, the ZZJ region and HK were also more affected by sources of the ocean and other countries and regions, which occupied about 24 % and 27 %, respectively.
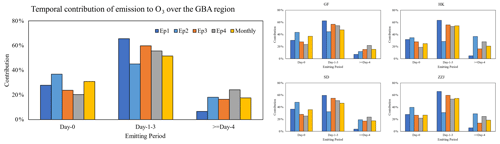
Figure 4Contribution of pollutants from different emitting periods to the average hourly O3 concentration over the GBA in different cases.
Regarding the average hourly O3 concentration over the GBA region in different episode periods, it can be found that, during the typhoon episodes (i.e., Ep1, Ep3, and Ep4), the contribution of non-local emissions has increased. The typhoon paths were quite similar in the Ep1 and Ep3 episodes (Fig. S3). Results show that the total contribution of GDo and the neighboring provinces has increased and reached more than 50 % for O3 over the GBA in these two typhoon episodes. As shown in Fig. S4, with the approaching of the typhoon, the wind speed increased, and the average wind direction over the GBA changed from south to north. Therefore, more pollutants from the surrounding provinces were transported to the GBA. Considering the typical circulation patterns of the typhoon periphery (Figs. S4 and S6), it is inferred that more pollutants may come from the Jiangxi, Fujian, and Hunan provinces. During the Ep1 and Ep3 episodes, the contribution of local emissions in different subregions slightly decreased. With the change in the wind direction from south to north in these two periods, the influence of pollutants within the GBA to O3 in the GF area decreased from 15 % to 8 %. The contribution of the GBA emissions to the O3 in other subregions increased, especially the ZZJ area and HK. This is because of the change in the wind direction and because these two regions are located in the downwind area of the GF and SD regions, which are the emission hotspots within the GBA. At the same time, the contribution of sources from the ocean and other countries and regions also decreased by approximately 10 %. The contribution of emissions from the GDo and the neighboring provinces to the O3 concentration in the GF, SD, and ZZJ regions and HK increased by 27 %, 21 %, 32 %, and 22 %, respectively.
In another typhoon process (Ep4), where the typhoon's moving path differed from the other two typhoon cases, an increase in the contribution from GDo and the neighboring provinces was observed due to the persistent northerly winds. Furthermore, it was observed that pollutants from eastern China (EC) and the North China Plain (NCP) could also influence the O3 levels in the GBA, accounting for approximately 12 %. Similar increases in terms of the impact of emissions from the EC and NCP were also found in the four subregions.
In Ep2, the GBA was mainly controlled by the subtropical high-pressure system with a prevailing southerly wind. However, the low wind speed was conducive to the accumulation of the pollutants. Hence, the local sources were the dominant contributors and accounted for about 44 %, while the contribution from GDo and the neighboring provinces decreased. For O3 in the GF region, as discussed above, the O3 in the GF region is more susceptible to emissions within the GBA under the prevailing southerly wind. Thus, not only the local contribution but also the GBA regional contribution largely increased in the GF region. The regional contribution is larger in the GF region, increasing from 15 % to 33 %. On the other hand, the main increase in other subregions was seen in the local contributions.
3.2 Emission period contributions
The contribution of pollutants emitted from different time periods to the average hourly O3 concentration in the GBA and its subregions is shown in Fig. 4 and Table S3. The background contribution was not considered in the temporal source contribution analysis. This is because the background contribution is primarily derived from boundary conditions, and its temporal contribution is calculated based on the time when the pollutants are transported into D1 instead of than the actual emission time.
Overall, under the general monthly condition, the emissions within 3 d (namely from Day-0 to Day-2) account for approximately 73 % of the monthly average O3 concentration within the GBA. The largest proportion of O3, around 31 %, was formed from the current day's emission (Day-0) and the contribution of pollutants from earlier emission periods decreased as time elapsed. For the monthly average O3 in different subregions, more O3 in the GF and SD regions was formed from the emission from Day-0, which contributed about 37 % and 36 %, respectively. The contribution of emissions from Day-1 decreased to about 23 % in these two regions. The contribution of Day-0 and Day-1 emissions was relatively small but stable for HK and the ZZJ region, which accounted for around 25 % and 27 %, respectively. The influence of pollutants emitted earlier than 3 d previously (i.e., Day-4) was generally lower than 20 %.
The situations are different during the pollution periods. The contribution of emissions from the current days to the average hourly O3 over the GBA decreased in the two typhoon cases with similar moving paths (Ep1 and Ep3). However, the contribution of emissions from Day-1 to Day-3 increased by 14 % and 8 %, respectively. And the influence of pollutants emitted earlier than 3 d previously (Day-4) decreased by 11 % in Ep1 and remained almost unchanged in Ep3. These findings indicate that these two ozone pollution events were caused by the accumulation of pollutants within the current 3 d.
For another typhoon case (Ep4), the contribution from Day-0 decreased approximately by 11 %, compared to the monthly contribution over the GBA. At the same time, the influence of pollutants from earlier emitting periods increased, especially for those emitted earlier than 3 d previously. It means that the O3 pollution during this period was a persistent pollution process. The major contributor should involve not only local emissions but also long-range transport. Similar trends in the temporal contribution variations were observed in different subregions, which also illustrated that O3 pollution is usually a regional problem.
For Ep2, the contribution of emissions from Day-0 increased by approximately 18 %, while the influence of emissions from Day-1 to Day-3 decreased by about 18 %. According to the source area contribution result, the source area of O3 over GBA in Ep2 is mainly local sources. Therefore, the contribution of freshly emitted pollutants was larger. The contribution of Day-4 emissions to HK and the ZZJ region in Ep2 was larger. This is probably because of the prevailing southerly wind direction which brought more airflow from the ocean. Compared with the emissions of the GF and SD regions, HK and the ZZJ region have lower emission amounts. At the same time, HK and the ZZJ region were located in the upwind region, and the pollutants from GBA would have a smaller influence on the O3 in these two regions. Hence, the number of fresh pollutants was smaller and contributed similarly to Day-4 emissions, which is an accumulated amount.
