the Creative Commons Attribution 4.0 License.
the Creative Commons Attribution 4.0 License.
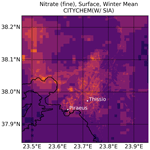
Analysis of secondary inorganic aerosols over the greater Athens area using the EPISODE–CityChem source dispersion and photochemistry model
Matthias Karl
Kim A. Weiss
Dimitris Karagiannis
Eleni Athanasopoulou
Anastasia Kakouri
Aikaterini Bougiatioti
Eleni Liakakou
Iasonas Stavroulas
Georgios Papangelis
Georgios Grivas
Despina Paraskevopoulou
Orestis Speyer
Nikolaos Mihalopoulos
Evangelos Gerasopoulos
Secondary inorganic aerosols (SIAs) are major components of fine particulate matter (PM2.5), having substantial implications for climate and air quality in an urban environment. In this study, a state-of-the-art thermodynamic model has been coupled to the source dispersion and photochemistry city-scale chemistry transport model EPISODE–CityChem, which is able to simulate pollutants at a horizontal resolution of 100 m×100 m, to determine the equilibrium between the inorganic gas and aerosol phases over the greater Athens area, Greece, for the year 2019. In agreement with in situ observations, sulfate () is calculated to have the highest annual mean surface concentration (2.15 ± 0.88 µg m−3) among SIAs in the model domain, followed by ammonium (; 0.58 ± 0.14 µg m−3) and fine nitrate (; 0.24 ± 0.22 µg m−3). Simulations denote that formation strongly depends on the local nitrogen oxide emissions, along with the ambient temperature, the relative humidity, and the photochemical activity. Additionally, we show that anthropogenic combustion sources may have an important impact on the formation in an urban area. During the cold period, the combined effect of decreased temperature in the presence of non-sea-salt potassium favors the partitioning of HNO3 in the aerosol phase in the model, raising the formation in the area. Overall, this work highlights the significance of atmospheric composition and the local meteorological conditions for the equilibrium distribution of nitrogen-containing semi-volatile compounds and the acidity of inorganic aerosols, especially in urban areas where atmospheric trace elements from natural and anthropogenic sources coexist.
- Article
(8934 KB) - Full-text XML
-
Supplement
(2030 KB) - BibTeX
- EndNote
Fine particulate matter (PM2.5) impacts the planet's radiative balance (IPCC, 2014) and severely affects air quality (WHO, 2021). Such effects strongly depend on the chemical and physical properties of PM2.5, e.g., shape, size distribution, and hygroscopicity. Among other particulate pollutants, like organic matter, elemental carbon, dust particles, and sea spray, secondary inorganic aerosols (SIAs) are identified as a critical PM2.5 fraction in an urban atmosphere. SIAs are formed in the atmosphere via gas-to-particle conversion of precursors of anthropogenic (e.g., coal combustion, biomass burning, vehicular), biomass burning, and natural (e.g., marine, soil, lightning, volcanoes) origin, with sulfate (), nitrate (), and ammonium () being the three major SIA species. However, primary emissions, such as those from residential combustion, have been found to increase their atmospheric abundance (Zhang et al., 2023). SIAs may further contribute significantly to air quality degradation; for example, sulfates can reduce visibility and contribute to acid rain (Liu et al., 2019), affecting forest and aquatic ecosystems (Driscoll et al., 2003, 2016) and damaging building materials (Ozga et al., 2013). On the other hand, SIAs are also linked to increased asthma exacerbation (Sarnat et al., 2015) and cardiovascular hospitalization (Basagaña et al., 2015) and can contribute to human mortality via the enhancement of fine particulate air pollution (Franklin et al., 2008; Pope et al., 2020). Focusing, in particular, on polluted environments, Nenes et al. (2020) indicated that atmospheric acidity, aerosol liquid water content (LWC), and meteorological conditions (temperature and relative humidity) are the main parameters that control SIA formation sensitivity, depending on the availability of ammonia (NH3) and nitric acid (HNO3).
Sulfates are primarily formed by the oxidation of sulfur dioxide (SO2) as a byproduct of fossil fuel burning, such as coal and oil in power plants and industrial processes, as well as residual oil burning in shipping. In the gas phase, the SO2 homogeneous oxidation via OH radicals during daytime strongly depends on temperature, while the heterogeneous oxidation of SO2 via may proceed in aqueous media such as cloud liquid water and in-cloud scavenging processes (Seinfeld and Pandis, 2006), enhanced in the presence of transition metal ions (e.g., Mn2+ and Fe3+; Alexander et al., 2009), and/or on aerosol surfaces such as dust aerosols (Wang et al., 2022). Aqueous-phase processes are nevertheless found to be responsible for about two-thirds of the total sulfate production in the global troposphere (e.g., Myriokefalitakis et al., 2022). Overall, it is well established that the amounts of precursor gases and oxidants, the cloud cover, the amount of available surface area, and meteorological factors such as temperature and relative humidity all affect the rate of SO2 conversion to sulfate (Liu et al., 2021).
Particulate nitrate formation depends on the emissions of nitrogen oxides (NOX) from traffic, power plants, and industrial activities, along with the gas-phase concentrations of ammonia (NH3) as emitted by agricultural activities, waste management, industrial processes, and the biosphere. The formation of is, however, a rather complex process and is strongly dependent on interactions of acidity, relative humidity, and temperature (Guo et al., 2017b; Nenes et al., 2020), with the partitioning of HNO3 to the particulate phase enhanced under lower ambient temperatures (Guo et al., 2017b). Nevertheless, since ammonium nitrate (NH4NO3) is formed when NH3 is in excess upon the formation of ammonium sulfate and ammonium bisulfate, such an equilibrium, besides the availability of NH3, also depends on the concentration of sulfuric acid (H2SO4). The relative humidity is, nevertheless, a key factor for SIA formation, determining the aerosol LWC and thus the aerosol acidity levels, which in turn impact the HNO3 partitioning in the aerosol phase. All in all, formation increases the aerosol LWC by lowering aerosol acidity via dilution and thus further promoting HNO3 partitioning into the particulate phase (Guo et al., 2017a).
Thermodynamic calculations (Clegg et al., 1998; Fountoukis and Nenes, 2007; Nenes et al., 1998) are considered to be the appropriate way of assessing non-linearities in an inorganic aerosol system and the behavior of a non-ideal liquid solution. Such methods can explicitly account for the equilibrium state of inorganic particles by calculating the gas- and particulate-phase fractions of the inorganics, along with the corresponding aerosol LWC and the respective levels of acidity (pH), both of which are very important for assessing the burden of the secondary inorganic PM fraction. The presence of non-volatile cations (NVCs) in thermodynamic calculations, however, has been shown to substantially affect the ion balance (Guo et al., 2018) and thus the partitioning of and species, especially in areas with abundant mineral dust and sea spray aerosols (Athanasopoulou et al., 2008, 2016), such as the Eastern Mediterranean. For example, calcium-containing dust particles may react with nitric acid to form calcium nitrate (Ca(NO3)2), significantly enhancing formation, especially when natural and anthropogenic particulate matter coexist. On the other hand, NVCs from combustion sources related to natural or anthropogenic activities may also critically impact the predictions of inorganic partitioning between the gas and the particulate phase in an urban environment (Cheng et al., 2022).
Regional chemistry transport models (CTMs) are promising tools for providing spatial information on environmental impacts occurring in the urban landscape. Although such modeling tools can successfully simulate the inorganic and organic components of atmospheric aerosols at the background scale (e.g., Brandt et al., 2003; Athanasopoulou et al., 2013; Zakoura and Pandis, 2018; Basla et al., 2022), they are not rather accurate in the representation of their spatial distribution in proximity to point and/or line sources (Santiago et al., 2022). On the contrary, urban-scale CTMs have the advantage of mapping air pollution levels in high resolution (≤1 km) (e.g., Hurley et al., 2005; Karl et al., 2019; Denby et al., 2020), although critical processes related to air quality, such as aerosol representation and its chemical speciation, may still be simplistically represented due to the high computational load of such modeling systems. Indeed, urban-scale CTMs usually estimate the bulk mass of particulate matter (PM2.5 and/or PM10) without having incorporated their chemical interactions with gaseous pollutants in the atmosphere, like in the case of inorganic chemical components in the aqueous and/or solid state. Such a simplistic aerosol parameterization in local/city-scale models, which still remains a common approach, nevertheless represents the next challenging issue of multi-scale modeling (Sokhi et al., 2022).
Several regional-scale numerical simulations of atmospheric pollution have been performed over the Eastern Mediterranean area (Athanasopoulou et al., 2015; Fountoukis et al., 2011; Im et al., 2013; Kontos et al., 2021; Kushta et al., 2019; Liora et al., 2022), focusing in particular on the urban area of Athens, one of the most polluted cities in the EU. Most of these studies highlight the complex interactions among nitrogen and sulfur oxides of anthropogenic origin with sea salt and/or dust particles of natural origin over the area (Athanasopoulou et al., 2008; Karydis et al., 2016). These findings are also confirmed by several local observational studies (Bardouki et al., 2003; Dimitriou et al., 2021; Koçak et al., 2004, 2012; Stavroulas et al., 2021). On the other hand, recent city-scale modeling studies over Athens (Gratsea et al., 2021; Grivas et al., 2020; Lasne et al., 2023; Ramacher et al., 2021) clearly demonstrate the difficulties in simulating the inorganic constituents of atmospheric aerosols in the area, in particular at the intra-urban scale.
To tackle the above challenges in the context of multi-pollutant studies at a city-scale spatial resolution, we here develop a framework for fine aerosol thermodynamic calculations to better understand the SIA formation mechanisms in an urban environment. Section 2 provides an overview of the model, focusing mostly on the new developments applied in this study. In particular, we describe the aqueous-phase chemistry scheme used to simulate the atmospheric production in cloud droplets and the respective developments for the thermodynamic calculations of semi-volatile inorganics, along with a series of code updates currently applied in the model. In Sect. 3, we present the model-derived , , and surface concentrations and their evaluation against in situ observations. Finally, in Sect. 4, we discuss the simulated processes and summarize the implications of explicitly resolving the secondary formation of PM2.5 in a city-scale chemistry transport model for air quality, as well as the plans for future model development.
The numerical atmospheric model system used for this study is the chemistry transport model (CTM) EPISODE–CityChem (Karl et al., 2019), coupled here to atmospheric processes relevant to SIA formation. The model is applied over the greater Athens area (Greece), centered at the urban center (Fig. 1), horizontally covering a domain of 45 km×45 km (SW corner at 23.4° E, 37.8° N; 1 km×1 km cell size, with a sub-grid of 100 m) and vertically representing a 24-layer atmosphere up to 3.7 km. For the current study, simulations of air quality are performed for 2019. Three simulations have been conducted in this work to investigate uncertainties regarding the impact of SIA formation in the greater Athens area (GAA): (i) the base simulation where a complete chemistry/thermodynamic scheme is accounted for in the model (W/ SIA); (ii) a simulation where the secondary production of , , and is neglected (W/O SIA), focusing on the impact of long-range transport to SIA concentrations in the study area; and (iii) an extra simulation where the impact of NVC emissions related to domestic burning on the SIA formation is neglected (W/O Kbb), focusing thus on the importance of potassium (K+) on the equilibrium distribution of nitrogen-containing semi-volatile compounds. Finally, available in situ measurements for the area and year of study are used to assess the accuracy of model predictions and the efficiency of the performed advancements.
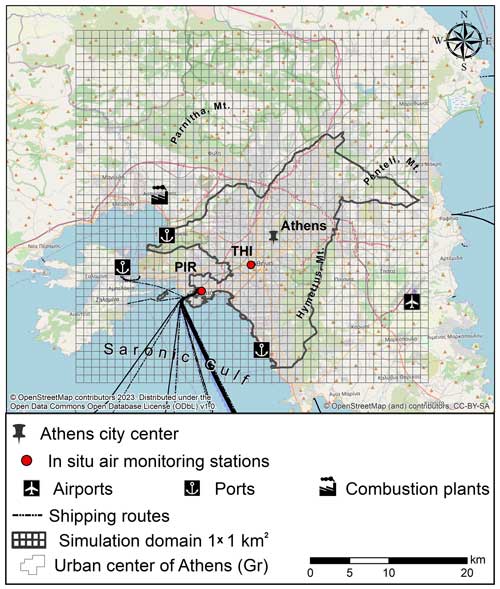
Figure 1Model domain of the greater Athens area, Greece. The gridded rectangles correspond to the air quality simulation domains, and the red dots correspond to the locations of the air quality monitoring sites of Thissio (THI) and Piraeus (PIR).
