the Creative Commons Attribution 4.0 License.
the Creative Commons Attribution 4.0 License.
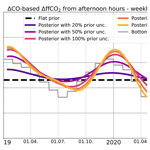
Potential of 14C-based vs. ΔCO-based ΔffCO2 observations to estimate urban fossil fuel CO2 (ffCO2) emissions
Fabian Maier
Christian Rödenbeck
Ingeborg Levin
Christoph Gerbig
Maksym Gachkivskyi
Samuel Hammer
Atmospheric transport inversions are a powerful tool for independently estimating surface CO2 fluxes from atmospheric CO2 concentration measurements. However, additional tracers are needed to separate the fossil fuel CO2 (ffCO2) emissions from non-fossil CO2 fluxes. In this study, we focus on radiocarbon (14C), the most direct tracer of ffCO2, and the continuously measured surrogate tracer carbon monoxide (CO), which is co-emitted with ffCO2 during incomplete combustion. In the companion paper by Maier et al. (2024), we determined discrete 14C-based and continuous ΔCO-based estimates of the ffCO2 excess concentration (ΔffCO2) compared with a clean-air reference for the urban Heidelberg observation site in southwestern Germany. The ΔCO-based ΔffCO2 concentration was calculated by dividing the continuously measured ΔCO excess concentration by an average 14C-based ratio. Here, we use the CarboScope inversion framework adapted for the urban domain around Heidelberg to assess the potential of both types of ΔffCO2 observations to investigate ffCO2 emissions and their seasonal cycle. We find that, although they are more precise, 14C-based ΔffCO2 observations from almost 100 afternoon flask samples collected in the 2 years of 2019 and 2020 are not well suited for estimating robust ffCO2 emissions in the main footprint of this urban area, which has a very heterogeneous distribution of sources including several point sources. The benefit of the continuous ΔCO-based ΔffCO2 estimates is that they can be averaged to reduce the impact of individual hours with an inadequate model performance. We show that the weekly averaged ΔCO-based ΔffCO2 observations allow for a robust reconstruction of the seasonal cycle of the area source ffCO2 emissions from temporally flat a priori emissions. In particular, the distinct COVID-19 signal – with a steep drop in emissions in spring 2020 – is clearly present in these data-driven a posteriori results. Moreover, our top-down results show a shift in the seasonality of the area source ffCO2 emissions around Heidelberg in 2019 compared with the bottom-up estimates from the Netherlands Organization for Applied Scientific Research (TNO). This highlights the huge potential of ΔCO-based ΔffCO2 to validate bottom-up ffCO2 emissions at urban stations if the ratios can be determined without biases.
- Article
(7843 KB) - Full-text XML
- Companion paper
- BibTeX
- EndNote
The combustion of fossil fuels (ff) like coal, oil, and gas is the major reason for the ongoing increase in the atmospheric CO2 concentration, which causes current global warming. About 70 % of the global ffCO2 emissions are released from urban hotspot regions (Duren and Miller, 2012). Fortunately, the atmospheric CO2 increase is weakened, as about half of the human-induced CO2 emissions are currently taken up by the terrestrial biosphere and the oceans in roughly equal shares (Friedlingstein et al., 2022). Indeed, there are large seasonal and interannual variations in the non-fossil CO2 sinks and sources that need to be better understood in order to make predictions about future changes in the carbon cycle owing to increased atmospheric CO2 levels.
The “atmospheric transport inversion” (Newsam and Enting, 1988) is a powerful tool for deducing surface CO2 fluxes from atmospheric CO2 observations. Hence, many studies have applied this top-down approach to constrain CO2 fluxes from terrestrial ecosystems and the oceans (e.g., Rödenbeck et al., 2003; Peylin et al., 2013; Jiang et al., 2016; Rödenbeck et al., 2018; Monteil et al., 2020; Liu et al., 2021). In these calculations, ffCO2 emissions are typically prescribed using bottom-up information from emission inventories. These bottom-up ffCO2 emission estimates are sometimes based on national annual activity data that describe the fuel consumption and sector-specific emission factors (Janssens-Maenhout et al., 2019). While annual national total ffCO2 emissions are associated with low uncertainties of typically a few percent for developed countries (Andres et al., 2012), their proxy-based distribution on individual spatial grid cells and individual months, days, or hours can dramatically increase the uncertainties (Peylin et al., 2013; Super et al., 2020). On the path to net-zero emissions, independent verification of the reported national CO2 emissions is essential. This includes the evaluation of the bottom-up statistics, especially on the relevant urban scales where uncertainties are larger and the most important emission reduction measures are implemented. Furthermore, the seasonal cycle of bottom-up ffCO2 emissions needs to be validated if they are used in CO2 inversions, in order to deduce biogenic CO2 fluxes that are dominated by a large seasonal cycle.
Atmospheric transport inversions can be used to validate these bottom-up ffCO2 emissions (e.g., Graven et al., 2018; Basu et al., 2020). However, their success relies on the ability of the used observational tracers to separate fossil fuel from non-fossil CO2 contributions (Shiga et al., 2014; Ciais et al., 2015; Basu et al., 2016; Bergamaschi et al., 2018). The most direct tracer of ffCO2 is radiocarbon (14C) in CO2. Radiocarbon has a half-life of 5700 years and is, therefore, no longer present in fossil fuels (Suess, 1955). Thus, the 14C depletion in ambient air CO2 compared with a clean-air reference site can directly be used to estimate the recently added ffCO2 excess (ΔffCO2) at the observation site (Levin et al., 2003; Turnbull et al., 2006). These ΔffCO2 estimates can then be implemented in regional inversions to evaluate bottom-up ffCO2 emissions in the footprints of the observation sites (Graven et al., 2018; Wang et al., 2018). However, a drawback of 14C-based ΔffCO2 estimates is that they have poor temporal and spatial coverage due to the labor-intensive and expensive 14C sampling and analysis methods. Therefore, continuously measured atmospheric excess concentrations of trace gases like CO, which is co-emitted with ffCO2, have been used as alternative proxies for ΔffCO2 (e.g., Gamnitzer et al., 2006; Turnbull et al., 2006; Levin and Karstens, 2007; Van Der Laan et al., 2010; Vogel et al., 2010). However, the construction of a high-resolution ΔCO-based ΔffCO2 record requires one to correctly determine the ratio in the footprint of the observation site. This can indeed be a big challenge: as the emission ratio depends on the combustion efficiency and applied end-of-pipe measures, it is very variable for different emission processes and changes with time due to technological progress (Dellaert et al., 2019).
In the companion paper by Maier et al. (2024), we calculated a ΔCO-based ΔffCO2 record for the urban Heidelberg observation site by dividing the continuous ΔCO record from Heidelberg by an average ratio derived from almost 350 14CO2 flask samples collected between 2019 and 2020. We refer to this continuous ΔffCO2 record as “ΔCO-based ΔffCO2” in the following but emphasize that we also used 14CO2 flask observations to estimate the ΔCO-based ΔffCO2 here. By comparing the hourly ΔCO-based ΔffCO2 with the direct 14C-based ΔffCO2 from the flasks, we estimated an uncertainty for these data of about 4 ppm, which is almost 4 times larger than typical 14C-based ΔffCO2 uncertainties. About half of this uncertainty could be attributed to the spatiotemporal variability in the ratios (Maier et al., 2024).
The goal of this study is to investigate which type of ΔffCO2 observations provides the greater benefit in an atmospheric transport inversion to validate bottom-up ffCO2 emission estimates in an urban region: (1) sparse 14C-based ΔffCO2 observations from flasks with a small uncertainty or (2) ΔCO-based ΔffCO2 estimates at high temporal resolution but with an increased uncertainty. For this, we adapt the CarboScope inversion framework (Rödenbeck, 2005) for the highly populated and industrialized Rhine Valley in southwestern Germany around the Heidelberg observation site. We perform separate inversion runs with the 14C- and ΔCO-based ΔffCO2 observations from Heidelberg. Thereby, we mainly focus on the seasonal cycle in the ffCO2 emissions and investigate which ΔffCO2 information leads to robust inversion results and is, thus, best suited to validate the seasonal cycle of the bottom-up emissions in the main footprint of Heidelberg.
2.1 Heidelberg observation site
Heidelberg is a medium-sized city with about 160 000 inhabitants; it is part of the Rhine–Neckar metropolitan area, which is home to over 2 million people. The Heidelberg observation site is located on the Heidelberg University campus in the northwestern part of the city. The sampling inlet line is 30 m above the ground on the roof of the institute's building. Local ffCO2 emissions originate mainly from traffic and residential heating, but there is also a nearby combined heat and power station as well as a large coal-fired power plant and the giant BASF industrial complex 15–20 km to the northwest. Due to its location in the Upper Rhine Valley, Heidelberg is frequently influenced by southwesterly air masses, which carry the signals from heterogeneous sources in the Rhine Valley. A more detailed description of the Heidelberg observation site can be found in Levin et al. (2011). The 14C-based and ΔCO-based ΔffCO2 observations from Heidelberg are presented in Sect. 2.2.3.
2.2 Inversion setup
The CarboScope inversion algorithm was initially introduced by Rödenbeck et al. (2003) to estimate interannual and spatial variability in global CO2 surface–atmosphere fluxes. The algorithm can also be applied to regional inversions (Rödenbeck et al., 2009). In the present study, we adapt this inversion modeling framework to estimate ffCO2 surface fluxes in the regional Rhine Valley domain (see Fig. 1) with ΔffCO2 observations from the Heidelberg observation site (see Fig. 2). This requires a high-resolution atmospheric transport model and a careful estimation of the lateral ΔffCO2 boundary conditions.
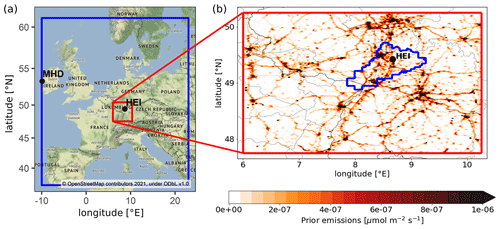
Figure 1(a) Map of the central European STILT domain (blue) and the high-resolution Rhine Valley STILT domain (red). The Heidelberg (HEI) observation site and the Mace Head (MHD) marine site, which we used as a background site to calculate the ΔffCO2 concentrations at Heidelberg (see Sect. 2.2.3), are indicated. (b) Zoomed view of the Rhine Valley domain showing the mean prior ffCO2 emissions from the TNO inventory for 2019–2020. The blue outline within panel (b) shows the “50 % footprint” range, i.e., the area accounting for 50 % of the Heidelberg average footprint within the Rhine Valley.
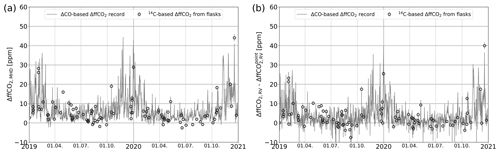
Figure 2Afternoon ΔffCO2 observations from the Heidelberg observation site. The gray curve indicates the ΔCO-based ΔffCO2 record and the black circles show the 14C-based ΔffCO2 estimates from flasks. Both the 14C-based and ΔCO-based ΔffCO2 observations are 2σ-selected. Panel (a) shows the ΔffCO2 excess compared with the Mace Head marine background site (i.e., ΔffCO2,MHD in Eq. 1). Panel (b) shows the ΔffCO2 excess compared with the Rhine Valley (RV) boundary (i.e., ΔffCO2,RV in Eq. 1) minus the modeled ΔffCO2,RV contributions from point sources within the Rhine Valley (ΔffCO). The data in panel (b) are effectively used to optimize the area source emissions in the Rhine Valley.