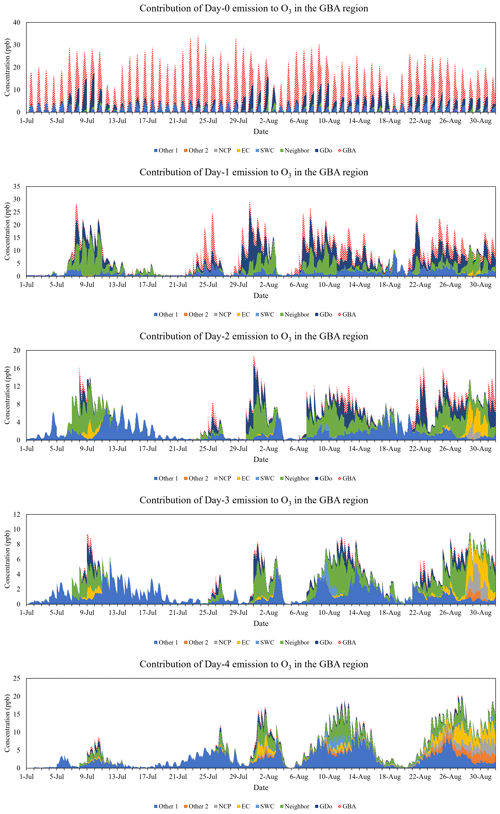
Figure 5Time series of contributions from different source areas and emitting periods to the O3 concentrations in the GBA. GDo represents areas outside the GBA region but within Guangdong province. Neighbor represents the provinces around Guangdong province. Other 1 represents the ocean and other countries and regions. Other 2 represents other areas within mainland China in the simulation domain.
3.3 Source area–time contributions
To further clarify the relationship between sources and the O3 concentration in target regions, the evolution of O3 from various source areas and periods was analyzed. Figure 5 shows the time series of the contributions from different source areas and precursor emission periods to the hourly average O3 concentration in the GBA region.
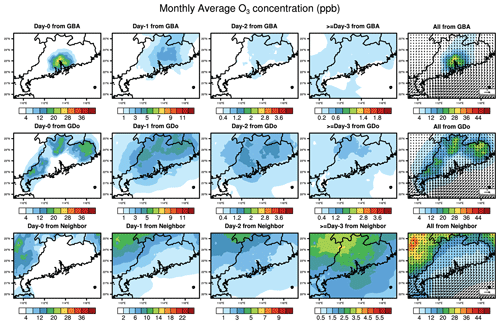
Figure 6Spatial distribution of monthly average O3 concentration between 09:00–17:00 LT (local time) contributed by emission of GBA, other regions within Guangdong province (GDo), and the neighboring provinces (Neighbor) from various periods (units in ppb). Due to the large variation in the contribution, the color bar range of each subfigure is different.
Regarding the monthly average O3 concentration over the GBA, the emission within the GBA was the major contributor and generally had a larger effect on the current day. Under the control of southerly wind, as shown in Fig. 6, the pollutants emitted 1 d previously (Day-1) were gradually transported out of the GBA, and the influence of the GBA's emission earlier than Day-1 diminished. Simultaneously, the pollutants of GDo and the neighboring provinces emitted 1 d previously began to have an impact on the O3 in the GBA. However, due to the prevailing southerly wind, the impact of aged pollutants from GDo and the neighboring provinces on the O3 in the GBA was relatively low.
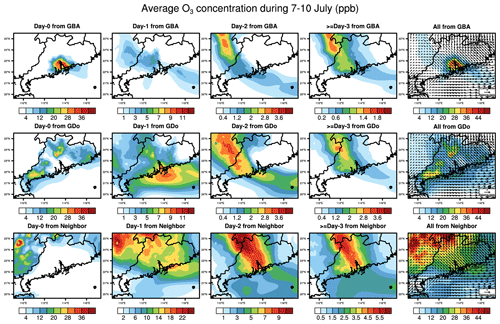
Figure 7Same as Fig. 6 but for the period of 7–10 July 2016.
However, regarding the O3 pollution between 7 and 10 July (Ep1), the major contributors changed. On 7 July, the GBA was under the control of the subtropical high-pressure system, and the typhoon was located near the east of Taiwan. The weather condition was unfavorable for pollutant dispersion, and the O3 sourced from the Day-1 emission within Guangdong province was trapped. The prevailing wind shifted to a northerly wind, bringing older pollutants from neighboring provinces to the GBA. With the approach of the typhoon from 8–10 July, the stronger northwesterly wind sped up the diffusion of pollutants, and the GBA local contribution decreased. However, it also transported more older pollutants from the northern inland region to the GBA. It can be found that the emissions from GDo on the present day also had a significant contribution. At the same time, the pollutants from the neighboring provinces dominated the emissions from Day-1 to Day-3. Moreover, the pollutants emitted 2 d previously in the EC were also transported southward and affected the O3 in the GBA on the current day. Figure 7 shows the spatial distribution of the average source contribution during the Ep1 period. Compared with the monthly average (Fig. 6), it was found that the older pollutants originating from the GBA can be transported back and influence the O3 concentration in the western part of the GBA during the Ep1 period. This is because easterly winds blew over the GBA from 5–6 July (before Ep1; Fig. S4). The pollutants emitted within the GBA were transported to the northwest inland region. However, under the influence of the northwesterly wind, they were transported back to the GBA again. It can also be seen that the pollutants from the GDo 1 d previously were transported downwind quickly, contributing to a high O3 concentration over the Pearl River estuary. According to the wind pattern, they mainly came from the northern and western parts of Guangdong province. Meanwhile, the neighboring provinces' emissions from Day-1 to Day-3 were also transported to the GBA by the northwesterly wind, thus continuously affecting the O3 over this region.
For the Ep3 O3 pollution process, results show that the pollutants from GDo and the neighboring provinces were also major contributors. From 30–31 July, the GBA was under the control of a high-pressure system, and weak northerly wind prevailed in this region. Afterward, the approaching of the typhoon (1 August) further strengthened the cross-regional transport of pollutants. The difference between Ep3 and Ep1 is that the emissions from GDo have a larger proportion in the Day-1 and Day-2 emissions. Additionally, while pollutants from neighboring provinces and EC in Day-4 emission only accounted for about 5 ppb in Ep1, they can still contribute to about 10 ppb in Ep3. The possible reason is that the northerly wind prevailed over the Fujian, Jiangxi, and Hunan provinces during the whole Ep1 period (Fig. S4). However, an easterly wind still blew over these provinces during the earlier period of Ep3 (30–31 July; Fig. S6), which slowed the transport and influence of pollutants from the neighboring provinces. Generally, the pathways of typhoons in the Ep1 and Ep3 episodes were quite similar, and the influence regions of typhoon wind field mainly covered Guangdong and the neighboring provinces. Therefore, the major source area and source time were quite similar in these two cases. To prevent this type of O3 pollution, earlier emission control (at least 3 d previously) and collaboration with neighboring provinces will result in a better control result.
