the Creative Commons Attribution 4.0 License.
the Creative Commons Attribution 4.0 License.
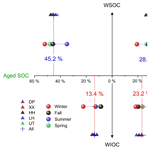
Characterizing water solubility of fresh and aged secondary organic aerosol in PM2.5 with the stable carbon isotope technique
Fenghua Wei
Xing Peng
Liming Cao
Mengxue Tang
Ning Feng
Xiaofeng Huang
Lingyan He
The investigation of the water-soluble characteristics of secondary organic carbon (SOC) is essential for a more comprehensive understanding of its climate effects. However, due to the limitations of the existing source apportionment methods, the water solubility of different types of SOC remains uncertain. This study analyzed stable carbon isotope and mass spectral signatures of total carbon (TC) and water-soluble organic carbon (WSOC) in ambient PM2.5 samples for 1 year and established stable carbon isotope profiles of fresh and aged SOC. Furthermore, a Bayesian stable isotope mixing (BSIM) model was employed to reveal the water solubility characteristics of fresh and aged SOC in a coastal megacity of China. WSOC was dominated by secondary sources, with fresh and aged SOC contributing 28.1 % and 45.2 %, respectively. Water-insoluble organic carbon (WIOC) was dominated by primary sources, to which fresh and aged SOC contributed 23.2 % and 13.4 %. We also found that the aging degree of SOC has considerable impacts on its water solubility due to the much higher water-soluble fraction of aged SOC (76.5 %) compared to fresh SOC (54.2 %). Findings of this study may provide a new perspective for further investigation of the hygroscopicity effects of SOC with different aging degrees on light extinction and climate change.
- Article
(3940 KB) - Full-text XML
-
Supplement
(971 KB) - BibTeX
- EndNote
As a major component of particulate matter (PM2.5), secondary organic aerosols (SOAs) not only contribute to haze formation but also exert a substantial influence on climate dynamics across various spatial scales, from local to global (Kaul et al., 2011; Shrivastava et al., 2017). Water solubility, considered one of the crucial physical properties of SOAs, has been extensively studied recently due to its significant effects on physicochemical processes in the atmosphere. The water solubility of SOAs varies with its aging degrees (Kirillova et al., 2013), while both the water solubility and the aging degree of organic aerosols contribute noticeably to hygroscopicity, which ultimately affects light extinction (Han et al., 2022; Liu et al., 2022). Hence, exploring the water solubility characteristics of SOAs with different aging degrees can help in elucidating the more detailed extinction mechanism of SOAs. In addition, recent studies have also shown that the formation of secondary particulates is one of the main processes determining the quantity of cloud condensation nuclei (CCN) in remote oceanic regions (Liu and Matsui, 2022). Therefore, investigating the water solubility of SOAs with different aging degrees is also meaningful for further exploring their indirect climate effects.
Investigating the contributions of SOAs with different aging degrees to both organic matter (OM) and water-soluble organic matter (WSOM) is imperative for determining quantified SOA water solubility. However, due to the constraints of reliable methods, only a limited number of studies have examined the water solubility of SOAs using mass spectrometry techniques. Qiu et al. (2019) conducted source apportionment of OM in PM1 and WSOM in PM2.5 based on online and offline AMS-PMF (aerosol mass spectrometry–positive matrix factorization) methods, respectively (Qiu et al., 2019). This approach faces challenges related to not only the inherent errors in online versus offline methods but also discrepancies in the measured particle sizes of OM and WSOM. Kondo et al. (2007) and Timonen et al. (2013) attempted to apportion water-soluble organic carbon (WSOC) through a multiple linear regression method based on the mass spectral information of OM, which still exhibits large indeterminateness (Timonen et al., 2013; Xiao et al., 2011; Kondo et al., 2007). The carbon isotopic technique offers a promising avenue for overcoming the aforementioned limitations, thereby enabling a more in-depth exploration of the water-soluble characteristics of SOAs. Carbon isotope techniques have garnered widespread attention and are increasingly employed in source apportionment studies of organic aerosols due to their robust source appointment capabilities. Radioactive carbon isotopes (14C) provide a precise method for quantitatively distinguishing between fossil and non-fossil organic aerosol sources (Fushimi et al., 2011; Zhang et al., 2014). The stable carbon isotope technique (13C), however, can quantitatively assess the contributions of various sources by integrating them into mass balance models (Yao et al., 2022; Widory et al., 2004). Bayesian mixing models stand out as one of the most widely utilized model types (Xiao et al., 2011; Xu and Xiao 2023; Tang et al., 2020). The stable carbon isotope technique can also be combined with other source tracers to further enhance the accuracy of the source apportionment of carbonaceous aerosols (Jiang et al., 2022; Plasencia Sánchez et al., 2023; Ceburnis et al., 2011; Lim et al., 2022). However, to our knowledge, no study has employed the carbon isotope technique to estimate the source contribution of both fresh and aged SOAs before, owing to the challenging measurement of the carbon isotope profiles for these two sources.
Previous studies have predominantly concentrated on assessing the water solubility of SOAs at inland urban sites, revealing a strong correlation between SOA water solubility and urban air pollution emissions as well as relative humidity (Wong et al., 2015; Zhou and Abbatt 2015; Pye et al., 2017; Favez et al., 2008; Salma et al., 2007; Weber et al., 2007; Miyazaki et al., 2006). Nevertheless, few researchers have addressed the differences between inland and coastal cities. As dynamic interfaces between urban and marine environments (Donaldson and George, 2012), coastal cities exhibit unique characteristics. Shenzhen is a typical representative city for coastal air pollution studies with a coastline spanning 260.5 km and a total sea area of 1145 m2. We measured the stable carbon isotope fingerprints of fresh and aged secondary organic carbon (SOC), which enabled us to investigate the source contributions of SOC with different aging degrees to WSOC and their respective water solubility levels in Shenzhen.
The aim of this study is to investigate the water solubility of SOC in PM2.5, with emphasis on Shenzhen as a representative coastal mega city in China. We analyzed stable carbon isotopes and mass spectral signatures of total carbon (TC) and WSOC in ambient PM2.5 samples, collected from five distinct sites in Shenzhen over 1 year, as well as specific emission sources. For the first time, we employed a Bayesian stable isotope mixing (BSIM) model and applied it to localized source profiles to quantify the contributions of fresh SOC and aged SOC to WSOC and water-insoluble organic carbon (WIOC). These results contribute to estimating the water solubility of both fresh and aged SOC, revealing direct or indirect implications for climate change.
2.1 Ambient PM2.5 sampling and chemical analysis
Shenzhen (22°27′–22°52′ N, 113°46′–114°37′ E), a megacity of the Pearl River Delta, China, is bordered by Daya Bay and Dapeng Bay to the east, the Pearl River estuary and Lingdingyang Estuary to the west, Hong Kong SAR to the south, and Dongguan and Huizhou to the north. As a typical coastal megacity in China, Shenzhen's air quality is predominantly affected by the continental air mass from northern Guangdong, the eastern coastal air mass, and the southern marine air mass (Fig. 1). For a comprehensive exploration of pollution characteristics in Shenzhen, PM2.5 samples were collected from five sites covering the western to eastern regions of the city. The selected sites are Xixiang (XX, urban site), University Town (UT, urban site), Longhua (LH, urban site), Honghu (HH, urban site), and Dapeng (DP, background site) (Fig. 1). Additional details about each sampling site are listed in Table S1 in the Supplement.