The CarboScope inversion system uses Bayesian inference to minimize the deviations between observed and modeled ΔffCO2 concentrations by finding the (global) minimum of the cost function (for technical details, see Appendix A and Rödenbeck, 2005). This cost function consists of a data constraint and an a priori flux constraint, which is needed to regularize the underdetermined problem and to prevent large and unrealistic spatiotemporal ffCO2 flux variabilities (Rödenbeck et al., 2018). The data constraint is weighted by the uncertainties of the transport model and the ΔffCO2 observations. Furthermore, the uncertainty applied for the a priori ffCO2 emissions determines the impact of the a priori constraint. Overall, the ratio between the model–data uncertainty and the a priori flux uncertainty controls the strength of the a priori constraint over the observational constraint (Rödenbeck, 2005; Kountouris et al., 2018; Munassar et al., 2022). The cost function is minimized by using a conjugate gradient algorithm with reorthogonalization after each iteration step (Rödenbeck, 2005). In this study, we optimize a single scalar on the a priori ffCO2 emissions field inside the Rhine Valley domain every day.
2.2.1 Atmospheric transport model
We use the Stochastic Time-Inverted Lagrangian Transport (STILT; Lin et al., 2003; Nehrkorn et al., 2010) model, driven by meteorological fields from the high-resolution Weather Research and Forecasting (WRF) model (version 3.9.1.1; Skamarock et al., 2008), to simulate the atmospheric transport in the Rhine Valley domain (see the red rectangle in Fig. 1). Hourly 0.25° resolution data from the fifth generation (ERA5; Hersbach et al., 2020) of the European Centre for Medium-Range Weather Forecasts (ECMWF) atmospheric reanalysis were used as boundary conditions for the WRF simulations. The WRF meteorological fields have a horizontal resolution of 2 km and were generated by applying the MYNN (Mellor–Yamada–Nakanishi–Niino; Nakanishi and Niino, 2009) planetary boundary layer (PBL) parameterization scheme. Finally, using STILT, we calculated the sensitivity of the Heidelberg observations to ffCO2 emissions from individual grid cells in the catchment area of the site (i.e., the so-called footprint in units of concentration per flux density) by computing the back-trajectories of 100 particles released from the Heidelberg receptor site for each hour. The hourly resolution footprints have a horizontal spatial resolution of about 1 km × 1 km (, long × lat). As there are many point source emissions within the Rhine Valley, we apply the STILT volume source influence (VSI) approach introduced by Maier et al. (2022) to model them. This modeling approach considers the effective heights (including plume rise) of the point source emissions, which are typically released from elevated chimney stacks. This approach substantially improved the simulation of ΔffCO2 concentrations at the Heidelberg site, especially during situations with low PBL heights (Maier et al., 2022). For the area source emissions, we apply the standard approach in STILT, which assumes that all emissions are released from the surface.
2.2.2 A priori information
We use the ffCO2 emissions from the Netherlands Organization for Applied Scientific Research (TNO; Dellaert et al., 2019; Denier van der Gon et al., 2019) with a horizontal resolution of about 1 km (° °, long × lat) as a priori estimates for our Rhine Valley inversion. The TNO emission inventory provides annual ffCO2 emissions for 15 different source sectors as well as sector-specific temporal profiles. In this study, we treat the ffCO2 emissions from the point-source-dominated “energy production” and “industry” TNO sectors separately for the following reasons:
-
While the VSI approach (see above) strongly improves the vertical representation of point source emissions in STILT (Maier et al., 2022), it still remains difficult to correctly describe the mixing and transport of narrow point source plumes with meteorological fields that have a resolution of 2 km.
-
Due to the elevated release of point source emissions from high stacks, the Heidelberg observation site, with an air intake height of only 30 m above the ground, is rarely influenced by distinct emission plumes from nearby point sources (see Fig. 4 in Maier et al., 2024, which shows the ratio analysis). This makes it difficult to evaluate those point source emissions with ΔffCO2 observations from the Heidelberg observation site alone.
-
As the energy and industry point source emissions in TNO are directly based on the European Pollutant and Transfer Register (E-PRTR) database, which provides information on the location and emission of the major facilities in Europe (Kuenen et al., 2014), we expect them to be better known than the more diffuse area source emissions in the Rhine Valley.
Thus, we focus on how well our observations are able to constrain area source emissions in the footprint of the Heidelberg site.
Hence, we prescribe the energy and industry emissions in our inversion setup and adjust only the area source emissions in the Rhine Valley, which mainly originate from the heating and traffic sector. TNO provides monthly profiles for the ffCO2 emissions from each of the 15 source sectors. We use the monthly profiles for 2019, which are European averages and, thus, identical for all countries within the central European STILT domain (see blue box in Fig. 1a). For 2020, TNO provides country-specific temporal profiles to account for the large variabilities in the length and intensity of the COVID-19 restrictions among the individual countries. As Germany and France are both part of the Rhine Valley domain, we decided to use the average of the German and French temporal profiles for 2020 to construct suitable sector-specific monthly profiles for the Rhine Valley domain in 2020. For all inversion runs performed in this study, we use these TNO monthly profiles to calculate monthly ffCO2 emissions for the energy and industry sectors from the corresponding annual total emissions.
In this study, we aim to evaluate the ΔffCO2 observation information regarding the seasonal cycle of the area source ffCO2 emissions. That is why we apply temporally constant (“flat”) a priori ffCO2 emissions for the area sources in our standard inversion runs. For this, we use the (high-spatial-resolution) 2-year average TNO area source emissions of the years 2019 and 2020. Finally, we also perform a sensitivity inversion run, in which we replace the temporally flat a priori area source emissions by monthly varying a priori area source emissions. For this, we use the monthly profiles from TNO described above (i.e., the European average monthly profiles in 2019 and the mean of the German and French monthly profiles in 2020) for both the area and the point source emissions.
2.2.3 Observations
In separate inversion runs, we use either the discrete 14C-based ΔffCO2 estimates from flasks, collected as integrals over 1 h, or the hourly ΔCO-based ΔffCO2 record from the Heidelberg observation site (see Fig. 2). The companion paper (Maier et al., 2024) describes the calculation of the 14C-based ΔffCO2 estimates in detail as well as the construction of the continuous ΔCO-based ΔffCO2 record. In short, the ΔCO-based ΔffCO2 record has been constructed by dividing the continuously measured hourly ΔCO offsets compared with the Mace Head (MHD) marine reference site by an average ratio of 8.44±0.07 ppb ppm−1, which was determined from ΔCO and 14C-based ΔffCO2 observations of almost 350 daytime and nighttime flask samples collected in 2019 and 2020. Correlation of these ΔCO and ΔffCO2 values showed only small variability, because heating and traffic emission ratios in the footprint of Heidelberg are currently very similar (around 8 ppb ppm−1). In the inversion, however, we only use the afternoon 14C-based and ΔCO-based ΔffCO2 observations between 11:00 and 16:00 UTC, as nighttime situations are associated with a poorer transport model performance. Times for the hourly integrated ΔffCO2 observations are reported as the start of the hour, e.g., 11:00 UTC corresponds to the time period between 11:00 and 12:00 UTC. The average uncertainties of the 14C- and ΔCO-based ΔffCO2 concentrations were estimated to 1.1 and 3.9 ppm, respectively (Maier et al., 2023a, 2024).
Furthermore, we apply a 2σ-selection criterion to the ΔffCO2 observations, as introduced by Rödenbeck et al. (2018). For this, we take the high-resolution annual total ffCO2 emissions from TNO and apply the hourly sector-specific temporal profiles. These hourly resolution ffCO2 emissions are then transported with the WRF-STILT model to simulate hourly ΔffCO2 concentrations. The mean difference between the simulated and the ΔCO-based ΔffCO2 observations is only −0.04 ppm during afternoon hours with a standard deviation of 6.76 ppm (i.e., almost 100 % of the mean value), which indicates that the model is able to reproduce, on average, the afternoon ΔCO-based ΔffCO2 observations without a significant mean bias. This directly allows the application of the 2σ-selection criterion, which means that we only use those ΔffCO2 observations whose deviation from the modeled ΔffCO2 is smaller than 2 times the standard deviation between the observed and modeled ΔffCO2 (i.e., within the 2σ range). Therewith, we exclude the data outside the 2σ range, which obviously cannot be represented with our transport model. Examples of such data are observations during very strong air stagnation events in winter, which are often underestimated in the model, or, vice versa, situations when the model overestimates the point source influence at the observation site. As the inversion system assumes a Gaussian distribution for the model–data mismatch, these extreme outlier events would have a strong impact on the inversion results (Rödenbeck et al., 2018). Thus, this 2σ-selection criterion can be seen as an additional regularization for the inversion to avoid using situations with unrealistic model simulations. We apply the 2σ-selection criterion to both the 14C-based ΔffCO2 observations from the afternoon flask samples and the afternoon hours of the ΔCO-based ΔffCO2 record.
2.2.4 Lateral boundary conditions
We set up the inversion system for the Rhine Valley domain (47.75–50.25° N, 6.00–10.25° E; red rectangle in Fig. 1a) around the Heidelberg observation site and run the inversion for the full 2 years (2019 and 2020) within this domain. However, as we calculated the 14C- and ΔCO-based ΔffCO2 excess compared with MHD (see Maier et al., 2024), we need to define a suitable ΔffCO2 background representative of the boundary of the Rhine Valley domain. In the following, we call this the “Rhine Valley ΔffCO2 background”. By definition, we assume that the Δ14CO2 observations from MHD correspond to ΔffCO2=0 ppm, which is reasonable because the MHD 14CO2 samples were only collected during situations with clean westerly air masses from the Atlantic. Therefore, it seems to be suitable to apply the MHD (ΔffCO2=0 ppm) background to the entire western boundary of the central European STILT domain (blue rectangle in Fig. 1a). However, this introduces the following question: “How representative is this background of the other boundaries of the central European domain?”. Maier et al. (2023a) estimated the representativeness bias of the MHD background for the eastern boundary of the central European domain, which is likely the most polluted border. They showed that the representativeness bias is on average smaller than 0.1 ppm for an observation site in central Europe. Therefore, we neglect this bias and also assume ΔffCO2=0 ppm at the non-western boundaries of the central European domain. To estimate the Rhine Valley ΔffCO2 background, we then use a nested STILT model approach with a 2 km horizontal resolution WRF meteorology in the Rhine Valley domain and a coarser (10 km) WRF resolution in the central European STILT domain outside the Rhine Valley. For both domains, we use hourly ffCO2 emissions from TNO (Dellaert et al., 2019; Denier van der Gon et al., 2019). This nested approach allows us to separate the ffCO2 contributions from each STILT domain. With this setup, we model the ΔffCO2 contributions from the central European domain outside the Rhine Valley (ΔffCO2,CE-RV) for the Heidelberg site for each hour during 2019 and 2020. We then subtract this modeled Rhine Valley ΔffCO2 background (ΔffCO2,CE-RV) from the estimated ΔffCO2 excess compared with MHD (ΔffCO2,MHD) to obtain the ΔffCO2 excess compared with the Rhine Valley boundary (ΔffCO2,RV):
The ΔffCO2,RV excess concentrations compared with the Rhine Valley boundary are then introduced into the inversion system to constrain the ffCO2 emissions within the Rhine Valley. Note, however, that the actual data constraint is the ΔffCO2,RV excess minus the modeled ΔffCO2 contribution from the point sources within the Rhine Valley (ΔffCO; see Fig. 2b), as we prescribe the point source emissions and only optimize for the area source emissions.