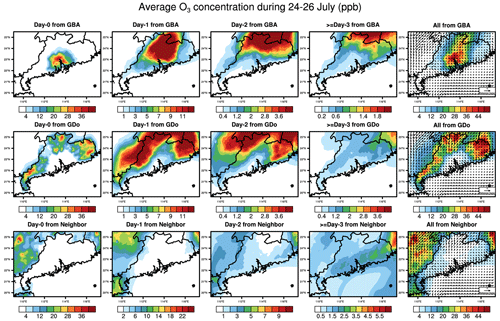
Figure 8Same as Fig. 6 but for the period of 24–26 July 2016.
On the other hand, the situation is different for the Ep2 ozone pollution. Under the control of the high-pressure system and weak southerly wind (Fig. S5), the major contributors were mainly the pollutants from the GBA and the ocean. Unlike Ep1 and Ep3, the pollutant emitted within the GBA was still dominant in the contribution of Day-1's emission. Under the influence of the southerly wind, there was a minimal migration of pollutants from the north inland regions to the GBA, and the local pollutants were gradually dispersing from the GBA. Thus, the pollutant emitted earlier than 2 d previously (≥ Day-2) had a smaller contribution. As shown in Fig. 8, the overall diffusion of pollutants within the Guangdong province was much slower during Ep2. The contribution of the GBA emissions can still reach more than 10 ppb in the Day-1 emission. These results indicate that this pollution process was mainly driven by the local pollutants within the current 2 d. Hence, emission control should focus on the local sources, and 1–2 d in advance is more efficient.
For the last O3 pollution process (Ep4), which occurred from 21 to 25 August, eastern and southern China were mainly controlled by the subtropical high-pressure system. Meanwhile, under the joint influence of the peripheral subsidence airflow of the typhoon, the wind speed over this region was slow (Fig. S7). The weak wind not only trapped the O3 formed from local emissions but also the O3 formed from cross-regional transported pollutants. The pollutants from the GBA sources mainly dominated the contribution of Day-0 and Day-1 emissions, while Day-2 and Day-3 emissions mainly consisted of pollutants from GDo and the neighboring provinces. Subsequently, as the typhoon moved northward, the stronger northerly wind further broadened the source areas of the O3 in the GBA (Fig. S7). The major contributor of the Day-2 emissions and the earlier periods' emissions changed to pollutants from the EC and NCP regions. The pollutants emitted earlier than 2 d previously from the EC had an important contribution which accounted for about 12 %. Furthermore, the pollutants emitted 3 d previously from the NCP can also have a noticeable impact on O3 over the GBA from 28–30 August, which can be up to 10 %. Therefore, to prevent the occurrence of this pollution, emission control measures should be implemented in a broader region and continuously enforced, as this pollution episode lasted longer compared to the other three cases.
Figure S8 shows the time series of the contributions from different source areas and precursor emission periods to the hourly average O3 concentration in the GF region and HK. The GF region is located at the inland of the GBA. It is the emission hotspot of the GBA with a higher O3 concentration (W. Chen et al., 2022). HK is located at the mouth of the PRD. According to previous source apportionment studies (Li et al., 2012, 2013), the pollution in HK is more attributed to the emissions outside the GBA compared to the other cities of the GBA. Regarding the O3 in the GF region, the Day-0 emission was usually contributed by both local emissions and regional transport within the GBA, with similar contributions. The major source areas of the Day-2 to Day-4 emissions contributing to O3 in the GF in different episodic cases varied in a manner similar to those contributing to the hourly average O3 in the GBA. Generally, the influence of local and GBA regional pollutants on O3 in the GF region diminished rapidly within 1 d. However, the regional emission can still have an important contribution to the episodic case with southerly winds, such as on 24–25 July (about 26 %) in Ep2 and 23–25 August (about 15 %) in Ep4. For the O3 in HK, the local emission amount is low, and its impact was also limited to the current day. In addition, the O3 in HK was also susceptible to the impact of pollutants from the ocean but less so from the GBA regional emissions. During Ep1 periods, it was observed that the contribution of the GBA regional sources largely increased in the Day-0 emission as the prevailing wind direction shifted to the north. On the other hand, the neighboring provinces' emissions dominated the contributions of emissions from Day-1 to Day-3. Unlike the GF region, the influence of EC emissions on the O3 in HK was also limited in Ep1. Similar conclusions can be drawn for the evolution of the spatiotemporal contribution of emissions in Ep3. As discussed above, the O3 pollution in Ep2 was mainly driven by local emissions. Thus, the O3 concentration in HK, located in the upwind region with fewer local emissions, was much lower than the O3 concentration in the GF region. In Ep4, the same as the GBA average and GF region, the impact of pollutants from EC and the NCP became important in the Day-2 and Day-3 emissions, which can contribute up to 20 % of O3. These results indicate that although O3 is usually considered a regional pollution problem, it is necessary to consider the local characteristics of different subregions when making more specific prevention and control policies.
3.4 Verification of the TSA by comparing to zero-out experiments
Here, the emission zero-out sensitivity experiments, another commonly used method for source apportionment, were also conducted to evaluate the results from the TSA method. The zero-out method needs to conduct two sets of simulations, including the control run and the zero-out run. In the control run, the simulations were conducted using the complete emissions. In the zero-out runs, the simulations were conducted with incomplete emissions; i.e., emissions were set to zero for specific time periods and areas. Subsequently, the contribution of the specific source area and source time was derived by calculating the difference between the control and zero-out simulations. For each target date, three types of emission area controls were implemented: type 1 involved the GBA region alone (GBA), type 2 included the emission within Guangdong province (GD; namely GBA + GDo), and type 3 expanded to encompass the emission within Guangdong province and the neighboring provinces (GD_Neighbor; namely GBA + GDo + Neighbor). The emission control period was set as being under continuous control beginning from the current day, which is also the target day (Day-0), from 1 d previously (Day-1), from 2 d previously (Day-2), and from 3 d previously (Day-3), respectively. The zero-out experiments were carried out for the periods between 7 and 10 July (typhoon case) and between 24 and 26 July (subtropical high case). More configurations can be found in Tables S4 and S5.
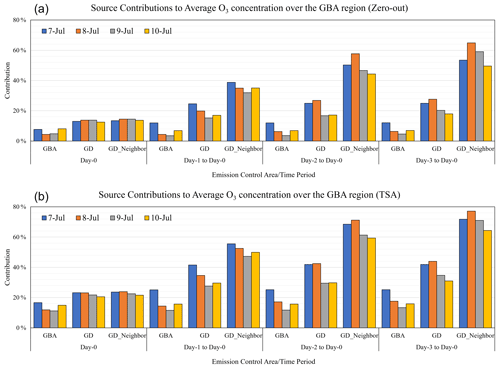
Figure 9The contribution of different source areas and time periods to the O3 concentration over the GBA in the typhoon case using the zero-out and TSA methods. Different colors represent different target dates. (a) Zero-out method. (b) TSA method.
