the Creative Commons Attribution 4.0 License.
the Creative Commons Attribution 4.0 License.
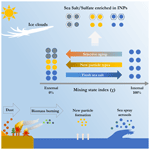
Diverse sources and aging change the mixing state and ice nucleation properties of aerosol particles over the western Pacific and Southern Ocean
Jiao Xue
Tian Zhang
Keyhong Park
Jinpei Yan
Young Jun Yoon
Jiyeon Park
Bingbing Wang
Atmospheric particles can impact cloud formation and play a critical role in regulating cloud properties. However, particle characteristics at the single-particle level and their ability to act as ice-nucleating particles (INPs) over the marine atmosphere are poorly understood. In this study, we present micro-spectroscopic characterizations and ice nucleation properties of particles collected during a cruise from South Korea to Antarctica in 2019. Most of the samples were dominated by fresh sea salt, aged sea salt, and sea salt mixed with sulfate particles, with total number percentages ranging from 48 % to 99 % over the western Pacific and the Southern Ocean. The mixing-state index of the particle population ranged from 50 % to 95 % over the Northern Hemisphere and Southern Hemisphere. Multiphase processes on sea salt particles resulted in chlorine deficiency. This selective aging process made the marine particle population more externally mixed. Ice nucleation onset conditions primarily for the deposition mode were measured and the investigated particles showed diverse ice nucleation abilities. The fresh sea salt particles with organic coatings exhibited the highest ice nucleation ability at a relative humidity with respect to ice as low as 121 %. The sea salt mixed sulfate particle was enriched in INPs by a factor of 1.9. Aging processes affected both the mixing state of the particles and their ice nucleation abilities. Our analysis shows that assuming an internally mixed particle population in the marine atmosphere can lead to errors of several orders of magnitude in predicting ice nucleation rates.
- Article
(11692 KB) - Full-text XML
-
Supplement
(3713 KB) - BibTeX
- EndNote
As 70.8 % of the Earth's surface is covered by oceans, marine aerosol particles are one of the most important types of natural aerosols in the global inventory (Myriokefalitakis et al., 2010; De Leeuw et al., 2011). Aerosols in marine environments can affect the ocean biogeochemical cycles and indirectly or directly affect the Earth system's radiation budget (Song et al., 2022). The sixth Intergovernmental Panel on Climate Change (IPCC) assessment report highlights a significant uncertainty in predicting the net effective radiative forcing of aerosols, with aerosol-cloud interactions contributing the most (IPCC, 2021). The impacts of atmospheric particles on cloud microphysical processes are still poorly understood. The physicochemical properties of particles determine their abilities to serve as cloud condensation nuclei (CCN) and ice-nucleating particles (INPs), thereby affecting cloud microphysical processes. Particles serving as INPs can potentially trigger ice nucleation via four pathways: (1) deposition ice nucleation (DIN), which forms ice by the direct deposition of water vapor onto particle surface; (2) immersion freezing (IMF), in which droplet freezing is triggered by immersed particles; (3) contact freezing, when supercooled droplets freeze by the contact with particles; and (4) condensation freezing, when droplets freeze as water vapor condenses on droplets or particles at temperatures below 0 °C. The majority of recent studies have focused on the IMF and DIN under mixed-phase and ice cloud conditions (Hoose and Möhler, 2012; Murray et al., 2012; Kanji et al., 2017; Knopf et al., 2018).
Diverse sources and atmospheric processes bring great challenges to the study of the physicochemical properties of marine particles. One of the major particle types in the marine atmosphere is sea spray aerosol (SSA) generated from wave breaking and bubble bursting over the ocean surface. Their compositions are mainly affected by seawater, sea ice, and biological activities and have minimum influence from anthropogenic activities (Kunwar et al., 2023). Other sources may also contribute to marine aerosols, such as ship emission (Ault et al., 2009, 2010) and long-range transport of aerosols from industrial emission, biomass burning, dust storms, and fossil fuel combustion (Han et al., 2006; Fu et al., 2013; Geng et al., 2019). In addition, atmospheric oxidation of volatile organic compounds from the ocean or anthropogenic activities results in gas-particle conversion, multiphase reactions, and the formation of secondary materials on particles (Cochran et al., 2017). For example, dimethyl sulfide (DMS) from marine sources can be oxidized to form methanesulfonic acid (MSA) and sulfuric acid (H2SO4) in the atmosphere (Barnes et al., 2006; Chen et al., 2018; Berndt et al., 2023). Moreover, multiphase reactions can occur on the surface or within particles, causing changes in their physicochemical properties of particles (Cochran et al., 2017). The oxidation products of DMS (H2SO4 and MSA) can react with sea salt particles, resulting in chloride depletion from acid displacements (Liu et al., 2011).
Due to their diverse sources and dynamic evolution in the atmosphere, individual particles become a mixture of various compositions and have complex morphologies (Riemer et al., 2019; Li et al., 2016). Chemical mixing state is a term used to describe how various chemical species are mixed within individual particles in an aerosol population (Riemer and West, 2013). Quantifying the chemical mixing state helps us to discuss how the population with different internal or external mixing affects its optical properties, cloud formation properties, and climatic impacts (Cziczo et al., 2017; Kanji et al., 2017; Knopf et al., 2018; Riemer et al., 2019; Burrows et al., 2022). Previous studies have quantified the chemical mixing state of an aerosol population using the mixing-state index (χ), which is based on the mass percentages of various chemical species in single particles within a population (Riemer et al., 2019). Thus, chemical characteristics of individual particles should be achieved by single-particle techniques to quantify χ. Previous studies have focused on mixing states, including (but not limited to) marine particles (Ault et al., 2010; Park et al., 2014; Tomlin et al., 2021), biomass burning aerosol (BBA) (Tomlin et al., 2022; Healy et al., 2013), and dust particles (Fraund et al., 2017; Adachi et al., 2020). However, the impacts of particle sources and atmospheric aging on the chemical mixing state of marine particles are not well understood.
Laboratory and field studies have shown that various particle types have potential impacts on ice crystal formation in marine atmosphere. Natural INPs include mineral dust, soil particles, volcanic ash, SSA, BBA, and bioaerosols. Anthropogenic INPs include agricultural particles, metals and metal oxides from industrial processes, and fossil fuel combustion particles (Hoose and Möhler, 2012; Murray et al., 2012; Kanji et al., 2017). Laboratory studies have shown that SSA, marine phytoplankton, and related organics can serve as INPs for both IMF and DIN (e.g., Knopf et al., 2011; Prather et al., 2013; Wilson et al., 2015; McCluskey et al., 2017; Schill and Tolbert, 2014; Wagner et al., 2018). SSA can be efficient INPs via IMF before complete deliquescence (Schill and Tolbert, 2014). Wagner et al. (2018) showed that SSA and desert dust particles have similar ice nucleation active surface site density (ns) for DIN. Previous studies have also shown that ns for SSA is 2–3 orders of magnitude lower than that for dust particles for IMF (DeMott et al., 2016; McCluskey et al., 2018a; Cornwell et al., 2019). Knopf et al. (2011) confirmed that intact cells or fragments of marine diatoms can act as INPs. Wilson et al. (2015) demonstrated that the sea surface microlayer is enriched with ice-nucleating materials. McCluskey et al. (2018b) identified two types of INPs from mesocosm experiments, which were dissolved organic-carbon-coated particles and particles like intact cells or cell fragments from microorganisms.
Most of the field investigations over oceans have focused on the INP concentration measurements for IMF and at mixed-phase cloud conditions with relatively warmer temperatures (Bigg, 1973; Rosinski et al., 1995; DeMott et al., 2016; Mason et al., 2015; McCluskey et al., 2018b; Welti et al., 2018, 2020; Ladino et al., 2019). For example, Welti et al. (2020) found that INP concentration from ship-based measurements over the oceans were 1 to 2 orders of magnitude lower than continental observations. INP concentrations were lowest in the polar regions and highest in the temperate climate ocean at about 258 K (Welti et al., 2020). Inoue et al. (2021) found high INP concentrations under high wave conditions which were related to the release of organic carbon from the ocean. Studies also found that active INPs via IMF are likely biological particles (e.g., Hartmann et al., 2020; McCluskey et al., 2018b; Gong et al., 2020).
There are several marine-related field studies focusing on DIN and INP identification (e.g., Ladino et al., 2016; China et al., 2017; Alpert et al., 2022; Knopf et al., 2022, 2023). Alpert et al. (2022) confirmed that INPs in the ambient and laboratory-generated SSA were the sea salt with organic matter, which were exudates released from planktonic microorganisms. Over the northeastern Pacific Ocean, Knopf et al. (2022, 2023) found different ice nucleation abilities between daytime and nighttime particles in marine boundary layer and between particles in the free troposphere and that in marine boundary layer. Recent field studies have used advanced micro-spectroscopes and mass spectrometry to image and characterize the INPs and ice crystal residuals to understand their nature (e.g., Cziczo et al., 2017; Knopf et al., 2018). INPs and ice residuals are often mixtures of chemical species. Ice nucleation is inherently related to the mixing state of particles because it depends on the particle surface properties. Quantifying the ice nucleation rate also depends on the composition, surface area, and the mixing state of the particle population. The importance of mixing state for INPs is well recognized, but there are limited studies focusing on its quantification, which is poorly understood (Kanji et al., 2017; Knopf et al., 2018; Riemer et al., 2019).
In this study, our main objectives are to quantify the mixing state and ice nucleation ability of marine particles over the western Pacific and Southern Ocean. We quantify ice formation potential and elemental composition of particles over coastal and open oceans using microscope-based ice nucleation instrumentation and computer-controlled scanning electron microscopy with energy dispersive X-ray spectroscopy (CCSEM/EDX), respectively. The mixing state of particle population based on the elemental composition is derived for 29 marine ambient particle populations extending from the Northern Hemisphere to the Southern Hemisphere. We measure the ice nucleation onset conditions and identify the nucleation pathways (DIN or IMF) for representative samples. In this study, we focus primarily on DIN and in some cases IMF below 240 K. Individual identified INPs are characterized and compared with the particle population to relate ice nucleation ability to the mixing state. Potential errors in predicting ice nucleation rate are briefly discussed if models assume an internally mixed population. We perform ice nucleation kinetic analysis based on the experimental data and provide parameterizations for cloud modeling.
2.1 Particle sampling
Aerosol particles were collected by a four-stage cascade impactor (SKC, Inc.) at a sample flow of 9 L min−1 on board the Korean ice breaker R/V Araon from 31 October to 12 December in 2019. Particles were collected on the third and fourth stages of the impactor with 50 % collection efficiency at aerodynamic sizes of 0.5 and 0.25 µm, respectively. The inlet was located on the third deck of the ship at about 13 m above sea surface level (Park et al., 2020). As shown in Fig. 1, the cruise crossed about 110 degrees of latitude from the western Pacific near South Korea (34.93° N) to the Ross Sea in the Southern Ocean (75.12° S). Samples were collected on transmission electron microscopy (TEM) copper grids (Carbon Type-B, Ted Pella, Inc.) for single-particle analysis by CCSEM/EDX and silicon wafer chips (Silson, Ltd.) with a hydrophobic coating (Si3N4) for ice nucleation experiments following our previous studies (Wang et al., 2012a, 2016a; Knopf et al., 2014). Particles were collected simultaneously on these two substrates, which were placed side by side in the same impactor. Particle samples were stored at room temperature in an airtight container with desiccant until analysis. This study primarily focuses on DIN at low temperatures and INP identification. Particles with a smaller size range may have a longer lifetime and can potentially be transported to higher altitudes in the atmosphere. Therefore, we limited our analysis to the samples collected on the fourth stage. Meteorological conditions and black carbon concentrations were measured by the onboard weather station and an aethalometer (AE22, Magee Scientific Co., USA), respectively. When the relative wind direction against the ship heading is between 110 and 260° and the relative wind speed is below 2 m s−1, samples may have been influenced by the ship exhaust (Park et al., 2020). Potentially contaminated samples were excluded from the analysis, resulting in a total of 29 selected samples. The sampling location and 72 h backward air mass trajectories are shown in Fig. 1. Backward air mass trajectories were computed using the Hybrid Single-Particle Lagrangian Integrated Trajectory (HYSPLIT) model (Stein et al., 2015; Rolph et al., 2017). Detailed sampling information is listed in Table S1 and includes the collecting time (Sect. S1), location, and the corresponding meteorological data. Black carbon concentration, air temperature, relative humidity, pressure, relative wind speed, and wind direction are also shown in Fig. S1 in the Supplement.
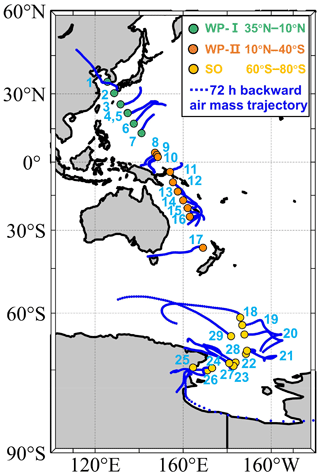
Figure 1Sampling locations with HYSPLIT 72 h backward air mass trajectories. Solid circles indicate the sample locations. Samples are labeled by numbers. Green, orange, and yellow circles represent the samples in 35–10° N of the western Pacific (WP-I), 10° N–40° S of the western Pacific (WP-II), and 60–80° S of the Southern Ocean (SO), respectively. Blue lines show the backward trajectories starting from 100 m above sea level.
2.2 Chemical imaging and characterization of particles and INPs
Chemical imaging and single-particle analysis were used to obtain information on the morphology, size, and elemental composition of the particle population and INPs. The methods have been described in detail in previous work (Laskin et al., 2002, 2006, 2012; Wang et al., 2012a; Knopf et al., 2014; O'Brien et al., 2015) and are briefly introduced here. Samples collected on the TEM grids were analyzed using a scanning electron microscope (Quanta 650, FEI Inc.) equipped with an energy dispersive X-ray spectroscopy (Genesis, EDAX Inc.) in the computer-controlled mode (CCSEM/EDX). CCSEM/EDX operating at 20 kV first detected the particles and determined their size. The particle size reported here is the equivalent circle diameter based on the two-dimensional projected area of the particle as determined by CCSEM/EDX analysis. Particles with a size (ECD) between 0.2 and 3 µm were included for analysis in this study. The elemental composition of each particle was then quantified by determining the relative atomic percentages of the selected elements, including C, N, O, Na, Mg, Al, Si, P, S, Cl, K, Ca, Mn, and Fe. We obtained the elemental composition for a significant number of particles, about 630–1480 particles for each sample depending on the particle loading on the substrates (Table S1). We manually performed SEM/EDX analysis to characterize the individual identified INPs on the silicon wafer chips after ice nucleation experiments, as described later. In addition, about 10 particles that did not nucleate ice (non-INPs) were randomly selected around each INP for SEM/EDX analysis. X-ray spectra for the INPs and non-INPs were collected at 10 kV. The relative atomic percentages of elements including C, O, Na, Mg, Al, P, S, Cl, K, and Ca were quantified. N and Si were not included in the quantification due to their presence in the background substrate.