We also want to emphasize that the ΔffCO2,RV excess concentrations rely on the STILT transport and the TNO emissions being correct. A potential bias in the modeled transport or the ffCO2 emissions outside the Rhine Valley would directly translate into a bias in the ΔffCO2,RV excess concentrations. This, in turn, might affect the deduced ffCO2 fluxes within the Rhine Valley domain. To assess the impact of the Rhine Valley ΔffCO2 background (ΔffCO2,CE-RV) on the a posteriori ffCO2 fluxes in the main footprint of Heidelberg, we also perform an inversion run with an alternative Rhine Valley ΔffCO2 background. We again model this alternative background with STILT but apply the 0.25° resolution forecasting meteorological data from the Integrated Forecasting System (IFS) instead of the WRF meteorology (see Sect. 2.2.1). Moreover, we replace the TNO emissions with the Emissions Database for Global Atmospheric Research (EDGAR, version 4.3.2; Janssens-Maenhout et al., 2019) emissions to prescribe the ffCO2 fluxes in Europe. This EDGAR inventory was updated to the years 2019 and 2020 by taking the British Petroleum (BP) statistics on fossil fuel consumption into account and was remapped on a grid with a horizontal resolution of 0.25°. Note that we only use this coarser STILT resolution for simulating the alternative Rhine Valley ΔffCO2 background. The inversion itself is again performed with the high-resolution WRF meteorology (see Sect. 3.3).
2.2.5 Model–data mismatch
The model–data mismatch (MDM) is calculated by subtracting the modeled from the observed ΔffCO2,RV concentrations. The uncertainties of the ΔCO-based and 14C-based ΔffCO2 observations are estimated to be 3.9 and 1.1 ppm, respectively (see Maier et al., 2024). The transport model uncertainty of urban, continental sites like Heidelberg with complex local circulations was assumed to be 5 ppm. The quadratically added observational and transport model uncertainties yield the total uncertainty of the model–data mismatch, which we call the MDM error in the following. To account for the temporal correlations of observations that are close together in time, we apply a data density weighting, as described in Rödenbeck (2005). It artificially increases the MDM error, so that all observations within 1 week lead to the same constraint as a single observation per week. The weighting interval was set to 1 week because this is a typical duration of synoptic weather patterns. Depending on the number of observations per week, the final MDM error of individual hourly observations ranges between 5.1 and 7.8 ppm in the case of 14C-based ΔffCO2 from flasks and between 23.7 and 37.5 ppm in the case of the ΔCO-based ΔffCO2 record.
Based on our analysis results presented in Sect. 3.1, we decided to apply weekly averaging in the case of the ΔCO-based ΔffCO2 inversion (see Sect. 3.2), which we briefly describe here. The MDM vector for the ΔCO-based ΔffCO2 inversion has a length of 3237, which represents the number of the (2σ-selected) afternoon hours with available ΔCO-based ΔffCO2 observations. Weekly averaging means that each hourly entry of the MDM vector within a week is replaced by the respective weighted average MDM of that week. The weight of the individual hours within a week is defined by the MDM error of the respective hours. This means that the weekly averaged MDM vector has the same length as the original MDM vector. We do not modify the hourly MDM errors when applying the weekly averaging. This means that the weekly averaging would not change anything if all hourly MDM entries within a week were initially (by chance) the same.
2.2.6 Degrees of freedom
As we only use ΔffCO2 observations from one single station in the Rhine Valley, we restrict the number of degrees of freedom in our inversion system so that the inverse problem is not too strongly underdetermined. Therefore, we only investigate the area source emissions in the Rhine Valley and prescribe the energy and industry emissions, as described above. Moreover, the inversion system adjusts only one spatial scaling factor per day, which increases or decreases the area source emissions in the whole Rhine Valley domain equally. Hence, we expect that the high-resolution TNO inventory is much better at describing the large spatial heterogeneity in the ffCO2 emissions within the Rhine Valley than our top-down approach. As we want to investigate the seasonal cycle of the ffCO2 emissions, additional temporal degrees of freedom are needed. For this, we choose a temporal correlation length of about 4 months (“Filt3T” in CarboScope notation), which should be appropriate to explore seasonal cycles. Finally, because the Heidelberg observations cannot be used to constrain the emissions in the whole Rhine Valley domain, we only analyze the a posteriori area source emissions in the (most constrained) near field of the observation site. We define the near field of Heidelberg as the area that accounts for 50 % of the temporally accumulated footprint in the Rhine Valley domain for the 2 years of 2019 and 2020 (blue outlined region in Fig. 1b).
3.1 Potential of flask-based ΔffCO2 estimates to investigate the seasonal cycle of ffCO2 emissions
First, we investigate the potential of flask-based ΔffCO2 estimates to resolve the seasonal cycle of the area source ffCO2 emissions around the urban Heidelberg observation site. For this, we use the average of the TNO area source ffCO2 emissions of the 2 years of 2019 and 2020 as a temporally constant prior estimate (see Sect. 2.2.2). To analyze the impact of the observational constraint on the a posteriori results, we apply different prior uncertainties, which effectively lead to different ratios between a priori and observational constraints (Fig. 3). In a first inversion run (Fig. 3a), we use the 14C-based ΔffCO2 observations from the 94 afternoon flasks collected in Heidelberg in the 2 years of 2019 and 2020. The distribution of the flask samplings over the 2 years can be seen in Fig. 2. For various reasons (e.g., testing of the flask sampler associated with frequent changes in the flask sampling strategy), the flasks were not evenly collected; specifically, the 2019–2020 winter has only limited flask coverage. The 14C-based a posteriori ffCO2 emissions show a clear seasonal cycle for the larger prior uncertainties that is mainly data-driven. However, large and unrealistic a posteriori flux variabilities emerge for prior uncertainties larger than 50 % of the flat a priori emissions. For example, the low flask coverage during the 2019–2020 winter period leads to a huge maximum in the area source ffCO2 emissions in November 2019 when the inversion algorithm tries to fit individual flask observations that have large model–data mismatches (see Fig. B1). Similarly, the flask samples in summer 2020 with near-zero or even negative ΔffCO2 estimates, which lead to negative model–data mismatches (cf. Figs. 2b and B1), cause a strong reduction in the a posteriori emissions.
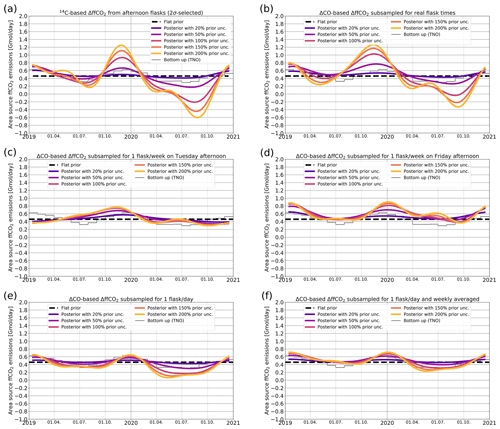
Figure 3Area source ffCO2 emissions in the near field (blue outlined area in Fig. 1b) of Heidelberg. Shown are the flat prior emissions (dashed black line), the a posteriori emissions for different prior uncertainties between 20 % and 200 % of the flat a priori emissions (solid colored lines) as well as the bottom-up estimates from TNO (gray line). In panel (a), 14C-based ΔffCO2 estimates from 94 2σ-selected afternoon flasks from Heidelberg were used as observational input (see Fig. 2). Panel (b) shows the inversion results if the ΔCO-based ΔffCO2 observations subsampled during the 94 flask sampling hours were used. In panels (c) and (d), the inversion was constrained with one hourly afternoon (at 13:00 UTC) ΔCO-based ΔffCO2 observation every week collected on the 2 random working days of Tuesday (c) or Friday (d). Panel (e) shows the results if one hypothetical flask is collected each day at 13:00 UTC. In panel (f), the seven afternoon flask observations within 1 week are averaged.
Figure B1 shows the agreement between the flat prior and the different a posteriori-based model results and the flask observations. The flat prior emissions lead to a mean bias (observed minus modeled ΔffCO2 concentration) of 0.68 ppm with a standard deviation of 5.61 ppm. The a posteriori emissions based on a 50 % prior uncertainty reduce the mean bias and the standard deviation to 0.35±5.23 ppm. However, for higher prior uncertainties, the mean bias increases again and only the standard deviation is further reduced; for example, a prior uncertainty of 150 % leads to a mean bias of 0.54±4.78 ppm between the observed and modeled ΔffCO2 concentration. This might be an indication of overfitting. To further investigate the performance of the inversion, we conducted a reduced chi-square () analysis. The values decrease from 1.10 (for a 50 % prior uncertainty) to 0.97 (for a 150 % prior uncertainty). Typically, values smaller than 1 are an indication of overfitting if the model–data mismatch error is chosen properly. However, as the values (for prior uncertainties between 50 % and 200 %) are all close to 1 (within ±10 %), the values might not be suitable to demonstrate overfitting in our case. Overall, this urban inversion setup obviously needs a very strong regularization through low prior uncertainties to prevent the fitting of individual flask observations and, thus, unrealistic variability in the a posteriori ffCO2 emissions. This indicates that the applied 2σ-filtering approach (see Sect. 2.2.3) is not sufficient in this urban setting.
We further investigate whether these overfitting patterns can be attributed to the uneven distribution of the flask samples. For this, we subsample the continuous ΔCO-based ΔffCO2 record. In a first step, we use the ΔCO-based ΔffCO2 observations from those 94 afternoon hours with flask samples as an observational constraint (Fig. 3b). For the most part, the subsampled ΔCO-based ΔffCO2 observations reproduce the a posteriori results of the 14C-based ΔffCO2 estimates. However, there are differences, like the shifted summer minimum in 2019. These differences can be explained by the deviations between the 14C-based and ΔCO-based ΔffCO2 estimates in Fig. 2, and thus the variability in the ratios that we fully neglected by using a constant mean ratio for constructing the ΔCO-based ΔffCO2 record. Therefore, when comparing the results with the TNO seasonality of emissions (gray histogram), it seems obvious that the 14C-based ΔffCO2 estimates provide more accurate data than the subsampled ΔCO-based ΔffCO2 record. However, the general similarity between both results means that we can use the continuous ΔCO-based ΔffCO2 record to investigate an even data coverage with hypothetical Heidelberg flask samples. Figure 3c and d show the inversion results if the ΔCO-based ΔffCO2 record is subsampled for one flask every week on Tuesday or on Friday afternoon, respectively. We chose Tuesday and Friday as random examples of 2 working days. The evenly distributed weekly flasks strongly dampen the variability in the a posteriori results. However, they show large differences depending on which day of the week the hypothetical afternoon flask was collected: whereas the Tuesday flasks lead to a quite unrealistic gradual increase in the ffCO2 emissions between January and November 2019, the Friday flasks show a more realistic seasonal cycle in this year. In contrast, both Tuesday and Friday flasks lead to an unexpected maximum in summer 2020. The comparison between the (hypothetical) flask concentrations and the a posteriori results illustrates again that the inversion mainly tries to fit those individual hours with the largest MDM (not shown). This implies that the a posteriori results are still dependent on the selection of the individual hypothetical flasks. Therefore, it seems that even a uniform data coverage with a realistic flask sampling frequency of one flask per week is not sufficient to determine a plausible seasonal cycle of the area source ffCO2 emissions around Heidelberg (as, e.g., suggested by the TNO inventory). However, the situation should be improved in the case of real, hourly integrated 14C flasks that are collected, e.g., once per week, as the average ratio used to construct the ΔCO-based ΔffCO2 record might be inappropriate for individual hours.
Finally, we investigated the benefit of an extremely high flask sampling frequency of one flask per afternoon (see Fig. 3e). Note that such a high-frequency flask sampling increases the MDM error because of the applied data density weighting (see Sect. 2.2.5). Here, the a posteriori results seem to approach the TNO bottom-up emissions in 2019. However, there are still unexpectedly strong deviations between the top-down and bottom-up estimates in the summer half-year of 2020 for increased prior uncertainties. These differences might be caused by individual afternoon hours with a negative MDM in summer 2020. To reduce the impact of such hours, we perform a separate inversion run in which we average the modeled and observational data of all seven hypothetical afternoon flasks within each week (Fig. 3f; see Sect. 2.2.5 for a description of the weekly averaging of the MDM vector). This further reduces the spread of a posteriori results, particularly in summer 2020, further approaching the seasonal amplitude of the bottom-up TNO emissions. Thus, several afternoon flasks per week would be needed so that the influence of individual flasks on the inversion results can be averaged out and a plausible seasonal cycle amplitude in the area source ffCO2 emissions around Heidelberg can be obtained.