From the results of zero-out experiments (Figs. 9 and S9), it can be seen that, for the typhoon case (Fig. 9), when only controlling the emission within the GBA, there is little difference between the results of controlling emissions 1 d and 3 d in advance. This is consistent with the TSA result that the influence of the emission within the GBA is usually limited to 2 d. Controlling emissions 1 d in advance in GD yields better results compared to solely controlling emissions within the GBA. There is less variation in the O3 concentration when controlling the emissions within GD 2 or 3 d in advance. Meanwhile, regarding only controlling emissions on Day-0, there is limited improvement in controlling the emission for a larger area (GD and GD_Neighbor) than solely within the GBA. This result aligns with the TSA result that the pollutants from neighboring provinces took effect on the O3 over the GBA region at least 1 d later. Joint control from Guangdong and the neighboring provinces has a better optimal effect in the simulations conducted from Day-2 to Day-0 and Day-3 to Day-0. The difference between GD_Neighbor and the GD result is more pronounced in these simulations, indicating that it is more effective to implement joint control with other provinces 2–3 d in advance.
For the subtropical high case (Fig. S9), whatever is controlling the emissions on the current day or 2 d ahead, the effect of solely controlling emissions within the GBA is similar to that of the joint control in a larger area (GD and GD_Neighbor). It supports our previous conclusion that the pollution is mainly contributed by the local sources. Additionally, there is a limited optimization effect when controlling the emission 2–3 d in advance compared to controlling 1 d in advance. To alleviate this ozone pollution, controlling the local emission in the short term should be effective. Although the contribution discrepancies between the source contribution (%) calculated from the zero-out method and those obtained from the TSA method can reach 20 %, which is due to the non-linear chemistry relationship between ozone and its precursors, as well as the differences in the methodology (Kwok et al., 2015; Clappier et al., 2017), similar relationships between source area/time and receptor can be drawn. These results also support the validity of the TSA approach.
3.5 Discussion
Previous studies mainly focused on exploring the contribution and control of various source areas and categories on O3 over the GBA. The analysis in this study illustrated that there could be a larger difference between the temporal contribution of emissions to the O3 pollution over the GBA under different weather patterns. This finding emphasizes the importance of understanding the contribution of pollutants from different emission periods and identifying the major periods, particularly in episodic cases, for effective policymaking in pollution control. In contrast to the zero-out method, which requires multiple simulations, our approach provides a comprehensive overview of source contributions within a single simulation. This method is suitable for applications involving more potential sources as it saves on computation costs.
In addition, meteorological conditions play an important role in affecting the effectiveness of the emission control area and period. The results here suggest that the approach of typhoons usually strengthens the cross-region transportation of pollutants to the GBA. Therefore, cross-province collaboration and control should be implemented at least 2–3 d ahead when the typhoon is predicted. The information obtained from the TSA results can contribute to the establishment of an early-warning and rapid-response system. It could help to facilitate collaboration, considering estimated timelines and the cost implications associated with emission reduction efforts, with the aim to achieve a balanced outcome across regions. In contrast, local emission control within 2 d is more effective when the GBA is under the influence of a high-pressure system. The primary focus for emission control measures should be on local vehicles and industries, as they are the major contributors of NOx and VOCs (Bian et al., 2019; Li et al., 2019). Implementing measures such as traffic restrictions based on even- and odd-numbered license plates and a temporary reduction in emissions from industries can be effective strategies to target these sources in advance. Our findings emphasize the importance of considering the impact of meteorological conditions when implementing control measures in advance. Here, our study primarily focuses on the summer season, which has been identified as the O3 pollution period in the GBA (Gao et al., 2018; T. Li et al., 2022). Typhoons and subtropical high-pressure systems are two significant weather patterns closely linked with O3 pollution events in southern China (Wang et al., 2017; Ouyang et al., 2022). The trajectories of typhoons in Ep1 and Ep3 (Fig. S3) are similar to one of the typical typhoon pathways often coinciding with O3 pollution events in the GBA (Qu et al., 2021; N. Wang et al., 2022). Meanwhile, the high 2 m temperature and low 2 m relative humidity over the GBA can be observed during the O3 episodes (Figs. S10 and S11). The prevailing wind across the GBA in the typhoon and subtropical high-pressure cases is northerly and southerly, respectively (Fig. S12). Overall, the weather conditions observed in the selected cases of this study are similar to those reported in other O3 pollution studies in this region (Qu et al., 2021; Ouyang et al., 2022; N. Wang et al., 2022). Nevertheless, it is crucial to underscore that the spatiotemporal source contribution may vary in O3 pollution – even under similar meteorological conditions. For instance, the change in the typhoon position and intensity could influence the large-scale circulation and precursor emissions (Zhan et al., 2020; N. Wang et al., 2022). Therefore, it is imperative to undertake further investigations and comparative studies on more similar O3 events over the GBA under the influence of typhoons and subtropical high-pressures in the future, which will contribute to attaining more widely applicable findings and offer valuable insights for developing emission control strategies. Additionally, the spatiotemporal influence of emissions to O3 over the GBA under other unfavorable conditions and seasons also needs to be further explored through the TSA method, which will help us to gain a more comprehensive understanding of the spatiotemporal sources of O3 over the GBA.
In the context of climate change, the occurrence of extreme weather, such as extreme heat waves (Coffel et al., 2018; Dong et al.,2023), is expected to become more frequent. These events will significantly impact the sources and sinks of pollutants through various physical and chemical processes. At the same time, governments in different countries will implement various emission control strategies in response to climate change, such as carbon neutrality (Liu et al., 2021; Zhang and Hanaoka, 2021), which will also alter the emission structure. How these extreme weather events and control measures influence the temporal characterization of sources, the formation of air pollution, and the spatiotemporal contribution of emissions from different countries, as well as their interactions, is also worth further investigation in the future. Such investigations can foster mutual cooperation among nations to collectively address environmental challenges.
However, it should be noted that the numerical model source apportionment results are usually influenced by the uncertainties in the emission inventory as most of the emission inventories are constructed with a bottom-up method and cannot be updated in a timely manner. With the increasing availability of different types of observations, including surface monitoring and satellite remote sensing data, different top-down methods such as data assimilation (East et al., 2022) and machine learning (Chen et al., 2023) have been applied to integrate observations and optimize the emissions. These methods should be implemented to update the emission inventory. Meanwhile, the air quality model results are also sensitive to the uncertainty in the weather forecast, potentially leading to variations in source apportionment results. To alleviate the impact of weather forecast uncertainty, different methods, such as ensemble simulation (Gilliam et al., 2015), data assimilation for the meteorological field simulation (Kwon et al., 2018), and the machine-learning method (Scher and Messori, 2018; Cho et al., 2020), should be applied to enhance the accuracy of meteorological field simulations.