2.1 The EPISODE–CityChem model
EPISODE–CityChem is a city-scale CTM designed for treating complex atmospheric chemistry in urban areas and improving the representation of near-field dispersion. EPISODE is an Eulerian dispersion model developed by the Norwegian Institute for Air Research (Slørdal et al., 2007) to simulate pollutant dispersion at the city scale and microscale simultaneously. EPISODE consists of a 3-D Eulerian grid that interacts with a sub-grid Gaussian dispersion model for the dispersion of pollutants emitted from both line and point sources (Hamer et al., 2020), allowing for the retrieval of pollutant concentrations at the sub-grid scale in an urban area. The CityChem extension offers an explicit description of the gaseous reactive pollutants' photochemistry on the Eulerian grid, along with the dispersion close to point emission sources (e.g., industrial stacks) and line emission sources (open roads and streets). For this work, however, supplementary emissions are incorporated into the model domain (see Sect. 2.1.3) to enable a realistic simulation of SIA formation.
2.1.1 Gas-phase chemistry
EPISODE–CityChem has several schemes for solving the chemistry of the gas phase. For the standard model configuration, the updated chemistry scheme, EmChem09-HET, is applied on the coarse grid, which supports more than 100 reactions, including photodissociation, thermal, and heterogeneous reactions, and about 70 species. However, for this study, the well-documented Carbon Bond mechanism 2005 (CB05; Yarwood et al., 2005), along with more recent modifications (mCB05) as introduced by Williams et al. (2013, 2017), are applied. For comparison, we note that the mCB05 configuration here uses 50 tracers with roughly 134 reactions, respectively. The Kinetic PreProcessor (KPP) software (version 2.2.3) (Damian et al., 2002; Sandu and Sander, 2006) is employed to integrate the mCB05 gas-phase chemical mechanism for this work, based on the generated Rosenbrock solver. A more detailed description and evaluation of the mCB05–KPP implementation can be found in Myriokefalitakis et al. (2020).
Photodissociation rates were calculated inline using the Tropospheric Ultraviolet and Visible (TUV) radiation model (Lee-Taylor and Madronich, 2002). The TUV module calculates actinic flux and photolysis rates in each vertical layer within a 1-D column of the Eulerian grid. The implemented wavelength grid ranges from 170–735 nm with 138 wavelength bins; 31 photodissociation reactions (out of 113 available in TUV) were selected for the implemented TUV module, covering all relevant photolysis rates in the lower troposphere. Additionally, the model includes a light-dependent ground surface source of nitrous acid (HONO) on top of the respective direct emissions from vehicles (Karl and Ramacher, 2021). In more detail, the ground surface reaction producing HONO was parameterized according to Zhang et al. (2016) with a NO uptake coefficient (gamma) depending on the light intensity. That is, when light intensity is less than 400 W m−2, a gamma value of is used; otherwise, a factor of light intensity of 400 is used to scale the gamma value.
Pollutant concentrations in the sub-grid components (i.e., the Gaussian models for line and point source dispersion) are determined by the EP10-Plume scheme, which contains 10 compounds and 17 reactions of O3, NO, NO2, HNO3, CO, and formaldehyde (HCHO). In the line source sub-grid model, the simplified street canyon model (SSCM) is applied to calculate pollutant dispersion in street canyons, and in the point source sub-grid model, the WMPP (Weak Wind Open Road Model (WORM) Meteorological Pre-Processor) is integrated to calculate the wind speed at plume height. The rate of change in the concentrations of NO, NO2, and O3 in a street canyon is described in terms of the chemical reaction, direct emissions, and the background concentration. The mixing processes are parameterized via the mixing time, which corresponds to the typical residence time of pollutants in a street canyon. More details on the sub-grid photochemistry schemes of EPISODE–CityChem can be found in Karl et al. (2019).
2.1.2 Extensions to the aerosol scheme
EPISODE–CityChem is extended for this work with online calculations of the main inorganic aerosol components, , , and , in the presence of non-volatile cations from sea salt, dust, and domestic heating. For this, the aqueous-phase formation of in cloud droplets and the thermodynamic calculations, which determine the gas/particle partitioning of and , are explicitly considered in the model. Aqueous-phase oxidation of the dissolved SO2 via H2O2 and O3 in cloud droplets is added to the model. H2O2 produced in the gas phase can be rapidly dissolved in the liquid phase due to its high solubility, and the dissolved H2O2 reacts rapidly with the . Note that the dissociation of H2O2 is neglected here. SO2 oxidation in the aqueous phase is much faster than its gas-phase homogeneous oxidation via OH radicals, with the dissolved H2O2 being the most effective oxidant in the aqueous phase (Seinfeld and Pandis, 2006), especially when the solution becomes acidic. At a higher pH (pH>4), the dissolved SO2 oxidation by ozone (O3) tends to dominate the S(IV) (HSO, SO) oxidation, even under dark conditions. Although laboratory studies have shown that dissolved SO2 can also be oxidized in the presence of transition metals via other pathways (Harris et al., 2013), such reactions are not currently considered in our model. Lastly, the acidity of clouds is calculated using the electro-neutrality of strong acids (i.e., H2SO4, , HNO3, ) and , along with the dissociations of CO2, SO2, and NH3. The reaction rates and the constants used to calculate the sulfur chemistry in the model are presented in Table 1.
Table 1Reaction rates and constants used in EPISODE–CityChem for this study.
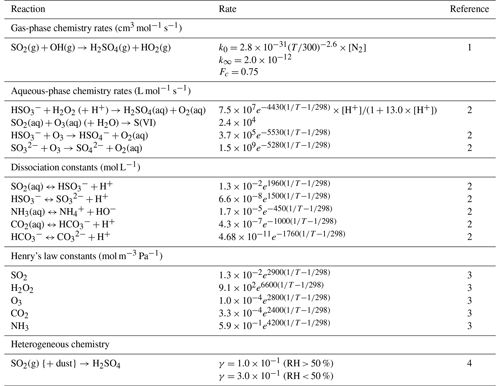
1 Atkinson et al. (2004). 2 Seinfeld and Pandis (2006). 3 Sander (2015). 4 Liao et al. (2003).
The ISORROPIA II thermodynamic equilibrium model (Fountoukis and Nenes, 2007) has been coupled to EPISODE–CityChem to calculate the gas/particle partitioning of inorganic aerosols. ISORROPIA II calculates the gas–liquid–solid equilibrium partitioning of the K+–Ca2+–Mg2+––Na+–––Cl−–H2O aerosol system, and it is applied here in the forward mode. NVCs from dust and sea salt aerosols are taken into account in the thermodynamic calculations considered here as a fraction of PM2.5 concentration. It is assumed here that constant mass ratios correspond to dust concentrations for Ca2+, Na+, K+, and Mg2+ of 1.2 %, 1.5 %, and 0.9 %, respectively (Sposito, 1989). Accordingly, for sea spray aerosols, mean mass fractions of 55.0 % for Cl−, 30.6 % for Na+, 7.7 % for , 3.7 % for Mg2+, 1.2 % for Ca2+, and 1.1 % for K+ are applied (Seinfeld and Pandis, 2006).
Inorganic particles can be solid and/or composed of an aqueous supersaturated solution. The assumption of aqueous aerosol for the acidity-sensitive semi-volatile inorganic species (e.g., and ) is found to perform quite well with a series of aerosol LWC observations when the relative humidity is above 40 % (Bougiatioti et al., 2016; Guo et al., 2015, 2017a). However, Cheng et al. (2022) recently indicated that although under conditions of high relative humidity, both assumptions may perform almost identically, the aqueous aerosol setup might perform better under conditions of high temperatures (>10 °C) and low relative humidity (<60 %), while the solid aerosol setup might perform better for lower temperatures. For this, the metastable aerosol setup is applied here for temperatures higher than 10 °C and RH lower than 60 % or higher than 83 %. On the other hand, the stable aerosol setup is applied mostly at lower temperatures (<5 °C). We note, however, that in our case, there were only minimal differences (less than 1 % in the simulated SIA concentrations during the cold period) between simulations only with assumptions of a metastable (liquid) and variable aerosol state in thermodynamic calculations.
Even though this work is focused on the SIA fine aerosol fraction, nitrate aerosols are explicitly calculated for both the fine and coarse modes (i.e., unlike and ). The rationale behind this is that nitrate is found to significantly contribute to the coarse aerosol sizes (Koulouri et al., 2008), indicating that a bulk approach may lead to an overestimation of the partitioning in the model, especially in areas with intense an NVC contribution, such as from sea salt and dust aerosols, and from anthropogenic combustion. For this practical application, kinetic limitations (Pringle et al., 2010) by mass transfer and transport between the nitric acid in the gas phase and the particulate nitrate in PM2.5 and PM10 are considered here, with ISORROPIA II then re-distributing the respective masses between the gas and the aerosol phases. We note, however, that the coarse nitrate is not further taken into account in the thermodynamic calculations, assuming for simplicity that the gaseous HNO3, able to condense in the coarse aerosol mode, partitions directly into the particulate bulk phase.
2.1.3 Emissions
Anthropogenic emissions were originally provided by the Copernicus Atmosphere Monitoring Service (CAMS). CAMS provides the annual emission rates of CO, NH3, NMVOCs (non-methane volatile organic compounds), NOX, PM10, PM2.5, and SO2 from the sectors of road, air, rail transport, maritime (local and international shipping), mobile machinery, fuel production, industry (paper, cement, minerals, metals, etc.), stationary combustion, agriculture, waste, solvent use, and public power. As is evident, forest fires or biogenic sources are not included in this dataset. CAMS adopts the GNFR (gridded nomenclature for reporting) sector classification and provides gridded data at 0.1°×0.05°. This dataset is then spatially refined over the domain of interest with the spatial-disaggregation approach and tool documented in Ramacher et al. (2021). In particular, the regional anthropogenic dataset is combined with GNFR-dependent high-resolution spatial proxies (based on data from the European Pollutant Release and Transfer Register, E-PRTR; Global Human Settlement population grid, GHS-POP; Coordination of Information on the Environment (CORINE) Land Cover, CLC; and OpenStreetMap, OSM) towards the spatial disaggregation at 1 km×1 km: (i) area sources emitted included 80 % in model layer 1 and 20 % in layer 2 for domestic heating; combustion in manufacturing industry, agriculture, and farming; and other mobile sources and machinery, except for shipping, where emissions are equally distributed in the first four layers of the model; (ii) point emissions (industrial sources emitted at the height of each stack); and (iii) line emissions (road and railway emissions emitted at 0 m). We note that the top heights of layers 1, 2, 3, and 4 are 17.5, 37.5, 62.5, and 87.5 m above the ground, respectively. Annual emissions are then converted into hourly emission rates using monthly, weekly, and daily distributions based on average European factors, except for the yearly profiles for public power, refineries, and road emissions, where CAMS-TEMPO (TEMPOral profiles) factors are applied (Guevara et al., 2021). The respective maps of the total annual emission fields are presented in Fig. S1 in the Supplement.
Sea salt emissions are calculated for this work following the parameterizations from Gong (2003) and Vignati et al. (2010). The flux of the number of sea spray particles is expressed as a function of the particle radius at 80 % humidity and the 10 m horizontal wind speed, assuming number median dry radius values of 0.09 and 0.794 µm for fine and coarse sea spray particles, along with geometric standard deviations of 1.5 and 2.0, respectively (Vignati et al., 2010). To further address the temperature effects on the sea spray source fluxes, polynomial expressions derived based on laboratory (chamber) experiments (Salter et al., 2015) have been implemented. The total annual sea salt emissions, as calculated online by the model, are presented in the Supplement (Fig. S1e).
Potassium (K+) emissions from anthropogenic wood-burning processes are further considered in our calculations, owing to their significance for the thermodynamic equilibrium distribution of nitrogen-containing semi-volatile compounds. For this, we apply monthly factors to the PM2.5 emissions from the domestic heating sector to derive the respective particle K+ fluxes. The non-sea-salt K+PM2.5 concentration ratios are derived from filter-based PM2.5 measurements in the center of Athens (Paraskevopoulou et al., 2014), specifically at the Thissio supersite (see Sect. 2.3). Assuming that in domestic wood combustion episodes K+ is directly emitted as part of the PM2.5, an average fraction of 3.52 % (weight concentration) contribution is here applied to the respective PM2.5 emissions for the winter months, as obtained by a positive matrix factorization (PMF) in central Athens (Thissio) from December 2013 to March 2016 (Theodosi et al., 2018). primary sources from residential combustion are also accounted for in the model, owing to the significance of their ambient concentrations, accounting here for a fraction of 3.7 % (Zhang et al., 2023) of the PM2.5 emitted during domestic wood combustion episodes. We note, however, that although Zhang et al. (2023) indicate that and can also be emitted from domestic combustion processes, the respective emission factors are found to be 3–4 times lower compared to , i.e., accounting for only up to 1 % of the PM2.5 emitted mass. For this reason, no primary emissions for , and are accounted for in this study. The total annual K+ emission field from anthropogenic wood-burning processes derived for this study is presented in Fig. S1f.