In this study, 24 h PM2.5 sampling was conducted every other day in 2019 at the UT site using a Thermo 2300 atmospheric particulate sampler (Thermo Fisher Scientific Inc., Waltham, Massachusetts, USA), yielding a total of 160 valid samples. For the remaining four sites, a total of 295 valid PM2.5 samples were collected every other day during typical months of the four seasons in 2019 (March, June, September, and December; Table S2 in the Supplement) using a model Th-16a atmospheric particulate sampler (Tianhong Corp., Wuhan, China). The PM2.5 samples collected by the quartz filter were used to determine the organic carbon (OC) and elemental carbon (EC) using an OC–EC analyzer (2001A, Desert Research Institute, Reno, Nevada, USA) following the IMPROVE-A procedure. In addition, the samples collected by Teflon filter in this study were analyzed for water-soluble ions (mainly , , , and Cl−) within PM2.5, and the mass concentrations of 23 metallic elements (primarily Na, Mg, Al, K, Ca, V, Fe, Ni, Zn, Pb, and Cd) within PM2.5 were also determined using an inductively coupled plasma mass spectrometer (ICP-MS, Aurora M90; Bruker, Germany). Relevant quality control information is described in the Supplement (Sect. S1).
For WSOC extraction, the PM2.5 sample underwent ultrasonication (20 min × 3) in 15 mL ultrapure water (18.2 MΩ cm), followed by filtration through a syringe with a 0.45 µm filter head to eliminate insoluble particles. The extracted PM2.5 samples were sequentially analyzed using a long-time-of-flight aerosol mass spectrometer (L-TOF-AMS, Aerodyne, USA) and an ultrasonic nebulizer (U5000AT+, CETAC Technologies Inc., USA) to measure elemental ratios, such as , as well as the mass spectrum signatures of the water-soluble organic fractions, including ion fragments like , , and . The concentration of WSOC was determined using a total organic carbon analyzer (multi 3100, Jena, Germany), and WIOC was calculated as the difference between OC and WSOC.
To investigate the stable carbon isotope signatures of carbonaceous aerosols, we built a stable isotope spectrometry system by integrating an OC–EC analyzer with a carbon dioxide isotope spectrometer (QCLAS, Aerodyne). This system reduces the carbon requirement for isotope analysis from 5 to 0.5 µg C and improves the accuracy of spectroscopic measurement methods to 0.2 ‰–0.3 ‰. The stable carbon isotope values of TC and WSOC in ambient PM2.5 were measured in this study.
2.2 Bayesian stable isotope mixing model
The BSIM model could quantify the contributions of multiple sources to the TC and WSOC based on the principle of mass conservation of stable isotopes, in which the Markov chain Monte Carlo (MCMC) method was employed. The methodology employed in the BSIM model was detailed in works by Parnell et al. (2010, 2013). In brief, the posterior distribution for the Bayesian neural network (BNN) was calculated utilizing the prior distribution and likelihood function based on Bayes' theorem. Implementation of the BSIM model in this study utilized the “simmr” package in R software (https://cran.r-project.org/web/packages/simmr/index.html, last access: 2 November 2023). Gelman diagnostic values, ranging from 1 to 1.01, all met the criteria of the posterior prediction test, indicating robust model performance and reliable results. Additionally, an uncertainty index (UI90) was employed here to further characterize the uncertainty strength of TC and WSOC source apportionments based on their posterior distribution. This index refers to the difference between the proportional contributions of the maximum and minimum values in the rapid-increase segment divided by 90 with a 90 % cumulative probability () (Zaryab et al., 2022; Ji et al., 2017).
2.3 Stable carbon isotope spectrum of PM2.5 sources
The BSIM model requires the input of potential sources for carbonaceous aerosols, along with their local source-specific stable carbon isotope values (fingerprints). In this study, we firstly employed the PMF model to identify the potential sources of TC and WSOC (Sect. S1), with the aim of reducing the uncertainty in the subsequent BSIM model and verifying the reliability of the BSIM results. The PMF results showed that traffic emissions, SOAs, and biomass burning are the major contributors to carbonaceous aerosols in Shenzhen, which is similar to previous results for Guangzhou (Huang et al., 2014). In addition, a literature review indicated that secondary conversion sources could be further subdivided into fresh SOC for the low-oxidation state and aged SOC for the high-oxidation state (Chen et al., 2019; Presto et al., 2009; Mahrt et al., 2022; Shen et al., 2017). Ultimately, traffic emissions, fresh SOC, aged SOC, and biomass burning (BB) were identified as the four potential sources of TC and WSOC for the BSIM model in this study. Since the PMF model lacks the mass spectral information of offline PM2.5 samples, it fails to distinguish between fresh SOC and aged SOC in TC, making it challenging to investigate the water solubility characteristics of the SOC based on PMF results. The BSIM model simultaneously quantified fresh and aged SOC separately in both TC and WSOC, thereby enabling an estimation of SOC water solubility. This capability is used for the final analysis in this study.
Recognizing the regional variability in stable carbon isotope fingerprints of PM2.5 sources (Yao et al., 2022), this work obtained representative and locally specific carbon isotope profiles for the four sources in Shenzhen. The measured profiles of the four sources were used as prior information in the BSIM model for the follow-up analyses. For the traffic emissions, we measured the stable carbon isotope values of TC and WSOC in PM2.5 that were collected from Tanglang Mountain Tunnel (dominated by diesel vehicles) and Jiuweiling Tunnel (dominated by gasoline vehicles) in Shenzhen. Fresh SOC was simulated through gasoline vehicle bench tests. The lowest stable carbon isotope values for TC and WSOC from the simulated samples were chosen as the fresh-SOC results. The oxygen-carbon ratios () of fresh-SOC samples in this study ranged from 0.51 to 0.62, indicating a low-oxidation state (Ding et al., 2012). Aged-SOC samples were obtained by collecting ambient PM2.5 samples at the National Ambient Air Background Monitoring Station (Mount Wuzhi site, Hainan, China), primarily influenced by regional pollution transported by northern continental air masses. These aged-SOC samples exhibited a high value of 0.98, suggesting their highly oxidized state (Zhu et al., 2016). Biomass burning emissions were simulated and analyzed by burning pine wood in the Laboratory of Biomass Burning Simulation at Peking University Shenzhen Graduate School (He et al., 2010). Additional details about the sampling process are available in the Supplement (Sect. S2). Table 1 summarizes the stable carbon isotope fingerprints of the four sources and f60 signatures used in this study. Table S3 in the Supplement compares source signatures in this study with global datasets. The stable carbon isotope measurements from the four sources align with the range observed in global datasets, thus affirming the reliability of the four source fingerprints utilized in this study. Previous research identified ( 60) as a reliable marker for biomass burning in Shenzhen, with a feature value of 1.61 % ± 0.68 % (Cao et al., 2018). This prior information was also incorporated into the BSIM model to estimate the biomass burning source. Although there is some overlap among the δ13C fingerprints of different sources, the Bayesian approach allows for probabilistic estimation of the contribution of different sources and can also integrate information from multiple markers and sources to mitigate the effects of overlap. In this study, the PMF model was used to reduce the uncertainty in interference from unrelated sources, and the chemical tracer marker of biomass burning source (f60) was also integrated to minimize the effect of this overlap.