In this study, we applied the CAMx–TSA method to analyze the spatiotemporal contribution of different sources to the O3 pollution in the GBA during summer. The result shows that the O3 over the GBA in summer is mainly contributed by the pollutants from local emissions, followed by pollutants originating from other regions within Guangdong province and the neighboring provinces. The O3 formation is predominantly attributed to pollutants emitted within a 3 d period, accounting for over 70 % of the total contribution. During the O3 episodes, when the typhoon moved from the Philippine Sea towards southern China, the prevailing wind shifted from southerly to northerly over the GBA. This facilitated the transport of pollutants from GDo and the neighboring provinces to the GBA, resulting in an increase in O3 concentrations. The pollutants emitted 3 d previously still have a significant contribution. When the typhoon remained located at the ocean of northeastern Philippines and moved northward, under the continuous influence of the northerly wind, the emissions from eastern China and even the North China Plain from 3 d previously can also have a noticeable impact on O3 over the GBA. In contrast, when the GBA was mainly under the control of the subtropical high-pressure system, the ozone pollution was mainly caused by the local pollutants within the current 2 d. The results indicated that implementing joint emission control measures with other provinces 2–3 d in advance is more effective for preventing the O3 pollution in the GBA when the typhoon is approaching southern China. On the other hand, it is more efficient to pay more attention to local source control within 2 d when the GBA is under the control of the high-pressure system.
Here, different surrounding provinces were categorized as one source area to save on computation resources for more potential source investigation. As the neighboring province was illustrated as a major contributor to the O3 in the GBA, it is necessary to further divide this source into several subsource areas and explore their individual impact in future work. Meanwhile, our preliminary findings indicate that pollutants emitted more than 3 d prior can still have a considerable impact on the O3 levels in the GBA. As a result, it would be valuable to conduct source apportionment analyses with finer source areas and earlier source periods for O3 pollution in different cities within the GBA. This further investigation would provide deeper insights into the unique O3 pollution characteristics of each city. In addition, individual source categories were not separated in this study, mainly due to the application of different emission inventories with different source category classifications, making it difficult to combine them. It is important to note that each source category has its own characteristic temporal profile, which can have different temporal impacts on O3 concentrations. Therefore, the temporal contribution of various source categories, including anthropogenic and biogenic emissions, should be also considered in future work. These future works can provide more spatiotemporal information about the O3 source over the GBA, enabling local governments to design and implement more targeted control measures more effectively and promptly.
Hourly O3 observation data were released by the China National Environmental Monitoring Centre, available at https://quotsoft.net/air/ (CNEMC, 2023), and the Hong Kong Environmental Protection Department, available at https://cd.epic.epd.gov.hk/EPICDI/air/station/?lang=en (HKEPD, 2023). The CAMx model code is freely available at https://www.camx.com/download/, last access: 24 December 2023 (CAMx, 2024).
The supplement related to this article is available online at: https://doi.org/10.5194/acp-24-8847-2024-supplement.
CY, LX, and JCHF designed the research. CY contributed to model development, simulation, and data analysis. LX and JCHF contributed to the resulting discussion. CY prepared the paper with contributions from all co-authors.
The contact author has declared that none of the authors has any competing interests.
Publisher's note: Copernicus Publications remains neutral with regard to jurisdictional claims made in the text, published maps, institutional affiliations, or any other geographical representation in this paper. While Copernicus Publications makes every effort to include appropriate place names, the final responsibility lies with the authors.
This work has been supported by the National Natural Science Foundation of China Grant (grant no. 42007203), the Research Grants Council of Hong Kong (grant no. C7041-21G), the Improvement on Competitiveness in Hiring New Faculties Funding Scheme of the Chinese University of Hong Kong (CUHK) (grant no. 4937115), the National Key Research and Development Program of China (project no. 2023YFC3709200), and the Guangzhou Scientific and Technological Planning Project (grant no. 2024A04J4128). We are grateful for the constructive comments from the anonymous reviewers and editor.
This research has been supported by the National Natural Science Foundation of China (grant no. 42007203), the Research Grants Council of Hong Kong (grant no. C7041-21G), the Improvement on Competitiveness in Hiring New Faculties Funding Scheme of the Chinese University of Hong Kong (CUHK) (grant no. 4937115), the National Key Research and Development Program of China (project no. 2023YFC3709200), and the Guangzhou Scientific and Technological Planning Project (grant no. 2024A04J4128).
This paper was edited by Stefano Galmarini and reviewed by two anonymous referees.
Bian, Y., Huang, Z., Ou, J., Zhong, Z., Xu, Y., Zhang, Z., Xiao, X., Ye, X., Wu, Y., Yin, X., Li, C., Chen, L., Shao, M., and Zheng, J.: Evolution of anthropogenic air pollutant emissions in Guangdong Province, China, from 2006 to 2015, Atmos. Chem. Phys., 19, 11701–11719, https://doi.org/10.5194/acp-19-11701-2019, 2019.
CAMx: Comprehensive Air Quality Model with Extensions, Ramboll Environ US Corporation [code], https://www.camx.com/download/, last access: 24 December 2023.
Cao, M., Fan, S., Jin, C., Cai, Q., and He, Y.: O3 pollution characteristics, weather classifications and local meteorological conditions in Guangdong from 2015 to 2020, Acta Scientiae Circumstantiae, 43, 19–31, https://doi.org/10.13671/j.hjkxxb.2022.0416, 2023 (in Chinese).
Cao, T., Wang, H., Li, L., Lu, X., Liu, Y., and Fan, S.: Fast spreading of surface ozone in both temporal and spatial scale in Pearl River Delta, J. Environ. Sci., 137, 540–552, https://doi.org/10.1016/j.jes.2023.02.025, 2024.
Chen, W., Chen, Y., Chu, Y., Zhang, J., Xian, C., Lin, C., Fung, Z., and Lu, X.: Numerical simulation of ozone source characteristics in the Pearl River Delta region, Acta Scientiae Circumstantiae, 42, 293–308, https://doi.org/10.13671/j.hjkxxb.2021.0328, 2022 (in Chinese).
Chen, X., Wang, N., Wang, G., Wang, Z., Chen, H., Cheng, C., Li, M., Zheng, L., Wu, L., Zhang, Q., Tang, M., Huang, B., Wang, X., and Zhou, Z.: The Influence of Synoptic Weather Patterns on Spatiotemporal Characteristics of Ozone Pollution Across Pearl River Delta of Southern China, J. Geophys. Res.-Atmos., 127, e2022JD037121, https://doi.org/10.1029/2022jd037121, 2022.