The relative atomic percentage data were analyzed using a rule-based classification method to assess the contributions of different particle types for each sample (Laskin et al., 2012; Wang et al., 2012a; China et al., 2018; Lata et al., 2021). As shown in Fig. 2, the classification scheme grouped particles into seven classes. The first being (i) “CNO” particles, which mainly contain C, N, and O elements with traces of other elements. These are carbonaceous particles such as black carbon, secondary or primary organic particles. The second class consists of (ii) “CNOS” particles, which mainly contain C, N, O, and S elements with traces of other elements and are sulfates and other sulfur containing particles including their internal mixtures with organics. The third class consists of (iii) “FreshSS” particles. These are fresh sea salt particles containing Na above a threshold level of 2 % (atomic percentage) and with the ratio > 0.8. At the same time, the total atomic percentage of Na, Mg, and Ca, which are the dominant cations in sea salt particles, is higher than that of other metals. CCSEM/EDX analysis of fresh sea salt particles generated by nebulizing seawater shows that the ratio is slightly higher than 0.8 for particles at about 0.2 µm (Fig. S2). The samples we investigated contain a large number of small sea salt particles. Thus, we use a ratio of 0.8 as the threshold value to distinguish between the fresh and aged sea salt particles. The fourth class is (iv) “AgedSS” particles. These are aged sea salt particles with Cl depletion and the ratio < 0.8. The fifth class is (v) “SS/Sulf” particles. These are aged sea salt particles mixed with sulfur-containing compounds (e.g., sulfate) containing mainly Na and S without Cl. The six class is (vi) “Dust” particles. These particles have the total atomic percentage of Al and Si above 4 % and higher than the total atomic percentage of Na and Mg. They are likely from dust storms and road or soil emissions. The final class consists of (vii) “Mixture” particles, which are all remaining particles that did not fit into the previous categories. The classification scheme for INPs and non-INPs on silicon wafer chips was modified to account for the Si and N background in the substrate. It is important to note that particles on either TEM grids or Si chips were collected at the same time using the same impactor, and therefore the contributions of the respective particle classes for the two are the same. Thus, we modified the classification scheme with this assumption.
2.3 Chemical mixing state
The chemical mixing state was derived using a previous methodology based on mass and entropy metrics (O'Brien et al., 2015; Riemer and West, 2013) and is described briefly here. Particle mass was estimated from the density and volume of each particle. Particles were assumed to be hemispherical, and the volume of each particle was calculated from the ECD obtained by CCSEM. Particle density was assigned according to its classification, with FreshSS, AgedSS, SS/Sulf, CNO, CNOS, Dust, and Mixture particles having a density of 2.0, 2.0, 1.7, 1.3, 1.3, 2.7, and 2.0 g cm−3, respectively (O'Brien et al., 2015; Tang et al., 2014). To calculate the mass of each element in a particle, the atomic percentage of each element obtained by EDX was converted to a weight percentage and then multiplied by the mass of the particle. For the particle i, the mass of element a is equal to
where a=1,..., A (number of elements), i=1,..., N (number of particles), and μi is the total mass of the ith particle. Here, a represents elements of Na, Mg, Al, Si, P, S, Cl, K, Ca, Mn, and Fe, while for this list A=11. Note that C, N, and O are not included, as these three elements are semi-quantitative when EDX analysis is used (Laskin et al., 2006).
First, the total mass of element a in the particle population (μa) and the total mass of the bulk particle population (μ) were calculated using the following equations:
and
Following this, the mass fraction of element a in the ith particle (, the mass fraction of individual particles (pi) in the particle population, and the mass fraction of element ain the bulk particle population (pa) are
and
Next, the following Shannon entropies (MacKay, 2003) were calculated, where the mixing entropy of the ith particle (Hi) is
the average particle mixing entropy (Ha) is
and the mass entropy of bulk particle population (Hγ) is
Hi and Hγ are used to describe the mass distribution of species (elements) with the ith particle or particle population, respectively. A higher entropy indicates a more uniform distribution of elements in the individual particle or particle population, whereas a lower entropy indicates a trend towards a non-uniform mass distribution.
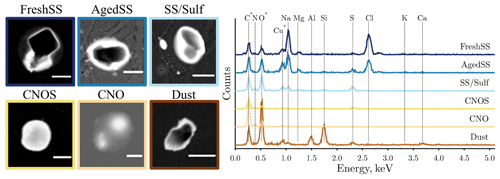
Figure 3Representative SEM images and the corresponding EDX spectra for particles from each class. The peaks in spectra for the C, O, and Cu elements (asterisked) may include some signal from substrate background of TEM grids. SEM images were captured at 20 kV using scanning transmission electron microscopy detector. The scale bar for all images is 1 µm.
Individual particle diversity (Di) is calculated by taking the exponent of Hi. Di means the effective number of elements in individual particles or in other words indicates the distribution of elements in single particles. Di ranges from the minimum value of 1 when the particle contains a single element to the maximum value of A when the particle is composed of all A elements with equal mass. Particle elemental diversity (Dα) and bulk population elemental diversity (Dγ) are calculated by taking the exponent of Ha and Hγ, respectively. Dα indicates the average effective number of elements in particles. Dγ indicates the effective number of elements in the whole particle sample.
The mixing-state index (χ) indicates the homogeneity or heterogeneity of the population and is defined as
χ ranges from 0 % for an externally mixed particle sample with a heterogeneous population composing single-component particles (Dα=1) to 100 % for an internally well-mixed particle sample with a homogeneous population where all particles have identical compositions.
2.4 Ice nucleation experiment and INP identification
Ice nucleation and water uptake by particles were examined following our previous studies (Knopf et al., 2011, 2014, 2022; Wang and Knopf, 2011; Wang et al., 2012b; Charnawskas et al., 2017; China et al., 2017; Alpert et al., 2022) and the methods are briefly introduced here. Onset conditions of the particle temperature (Tp) and relative humidity with respect to ice (RHice), when ice nucleation and water uptake occurred, were determined using a custom-built cryo-cooling system. The system consists of a water vapor control component, an ice nucleation cell (INC), and an optical microscope (OM). Prior to ice nucleation experiments, a particle sample collected on a silicon wafer was placed in the INC. Following this, a humidified N2 gas with targeted water vapor partial pressure was continuously introduced into INC at a flow rate of 1 SLPM (standard liter per minute). The water partial pressure in the INC was determined by the dew point temperature (Td) of the gas, which was measured using a chilled mirror hygrometer (GE Sensing, Optica). After Td was stable, Tp was set to about Td+3 K and RHice<100 %. An ice nucleation experiment was started, and Tp was cooled at a rate of 0.2 K min−1. Images of particle sample were recorded by OM every 0.02 K. Td and Tp were recorded every second throughout the experiment. Once ice formation was observed, the sample was gradually warmed to 298 K to sublime and remove any ice that may be retained in the pores or cavities of particles. Images of ice crystals were acquired during sublimation at high magnification, and the visible particle that served as the INP was identified after complete crystal sublimation. The experiment was repeated three to seven times at similar Td for reproducibility. Water uptake and ice formation by particles were determined through visual observation and the changes in particle phase or size obtained by analyzing the recorded images using ImageJ software. DIN and IMF were discriminated based on whether particles took up water before ice formation. The freezing mechanism within the small droplets after the water uptake could not be visualized due to the limited spatial resolution and imaging speed of the OM used in this study. Thus, we assume that IMF has occurred if the RHice onset of freezing is lower than the homogeneous freezing limits. Only the temperature and RHice conditions when the first ice crystal formed were reported. RHice was derived from the measured Td and Tp (Wang and Knopf, 2011). Particle surface area available for ice nucleation for each sample was estimated from the particle number and size derived from OM images, assuming that the particles were hemispheres. The conservative uncertainty of a factor of 2 for particle surface area was estimated using the standard deviation of the means with assumption of particles being flat or spherical. This assumption likely underestimates the actual surface area since most of the particles deposited on the substrate exhibited non-spherical geometry and may have had rough surfaces including cracks or cavities. The particle surface areas were later used for ice nucleation kinetic analysis. INPs were identified using the recorded optical images during ice nucleation, ice growth, and ice sublimation. Using these optical images, INPs were relocated in the SEM using digital pattern recognition and triangulation and then were imaged and analyzed by SEM/EDX (Knopf et al., 2014).
The temperature and water vapor distribution within the INC were calibrated and validated following our previous work (Wang and Knopf, 2011; Wang et al., 2016b). Homogeneous water vapor distribution in the INC was confirmed by uniform condensation and evaporation of micrometer-sized water droplets across the 0.5 mm2 sample area. Tp was calibrated by the melting points of ice, dodecane, decane, octane, and heptane. The calibration confirmed that the response of Tp is linear in the range of 180–273 K with an uncertainty less than 0.3 K. Conservative uncertainty in RHice for this experimental system comes from the uncertainty in Td and Tp ( K and K), resulting in ΔRH % at 200 K and % at 260 K.
3.1 Particle characterization
Figure 3 shows the typical SEM images and the corresponding EDX spectra of the identified particle classes. FreshSS and AgedSS particles were dominated by Na with different contents of Cl. The FreshSS particle exhibited a cubic NaCl crystal morphology (solid black square) with irregular materials (bright coating) under the transmission detector of SEM at dark field mode. The coating likely comprised other materials in seawater including MgSO4 and CaSO4 (Xiao et al., 2008). The AgedSS particle showed a non-cubic-shaped NaCl crystal shape as its core is surrounded by substances containing Na with depletion of Cl. The depletion of Cl indicates that the particle had been aged, possibly due to the formation of gaseous HCl by chemical reactions with nitric acid, sulfuric acid, and organic acids in the atmosphere (Laskin et al., 2012; Wang et al., 2015; Angle et al., 2021; Su et al., 2022). The SS/Sulf particle shown in Fig. 3 had a core shell structure and was mainly composed of Na and S without Cl. This suggests that it was a completely aged sea salt particle coated with sulfur-containing components, such as sulfate. The CNOS and CNO particles showed a round-shaped morphology. The Dust particle exhibited clear Al and Si peaks with minor Ca. Figure 4 shows the particle classification results of over 30 000 particles in 29 samples investigated by CCSEM/EDX. The size-resolved chemical distributions for all samples are shown in Fig. S3, with FreshSS and AgedSS particles dominating all size bins. The majority of SS/Sulf particles were larger than 0.5 µm, while the CNOS and CNO particles were mainly in the sub-micrometer size range. As shown in Figs. 1 and 4, the samples (S1–S29) were separated into the following three regions according to the latitudes of the sampling locations, 35–10° N of the western Pacific (WP-I), 10° N–40° S of the western Pacific (WP-II), and 60–80° S of the Southern Ocean (SO).
Figure 4 shows that in the WP-I region (35–10° N, S1–S7), the proportion of FreshSS and AgedSS particles increased from 10 % to 91 % as the ship moved away from the land, indicating an increased contribution of SSA. The contribution of the Dust particle class decreased from 59 % to 8 % for S1 to S3. The Navy Aerosol Analysis and Prediction System reanalysis (NAAPS-RA) product indicated a dust storm during the S1 and S2 sampling periods (Fig. S4). The 72 h backward air mass trajectories also showed that the air mass passed through areas affected by the dust storm.
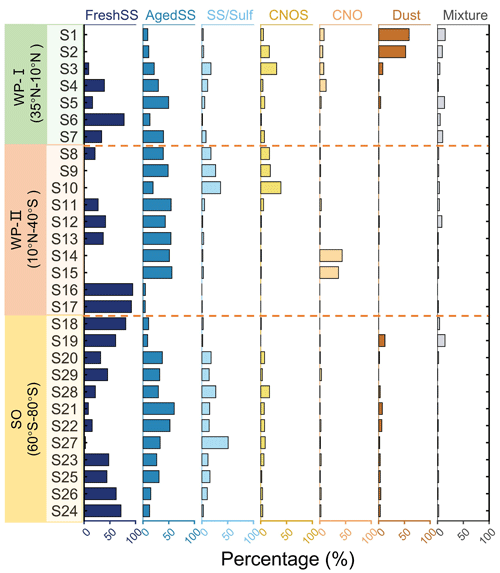
Figure 4Relative percentages of the seven particle classes (FreshSS, AgedSS, SS/Sulf, CNOS, CNO, Dust, and Mixture) for each sample sorted by the latitudes of sampling locations and separated into three regions (see Fig. 1).
In the WP-II region (10° N–40° S, S8–S17), the backward trajectories (Fig. 1) display that the air mass of most samples resided over the ocean. However, the samples demonstrated large variations in the particle composition. S8–S10 were dominated by AgedSS, SS/Sulf, and CNOS particles. The percentage of SS/Sulf and CNOS particles increased from 18 % to 36 % and from 17 % to 39 %, respectively, from S8 to S10. The contributions of these two sulfur-containing particle classes increased simultaneously, implying the same sulfur source. This is consistent with the results showing high sulfate aerosol optical depth (AOD) from the NAAPS-RA products at the same period (Fig. S4D–F). The average black carbon (BC) concentration was 107.4±70.6 ng m−3 for S8 (Table S1). It likely originated from combustion emissions transported from land. As shown in Fig. S4C, this is further supported by the air mass for S8 originated around the region where fire spots were detected in NASA Fire Information for Resource Management System (FIRMS). S14 and S15 were dominated only by the AgedSS and CNO particles, with the latter accounting for 43 % and 36 %, respectively. These higher fractions of CNO particles compared to other samples (Fig. 4) are likely related to BBA. As shown in Fig. S1, the average BC concentrations of S14 and S15 were as high as 674 and 356 ng m−3, respectively. Backward trajectories showed that the air mass passed through the high smoke AOD regions (Fig. S4G–H). These two samples were collected on 13 and 14 November of 2019, when large wildfires occurred in Australia during the austral summer of 2019–2020 (Hirsch and Koren, 2021). Dense fire spots along the east coast of Australia at the time of sampling are shown in Fig. S4I. Chemical imaging of elements (Fig. S5) showed that typical CNO particles from these two samples had thick organic coatings with high carbon signal inclusions and likely are aged BC. This type of aged BBA or smoke particle with organic coating has been observed in the stratosphere (Ditas et al., 2018). Similar complex organic compounds have also been observed in the tropospheric smoke aerosol (Palm et al., 2020). Other samples in the WP-II region (S11, S12, S13, S16, and S17) were dominated by the FreshSS and AgedSS particles.
In the SO region (60–80° S, sample S18–S24), the number percentages of FreshSS particles decreased first and then increased moving toward higher latitudes, whereas the AgedSS, SS/Sulf, and CNOS particles had opposite trends. These three particle types contributed to 11 % to 93 % of the total particles in the sample. As shown in Fig. S6, the contributions of AgedSS, SS/Sulf, and CNOS particles from the middle of the Ross Sea (S28, S21, S22, S27, S23) were significantly higher than those from the north (S18, S19, S20, S29) and southwest (S25, S26, S24) of the Ross Sea. The increased contribution of these three sulfur-containing particle classes may be related to the biogenic sulfur emission from polynyas (areas of open water surrounded by sea ice) in the central Ross Sea (Fig. S7) (Brean et al., 2021; Baccarini et al., 2021; McCoy et al., 2021; Jang et al., 2019; Zhang et al., 2015). The formation of polynyas during the austral summer allowed phytoplankton to grow and produce DMS, which can be transferred into the atmosphere and oxidized. The products increase the sulfur content in aerosol particles. Previous work found that the MSA concentration over the Southern Ocean from November 1994 to February 1995 was about 2 times higher than that of the western Pacific (Kunwar et al., 2023). High MSA levels were observed in the Ross Sea and were associated with the dynamic sea ice edge at ∼ 64° S in early December (Yan et al., 2020). This was caused by the increase in phytoplankton from the release of algae from the melting sea ice. This also suggests the potential impacts by the biogenic emission of DMS on these samples.