2.1.4 Boundary conditions
The CAMS European regional ensemble reanalysis data are used to create boundary conditions for O3, NO, NO2, CO, NH3, PM2.5, PM10, peroxyacetyl nitrate (PAN), SO2, and NMVOC concentrations (chemically split to the species treated by the local model). Due to the chemical advancements of the current work (SIA treatment), the CAMS global atmospheric composition forecast supports the boundary conditions for H2O2, N2O5, HNO3, methacrolein/methyl vinyl ketone, N2O5, HNO3, and HNO4, along with sea salt aerosols in the fine and coarse sizes (not available on the regional scale). Furthermore, since the CAMS European regional ensemble reanalysis data only provide SIA concentrations as lumped species, we also use the respective global atmospheric composition forecast to derive the individual species (i.e., , , and ) normalized to the regional ensemble models' SIA concentration data. The same assumption is applied for dust aerosols since dust concentrations are only provided as a part of the PM10 fraction in the regional reanalysis data. in the coarse mode is directly derived from the global forecast data (where available). The boundary conditions interface (BCONCAMS) developed at Helmholtz-Zentrum Hereon was utilized for the interpolation of the CAMS regional ensemble and global forecast product and the mapping of the chemical species to the urban air quality model. The interface performs bilinear interpolation in the horizontal and linear interpolation in the vertical and projects the pollutant concentrations to the city domain. We note that several utilities allow for the preparation of input files or the conversion of these to the necessary CityChem input formats. More details on the utilities used to produce the EPISODE–CityChem input files can be found in Karl et al. (2019).
2.1.5 Meteorology
The CTM EPISODE–CityChem is coupled offline with the mesoscale numerical weather prediction system Weather Research and Forecasting (WRF) (Skamarock et al., 2021). WRF is set up with the Lambert conformal conic projection, two-way nesting, the Thompson graupel scheme for microphysics (Thompson et al., 2008), the Monin–Obukhov (Janjić, 2002) scheme for surface-layer parameterization, the Noah land surface model (Tewari et al., 2004), and the Mellor–Yamada–Janjić (Janjić, 1994) turbulent kinetic energy (TKE) scheme for boundary layer. The meteorological simulations are initialized by the ensemble means of the synoptic-scale meteorological reanalysis ECMWF ERA5 (Hersbach et al., 2020). For this work, WRF is applied with telescoping nesting (three domains), with the innermost domain covering the CTM area with a 1 km × 1 km spatial resolution. Hourly varying meteorological variables used as inputs in the EPISODE–CityChem include pressure, air temperature, temperature gradient, relative humidity, sensible and latent heat fluxes, total solar radiation, cloud fraction, and the cloud LWC.
2.2 In situ measurements
In situ aerosol chemical composition measurements conducted at (1) the Thissio air monitoring supersite and (2) the Piraeus monitoring station, both operated by the National Observatory of Athens, are here used to evaluate the model's ability to simulate the main SIAs (, , and ) in the GAA, Greece. The Thissio air monitoring supersite (37.9732° N, 23.7180° E; 105 m a.s.l.) is located on the premises of the National Observatory of Athens, in central Athens. The site is far from major traffic roads, in a moderately populated residential area, and thus is considered representative of urban background conditions in the center of the Athens basin (Grivas et al., 2019; Panopoulou et al., 2020), as it serves as a receptor of pollutants from different sources and with various degrees of processing. Online measurements took place throughout 2019 at Thissio, apart from the period when the instrumentation for online aerosol SIA measurements was moved to the Piraeus site. Measurements were conducted at a central location during two periods, in winter (10 December 2018–16 January 2019) and summer (11 June 2019–9 July 2019). The instruments in Piraeus were located on the first floor (9 m above ground) of the Athens Metro terminal station building (Urban Rail Transport S.A., Athens, Greece; 37.9479° N, 23.6429° E; 10 m a.s.l.). The site is influenced to an extent by traffic emissions, being at a distance of 70 m from the coastal avenue in the Piraeus port area (Stavroulas et al., 2021).
High-temporal-resolution measurements of non-refractory submicron aerosol chemical composition at both sites were performed with a quadrupole Aerosol Chemical Speciation Monitor (ACSM, Aerodyne Research Inc.; Ng et al., 2011), operated at 30 min temporal resolution. The instrument provides concentrations of ammonium, sulfate, nitrate, chloride, and organic matter. Details on the limits of detection, sampling, and calibration of the instrument can be found in Stavroulas et al. (2019, 2021). QA/QC (quality assurance/quality control) of ACSM measurements included the comparison of the daily average online and offline measurements. The estimation of sea salt, non-sea-salt potassium, and dust was performed using the methodology of Sciare et al. (2005). Although the evaluation of dust concentrations is out of the scope of this study, we note that the estimation of atmospheric dust levels using the calcium ion as a proxy (Sciare et al., 2005) may be largely uncertain due to major contributions from other sources. It should also be noted that since ion chromatography mostly quantifies the inorganic components, it is expected that interferences may occur for sulfate and nitrate, especially during winter, when nitrites, organic nitrates, and sulfates are variably present (Guo et al., 2016).
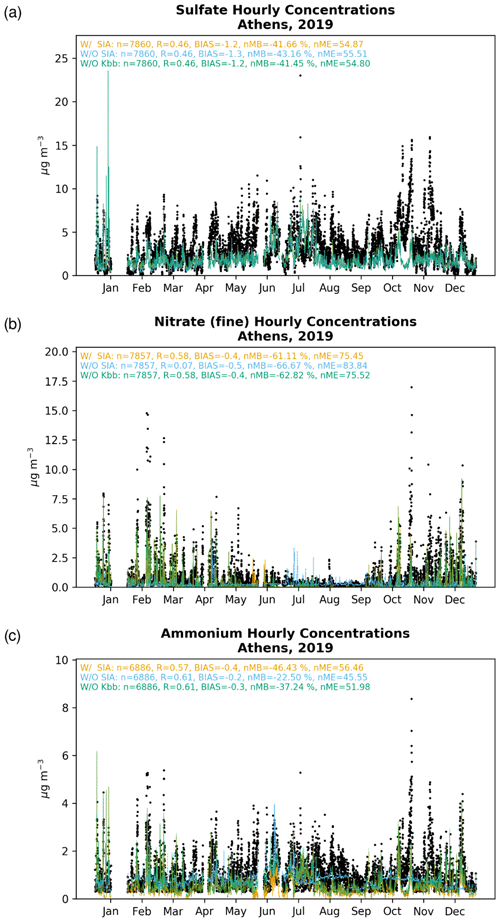
Figure 2Comparison (µg m−3) of hourly mean observations (black dots) in Piraeus (1–16 January 2019 and 11 June–9 July 2019) and in Thissio (17 January–10 June 2019 and 10 July–31 December 2019), for (a) sulfate, (b) fine nitrate, and (c) ammonium, to the EPISODE–CityChem simulations for base case simulation (W/ SIA; orange line), without considering SIA formation (W/O SIA; blue line) and without considering the contribution of K+ from domestic heating processes in thermodynamic calculations (W/O Kbb; green line). The respective correlation statistics for the Pearson correlation coefficient (r), the calculated bias (BIAS), the normalized mean bias (nMB), and the normalized mean error (nME) are also provided.
3.1 Model evaluation
3.1.1 Sulfate
Observations indicate that the hourly concentrations present only a slightly increasing trend from winter to summer 2019 over Athens (Fig. 2a). During the warm season, however, the scarcity or absence of precipitation in the region, along with increased transport through the boundaries and mainly the higher oxidation potential, resulted in an overall increase in concentrations in the model (W/ SIA), although still lower compared to observations. The enhanced summer concentrations in the model are nonetheless in accordance with previous observational and modeling studies in the area (Athanasopoulou et al., 2015; Grivas et al., 2018; Paraskevopoulou et al., 2015; Theodosi et al., 2018), suggesting that the majority of mainly originates from long-range transport (e.g., Balkan countries and the wider Black Sea region) due to fossil fuel combustion in power production and other industrial plants (Aksoyoglu et al., 2017). This is also clearly shown by the present sensitivity model calculations, indicating that when the local production via aqueous-phase SO2 oxidation is neglected (W/O SIA), the simulated concentrations remain practically unchanged in most of the cases, especially during the warm periods.
EPISODE–CityChem captures the observed hourly surface levels in Piraeus and Thissio, with a correlation coefficient (r) of 0.46 and an annual mean bias of −1.2 µg m−3 (Fig. 2a), partly attributed to the temporal variation in emissions, which is largely based on Europe-wide factors provided along with the CAMS-REG (regional) yearly data. The model tends to overall underestimate the observations, presenting for 2019 a negative normalized mean bias (nMB) of −42 %. Our simulations nevertheless indicate that the underestimation of the modeled concentrations should be mainly due to too low a long-range transport in the area through the boundaries, since only a weak local formation is supported by the model. Indeed, when comparing the base simulation (W/ SIA) with the W/O SIA sensitivity simulation, where the local production in cloud droplets is neglected, only a slight increase in the surface concentrations is illustrated, without any noteworthy change (<1 %) in the respective correlation statistics. On the other hand, the underrepresentation of SOx () local emissions, or even too slow a SO2 oxidation in the gas phase, could further contribute to the model's underestimation of the observed values. Last, although WRF accurately predicts temperature and humidity fields, it tends to overestimate wind speed (see Table S1 in the Supplement). This has the effect of enhanced ventilation and dispersion of (and the rest of SIAs), which may partially explain the observed negative biases in the simulated pollutants under study.
3.1.2 Nitrate
The observed and modeled concentrations increase during the cold period (Fig. 2b), presenting a pronounced seasonality. The increase mentioned above is caused by both the formation of NH4NO3 under low ambient temperatures (Theodosi et al., 2018) and the presence of non-volatile potassium cations from domestic heating processes, which can significantly promote the formation of KNO3. On the contrary, the observed concentrations are considerably reduced in summer (Liakakou et al., 2022; Paraskevopoulou et al., 2015), owing to the thermal instability and volatilization of the NH4NO3.
Sensitivity model simulations clearly indicate that is a local pollutant rather than a transported one in the GAA. This agrees with previous modeling studies where road traffic is found to significantly contribute to in eastern Europe (Aksoyoglu et al., 2017). Indeed, when the thermodynamics are neglected in model calculations (W/O SIA), concentrations are severely underestimated over the GAA in almost all seasons of the year, except for some cases during the summer months (July and, to a lesser extent, August). Although EPISODE–CityChem tends to calculate very low levels during the warm period for the base simulation (W/ SIA), the maxima during July and August due to the contribution of long-range transport from biomass-burning processes (such as wildfires in Attica and on the island of Evia in July and August 2019) seem not to be well captured by the model. On the other hand, the W/O Kbb sensitivity model simulation clearly indicates that the presence of K+ associated with domestic heating processes plays a crucial role in formation during the winter period, leading overall to a lower nMB compared to the base simulation (i.e., −63 % vs. −61 %). This, yet, is in accordance with previous findings in the region, where a good correlation between K+ with organic carbon (OC), elemental carbon (EC), and has been observed during winter (Fourtziou et al., 2017; Theodosi et al., 2018), thus clearly supporting their common origin during the cold period. In more detail, residential biomass-burning processes are found to be directly responsible for the winter maxima measured over the GAA, while intense Saharan dust episodes under the local southerly winds may also have an impact on the spring peak.
A better correlation compared to those of is found when comparing the model and the measurements for the hourly surface levels (r=0.58). The model, however, tends to overall underestimate the observations, with a slightly negative bias of around −0.4 µg m−3 and an nMB of roughly −61 % for the year 2019, mainly due to too low a summertime simulated concentration (Fig. 2b). We note, nevertheless, that an underestimation of the observed concentrations is here expected since, on the one hand, some ammonia sources, such as from agricultural soils, are currently omitted in the model and on the other hand, species that may be accounted for in the observational data, such as nitrites and organic nitrates, which may also be present during winter and partition in the particulate phase (Guo et al., 2016), are not yet included in the presented chemical mechanism. Still, correlation statistics clearly denote that the local HNO3 partitioning in the particulate phase is the main pathway of fine particulate formation in the study area and, more specifically, that the presence of K+, either from domestic burning processes or dust events, plays a crucial role in the concentration distributions.
3.1.3 Ammonium
The observed concentrations (Fig. 2c) generally present a similar seasonal pattern to that of , although somehow with a more intense trend during the warm season. As already found in previous works (Mantas et al., 2014; Paraskevopoulou et al., 2015; Theodosi et al., 2018), observations of and are highly correlated (r=0.79), indicating that non-sea-salt can be considered at least partially neutralized by . The simulated sulfate/ammonium ratio for the base simulation (r=0.73) shows that sulfate exists mainly in the model in the form of NH4HSO4 and (NH4)2SO4 at the two sites (i.e., Thissio and Piraeus), in agreement with previous studies in the wider eastern Mediterranean area (Athanasopoulou et al., 2008; Bardouki et al., 2003; Fourtziou et al., 2017; Koulouri et al., 2008). Nevertheless, during the cold season, is also found to be significantly correlated with (r=0.62), indicative of NH4NO3 formation. Note, however, that for this work, the non-sea-salt chloride is not considered in model calculations, so the non-sea-salt NH4Cl does not contribute to the calculated NH4 concentrations.