2.4 Contributions of SOC to WIOC
Based on the source apportionment results from the BSIM model for TC and WSOC, the contributions of fresh SOC and aged SOC to WIOC were calculated according to Eqs. (1)–(2). The uncertainties (u) in concentrations of Fresh SOC(WIOC) and Aged SOC(WIOC) were assessed using the uncertainty transfer equations (Eqs. 3–4). Fresh-SOC and aged-SOC uncertainties in both TC (14.9 %, 30.1 %) and WSOC (24.1 %, 20.9 %) were determined using the BSIM model. Our findings reveal that the calculated uncertainties in [Fresh SOC(WIOC)] and [Aged SOC(WIOC)] were 28.3 % and 36.8 %, respectively.
3.1 Overview of PM2.5 and carbonaceous components
The annual mean concentration of PM2.5 in Shenzhen was 24.9 µg m−3 in 2019, with TC being the predominant component and exhibiting an annual mean concentration of 7.1 µg m−3 (5.8 and 1.3 µg m−3 for OC and EC, respectively). WSOC accounts for 48 % of OC, presenting an annual mean concentration of 2.8 µg m−3. The mean stable carbon isotope values for TC () and WSOC () were −26.64 ‰ ± 0.79 ‰ and −25.80 ‰ ± 0.88 ‰, respectively, which is lower than the results of northern cities in China (Wu et al., 2020). This can be attributed to the limited impact of coal combustion (which has high 13C values) on PM2.5 in Shenzhen (Yao et al., 2022; Vodicka et al., 2022).
Seasonal variation revealed that TC, OC, WSOC, and EC exhibited elevated levels in winter and decreased levels in summer (Fig. 2a). This pattern primarily stems from pollution air masses originating from continental regions in the fall and winter and clean air masses from the Southern Ocean during the summer months (Fig. S1 in the Supplement). The OC-to-EC ratio, averaging 4.5, was also higher in winter than in summer, consistent with the oxygen-to-carbon () ratio results for WSOC (Fig. 2a), indicating a large influence of aged SOC on carbonaceous aerosols in winter. The stable carbon isotope results support this observation. Figure 2b depicts relatively high and values in spring (−26.59 ‰, −25.26 ‰), fall (−26.38 ‰, −25.44 ‰), and winter (−26.46 ‰, −26.27 ‰). These higher values are attributed to greater contributions of aged SOC from northern and northeastern regional transport processes during these seasons (Fig. S1). In summer, observed low and values of −27.29 ‰ and −26.57 ‰, respectively, suggest relatively high contributions of fresh SOC to PM2.5. Shenzhen experiences high temperatures in summer, leading to increased gaseous precursor emissions from terrestrial biogenic sources, especially C3 plants. Intense solar radiation and high temperature favor photochemical reactions to generate fresh SOC that depletes 13C in particulate matter during summer (Kirillova et al., 2013).
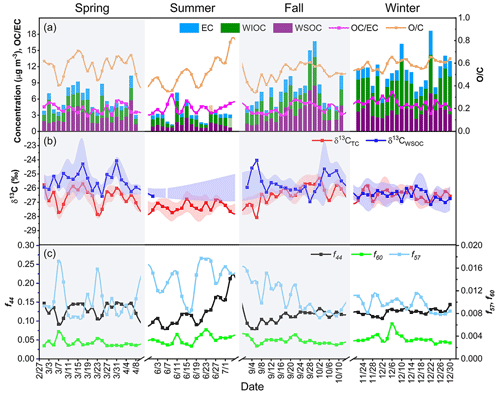
Figure 2Time series of carbonaceous components (a), stable carbon isotope characteristics of TC and WSOC (b), and mass spectral signatures of WSOC in PM2.5 (c) from Shenzhen. Each data point was averaged from five sampling sites. (Note that summer samples exhibit elevated analytical errors due to low concentrations and that values are computed from combined summer samples.) The date is given in the format month/day.
Mass spectral characteristics of ( 44), ( 57), and ( 60) in WSOC were measured to represent oxidized organic aerosol (OOA), hydrocarbon-like organic aerosol (HOA), and biomass burning organic aerosol (BBOA), respectively. The abundance of these ion fragments, denoted as f44, f57, and f60, is determined by the ratios of signal intensities at 44, 57, and 60 to the sum of signal intensities from all signals in the organic mass spectra. As depicted in Fig. 2c, f44 obtained higher values in spring (0.131) and winter (0.125) compared to summer (0.120) and fall (0.112), further indicating an elevated oxidation level of OOA during spring and winter. Considering that f60 exceeds 0.0030 when biomass burning influences carbonaceous aerosol (Docherty et al., 2008; DeCarlo et al., 2008), the annual average value of f60 was 0.0032, suggesting biomass burning was an important source of carbon components in Shenzhen. Winter exhibited higher levels of f60 (0.0035) compared to other seasons, suggesting relatively strong impacts of biomass burning on WSOC in winter. Conversely, f57 reached its highest level in summer (0.014) and its lowest in winter (0.009), with an annual average value of 0.011, possibly associated with a notable increase in hydrocarbon organic aerosol emissions from traffic and biogenic sources during the summer period.
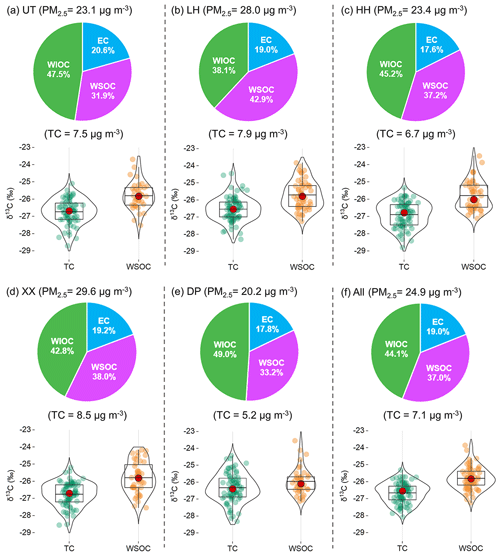
Figure 3Chemical compositions of EC, WIOC, and WSOC in TC at urban sites (a–d), at the background site (e), and averaged from all five sites (f). The violin and box-and-whisker plots below each pie chart display spatial variations in and at each site, featuring mean values (black lines) and median values (red dots).