Except for the two samples impacted by the dust storm, most of the samples collected during this cruise were predominantly sea-salt-containing particles, including the FreshSS, AgedSS, and SS/Sulf classes, with total number percentages ranging from 48 % to 99 %. The varying proportions of these three particle classes indicated the contribution of marine emission, but with different degrees of aging which will be discussed in the next section. Figures S8A and S9A show the classification results for super-micrometer particles (diameter > 1 µm) and submicrometer particles (diameter between 0.2 and 1 µm), respectively. The sea-salt-containing particles including FreshSS, AgedSS, and SS/Sulf classes had higher percentages in the super-micrometer size range. The majority of CNOS particles were in the submicrometer size range. CNOS represents sulfur containing particles (e.g., sulfate) including their mixtures with organics. CNOS particles were predominantly in the submicrometer range, likely due to new particle formation of MSA and H2SO4 and their condensation onto pre-existing particles (Hopkins et al., 2008; Yan et al., 2020; Beck et al., 2021). Heterogeneous aqueous chemical reactions may also contribute to CNOS particles, including cloud processing (e.g., Ervens et al., 2018).
3.2 Mixing state of particle population
The mixing state described here is based on the elemental composition and is referred to as the chemical mixing state. Figure 5a shows the elemental mass percentages of the particle population for each sample. Most of the samples were dominated by Na, Cl, and S, except the samples affected by dust storm (S1, S2). The proportion of Na in each sample was relatively stable at about 38 %. The rest was contributed mostly by Cl and S. The aging of sea salt particles can be evaluated by the elemental ratio of Cl to Na (). As shown in Figs. 5a and S10, the ratio ranges from 0 to close to 1, indicating complete Cl depletion and no aging on sea salt particles, respectively. The samples with more S have less Cl. This is consistent with the increase in the proportion of sea salt with different degrees of aging (AgedSS and SS/Sulf). The relationship between S and Cl indicates that the chlorine loss in particles over ocean is attributed to the acid displacement by H2SO4 and MSA. The samples affected by the dust storm were dominated by the elements of Si, Al, and Fe, all of which are common in mineral dust with traces of Ca and K. As the elements of C, N, and O were not considered in the mass percentage calculation, the CNO particles prominent in S14 and S15 are not reflected in the mass percentages (Fig. 5a). The elements of K, Si, and Fe presented in the same samples are related to biomass burning (Chen et al., 2017). It is consistent with the air mass from which they were sampled being impacted by biomass burning plumes.
The mixing-state parameters, namely Dα, Dγ, and χ for each sample, are presented in Fig. 5b. The range of Dα and Dγ was from 2.4 to 4.9 and 2.7 to 6.4, respectively. χ ranged from 50 % to 95 %. In the WP-I region, the Dust-dominated S1 had the highest Dα of 4.9 and Dγ of 6.4. As the contribution of the Dust particles decreased from 59 % to 1 % for S1 to S4, Dα and Dγ decreased from 4.9 to 3.2 and 6.4 to 4.3, respectively. χ first decreased from 72 % to 50 % (S1 to S3) and then increased to 68 % (S4) as the dominant particle type switched from Dust to sea-salt-containing particles. χ further increased to 86 % (S6) when FreshSS particles dominated. In the WP-II region, Dα and Dγ decreased as CNOS and SS/Sulf particles increased and the mass percentage of S increased from S8 to S10, corresponding to a slight increase in χ from 72 % to 82 %. When comparing the wildfire-influenced samples (S14, S15) and the FreshSS-dominated samples (S16, S17), the Dα values are very similar, whereas Dγ values are quite different (3.8 and 4.8 vs. 2.8 and 2.9) resulting in contrasting χ values. The highest χ of about 95 % for S16 and S17 indicated that these two samples dominated by Na and Cl were largely internally mixed. This can be expected since they were dominated by FreshSS particles from the single marine source. AgedSS particles from marine emission with additional atmospheric processing and CNO particles from biomass burning had similar contributions to S14 and S15. This was reflected in χ of about 58 % for S14 and S15. The main elements of Na and S in these two samples are more externally mixed. In the SO region, Dα and Dγ were similar to each other, resulting in relatively stable χ values. The changes in Dα and Dγ are mainly due to the changes in the contribution of sulfur-containing particles. Figure S8 and Fig. S9 show the elemental mass percentages, Dα, Dγ, and χ for super-micrometer and submicrometer particles, respectively. For submicrometer particles of each sample, Dα and Dγ are 1.9–3.9 and 2.4–7.1, respectively. χ ranges from about 38 % to 95 %. For super-micrometer particles of each sample, Dα and Dγ are 2.5–5.1 and 2.7–6.6, respectively. χ ranges from about 54 %–96 %. In general, Dα and χ for the submicrometer particles are lower than super-micrometer particles in most samples, whereas Dγ is similar. This suggests that the main elements of Na, S, and Cl and the minor elements of Mg, K, Fe, Ca, and Si in super-micrometer particles tend to be more internally mixed than in submicrometer particles (Figs. S8B and S9B).
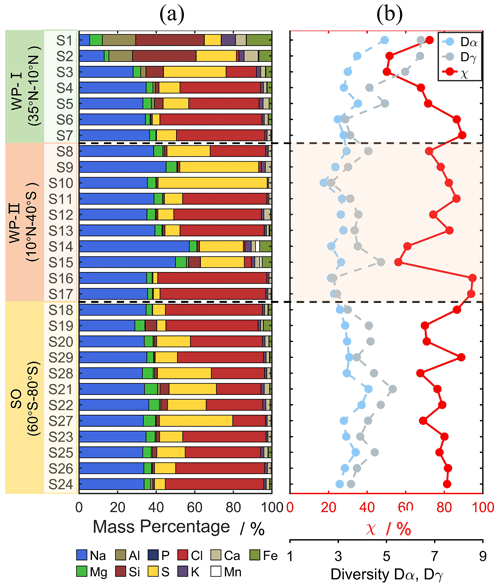
Figure 5Relative mass percentages of elements (a) and mixing state (b) of all samples. Color codes for the elements are shown at bottom. Light blue, gray, and red circles represent the particle elemental diversity (Dα), population elemental diversity (Dγ), and mixing-state index (χ), respectively.
To focus on the effect of particle aging on the mixing state of marine aerosols, we excluded four samples from two events from the analysis, i.e., the Dust-dominated samples from a dust storm (S1 and S2) and the BBA-dominated samples from wildfire emissions (S14 and S15). Figure 6 shows the relationship between χ and the percentages of FreshSS, AgedSS and SS/Sulf, and CNOS particles in different size ranges. To minimize the potential influence of extreme data points, the linear regression was performed using the binned data based on the number percentage of particle type. We found a strong correlation between the percentages of FreshSS particles in the samples and their χ (Fig. 6a, R2=0.66, p=0.008), especially for submicrometer particles (R2=0.85). χ increased as the contribution of FreshSS particles increased. There is a negative correlation between χ and the total percentage of AgedSS and SS/Sulf particles (Fig. 6b, R2=0.46, p<0.05). We also found a strong negative correlation between χand the contribution of CNOS particles (Fig.6c, R2=0.86, p=0.002). The results of the above-mentioned relationship also hold for both submicrometer and super-micrometer size range particles. The positive correlation between the contribution of FreshSS particles and χ indicates that the particle population became more internally mixed as more fresh SSA were added to the air parcel. When the FreshSS particles became the dominant type, the particle population was close to the complete internal mixing (χ=100 %), such as S16 and S17 as discussed above. Adding CNOS particles (a new particle class) decreases the χ and makes the population more externally mixed. These results verify the schematic descriptions on the evolution of the mixing state described by Riemer et al. (2019) after adding new particles or particles of a dominant type.
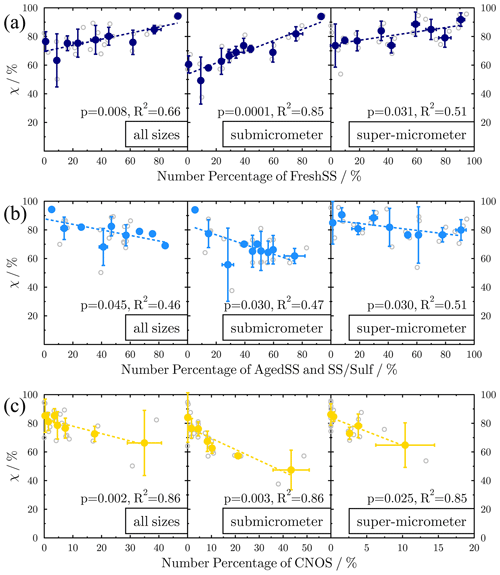
Figure 6Variation of mixing state index (χ) with the number percentages of different particle classes: (a) FreshSS, (b) AgedSS and SS/Sulf, and (c) CNOS. Panels, from left to right, are for particles with different size ranges: all particle size (all sizes), 0.2–1 µm (submicrometer), and larger than 1 µm in diameter (super-micrometer). Original and binned data are shown as open circles and solid color circles, respectively. Linear regression (dashed line) is based on the binned data.
Atmospheric aging processes can change the composition and mixing state of aerosol population. In marine environments, the degree of aging on sea salt particles can be reflected by the contribution of AgedSS and SS/Sulf particles in the population. The negative correlation between the number percentages of AgedSS and SS/Sulf particles and χ indicates that aging has resulted in a more externally mixed particle population. This seems to contradict the view that aging tends to drive a particle population towards a more internally mixed state (Riemer et al., 2019). If an aging process (e.g., condensation of secondary organics) occurs uniformly on each particle and further aging makes them more homogeneous, the population tends to be more internally mixed. On the other hand, if the aging process occurs only on a specific type of particle, such as acid displacement turning fresh sea salt into aged sea salt, this will increase particle diversity, and the population may move to a more externally mixed state. This may be particularly true as fresh sea salt is continuously released even as aged sea salt particles form. The availability of acids and the size distribution of fresh and aged sea salt particles at different locations could result in particles with different chlorine deficiencies and greater diversity. In this case, aging will increase the inhomogeneity of particles and move the population toward a more externally mixed state.
3.3 Ice nucleation properties at cirrus conditions
We conducted ice nucleation experiments on six samples dominated by different particle classes. These samples are separated into four groups: (i) Dust-dominated samples (S1), where 59 % of the particles are dust-like; (ii) BBA-influenced samples (S14) in which CNO and AgedSS particles contributed 43 % and 51 %, respectively; (iii) CNOS- and SS/Sulf-dominated samples (S10) with percentages of 39 % and 36 %, respectively; and (iv) FreshSS- and AgedSS-dominated samples (S4, S11, and S12), with the total number percentage of these two particle types being greater than 70 %. Table 1 shows the dominant particle class, total particle surface area, number of particles available for ice nucleation during the experiments, IN-activated fraction at ice nucleation onsets, average ECD of INPs and non-INPs, and average ECD of INPs.
Table 1Information of particle samples, including the total particle surface area and number of particles, IN-activated fraction at ice nucleation onset conditions, the average ECD of INPs and non-INPs, and the average ECD of INPs.
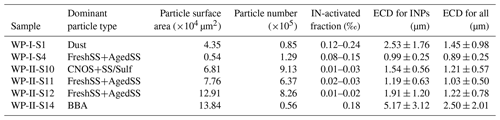
Figure 7 shows the onset conditions of Tp and RHice for water uptake and ice nucleation on the representative samples. Below 235 K, only DIN was observed for the Dust and BBA samples (Fig. 7a). Particles on the Dust sample nucleated ice heterogeneously at RHice from 130 % ± 9 % to 153 % ± 8 % at Tp from 235 to 209 K. These values are below the homogeneous nucleation limits of aqueous droplets (Koop et al., 2000; Koop and Zobrist, 2009). RHice onsets of the BBA sample ranged from 145 % ± 5 % to 169 % ± 1 % at Tp from 229 to 209 K. The RHice onsets were only about 3 % below the homogeneous nucleation limits between 220 and 228 K, and thus the sample dominated by BBA may not have been efficient heterogeneous ice nuclei. As Tp decreased, the RHice onset of both the BBA and Dust samples gradually increased. Particles on the Dust sample initiated DIN at RHice lower than the BBA sample by 7 % to 18 % at each Tp. For the CNOS- and SS/Sulf-dominated sample (Fig. 7a), particles first took up water when RHw reached about 83 % and then froze via IMF or homogeneous nucleation with RHice around the homogeneous line at the temperature close to 230 K. Particles formed ice via DIN below 225 K at RHice from 136 % ± 4 % to 159 % ± 11 %. Figure 7b shows that the ice nucleated on particles from the FreshSS- and AgedSS-dominated samples via DIN and IMF pathways. The transition temperature between DIN and IMF pathways was about 225 K. At about 225 K, particles on S4 and S12 both took up water at 78 % ± 2 % and then formed ice upon further cooling. The subsequent ice nucleation of S12 could be homogeneous nucleation or IMF since the onset RHice of 150 % ± 7 % is close to the homogeneous nucleation limit. However, particles of S4 nucleated ice after water uptake through IMF at RHice of 140 % ± 8 %, which is about 8 % lower than the homogeneous nucleation limit. Below 225 K, particles of S4 and S12 nucleated ice via DIN at RHice from 133 % ± 4 % to 170 % ± 8 %. DIN occurred on the S11 particles at about 121 % ± 3 % to 130 % ± 3 % RHice below 225 K. S11 showed the lowest onset RHice among the investigated samples indicating that its ice nucleation efficiency was higher than other samples. The results of ice nucleation onset conditions for the investigated samples showed distinct variations in the ice nucleation ability for particles in the different marine atmospheres, even for the samples (S11, S12) with similar elemental composition and the dominant particle classes. The possible reasons for this are discussed in the later section.
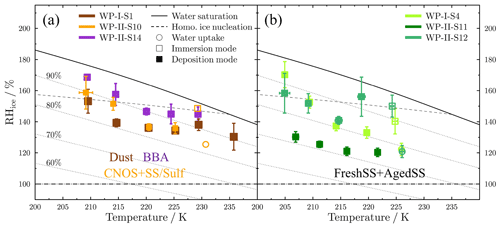
Figure 7Onset conditions of freezing temperature and RHice for water uptake (open circles), immersion freezing (open squares), and deposition ice nucleation (solid squares) on particle samples. (a) Dust-dominated samples (S1), BBA-influenced samples (S14), and CNOS- and SS/Sulf-dominated samples (S10). (b) FreshSS- and AgedSS-dominated samples (S4, S11, S12). The dashed line indicates the homogeneous freezing limits for aqueous droplets of 0.3 µm in diameter (Koop et al., 2000; Koop and Zobrist, 2009). Dotted lines represent different relative humidity (RHw) shown in panel (a). Solid and dashed–dotted lines represent water saturation (100 % RHw) and ice saturation (100 % RHice), respectively.