The base case simulation of EPISODE–CityChem captures the observed hourly surface observations in Piraeus and Thissio with a correlation coefficient (r) of 0.57 and a negative mean bias of −0.4 µg m−3 (Fig. 2f), with a calculated negative nMB of −46 % for 2019. However, when the thermodynamic calculations are neglected in the model in the W/O SIA simulation (i.e., accounting only for the transport from the model's boundaries contributing to the levels), the agreement tends to improve, leading to a correlation coefficient (r) of 0.61, a negative mean bias of −0.2 µg m−3, and a negative nMB of −22 % (Fig. 2f). This can be explained due to the volatilization of for the base simulation when thermodynamics are taken into account in the model, which overall shifts to gas-phase NH3 (Guo et al., 2018).
The significance of aerosol pH levels on volatilization is further supported by the model sensitivity simulation when the contribution of K+ from domestic heating processes is neglected in the thermodynamic calculations (W/O Kbb). Indeed, the absence of K+ increases the acidity in the aerosol phase and thus enhances the gas/particle partitioning of . Overall, the improved correlation of model results with observations in the absence of K+ from domestic heating compared to the base case simulation (i.e., r=0.61, , and ) indicates that the model misses primary NH3 emissions from sources like road transport that could contribute up to 10 % to ambient levels (Aksoyoglu et al., 2017), as well as from non-urban sources, such as the volatilization of animal waste and synthetic fertilizers, biomass combustion, and losses from natural soils (e.g., Fameli and Assimakopoulos, 2016)
3.2 Spatial model concentration fields
The mapping of the main SIAs at ground level, as simulated by EPISODE–CityChem averaged for the winter (January, February, and December 2019) and summer (June, July, and August 2019) periods, is presented in Fig. 3. Additionally, the spatiotemporal totals of the SIA-related tracer emissions are presented in Fig. S2. During both seasons, high levels are simulated near the major emission hotspots, such as shipping routes around the ports of Piraeus and Eleusis and large combustion plants in the Thriassion Plain (Fig. 1), where the vast majority of the surface SO2 emissions in the GAA occur. During summer (Fig. 3b), the predicted surface concentrations are close to 2.86 ± 0.92 µg m−3 in the center of Athens and the suburbs, while during winter (Fig. 3a) the season mean simulated concentrations vary between 1.31 and 4.45 µg m−3. The results indicate that long-range transport is an important contributor to surface concentrations in the area, which are maximized during the summer due to enhanced photochemical activity. On average, is calculated to have an annual mean surface concentration of 2.15 ± 0.88 µg m−3 for the year 2019 in the model domain. Sensitivity model calculations confirmed this, showing that chemical production in the model domain can support up to 10 % of the wintertime surface concentrations (Fig. 4a), mainly in higher-altitude areas, such as Mt. Parnitha (Fig. 1), where cloudiness is enhanced and the increased cloud LWC promotes the aqueous-phase oxidation of ambient SO2. Around the city center, however, the chemical formation contribution to the surface levels is calculated to be rather low (roughly 4 %).
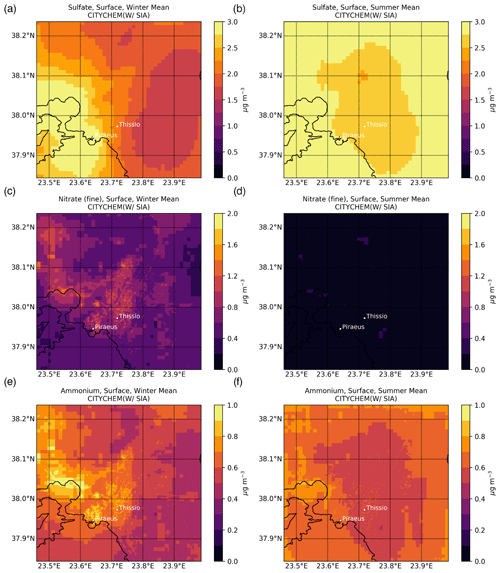
Figure 3Surface concentrations (µg m−3) over the greater Athens area of (a, b) sulfate, (c, d) nitrate, and (e, f) ammonium, as simulated by EPISODE–CityChem at a horizontal resolution of 100 m×100 m for (a, c, e) winter (December, January, and February) and (b, d, f) summer (June, July, and August) 2019.
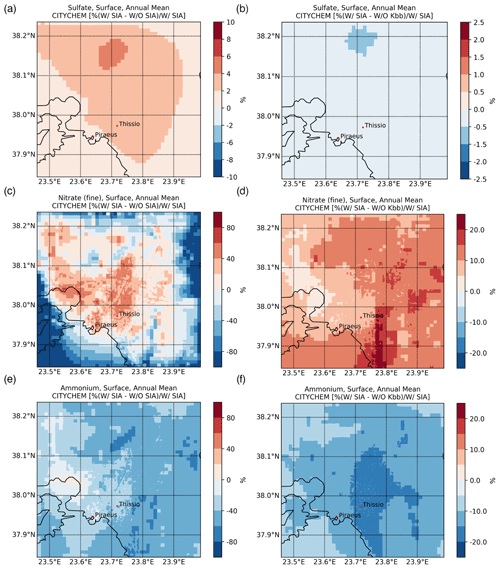
Figure 4Annual mean percentage (%) contribution of secondary formation processes to the surface concentrations of (a) sulfate, (c) nitrate, and (e) ammonium and (b, d, f) the respective impact of including the K+ from domestic burning in thermodynamic calculations, as simulated by EPISODE–CityChem at a horizontal resolution of 100 m×100 m over the greater Athens area for the year 2019.
The average fine concentrations in the model are calculated to vary between 0.05 and 1.50 µg m−3 during the cold period (Fig. 3c). A mean concentration of 0.51 ± 0.20 µg m−3 is simulated during the winter months, while only 0.05 ± 0.05 µg m−3 is calculated during the summer (Fig. 3d). In the model domain, registered an annual mean surface concentration of 0.24 ± 0.23 µg m−3 for 2019. In contrast, however, to the surface concentrations, levels present notable spatial variability, with much higher concentrations calculated near rather than away from the city center during the winter, compared to the negligible concentrations in the warm season. This indicates that formation significantly depends on the local meteorological conditions (i.e., temperature and relative humidity) along with the anthropogenic emissions of precursor species that are widespread in the GAA (Fig. 1), mainly from traffic, and especially the presence of NVCs such as K+ from domestic heating in winter that can support an increase in surface concentrations of about 10 % ± 4 % in the model domain (Fig. 4d). It is noted that, in this study, is only either formed in the model domain as a secondary product or transported from the boundaries, without any primary contribution. However, the enhanced concentrations around the city center in the model clearly indicate that is mainly a local pollutant rather than one transported over the GAA. The latter is further supported through sensitivity model calculations performed for this work, demonstrating that when thermodynamics are accounted for in the model (Fig. 4c), more than 80 % of the surface concentrations on an annual basis in downtown Athens (Fig. 1) are directly attributed to the HNO3 gas/particle partitioning.
The average surface concentrations in the model are calculated to be without strong annual variability in the GAA for 2019. For the cold period (Fig. 3e), the model simulates that concentration varies between 0.30 in the suburbs and 1.40 µg m−3 around the city center, while for the summertime it is between 0.33 and 1.18 µg m−3, respectively (Fig. 3f). For the year 2019, is calculated to have an annual mean surface concentration of 0.58 ± 0.14 µg m−3 in the model domain. Model simulations show that the available anthropogenic NH3 emissions in the model domain tend to quickly neutralize the primary strong acids present in the urban atmosphere of Athens, like H2SO4 and HNO3, which subsequently partition into the aerosol aqueous phase in the form of the ammonium ion. As already discussed in Sect. 3.1.3, when thermodynamics are included in model simulation, an increase in volatilization of is calculated that, on an annual basis, can support a decrease in its concentrations up to 70 % in the suburbs (Fig. 4e). On the other hand, much lower volatilization is calculated around the Thriassion Plain, where combustion plants exist, as well as in the region's major port zones (Fig. 1), due to primary NH3 sources. The latter indicates that although traffic, solid- and water-waste management facilities, and certain industrial processes (e.g., refineries, cement manufacturing, livestock production) seem to be the main NH3 sources in urban areas (Liakakou et al., 2023), ammonia may also be strongly related to agricultural activities and soil sources in rural areas, which in turn significantly impacts concentrations; however, NH3 emissions from such sectors are not currently included in our model. Additionally, emissions from domestic heating may also have some impact on NH3 surface levels (Fig. 4f), since in the presence of non-volatile potassium cation such as from biomass-burning processes, the formation of KNO3 is promoted and thus the gas/particle partitioning of NH3 is reduced (12 % ± 2 %).
3.3 Atmospheric water and acidity
Particle LWC and aerosol pH are important parameters of the aerosol phase; however, limited in situ observations of aerosol pH exist due to the non-conserved nature of H+ and the dissociation of inorganic and organic electrolytes in the particulate phase. Since ion balance proxy methods generally lead to miscalculations of aerosol acidity levels, thermodynamic model calculations can be considered a reliable tool for such calculations (Hennigan et al., 2015). The acidity levels in an urban environment are primarily governed by the atmospheric LWC and the aqueous-phase proton concentration ([H+]). The impacts of cloud LWC and aerosol water on atmospheric acidity significantly differ; cloud liquid water is primarily determined by meteorological conditions, while aerosol water is subject to chemical equilibrium with atmospheric water vapors. Thus, the hygroscopicity of the dissolved aerosol species plays a significant role in governing the aerosol water content under specific relative humidity levels (Pye et al., 2020). Moreover, the pH levels in clouds are found to be notably higher (up to four units; Seinfeld and Pandis, 2006) in comparison to aerosols, owing to the dilute conditions prevailing in them. On the contrary, aerosol acidity is determined not only by the equilibrium between acidic and alkaline species but also by the relative concentrations of such species that partition in the particulate phase under favorable temperature and relative humidity conditions (Pye et al., 2020).
Figure 5 illustrates the average pH levels of fine aerosols and the respective particulate water content on the surface for winter and summer, as calculated by EPISODE–CityChem. The calculated pH for fine aerosols over the GAA can be rather acidic, with an average value of 4.81 ± 0.93 during winter (Fig. 5a) and 2.20 ± 1.00 during summer (Fig. 5b), varying spatially between 2.38–7.58 and 0.79–5.82, respectively. Minimum pH values are calculated to differ by roughly one unit, indicating an overall 10-fold increase in the aerosol H+ LWC ratio from the summer to winter periods in the model. Such a variation in the aerosol acidity can be directly attributed to the significantly different meteorological parameters between the two seasons, illustrating the importance of the increased relative humidity levels on aerosol pH values during the cold period. Nonetheless, the persistence of strong acidity in fine aerosols can also be attributed to the thermodynamic equilibrium between and NH3 that overall promotes the NHx () volatilization in the model. This is in accordance with other studies where, although gaseous NH3 may be abundant, the pH values of PM2.5 remain acidic (Ding et al., 2019). Note, however, that for this study we only account for the water associated with the inorganic aerosol component; thus, the model may slightly underestimate the particulate water by 0.15–0.23 units compared to the pH predicted with total water (Guo et al., 2015). This value is likely an upper bound of the error since organic aerosol mass fractions in the specific location were high (∼60 %; Guo et al., 2015; Stavroulas et al., 2019). On the other hand, the significantly higher maximum pH values during wintertime cannot be only attributed to meteorology. In accordance with previous studies, higher aerosol pH levels can be directly associated with the presence of NVCs emanating from wood-burning processes (Bougiatioti et al., 2016; Zhang et al., 2015). For completeness, the calculated surface aerosol water concentrations for winter (Fig. 5c) and summer (Fig. 5d) are also provided.
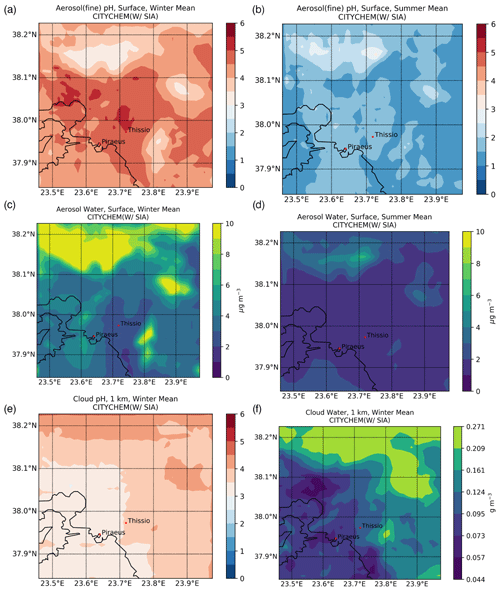
Figure 5(a, b) Surface fine aerosol pH and (c, d) aerosol water content (µg m−3) for (a, c) winter (December, January, February) and (b, d) summer (June, July, August) 2019, along with the cloud pH at 1 km altitude for (e) winter as simulated by EPISODE–CityChem at a horizontal resolution of 1 km×1 km over the greater Athens area. (f) The respective cloud water content (g m−3) is also presented.