Overall, these results show that the a posteriori estimates are very sensitive to individual flask observations in the Heidelberg target region, which has very heterogeneously distributed ffCO2 sources. Obviously, the transport model fails to appropriately simulate the ΔffCO2 concentrations for individual afternoon hours. This can be explained by remaining shortcomings in the transport model as well as by the enormous heterogeneity in the ffCO2 emissions in the footprint of the Heidelberg observation site. As already mentioned in Sect. 2, modeling individual plumes from point source emissions is a particular challenge in this urban region, and, for example, the forward model estimates of point source signals, even with the improved VSI approach, often seem incorrect, at least at a temporal resolution of 1 h. Moreover, there might also be inaccuracies in the TNO point source emissions themselves. Finally, part of the MDM can also be explained by uncertainties in the proxies used to spatially disaggregate the area source emissions in the TNO inventory (Super et al., 2020).
3.2 Potential of continuous ΔCO-based ΔffCO2 estimates to investigate the seasonal cycle of ffCO2 emissions
The big advantage of the continuous ΔCO-based ΔffCO2 record is that it provides full temporal coverage of the inversion period and can, thus, also be averaged such that the sensitivity of the a posteriori results to individual (hourly) model–data mismatches with poor model performance is strongly reduced. In Appendix C, we investigate the time interval over which the hourly ΔCO-based and modeled ΔffCO2 concentrations should be averaged to sufficiently reduce the impact of the point source emissions on the a posteriori area source emissions. For this, in addition to the standard inversion runs with fixed point source emissions, we perform further sensitivity runs with adjustable point source emissions. Ideally (i.e., if the point source emissions are well described in TNO), the a posteriori area source emissions are identical for both inversion runs, meaning that the modeling of the better-known point source emissions has no impact on the area source emissions. It turns out that the averaging interval of 1 week strongly reduces the impact of the point sources on the a posteriori area source emissions (see blue curves in Fig. C1). It limits the differences between the a posteriori area source emissions of the inversion runs with fixed and adjustable point source emissions to below 30 % for individual seasons. Averaged over the 2 years (2019 and 2020), these differences are below 10 %. Therefore, for the ΔCO-based ΔffCO2 inversion, we apply weekly averaging to the MDM vector (as described in Sect. 2.2.5).
In the following, we use the weekly averaged afternoon ΔCO-based ΔffCO2 observations to investigate the seasonal cycle of the area source ffCO2 emissions around Heidelberg (see Fig. 4a). If the chosen prior uncertainty is large enough, the seasonal cycle amplitude of the a posteriori estimates agrees with that of the TNO inventory reasonably well. Moreover, the data-driven inversion results distinctly show the effect of the COVID-19 restrictions, with lower emissions in 2020 compared with 2019. In southwestern Germany, the first COVID-19 lockdown started in mid-March 2020. Indeed, the inversion results show a strong decrease in the area source ffCO2 emissions at that time. In particular, the decline in the a posteriori ffCO2 emissions is much steeper in spring 2020 than in spring 2019 and the minimum of the seasonal cycle is flatter in 2020 (as it extends over several summer months). The sharp drop in emissions at the beginning of the COVID-19 restrictions in spring 2020 can also be seen in Fig. D1, where we plot the difference between the seasonal cycles of the 2 years.
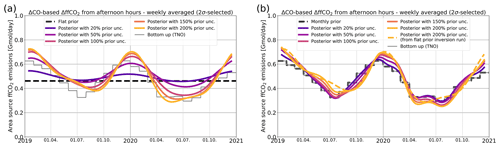
Figure 4Area source ffCO2 emissions in the near field (blue outlined area in Fig. 1b) of Heidelberg. In panel (a), a flat prior (dashed black line) was used for the area source emissions; in panel (b), a monthly prior, i.e., the monthly bottom-up estimates from TNO (dashed gray line), was used as the a priori estimate. Shown are the a posteriori emissions for different prior uncertainties between 20 and 200 % (solid colored lines). The inversion was constrained with weekly averages of hourly, 2σ-selected afternoon ΔCO-based ΔffCO2 observations from Heidelberg. For comparison, the posterior emissions with 200 % prior uncertainty from panel (a) are shown as a dashed yellow line in panel (b).
The agreement with the phasing of the seasonal cycle of the TNO inventory seems to be better in 2020 than in 2019. As described in Sect. 2.2.2, TNO provides country-specific “COVID-19” time profiles for 2020 that consider the timing and the strength of the respective national restrictions. For this year, Fig. 4 shows the average of the monthly time profiles from Germany and France applied to the annual Rhine Valley emissions (gray histogram). This seasonal cycle for 2020 seems to be confirmed by our observations. However, the TNO seasonal cycle shown for 2019 is a general estimate constructed for the whole central European domain (see Fig. 1) that is neither specific for individual countries nor for the year 2019. It assumes minimum emissions in July, whereas our observations show minimum emissions in August and September. Indeed, this shifted minimum of the seasonal cycle coincides with the summer holidays in southwestern Germany, which are from August to mid-September.
We further investigate the consistency of the seasonal cycles from the bottom-up and the top-down estimates. For this, we explore the effect of using the monthly TNO bottom-up seasonal cycle for the a priori emissions (see Fig. 4b). As expected, the phase of the a posteriori seasonal cycle is in agreement with the TNO inventory in 2020. However, the a priori information pulls the summer emission minimum to July in 2019. With weakening regularization of the prior, the inversion algorithm tries to shift the minimum of the a posteriori seasonal cycle from July towards August and September. Due to the limited temporal degrees of freedom of the inversion, this shifting artificially increases the emissions in May 2019 and lowers them in October. Hence, these results might point to some inconsistencies in the seasonality of the TNO emissions in the main footprint of the Heidelberg observation site. In fact, correct phasing of the fossil emissions is essential when prescribed ffCO2 emissions are used in CO2 model inversions to separate the fossil from the biogenic contribution in atmospheric CO2 observations and constrain CO2 fluxes from the biosphere. Although these biospheric CO2 signals are typically estimated with observations from sites that are more remote and rural than the urban Heidelberg site, the correct seasonality in prescribed ffCO2 emissions is still important when deducing the month-to-month variations in the biospheric CO2 fluxes.
Overall, the (weekly averaged) ΔCO-based ΔffCO2 record seems to be well suited to estimate (and validate) the seasonal cycle of bottom-up ffCO2 emissions in the near field of the Heidelberg observation site. This is a very promising result, especially considering how simply the ΔCO-based ΔffCO2 record was constructed. It is based on the 2-year average ratio estimated from 14C measurements on flask samples, and a potential seasonal cycle in the ratios was fully neglected.
3.3 Robustness of the ΔCO-based ΔffCO2 inversion results
In the following, we investigate the robustness of the (weekly averaged) ΔCO-based ΔffCO2 inversion results. For this, we (1) reduce the flat prior emissions by 20 %, (2) assume a seasonal cycle in the ratios, and (3) apply an alternative Rhine Valley ΔffCO2 background. In Fig. 5, we show the respective a posteriori results for a prior uncertainty of 150 %, which constitutes enough weighting on the (weekly averaged) ΔCO-based ΔffCO2 observations to reconstruct the seasonal cycle from the flat a priori area source ffCO2 emissions (see Fig. 4a). Moreover, the 150 % prior uncertainty reduces the mean bias between the weekly averaged ΔCO-based ΔffCO2 observations and the a posteriori fits to 0.10±1.89 ppm (compared with the mean bias of 0.65±2.23 ppm, which one gets when using the flat prior emissions). Further increasing the prior uncertainty to 200 % leads to only neglectable changes in the mean bias (0.11±1.88 ppm).
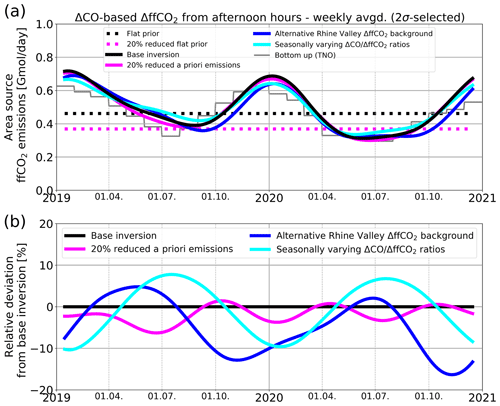
Figure 5(a) Area source ffCO2 emissions in the near field (blue outlined area in Fig. 1b) of Heidelberg for different sensitivity runs. Shown are the a posteriori results for a 20 % reduction in the flat a priori emissions (solid magenta line), for an alternative Rhine Valley ΔffCO2 background modeled with EDGAR emissions (blue), and for an assumed seasonal cycle in the ratios (cyan; see Fig. E1). As a reference, the a posteriori result of the base inversion from Fig. 4a is shown in black. All a posteriori results correspond to a 150 % prior uncertainty. The dotted lines indicate the flat prior emissions (black) and the prior emissions reduced by 20 % (magenta). Panel (b) shows the relative deviations between the different a posteriori area source emissions of the sensitivity runs and the base inversion (in %).
First, if the a priori area source ffCO2 emissions in the Rhine Valley domain are equally reduced by 20 % (see dotted magenta line in Fig. 5a), the ΔCO-based ΔffCO2 inversion manages to compensate for almost all of this bias (compare the magenta curves with the black curves in Fig. 5). The deviations between the a posteriori emissions of the inversion runs with perturbed and unperturbed flat prior emissions is typically below 5 % for all seasons (Fig. 5b). Accordingly, the a posteriori seasonal cycle of the ffCO2 emissions is hardly affected by a potential bias in the flat prior emissions. The deviations between the annual totals of the a posteriori estimates of the perturbed and unperturbed prior inversion runs is only 2 % for both years. This means that about 90 % of this 20 % bias in the perturbed flat prior could be corrected for with the observational constraint on an annual scale.
With the second sensitivity test, we want to investigate the effect of the ratios used to construct the ΔCO-based ΔffCO2 record. For our base inversion, the ΔCO-based ΔffCO2 record was constructed using the average ratio of 8.44±0.07 ppb ppm−1, which was calculated from all flask samples collected in Heidelberg in 2019 and 2020. However, as discussed in Maier et al. (2024), the ratio during summer with lower signals is hard to determine and, thus, less constrained. Indeed, the winter flasks show a slightly higher mean ratio (8.52±0.08 ppb ppm−1, R2=0.89) compared with the summer flasks (8.08±0.17 ppb ppm−1, R2=0.36). This introduces the following question: “How would our inversion results change if the ratios would have a (small) seasonal cycle?”. For this, we assume a seasonal cycle in the ratios for the 2 years, with 5 % lower ratios in the summer half-year and, correspondingly, 5 % larger ratios in the winter half-year; the 2-year mean is still 8.44 ppb ppm−1 (see Fig. E1). Notice that we use the ratios to calculate the ΔffCO2,MHD excess compared with the MHD background site and then subtract the modeled Rhine Valley ΔffCO2,CE-RV background to get the ΔffCO2,RV observations for our Rhine Valley inversion (see Eq. 1). This effectively results in summer and winter ΔffCO2 concentrations being more than 5 % higher and lower than the ΔffCO2 concentrations based on the average ratio, respectively. Obviously, this leads to larger a posteriori emissions (cyan curve in Fig. 5) during summer and lower emissions in winter compared with the base inversion results. The largest seasonal deviations from the base inversion a posteriori emissions are 10 %. As, by construction, the mean of the seasonally varying ratios corresponds to the average ratio used for the base inversion, the effect on the annual totals of the a posteriori ffCO2 emissions is neglectable.