3.4 INP characterization
In total, 132 INPs together with 1317 non-INPs on the silicon wafer substrates were identified and characterized individually for their morphology and elemental composition. Figure 8 shows the representative SEM images and the corresponding EDX spectra of different types of INPs. SEM images for each identified INP at different temperatures are shown in Figs. S11, S12, and S13. The average ECD of INPs was generally greater than the ECD of the population considering both INPs and non-INPs together for each sample (Table 1). Of the 132 INPs, 71 % of them were super-micrometer particles, and the rest were submicrometer particles. The INPs exhibited different morphologies, such as crystalline irregular shapes (e.g., INPs classified as FreshSS, Aged SS, and Dust) and spherical shapes (e.g., INPs classified as CNOS). We found that the majority of INPs from S14 influenced by BBA had thick organic coatings (Figs. 8 and S11). The organic coating thickness of BBA INPs (1.07±0.68 µm, n=13) is significantly thicker than that of other BBA (0.53±0.37 µm, n=112) at the significant level of 0.001. Different number fractions of INPs identified in the FreshSS- and AgedSS-dominated samples (S4, S11, and S12) have organic coatings. More than 80 % of INPs in S11 were coated with organics, which is a greater percentage compared to S4 (33 %) and S12 (30 %). Figure S14 shows the elemental mapping of a representative INP with a thin organic coating. The mapping shows that Na and Cl were distributed in the core, while C and O were present throughout the whole 2-D projected area of this AgedSS INP. Previous studies have shown that solid organics can form ice via DIN at low temperatures (Wang et al., 2012b; Knopf et al., 2018; Lata et al., 2021; Alpert et al., 2022). We speculate that the organic coatings on the particles of S11 triggered the ice nucleation and have different ice nucleation abilities compared to S4 and S12.
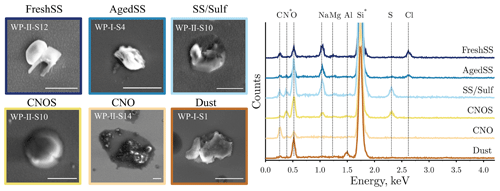
Figure 8Representative SEM images and the corresponding EDX spectra for six classes of INPs. The spectra include the substrate background signal of N and Si (asterisked) from a silicon wafer coated with Si3N4. Labels on SEM images are the name of samples. The scale bar is 2 µm for all images.
All the identified INPs and non-INPs on the substrates used in the ice nucleation experiments were categorized based on the SEM/EDX data. Figure 9 shows the percentages of different classes for INPs and the particle population in stacked bars marked with “INPs” and “All”, respectively. Taking S1 as an example, six particle classes were identified in the particle population, and the 19 identified INPs are from five particle classes, except for the CNO class. Similar to S1, the dominant particle classes in the population of all respective samples are also the dominant classes of INPs. This result suggests that all these major particle classes identified in our experiments are potential INPs. We calculated the average elemental composition of INPs and non-INPs for each particle type as shown in Fig. S15. A two-sample t test was also performed on the elemental compositions of INPs and non-INPs for each particle type. At the significance level of 0.05, there is not sufficient evidence to show that the elemental compositions of INPs and non-INPs are different. We also found that the contributions of the same particle class in INPs and the particle population are different in some cases. The most notable example is S10, where SS/Sulf particles contributed 77 % of INPs but only 51 % of the population.
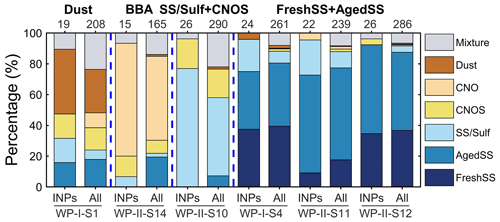
Figure 9The number percentages of different particle classes for INPs and the particle population. Data marked with “All” include both INPs and non-INPs. The corresponding numbers on the top represent the investigated particle number. Dashed blue lines separate the samples with different particle classes.
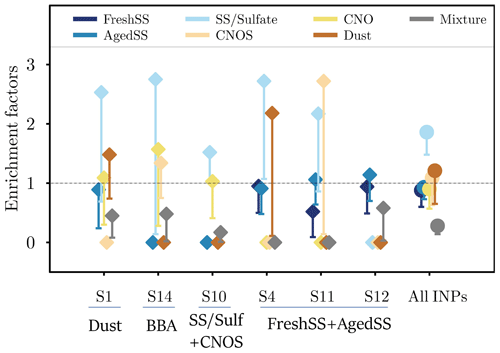
Figure 10The EF of all INP classes for each sample (diamonds) and all INPs (circles). The dashed line indicates EF of 1. The lower limits of EF were calculated by Poisson distribution at the confidence level of 95 %. For readability, the upper limits were not plotted here but the values are listed in Table S2.
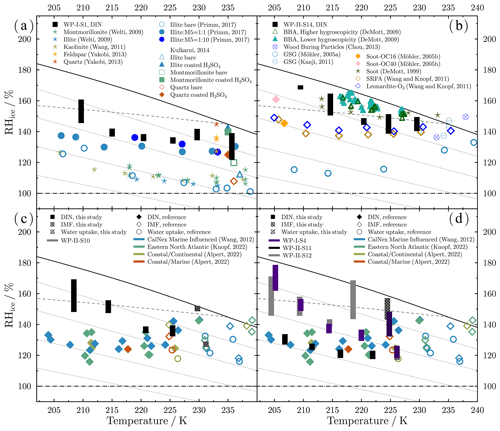
Figure 11Ice nucleation and water uptake onset conditions (bars) for (a) Dust-dominated samples (S1), (b) BBA-influenced samples (S14), (c) CNOS- and SS/Sulf-dominated samples (S10), and (d) FreshSS- and AgedSS-dominated samples (S4, S11, S12) compared with previous studies (symbols) (DeMott et al., 1999, 2009; Möhler et al., 2005a, b; Welti et al., 2009; Kanji et al., 2011; Wang and Knopf, 2011; Wang et al., 2012a; Yakobi-Hancock et al., 2013; Kulkarni et al., 2014; Primm et al., 2017; Alpert et al., 2022; Knopf et al., 2022). Descriptions for the lines are the same as in Fig. 7.
To further explore whether a particle class is unique when acting as INPs, we calculated the enrichment factor (EF) for a given particle class (A) according to the following formula:
where N is the number of particles. EF(A) is the ratio of the contributions of the particle class A in the INPs to the whole particle population (INPs + non-INPs). EF values of each particle class for different samples are shown in Fig. 10. EF values with upper and lower limits determined from Poisson distributed errors at 95 % confidence level are listed in Table S2. Although the enriched particle classes are diverse for different samples, EF of the SS/Sulf class is greater than 1 for most of the samples except S12. No INP was identified as SS/Sulf particle for S12, which only has 4 % of SS/Sulf in the population. The overall EF of the SS/Sulf class is 1.9 with upper and lower limits of 9.2 and 1.2, respectively. This indicates that the SS/Sulf particles were enriched in INPs in these marine environments.
3.5 Comparison of ice nucleation properties with previous studies
Figure 11 shows the comparison of ice nucleation data from our work and previous studies. The DIN onset conditions of the Dust-dominated sample (S1) are similar to the feldspar between 230 and 235 K (Yakobi-Hancock et al., 2013) and less efficient compared to bare dust particles, including montmorillonite (Welti et al., 2009; Kulkarni et al., 2014), illite (Welti et al., 2009; Kulkarni et al., 2014), quartz (Kulkarni et al., 2014), and kaolinite (Wang and Knopf, 2011). Previous laboratory studies showed that the aging processes can reduce the ice nucleation ability of mineral dust at temperatures relevant to cirrus clouds (Tang et al., 2016). For example, Primm et al. (2017) indicated that the ice nucleation ability of illite can be slightly suppressed by organic acids when mixed with a mixture of five dicarboxylic acids (referred to as M5) at an organic-illite mass ratio greater than 1:1. The DIN onset conditions of S1 are similar to the M5/illite (10:1) mixtures (Fig. 11a). We also found that coated particles (Fig. S16) in S1 serving as INPs have a similar coating thickness (Fig. S11) as organic coatings on M5/illite greater than 1:1. Kulkarni et al. (2014) observed a decrease in ice nucleation ability of montmorillonite, illite, and quartz at 235 K when coated with H2SO4. These H2SO4-coated dust particles had a similar onset RHice as compared to particles on S1 at 235 K. During the cruise, S1 and S2 in the WP-I region were impacted by the dust storm originating from western Asia. The dust particles were aged during the transport to the coastal areas and some of them had clear coatings (Figs. S11 and S16). This is consistent with the finding by Jang et al. (2023) from the same cruise that aerosol particles in the S1 sample area have a high fraction of lignin-like organics. S1 had a similar ice nucleation ability as dust particles with organic coatings. We suspect that the ice nucleation efficiency of the dust particles at temperatures where DIN occurred on the INP's surface was affected by coatings after aging.
Our results for S14 suggest that BBA from the Australian wildfires may have served as INPs via DIN below 230 K. Combustion and burning processes produce a large number of particles with mixed organic and inorganic components into the atmosphere (e.g., Fuzzi et al., 2007; Chen et al., 2017; Hodshire et al., 2019). Previous studies have shown that soot, combustion ash, and BBA particles from different burning sources demonstrated various ice nucleation properties (e.g., Jahl et al., 2021; Jahn et al., 2020; Kanji et al., 2017 and references therein). Soot particles or their organic coatings on S14 may have played a role in the observed ice nucleation events. Several studies have reported that combustion-related particles can serve as INPs via DIN below 233 K (DeMott et al., 1999; Möhler et al., 2005a, b; Koehler et al., 2009; Kanji et al., 2011; Chou et al., 2013). Kanji et al. (2011) showed the ice nucleation activated by soot generated using a graphite spark generator (GSG) at about 145 % RHice, a value similar to S14 at 230 K. Chou et al. (2013) showed that wood burning particles nucleated ice at about 136 % RHice at about 233 K. DeMott et al. (1999) reported that the freezing RHice of soot was close to the homogeneous freezing limits. S14 nucleated ice at higher RHice than soot generated by GSG and particles containing organic carbon (Möhler et al., 2005a, b) but lower than the soot studied by DeMott et al. (1999). Suwannee River standard fulvic acid (SRFA) and leonardite were used as surrogates of atmospheric organics from biomass burning (Wang and Knopf, 2011). Ice nucleation onset conditions of S14 are very similar to those of SRFA and ozone aged leonardite particles above 220 K but higher at lower temperatures.
Figures 11c and d show the comparison of data on marine-related particles from previous studies with our data on sea-salt-containing particles. Several studies have shown that the aerosol particles collected in marine environments or coastal areas can act as efficient INPs at RHice between 115 % and 143 % (e.g., Wang et al., 2012a; Alpert et al., 2022; Knopf et al., 2022). Using the micro-spectroscopic analysis, these studies identified the INPs and demonstrated that the major particle types in the population initiate ice formation. These particle types include the marine-influenced particles with thin organic coatings collected during the CalNex field campaign (Wang et al., 2012a), the relatively fresh sea salt particles with marine-produced organics collected from the coast of Long Island, New York (Alpert et al., 2022), and the processed sea-salt-containing particles collected over the eastern North Atlantic (Knopf et al., 2022). There is one FreshSS- and AgedSS-dominated sample (S11) that nucleated ice at the same RHice onsets as compared to those marine-influenced particles from above-mentioned studies. The other sea-salt-containing samples in this work including the CNOS- and SS/Sulf-dominated sample (S10) and the FreshSS- and AgedSS-dominated samples (S4, S12) showed very similar RHice onsets only at temperatures above 220 K but higher onsets below 220 K. The reason for this discrepancy at lower temperatures is not clear. Future work on the coating composition may provide more insight. Together with previous studies, we show that these marine-influenced particles exhibit different ice nucleation efficiencies. These variations likely contributed not only to the complex compositions but also to the physical and chemical mixing state of these particles.
3.6 Ice nucleation kinetics
Ice nucleation kinetic analysis was conducted using the experimental data, including the RHice and temperature onsets, particle surface area, number of nucleation events, and nucleation time. Classical nucleation theory (CNT) has been widely used in cloud models (e.g., Pruppacher and Klett, 2010; Khvorostyanov and Curry, 2004; Liu and Penner, 2005). In this study, heterogeneous ice nucleation rate coefficient (Jhet), contact angle (θ), and their parameterizations are presented and discussed. The ice-nucleation-activated fraction of each sample is listed in Table 1 and discussed in Sect. S2. ns is based on the singular hypothesis (Vali, 1971; Connolly et al., 2009), and its parameterizations are also presented and discussed in Sect. S2, Figs. S17, and S18.
3.6.1 Heterogeneous ice nucleation rate coefficient (Jhet)
According to CNT, ice nucleation is a stochastic process and continues with time, in contrast to the singular hypothesis (Pruppacher and Klett, 2010). Jhet depends on temperature and RHice. Jhet was derived following the approach in previous works (Wang and Knopf, 2011; Wang et al., 2012a; China et al., 2017; Knopf et al., 2022). Using the observed ice nucleation data, , where Nice is the number of the observed ice nucleation events that occurred in a temperature interval, Atot is the total particle surface area available for ice nucleation experiment in the temperature interval, t of 6 s is the time interval between two consecutive OM images used to monitor ice formation. In the four groups of particle samples we investigated, Jhet for DIN ranged from 130 to 1370 cm−2 s−1, 40 to 2970 cm−2 s−1, 40 to 1480 cm−2 s−1, and 10 to 390 cm−2 s−1 for the Dust-dominated, BBA-influenced, CNOS- and SS/Sulf-dominated, and FreshSS- and AgedSS-dominated samples, respectively. Jhet for IMF ranged from 540 to 4780 cm−2 s−1 for the FreshSS- and AgedSS-dominated samples.
3.6.2 Parameterizations of Jhet and θ
The experimentally derived Jhet was parameterized based on the water-activity-based approach. The water-activity-based approach has been widely used to describe the homogeneous ice nucleation of droplets and depends only on a droplet's water activity (aw) and Tp (Koop et al., 2000). Homogeneous ice nucleation temperature follows a single curve as a function of aw and is parameterized by a constant shift of aw (i.e., Δaw) from the ice melting temperature curve (Koop and Zobrist, 2009). This approach has also been applied to heterogeneous ice nucleation including DIN and IMF (Koop and Zobrist, 2009; Knopf and Alpert, 2013; China et al., 2017). It is assumed that particles were in equilibrium with the gas phase during the experiments, so . For each nucleation event, Δaw(Tf) is the difference between the RHw at which ice nucleated and the RHw on the ice melting curve at the observed ice nucleation temperature (Tf), or aw(Tf) and , respectively. Following this, (Knopf and Alpert, 2013; Koop and Zobrist, 2009), which can be calculated for each ice nucleation event using the ice nucleation onset temperature and RHw. Jhet was calculated for all ice nucleation events and parameterized as a function of Δaw following the previous works (China et al., 2017; Alpert et al., 2022; Knopf et al., 2022, 2023). Figure 12 presents the Jhet(Δaw) for the DIN and IMF data along with the parameterizations according to . The values of c and d parameters are listed in Table 2. The Dust-dominated sample has higherJhet at the same Δaw compared with the other samples. The BBA influenced sample only nucleated ice at higher Δaw. The DIN Jhet for the FreshSS and AgedSS samples changes by 2 orders of magnitude over a wide range of Δaw. For the FreshSS and AgedSS samples, IMFJhet with a larger c displays a steeper slope than DIN Jhet.