The long-range transport of SIAs along with the emissions of its precursors, such as SO2 and NOX, tend to also decrease local cloud pH levels in the model. It should be mentioned that EPISODE–CityChem does not calculate but instead only reads the cloud LWC and the cloud cover from meteorological files in the region, meaning that no transport of clouds exists in the model domain. During the winter season (Fig. 5e), higher cloud pH values have been generally calculated as compared to the summer season (not shown). The cloud pH is calculated to vary between 3.50 and 6.70 during the wintertime over Athens, mostly in the suburbs where higher levels of cloudiness exist, owing to the orography of the regions. On the contrary, during the summer, the model calculates clouds of higher acidity (i.e., up to ∼0.28 pH units). This, however, can be attributed mainly to the relative absence of cloud water during the warm period in the model, along with the increased transport of acidic compounds like sulfates (i.e., very concentrated solutions lead to strongly acidic pH values). Note, however, that the potential impact of mineral dust, particularly calcium, on cloud H+ concentrations has been neglected in the above calculations. Such an assumption could potentially lead to an overestimation of cloud acidity, especially during the dust event periods (mainly late winter and spring). A further constraint in the assessment of cloud acidity pertains to the exclusion of low-molecular-weight organic acids (e.g., formic and acetic acids), which may also result in some degree of miscalculation of the cloud acidity levels.
For this work, the ISORROPIA II thermodynamic model has been coupled to the urban-scale EPISODE–CityChem CTM to calculate the gas/particle partitioning of and , providing insights into complex interactions in a major Eastern Mediterranean urban agglomeration. While the model tends to generally underestimate the SIA measurements over the greater Athens area, it tracks their observed daily variation at the Piraeus and Thissio monitoring stations. SIA formation appears to significantly depend on the ambient precursor gases (SO2, NOX, and NH3), which represent an important fraction (i.e., up to 20 % during both cold and warm periods) of PM2.5 in the model domain, especially in suburban areas. Nevertheless, long-range transport is found to be a critical factor in determining the SIA ambient concentrations, especially for . Indeed, the model's underestimation of observed concentrations indicates transport that is too weak from the boundaries rather than too low a secondary formation from primary SOx sources. Respectively, the lower concentrations compared to the observed values generally follow the variations in the simulated , as also denoted by the derived sulfate ammonium ratio, where sulfate is mainly in the form of NH4HSO4 and (NH4)2SO4. Specifically, during the cold period, the relatively basic aerosol pH values enhance the volatilization of in the model, and, along with possibly missing NH3 sources, the model tends to underestimate the measurements.
The inclusion of non-volatile cations originating from biomass-burning sources, along with sea salt and dust aerosols, has nevertheless had a critical impact on the ion balance and thus the partitioning of semi-volatile compounds (i.e., nitrate and ammonium) in the model. Although this study is focused on the fine PM fraction, we note that high nitrate concentrations have been found to be well correlated with increased concentrations of supermicron dust and sea spray particles (Allen et al., 2015), indicating the importance of crustal species in the partitioning. Still, besides the presence of sea salt and dust aerosols, the inclusion of K+ from domestic wood-burning combustion sources in the aerosol thermodynamic calculations is found to affect the ion balance and thus the formation of , due to a more effective partitioning in the aerosol phase during the cold and humid conditions. concentrations for 2019 exhibit a relatively lower value in comparison to the observations, with a correlation coefficient of 0.58 and a normalized mean bias of −61 %. However, the model performs better in simulating concentrations as compared to (i.e., r=0.46) and (i.e., r=0.57), suggesting a satisfactory representation of the partitioning. On the other hand, the volatilization of due to a decrease in aerosol pH (especially during the warm period) tends to shift to the gas phase, further indicating missing NH3 sources in the model.
It is well established that SIAs play an important role in urban air quality and can have detrimental effects on the environment and human health. Thus, reducing emissions of their precursors via measures including, inter alia, the extensive use of sustainable energy, mobility, and agriculture is crucial for mitigating such effects in the long term. The continuation of such policies on the European level appears critical, especially in view of new air quality management legislation in the EU that will set very ambitious PM standards for the protection of public health, which will be difficult to attain unless sweeping emission cutbacks are implemented. Moreover, despite the fact that the reduction in primary pollutants like SO2 and NOX can also reduce the acidity of cloud water and precipitation (Watmough et al., 2016), fine aerosols may still remain highly acidic in a future atmosphere due to the respective NH3 volatilization in the particulate phase (Baker et al., 2021; Lawal et al., 2018). On the other hand, it is shown here that, accounting for the K+ sources from domestic wood burning in the model, significantly higher aerosol pH values can be locally supported during the winter compared to the warm period, where K+ is mostly associated with Saharan dust outbreaks in the GAA (Theodosi et al., 2018). Nevertheless, such a discrepancy in the response of the fine aerosol pH to emission controls also has noteworthy implications for the toxicity of atmospheric particles, especially during the cold period, where domestic wood burning may consist of an additional source of harmful air pollution. Such complex chemical interactions have not yet, however, been comprehensively acknowledged in urban-scale studies (Zhang et al., 2022).
In this study, the high spatiotemporal resolution of EPISODE–CityChem (up to 100 m×100 m, 1 h) with the improved representation of the inorganic aerosols delivers a beyond-the-state-of-the-art characterization of the fine particulate load over the GAA. Focusing further on air quality studies in polluted urban domains, several initiatives underway are aimed at improving EPISODE–CityChem's modeling of aerosols and the respective chemical processes. These include coupling with aerosol microphysical schemes that will account for new particle formation and aerosol aging through coalescence and condensation (e.g., Karl et al., 2023). Such developments aim to improve the model's ability to represent the composition and size distribution of fine PM. A better characterization of aerosol chemical speciation, like the consideration of organic aerosols, is expected to also improve the aerosol hygroscopicity calculations in the model, especially within the boundary layer where the contribution of water-soluble organics to total aerosol mass is important (i.e., Bougiatioti et al., 2016). The KPP software for solving stiff numerical systems has already been coupled to the model to integrate the gas-phase photochemistry schemes, and, based on this development, more explicit chemical schemes are planned to be available in the model. Furthermore, gas-phase organic species can also be oxidized in the interstitial cloud space and form water-soluble compounds like aldehydes, which rapidly partition into droplets. In the presence of oxidants (e.g., OH and NO3 radicals), the dissolved organics can also undergo chemical conversions and form low-volatility organics that remain partially in the aerosol phase. A state-of-the-art multiphase chemical scheme (e.g., Myriokefalitakis et al., 2022) is therefore planned to be coupled to the model to account for such an aerosol formation process in an urban area. Additionally, parameterizations of marine dimethylsulfide (DMS) and organic aerosol primary sources in the model are currently under consideration.
The proposed model framework, coupled with emission reduction scenarios, may enable a thorough investigation of the regulations and interventions that can efficiently curtail air pollution in the GAA and, consequently, its health impacts, providing a valuable decision support tool to the air quality management authorities in view of the realignment with the new air quality standards that will be inevitable in the near future, given the present state of air quality in Greece. Anticipated advancements in model parallelization and code architecture are expected to facilitate computationally efficient urban simulations and the creation of state-of-the-art forecasting systems capable of generating high-resolution air quality predictions for multiple urban regions in Europe up to the street level. Overall, providing such an urban-scale modeling tool allows for a more accurate articulation of strategies for air quality management, including the optimization of monitoring protocols as well as the mitigation of emissions from various activity sectors and adverse effects.
The source codes of the EPISODE–CityChem model (version 1.8) and the preprocessing utilities are accessible in the release under the Reciprocal Public License (RPL) at https://doi.org/10.5281/zenodo.8063985 (Karl, 2023). The thermodynamic equilibrium model ISORROPIA II is available at https://www.epfl.ch/labs/lapi/software/isorropia/ (last access: 5 July 2024).
The supplement related to this article is available online at: https://doi.org/10.5194/acp-24-7815-2024-supplement.
SM made the couplings for including thermodynamic calculations in the model, developed the chemistry code, performed the simulations, and evaluated the model. MK was responsible for all developments, updates, and the maintenance of the CityChem extension of the model. EA and AK developed the emission fields. KAW contributed to the creation of the emission and boundary fields. DK and GP performed the meteorological simulations, developed and provided model evaluation tools, and collected observational meteorological data. GG prepared and provided data for the preparation of potassium emission fields from anthropogenic wood-burning processes. AB, EL, IS, GG, DP, and NM collected, prepared, and provided atmospheric data used for model evaluation. SM, MK, EA, OS, and EG developed the methodology for this work. SM conceptualized and wrote the paper, and all co-authors contributed to the preparation of the paper, the discussion of the results, and provided comments on the final version of the paper.
The contact author has declared that none of the authors has any competing interests.
Publisher's note: Copernicus Publications remains neutral with regard to jurisdictional claims made in the text, published maps, institutional affiliations, or any other geographical representation in this paper. While Copernicus Publications makes every effort to include appropriate place names, the final responsibility lies with the authors.
This work was supported by the EU H2020 project Research Infrastructures Services Reinforcing Air Quality Monitoring Capacities in European Urban & Industrial AreaS (RI-URBANS) (grant no. 101036245). This work was supported by computational time granted from the National Infrastructures for Research and Technology S.A. (GRNET S.A.) in the Advanced Research Information System (ARIS) national high-performance computing (HPC) facility (project ID 014008; ANIONIC). The authors would like to thank Athanasios Nenes. for the use of the ISORROPIA II code in this work. The authors thank Vassiliki Kotroni and Konstantinos Lagouvardos for the use of in situ measurements from the weather station network operated by the METEO unit of the National Observatory of Athens. The authors are also grateful to two anonymous reviewers for their comments, which contributed significantly to the improvement of the final version of this paper.
This research has been supported by the European Union H2020 research infrastructures (grant no. 101036245).
This paper was edited by Stefano Galmarini and reviewed by two anonymous referees.
Aksoyoglu, S., Ciarelli, G., El-Haddad, I., Baltensperger, U., and Prévôt, A. S. H.: Secondary inorganic aerosols in Europe: sources and the significant influence of biogenic VOC emissions, especially on ammonium nitrate, Atmos. Chem. Phys., 17, 7757–7773, https://doi.org/10.5194/acp-17-7757-2017, 2017.
Alexander, B., Park, R. J., Jacob, D. J., and Gong, S.: Transition metal-catalyzed oxidation of atmospheric sulfur: Global implications for the sulfur budget, J. Geophys. Res., 114, D02309, https://doi.org/10.1029/2008JD010486, 2009.
Allen, H. M., Draper, D. C., Ayres, B. R., Ault, A., Bondy, A., Takahama, S., Modini, R. L., Baumann, K., Edgerton, E., Knote, C., Laskin, A., Wang, B., and Fry, J. L.: Influence of crustal dust and sea spray supermicron particle concentrations and acidity on inorganic aerosol during the 2013 Southern Oxidant and Aerosol Study, Atmos. Chem. Phys., 15, 10669–10685, https://doi.org/10.5194/acp-15-10669-2015, 2015.
Athanasopoulou, E., Tombrou, M., Pandis, S. N., and Russell, A. G.: The role of sea-salt emissions and heterogeneous chemistry in the air quality of polluted coastal areas, Atmos. Chem. Phys., 8, 5755–5769, https://doi.org/10.5194/acp-8-5755-2008, 2008.
Athanasopoulou, E., Vogel, H., Vogel, B., Tsimpidi, A. P., Pandis, S. N., Knote, C., and Fountoukis, C.: Modeling the meteorological and chemical effects of secondary organic aerosols during an EUCAARI campaign, Atmos. Chem. Phys., 13, 625–645, https://doi.org/10.5194/acp-13-625-2013, 2013.
Athanasopoulou, E., Protonotariou, A. P., Bossioli, E., Dandou, A., Tombrou, M., Allan, J. D., Coe, H., Mihalopoulos, N., Kalogiros, J., Bacak, A., Sciare, J., and Biskos, G.: Aerosol chemistry above an extended archipelago of the eastern Mediterranean basin during strong northern winds, Atmos. Chem. Phys., 15, 8401–8421, https://doi.org/10.5194/acp-15-8401-2015, 2015.
Athanasopoulou, E., Protonotariou, A., Papangelis, G., Tombrou, M., Mihalopoulos, N., and Gerasopoulos, E.: Long-range transport of Saharan dust and chemical transformations over the Eastern Mediterranean, Atmos. Environ., 140, 592–604, https://doi.org/10.1016/j.atmosenv.2016.06.041, 2016.
Atkinson, R., Baulch, D. L., Cox, R. A., Crowley, J. N., Hampson, R. F., Hynes, R. G., Jenkin, M. E., Rossi, M. J., and Troe, J.: Evaluated kinetic and photochemical data for atmospheric chemistry: Volume I – gas phase reactions of Ox, HOx, NOx and SOx species, Atmos. Chem. Phys., 4, 1461–1738, https://doi.org/10.5194/acp-4-1461-2004, 2004.