Obvious spatial variations in PM2.5 mass concentrations across Shenzhen during 2019 were observed, with the XX site registering the highest concentration (29.6 µg m−3), followed by LH (28.0 µg m−3), HH (23.4 µg m−3), UT (23.1 µg m−3), and DP (20.2 µg m−3). Figure 3 illustrates that TC made more substantial contributions (28.2 %–32.5 %) to PM2.5 at the four urban sites in the central and western regions of Shenzhen compared to the background site (DP, 25.7 %). This suggests that local pollutant emissions significantly influence carbonaceous aerosols in Shenzhen's urban areas. The percentage of WSOC in TC was also higher in urban areas (37.5 % ± 3.9 %) compared to the background area (DP, 33.2 %), reaching the highest value at the LH site (42.9 %). However, the percentage of WIOC in TC displayed the opposite trend, suggesting carbonaceous aerosols in urban areas of Shenzhen exhibit higher water solubility than in background areas. Distinct spatial distribution characteristics were also observed in the stable carbon isotopes of TC and WSOC. The background site exhibits higher values (−26.33 ‰) than the four urban sites (−26.72 ‰ ± 0.13 ‰). This difference may be attributed to the increased contribution of traffic or fresh-SOC sources to carbonaceous aerosols at urban sites and the relatively high contribution of aged SOC at the background site. Atmospheric aging processes of organics through photochemical reactions can deplete 13C in aged SOC and enrich 13C in fresh SOC and other related reactants simultaneously (Pavuluri and Kawamura, 2017), and the close proximity of the values at urban sites (−25.77 ‰ ± 0.04 ‰) to the background site (DP, −25.96 ‰) suggests that the WSOC in different areas of Shenzhen may share a similar origin.
3.2 Source apportionment results for TC and WSOC
The BSIM model assessed the contributions of traffic, fresh SOC, aged SOC, and biomass burning (BB) to TC and WSOC, as shown in Fig. 4. On average, SOC (total of fresh and aged SOC) and traffic emerged as the two major contributors to TC, accounting for 43 % and 40 %, respectively, while biomass burning contributed 17 % to TC. The contribution of aged SOC to TC (23 %) is comparable with fresh SOC (20 %). Regarding WSOC, SOC was the dominant source, comprising 45 % of aged SOC and 28 % of fresh SOC, followed by BB (18 %) and traffic (9 %). The noteworthy contribution of aged SOC to WSOC suggests a comparatively high water solubility of aged SOC in Shenzhen.
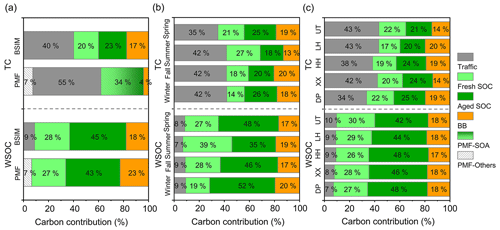
Figure 4(a) Comparison of source apportionment results between the BSIM model and PMF model for TC and WSOC and (b) seasonal and (c) spatial distributions of source apportionment results for TC and WSOC based on the BSIM model.
To evaluate the BSIM model's performance, we employed the PMF model to apportion the sources of TC and WSOC. The obtained results were subsequently compared with those from the BSIM model, as depicted in Fig. 4a. A total of 17 chemical species of PM2.5 were applied as the PMF model input to estimate source contributions to TC, encompassing carbon components, soluble inorganic ions, and elements. For the apportionment of WSOC sources, five species including WSOC, WIOC, and three organic mass spectra were applied as the PMF model input. More details about the PMF model and results can be found in the Supplement (Sect. S1, Figs. S2–S5). PMF identified the traffic as the predominant contributor to TC (55 %), followed by SOC (34 %) and biomass burning (4 %). Concerning WSOC, aged SOC and fresh SOC were the two major sources as well, accounting for 43 % and 27 %, respectively. The traffic contribution to TC apportioned by the PMF model is higher than that of the BSIM model (55 % vs. 40 %), which may be due to the fact that some of the fresh SOC generated by the conversion of primary vehicle emissions was improperly apportioned to the traffic source in the PMF model (Li et al., 2022; Zhao et al., 2014). A previous study also showed that SOAs contribute more to carbonaceous aerosols in Shenzhen than the traffic source (Cao et al., 2022). The PMF model results for WSOC were generally consistent with BSIM model results, with deviations primarily attributed to the differences in the principles of and uncertainties in the two models.
Furthermore, this study examined cumulative frequency distributions to elucidate the inherent uncertainty in source apportionments of TC and WSOC. As shown in Fig. 5a and b, the proportional contributions of the BB source to both TC and WSOC were quite stable during the research periods due to its low UI90 value (0.02). This may be attributed to the incorporation of mass spectral constraints for the BB source in the BSIM model used in this study. For TC source apportionment results, the largest UI90 value (0.46) was observed for the traffic source, indicating that its contribution to TC exhibited relatively high uncertainty. With 90 % probability, its contribution ranged from 19.4 % to 60.9 %. The UI90 values for fresh and aged SOC were 0.15 and 0.30, respectively. Regarding WSOC, the calculated UI90 value of traffic, fresh SOC, and aged SOC ranged from 0.18 to 0.24. The UI90 values obtained through the BSIM model remained within reasonable limits and were smaller than those calculated in previous, related studies (0.23–0.62) (Zaryab et al., 2022; Ji et al., 2017). Consequently, the source contributions of TC and WSOC estimated by the BSIM model in this study were deemed reasonable.
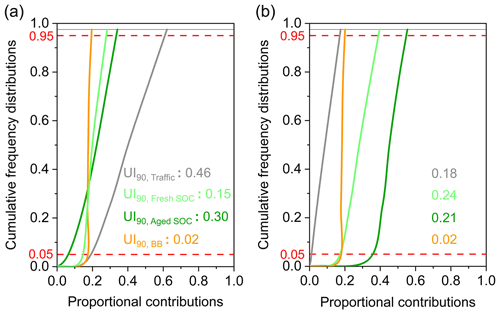
Figure 5Cumulative frequency distributions of the proportional contributions from potential sources of TC (a) and WSOC (b) based on the BSIM model.
For seasonal variations, as shown in Fig. 4b, SOC was still the major source of TC and WSOC during all four seasons, ranging from 38 % to 46 % and from 71 % to 75 %, respectively. Significant high contributions of fresh SOC to TC and WSOC occurred in summer (27 %, 39 %), and relatively high contributions of aged SOC to TC and WSOC were observed in winter (26 %, 52 %). This is because meteorological conditions in winter characterized by inversions and stagnant winds facilitate the accumulation of air pollutants, and Shenzhen is significantly influenced by regional pollution transport in winter, favoring the formation of aged SOC (Huang et al., 2018). In contrast, favorable meteorological conditions (e.g., intense and prolonged solar radiation, high temperatures, and relative humidity) in summer enhance photochemical reactions to generate fresh SOC. In terms of spatial distributions (Fig. 4c), the contributions of the traffic source to TC were higher at urban sites (38 % to 43 %) compared to the background site (34 %). This finding aligns with expectations due to increased human activity and vehicle numbers in urban locations. At the DP site, the contributions of SOC to TC were higher than those of other sources (47 %), signifying a predominant influence of regionally transported pollutant emissions on TC at the background site. However, the contributions of SOC and the other two primary sources at both urban and background sites were all close to each other, indicating the source composition of WSOC in Shenzhen is less affected by the air pollution degree compared to TC.