In Fig. 12, we compared our Jhet parameterizations with previous studies. DIN Jhet of the Dust-dominated sample is similar to the particles collected during the Aerosol and Cloud Experiments in the Eastern North Atlantic (ACE ENA) field campaign within the marine boundary layer (ACE ENA MBL) (Knopf et al., 2022, 2023). It is about 1–2 orders of magnitude lower than particles collected from the free troposphere (ACE ENA FT). DIN Jhet of the other particle types investigated in this study are lower than particles collected from ACE ENA FT, ground site (ACE ENA GD), and ACE ENA MBL. This is consistent with the results showing that our particles nucleated ice at higher RHice than particles collected during ACE ENA (Fig. 11). DIN Jhet for the FreshSS and AgedSS samples is similar to the SSA investigated by Alpert et al. (2022) in the lower Δaw range (less than 0.25). This is likely because S11 nucleated ice in this Δaw range (i.e., RHice) is similar to the SSA INP investigated by Alpert et al. (2022) as they have similar morphology and composition. IMF Jhet of the FreshSS and AgedSS samples is slightly higher than particles from ACE ENA GD and 1-2 orders of magnitude higher than the other field samples (Fig. 12C). The IMF Jhet of the FreshSS and AgedSS samples displays a smaller slope with Δaw compared to the laboratory-generated particles, such as illite (Knopf and Alpert, 2013), natural dust (Alpert and Knopf, 2016; Niemand et al., 2012), leonardite particles (Knopf and Alpert, 2013), and diatomaceous material (Knopf and Alpert, 2013; Knopf et al., 2011; Alpert et al., 2011a, b). This is consistent with the study by Knopf et al. (2022), which suggested that the diversity of ambient particles exhibit different ice nucleation efficiencies compared to single-component INP types studied in the laboratory at different temperature ranges. Our results emphasize the importance of studying the ice nucleation ability of particles and their parameterizations from different geological areas with different diversities in particle characteristics.
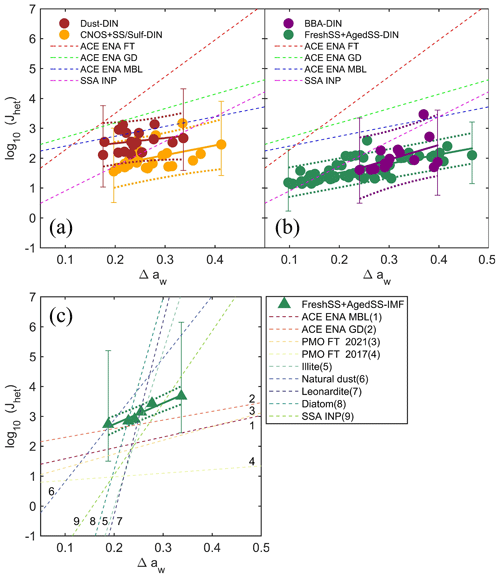
Figure 12Jhet as a function of Δaw. Circles represent DIN Jhet for (a) Dust-, CNOS-, and SS/Sulf-dominated samples and (b) BBA-, FreshSS- and AgedSS-dominated samples. Triangles represent IMF Jhet (c) for FreshSS- and AgedSS-dominated samples. Solid lines indicate the fittings according to with associated dotted lines representing the 95 % prediction intervals. Representative ΔJhet (error bars) are also plotted (Sect. S2). (a–b) Dashed red, green, and blue lines represent DIN Jhet parameterizations of particles collected from ACE ENA FT (Knopf et al., 2023), ACE ENA GD (Knopf et al., 2022), and ACE ENA MBL (Knopf et al., 2023), respectively. The dashed magenta line indicates Jhet for SSA INPs (Alpert et al., 2022). (c) Dashed lines with the number label of 1–4 represent IMF Jhet for particles from ACE ENA MBL (Knopf et al., 2023), ACE-ENA GD (Knopf et al., 2022), and the Pico Mountain Observatory (PMO) under free-tropospheric (FT) conditions in the Azores (PMO FT 2017 and PMO FT 2021) (China et al., 2017; Lata et al., 2021), respectively. Dashed lines with the number label of 5–8 represent IMF Jhet for illite particles (Knopf and Alpert, 2013), natural dust (Alpert and Knopf, 2016; Niemand et al., 2012), leonardite particles (Knopf and Alpert, 2013), diatomaceous material (Diatom) (Knopf and Alpert, 2013; Knopf et al., 2011; Alpert et al., 2011a, b), and SSA INP (Alpert et al., 2022), respectively.
θ for DIN is derived based on CNT following our previous studies (Wang and Knopf, 2011; Wang et al., 2012a). θ is calculated from Jhet and the ice nucleation onset temperature and RHice using the following formula: , where A is a pre-exponential factor and k is the Boltzmann constant. ΔFg,het is the free energy of ice embryo formation, which is defined as , where R is the universal gas constant, ρ is the density of ice, Sice is the ice saturation ratio (, Mw is the water molecular weight, and is the surface tension at the ice vapor interface. f(m) is the geometric factor and is defined as , where m=cos(θ). A smaller value of θ implies higher ice nucleation efficiency and should translate to relatively low RHice onsets in our experiments. Figure 13 represents the mean θ as a function of temperature for INPs of all sample types. When comparing θ at the same temperature, the FreshSS- and AgedSS-dominated samples, in general, have the smallest θ, followed by the Dust-dominated, CNOS- and SS/Sulf-dominated, and BBA-influenced samples. For all the investigated samples, θ was relatively constant above 220 K and increased as temperature decreased to 205 K. We propose new parameterizations of θ as a function of T3ln[RHice]2 (Sect. S2). θ for various particle types fall tightly into the parameterization as shown in Fig. S19.
3.7 Ice nucleation propensity and mixing state of particle population
Previous studies have used χ to estimate the error in predicting aerosol CCN activity and optical properties when assuming idealized mixing states (Ching et al., 2017; Yao et al., 2022). For example, Ching et al. (2017) quantified the error in predicting CCN concentration as a function of χ. When internal mixing was assumed, errors of up to 100 % overpredicting CCN concentration were found for populations with more external mixing (χ<60 %). However, CCN concentrations were well predicted for populations with χ>75 %. Inspired by these studies, we used θ as a measure of particle ice nucleation propensity to discuss the potential link between the mixed state of population and particle ice nucleation propensity. We investigated the relative difference (error) in θ for the field samples compared to pure NaCl particles. Ice nucleation experiments were conducted on the laboratory-generated NaCl particles (Sect. S3), and θ was calculated for NaCl particles (see Sect. 3.6.2 for the calculation details). Pure NaCl particles were used as a surrogate of complete internally mixed marine aerosols (χ=100 %). We analyzed the relationship between the relative error in θ of INPs (Err) and the mixing state of the population in which the INPs were identified. Err of each INP from a field sample with a given mixing state (χ) was defined as follows:
where θ (100 %) is the average θ for the NaCl standard. As shown in Fig. 14, χ for the six investigated samples changed from 61 % to 86 %. Five samples (S1, S4, S10, S12, and S14) exhibited positive mean Err ranging from 2.3 % ± 7.5 % to 16.3 % ± 6.6 %, while S11 had a negative mean Err of -18.6 % ± 6.2 %. The FreshSS- and AgedSS-dominated samples, S4 and S12, have a mean Err of 6.9 % ± 12.4 % and 13.0 % ± 9.2 %, respectively.
The θ for particles in the marine environment is different from that for NaCl particles. This can lead to errors in Jhet of several orders of magnitude if the model assumes an internally mixed particle population. According to CNT, we calculated DIN Jhet for NaCl and particles with Err of 5 % and 10 % under different temperature and humidity conditions (Fig. S20). Between 135 % and 180 % RHice, Err of 5 % and 10 % will result in 1.5–4 and 2–10 orders of magnitude differences in Jhet, respectively. Moreover, the lower the temperature and RHice, the larger the error in Jhet. This suggests that the effects of aging processes and mixing state need to be considered in the ice nucleation modeling for different oceans with complex particle sources, such as coastal regions. Instead of pure NaCl, better proxies for SSA should be used in future studies to obtain better estimations in Err and thus Jhet. No specific correlation or trend was found between χ and the Err from this data set with the limited number of samples. This may be expected since ice nucleation is controlled by various physiochemical properties of individual particles, particularly for DIN. For example, one crucial factor is the physical mixing state of particle, such as coating. Organic coatings play a significant role in altering the θ of sea salt particles, as exemplified by S11 compared to S4 and S12. In contrast to CCN, which in general can be described by the κ-Köhler theory, there are still no appropriate physical models to describe INPs. In addition, no direct connection between Err (i.e., θ) and χ for DIN is likely attributed to the fact that ice nucleation propensity for DIN depends on the particle surface composition and morphology on the individual particle basis, whereas χ characterizes the overall mixing of species (i.e., composition) within the particle population which may not sensitive to the particle surface characteristics. There is a large gap in the understanding of how physical and chemical mixing state affects ice nucleation potential of particle population. Other ice nucleation variables, e.g., INP concentration, can be used to estimate the potential error in cloud modeling if internal mixing is assumed for the population. Mixing state metrics reflecting the underlying physical process and the particle physicochemical properties controlling ice nucleation ability are needed in future studies, for example, metrics measuring the morphological characteristics of particles.
We found that the distinct mixing states of particle populations were affected by several processes over different oceans. In the marine atmosphere that already has a contribution of sea salt particles, adding more fresh sea salt particles makes the population more internally mixed (Fig. 6). We suspect that over open oceans where sea salt particle flux is large, the population will be mostly internally mixed. The addition of new particle types from other sources, such as dust storms (WP-I, S1, S2), biomass burning (WP-II, S14, S15), and secondary sources (CNOS in the SO region), will decrease the mixing state index, and the population will become more externally mixed. These results are consistent with the proposed schematic description by Riemer et al. (2019) on the evolution of particle mixing state. We showed that multiphase processes lead to the aging of fresh sea salt particles resulting in chlorine deficiency and thus changing the mixing state of the population (Fig. 5). This is a selective process that occurred on the sea salt particles and is different from the aging by condensation of secondary materials. As discussed in Sect. 3.2, these types of selective or inhomogeneous aging on particles move the population toward a more externally mixed state. To the best of our knowledge, this is not considered in the models predicting the mixing state of aerosols, even for the particle-resolved model. Our study suggests that when discussing the influence of aging on the evolution of the mixing state it is necessary to consider whether the aging processes occur uniformly on all particles.
We characterized chemical compositions for particle population and INPs at the single-particle level. We found that all the identified particle types from different sources have the potential to serve as INPs but have different ice nucleation efficiencies (Figs. 7 and 9). We found that S11 showed the highest ice nucleation efficiency among the FreshSS- and AgedSS-dominated samples (Fig. 7), which we attributed to the organic coatings on the particles. This indicates the importance of characterizing the physical mixing state of particles (i.e., morphology). INPs were enriched in SS/Sulf class, suggesting that the aging process alters ice nucleation ability by changing the chemical mixing state of particles. Considering the characterizations of individual particles and INPs, the ice nucleation onset conditions, and the derived freezing kinetics, it is suggested that the ice nucleation abilities at cirrus conditions of these complex marine aerosols are affected not only by the particle compositions but also by the mixing state of particle population. Future investigations on how the chemical and physical mixing state of particles affects ice nucleation in the atmosphere are needed for a better understanding of aerosol–cloud interactions in the climate system.
According to the CNT, the ice nucleation kinetic parameter, Jhet, was derived from the experimental data. We proposed parameterizations of Jhet as a function of Δaw for IMF and DIN based on the water-activity-based theory. In addition, θ was derived for DIN based on CNT. We proposed new parameterizations of θ taking into account both temperature and RHice. These parameterizations can be used to estimate the INP concentrations or ice crystal production rates when particle surface area data are available. These parameterizations can be used in cloud models with different ice nucleation descriptions to evaluate the potential sources and impacts of different particles on cloud formation in the marine atmosphere.
Composition, mixing state, and ice nucleation properties were investigated for the aerosol particles collected over the western Pacific and Southern Ocean during a cruise from South Korea (34.93 °N) to the Ross Sea (75.12 °S) in 2019. Seven particle classes were identified including fresh sea salt, aged sea salt, sea salt mixed with sulfate, carbonaceous particle, sulfur-containing particle, dust, and a mixed class. Contributions of these particles varied across oceans at different latitudes. Except for the samples impacted by dust storm, fresh sea salt, aged sea salt, and sea salt mixed with sulfate particles were the most prevalent particle types, with the total number percentage ranging from 48 % to 99 %. Particles showed different degrees of chloride depletion due to the aging processes of acid displacements on sea salt particles. Significant contributions of sulfur-containing particles up to 93 % in number were found in the Ross Sea, which was affected by phytoplankton blooms in the austral summer. The chemical mixing state index of particle population ranges from 50 % to 95 %. The mixing state index was positively correlated with the number percentages of fresh sea salt and negatively correlated with the proportion of sulfur-containing particles. Particle populations tended to be more internally mixed as the fresh sea salt fraction increased. When sea salt particles were a background or dominant particle type, inhomogeneous aging processes and new particle sources made the population more externally mixed.
We showed that different types of particle samples demonstrated a variety of ice nucleation abilities at cirrus conditions. The sample dominated by the fresh sea salt particles with organic coatings exhibited the highest ice nucleation efficiency with RHice onset as low as 121 %, while particles influenced by biomass burning were the least efficient among the investigated samples. According to the individual INP characterizations, the dominant particle types in the population all contribute to the identified INPs but with different enrichment factors. The sea salt mixed with sulfate particles were enriched in INPs with an overall factor of about 1.9. Ice nucleation abilities of marine aerosol particles were affected not only by composition but also by the chemical and physical mixing state of the particle population. Ice nucleation kinetic parameters, ice nucleation rate coefficient, and contact angle were derived from the experimental data. The corresponding parameterizations for different particle types are provided for cloud modeling. In this study, we demonstrated the diversity in the particle characteristics at the single-particle level, the mixing state of particle population, and particles' abilities to form ice crystals in different marine atmospheres in both the Northern Hemisphere and Southern Hemisphere. The results motivate the need for further investigations into how the mixing state of particles affects ice nucleation in the atmosphere.
All data are given in the main text or in the Supplement. HYSPLIT data were obtained through the NOAA website (https://www.ready.noaa.gov/hypub-bin/trajasrc.pl, NOAA Air Resources Laboratory, 2023). NAAPS aerosol reanalysis data were obtained from https://nrlgodae1.nrlmry.navy.mil/ftp/outgoing/nrl/NAAPS-REANALYSIS/2019/ (Office of Naval Research, 2022). FIRMS data were obtained from https://firms.modaps.eosdis.nasa.gov/ (Fire Information for Resource Management System US/Canada, 2023).
The supplement related to this article is available online at: https://doi.org/10.5194/acp-24-7731-2024-supplement.