Baker, A. R., Kanakidou, M., Nenes, A., Myriokefalitakis, S., Croot, P. L., Duce, R. A., Gao, Y., Guieu, C., Ito, A., Jickells, T. D., Mahowald, N. M., Middag, R., Perron, M. M. G., Sarin, M. M., Shelley, R., and Turner, D. R.: Changing atmospheric acidity as a modulator of nutrient deposition and ocean biogeochemistry, Science Advances, 7, eabd8800, https://doi.org/10.1126/sciadv.abd8800, 2021.
Bardouki, H., Liakakou, H., Economou, C., Sciare, J., Smolík, J., Ždímal, V., Eleftheriadis, K., Lazaridis, M., Dye, C., and Mihalopoulos, N.: Chemical composition of size-resolved atmospheric aerosols in the eastern Mediterranean during summer and winter, Atmos. Environ., 37, 195–208, https://doi.org/10.1016/S1352-2310(02)00859-2, 2003.
Basagaña, X., Jacquemin, B., Karanasiou, A., Ostro, B., Querol, X., Agis, D., Alessandrini, E., Alguacil, J., Artiñano, B., Catrambone, M., De La Rosa, J. D., Díaz, J., Faustini, A., Ferrari, S., Forastiere, F., Katsouyanni, K., Linares, C., Perrino, C., Ranzi, A., Ricciardelli, I., Samoli, E., Zauli-Sajani, S., Sunyer, J., and Stafoggia, M.: Short-term effects of particulate matter constituents on daily hospitalizations and mortality in five South-European cities: Results from the MED-PARTICLES project, Environ. Int., 75, 151–158, https://doi.org/10.1016/j.envint.2014.11.011, 2015.
Basla, B., Agresti, V., Balzarini, A., Giani, P., Pirovano, G., Gilardoni, S., Paglione, M., Colombi, C., Belis, C. A., Poluzzi, V., Scotto, F., and Lonati, G.: Simulations of Organic Aerosol with CAMx over the Po Valley during the Summer Season, Atmosphere, 13, 1996, https://doi.org/10.3390/atmos13121996, 2022.
Bougiatioti, A., Nikolaou, P., Stavroulas, I., Kouvarakis, G., Weber, R., Nenes, A., Kanakidou, M., and Mihalopoulos, N.: Particle water and pH in the eastern Mediterranean: source variability and implications for nutrient availability, Atmos. Chem. Phys., 16, 4579–4591, https://doi.org/10.5194/acp-16-4579-2016, 2016.
Brandt, F., Bosbach, D., Krawczyk-Barsch, E., Arnold, T., and Bernhard, G.: Chlorite dissolution in the acid ph-range: a combined microscopic and macroscopic approach, Geochim. Cosmochim. Ac., 67, 1451–1461, https://doi.org/10.1016/S0016-7037(02)01293-0, 2003.
Cheng, B., Wang-Li, L., Classen, J., and Bloomfield, P.: Performance of a Thermodynamic Model for Predicting Inorganic Aerosols in the Southeastern U. S., Atmosphere, 13, 1977, https://doi.org/10.3390/atmos13121977, 2022.
Clegg, S. L., Brimblecombe, P., and Wexler, A. S.: Thermodynamic Model of the System H+––––H2O at Tropospheric Temperatures, J. Phys. Chem. A, 102, 2137–2154, https://doi.org/10.1021/jp973042r, 1998.
Damian, V., Sandu, A., Damian, M., Potra, F., and Carmichael, G. R.: The kinetic preprocessor KPP-a software environment for solving chemical kinetics, Comput. Chem. Eng., 26, 1567–1579, https://doi.org/10.1016/S0098-1354(02)00128-X, 2002.
Denby, B. R., Gauss, M., Wind, P., Mu, Q., Grøtting Wærsted, E., Fagerli, H., Valdebenito, A., and Klein, H.: Description of the uEMEP_v5 downscaling approach for the EMEP MSC-W chemistry transport model, Geosci. Model Dev., 13, 6303–6323, https://doi.org/10.5194/gmd-13-6303-2020, 2020.
Dimitriou, K., Grivas, G., Liakakou, E., Gerasopoulos, E., and Mihalopoulos, N.: Assessing the contribution of regional sources to urban air pollution by applying 3D-PSCF modeling, Atmos. Res., 248, 105187, https://doi.org/10.1016/j.atmosres.2020.105187, 2021.
Ding, J., Zhao, P., Su, J., Dong, Q., Du, X., and Zhang, Y.: Aerosol pH and its driving factors in Beijing, Atmos. Chem. Phys., 19, 7939–7954, https://doi.org/10.5194/acp-19-7939-2019, 2019.
Driscoll, C. T., Driscoll, K. M., Mitchell, M. J., and Raynal, D. J.: Effects of acidic deposition on forest and aquatic ecosystems in New York State, Environ. Pollut., 123, 327–336, https://doi.org/10.1016/s0269-7491(03)00019-8, 2003.
Driscoll, C. T., Driscoll, K. M., Fakhraei, H., and Civerolo, K.: Long-term temporal trends and spatial patterns in the acid-base chemistry of lakes in the Adirondack region of New York in response to decreases in acidic deposition, Atmos. Environ., 146, 5–14, https://doi.org/10.1016/j.atmosenv.2016.08.034, 2016.
Fameli, K.-M. and Assimakopoulos, V. D.: The new open Flexible Emission Inventory for Greece and the Greater Athens Area (FEI-GREGAA): Account of pollutant sources and their importance from 2006 to 2012, Atmos. Environ., 137, 17–37, https://doi.org/10.1016/j.atmosenv.2016.04.004, 2016.
Fountoukis, C. and Nenes, A.: ISORROPIA II: a computationally efficient thermodynamic equilibrium model for K+–Ca2+–Mg2+––Na+–––Cl−–H2O aerosols, Atmos. Chem. Phys., 7, 4639–4659, https://doi.org/10.5194/acp-7-4639-2007, 2007.
Fountoukis, C., Racherla, P. N., Denier van der Gon, H. A. C., Polymeneas, P., Charalampidis, P. E., Pilinis, C., Wiedensohler, A., Dall'Osto, M., O'Dowd, C., and Pandis, S. N.: Evaluation of a three-dimensional chemical transport model (PMCAMx) in the European domain during the EUCAARI May 2008 campaign, Atmos. Chem. Phys., 11, 10331–10347, https://doi.org/10.5194/acp-11-10331-2011, 2011.
Fourtziou, L., Liakakou, E., Stavroulas, I., Theodosi, C., Zarmpas, P., Psiloglou, B., Sciare, J., Maggos, T., Bairachtari, K., Bougiatioti, A., Gerasopoulos, E., Sarda-Estève, R., Bonnaire, N., and Mihalopoulos, N.: Multi-tracer approach to characterize domestic wood burning in Athens (Greece) during wintertime, Atmos. Environ., 148, 89–101, https://doi.org/10.1016/j.atmosenv.2016.10.011, 2017.
Franklin, M., Koutrakis, P., and Schwartz, J.: The Role of Particle Composition on the Association Between PM2.5 and Mortality, Epidemiology, 19, 680–689, https://doi.org/10.1097/EDE.0b013e3181812bb7, 2008.
Gong, S. L.: A parameterization of sea-salt aerosol source function for sub- and super-micron particles, Global Biogeochem. Cy., 17, 1097, https://doi.org/10.1029/2003GB002079, 2003.
Gratsea, M., Athanasopoulou, E., Kakouri, A., Richter, A., Seyler, A., and Gerasopoulos, E.: Five Years of Spatially Resolved Ground-Based MAX-DOAS Measurements of Nitrogen Dioxide in the Urban Area of Athens: Synergies with In Situ Measurements and Model Simulations, Atmosphere, 12, 1634, https://doi.org/10.3390/atmos12121634, 2021.
Grivas, G., Cheristanidis, S., Chaloulakou, A., Koutrakis, P., and Mihalopoulos, N.: Elemental Composition and Source Apportionment of Fine and Coarse Particles at Traffic and Urban Background Locations in Athens, Greece, Aerosol Air Qual. Res., 18, 1642–1659, https://doi.org/10.4209/aaqr.2017.12.0567, 2018.
Grivas, G., Stavroulas, I., Liakakou, E., Kaskaoutis, D. G., Bougiatioti, A., Paraskevopoulou, D., Gerasopoulos, E., and Mihalopoulos, N.: Measuring the spatial variability of black carbon in Athens during wintertime, Air Qual. Atmos. Hlth., 12, 1405–1417, https://doi.org/10.1007/s11869-019-00756-y, 2019.
Grivas, G., Athanasopoulou, E., Kakouri, A., Bailey, J., Liakakou, E., Stavroulas, I., Kalkavouras, P., Bougiatioti, A., Kaskaoutis, D. G., Ramonet, M., Mihalopoulos, N., and Gerasopoulos, E.: Integrating in situ Measurements and City Scale Modelling to Assess the COVID–19 Lockdown Effects on Emissions and Air Quality in Athens, Greece, Atmosphere, 11, 1174, https://doi.org/10.3390/atmos11111174, 2020.
Guevara, M., Jorba, O., Tena, C., Denier van der Gon, H., Kuenen, J., Elguindi, N., Darras, S., Granier, C., and Pérez García-Pando, C.: Copernicus Atmosphere Monitoring Service TEMPOral profiles (CAMS-TEMPO): global and European emission temporal profile maps for atmospheric chemistry modelling, Earth Syst. Sci. Data, 13, 367–404, https://doi.org/10.5194/essd-13-367-2021, 2021.
Guo, H., Xu, L., Bougiatioti, A., Cerully, K. M., Capps, S. L., Hite Jr., J. R., Carlton, A. G., Lee, S.-H., Bergin, M. H., Ng, N. L., Nenes, A., and Weber, R. J.: Fine-particle water and pH in the southeastern United States, Atmos. Chem. Phys., 15, 5211–5228, https://doi.org/10.5194/acp-15-5211-2015, 2015.
Guo, H., Sullivan, A. P., Campuzano-Jost, P., Schroder, J. C., Lopez-Hilfiker, F. D., Dibb, J. E., Jimenez, J. L., Thornton, J. A., Brown, S. S., Nenes, A., and Weber, R. J.: Fine particle pH and the partitioning of nitric acid during winter in the northeastern United States, J. Geophys. Res.-Atmos., 121, 10355–10376, https://doi.org/10.1002/2016JD025311, 2016.
Guo, H., Liu, J., Froyd, K. D., Roberts, J. M., Veres, P. R., Hayes, P. L., Jimenez, J. L., Nenes, A., and Weber, R. J.: Fine particle pH and gas–particle phase partitioning of inorganic species in Pasadena, California, during the 2010 CalNex campaign, Atmos. Chem. Phys., 17, 5703–5719, https://doi.org/10.5194/acp-17-5703-2017, 2017a.
Guo, H., Weber, R. J., and Nenes, A.: High levels of ammonia do not raise fine particle pH sufficiently to yield nitrogen oxide-dominated sulfate production, Sci. Rep.-UK, 7, 12109, https://doi.org/10.1038/s41598-017-11704-0, 2017b.
Guo, H., Nenes, A., and Weber, R. J.: The underappreciated role of nonvolatile cations in aerosol ammonium-sulfate molar ratios, Atmos. Chem. Phys., 18, 17307–17323, https://doi.org/10.5194/acp-18-17307-2018, 2018.
Hamer, P. D., Walker, S.-E., Sousa-Santos, G., Vogt, M., Vo-Thanh, D., Lopez-Aparicio, S., Schneider, P., Ramacher, M. O. P., and Karl, M.: The urban dispersion model EPISODE v10.0 – Part 1: An Eulerian and sub-grid-scale air quality model and its application in Nordic winter conditions, Geosci. Model Dev., 13, 4323–4353, https://doi.org/10.5194/gmd-13-4323-2020, 2020.
Harris, E., Sinha, B., Pinxteren, D. V., Tilgner, A., Fomba, K. W., Schneider, J., Roth, A., Gnauk, T., Fahlbusch, B., Mertes, S., Lee, T., Collett, J., Foley, S., Borrmann, S., Hoppe, P., and Herrmann, H.: Enhanced Role of Transition Metal, Science, 340, 727–730, https://doi.org/10.1126/science.1230911, 2013.
Hennigan, C. J., Izumi, J., Sullivan, A. P., Weber, R. J., and Nenes, A.: A critical evaluation of proxy methods used to estimate the acidity of atmospheric particles, Atmos. Chem. Phys., 15, 2775–2790, https://doi.org/10.5194/acp-15-2775-2015, 2015.