Finally, we investigate the impact of the lateral ΔffCO2 boundaries on the area source ffCO2 emissions estimates. For our base inversion, we used the high-resolution TNO emission inventory and WRF-STILT to model (for the Heidelberg observation site) the ΔffCO2 contributions from the European STILT domain outside the Rhine Valley (see Sect. 2.2.4). For the following sensitivity run (blue curve in Fig. 5), we model the Rhine Valley ΔffCO2 background with ffCO2 emissions based on the EDGAR emissions and with a coarser meteorology in STILT (see Sect. 2.2.4). The application of this alternative Rhine Valley ΔffCO2 background leads to more than 10 % lower emissions in the autumn of both years, which can be explained by strong deviations between the weekly averages of the two modeled background concentrations during these periods (see Fig. F1). Thus, the Rhine Valley background affects the seasonal cycle of the area source ffCO2 emissions. During summer, the deviations from the base inversion results are below 5 %. The annual totals of the area source ffCO2 emissions around Heidelberg are 3 % and 7 % lower in the years 2019 and 2020, respectively, if the alternative Rhine Valley ΔffCO2 background is used.
In the present study, we investigate the potential of 14C-based and ΔCO-based ΔffCO2 observations to evaluate the ffCO2 emissions and their seasonal cycle in an urban region around the Heidelberg observation site. This urban area is characterized by complex topography and large spatial heterogeneity in ffCO2 sources, including several nearby point sources. Thus, deficits in the transport model as well as inaccuracies in the driving meteorology and the prescribed point source emissions strongly impact the model–data mismatch at the observation site, which will be minimized by the inversion algorithm. We focus on the estimation of the ffCO2 emissions from area sources, as the observations from the Heidelberg site with an air intake height of 30 m above the ground are not suitable to constrain the emissions of nearby point sources with elevated stack heights. Indeed, the analysis of the ratios in Maier et al. (2024) showed that the Heidelberg observation site is hardly influenced by pure point source emission plumes. Moreover, we expect that point source emissions can be better quantified a priori from the bottom up compared with area source emissions. Therefore, we prescribe the better-known point source ffCO2 emissions in the inversion setup and only adjust the area source emissions in the Rhine Valley domain.
4.1 Can flask-based ΔffCO2 observations be used to predict the seasonal cycle of ffCO2 emissions at an urban site?
To investigate the potential of ΔffCO2 observations to predict the seasonal cycle of the area source ffCO2 emissions around Heidelberg, we applied temporally constant (flat) a priori ffCO2 emissions in our inversion system, such that all seasonal information comes from the atmospheric data. We have shown that 14C-based ΔffCO2 observations from almost 100 hourly flask samples collected in the 2 years of 2019 and 2020 are not sufficient to reconstruct a robust seasonal cycle from the flat a priori estimate. As the Bayesian inversion setup assumes a Gaussian distribution for the model–data mismatch, the inversion algorithm tries to primarily reduce the largest model–data differences. Therefore, we applied a 2σ-selection criterion to exclude the flask events with the largest model–data mismatches and, thus, poorer model performance. However, the a posteriori ffCO2 emissions are still very sensitive to individual flask observations. This may suggest that the 2σ filter is not sufficient in a densely populated high-fossil-fuel environment like Heidelberg with a complex topography. Therefore, strong regularization through small a priori uncertainties (i.e., < 50 % prior uncertainty; Fig. 3a) is needed in the case of flask observations to avoid large overfitting patterns in the inversion results.
Due to the fortunate circumstance of currently having similar heating and traffic emission ratios in the main footprint of Heidelberg, we decided to use the 2-year average 14C-based ratio from the flasks to construct a continuous ΔCO-based ΔffCO2 record (see Maier et al., 2024). By subsampling this ΔCO-based ΔffCO2 record, we further investigate the potential of uniform data coverage with one hypothetical afternoon flask per week to reliably estimate the seasonal cycle in the area source emissions. Indeed, several afternoon flask samples per week are needed, in addition to an averaging of the flask observations within 1 week so that the overfitting of individual flask data is reduced. However, the situation should be better for real, e.g., sub-weekly, 14C flasks compared with the subsampled ΔCO-based ΔffCO2 record. As the applied 2-year average ratio may be inappropriate for individual hours, this could amplify the sensitivity to the individual hypothetical flasks.
4.2 What is an appropriate averaging interval for urban observations?
The main advantage of the ΔCO-based ΔffCO2 record is its continuous data coverage that allows an averaging so that the influence of individual hours with poor model performance on the inversion results is strongly reduced. The comparison of Fig. 3e with Fig. 3f and Fig. 4a clearly illustrates that the averaging of multiple hourly observations leads to a reduction in the a posteriori flux variability. In this urban region, such an averaging is especially necessary because of the shortcomings in the STILT model and its driving meteorology with respect to describing the transport and mixing of nearby point source emissions. Imagine that the plume of a point source arrives at the observation site a few hours earlier or later than simulated by STILT. In such cases, averaging is inevitable in order to prevent the incorrect adjustment of the ffCO2 emissions. Moreover, the STILT-VSI approach itself has its deficits, as it assumes mean effective emission height profiles for all meteorological situations and ignores the stack heights of individual power plants. Furthermore, the VSI approach still relies on correct vertical mixing in STILT and an accurate point source emission inventory. Although, we could show that the VSI approach strongly improves the agreement between modeled and observed ΔffCO2 concentrations from 2-week integrated samples in Maier et al. (2022), it may still overestimate the point source contributions for individual hours. Therefore, an averaging of the observations is very helpful when a transport model like STILT is used to describe the transport and mixing of nearby point source emissions.
We investigate how to appropriately average the observational and modeled data. Ideally, the a posteriori area source ffCO2 emissions are independent of an incorrect modeling of the point source emissions. Thus, they should not be affected by whether the a priori point source emissions are fixed or adjustable in the inversion framework, provided that the point source emissions are well represented in the emission inventory. We found that an averaging interval of 1-week limits the differences between the a posteriori area source ffCO2 emissions of the inversion runs with fixed and adjustable point source emissions to below 30 % for all seasons. This deviation can be used as a measure of the uncertainty of the a posteriori area source ffCO2 emissions that is induced by an inadequate modeling of the point source emissions. A longer, e.g., monthly, averaging interval further reduces this difference (see Appendix C), but it also involves averaging over very different meteorological situations and, thus, reduces the spatiotemporal information comprised in the observations. This might be especially important if there are several observation sites and the inversion system optimizes the ΔffCO2 gradients between these different stations. The averaging interval of a week corresponds to the typical length scale of synoptic weather patterns. Therefore, the model–data mismatch error has already been increased to account for the potential correlations between the hourly observations within 1 week. The weekly averaging should, thus, not destroy too much information. Hence, an averaging interval of 1 week should be seen as a compromise between reducing the impact of hours with an inadequate model performance and using as much observational information as possible.
4.3 What is the potential of ΔCO-based ΔffCO2 to estimate the seasonal cycle of urban ffCO2 emissions?
The weekly averaged ΔCO-based ΔffCO2 observations lead to robust seasonal cycles in ffCO2 emissions with a plausible amplitude and phasing, based on comparison with the TNO inventory. Figure G1 further compares the TNO ffCO2 emissions in the near-field area of Heidelberg (blue outlined area in Fig. 1b) with emissions from the EDGAR inventory (as introduced in Sect. 2.2.4) and the GridFEDv2021.2 inventory (Jones et al., 2021). While the EDGAR emissions are on average about 25 % lower than the TNO emissions over the 2 years (2019 and 2020), the GridFED inventory shows ca. 23 % larger emissions than TNO. This illustrates the uncertainty of the emission inventories at regional scales. In 2019, the amplitude and phasing of the seasonal cycle are very similar for the EDGAR and TNO inventory. However, the normalized seasonal cycles of the two inventories show differences of up to roughly 20 % in spring 2020, which can be explained by the fact that the shown seasonal cycle of the EDGAR inventory does not take the COVID-19 restrictions into account. In contrast, the seasonal cycles from GridFED include the effect of the COVID-19 restrictions in spring 2020, similar to TNO, but show a smaller seasonal cycle amplitude compared with EDGAR and TNO in 2019. Overall, the seasonal cycles of our top-down estimate are in the range covered by all three bottom-up inventories, thus inferring that we could indeed reliably reconstruct the amplitude and the phasing of the seasonal cycle from flat a priori area source ffCO2 emissions with the ΔCO-based ΔffCO2 observations.
Further, we could detect the COVID-19 signal in 2020, which is characterized by lower emissions compared with 2019 and a very steep decline in the emissions in spring 2020 (see Fig. D1). This is in accordance with what is reported by TNO for 2020. However, our ΔCO-based ΔffCO2 results for 2019 suggest the summer minimum of the (restricted) Rhine Valley area source ffCO2 emissions to be in August and September (instead of July), when local summer holidays take place in that part of Germany. Thus, this result of the Heidelberg inversion might point to some inconsistencies in the seasonality of TNO emissions in the footprint of the station. The correct phasing of the fossil emissions is essential when prescribed ffCO2 emissions and associated forward modeling results are used in atmospheric transport inversions to constrain the CO2 fluxes from the biosphere.
In contrast to the inversion with flask-14C-based ΔffCO2 observations, the ΔCO-based ΔffCO2 inversion with weekly averaging allows a weakening of the regularization strength without generating unrealistic variabilities in the seasonal cycle of the ffCO2 emissions. This implies that the a posteriori results are less dependent on a potential bias in the a priori emissions (see Fig. G1). Indeed, a sensitivity run with a 20 % reduction in the flat prior estimate for the area source ffCO2 emissions leads to similar results to the base inversion run with unperturbed prior estimate, when sufficiently large prior uncertainties are used. Thus, the ΔCO-based ΔffCO2 inversion is able to simultaneously reconstruct the seasonal cycle from a flat prior and correct a potential bias in the total a priori emissions.
However, the ΔCO-based ΔffCO2 inversion results strongly depend on a potential bias in the ratios that are applied to calculate the ΔffCO2 estimates. As there is no evidence of a strong seasonal cycle in the ratios at the Heidelberg observation site, we used a constant average ratio to calculate the ΔCO-based ΔffCO2 record for the 2 years of 2019 and 2020 (see Maier et al., 2024). However, due to the low signals and the weak correlation between ΔCO and ΔffCO2 during summer, it is hard to determine separate summer ratios. Nevertheless, our results indicate that there might be a small seasonal cycle of the order of 5 % in the ratio. We have shown that a hypothetical seasonal cycle with 5 % lower and 5 % larger ratios in summer and winter, respectively, would lead to changes in the area source ffCO2 emissions of up to 10 % for individual seasons. This emphasizes the importance of a thorough determination of the ratios to prevent biases in estimates of total fluxes and the seasonal cycle of the ffCO2 emissions.
Indeed, we are currently in a fortunate situation in Heidelberg, as the emission ratios of the traffic and heating sectors seem to be quite similar in the main footprint of the station (see Maier et al., 2024). Hence, despite the varying share of traffic and heating over the course of a year, this allowed the usage of a constant average flask-based ratio for constructing the ΔCO-based ΔffCO2 record. Of course, it is much more challenging to determine continuous ΔCO-based ΔffCO2 estimates for stations where the ratios show large seasonal or even diurnal variability. In principle, also bottom-up estimates of the seasonal contributions of each ffCO2 sector and its characteristic emission ratio could be used to construct the seasonal cycle of the ratios. However, in the companion paper (Maier et al., 2024), we show that there can be large discrepancies between 14C-based and inventory-based ratios. Therefore, we recommend validating those bottom-up ratios with observations before using them to estimate a continuous ΔffCO2 record.
The ΔCO-based ΔffCO2 inversion can be seen as a simplification of a multispecies inversion, which is based on co-located CO2 and CO observations. Such a multispecies inversion exploits the fact that the co-located CO2 and CO observations are affected by the same atmospheric transport and that these two species have partially overlapping emission patterns (Boschetti et al., 2018). Boschetti et al. (2018) show that the consideration of these interspecies correlations leads to a reduction in the respective a posteriori uncertainties of the ffCO2 (and CO) emissions. While our ΔCO-based ΔffCO2 inversion assumes a constant but observation-based ratio, the multispecies inversion intrinsically considers the spatiotemporal variability in the ratios. However, this requires reliable a priori estimates of the emission ratios and their uncertainties as well as neglectable non-fossil CO sources and sinks.