3.3 Water solubility of fresh SOC and aged SOC
The contributions of fresh SOC and aged SOC to WIOC were the differences between the contributions of those two SOC sources to TC and WSOC from the BSIM model (Sect. 2.4) in this study. As shown in Fig. 6a, fresh SOC and aged SOC made contributions of 23.2 % ± 4.2 % and 13.4 % ± 3.8 % to WIOC, respectively, implying that primary sources are the dominant contributors to WIOC. Further support for this finding is evident in the strong correlation between WIOC and EC, as depicted in Fig. 6b. A higher slope was observed in winter (2.4) than in other seasons, consistent with the highest contributions of aged SOC to WIOC in winter (22 %). This observation implies that WIOC in winter is influenced not only by local primary sources but also by the promotion of secondary pollution.
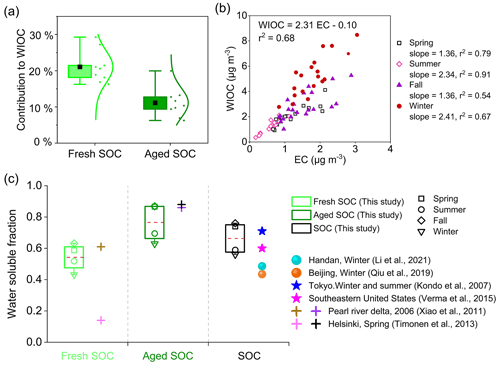
Figure 6(a) Box-and-whisker plots of fresh-SOC and aged-SOC contributions to WIOC, with the upper and lower edges of the boxes representing the 75th and 25th percentiles and the black squares representing mean values. The dots to the right of each box show the contribution of fresh and aged SOC to WIOC across seasons and sites, and the curves demonstrate its normal distribution. (b) A scatterplot of WIOC versus EC by season. (c) Comparison of the water-soluble fraction of SOC (fresh SOC, aged SOC, SOC) in this study (box-and-whisker plots) with those in other related literature (colored markings on the right). The upper and lower edges of the boxes represent the 75th and 25th percentiles, and the dashed red lines indicate mean values.
To investigate in-depth the water solubility characteristics of fresh and aged SOC, we then calculated their water-soluble fractions by comparing their water-soluble portions to the ambient fraction (), where [c]water-soluble and [c]water-insoluble are the concentrations of fresh SOC or aged SOC in WSOC and WIOC, respectively) (Li et al., 2021). As shown in Fig. 6c, the overall water-soluble fraction of SOC in this study was 66.2 %, with a range from 58.9 % to 76.0 %. Fresh SOC exhibited a much lower water solubility of 54.2 %, whereas aged SOC displayed a comparatively high water solubility of 76.5 %. The higher water solubility of aged SOC compared to fresh SOC might be due to the positive correlation between aerosol hygroscopicity and oxidation in the sub-saturated state. The water-soluble fraction of SOC in this study was close to that reported in other coastal urban areas (Tokyo (71 %) and the southeastern United States (60 %)) (Kondo et al., 2007; Verma et al., 2015), while it was much higher than that reported in northern Chinese cities (Beijing (42 %–45 %) and Handan (49 %)) (Li et al., 2021; Qiu et al., 2019). In addition, the water-soluble fraction of both fresh SOC and aged SOC, as calculated in this study, was comparable to that reported in Guangzhou (61 % and 86 % for fresh and aged SOC, respectively) (Xiao et al., 2011). This could be attributed to Shenzhen's coastal location, which is markedly influenced by regional transport from neighboring urban areas and the eastern seaboard air masses. The high relative humidity facilitates the conversion of aged SOC into WSOC during the pollution transport process. This result is in accordance with previous findings that air masses influenced by anthropogenic emissions could promote the formation of high levels of water-soluble SOAs under high relative humidity in urban environments (Miyazaki et al., 2006; Salma et al., 2007; Weber et al., 2007). Given that the aging process of SOAs dissolved in water could enhance the cloud condensation nuclei (CCN) activity of the particles (Liu and Matsui, 2022), high levels of water-soluble aged SOC in Shenzhen might have significant impacts on the activity of CCN, potentially resulting in more important indirect climate effects.
The water-soluble fraction of SOC (especially aged SOC) in Shenzhen exhibits obvious seasonal characteristics, with the highest in fall (76.0 %) and the lowest in winter (56.0 %). This phenomenon is primary related to the robust atmospheric oxidizing capacity during fall in Shenzhen, since atmospheric oxidants such as OH and NO3 radicals play pivotal roles in driving the secondary generation of WSOC (Wang et al., 2023). Conversely, during winter, the temperature and relative humidity are at their lowest levels, and the relatively diminished atmospheric oxidizing capacity also constrains the secondary generation of WSOC.
Assessing the impacts of different oxidational SOC on air quality and its water solubility has been challenging, and this work successfully evaluated the water-soluble fraction of fresh and aged SOC, employing the BSIM model and applying it to 1-year observational data for stable carbon isotopes and mass spectra of TC and WSOC in Shenzhen, China. Compared with other methods, e.g., PMF models, EC tracers, and multiple linear regression analyses, the BSIM model successfully calculated the contributions of fresh SOC and aged SOC to WSOC and WIOC, owing to prior and localized information about stable carbon isotopes and mass spectra of PM2.5 sources. Therefore, establishing localized carbonaceous aerosol source profiles for stable carbon isotopes becomes crucial for comprehending the relationship between the aging degree and water solubility of SOC.
The observed average mass concentration of PM2.5 during the sampling period in Shenzhen was 24.9 µg m−3, and WSOC accounts for 48 % of OC. The mean stable carbon isotope values for TC () and WSOC () were −26.64 ‰ ± 0.79 ‰ and −25.80 ‰ ± 0.88 ‰, respectively. WSOC was dominated by secondary sources, while WIOC was dominated by primary sources. The contribution of fresh SOC and aged SOC to WSOC (WIOC) were 28.1 % and 45.2 % (23.2 % and 13.4 %), respectively. The overall water-soluble fraction of SOC in this study was 66.2 %, with aged SOC constituting 76.5 % and fresh SOC constituting 54.2 %. The water-soluble fraction of aged SOC was 22 % higher than fresh SOC, even though both of them demonstrated remarkable water-soluble characteristics in Shenzhen. This finding highlights the important role of aged SOC in the water uptake process of particulate matter. Considering the strong correlation between the water solubility of SOC and its light extinction effect, further exploration of the extinction effect of SOC with different aging degrees will greatly contribute to a more profound understanding of the extinction mechanism of SOC. Besides, the water solubility of SOC in coastal cities was observed to be higher than that in inland cities, suggesting a more pronounced climate effect of SOC in coastal cities. Therefore, there should be increased emphasis on enhancing the control of SOA precursors in coastal urban areas to better integrate air pollution and climate change management. This is particularly crucial given the observed rise in the proportion of SOAs in particulate matter in recent years. Moreover, the results of our study further indicated that the notable water solubility of SOC, particularly aged SOC, may contribute a lot to the formation of CCN above coastal cities, a finding which is also helpful in developing a better understanding of the cloud microphysical processes and the indirect climate effect of SOC in coastal urban regions.