Hersbach, H., Bell, B., Berrisford, P., Hirahara, S., Horányi, A., Muñoz-Sabater, J., Nicolas, J., Peubey, C., Radu, R., Schepers, D., Simmons, A., Soci, C., Abdalla, S., Abellan, X., Balsamo, G., Bechtold, P., Biavati, G., Bidlot, J., Bonavita, M., De Chiara, G., Dahlgren, P., Dee, D., Diamantakis, M., Dragani, R., Flemming, J., Forbes, R., Fuentes, M., Geer, A., Haimberger, L., Healy, S., Hogan, R. J., Hólm, E., Janisková, M., Keeley, S., Laloyaux, P., Lopez, P., Lupu, C., Radnoti, G., de Rosnay, P., Rozum, I., Vamborg, F., Villaume, S., and Thépaut, J.-N.: The ERA5 global reanalysis, Q. J. Roy. Meteorol. Soc., 146, 1999–2049, https://doi.org/10.1002/qj.3803, 2020.
Hurley, P. J., Physick, W. L., and Luhar, A. K.: TAPM: a practical approach to prognostic meteorological and air pollution modelling, Environ. Modell. Softw., 20, 737–752, https://doi.org/10.1016/j.envsoft.2004.04.006, 2005.
Im, U., Christodoulaki, S., Violaki, K., Zarmpas, P., Kocak, M., Daskalakis, N., Mihalopoulos, N., and Kanakidou, M.: Atmospheric deposition of nitrogen and sulfur over southern Europe with focus on the Mediterranean and the Black Sea, Atmos. Environ., 81, 660–670, https://doi.org/10.1016/j.atmosenv.2013.09.048, 2013.
IPCC – Intergovernmental Panel on Climate Change (Ed.): Anthropogenic and Natural Radiative Forcing, in: Climate Change 2013 – The Physical Science Basis: Working Group I Contribution to the Fifth Assessment Report of the Intergovernmental Panel on Climate Change, Cambridge University Press, Cambridge, 659–740, https://doi.org/10.1017/CBO9781107415324.018, 2014.
Janjić, Z. I.: The Step-Mountain Eta Coordinate Model: Further Developments of the Convection, Viscous Sublayer, and Turbulence Closure Schemes, Mon. Weather Rev., 122, 927–945, https://doi.org/10.1175/1520-0493(1994)122<0927:TSMECM>2.0.CO;2, 1994.
Janjić, Z. I.: Nonsingular implementation of the Mellor-Yamada Level 2.5 Scheme in the NCEP Meso model, NCEP Office Note No. 437, NCEP, 61 pp., https://repository.library.noaa.gov/view/noaa/11409 (last access: 8 June 2024), 2002.
Karl, M.: City-scale Chemistry Transport Model EPISODE-CityChem (1.8), Zenodo [code], https://doi.org/10.5281/zenodo.8063985, 2023.
Karl, M. and Ramacher, M. O. P.: Urban Atmospheric Chemistry with the EPISODE-CityChem Model, in: Air Pollution Modeling and its Application XXVII, edited by: Mensink, C. and Matthias, V., Springer Berlin Heidelberg, Berlin, Heidelberg, 235–239, https://doi.org/10.1007/978-3-662-63760-9_33, 2021.
Karl, M., Walker, S.-E., Solberg, S., and Ramacher, M. O. P.: The Eulerian urban dispersion model EPISODE – Part 2: Extensions to the source dispersion and photochemistry for EPISODE–CityChem v1.2 and its application to the city of Hamburg, Geosci. Model Dev., 12, 3357–3399, https://doi.org/10.5194/gmd-12-3357-2019, 2019.
Karl, M., Ramacher, M. O. P., Oppo, S., Lanzi, L., Majamäki, E., Jalkanen, J.-P., Lanzafame, G. M., Temime-Roussel, B., Le Berre, L., and D'Anna, B.: Measurement and Modeling of Ship-Related Ultrafine Particles and Secondary Organic Aerosols in a Mediterranean Port City, Toxics, 11, 771, https://doi.org/10.3390/toxics11090771, 2023.
Karydis, V. A., Tsimpidi, A. P., Pozzer, A., Astitha, M., and Lelieveld, J.: Effects of mineral dust on global atmospheric nitrate concentrations, Atmos. Chem. Phys., 16, 1491–1509, https://doi.org/10.5194/acp-16-1491-2016, 2016.
Koçak, M., Kubilay, N., and Mihalopoulos, N.: Ionic composition of lower tropospheric aerosols at a Northeastern Mediterranean site: implications regarding sources and long-range transport, Atmos. Environ., 38, 2067–2077, https://doi.org/10.1016/j.atmosenv.2004.01.030, 2004.
Koçak, M., Theodosi, C., Zarmpas, P., Séguret, M. J. M., Herut, B., Kallos, G., Mihalopoulos, N., Kubilay, N., and Nimmo, M.: Influence of mineral dust transport on the chemical composition and physical properties of the Eastern Mediterranean aerosol, Atmos. Environ., 57, 266–277, https://doi.org/10.1016/j.atmosenv.2012.04.006, 2012.
Kontos, S., Kakosimos, K., Liora, N., Poupkou, A., and Melas, D.: Towards a regional dust modeling system in the central Middle East: Evaluation, uncertainties and recommendations, Atmos. Environ., 246, 118160, https://doi.org/10.1016/j.atmosenv.2020.118160, 2021.
Koulouri, E., Saarikoski, S., Theodosi, C., Markaki, Z., Gerasopoulos, E., Kouvarakis, G., Mäkelä, T., Hillamo, R., and Mihalopoulos, N.: Chemical composition and sources of fine and coarse aerosol particles in the Eastern Mediterranean, Atmos. Environ., 42, 6542–6550, https://doi.org/10.1016/j.atmosenv.2008.04.010, 2008.
Kushta, J., Georgiou, G. K., Proestos, Y., Christoudias, T., Thunis, P., Savvides, C., Papadopoulos, C., and Lelieveld, J.: Evaluation of EU air quality standards through modeling and the FAIRMODE benchmarking methodology, Air Qual. Atmos. Hlth., 12, 73–86, https://doi.org/10.1007/s11869-018-0631-z, 2019.
Lasne, J., Lostier, A., Salameh, T., Athanasopoulou, E., Karagiannis, D., Kakouri, A., Vassaux, S., Lesueur, D., and Romanias, M. N.: NOx emissions by real-world fresh and old asphalt mixtures: Impact of temperature, relative humidity, and UV-irradiation, Urban Climate, 49, 101457, https://doi.org/10.1016/j.uclim.2023.101457, 2023.
Lawal, A. S., Guan, X., Liu, C., Henneman, L. R. F., Vasilakos, P., Bhogineni, V., Weber, R. J., Nenes, A., and Russell, A. G.: Linked Response of Aerosol Acidity and Ammonia to SO2 and NOx Emissions Reductions in the United States, Environ. Sci. Technol., 52, 9861–9873, https://doi.org/10.1021/acs.est.8b00711, 2018.
Lee-Taylor, J. and Madronich, S.: Calculation of actinic fluxes with a coupled atmosphere–snow radiative transfer model, J. Geophys. Res., 107, 660–670, https://doi.org/10.1029/2002JD002084, 2002.
Liakakou, E., Fourtziou, L., Paraskevopoulou, D., Speyer, O., Lianou, M., Grivas, G., Myriokefalitakis, S., and Mihalopoulos, N.: High-Resolution Measurements of SO2, HNO3 and HCl at the Urban Environment of Athens, Greece: Levels, Variability and Gas to Particle Partitioning, Atmosphere, 13, 218, https://doi.org/10.3390/atmos13020218, 2022.
Liakakou, E., Mihalopoulos, N., Theodosi, C., Tsiodra, E., Kaskaoutis, D. G., Koukouli, M.-E., Balis, D., Kharol, S. K., Shephard, M. W., Dammers, E., and Cady-Pereira, K. E.: Inorganic Aerosol Precursors in the Mediterranean Atmosphere, in: Atmospheric Chemistry in the Mediterranean Region: Vol. 1 – Background Information and Pollutant Distribution, edited by: Dulac, F., Sauvage, S., and Hamonou, E., Springer International Publishing, Cham, 471–503, https://doi.org/10.1007/978-3-031-12741-0_14, 2023.
Liao, H., Adams, P. J., Chung, S. H., Seinfeld, J. H., Mickley, L. J., and Jacob, D. J.: Interactions between tropospheric chemistry and aerosols in a unified general circulation model, J. Geophys. Res., 108, 4001, https://doi.org/10.1029/2001JD001260, 2003.
Liora, N., Kontos, S., Parliari, D., Akritidis, D., Poupkou, A., Papanastasiou, D. K., and Melas, D.: “On-Line” Heating Emissions Based on WRF Meteorology – Application and Evaluation of a Modeling System over Greece, Atmosphere, 13, 568, https://doi.org/10.3390/atmos13040568, 2022.
Liu, M., Huang, X., Song, Y., Tang, J., Cao, J., Zhang, X., Zhang, Q., Wang, S., Xu, T., Kang, L., Cai, X., Zhang, H., Yang, F., Wang, H., Yu, J. Z., Lau, A. K. H., He, L., Huang, X., Duan, L., Ding, A., Xue, L., Gao, J., Liu, B., and Zhu, T.: Ammonia emission control in China would mitigate haze pollution and nitrogen deposition, but worsen acid rain, P. Natl. Acad. Sci. USA, 116, 7760–7765, https://doi.org/10.1073/pnas.1814880116, 2019.
Liu, T., Chan, A. W. H., and Abbatt, J. P. D.: Multiphase Oxidation of Sulfur Dioxide in Aerosol Particles: Implications for Sulfate Formation in Polluted Environments, Environ. Sci. Technol., 55, 4227, https://doi.org/10.1021/acs.est.0c06496, 2021.
Mantas, E., Remoundaki, E., Halari, I., Kassomenos, P., Theodosi, C., Hatzikioseyian, A., and Mihalopoulos, N.: Mass closure and source apportionment of PM2.5 by Positive Matrix Factorization analysis in urban Mediterranean environment, Atmos. Environ., 94, 154–163, https://doi.org/10.1016/j.atmosenv.2014.05.002, 2014.
Myriokefalitakis, S., Daskalakis, N., Gkouvousis, A., Hilboll, A., van Noije, T., Williams, J. E., Le Sager, P., Huijnen, V., Houweling, S., Bergman, T., Nüß, J. R., Vrekoussis, M., Kanakidou, M., and Krol, M. C.: Description and evaluation of a detailed gas-phase chemistry scheme in the TM5-MP global chemistry transport model (r112), Geosci. Model Dev., 13, 5507–5548, https://doi.org/10.5194/gmd-13-5507-2020, 2020.
Myriokefalitakis, S., Bergas-Massó, E., Gonçalves-Ageitos, M., Pérez García-Pando, C., van Noije, T., Le Sager, P., Ito, A., Athanasopoulou, E., Nenes, A., Kanakidou, M., Krol, M. C., and Gerasopoulos, E.: Multiphase processes in the EC-Earth model and their relevance to the atmospheric oxalate, sulfate, and iron cycles, Geosci. Model Dev., 15, 3079–3120, https://doi.org/10.5194/gmd-15-3079-2022, 2022.
Nenes, A., Pandis, S. N., and Pilinis, C.: ISORROPIA: A New Thermodynamic Equilibrium Model for Multiphase Multicomponent Inorganic Aerosols, Aquat. Geochem., 4, 123–152, https://doi.org/10.1023/A:1009604003981, 1998.
Nenes, A., Pandis, S. N., Weber, R. J., and Russell, A.: Aerosol pH and liquid water content determine when particulate matter is sensitive to ammonia and nitrate availability, Atmos. Chem. Phys., 20, 3249–3258, https://doi.org/10.5194/acp-20-3249-2020, 2020.
Ng, N. L., Herndon, S. C., Trimborn, A., Canagaratna, M. R., Croteau, P. L., Onasch, T. B., Sueper, D., Worsnop, D. R., Zhang, Q., Sun, Y. L., and Jayne, J. T.: An Aerosol Chemical Speciation Monitor (ACSM) for Routine Monitoring of the Composition and Mass Concentrations of Ambient Aerosol, Aerosol Sci. Tech., 45, 780–794, https://doi.org/10.1080/02786826.2011.560211, 2011.
Ozga, I., Bonazza, A., Ait Lyazidi, S., Haddad, M., Ben-Ncer, A., Ghedini, N., and Sabbioni, C.: Pollution impact on the ancient ramparts of the Moroccan city Salé, J. Cult. Herit., 14, S25–S33, https://doi.org/10.1016/j.culher.2012.10.018, 2013.
Panopoulou, A., Liakakou, E., Sauvage, S., Gros, V., Locoge, N., Stavroulas, I., Bonsang, B., Gerasopoulos, E., and Mihalopoulos, N.: Yearlong measurements of monoterpenes and isoprene in a Mediterranean city (Athens): Natural vs. anthropogenic origin, Atmos. Environ., 243, 117803, https://doi.org/10.1016/j.atmosenv.2020.117803, 2020.