Chen, Y., Fung, J. C. H., Huang, Y., Lu, X., Wang, Z., Louie, P. K. K., Chen, W., Yu, C. W., Yu, R., and Lau, A. K. H.: Temporal Source Apportionment of PM2.5 Over the Pearl River Delta Region in Southern China, J. Geophys. Res.-Atmos., 127, e2021JD035271, https://doi.org/10.1029/2021jd035271, 2022.
Chen, Y., Fung, J. C. H., Yuan, D., Chen, W., Fung, T., and Lu, X.: Development of an integrated machine-learning and data assimilation framework for NOx emission inversion, Sci. Total Environ., 871, 161951, https://doi.org/10.1016/j.scitotenv.2023.161951, 2023.
Cho, D., Yoo, C., Im, J., and Cha, D. H.: Comparative Assessment of Various Machine Learning-Based Bias Correction Methods for Numerical Weather Prediction Model Forecasts of Extreme Air Temperatures in Urban Areas, Earth Space Sci., 7, e2019EA000740, https://doi.org/10.1029/2019ea000740, 2020.
Clappier, A., Belis, C. A., Pernigotti, D., and Thunis, P.: Source apportionment and sensitivity analysis: two methodologies with two different purposes, Geosci. Model Dev., 10, 4245–4256, https://doi.org/10.5194/gmd-10-4245-2017, 2017.
CNEMC: Real-time National Air Quality, China National Environmental Monitoring Centre [data set], https://quotsoft.net/air/, last access: 24 December 2023.
Coffel, E. D., Horton, R. M., and de Sherbinin, A.: Temperature and humidity based projections of a rapid rise in global heat stress exposure during the 21st century, Environ. Res. Lett., 13, 014001, https://doi.org/10.1088/1748-9326/aaa00e, 2018.
Deng, T., Wang, T., Wang, S., Zou, Y., Yin, C., Li, F., Liu, L., Wang, N., Song, L., Wu, C., and Wu, D.: Impact of typhoon periphery on high ozone and high aerosol pollution in the Pearl River Delta region, Sci. Total Environ., 668, 617–630, https://doi.org/10.1016/j.scitotenv.2019.02.450, 2019.
Dong, W., Jia, X., Qian, Q., and Li, X.: Rapid Acceleration of Dangerous Compound Heatwaves and Their Impacts in a Warmer China, Geophys. Res. Lett., 50, e2023GL104850, https://doi.org/10.1029/2023gl104850, 2023.
East, J. D., Henderson, B. H., Napelenok, S. L., Koplitz, S. N., Sarwar, G., Gilliam, R., Lenzen, A., Tong, D. Q., Pierce, R. B., and Garcia-Menendez, F.: Inferring and evaluating satellite-based constraints on NOx emissions estimates in air quality simulations, Atmos. Chem. Phys., 22, 15981–16001, https://doi.org/10.5194/acp-22-15981-2022, 2022.
Emery, C. and Tai, E.: Enhanced Meteorological Modeling and Performance Evaluation for Two Texas Ozone Episodes, Texas Natural Resource Conservation Commission, ENVIRON International Corp, https://api.semanticscholar.org/CorpusID:127579774 (last access: 31 July 2024), 2001.
ERA5: ECMWF Reanalysis v5 data, ERA5 [data set], https://www.ecmwf.int/en/forecasts/dataset/ecmwf-reanalysis-v5 (last access: 17 May 2024), 2024.
Fang, T., Zhu, Y., Wang, S., Xing, J., Zhao, B., Fan, S., Li, M., Yang, W., Chen, Y., and Huang, R.: Source impact and contribution analysis of ambient ozone using multi-modeling approaches over the Pearl River Delta region, China, Environ. Pollut., 289, 117860, https://doi.org/10.1016/j.envpol.2021.117860, 2021.
Feng, X., Guo, J., Wang, Z., Gu, D., Ho, K.-F., Chen, Y., Liao, K., Cheung, V. T. F., Louie, P. K. K., Leung, K. K. M., Yu, J. Z., Fung, J. C. H., and Lau, A. K. H.: Investigation of the multi-year trend of surface ozone and ozone-precursor relationship in Hong Kong, Atmos. Environ., 315, 120139, https://doi.org/10.1016/j.atmosenv.2023.120139, 2023.
Gao, X., Deng, X., Tan, H., Wang, C., Wang, N., and Yue, D.: Characteristics and analysis on regional pollution process and circulation weather types over Guangdong Province, Acta Scientiae Circumstantiae, 38, 1708–1716, https://doi.org/10.13671/j.hjkxxb.2017.0473, 2018 (in Chinese).
Gilliam, R. C., Hogrefe, C., Godowitch, J. M., Napelenok, S., Mathur, R., and Rao, S. T.: Impact of inherent meteorology uncertainty on air quality model predictions, J. Geophys. Res.-Atmos., 120, 12259–12280, https://doi.org/10.1002/2015jd023674, 2015.
Gong, C., Liao, H., Yue, X., Ma, Y., and Lei, Y.: Impacts of Ozone-Vegetation Interactions on Ozone Pollution Episodes in North China and the Yangtze River Delta, Geophys. Res. Lett., 48, e2021GL093814, https://doi.org/10.1029/2021gl093814, 2021.
Gong, S., Zhang, L., Liu, C., Lu, S., Pan, W., and Zhang, Y.: Multi-scale analysis of the impacts of meteorology and emissions on PM2.5 and O3 trends at various regions in China from 2013 to 2020 2. Key weather elements and emissions, Sci. Total Environ., 824, 153847, https://doi.org/10.1016/j.scitotenv.2022.153847, 2022.
Han, H., Liu, J., Shu, L., Wang, T., and Yuan, H.: Local and synoptic meteorological influences on daily variability in summertime surface ozone in eastern China, Atmos. Chem. Phys., 20, 203–222, https://doi.org/10.5194/acp-20-203-2020, 2020.
He, Z., Wang, X., Ling, Z., Zhao, J., Guo, H., Shao, M., and Wang, Z.: Contributions of different anthropogenic volatile organic compound sources to ozone formation at a receptor site in the Pearl River Delta region and its policy implications, Atmos. Chem. Phys., 19, 8801–8816, https://doi.org/10.5194/acp-19-8801-2019, 2019.
HKEPD: Hong Kong Air Quality Data, Hong Kong Environmental Protection Department [data set], https://cd.epic.epd.gov.hk/EPICDI/air/station/?lang=en, last access: 24 December 2023.