Datasets are available upon request to the corresponding author, Xing Peng (pengxing@pku.edu.cn).
The supplement related to this article is available online at: https://doi.org/10.5194/acp-24-8507-2024-supplement.
XP and XH conceptualized the study. FW, LC, MT, and NF retrieved and constructed the dataset. FW and XP carried out the statistical analysis. FW prepared the first draft of the manuscript, which was commented on and revised by XP, LH, and XH. All authors reviewed and approved the final version for publication.
The contact author has declared that none of the authors has any competing interests.
Publisher's note: Copernicus Publications remains neutral with regard to jurisdictional claims made in the text, published maps, institutional affiliations, or any other geographical representation in this paper. While Copernicus Publications makes every effort to include appropriate place names, the final responsibility lies with the authors.
We gratefully acknowledge the anonymous reviewers for their helpful comments that significantly improved the quality of this paper.
This research has been supported by the National Key Research and Development Program of China (grant no. 2023YFC3709203) and the Science and Technology Plan of Shenzhen Municipality (grant no. JCYJ20220818100812028).
This paper was edited by Zhibin Wang and reviewed by two anonymous referees.
Cao, L.-M., Huang, X.-F., Li, Y.-Y., Hu, M., and He, L.-Y.: Volatility measurement of atmospheric submicron aerosols in an urban atmosphere in southern China, Atmos. Chem. Phys., 18, 1729–1743, https://doi.org/10.5194/acp-18-1729-2018, 2018.
Cao, L. M., Wei, J., He, L. Y., Zeng, H., Li, M. L., Zhu, Q., Yu, G. H., and Huang, X. F.: Aqueous aging of secondary organic aerosol coating onto black carbon: Insights from simultaneous L-ToF-AMS and SP-AMS measurements at an urban site in southern China, J. Clean. Prod., 330, 129888, https://doi.org/10.1016/j.jclepro.2021.129888, 2022.
Ceburnis, D., Garbaras, A., Szidat, S., Rinaldi, M., Fahrni, S., Perron, N., Wacker, L., Leinert, S., Remeikis, V., Facchini, M. C., Prevot, A. S. H., Jennings, S. G., Ramonet, M., and O'Dowd, C. D.: Quantification of the carbonaceous matter origin in submicron marine aerosol by 13C and 14C isotope analysis, Atmos. Chem. Phys., 11, 8593–8606, https://doi.org/10.5194/acp-11-8593-2011, 2011.
Chen, T., Liu, Y., Chu, B., Liu, C., Liu, J., Ge, Y., Ma, Q., Ma, J., and He, H.: Differences of the oxidation process and secondary organic aerosol formation at low and high precursor concentrations, J. Environ. Sci.-China, 79, 256–263, 2019.
DeCarlo, P. F., Dunlea, E. J., Kimmel, J. R., Aiken, A. C., Sueper, D., Crounse, J., Wennberg, P. O., Emmons, L., Shinozuka, Y., Clarke, A., Zhou, J., Tomlinson, J., Collins, D. R., Knapp, D., Weinheimer, A. J., Montzka, D. D., Campos, T., and Jimenez, J. L.: Fast airborne aerosol size and chemistry measurements above Mexico City and Central Mexico during the MILAGRO campaign, Atmos. Chem. Phys., 8, 4027–4048, https://doi.org/10.5194/acp-8-4027-2008, 2008.
Ding, X., Wang, X. M., Gao, B., Fu, X. X., He, Q. F., Zhao, X. Y., Yu, J. Z., and Zheng, M.: Tracer-based estimation of secondary organic carbon in the Pearl River Delta, south China, J. Geophys. Res.-Atmos., 117, 1–14, 2012.
Docherty, K. S., Stone, E. A., Ulbrich, I. M., DeCarlo, P. F., Snyder, D. C., Schauer, J. J., Peltier, R. E., Weber, R. J., Murphy, S. M., Seinfeld, J. H., Grover, B. D., Eatough, D. J., and Jimenez, J. L.: Apportionment of primary and secondary organic aerosols in Southern California during the 2005 study of organic aerosols in riverside (SOAR-1), Environ. Sci. Technol., 42, 7655–7662, 2008.
Donaldson, D. J. and George, C.: Sea-surface chemistry and its impact on the marine boundary layer, Environ. Sci. Technol., 46, 10385–10389, 2012.
Favez, O., Sciare, J., Cachier, H., Alfaro, S. C., and Abdelwahab, M. M.: Significant formation of water-insoluble secondary organic aerosols in semi-arid urban environment, Geophys. Res. Lett., 35, L15801, https://doi.org/10.1029/2008GL034446, 2008.
Fushimi, A., Wagai, R., Uchida, M., Hasegawa, S., Takahashi, K., Kondo, M., Hirabayashi, M., Morino, Y., Shibata, Y., Ohara, T., Kobayashi, S., and Tanabe, K.: Radiocarbon (14C) diurnal variations in fine particles at sites downwind from Tokyo, Japan in summer, Environ. Sci. Technol., 45, 6784–6792, 2011.
Han, S., Hong, J., Luo, Q., Xu, H., Tan, H., Wang, Q., Tao, J., Zhou, Y., Peng, L., He, Y., Shi, J., Ma, N., Cheng, Y., and Su, H.: Hygroscopicity of organic compounds as a function of organic functionality, water solubility, molecular weight, and oxidation level, Atmos. Chem. Phys., 22, 3985–4004, https://doi.org/10.5194/acp-22-3985-2022, 2022.
He, L.-Y., Lin, Y., Huang, X.-F., Guo, S., Xue, L., Su, Q., Hu, M., Luan, S.-J., and Zhang, Y.-H.: Characterization of high-resolution aerosol mass spectra of primary organic aerosol emissions from Chinese cooking and biomass burning, Atmos. Chem. Phys., 10, 11535–11543, https://doi.org/10.5194/acp-10-11535-2010, 2010.
Huang, R. J., Zhang, Y., Bozzetti, C., Ho, K. F., Cao, J. J., Han, Y., Daellenbach, K. R., Slowik, J. G., Platt, S. M., Canonaco, F., Zotter, P., Wolf, R., Pieber, S. M., Bruns, E. A., Crippa, M., Ciarelli, G., Piazzalunga, A., Schwikowski, M., Abbaszade, G., Schnelle-Kreis, J., Zimmermann, R., An, Z., Szidat, S., Baltensperger, U., El Haddad, I., and Prevot, A. S.: High secondary aerosol contribution to particulate pollution during haze events in China, Nature, 514, 218–222, 2014.
Huang, X.-F., Zou, B.-B., He, L.-Y., Hu, M., Prévôt, A. S. H., and Zhang, Y.-H.: Exploration of PM2.5 sources on the regional scale in the Pearl River Delta based on ME-2 modeling, Atmos. Chem. Phys., 18, 11563–11580, https://doi.org/10.5194/acp-18-11563-2018, 2018.