Paraskevopoulou, D., Liakakou, E., Gerasopoulos, E., Theodosi, C., and Mihalopoulos, N.: Long-term characterization of organic and elemental carbon in the PM2.5 fraction: the case of Athens, Greece, Atmos. Chem. Phys., 14, 13313–13325, https://doi.org/10.5194/acp-14-13313-2014, 2014.
Paraskevopoulou, D., Liakakou, E., Gerasopoulos, E., and Mihalopoulos, N.: Sources of atmospheric aerosol from long-term measurements (5 years) of chemical composition in Athens, Greece, Sci. Total Environ., 527–528, 165–178, https://doi.org/10.1016/j.scitotenv.2015.04.022, 2015.
Pope, C. A., Coleman, N., Pond, Z. A., and Burnett, R. T.: Fine particulate air pollution and human mortality: 25+ years of cohort studies, Environ. Res., 183, 108924, https://doi.org/10.1016/j.envres.2019.108924, 2020.
Pringle, K. J., Tost, H., Metzger, S., Steil, B., Giannadaki, D., Nenes, A., Fountoukis, C., Stier, P., Vignati, E., and Lelieveld, J.: Corrigendum to “Description and evaluation of GMXe: a new aerosol submodel for global simulations (v1)” published in Geosci. Model Dev., 3, 391–412, 2010, Geosci. Model Dev., 3, 413–413, https://doi.org/10.5194/gmd-3-413-2010, 2010.
Pye, H. O. T., Nenes, A., Alexander, B., Ault, A. P., Barth, M. C., Clegg, S. L., Collett Jr., J. L., Fahey, K. M., Hennigan, C. J., Herrmann, H., Kanakidou, M., Kelly, J. T., Ku, I.-T., McNeill, V. F., Riemer, N., Schaefer, T., Shi, G., Tilgner, A., Walker, J. T., Wang, T., Weber, R., Xing, J., Zaveri, R. A., and Zuend, A.: The acidity of atmospheric particles and clouds, Atmos. Chem. Phys., 20, 4809–4888, https://doi.org/10.5194/acp-20-4809-2020, 2020.
Ramacher, M. O. P., Kakouri, A., Speyer, O., Feldner, J., Karl, M., Timmermans, R., Denier van der Gon, H., Kuenen, J., Gerasopoulos, E., and Athanasopoulou, E.: The UrbEm Hybrid Method to Derive High-Resolution Emissions for City-Scale Air Quality Modeling, Atmosphere, 12, 1404, https://doi.org/10.3390/atmos12111404, 2021.
Salter, M. E., Zieger, P., Acosta Navarro, J. C., Grythe, H., Kirkevåg, A., Rosati, B., Riipinen, I., and Nilsson, E. D.: An empirically derived inorganic sea spray source function incorporating sea surface temperature, Atmos. Chem. Phys., 15, 11047–11066, https://doi.org/10.5194/acp-15-11047-2015, 2015.
Sander, R.: Compilation of Henry's law constants (version 4.0) for water as solvent, Atmos. Chem. Phys., 15, 4399–4981, https://doi.org/10.5194/acp-15-4399-2015, 2015.
Sandu, A. and Sander, R.: Technical note: Simulating chemical systems in Fortran90 and Matlab with the Kinetic PreProcessor KPP-2.1, Atmos. Chem. Phys., 6, 187–195, https://doi.org/10.5194/acp-6-187-2006, 2006.
Santiago, J. L., Rivas, E., Gamarra, A. R., Vivanco, M. G., Buccolieri, R., Martilli, A., Lechón, Y., and Martín, F.: Estimates of population exposure to atmospheric pollution and health-related externalities in a real city: The impact of spatial resolution on the accuracy of results, Sci. Total Environ., 819, 152062, https://doi.org/10.1016/j.scitotenv.2021.152062, 2022.
Sarnat, S. E., Winquist, A., Schauer, J. J., Turner, J. R., and Sarnat, J. A.: Fine Particulate Matter Components and Emergency Department Visits for Cardiovascular and Respiratory Diseases in the St. Louis, Missouri–Illinois, Metropolitan Area, Environ. Health Persp., 123, 437–444, https://doi.org/10.1289/ehp.1307776, 2015.
Sciare, J., Oikonomou, K., Cachier, H., Mihalopoulos, N., Andreae, M. O., Maenhaut, W., and Sarda-Estève, R.: Aerosol mass closure and reconstruction of the light scattering coefficient over the Eastern Mediterranean Sea during the MINOS campaign, Atmos. Chem. Phys., 5, 2253–2265, https://doi.org/10.5194/acp-5-2253-2005, 2005.
Seinfeld, J. H. and Pandis, S. N.: Atmospheric chemistry and physics: from air pollution to climate change, in: 2nd Edn., J. Wiley, Hoboken, NJ, 1203 pp., ISBN 978-0-471-72017-1, ISBN 978-0-471-72018-8, 2006.
Skamarock, C., Klemp, B., Dudhia, J., Gill, O., Liu, Z., Berner, J., Wang, W., Powers, G., Duda, G., Barker, D., and Huang, X.: A Description of the Advanced Research WRF Model Version 4.3, UCAR, https://doi.org/10.5065/1dfh-6p97, 2021.
Slørdal, L. H., McInnes, H., and Krognes, T.: The Air Quality Management System AirQUIS, in: Information Technologies in Environmental Engineering, Springer, erlin, Heidelberg, 21–33, https://doi.org/10.1007/978-3-540-71335-7_6, 2007.
Sokhi, R. S., Moussiopoulos, N., Baklanov, A., Bartzis, J., Coll, I., Finardi, S., Friedrich, R., Geels, C., Grönholm, T., Halenka, T., Ketzel, M., Maragkidou, A., Matthias, V., Moldanova, J., Ntziachristos, L., Schäfer, K., Suppan, P., Tsegas, G., Carmichael, G., Franco, V., Hanna, S., Jalkanen, J.-P., Velders, G. J. M., and Kukkonen, J.: Advances in air quality research – current and emerging challenges, Atmos. Chem. Phys., 22, 4615–4703, https://doi.org/10.5194/acp-22-4615-2022, 2022.
Sposito, G.: The Chemistry of Soils, Oxford University Press, Oxford, 277 pp., ISBN 9780195313697, 1989.
Stavroulas, I., Bougiatioti, A., Grivas, G., Paraskevopoulou, D., Tsagkaraki, M., Zarmpas, P., Liakakou, E., Gerasopoulos, E., and Mihalopoulos, N.: Sources and processes that control the submicron organic aerosol composition in an urban Mediterranean environment (Athens): a high temporal-resolution chemical composition measurement study, Atmos. Chem. Phys., 19, 901–919, https://doi.org/10.5194/acp-19-901-2019, 2019.
Stavroulas, I., Grivas, G., Liakakou, E., Kalkavouras, P., Bougiatioti, A., Kaskaoutis, D. G., Lianou, M., Papoutsidaki, K., Tsagkaraki, M., Zarmpas, P., Gerasopoulos, E., and Mihalopoulos, N.: Online Chemical Characterization and Sources of Submicron Aerosol in the Major Mediterranean Port City of Piraeus, Greece, Atmosphere, 12, 1686, https://doi.org/10.3390/atmos12121686, 2021.
Tewari, M., Chen, F., Wang, W., Dudhia, J., Lemone, A., Mitchell, E., Ek, M., Gayno, G., Wegiel, W., and Cuenca, R.: Implementation and verification of the unified Noah land-surface model in the WRF model [presentation], http://n2t.net/ark:/85065/d7fb523p (last access: 21 June 2023), 2004.
Theodosi, C., Tsagkaraki, M., Zarmpas, P., Grivas, G., Liakakou, E., Paraskevopoulou, D., Lianou, M., Gerasopoulos, E., and Mihalopoulos, N.: Multi-year chemical composition of the fine-aerosol fraction in Athens, Greece, with emphasis on the contribution of residential heating in wintertime, Atmos. Chem. Phys., 18, 14371–14391, https://doi.org/10.5194/acp-18-14371-2018, 2018.
Thompson, G., Field, P. R., Rasmussen, R. M., and Hall, W. D.: Explicit Forecasts of Winter Precipitation Using an Improved Bulk Microphysics Scheme. Part II: Implementation of a New Snow Parameterization, Mon. Weather Rev., 136, 5095–5115, https://doi.org/10.1175/2008MWR2387.1, 2008.
Vignati, E., Facchini, M. C., Rinaldi, M., Scannell, C., Ceburnis, D., Sciare, J., Kanakidou, M., Myriokefalitakis, S., Dentener, F., and ODowd, C. D.: Global scale emission and distribution of sea-spray aerosol: Sea-salt and organic enrichment, Atmos. Environ., 44, 670–677, https://doi.org/10.1016/j.atmosenv.2009.11.013, 2010.
Wang, T., Liu, Y., Cheng, H., Wang, Z., Fu, H., Chen, J., and Zhang, L.: Significant formation of sulfate aerosols contributed by the heterogeneous drivers of dust surface, Atmos. Chem. Phys., 22, 13467–13493, https://doi.org/10.5194/acp-22-13467-2022, 2022.
Watmough, S. A., Eimers, C., and Baker, S.: Impediments to recovery from acid deposition, Atmos. Environ., 146, 15–27, https://doi.org/10.1016/j.atmosenv.2016.03.021, 2016.
WHO: WHO global air quality guidelines: particulate matter (PM2.5 and PM10), ozone, nitrogen dioxide, sulfur dioxide and carbon monoxide, 273 pp., ISBN 9789240034228, 2021.
Williams, J. E., van Velthoven, P. F. J., and Brenninkmeijer, C. A. M.: Quantifying the uncertainty in simulating global tropospheric composition due to the variability in global emission estimates of Biogenic Volatile Organic Compounds, Atmos. Chem. Phys., 13, 2857–2891, https://doi.org/10.5194/acp-13-2857-2013, 2013.
Williams, J. E., Boersma, K. F., Le Sager, P., and Verstraeten, W. W.: The high-resolution version of TM5-MP for optimized satellite retrievals: description and validation, Geosci. Model Dev., 10, 721–750, https://doi.org/10.5194/gmd-10-721-2017, 2017.
Yarwood, G., Rao, S., and Yocke, M.: Updates to the carbon bond chemical mechanism: CB05 – Prepared for Deborah Luecken, U.S. Environmental Protection Agency Research Triangle Park, NC 27703, Yocke and Company, https://www.camx.com/Files/CB05_Final_Report_120805.pdf (last access: 16 March 2017), 2005.
Zakoura, M. and Pandis, S. N.: Overprediction of aerosol nitrate by chemical transport models: The role of grid resolution, Atmos. Environ., 187, 390–400, https://doi.org/10.1016/j.atmosenv.2018.05.066, 2018.
Zhang, L., Wang, T., Zhang, Q., Zheng, J., Xu, Z., and Lv, M.: Potential sources of nitrous acid (HONO) and their impacts on ozone: A WRF-Chem study in a polluted subtropical region: Modeling HONO in a Subtropical Region, J. Geophys. Res.-Atmos., 121, 3645–3662, https://doi.org/10.1002/2015JD024468, 2016.
Zhang, Y., Yang, Y., Zhang, L., Xu, H., Sun, J., Wang, T., Li, F., Chang, X., Ho, S. S. H., Li, B., Wang, B., Cao, J., and Shen, Z.: Insight into the contributions of primary emissions of sulfate, nitrate, and ammonium from residential solid fuels to ambient PM2.5, Atmos. Res., 290, 106790, https://doi.org/10.1016/j.atmosres.2023.106790, 2023.
Zhang, Z., Gao, J., Engling, G., Tao, J., Chai, F., Zhang, L., Zhang, R., Sang, X., Chan, C., Lin, Z., and Cao, J.: Characteristics and applications of size-segregated biomass burning tracers in China's Pearl River Delta region, Atmos. Environ., 102, 290–301, https://doi.org/10.1016/j.atmosenv.2014.12.009, 2015.
Zhang, Z.-H., Hartner, E., Utinger, B., Gfeller, B., Paul, A., Sklorz, M., Czech, H., Yang, B. X., Su, X. Y., Jakobi, G., Orasche, J., Schnelle-Kreis, J., Jeong, S., Gröger, T., Pardo, M., Hohaus, T., Adam, T., Kiendler-Scharr, A., Rudich, Y., Zimmermann, R., and Kalberer, M.: Are reactive oxygen species (ROS) a suitable metric to predict toxicity of carbonaceous aerosol particles?, Atmos. Chem. Phys., 22, 1793–1809, https://doi.org/10.5194/acp-22-1793-2022, 2022.
A state-of-the-art thermodynamic model has been coupled with the city-scale chemistry transport model EPISODE–CityChem to investigate the equilibrium between the inorganic gas and aerosol phases over the greater Athens area, Greece. The simulations indicate that the formation of nitrates in an urban environment is significantly affected by local nitrogen oxide emissions, as well as ambient temperature, relative humidity, photochemical activity, and the presence of non-volatile cations.
A state-of-the-art thermodynamic model has been coupled with the city-scale chemistry transport...