Kwok, R. H. F., Baker, K. R., Napelenok, S. L., and Tonnesen, G. S.: Photochemical grid model implementation and application of VOC, NOx, and O3 source apportionment, Geosci. Model Dev., 8, 99–114, https://doi.org/10.5194/gmd-8-99-2015, 2015.
Kwon, I.-H., English, S., Bell, W., Potthast, R., Collard, A., and Ruston, B.: Assessment of Progress and Status of Data Assimilation in Numerical Weather Prediction, B. Am. Meteorol. Soc., 99, ES75–ES79, https://doi.org/10.1175/bams-d-17-0266.1, 2018.
Li, M., Liu, H., Geng, G., Hong, C., Liu, F., Song, Y., Tong, D., Zheng, B., Cui, H., Man, H., Zhang, Q., and He, K.: Anthropogenic emission inventories in China: a review, Natl. Sci. Rev., 4, 834–866, https://doi.org/10.1093/nsr/nwx150, 2017.
Li, M., Zhang, Q., Zheng, B., Tong, D., Lei, Y., Liu, F., Hong, C., Kang, S., Yan, L., Zhang, Y., Bo, Y., Su, H., Cheng, Y., and He, K.: Persistent growth of anthropogenic non-methane volatile organic compound (NMVOC) emissions in China during 1990–2017: drivers, speciation and ozone formation potential, Atmos. Chem. Phys., 19, 8897–8913, https://doi.org/10.5194/acp-19-8897-2019, 2019.
Li, T., Chen, J., Weng, J., Shen, J., and Gong, Y.: Ozone pollution synoptic patterns and their variation characteristics in Guangdong Province, China Environmental Science, 42, 2015–2024, https://doi.org/10.19674/j.cnki.issn1000-6923.2022.0102, 2022 (in Chinese).
Li, Y., Lau, A. K. H., Fung, J. C. H., Zheng, J. Y., Zhong, L. J., and Louie, P. K. K.: Ozone source apportionment (OSAT) to differentiate local regional and super-regional source contributions in the Pearl River Delta region, China, J. Geophys. Res.-Atmos., 117, 1–18, https://doi.org/10.1029/2011jd017340, 2012.
Li, Y., Lau, A. K. H., Fung, J. C. H., Ma, H., and Tse, Y.: Systematic evaluation of ozone control policies using an Ozone Source Apportionment method, Atmos. Environ., 76, 136–146, https://doi.org/10.1016/j.atmosenv.2013.02.033, 2013.
Li, Y., Zhao, X., Deng, X., and Gao, J.: The impact of peripheral circulation characteristics of typhoon on sustained ozone episodes over the Pearl River Delta region, China, Atmos. Chem. Phys., 22, 3861–3873, https://doi.org/10.5194/acp-22-3861-2022, 2022.
Lin, X., Yuan, Z., Yang, L., Luo, H., and Li, W.: Impact of Extreme Meteorological Events on Ozone in the Pearl River Delta, China, Aerosol Air Qual. Res., 19, 1307–1324, https://doi.org/10.4209/aaqr.2019.01.0027, 2019.
Liu, H., Zhang, M., and Han, X.: A review of surface ozone source apportionment in China, Atmospheric and Oceanic Science Letters, 13, 470–484, https://doi.org/10.1080/16742834.2020.1768025, 2020.
Liu, Y. and Wang, T.: Worsening urban ozone pollution in China from 2013 to 2017 – Part 1: The complex and varying roles of meteorology, Atmos. Chem. Phys., 20, 6305–6321, https://doi.org/10.5194/acp-20-6305-2020, 2020.
Liu, Y., Geng, G., Cheng, J., Liu, Y., Xiao, Q., Liu, L., Shi, Q., Tong, D., He, K., and Zhang, Q.: Drivers of Increasing Ozone during the Two Phases of Clean Air Actions in China 2013–2020, Environ. Sci. Technol., 57, 8954–8964, https://doi.org/10.1021/acs.est.3c00054, 2023.
Liu, Z., Deng, Z., He, G., Wang, H., Zhang, X., Lin, J., Qi, Y., and Liang, X.: Challenges and opportunities for carbon neutrality in China, Nature Reviews Earth & Environment, 3, 141–155, https://doi.org/10.1038/s43017-021-00244-x, 2021.
Lu, X., Yao, T., Li, Y., Fung, J. C. H., and Lau, A. K. H.: Source apportionment and health effect of NOx over the Pearl River Delta region in southern China, Environ. Pollut., 212, 135–146, https://doi.org/10.1016/j.envpol.2016.01.056, 2016.
Lu, X., Zhang, L., and Shen, L.: Meteorology and Climate Influences on Tropospheric Ozone: a Review of Natural Sources, Chemistry, and Transport Patterns, Current Pollution Reports, 5, 238–260, https://doi.org/10.1007/s40726-019-00118-3, 2019.
Maji, K. J., Ye, W.-F., Arora, M., and Nagendra, S. M. S.: Ozone pollution in Chinese cities: Assessment of seasonal variation, health effects and economic burden, Environ. Pollut., 247, 792–801, https://doi.org/10.1016/j.envpol.2019.01.049, 2019.
Ouyang, S., Deng, T., Liu, R., Chen, J., He, G., Leung, J. C.-H., Wang, N., and Liu, S. C.: Impact of a subtropical high and a typhoon on a severe ozone pollution episode in the Pearl River Delta, China, Atmos. Chem. Phys., 22, 10751–10767, https://doi.org/10.5194/acp-22-10751-2022, 2022.
Qu, K., Wang, X., Yan, Y., Shen, J., Xiao, T., Dong, H., Zeng, L., and Zhang, Y.: A comparative study to reveal the influence of typhoons on the transport, production and accumulation of O3 in the Pearl River Delta, China, Atmos. Chem. Phys., 21, 11593–11612, https://doi.org/10.5194/acp-21-11593-2021, 2021.
Sahu, S. K., Liu, S., Liu, S., Ding, D., and Xing, J.: Ozone pollution in China: Background and transboundary contributions to ozone concentration & related health effects across the country, Sci. Total Environ., 761, https://doi.org/10.1016/j.scitotenv.2020.144131, 2021.
Scher, S. and Messori, G.: Predicting weather forecast uncertainty with machine learning, Q. J. Roy. Meteor. Soc., 144, 2830–2841, https://doi.org/10.1002/qj.3410, 2018.
US EPA: Guidance on the Use of Models and Other Analyses for Demonstrating Attainment of Air Quality Goals for Ozone, PM2.5, and Regional Haze, Office of Air Quality Planning and Standards, Research Triangle Park, North Carolina, USA, EPA-454/B-07-002, 262 pp., 2007.