Ji, X. L., Xie, R. T., Hao, Y., and Lu, J.: Quantitative identification of nitrate pollution sources and uncertainty analysis based on dual isotope approach in an agricultural watershed, Environ. Pollut., 229, 586–594, 2017.
Jiang, F., Liu, J., Cheng, Z., Ding, P., Xu, Y., Zong, Z., Zhu, S., Zhou, S., Yan, C., Zhang, Z., Zheng, J., Tian, C., Li, J., and Zhang, G.: Dual-carbon isotope constraints on source apportionment of black carbon in the megacity Guangzhou of the Pearl River Delta region, China for 2018 autumn season, Environ. Pollut., 294, 118638, https://doi.org/10.1016/j.envpol.2021.118638, 2022.
Kaul, D. S., Gupta, T., Tripathi, S. N., Tare, V., and Collett, J. L.: Secondary organic aerosol: a comparison between foggy and nonfoggy days, Environ. Sci. Technol., 45, 7307–7313, 2011.
Kirillova, E. N., Andersson, A., Sheesley, R. J., Kruså, M., Praveen, P. S., Budhavant, K., Safai, P. D., Rao, P. S. P., and Gustafsson, Ö.: 13C- and 14C-based study of sources and atmospheric processing of water-soluble organic carbon (WSOC) in South Asian aerosols, J. Geophys. Res.-Atmos., 118, 614–626, 2013.
Kondo, Y., Miyazaki, Y., Takegawa, N., Miyakawa, T., Weber, R. J., Jimenez, J. L., Zhang, Q., and Worsnop, D. R.: Oxygenated and water-soluble organic aerosols in Tokyo, J. Geophys. Res.-Atmos., 112, D01203, https://doi.org/10.1029/2006JD007056, 2007.
Li, H., Zhang, Q., Jiang, W., Collier, S., Sun, Y., Zhang, Q., and He, K.: Characteristics and sources of water-soluble organic aerosol in a heavily polluted environment in Northern China, Sci. Total Environ., 758, 143970, https://doi.org/10.1016/j.scitotenv.2020.143970, 2021.
Li, S., Liu, D., Kong, S., Wu, Y., Hu, K., Zheng, H., Cheng, Y., Zheng, S., Jiang, X., Ding, S., Hu, D., Liu, Q., Tian, P., Zhao, D., and Sheng, J.: Evolution of source attributed organic aerosols and gases in a megacity of central China, Atmos. Chem. Phys., 22, 6937–6951, https://doi.org/10.5194/acp-22-6937-2022, 2022.
Lim, S., Hwang, J., Lee, M., Czimczik, C. I., Xu, X., and Savarino, J.: Robust Evidence of 14C, 13C, and 15N Analyses Indicating Fossil Fuel Sources for Total Carbon and Ammonium in Fine Aerosols in Seoul Megacity, Environ. Sci. Technol., 56, 6894–6904, 2022.
Liu, L., Kuang, Y., Zhai, M., Xue, B., He, Y., Tao, J., Luo, B., Xu, W., Tao, J., Yin, C., Li, F., Xu, H., Deng, T., Deng, X., Tan, H., and Shao, M.: Strong light scattering of highly oxygenated organic aerosols impacts significantly on visibility degradation, Atmos. Chem. Phys., 22, 7713–7726, https://doi.org/10.5194/acp-22-7713-2022, 2022.
Liu, M. X. and Matsui, H.: Secondary organic aerosol formation regulates cloud condensation nuclei in the global remote troposphere, Geophys. Res. Lett., 49, e2022GL100543, https://doi.org/10.1029/2022GL100543, 2022.
Mahrt, F., Peng, L., Zaks, J., Huang, Y., Ohno, P. E., Smith, N. R., Gregson, F. K. A., Qin, Y., Faiola, C. L., Martin, S. T., Nizkorodov, S. A., Ammann, M., and Bertram, A. K.: Not all types of secondary organic aerosol mix: two phases observed when mixing different secondary organic aerosol types, Atmos. Chem. Phys., 22, 13783–13796, https://doi.org/10.5194/acp-22-13783-2022, 2022.
Miyazaki, Y., Kondo, Y., Takegawa, N., Komazaki, Y., Fukuda, M., Kawamura, K., Mochida, M., Okuzawa, K., and Weber, R. J.: Time-resolved measurements of water-soluble organic carbon in Tokyo, J. Geophys. Res.-Atmos., 111, D23206, https://doi.org/10.1029/2006JD007125, 2006.
Parnell, A. C., Inger, R., Bearhop, S., and Jackson, A. L.: Source partitioning using stable isotopes: coping with too much variation, PLoS One, 5, e9672, https://doi.org/10.1371/journal.pone.0009672, 2010.
Parnell, A. C., Phillips, D. L., Bearhop, S., Semmens, B. X., Ward, E. J., Moore, J. W., Jackson, A. L., Grey, J., Kelly, D. J., and Inger, R.: Bayesian stable isotope mixing models, Environmetrics, 24, 387–399, 2013.
Pavuluri, C. M. and Kawamura, K.: Seasonal changes in TC and WSOC and their 13C isotope ratios in Northeast Asian aerosols: land surface-biosphere-atmosphere interactions, Acta Geochimica, 36, 355–358, 2017.
Plasencia Sánchez, E., Sánchez-Soberón, F., Rovira, J., Sierra, J., Schuhmacher, M., Soler, A., Torrentó, C., and Rosell, M.: Integrating dual C and N isotopic approach to elemental and mathematical solutions for improving the PM source apportionment in complex urban and industrial cities: Case of Tarragona – Spain, Atmos. Environ., 293, 119449, https://doi.org/10.1016/j.atmosenv.2022.119449, 2023.
Presto, A., Miracolo, M., Kroll, J., Worsnop, D., Robinson, A., and Donahue, N.: Intermediate-volatility organic compounds: a potential source of ambient oxidized organic aerosol, Environ. Sci. Technol., 43, 4744–4749, 2009.
Pye, H. O. T., Murphy, B. N., Xu, L., Ng, N. L., Carlton, A. G., Guo, H., Weber, R., Vasilakos, P., Appel, K. W., Budisulistiorini, S. H., Surratt, J. D., Nenes, A., Hu, W., Jimenez, J. L., Isaacman-VanWertz, G., Misztal, P. K., and Goldstein, A. H.: On the implications of aerosol liquid water and phase separation for organic aerosol mass, Atmos. Chem. Phys., 17, 343–369, https://doi.org/10.5194/acp-17-343-2017, 2017.
Qiu, Y., Xie, Q., Wang, J., Xu, W., Li, L., Wang, Q., Zhao, J., Chen, Y., Chen, Y., Wu, Y., Du, W., Zhou, W., Lee, J., Zhao, C., Ge, X., Fu, P., Wang, Z., Worsnop, D. R., and Sun, Y.: Vertical characterization and source apportionment of water-soluble organic aerosol with high-resolution aerosol mass spectrometry in Beijing, China, ACS Earth Space Chem., 3, 273–284, 2019.