A common challenge in regional inversions is the determination of the lateral boundary conditions (Munassar et al., 2023). In this study, we used two different emission inventories and meteorological fields to estimate the ΔffCO2 background for the Rhine Valley domain by modeling the contributions from the central European ffCO2 emissions outside the Rhine Valley. For individual seasons, the a posteriori area source ffCO2 emissions around Heidelberg can differ by more than 10 %. This highlights the strong need for appropriate boundary conditions. In Europe, the Integrated Carbon Observation System (ICOS; Heiskanen et al., 2022) provides high-quality atmospheric in situ data from a network of tall-tower stations that cover a large part of the European continent. These observations may help to verify the ffCO2 emissions in Europe. The optimized European ffCO2 emissions could then be used to more reliably estimate the ΔffCO2 background for the Rhine Valley domain.
Overall, our results demonstrate that the weekly averaged ΔCO-based ΔffCO2 observations are currently well suited to investigate the amplitude and the phasing of the seasonal cycle of the area source ffCO2 emissions in the main footprint of the Heidelberg observation site. The different sensitivity runs suggest that ΔCO-based ΔffCO2 allows a reconstruction of this seasonal cycle from temporally constant a priori estimates with an uncertainty of below ca. 30 % for all seasons. Thus, if the ratios can be determined accurately, we recommend applying this ΔCO-based ΔffCO2 inversion at further urban sites with a strong heterogeneity in the local ffCO2 sources. If ratios from bottom-up inventories are not trusted or the urban region is influenced by CO emissions from the biosphere, the ratios are most reliably calculated from 14C flasks. Then, at least some of the summer 14C flasks should be collected during situations with significant CO and ffCO2 signals, so that a possible seasonal cycle in the ratios could be identified. At remote sites, such as at several ICOS atmosphere stations with low ffCO2 signals and predominant biosphere influence, the calculation of ratios and the construction of a bias-free ΔCO-based ΔffCO2 record might be more challenging than at an urban site. However, the model performance is expected to be better at remote sites with a typically higher air intake above the ground and a much lower heterogeneity in the surrounding ffCO2 sources with minor influences from nearby point sources. Consequently, the outcome of our urban study cannot directly be transferred to remote sites; further studies are needed to investigate the potential of 14C-based vs. ΔCO-based ΔffCO2 to estimate ffCO2 emissions at such sites.
Finally, the good performance of the continuous but less precise ΔCO-based ΔffCO2 observations in our regional inversion suggests that there may be the potential for continuously measured 14CO2 (e.g., via optical spectrometry) to estimate urban ffCO2 emissions, even if those continuous 14CO2 measurements have larger uncertainties.
A detailed description of the CarboScope inversion system can be found in Rödenbeck (2005). In the following, we summarize the main characteristics for reference.
In this study, the CarboScope inversion framework is used to minimize the model–data mismatch m between observed and modeled ΔffCO2 concentrations. For this, the ffCO2 flux field f is written in terms of a fixed a priori estimate ffix and a vector p with dimensionless adjustable parameters:
where the matrix F describes the uncertainty of the a priori fluxes and their spatiotemporal correlations. The a priori realization of the parameters ppri is assumed to have a zero mean, i.e., 〈ppri〉=0, and the variance , where 1 is the identity matrix and μ is a scaling factor. This leads to the following cost function:
The first term of this cost function describes the data constraint, which is weighted by the model–data mismatch covariance matrix Qm. The a priori constraint is included in the second term of the cost function. It is scaled by the parameter μ, which effectively represents the ratio between a priori and data constraint (e.g., the a priori term vanishes for μ→0, in accordance with the a priori covariance matrix going to infinity; note that Qf,pri does not explicitly appear in the CarboScope implementation). The last constant C contains all terms that are independent of p.
The minimum of the cost function is calculated using the following expression:
where ppost describes the a posteriori realizations of the parameter vector p. For this, a conjugate gradient algorithm is used, which is described in detail in Rödenbeck (2005).
In Fig. B1, we show the agreement between the flat prior and the different a posteriori-based model results and the flask observations. It illustrates that the inversion mainly reduces the largest model–data mismatches of individual winter flasks and the negative model–data mismatches in summer 2020.
To investigate the influence of inadequate point source modeling on the a posteriori area source ffCO2 emissions, we use two different ΔCO-based ΔffCO2 inversion setups: (1) an inversion with fixed point source emissions (“INV_fix”) and (2) an inversion with adjustable point source emissions (“INV_adj”). The first inversion setup corresponds to the inversion described in Sect. 2. It optimizes the flat a priori area source emissions by using fixed monthly point source emissions. The second inversion setup optimizes both the flat a priori area source emissions and the monthly a priori point source emissions. Thus, the point source emissions from the respective energy production sector and industry sector get the same temporal (i.e., “Filt3T” in CarboScope notation; see Sect. 2.2.6) and spatial (i.e., one spatial scaling factor) degrees of freedom as the area source emissions. Ideally, both inversion setups should lead to the same a posteriori area source emissions, meaning that the modeling of the better-known point source emissions has no influence on the area source emission estimates. Obviously, this is not the case. If the observed and modeled hourly ΔffCO2 concentrations (i.e., the model–data mismatches) are not averaged over a certain period of time, the INV_fix inversion leads to much lower area source emissions estimates than the INV_adj inversion (see cyan curves in Fig. C1). For individual seasons, e.g., in summer 2020, the differences are larger than 150 %. Thus, the INV_fix inversion tends to decrease the area source emissions to compensate for an inadequate modeling of the (fixed) point source emissions. This indicates that the transport model (even with the VSI approach) and/or the TNO emission inventory seems to overestimate the contributions from point sources at the Heidelberg observation site for individual hours.
The averaging over one afternoon (magenta curve in Fig. C1) only leads to minor improvements; there are still deviations larger than 100 % in summer 2020. In contrast, the averaging interval of 1 week (blue curve) limits the largest deviations in summer 2020 to below 30 %. Averaged over the 2 years of 2019 and 2020, these deviations between the INV_fix and INV_adj a posteriori area source emissions are less than 10 %. A monthly averaging interval (pink curve) further reduces the deviations to below 20 % in summer 2020.
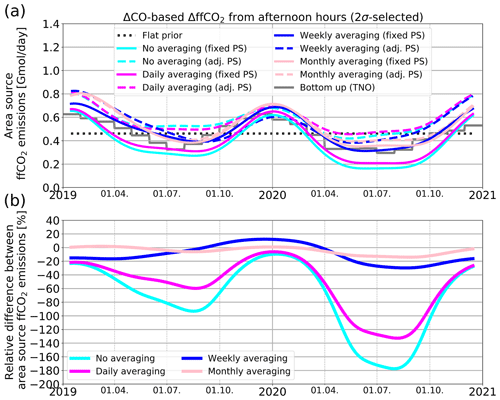
Figure C1(a) Area source ffCO2 emissions in the near field (blue outlined area in Fig. 1b) of Heidelberg. Shown are the results of the ΔCO-based ΔffCO2 inversion with fixed point sources (solid lines, “fixed PS”) and adjustable point sources (dashed lines, “adj. PS”) for different averaging intervals ranging from no averaging at all (cyan) to daily averaging of the 5 h (11:00–16:00 UTC) of each afternoon (magenta) and weekly (blue) and monthly (pink) averaging. All a posteriori results correspond to a 150 % prior uncertainty. The flat a priori emissions and the bottom-up emissions are shown as a reference in black and gray, respectively. Panel (b) shows the relative differences (fixed PS minus adj. PS) between the a posteriori area source ffCO2 emissions of the inversion runs with adjustable and fixed point source emissions (in %).
We also investigate if the sum of the a posteriori area source and point source emissions is similar for the INV_fix and the INV_adj inversion runs (see Fig. C2). If no averaging or only a daily averaging is applied, the INV_adj run leads to up to 50 % lower total (i.e., area plus point source) ffCO2 emissions than the INV_fix run. This again shows that the point source emissions are strongly reduced in the INV_adj inversion run. A weekly averaging (blue curve) restricts the relative differences between INV_fix and INV_adj to below ca. 20 % if the first and the last 2 months of the 2-year period are disregarded. The monthly averaging again shows the smallest differences (below 10 %) between the INV_fix and the INV_adj run.
Figure C3 shows the comparison between the modeled ΔffCO2 concentrations based on the INV_fix and INV_adj a posteriori emissions and the ΔCO-based ΔffCO2 observations if a weekly averaging is applied (i.e., all hourly entries within 1 week are averaged in the model–data mismatch vector). The mean bias and the standard deviation between weekly averaged observed and modeled ΔffCO2 are very similar for both inversion runs (0.10±1.89 ppm in the case of INV_fix and 0.13±1.80 ppm in the case of INV_adj). For comparison, the flat prior emissions lead to a mean bias of 0.65±2.23 ppm. Hence, there are no significant changes in the fits to the observational data when additional degrees of freedom are introduced for the point source emissions.
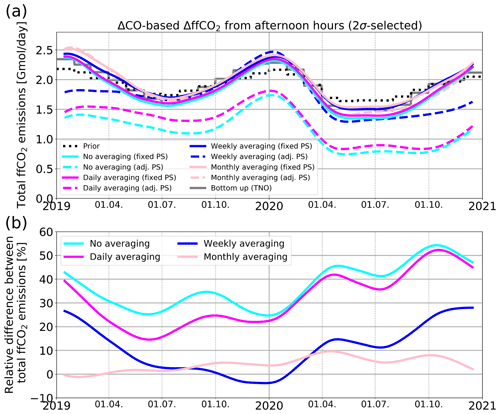
Figure C2(a) Area plus point source (i.e., “total”) ffCO2 emissions in the near field (blue outlined area in Fig. 1b) of Heidelberg. Shown are the results of the ΔCO-based ΔffCO2 inversion with fixed point sources (solid lines, “fixed PS”) and adjustable point sources (dashed lines, “adj. PS”) for different averaging intervals ranging from no averaging at all (cyan) to daily averaging of the 5 h (11:00–16:00 UTC) of each afternoon (magenta) and weekly (blue) and monthly (pink) averaging. All a posteriori results correspond to a 150 % prior uncertainty. The a priori emissions and the bottom-up emissions are shown as a reference in black and gray, respectively. Panel (b) shows the relative differences (fixed PS minus adj. PS) between the a posteriori total ffCO2 emissions of the inversion runs with adjustable and fixed point source emissions (in %).
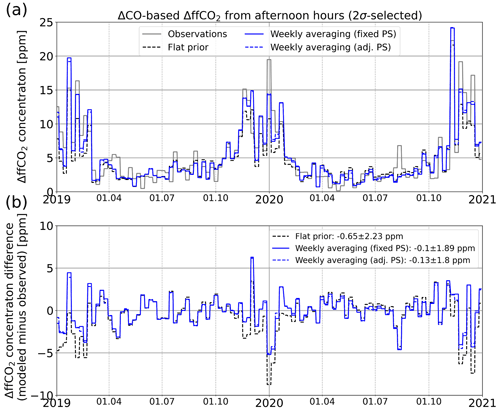
Figure C3Comparison between the weekly averaged observed ΔCO-based ΔffCO2 concentrations (gray) and the modeled ΔffCO2 concentrations based on the flat prior (black) and the a posteriori emissions with a prior uncertainty of 150 % (blue). Shown are the results for the standard INV_fix inversion setup with fixed point source emissions (solid) and the INV_adj inversion setup with adjustable point source emissions (dashed). In both inversion setups, the hourly entries of the model–data mismatch vector within 1 week were averaged.