JX performed the experiments and wrote the first draft of the manuscript. JX, TZ, JP, and BW performed the data analysis. JP and KP collected the samples. BW, JP and KP initiated and designed the study. BW supervised the project. BW, YJY, and JX led the discussion and all authors contributed to the data interpretation and the manuscript writing.
The contact author has declared that none of the authors has any competing interests.
Publisher's note: Copernicus Publications remains neutral with regard to jurisdictional claims made in the text, published maps, institutional affiliations, or any other geographical representation in this paper. While Copernicus Publications makes every effort to include appropriate place names, the final responsibility lies with the authors.
We thank the staff onboard R/V Araon helping with the sampling during the Antarctic cruise and Peter A. Alpert for helpful discussion and manuscript revision. Tian Zhang acknowledges support from the PhD Fellowship of the State Key Laboratory of Marine Environmental Science at Xiamen University.
This research has been supported by National Natural Science Foundation of China (grant nos. 42075076 and 41775133), Fundamental Research Funds for the Central Universities (grant nos. 20720160111 and 20720190147), the Korea Polar Research Institute research grant (grant nos. PE24110 and PE24030), and the Fund of Key Laboratory of Global Change and Marine-Atmospheric Chemistry, MNR (grant no. GCMAC2004).
This paper was edited by Luis A. Ladino and reviewed by Jie Chen and two anonymous referees.
Adachi, K., Oshima, N., Gong, Z., de Sá, S., Bateman, A. P., Martin, S. T., de Brito, J. F., Artaxo, P., Cirino, G. G., Sedlacek III, A. J., and Buseck, P. R.: Mixing states of Amazon basin aerosol particles transported over long distances using transmission electron microscopy, Atmos. Chem. Phys., 20, 11923–11939, https://doi.org/10.5194/acp-20-11923-2020, 2020.
Alpert, P. A. and Knopf, D. A.: Analysis of isothermal and cooling-rate-dependent immersion freezing by a unifying stochastic ice nucleation model, Atmos. Chem. Phys., 16, 2083–2107, https://doi.org/10.5194/acp-16-2083-2016, 2016.
Alpert, P. A., Aller, J. Y., and Knopf, D. A.: Ice nucleation from aqueous NaCl droplets with and without marine diatoms, Atmos. Chem. Phys., 11, 5539–5555, https://doi.org/10.5194/acp-11-5539-2011, 2011a.
Alpert, P. A., Aller, J. Y., and Knopf, D. A.: Initiation of the ice phase by marine biogenic surfaces in supersaturated gas and supercooled aqueous phases, Phys. Chem. Chem. Phys., 13, 19882, https://doi.org/10.1039/c1cp21844a, 2011b.
Alpert, P. A., Kilthau, W. P., O'Brien, R. E., Moffet, R. C., Gilles, M. K., Wang, B., Laskin, A., Aller, J. Y., and Knopf, D. A.: Ice-nucleating agents in sea spray aerosol identified and quantified with a holistic multimodal freezing model, Sci. Adv., 8, 1–11, https://doi.org/10.1126/sciadv.abq6842, 2022.
Angle, K. J., Crocker, D. R., Simpson, R. M. C., Mayer, K. J., Garofalo, L. A., Moore, A. N., Mora Garcia, S. L., Or, V. W., Srinivasan, S., Farhan, M., Sauer, J. S., Lee, C., Pothier, M. A., Farmer, D. K., Martz, T. R., Bertram, T. H., Cappa, C. D., Prather, K. A., and Grassian, V. H.: Acidity across the interface from the ocean surface to sea spray aerosol, P. Natl. Acad. Sci. USA, 118, 1–6, https://doi.org/10.1073/pnas.2018397118, 2021.
Ault, A. P., Moore, M. J., Furutani, H., and Prather, K. A.: Impact of emissions from the Los Angeles Port Region on San Diego air quality during regional transport events, Environ. Sci. Technol., 43, 3500–3506, https://doi.org/10/d7kt9s, 2009.
Ault, A. P., Gaston, C. J., Wang, Y., Dominguez, G., Thiemens, M. H., and Prather, K. A.: Characterization of the single particle mixing state of individual ship plume events measured at the Port of Los Angeles, Environ. Sci. Technol., 44, 1954–1961, https://doi.org/10.1021/es902985h, 2010.
Baccarini, A., Dommen, J., Lehtipalo, K., Henning, S., Modini, R. L., Gysel-Beer, M., Baltensperger, U., and Schmale, J.: Low-volatility vapors and new particle formation over the Southern Ocean during the Antarctic circumnavigation expedition, J. Geophys. Res.-Atmos., 126, e2021JD035126, https://doi.org/10.1029/2021JD035126, 2021.
Barnes, I., Hjorth, J., and Mihalopoulos, N.: Dimethyl sulfide and dimethyl sulfoxide and their oxidation in the atmosphere, Chem. Rev., 106, 940–975, https://doi.org/10.1021/cr020529+, 2006.
Beck, L. J., Sarnela, N., Junninen, H., Hoppe, C. J. M., Garmash, O., Bianchi, F., Riva, M., Rose, C., Peräkylä, O., Wimmer, D., Kausiala, O., Jokinen, T., Ahonen, L., Mikkilä, J., Hakala, J., He, X., Kontkanen, J., Wolf, K. K. E., Cappelletti, D., Mazzola, M., Traversi, R., Petroselli, C., Viola, A. P., Vitale, V., Lange, R., Massling, A., Nøjgaard, J. K., Krejci, R., Karlsson, L., Zieger, P., Jang, S., Lee, K., Vakkari, V., Lampilahti, J., Thakur, R. C., Leino, K., Kangasluoma, J., Duplissy, E., Siivola, E., Marbouti, M., Tham, Y. J., Saiz-Lopez, A., Petäjä, T., Ehn, M., Worsnop, D. R., Skov, H., Kulmala, M., Kerminen, V., and Sipilä, M.: Differing mechanisms of new particle formation at two Arctic sites, Geophys. Res. Lett., 48, 1–11, https://doi.org/10/gjrb6h, 2021.
Berndt, T., Hoffmann, E. H., Tilgner, A., Stratmann, F., and Herrmann, H.: Direct sulfuric acid formation from the gas-phase oxidation of reduced-sulfur compounds, Nat. Commun., 14, 4849, https://doi.org/10/gs3whz, 2023.
Bigg, E. K.: Ice nucleus concentrations in remote areas, J. Atmos. Sci., 30, 1153–1157, https://doi.org/10.1175/1520-0469(1973)030<1153:INCIRA>2.0.CO;2, 1973.
Brean, J., Dall'Osto, M., Simó, R., Shi, Z., Beddows, D. C. S., and Harrison, R. M.: Open ocean and coastal new particle formation from sulfuric acid and amines around the Antarctic Peninsula, Nat. Geosci., 14, 383–388, https://doi.org/10.1038/s41561-021-00751-y, 2021.
Burrows, S. M., McCluskey, C. S., Cornwell, G., Steinke, I., Zhang, K., Zhao, B., Zawadowicz, M., Raman, A., Kulkarni, G., China, S., Zelenyuk, A., and DeMott, P. J.: Ice-nucleating particles that impact clouds and climate: observational and modeling research needs, Rev. Geophys., 60, 1–45, https://doi.org/10/gs6w5x, 2022.
Charnawskas, J. C., Alpert, P. A., Lambe, A. T., Berkemeier, T., O'Brien, R. E., Massoli, P., Onasch, T. B., Shiraiwa, M., Moffet, R. C., Gilles, M. K., Davidovits, P., Worsnop, D. R., and Knopf, D. A.: Condensed-phase biogenic-anthropogenic interactions with implications for cold cloud formation, Faraday Discuss., 200, 165–194, https://doi.org/10.1039/C7FD00010C, 2017.
Chen, J., Li, C., Ristovski, Z., Milic, A., Gu, Y., Islam, M. S., Wang, S., Hao, J., Zhang, H., He, C., Guo, H., Fu, H., Miljevic, B., Morawska, L., Thai, P., Lam, Y. F., Pereira, G., Ding, A., Huang, X., and Dumka, U. C.: A review of biomass burning: Emissions and impacts on air quality, health and climate in China, Sci. Total Environ., 579, 1000–1034, https://doi.org/10.1016/j.scitotenv.2016.11.025, 2017.
Chen, Q., Sherwen, T., Evans, M., and Alexander, B.: DMS oxidation and sulfur aerosol formation in the marine troposphere: a focus on reactive halogen and multiphase chemistry, Atmos. Chem. Phys., 18, 13617–13637, https://doi.org/10.5194/acp-18-13617-2018, 2018.
China, S., Alpert, P. A., Zhang, B., Schum, S., Dzepina, K., Wright, K., Owen, R. C., Fialho, P., Mazzoleni, L. R., Mazzoleni, C., and Knopf, D. A.: Ice cloud formation potential by free tropospheric particles from long-range transport over the Northern Atlantic Ocean, J. Geophys. Res.-Atmos., 122, 3065–3079, https://doi.org/10.1002/2016JD025817, 2017.
China, S., Burrows, S. M., Wang, B., Harder, T. H., Weis, J., Tanarhte, M., Rizzo, L. V., Brito, J., Cirino, G. G., Ma, P.-L., Cliff, J., Artaxo, P., Gilles, M. K., and Laskin, A.: Fungal spores as a source of sodium salt particles in the Amazon basin, Nat. Commun., 9, 4793, https://doi.org/10.1038/s41467-018-07066-4, 2018.
Ching, J., Fast, J., West, M., and Riemer, N.: Metrics to quantify the importance of mixing state for CCN activity, Atmos. Chem. Phys., 17, 7445–7458, https://doi.org/10.5194/acp-17-7445-2017, 2017.
Chou, C., Kanji, Z. A., Stetzer, O., Tritscher, T., Chirico, R., Heringa, M. F., Weingartner, E., Prévôt, A. S. H., Baltensperger, U., and Lohmann, U.: Effect of photochemical ageing on the ice nucleation properties of diesel and wood burning particles, Atmos. Chem. Phys., 13, 761–772, https://doi.org/10.5194/acp-13-761-2013, 2013.
Cochran, R. E., Ryder, O. S., Grassian, V. H., and Prather, K. A.: Sea spray aerosol: The chemical link between the oceans, atmosphere, and climate, Acc. Chem. Res., 50, 599–604, https://doi.org/10.1021/acs.accounts.6b00603, 2017.
Connolly, P. J., Möhler, O., Field, P. R., Saathoff, H., Burgess, R., Choularton, T., and Gallagher, M.: Studies of heterogeneous freezing by three different desert dust samples, Atmos. Chem. Phys., 9, 2805–2824, https://doi.org/10.5194/acp-9-2805-2009, 2009.
Cornwell, G. C., McCluskey, C. S., Levin, E. J. T., Suski, K. J., DeMott, P. J., Kreidenweis, S. M., and Prather, K. A.: Direct online mass spectrometry measurements of ice nucleating particles at a California coastal site, J. Geophys. Res.-Atmos., 124, 12157–12172, https://doi.org/10.1029/2019JD030466, 2019.
Cziczo, D. J., Ladino, L., Boose, Y., Kanji, Z. A., Kupiszewski, P., Lance, S., Mertes, S., and Wex, H.: Measurements of ice nucleating particles and ice residuals, Meteorol. Monogr., 58, 8.1–8.13, https://doi.org/10.1175/AMSMONOGRAPHS-D-16-0008.1, 2017.
De Leeuw, G., Andreas, E. L., Anguelova, M. D., Fairall, C. W., Lewis, E. R., O'Dowd, C., Schulz, M., and Schwartz, S. E.: Production flux of sea spray aerosol, Rev. Geophys., 49, 1–39, https://doi.org/10.1029/2010RG000349, 2011.
DeMott, P. J., Chen, Y., Kreidenweis, S. M., Rogers, D. C., and Sherman, D. E.: Ice formation by black carbon particles, Geophys. Res. Lett., 26, 2429–2432, https://doi.org/10.1029/1999GL900580, 1999.
DeMott, P. J., Petters, M. D., Prenni, A. J., Carrico, C. M., Kreidenweis, S. M., Collett, J. L., and Moosmüller, H.: Ice nucleation behavior of biomass combustion particles at cirrus temperatures, J. Geophys. Res.-Atmos., 114, 1–13, https://doi.org/10.1029/2009JD012036, 2009.
DeMott, P. J., Hill, T. C. J., McCluskey, C. S., Prather, K. A., Collins, D. B., Sullivan, R. C., Ruppel, M. J., Mason, R. H., Irish, V. E., Lee, T., Hwang, C. Y., Rhee, T. S., Snider, J. R., McMeeking, G. R., Dhaniyala, S., Lewis, E. R., Wentzell, J. J. B., Abbatt, J., Lee, C., Sultana, C. M., Ault, A. P., Axson, J. L., Diaz Martinez, M., Venero, I., Santos-Figueroa, G., Stokes, M. D., Deane, G. B., Mayol-Bracero, O. L., Grassian, V. H., Bertram, T. H., Bertram, A. K., Moffett, B. F., and Franc, G. D.: Sea spray aerosol as a unique source of ice nucleating particles, P. Natl. Acad. Sci. USA, 113, 5797–5803, https://doi.org/10.1073/pnas.1514034112, 2016.
Ditas, J., Ma, N., Zhang, Y., Assmann, D., Neumaier, M., Riede, H., Karu, E., Williams, J., Scharffe, D., Wang, Q., Saturno, J., Schwarz, J. P., Katich, J. M., McMeeking, G. R., Zahn, A., Hermann, M., Brenninkmeijer, C. A. M., Andreae, M. O., Pöschl, U., Su, H., and Cheng, Y.: Strong impact of wildfires on the abundance and aging of black carbon in the lowermost stratosphere, P. Natl. Acad. Sci. USA, 115, E11595–E11603, https://doi.org/10.1073/pnas.1806868115, 2018.
Ervens, B., Sorooshian, A., Aldhaif, A. M., Shingler, T., Crosbie, E., Ziemba, L., Campuzano-Jost, P., Jimenez, J. L., and Wisthaler, A.: Is there an aerosol signature of chemical cloud processing?, Atmos. Chem. Phys., 18, 16099–16119, https://doi.org/10.5194/acp-18-16099-2018, 2018.
Fire Information for Resource Management System US/Canada: Providing Active Fire Data for Near-Real Time Monitoring and Applications, https://firms.modaps.eosdis.nasa.gov/ (last access: 5 January 2023), 2023.
Fraund, M., Pham, D., Bonanno, D., Harder, T., Wang, B., Brito, J., de Sá, S., Carbone, S., China, S., Artaxo, P., Martin, S., Pöhlker, C., Andreae, M., Laskin, A., Gilles, M., and Moffet, R.: Elemental mixing state of aerosol particles collected in central Amazonia during GoAmazon2014/15, Atmosphere, 8, 1–27, https://doi.org/10.3390/atmos8090173, 2017.
Fu, P. Q., Kawamura, K., Chen, J., Charrière, B., and Sempéré, R.: Organic molecular composition of marine aerosols over the Arctic Ocean in summer: contributions of primary emission and secondary aerosol formation, Biogeosciences, 10, 653–667, https://doi.org/10.5194/bg-10-653-2013, 2013.