Wang, N., Huang, X., Xu, J., Wang, T., Tan, Z.-m., and Ding, A.: Typhoon-boosted biogenic emission aggravates cross-regional ozone pollution in China, Science Advances, 8, eabl6166, https://doi.org/10.1126/sciadv.abl6166, 2022.
Wang, T., Xue, L., Brimblecombe, P., Lam, Y. F., Li, L., and Zhang, L.: Ozone pollution in China: A review of concentrations, meteorological influences, chemical precursors, and effects, Sci. Total Environ., 575, 1582–1596, https://doi.org/10.1016/j.scitotenv.2016.10.081, 2017.
Wang, T., Xue, L., Feng, Z., Dai, J., Zhang, Y., and Tan, Y.: Ground-level ozone pollution in China: a synthesis of recent findings on influencing factors and impacts, Environ. Res. Lett., 17, 063003, https://doi.org/10.1088/1748-9326/ac69fe, 2022.
Wang, Y., Wild, O., Ashworth, K., Chen, X., Wu, Q., Qi, Y., and Wang, Z.: Reductions in crop yields across China from elevated ozone, Environ. Pollut., 292, 118218, https://doi.org/10.1016/j.envpol.2021.118218, 2022.
Wang, W., Parrish, D. D., Wang, S., Bao, F., Ni, R., Li, X., Yang, S., Wang, H., Cheng, Y., and Su, H.: Long-term trend of ozone pollution in China during 2014–2020: distinct seasonal and spatial characteristics and ozone sensitivity, Atmos. Chem. Phys., 22, 8935–8949, https://doi.org/10.5194/acp-22-8935-2022, 2022.
Wu, Y., Chen, W., You, Y., Xie, Q., Jia, S., and Wang, X.: Quantitative impacts of vertical transport on the long-term trend of nocturnal ozone increase over the Pearl River Delta region during 2006–2019, Atmos. Chem. Phys., 23, 453–469, https://doi.org/10.5194/acp-23-453-2023, 2023.
Xie, X., Shi, Z., Ying, Q., Zhang, H., and Hu, J.: Age-Resolved Source and Region Contributions to Fine Particulate Matter During an Extreme Haze Episode in China, Geophys. Res. Lett., 48, e2021GL095388, https://doi.org/10.1029/2021gl095388, 2021.
Xie, X., Hu, J., Qin, M., Guo, S., Hu, M., Ji, D., Wang, H., Lou, S., Huang, C., Liu, C., Zhang, H., Ying, Q., Liao, H., and Zhang, Y.: Evolution of atmospheric age of particles and its implications for the formation of a severe haze event in eastern China, Atmos. Chem. Phys., 23, 10563–10578, https://doi.org/10.5194/acp-23-10563-2023, 2023.
Xu, J., Zhao, Z., Wu, Y., Zhang, Y., Wang, Y., Su, B., Liang, Y., Hu, T., and Liu, R.: Impacts of Meteorological Conditions on Autumn Surface Ozone During 2014–2020 in the Pearl River Delta, China, Earth Space Sci., 10, e2022EA002742, https://doi.org/10.1029/2022ea002742, 2023.
Xu, Y., Shen, A., Jin, Y., Liu, Y., Lu, X., Fan, S., Hong, Y., and Fan, Q.: A quantitative assessment and process analysis of the contribution from meteorological conditions in an O3 pollution episode in Guangzhou, China, Atmos. Environ., 303, 119757, https://doi.org/10.1016/j.atmosenv.2023.119757, 2023.
Yang, J. and Zhao, Y.: Performance and application of air quality models on ozone simulation in China – A review, Atmos. Environ., 293, 119446, https://doi.org/10.1016/j.atmosenv.2022.119446, 2023.
Yang, L., Luo, H., Yuan, Z., Zheng, J., Huang, Z., Li, C., Lin, X., Louie, P. K. K., Chen, D., and Bian, Y.: Quantitative impacts of meteorology and precursor emission changes on the long-term trend of ambient ozone over the Pearl River Delta, China, and implications for ozone control strategy, Atmos. Chem. Phys., 19, 12901–12916, https://doi.org/10.5194/acp-19-12901-2019, 2019.
Yang, W., Chen, H., Wang, W., Wu, J., Li, J., Wang, Z., Zheng, J., and Chen, D.: Modeling study of ozone source apportionment over the Pearl River Delta in 2015, Environ. Pollut., 253, 393–402, https://doi.org/10.1016/j.envpol.2019.06.091, 2019.
Yin, P., Chen, R., Wang, L., Meng, X., Liu, C., Niu, Y., Lin, Z., Liu, Y., Liu, J., Qi, J., You, J., Zhou, M., and Kan, H.: Ambient Ozone Pollution and Daily Mortality: A Nationwide Study in 272 Chinese Cities, Environ. Health Persp., 125, https://doi.org/10.1289/ehp1849, 2017.
Ying, Q., Zhang, J., Zhang, H., Hu, J., and Kleeman, M. J.: Atmospheric Age Distribution of Primary and Secondary Inorganic Aerosols in a Polluted Atmosphere, Environ. Sci. Technol., 55, 5668–5676, https://doi.org/10.1021/acs.est.0c07334, 2021.
Zeren, Y., Zhou, B., Zheng, Y., Jiang, F., Lyu, X., Xue, L., Wang, H., Liu, X., and Guo, H.: Does Ozone Pollution Share the Same Formation Mechanisms in the Bay Areas of China?, Environ. Sci. Technol., 56, 14326–14337, https://doi.org/10.1021/acs.est.2c05126, 2022.
Zhan, C., Xie, M., Huang, C., Liu, J., Wang, T., Xu, M., Ma, C., Yu, J., Jiao, Y., Li, M., Li, S., Zhuang, B., Zhao, M., and Nie, D.: Ozone affected by a succession of four landfall typhoons in the Yangtze River Delta, China: major processes and health impacts, Atmos. Chem. Phys., 20, 13781–13799, https://doi.org/10.5194/acp-20-13781-2020, 2020.
Zhang, R. and Hanaoka, T.: Deployment of electric vehicles in China to meet the carbon neutral target by 2060: Provincial disparities in energy systems, CO2 emissions, and cost effectiveness, Resour. Conserv. Recy., 170, 105622, https://doi.org/10.1016/j.resconrec.2021.105622, 2021..
Zheng, H., Kong, S., He, Y., Song, C., Cheng, Y., Yao, L., Chen, N., and Zhu, B.: Enhanced ozone pollution in the summer of 2022 in China: The roles of meteorology and emission variations, Atmos. Environ., 301, 119701, https://doi.org/10.1016/j.atmosenv.2023.119701, 2023.