We used the 14C- and ΔCO-based ΔffCO2 data published with Maier et al. (2024, https://doi.org/10.5194/egusphere-2023-1237). They can be found in Maier et al. (2023b, https://doi.org/10.11588/data/GRSSBN).
FM designed the study with input from all co-authors. FM performed the inverse modeling and processed the inversion results. CR helped with applying the CarboScope inversion framework for the Rhine Valley domain. CG modeled the alternative Rhine Valley ΔffCO2 background with emissions based on EDGAR. The various inversion results were discussed by all authors. FM wrote the manuscript with help from all co-authors.
At least one of the (co-)authors is a member of the editorial board of Atmospheric Chemistry and Physics. The peer-review process was guided by an independent editor, and the authors also have no other competing interests to declare.
Publisher’s note: Copernicus Publications remains neutral with regard to jurisdictional claims made in the text, published maps, institutional affiliations, or any other geographical representation in this paper. While Copernicus Publications makes every effort to include appropriate place names, the final responsibility lies with the authors.
We are grateful to the TNO staff at the Department of Climate, Air and Sustainability in Utrecht for providing the emission inventory as well as to Julia Marshall and Michał Gałkowski for computing and processing the high-resolution WRF meteorology for the Rhine Valley.
This research has been supported by the German Weather Service (DWD), the ICOS Research Infrastructure, and VERIFY (grant no. 776810, EU Horizon 2020 framework). The ICOS Central Radiocarbon Laboratory is funded by the German Federal Ministry of Transport and Digital Infrastructure.
This paper was edited by Abhishek Chatterjee and reviewed by Jocelyn Turnbull and John Miller.
Andres, R. J., Boden, T. A., Bréon, F.-M., Ciais, P., Davis, S., Erickson, D., Gregg, J. S., Jacobson, A., Marland, G., Miller, J., Oda, T., Olivier, J. G. J., Raupach, M. R., Rayner, P., and Treanton, K.: A synthesis of carbon dioxide emissions from fossil-fuel combustion, Biogeosciences, 9, 1845–1871, https://doi.org/10.5194/bg-9-1845-2012, 2012.
Basu, S., Miller, J. B., and Lehman, S.: Separation of biospheric and fossil fuel fluxes of CO2 by atmospheric inversion of CO2 and 14CO2 measurements: Observation System Simulations, Atmos. Chem. Phys., 16, 5665–5683, https://doi.org/10.5194/acp-16-5665-2016, 2016.
Basu, S., Lehman, S. J., Miller, J. B., Andrews, A. E., Sweeney, C., Gurney, K. R., Xue, X., Southon, J., and Tans, P. P.: Estimating US fossil fuel CO2 emissions from measurements of 14C in atmospheric CO2, P. Natl. Acad. Sci. USA, 117, 13300–13307, https://doi.org/10.1073/pnas.1919032117, 2020.
Bergamaschi, P., Danila, A., Weiss, R. F., Ciais, P., Thompson, R. L., Brunner, D., Levin, I., Meijer, Y., Chevallier, F., Janssens-Maenhout, G., Bovensmann, H., Crisp, D., Basu, S., Dlugokencky, E., Engelen, R., Gerbig, C., Günther, D., Hammer, S., Henne, S., Houweling, S., Karstens, U., Kort, E., Maione, M., Manning, A. J., Miller, J., Montzka, S., Pandey, S., Peters, W., Peylin, P., Pinty, B., Ramonet, M., Reimann, S., Röckmann, T., Schmidt, M., Strogies, M., Sussams, J., Tarasova, O., van Aardenne, J., Vermeulen, A. T., and Vogel, F.: Atmospheric monitoring and inverse modelling for verification of greenhouse gas inventories, EUR 29276 EN, Publications Office of the European Union, Luxembourg, JRC111789, https://doi.org/10.2760/759928, ISBN 978-92-79-88938-7, 2018.
Boschetti, F., Thouret, V., Maenhout, G. J., Totsche, K. U., Marshall, J., and Gerbig, C.: Multi-species inversion and IAGOS airborne data for a better constraint of continental-scale fluxes, Atmos. Chem. Phys., 18, 9225–9241, https://doi.org/10.5194/acp-18-9225-2018, 2018.
Ciais, P., Crisp, D., Denier Van Der Gon, H., Engelen, R., Heimann, M., Janssens-Maenhout, G., Rayner, P., and Scholze, M.: Towards a European Operational Observing System to Monitor Fossil CO2 Emissions. Final Report from the Expert Group, European Commission, ISBN 978-92-79-53482-9, https://doi.org/10.2788/350433, 2015.
Dellaert, S., Super, I., Visschedijk, A., and Denier van der Gon, H.: High resolution scenarios of CO2 and CO emissions, CHE deliverable D4.2, https://www.che-project.eu/sites/default/files/2019-05/CHE-D4-2-V1-0.pdf (last access: 28 March 2023), 2019.
Denier van der Gon, H., Kuenen, J., Boleti, E., Maenhout, G., Crippa, M., Guizzardi, D., Marshall, J., and Haussaire, J.: Emissions and natural fluxes Dataset, CHE deliverable D2.3, https://www.che-project.eu/sites/default/files/2019-02/CHE-D2-3-V1-1.pdf (last access: 28 March 2023), 2019.
Duren, R. and Miller, C.: Measuring the carbon emissions of megacities, Nat. Clim. Change, 2, 560–562, https://doi.org/10.1038/nclimate1629, 2012.
Friedlingstein, P., O'Sullivan, M., Jones, M. W., Andrew, R. M., Gregor, L., Hauck, J., Le Quéré, C., Luijkx, I. T., Olsen, A., Peters, G. P., Peters, W., Pongratz, J., Schwingshackl, C., Sitch, S., Canadell, J. G., Ciais, P., Jackson, R. B., Alin, S. R., Alkama, R., Arneth, A., Arora, V. K., Bates, N. R., Becker, M., Bellouin, N., Bittig, H. C., Bopp, L., Chevallier, F., Chini, L. P., Cronin, M., Evans, W., Falk, S., Feely, R. A., Gasser, T., Gehlen, M., Gkritzalis, T., Gloege, L., Grassi, G., Gruber, N., Gürses, Ö., Harris, I., Hefner, M., Houghton, R. A., Hurtt, G. C., Iida, Y., Ilyina, T., Jain, A. K., Jersild, A., Kadono, K., Kato, E., Kennedy, D., Klein Goldewijk, K., Knauer, J., Korsbakken, J. I., Landschützer, P., Lefèvre, N., Lindsay, K., Liu, J., Liu, Z., Marland, G., Mayot, N., McGrath, M. J., Metzl, N., Monacci, N. M., Munro, D. R., Nakaoka, S.-I., Niwa, Y., O'Brien, K., Ono, T., Palmer, P. I., Pan, N., Pierrot, D., Pocock, K., Poulter, B., Resplandy, L., Robertson, E., Rödenbeck, C., Rodriguez, C., Rosan, T. M., Schwinger, J., Séférian, R., Shutler, J. D., Skjelvan, I., Steinhoff, T., Sun, Q., Sutton, A. J., Sweeney, C., Takao, S., Tanhua, T., Tans, P. P., Tian, X., Tian, H., Tilbrook, B., Tsujino, H., Tubiello, F., van der Werf, G. R., Walker, A. P., Wanninkhof, R., Whitehead, C., Willstrand Wranne, A., Wright, R., Yuan, W., Yue, C., Yue, X., Zaehle, S., Zeng, J., and Zheng, B.: Global Carbon Budget 2022, Earth Syst. Sci. Data, 14, 4811–4900, https://doi.org/10.5194/essd-14-4811-2022, 2022.
Gamnitzer, U., Karstens, U., Kromer, B., Neubert, R. E. M., Meijer, H. A. J., Schroeder, H., and Levin, I.: Carbon monoxide: A quantitative tracer for fossil fuel CO2?, J. Geophys. Res., 111, D22302, https://doi.org/10.1029/2005JD006966, 2006.
Graven, H., Fischer, M. L., Lueker, T., Jeong, S., Guilderson, T. P., Keeling, R. F., Bambha, R., Brophy, K., Callahan, W., Cui, X., Frankenberg, C., Gurney, K. R., LaFranchi, B. W., Lehman, S. J., Michelsen, H., Miller, J. B., Newman, S., Paplawsky, W., Parazoo, N. C., Sloop, C., and Walker, S. J.: Assessing fossil fuel CO2 emissions in California using atmospheric observations and models, Environ. Res. Lett., 13, 065007, https://doi.org/10.1088/1748-9326/aabd43, 2018.
Heiskanen, J., Brümmer, C., Buchmann, N., Calfapietra, C., Chen, H., Gielen, B., Gkritzalis, T., Hammer, S., Hartman, S., Herbst, M., Janssens, I. A., Jordan, A., Juurola, E., Karstens, U., Kasurinen, V., Kruijt, B., Lankreijer, H., Levin, I., Linderson, M., Loustau, D., Merbold, L., Myhre, C. L., Papale, D., Pavelka, M., Pilegaard, K., Ramonet, M., Rebmann, C., Rinne, J., Rivier, L., Saltikoff, E., Sanders, R., Steinbacher, M., Steinhoff, T., Watson, A., Vermeulen, A. T., Vesala, T., Vítková, G., and Kutsch, W.: The Integrated Carbon Observation System in Europe, B. Am. Meteorol. Soc., 103, E855–E872, https://doi.org/10.1175/BAMS-D-19-0364.1, 2022.
Hersbach, H., Bell, B., Berrisford, P., Hirahara, S., Horányi, A., Muñoz-Sabater, J., Nicolas, J., Peubey, C., Radu, R., Schepers, D., Simmons, A., Soci, C., Abdalla, S., Abellan, X., Balsamo, G., Bechtold, P., Biavati, G., Bidlot, J., Bonavita, M., De Chiara, G., Dahlgren, P., Dee, D., Diamantakis, M., Dragani, R., Flemming, J., Forbes, R., Fuentes, M., Geer, A., Haimberger, L., Healy, S., Hogan, R. J., Hólm, E., Janisková, M., Keeley, S., Laloyaux, P., Lopez, P., Lupu, C., Radnoti, G., de Rosnay, P., Rozum, I., Vamborg, F., Villaume, S., and Thépaut, J.-N.: The ERA5 global reanalysis, Q. J. Roy. Meteor. Soc., 146, 1999–2049, https://doi.org/10.1002/qj.3803, 2020.
Janssens-Maenhout, G., Crippa, M., Guizzardi, D., Muntean, M., Schaaf, E., Dentener, F., Bergamaschi, P., Pagliari, V., Olivier, J. G. J., Peters, J. A. H. W., van Aardenne, J. A., Monni, S., Doering, U., Petrescu, A. M. R., Solazzo, E., and Oreggioni, G. D.: EDGAR v4.3.2 Global Atlas of the three major greenhouse gas emissions for the period 1970–2012, Earth Syst. Sci. Data, 11, 959–1002, https://doi.org/10.5194/essd-11-959-2019, 2019.
Jiang, F., Chen, J. M., Zhou, L., Ju, W., Zhang, H., Machida, T., Ciais, P., Peters, W., Wang, H., Chen, B., Liu, L., Zhang, C., Matsueda, H., and Sawa, Y.: A comprehensive estimate of recent carbon sinks in China using both top-down and bottom-up approaches, Sci. Rep.-UK, 6, 22130, https://doi.org/10.1038/srep22130, 2016.
Jones, M. W., Andrew, R. M., Peters, G. P., Janssens-Maenhout, G., De-Gol, A. J., Dou, X., Liu, Z., Ciais, P., Patra, P. K., Chevallier, F., and Le Quéré, C.: Gridded fossil CO2 emissions and related O2 combustion consistent with national inventories 1959–2020, Version GCP-GridFEDv2021.2, Zenodo [data set], https://doi.org/10.5281/zenodo.5565199, 2021.