Fuzzi, S., Decesari, S., Facchini, M. C., Cavalli, F., Emblico, L., Mircea, M., Andreae, M. O., Trebs, I., Hoffer, A., Guyon, P., Artaxo, P., Rizzo, L. V., Lara, L. L., Pauliquevis, T., Maenhaut, W., Raes, N., Chi, X., Mayol-Bracero, O. L., Soto-García, L. L., Claeys, M., Kourtchev, I., Rissler, J., Swietlicki, E., Tagliavini, E., Schkolnik, G., Falkovich, A. H., Rudich, Y., Fisch, G., and Gatti, L. V.: Overview of the inorganic and organic composition of size-segregated aerosol in Rondônia, Brazil, from the biomass-burning period to the onset of the wet season, J. Geophys. Res.-Atmos., 112, 1–35, https://doi.org/10.1029/2005JD006741, 2007.
Geng, X., Zhong, G., Li, J., Cheng, Z., Mo, Y., Mao, S., Su, T., Jiang, H., Ni, K., and Zhang, G.: Molecular marker study of aerosols in the northern South China Sea: Impact of atmospheric outflow from the Indo-China Peninsula and South China, Atmos. Environ., 206, 225–236, https://doi.org/10.1016/j.atmosenv.2019.02.033, 2019.
Gong, X., Wex, H., van Pinxteren, M., Triesch, N., Fomba, K. W., Lubitz, J., Stolle, C., Robinson, T.-B., Müller, T., Herrmann, H., and Stratmann, F.: Characterization of aerosol particles at Cabo Verde close to sea level and at the cloud level – Part 2: Ice-nucleating particles in air, cloud and seawater, Atmos. Chem. Phys., 20, 1451–1468, https://doi.org/10.5194/acp-20-1451-2020, 2020.
Han, Y., Fang, X., Xi, X., Song, L., and Yang, S.: Dust storm in Asia continent and its bio-environmental effects in the North Pacific: A case study of the strongest dust event in April, 2001 in central Asia, Sci. Bull., 51, 723–730, https://doi.org/10.1007/s11434-006-0723-2, 2006.
Hartmann, M., Adachi, K., Eppers, O., Haas, C., Herber, A., Holzinger, R., Hünerbein, A., Jäkel, E., Jentzsch, C., Pinxteren, M., Wex, H., Willmes, S., and Stratmann, F.: Wintertime Airborne Measurements of Ice Nucleating Particles in the High Arctic: A Hint to a Marine, Biogenic Source for Ice Nucleating Particles, Geophys. Res. Lett., 47, e2020GL087770, https://doi.org/10.1029/2020GL087770, 2020.
Healy, R. M., Sciare, J., Poulain, L., Crippa, M., Wiedensohler, A., Prévôt, A. S. H., Baltensperger, U., Sarda-Estève, R., McGuire, M. L., Jeong, C.-H., McGillicuddy, E., O'Connor, I. P., Sodeau, J. R., Evans, G. J., and Wenger, J. C.: Quantitative determination of carbonaceous particle mixing state in Paris using single-particle mass spectrometer and aerosol mass spectrometer measurements, Atmos. Chem. Phys., 13, 9479–9496, https://doi.org/10.5194/acp-13-9479-2013, 2013.
Hirsch, E. and Koren, I.: Record-breaking aerosol levels explained by smoke injection into the stratosphere, Science, 371, 1269–1274, https://doi.org/10.1126/science.abe1415, 2021.
Hodshire, A. L., Akherati, A., Alvarado, M. J., Brown-Steiner, B., Jathar, S. H., Jimenez, J. L., Kreidenweis, S. M., Lonsdale, C. R., Onasch, T. B., Ortega, A. M., and Pierce, J. R.: Aging effects on biomass burning aerosol mass and composition: a critical review of field and laboratory studies, Environ. Sci. Technol., 53, 10007–10022, https://doi.org/10.1021/acs.est.9b02588, 2019.
Hoose, C. and Möhler, O.: Heterogeneous ice nucleation on atmospheric aerosols: a review of results from laboratory experiments, Atmos. Chem. Phys., 12, 9817–9854, https://doi.org/10.5194/acp-12-9817-2012, 2012.
Hopkins, R. J., Desyaterik, Y., Tivanski, A. V., Zaveri, R. A., Berkowitz, C. M., Tyliszczak, T., Gilles, M. K., and Laskin, A.: Chemical speciation of sulfur in marine cloud droplets and particles: Analysis of individual particles from the marine boundary layer over the California current, J. Geophys. Res.-Atmos., 113, 1–15, https://doi.org/10.1029/2007JD008954, 2008.
Inoue, J., Tobo, Y., Taketani, F., and Sato, K.: Oceanic supply of ice-nucleating particles and its effect on ice cloud formation: A case study in the Arctic Ocean during a cold-air outbreak in early winter, Geophys. Res. Lett., 48, e2021GL094646, https://doi.org/10.1029/2021GL094646, 2021.
IPCC: Climate change 2021: the physical science basis, Cambridge University Press, 2391 pp., https://doi.org/10.1017/9781009157896, 2021.
Jahn, L. G., Polen, M. J., Jahl, L. G., Brubaker, T. A., Somers, J., and Sullivan, R. C.: Biomass combustion produces ice-active minerals in biomass-burning aerosol and bottom ash, P. Natl. Acad. Sci. USA, 117, 21928–21937, https://doi.org/10.1073/pnas.1922128117, 2020.
Jahl, L. G., Brubaker, T. A., Polen, M. J., Jahn, L. G., Cain, K. P., Bowers, B. B., Fahy, W. D., Graves, S., and Sullivan, R. C.: Atmospheric aging enhances the ice nucleation ability of biomass-burning aerosol, Sci. Adv., 7, 1–9, https://doi.org/10.1126/sciadv.abd3440, 2021.
Jang, E., Park, K.-T., Yoon, Y. J., Kim, T.-W., Hong, S.-B., Becagli, S., Traversi, R., Kim, J., and Gim, Y.: New particle formation events observed at the King Sejong Station, Antarctic Peninsula – Part 2: Link with the oceanic biological activities, Atmos. Chem. Phys., 19, 7595–7608, https://doi.org/10.5194/acp-19-7595-2019, 2019.
Jang, J., Park, J., Park, J., Yoon, Y. J., Dall'Osto, M., Park, K.-T., Jang, E., Lee, J. Y., Cho, K. H., and Lee, B. Y.: Ocean-atmosphere interactions: Different organic components across Pacific and Southern Oceans, Sci. Total Environ., 878, 1–12, https://doi.org/10/gs3zkw, 2023.
Kanji, Z. A., DeMott, P. J., Möhler, O., and Abbatt, J. P. D.: Results from the University of Toronto continuous flow diffusion chamber at ICIS 2007: instrument intercomparison and ice onsets for different aerosol types, Atmos. Chem. Phys., 11, 31–41, https://doi.org/10.5194/acp-11-31-2011, 2011.
Kanji, Z. A., Ladino, L. A., Wex, H., Boose, Y., Burkert-Kohn, M., Cziczo, D. J., and Krämer, M.: Overview of ice nucleating particles, Meteorol. Monogr., 58, 1.1–1.33, https://doi.org/10.1175/AMSMONOGRAPHS-D-16-0006.1, 2017.
Khvorostyanov, V. I. and Curry, J. A.: The theory of ice nucleation by heterogeneous freezing of deliquescent mixed CCN. Part I: Critical radius, energy, and nucleation rate, J. Atmos. Sci., 61, 2676–2691, https://doi.org/10/b7nhwf, 2004.
Knopf, D. A. and Alpert, P. A.: A water activity based model of heterogeneous ice nucleation kinetics for freezing of water and aqueous solution droplets, Faraday Discuss., 165, 513–534, https://doi.org/10.1039/c3fd00035d, 2013.
Knopf, D. A., Alpert, P. A., Wang, B., and Aller, J. Y.: Stimulation of ice nucleation by marine diatoms, Nat. Geosci., 4, 88–90, https://doi.org/10.1038/ngeo1037, 2011.
Knopf, D. A., Alpert, P. A., Wang, B., O'Brien, R. E., Kelly, S. T., Laskin, A., Gilles, M. K., and Moffet, R. C.: Microspectroscopic imaging and characterization of individually identified ice nucleating particles from a case field study, J. Geophys. Res.-Atmos., 119, 10365–10381, https://doi.org/10.1002/2014JD021866, 2014.
Knopf, D. A., Alpert, P. A., and Wang, B.: The role of organic aerosol in atmospheric ice nucleation: a review, ACS Earth Space Chem., 2, 168–202, https://doi.org/10.1021/acsearthspacechem.7b00120, 2018.
Knopf, D. A., Charnawskas, J. C., Wang, P., Wong, B., Tomlin, J. M., Jankowski, K. A., Fraund, M., Veghte, D. P., China, S., Laskin, A., Moffet, R. C., Gilles, M. K., Aller, J. Y., Marcus, M. A., Raveh-Rubin, S., and Wang, J.: Micro-spectroscopic and freezing characterization of ice-nucleating particles collected in the marine boundary layer in the eastern North Atlantic, Atmos. Chem. Phys., 22, 5377–5398, https://doi.org/10.5194/acp-22-5377-2022, 2022.
Knopf, D. A., Wang, P., Wong, B., Tomlin, J. M., Veghte, D. P., Lata, N. N., China, S., Laskin, A., Moffet, R. C., Aller, J. Y., Marcus, M. A., and Wang, J.: Physicochemical characterization of free troposphere and marine boundary layer ice-nucleating particles collected by aircraft in the eastern North Atlantic, Atmos. Chem. Phys., 23, 8659–8681, https://doi.org/10.5194/acp-23-8659-2023, 2023.
Koehler, K. A., DeMott, P. J., Kreidenweis, S. M., Popovicheva, O. B., Petters, M. D., Carrico, C. M., Kireeva, E. D., Khokhlova, T. D., and Shonija, N. K.: Cloud condensation nuclei and ice nucleation activity of hydrophobic and hydrophilic soot particles, Phys. Chem. Chem. Phys., 11, 7906–7920, https://doi.org/10.1039/b905334b, 2009.
Koop, T. and Zobrist, B.: Parameterizations for ice nucleation in biological and atmospheric systems, Phys. Chem. Chem. Phys., 11, 10839, https://doi.org/10.1039/b914289d, 2009.
Koop, T., Luo, B., Tsias, A., and Peter, T.: Water activity as the determinant for homogeneous ice nucleation in aqueous solutions, Nature, 406, 611–614, https://doi.org/10.1038/35020537, 2000.
Kulkarni, G., Sanders, C., Zhang, K., Liu, X., and Zhao, C.: Ice nucleation of bare and sulfuric acid-coated mineral dust particles and implication for cloud properties: Ice formation on dust particles, J. Geophys. Res.-Atmos., 119, 9993–10011, https://doi.org/10.1002/2014JD021567, 2014.
Kunwar, B., Pokhrel, A., Niwai, T., and Kawamura, K.: Spatial and longitudinal distributions of total carbon, nitrogen and sulfur together with water-soluble major ions in marine aerosols collected from the Western Pacific and Southern Ocean, J. Geophys. Res.-Atmos., 128, 1–23, https://doi.org/10.1029/2022JD037874, 2023.
Ladino, L. A., Yakobi-Hancock, J. D., Kilthau, W. P., Mason, R. H., Si, M., Li, J., Miller, L. A., Schiller, C. L., Huffman, J. A., Aller, J. Y., Knopf, D. A., Bertram, A. K., and Abbatt, J. P. D.: Addressing the ice nucleating abilities of marine aerosol: A combination of deposition mode laboratory and field measurements, Atmos. Environ., 132, 1–10, https://doi.org/10.1016/j.atmosenv.2016.02.028, 2016.
Ladino, L. A., Raga, G. B., Alvarez-Ospina, H., Andino-Enríquez, M. A., Rosas, I., Martínez, L., Salinas, E., Miranda, J., Ramírez-Díaz, Z., Figueroa, B., Chou, C., Bertram, A. K., Quintana, E. T., Maldonado, L. A., García-Reynoso, A., Si, M., and Irish, V. E.: Ice-nucleating particles in a coastal tropical site, Atmos. Chem. Phys., 19, 6147–6165, https://doi.org/10.5194/acp-19-6147-2019, 2019.
Laskin, A., Iedema, M. J., and Cowin, J. P.: Quantitative time-resolved monitoring of nitrate formation in sea salt particles using a CCSEM/EDX single particle analysis, Environ. Sci. Technol., 36, 4948–4955, https://doi.org/10.1021/es020551k, 2002.
Laskin, A., Cowin, J. P., and Iedema, M. J.: Analysis of individual environmental particles using modern methods of electron microscopy and X-ray microanalysis, J. Electron. Spectrosc. Relat. Phenom., 150, 260–274, https://doi.org/10.1016/j.elspec.2005.06.008, 2006.
Laskin, A., Moffet, R. C., Gilles, M. K., Fast, J. D., Zaveri, R. A., Wang, B., Nigge, P., and Shutthanandan, J.: Tropospheric chemistry of internally mixed sea salt and organic particles: Surprising reactivity of NaCl with weak organic acids, J. Geophys. Res.-Atmos., 117, 1–12, https://doi.org/10.1029/2012JD017743, 2012.
Lata, N. N., Zhang, B., Schum, S., Mazzoleni, L., Brimberry, R., Marcus, M. A., Cantrell, W. H., Fialho, P., Mazzoleni, C., and China, S.: Aerosol composition, mixing state, and phase state of free tropospheric particles and their role in ice cloud formation, ACS Earth Space Chem., 5, 3499–3510, https://doi.org/10.1021/acsearthspacechem.1c00315, 2021.
Li, W., Shao, L., Zhang, D., Ro, C.-U., Hu, M., Bi, X., Geng, H., Matsuki, A., Niu, H., and Chen, J.: A review of single aerosol particle studies in the atmosphere of East Asia: morphology, mixing state, source, and heterogeneous reactions, J. Clean. Prod., 112, 1330–1349, https://doi.org/10.1016/j.jclepro.2015.04.050, 2016.
Liu, X. and Penner, J. E.: Ice nucleation parameterization for global models, Meteor. Z., 14, 499–514, https://doi.org/10/b3b83s, 2005.
Liu, Y., Minofar, B., Desyaterik, Y., Dames, E., Zhu, Z., Cain, J. P., Hopkins, R. J., Gilles, M. K., Wang, H., Jungwirth, P., and Laskin, A.: Internal structure, hygroscopic and reactive properties of mixed sodium methanesulfonate-sodium chloride particles, Phys. Chem. Chem. Phys., 13, 11846–11857, https://doi.org/10.1039/c1cp20444k, 2011.
MacKay, D. J. C.: Information theory, inference, and learning algorithms, Cambridge University Press, Cambridge, 628 pp., https://assets.cambridge.org/97805216/42989/frontmatter/9780521642989_frontmatter.pdf (last access: 6 October 2023), 2003.