Salma, I., Ocskay, R., Chi, X., and Maenhaut, W.: Sampling artefacts, concentration and chemical composition of fine water-soluble organic carbon and humic-like substances in a continental urban atmospheric environment, Atmos. Environ., 41, 4106–4118, 2007.
Shen, Z., Zhang, Q., Cao, J., Zhang, L., Lei, Y., Huang, Y., Huang, R. J., Gao, J., Zhao, Z., Zhu, C., Yin, X., Zheng, C., Xu, H., and Liu, S.: Optical properties and possible sources of brown carbon in PM2.5 over Xi'an, China, Atmos. Environ., 150, 322–330, 2017.
Shrivastava, M., Cappa, C. D., Fan, J. W., Goldstein, A. H., Guenther, A. B., Jimenez, J. L., Kuang, C., Laskin, A., Martin, S. T., Ng, N. L., Petaja, T., Pierce, J. R., Rasch, P. J., Roldin, P., Seinfeld, J. H., Shilling, J., Smith, J. N., Thornton, J. A., Volkamer, R., Wang, J., Worsnop, D. R., Zaveri, R. A., Zelenyuk, A., and Zhang, Q.: Recent advances in understanding secondary organic aerosol: Implications for global climate forcing, Rev. Geophys., 55, 509–559, 2017.
Tang, T., Cheng, Z., Xu, B., Zhang, B., Zhu, S., Cheng, H., Li, J., Chen, Y., and Zhang, G.: Triple Isotopes δ13C, δ2H, and δ14C Compositions and Source Apportionment of Atmospheric Naphthalene: A Key Surrogate of Intermediate-Volatility Organic Compounds (IVOCs), Environ. Sci. Technol., 54, 5409–5418, 2020.
Timonen, H., Carbone, S., Aurela, M., Saarnio, K., Saarikoski, S., Ng, N. L., Canagaratna, M. R., Kulmala, M., Kerminen, V.-M., Worsnop, D. R., and Hillamo, R.: Characteristics, sources and water-solubility of ambient submicron organic aerosol in springtime in Helsinki, Finland, J. Aerosol Sci., 56, 61–77, 2013.
Verma, V., Fang, T., Xu, L., Peltier, R. E., Russell, A. G., Ng, N. L., and Weber, R. J.: Organic aerosols associated with the generation of reactive oxygen species (ROS) by water-soluble PM2.5, Environ. Sci. Technol., 49, 4646–4656, 2015.
Vodicka, P., Kawamura, K., Schwarz, J., and Zdimal, V.: Seasonal changes in stable carbon isotopic composition in the bulk aerosol and gas phases at a suburban site in Prague, Sci. Total Environ., 803, 149767, https://doi.org/10.1016/j.scitotenv.2021.149767, 2022.
Wang, Y., Feng, Z., Yuan, Q., Shang, D., Fang, Y., Guo, S., Wu, Z., Zhang, C., Gao, Y., Yao, X., Gao, H., and Hu, M.: Environmental factors driving the formation of water-soluble organic aerosols: A comparative study under contrasting atmospheric conditions, Sci. Total Environ., 866, 161364, https://doi.org/10.1016/j.scitotenv.2022.161364, 2023.
Weber, R. J., Sullivan, A. P., Peltier, R. E., Russell, A., Yan, B., Zheng, M., de Gouw, J., Warneke, C., Brock, C., Holloway, J. S., Atlas, E. L., and Edgerton, E.: A study of secondary organic aerosol formation in the anthropogenic-influenced southeastern United States, J. Geophys. Res.-Atmos., 112, D13302, https://doi.org/10.1029/2007JD008408, 2007.
Widory, D., Roy, S., Le Moullec, Y., Goupil, G., Cocherie, A., and Guerrot, C.: The origin of atmospheric particles in Paris: a view through carbon and lead isotopes, Atmos. Environ., 38, 953–961, 2004.
Wong, J. P., Zhou, S., and Abbatt, J. P.: Changes in secondary organic aerosol composition and mass due to photolysis: relative humidity dependence, J. Phys. Chem. A, 119, 4309–4316, 2015.
Wu, Y., Huang, X., Jiang, Z., Liu, S., and Cui, L.: Composition and sources of aerosol organic matter in a highly anthropogenic influenced semi-enclosed bay: Insights from excitation-emission matrix spectroscopy and isotopic evidence, Atmos. Res., 241, 104958, https://doi.org/10.1016/j.atmosres.2020.104958, 2020.
Xiao, H. W., Xu, Y., and Xiao, H. Y.: Source apportionment of black carbon aerosols in winter across China, Atmos. Environ., 298, 119622, https://doi.org/10.1016/j.atmosenv.2023.119622, 2023.
Xiao, R., Takegawa, N., Zheng, M., Kondo, Y., Miyazaki, Y., Miyakawa, T., Hu, M., Shao, M., Zeng, L., Gong, Y., Lu, K., Deng, Z., Zhao, Y., and Zhang, Y. H.: Characterization and source apportionment of submicron aerosol with aerosol mass spectrometer during the PRIDE-PRD 2006 campaign, Atmos. Chem. Phys., 11, 6911–6929, https://doi.org/10.5194/acp-11-6911-2011, 2011.
Yao, P., Huang, R. J., Ni, H. Y., Kairys, N., Yang, L., Meijer, H. A. J., and Dusek, U.: 13C signatures of aerosol organic and elemental carbon from major combustion sources in China compared to worldwide estimates, Sci. Total Environ., 810, 151284, https://doi.org/10.1016/j.scitotenv.2021.151284, 2022.
Zaryab, A., Nassery, H. R., Knoeller, K., Alijani, F., and Minet, E.: Determining nitrate pollution sources in the Kabul Plain aquifer (Afghanistan) using stable isotopes and Bayesian stable isotope mixing model, Sci. Total Environ., 823, 153749, https://doi.org/10.1016/j.scitotenv.2022.153749, 2022.
Zhang, Y. L., Li, J., Zhang, G., Zotter, P., Huang, R. J., Tang, J. H., Wacker, L., Prevot, A. S., and Szidat, S.: Radiocarbon-based source apportionment of carbonaceous aerosols at a regional background site on Hainan Island, South China, Environ. Sci. Technol., 48, 2651–2659, 2014.
Zhao, Y. L., Hennigan, C. J., May, A. A., Tkacik, D. S., de Gouw, J. A., Gilman, J. B., Kuster, W. C., Borbon, A., and Robinson, A. L.: Intermediate-volatility organic compounds: a large source of secondary organic aerosol, Environ. Sci. Technol., 48, 13743–13750, 2014.
Zhu, Q., He, L.-Y., Huang, X.-F., Cao, L.-M., Gong, Z.-H., Wang, C., Zhuang, X., and Hu, M.: Atmospheric aerosol compositions and sources at two national background sites in northern and southern China, Atmos. Chem. Phys., 16, 10283–10297, https://doi.org/10.5194/acp-16-10283-2016, 2016.