Kountouris, P., Gerbig, C., Rödenbeck, C., Karstens, U., Koch, T. F., and Heimann, M.: Technical Note: Atmospheric CO2 inversions on the mesoscale using data-driven prior uncertainties: methodology and system evaluation, Atmos. Chem. Phys., 18, 3027–3045, https://doi.org/10.5194/acp-18-3027-2018, 2018.
Kuenen, J. J. P., Visschedijk, A. J. H., Jozwicka, M., and Denier van der Gon, H. A. C.: TNO-MACC_II emission inventory; a multi-year (2003–2009) consistent high-resolution European emission inventory for air quality modelling, Atmos. Chem. Phys., 14, 10963–10976, https://doi.org/10.5194/acp-14-10963-2014, 2014.
Levin, I. and Karstens, U.: Inferring high-resolution fossil fuel CO2 records at continental sites from combined 14CO2 and CO observations, Tellus B, 59, 245–250, https://doi.org/10.1111/j.1600-0889.2006.00244.x, 2007.
Levin, I., Kromer, B., Schmidt, M., and Sartorius, H.: A novel approach for independent budgeting of fossil fuel CO2 over Europe by 14CO2 observations, Geophys. Res. Lett., 30, 2194, https://doi.org/10.1029/2003GL018477, 2003.
Levin, I., Hammer, S., Eichelmann, E., and Vogel, F.: Verification of greenhouse gas emission reductions: The prospect of atmospheric monitoring in polluted areas, Philos. T. Roy. Soc. A, 369, 1906–1924, https://doi.org/10.1098/rsta.2010.0249, 2011.
Lin, J. C., Gerbig, C., Wofsy, S. C., Andrews, A. E., Daube, B. C., Davis, K. J., and Grainger, C. A.: A near-field tool for simulating the upstream influence of atmospheric observations: The Stochastic Time-Inverted Lagrangian Transport (STILT) model, J. Geophys. Res., 108, 4493, https://doi.org/10.1029/2002JD003161, 2003.
Liu, J., Baskaran, L., Bowman, K., Schimel, D., Bloom, A. A., Parazoo, N. C., Oda, T., Carroll, D., Menemenlis, D., Joiner, J., Commane, R., Daube, B., Gatti, L. V., McKain, K., Miller, J., Stephens, B. B., Sweeney, C., and Wofsy, S.: Carbon Monitoring System Flux Net Biosphere Exchange 2020 (CMS-Flux NBE 2020), Earth Syst. Sci. Data, 13, 299–330, https://doi.org/10.5194/essd-13-299-2021, 2021.
Maier, F., Gerbig, C., Levin, I., Super, I., Marshall, J., and Hammer, S.: Effects of point source emission heights in WRF–STILT: a step towards exploiting nocturnal observations in models, Geosci. Model Dev., 15, 5391–5406, https://doi.org/10.5194/gmd-15-5391-2022, 2022.
Maier, F., Levin, I., Gachkivskyi, M., Rödenbeck, C., and Hammer, S.: Estimating regional fossil-fuel CO2 concentrations from 14CO2 observations: Challenges and uncertainties, Philos. T. Roy. Soc. A., 381, 20220203, https://doi.org/10.1098/rsta.2022.0203, 2023a.
Maier, F., Levin, I., Hammer, S., Conil, S., and Preunkert, S.: 14C-based ΔffCO2 estimates for Heidelberg and OPE and input data for the Rhine Valley ffCO2 inversion (2019-2020), heiDATA [data set], https://doi.org/10.11588/data/GRSSBN, 2023b.
Maier, F. M., Levin, I., Conil, S., Gachkivskyi, M., Denier van der Gon, H., and Hammer, S.: Uncertainty in continuous ΔCO-based ΔffCO2 estimates derived from 14C flask and bottom-up ΔCO ΔffCO2 ratios, Atmos. Chem. Phys., 24, 8205–8223, https://doi.org/10.5194/acp-24-8205-2024, 2024.
Monteil, G., Broquet, G., Scholze, M., Lang, M., Karstens, U., Gerbig, C., Koch, F.-T., Smith, N. E., Thompson, R. L., Luijkx, I. T., White, E., Meesters, A., Ciais, P., Ganesan, A. L., Manning, A., Mischurow, M., Peters, W., Peylin, P., Tarniewicz, J., Rigby, M., Rödenbeck, C., Vermeulen, A., and Walton, E. M.: The regional European atmospheric transport inversion comparison, EUROCOM: first results on European-wide terrestrial carbon fluxes for the period 2006–2015, Atmos. Chem. Phys., 20, 12063–12091, https://doi.org/10.5194/acp-20-12063-2020, 2020.
Nakanishi, M. and Niino, H.: Development of an improved turbulence closure model for the atmospheric boundary layer, J. Meteorol. Soc. Jpn. Ser. II, 87, 895–912, 2009.
Munassar, S., Rödenbeck, C., Koch, F.-T., Totsche, K. U., Gałkowski, M., Walther, S., and Gerbig, C.: Net ecosystem exchange (NEE) estimates 2006–2019 over Europe from a pre-operational ensemble-inversion system, Atmos. Chem. Phys., 22, 7875–7892, https://doi.org/10.5194/acp-22-7875-2022, 2022.
Munassar, S., Monteil, G., Scholze, M., Karstens, U., Rödenbeck, C., Koch, F.-T., Totsche, K. U., and Gerbig, C.: Why do inverse models disagree? A case study with two European CO2 inversions, Atmos. Chem. Phys., 23, 2813–2828, https://doi.org/10.5194/acp-23-2813-2023, 2023.
Nehrkorn, T., Eluszkiewicz, J., Wofsy, S. C., Lin, J. C., Gerbig, C., Longo, M., and Freitas, S.: Coupled weather research and forecasting–stochastic time-inverted lagrangian transport (WRF–STILT) model, Meteorol. Atmos. Phys., 107, 51–64, https://doi.org/10.1007/s00703-010-0068-x, 2010.
Newsam, G. N. and Enting, I. G.: Inverse problems in atmospheric constituent studies: I. Determination of surface sources under a diffusive transport approximation, Inverse Probl., 4, 1037–1054, 1988.
Peylin, P., Law, R. M., Gurney, K. R., Chevallier, F., Jacobson, A. R., Maki, T., Niwa, Y., Patra, P. K., Peters, W., Rayner, P. J., Rödenbeck, C., van der Laan-Luijkx, I. T., and Zhang, X.: Global atmospheric carbon budget: results from an ensemble of atmospheric CO2 inversions, Biogeosciences, 10, 6699–6720, https://doi.org/10.5194/bg-10-6699-2013, 2013.
Rödenbeck, C.: Estimating CO2 sources and sinks from atmospheric mixing ratio measurements using a global inversion of atmospheric transport, Tech. Rep. 6, Max Planck Institute for Biogeochemistry, Jena, Germany, ISSN 1615-7400, http://www.bgc-jena.mpg.de/CarboScope/s/tech_report6.pdf (last access: 24 January 2024), 2005.
Rödenbeck, C., Houweling, S., Gloor, M., and Heimann, M.: CO2 flux history 1982–2001 inferred from atmospheric data using a global inversion of atmospheric transport, Atmos. Chem. Phys., 3, 1919–1964, https://doi.org/10.5194/acp-3-1919-2003, 2003.
Rödenbeck, C., Gerbig, C., Trusilova, K., and Heimann, M.: A two-step scheme for high-resolution regional atmospheric trace gas inversions based on independent models, Atmos. Chem. Phys., 9, 5331–5342, https://doi.org/10.5194/acp-9-5331-2009, 2009.
Rödenbeck, C., Zaehle, S., Keeling, R., and Heimann, M.: How does the terrestrial carbon exchange respond to inter-annual climatic variations? A quantification based on atmospheric CO2 data, Biogeosciences, 15, 2481–2498, https://doi.org/10.5194/bg-15-2481-2018, 2018.
Shiga, Y. P., Michalak, A. M., Gourdji, S. M., Mueller, K. L., and Yadav, V.: Detecting fossil fuel emissions patterns from subcontinental regions using North American in situ CO2 measurements, Geophys. Res. Lett., 41, 4381–4388, https://doi.org/10.1002/2014GL059684, 2014.
Skamarock, W. C., Klemp, J. B., Dudhia, J., Gill, D. O., Barker, D. M., Wang, W., and Powers, J. G.: A description of the Advanced Research WRF version 3, NCAR Technical note-475+STR, 2008.
Suess, H.: Radiocarbon Concentration in Modern Wood, Science, 122, 415–417, https://doi.org/10.1126/science.122.3166.415.b, 1955.
Super, I., Dellaert, S. N. C., Visschedijk, A. J. H., and Denier van der Gon, H. A. C.: Uncertainty analysis of a European high-resolution emission inventory of CO2 and CO to support inverse modelling and network design, Atmos. Chem. Phys., 20, 1795–1816, https://doi.org/10.5194/acp-20-1795-2020, 2020.
Turnbull, J. C., Miller, J. B., Lehman, S. J., Tans, P. P., Sparks, R. J., and Southon, J.: Comparison of 14CO2, CO, and SF6 as tracers for recently added fossil fuel CO2 in the atmosphere and implications for biological CO2 exchange, Geophys. Res. Lett., 33, L01817, https://doi.org/10.1029/2005GL024213, 2006.
Van Der Laan, S., Karstens, U., Neubert, R. E. M., Van Der Laan-Luijkx, I. T., and Meijer, H. A. J.: Observation-based estimates of fossil fuel-derived CO2 emissions in the Netherlands using Δ14C, CO and 222Radon, Tellus B, 62, 389–402, https://doi.org/10.1111/j.1600-0889.2010.00493.x, 2010.
Vogel, F., Hammer, S., Steinhof, A., Kromer, B., and Levin, I.: Implication of weekly and diurnal 14C calibration on hourly estimates of CO-based fossil fuel CO2 at a moderately polluted site in southwestern Germany, Tellus B, 62, 512–520, https://doi.org/10.1111/j.1600-0889.2010.00477.x, 2010.
Wang, Y., Broquet, G., Ciais, P., Chevallier, F., Vogel, F., Wu, L., Yin, Y., Wang, R., and Tao, S.: Potential of European 14CO2 observation network to estimate the fossil fuel CO2 emissions via atmospheric inversions, Atmos. Chem. Phys., 18, 4229–4250, https://doi.org/10.5194/acp-18-4229-2018, 2018.
- Abstract
- Introduction
- Methods
- Results
- Discussion and conclusions
- Appendix A: Description of the CarboScope inversion framework
- Appendix B: Fits to the flask observations
- Appendix C: Impact of the averaging interval and additional degrees of freedom for the point sources emissions
- Appendix D: Anomaly in the seasonal cycle of the area source ffCO2 emissions in 2020
- Appendix E: Hypothetical seasonal cycle in the ΔCOffCO2 ratios
- Appendix F: Comparison between two modeled Rhine Valley ΔffCO2 backgrounds
- Appendix G: Comparison between TNO and other emission inventories
- Data availability
- Author contributions
- Competing interests
- Disclaimer
- Acknowledgements
- Financial support
- Review statement
- References
- Abstract
- Introduction
- Methods
- Results
- Discussion and conclusions
- Appendix A: Description of the CarboScope inversion framework
- Appendix B: Fits to the flask observations
- Appendix C: Impact of the averaging interval and additional degrees of freedom for the point sources emissions
- Appendix D: Anomaly in the seasonal cycle of the area source ffCO2 emissions in 2020
- Appendix E: Hypothetical seasonal cycle in the ΔCOffCO2 ratios
- Appendix F: Comparison between two modeled Rhine Valley ΔffCO2 backgrounds
- Appendix G: Comparison between TNO and other emission inventories
- Data availability
- Author contributions
- Competing interests
- Disclaimer
- Acknowledgements
- Financial support
- Review statement
- References