Mason, R. H., Si, M., Li, J., Chou, C., Dickie, R., Toom-Sauntry, D., Pöhlker, C., Yakobi-Hancock, J. D., Ladino, L. A., Jones, K., Leaitch, W. R., Schiller, C. L., Abbatt, J. P. D., Huffman, J. A., and Bertram, A. K.: Ice nucleating particles at a coastal marine boundary layer site: correlations with aerosol type and meteorological conditions, Atmos. Chem. Phys., 15, 12547–12566, https://doi.org/10.5194/acp-15-12547-2015, 2015.
McCluskey, C. S., Hill, T. C. J., Malfatti, F., Sultana, C. M., Lee, C., Santander, M. V., Beall, C. M., Moore, K. A., Cornwell, G. C., Collins, D. B., Prather, K. A., Jayarathne, T., Stone, E. A., Azam, F., Kreidenweis, S. M., and DeMott, P. J.: A dynamic link between ice nucleating particles released in nascent sea spray aerosol and oceanic biological activity during two mesocosm experiments, J. Atmos. Sci., 74, 151–166, https://doi.org/10.1175/JAS-D-16-0087.1, 2017.
McCluskey, C. S., Ovadnevaite, J., Rinaldi, M., Atkinson, J., Belosi, F., Ceburnis, D., Marullo, S., Hill, T. C. J., Lohmann, U., Kanji, Z. A., O'Dowd, C., Kreidenweis, S. M., and DeMott, P. J.: Marine and terrestrial organic ice-nucleating particles in pristine marine to continentally influenced Northeast Atlantic air masses, J. Geophys. Res.-Atmos., 123, 6196–6212, https://doi.org/10/gpjrfh, 2018a.
McCluskey, C. S., Hill, T. C. J., Humphries, R. S., Rauker, A. M., Moreau, S., Strutton, P. G., Chambers, S. D., Williams, A. G., McRobert, I., Ward, J., Keywood, M. D., Harnwell, J., Ponsonby, W., Loh, Z. M., Krummel, P. B., Protat, A., Kreidenweis, S. M., and DeMott, P. J.: Observations of ice nucleating particles over Southern Ocean waters, Geophys. Res. Lett., 45, 11–989, https://doi.org/10.1029/2018GL079981, 2018b.
McCoy, I. L., Bretherton, C. S., Wood, R., Twohy, C. H., Gettelman, A., Bardeen, C. G., and Toohey, D. W.: Influences of recent particle formation on Southern Ocean aerosol variability and low cloud properties, J. Geophys. Res.-Atmos., 126, e2020JD033529, https://doi.org/10.1029/2020JD033529, 2021.
Möhler, O., Büttner, S., Linke, C., Schnaiter, M., Saathoff, H., Stetzer, O., Wagner, R., Krämer, M., Mangold, A., Ebert, V., and Schurath, U.: Effect of sulfuric acid coating on heterogeneous ice nucleation by soot aerosol particles, J. Geophys. Res.-Atmos., 110, 1–12, https://doi.org/10.1029/2004JD005169, 2005a.
Möhler, O., Linke, C., Saathoff, H., Schnaiter, M., Wagner, R., Mangold, A., Krämer, M., and Schurath, U.: Ice nucleation on flame soot aerosol of different organic carbon content, Meteor. Z., 14, 477–484, https://doi.org/10.1127/0941-2948/2005/0055, 2005b.
Murray, B. J., O'Sullivan, D., Atkinson, J. D., and Webb, M. E.: Ice nucleation by particles immersed in supercooled cloud droplets, Chem. Soc. Rev., 41, 6519–6554, https://doi.org/10.1039/c2cs35200a, 2012.
Myriokefalitakis, S., Vignati, E., Tsigaridis, K., Papadimas, C., Sciare, J., Mihalopoulos, N., Facchini, M. C., Rinaldi, M., Dentener, F. J., Ceburnis, D., Hatzianastasiou, N., O'Dowd, C. D., van Weele, M., and Kanakidou, M.: Global modeling of the oceanic source of organic aerosols, Adv. Meteorol., 2010, 1–16, https://doi.org/10.1155/2010/939171, 2010.
Niemand, M., Möhler, O., Vogel, B., Vogel, H., Hoose, C., Connolly, P., Klein, H., Bingemer, H., DeMott, P., Skrotzki, J., and Leisner, T.: A particle-surface-area-based parameterization of immersion freezing on desert dust particles, J. Atmos. Sci., 69, 3077–3092, https://doi.org/10/f4bkkr, 2012.
NOAA Air Resources Laboratory: Meteorology & Starting Location(s), NOAA [data set], https://www.ready.noaa.gov/hypub-bin/trajasrc.pl (last access: 16 January 2023), 2023.
O'Brien, R. E., Wang, B., Laskin, A., Riemer, N., West, M., Zhang, Q., Sun, Y., Yu, X., Alpert, P., Knopf, D. A., Gilles, M. K., and Moffet, R. C.: Chemical imaging of ambient aerosol particles: Observational constraints on mixing state parameterization, J. Geophys. Res.-Atmos., 120, 9591–9605, https://doi.org/10.1002/2015JD023480, 2015.
Office of Naval Research: Aerosol reanalysis data set, https://nrlgodae1.nrlmry.navy.mil/ftp/outgoing/nrl/NAAPS-REANALYSIS/2019/ (last access: 24 December 2022), 2022.
Palm, B. B., Peng, Q., Fredrickson, C. D., Lee, B. H., Garofalo, L. A., Pothier, M. A., Kreidenweis, S. M., Farmer, D. K., Pokhrel, R. P., Shen, Y., Murphy, S. M., Permar, W., Hu, L., Campos, T. L., Hall, S. R., Ullmann, K., Zhang, X., Flocke, F., Fischer, E. V., and Thornton, J. A.: Quantification of organic aerosol and brown carbon evolution in fresh wildfire plumes, P. Natl. Acad. Sci. USA, 117, 29469–29477, https://doi.org/10.1073/pnas.2012218117, 2020.
Park, J., Dall'Osto, M., Park, K., Gim, Y., Kang, H. J., Jang, E., Park, K.-T., Park, M., Yum, S. S., Jung, J., Lee, B. Y., and Yoon, Y. J.: Shipborne observations reveal contrasting Arctic marine, Arctic terrestrial and Pacific marine aerosol properties, Atmos. Chem. Phys., 20, 5573–5590, https://doi.org/10.5194/acp-20-5573-2020, 2020.
Park, J. Y., Lim, S., and Park, K.: Mixing state of submicrometer sea spray particles enriched by insoluble species in bubble-bursting experiments, J. Atmos. Ocean. Technol., 31, 93–104, https://doi.org/10.1175/JTECH-D-13-00086.1, 2014.
Prather, K. A., Bertram, T. H., Grassian, V. H., Deane, G. B., Stokes, M. D., DeMott, P. J., Aluwihare, L. I., Palenik, B. P., Azam, F., Seinfeld, J. H., Moffet, R. C., Molina, M. J., Cappa, C. D., Geiger, F. M., Roberts, G. C., Russell, L. M., Ault, A. P., Baltrusaitis, J., Collins, D. B., Corrigan, C. E., Cuadra-Rodriguez, L. A., Ebben, C. J., Forestieri, S. D., Guasco, T. L., Hersey, S. P., Kim, M. J., Lambert, W. F., Modini, R. L., Mui, W., Pedler, B. E., Ruppel, M. J., Ryder, O. S., Schoepp, N. G., Sullivan, R. C., and Zhao, D.: Bringing the ocean into the laboratory to probe the chemical complexity of sea spray aerosol, P. Natl. Acad. Sci. USA, 110, 7550–7555, https://doi.org/10.1073/pnas.1300262110, 2013.
Primm, K. M., Schill, G. P., Veghte, D. P., Freedman, M. A., and Tolbert, M. A.: Depositional ice nucleation on NX illite and mixtures of NX illite with organic acids, J. Atmos. Chem., 74, 55–69, https://doi.org/10.1007/s10874-016-9340-x, 2017.
Pruppacher, H. R. and Klett, J. D.: Microphysics of clouds and precipitation, Springer Netherlands, Dordrecht, 954 pp., https://doi.org/10.1007/978-0-306-48100-0, 2010.
Riemer, N. and West, M.: Quantifying aerosol mixing state with entropy and diversity measures, Atmos. Chem. Phys., 13, 11423–11439, https://doi.org/10.5194/acp-13-11423-2013, 2013.
Riemer, N., Ault, A. P., West, M., Craig, R. L., and Curtis, J. H.: Aerosol mixing state: measurements, modeling, and impacts, Rev. Geophys., 57, 187–249, https://doi.org/10.1029/2018RG000615, 2019.
Rolph, G., Stein, A., and Stunder, B.: Real-time environmental applications and display system: ready, Environ. Model Softw., 95, 210–228, https://doi.org/10.1016/j.envsoft.2017.06.025, 2017.
Rosinski, J., Nagamoto, C. T., and Zhou, M. Y.: Ice-forming nuclei over the East China Sea, Atmos. Res., 36, 95–105, https://doi.org/10.1016/0169-8095(94)00029-D, 1995.
Schill, G. P. and Tolbert, M. A.: Heterogeneous ice nucleation on simulated sea-spray aerosol using Raman microscopy, J. Phys. Chem. C, 118, 29234–29241, https://doi.org/10.1021/jp505379j, 2014.
Song, Y., Qiao, F., Liu, J., Shu, Q., Bao, Y., Wei, M., and Song, Z.: Effects of sea spray on large-scale climatic features over the Southern Ocean, J. Climate, 35, 4645–4663, https://doi.org/10.1175/JCLI-D-21-0608.1, 2022.
Stein, A. F., Draxler, R. R., Rolph, G. D., Stunder, B. J. B., Cohen, M. D., and Ngan, F.: NOAA's HYSPLIT atmospheric transport and dispersion modeling system, B. Am. Meteorol. Soc., 96, 2059–2077, https://doi.org/10.1175/BAMS-D-14-00110.1, 2015.
Su, B., Wang, T., Zhang, G., Liang, Y., Lv, C., Hu, Y., Li, L., Zhou, Z., Wang, X., and Bi, X.: A review of atmospheric aging of sea spray aerosols: Potential factors affecting chloride depletion, Atmos. Environ., 290, 1–24, https://doi.org/10.1016/j.atmosenv.2022.119365, 2022.
Tang, M., Cziczo, D. J., and Grassian, V. H.: Interactions of water with mineral dust aerosol: water adsorption, hygroscopicity, cloud condensation, and ice nucleation, Chem. Rev., 116, 4205–4259, https://doi.org/10.1021/acs.chemrev.5b00529, 2016.
Tang, M. J., Schuster, G., and Crowley, J. N.: Heterogeneous reaction of N2O5 with illite and Arizona test dust particles, Atmos. Chem. Phys., 14, 245–254, https://doi.org/10.5194/acp-14-245-2014, 2014.
Tomlin, J. M., Jankowski, K. A., Veghte, D. P., China, S., Wang, P., Fraund, M., Weis, J., Zheng, G., Wang, Y., Rivera-Adorno, F., Raveh-Rubin, S., Knopf, D. A., Wang, J., Gilles, M. K., Moffet, R. C., and Laskin, A.: Impact of dry intrusion events on the composition and mixing state of particles during the winter Aerosol and Cloud Experiment in the Eastern North Atlantic (ACE-ENA), Atmos. Chem. Phys., 21, 18123–18146, https://doi.org/10.5194/acp-21-18123-2021, 2021.
Tomlin, J. M., Weis, J., Veghte, D. P., China, S., Fraund, M., He, Q., Reicher, N., Li, C., Jankowski, K. A., Rivera-Adorno, F. A., Morales, A. C., Rudich, Y., Moffet, R. C., Gilles, M. K., and Laskin, A.: Chemical composition and morphological analysis of atmospheric particles from an intensive bonfire burning festival, Environ. Sci.-Atmos., 2, 616–633, https://doi.org/10.1039/D2EA00037G, 2022.
Vali, G.: Qauantitative evaluation of experimental results on the heterogeneous freezing nucleation of supercooled liquids, J. Atmos. Sci., 28, 402–409, https://doi.org/10.1175/1520-0469(1971)028<0402:QEOERA>2.0.CO;2, 1971.
Wagner, R., Kaufmann, J., Möhler, O., Saathoff, H., Schnaiter, M., Ullrich, R., and Leisner, T.: Heterogeneous ice nucleation ability of NaCl and sea salt aerosol particles at cirrus temperatures, J. Geophys. Res.-Atmos., 123, 2841–2860, https://doi.org/10.1002/2017JD027864, 2018.
Wang, B. and Knopf, D. A.: Heterogeneous ice nucleation on particles composed of humic-like substances impacted by O3, J. Geophys. Res.-Atmos., 116, 1–14, https://doi.org/10.1029/2010JD014964, 2011.
Wang, B., Laskin, A., Roedel, T., Gilles, M. K., Moffet, R. C., Tivanski, A. V., and Knopf, D. A.: Heterogeneous ice nucleation and water uptake by field-collected atmospheric particles below 273 K, J. Geophys. Res.-Atmos., 117, 1–15, https://doi.org/10.1029/2012JD017446, 2012a.
Wang, B., Lambe, A. T., Massoli, P., Onasch, T. B., Davidovits, P., Worsnop, D. R., and Knopf, D. A.: The deposition ice nucleation and immersion freezing potential of amorphous secondary organic aerosol: Pathways for ice and mixed-phase cloud formation, J. Geophys. Res.-Atmos., 117, 1–12, https://doi.org/10.1029/2012JD018063, 2012b.
Wang, B., O'Brien, R. E., Kelly, S. T., Shilling, J. E., Moffet, R. C., Gilles, M. K., and Laskin, A.: Reactivity of liquid and semisolid secondary organic carbon with chloride and nitrate in atmospheric aerosols, J. Phys. Chem. A, 119, 4498–4508, https://doi.org/10.1021/jp510336q, 2015.
Wang, B., Harder, T. H., Kelly, S. T., Piens, D. S., China, S., Kovarik, L., Keiluweit, M., Arey, B. W., Gilles, M. K., and Laskin, A.: Airborne soil organic particles generated by precipitation, Nat. Geosci., 9, 433–437, https://doi.org/10.1038/ngeo2705, 2016a.
Wang, B., Knopf, D. A., China, S., Arey, B. W., Harder, T. H., Gilles, M. K., and Laskin, A.: Direct observation of ice nucleation events on individual atmospheric particles, Phys. Chem. Chem. Phys., 18, 29721–29731, https://doi.org/10.1039/C6CP05253C, 2016b.
Welti, A., Lüönd, F., Stetzer, O., and Lohmann, U.: Influence of particle size on the ice nucleating ability of mineral dusts, Atmos. Chem. Phys., 9, 6705–6715, https://doi.org/10.5194/acp-9-6705-2009, 2009.
Welti, A., Müller, K., Fleming, Z. L., and Stratmann, F.: Concentration and variability of ice nuclei in the subtropical maritime boundary layer, Atmos. Chem. Phys., 18, 5307–5320, https://doi.org/10.5194/acp-18-5307-2018, 2018.
Welti, A., Bigg, E. K., DeMott, P. J., Gong, X., Hartmann, M., Harvey, M., Henning, S., Herenz, P., Hill, T. C. J., Hornblow, B., Leck, C., Löffler, M., McCluskey, C. S., Rauker, A. M., Schmale, J., Tatzelt, C., van Pinxteren, M., and Stratmann, F.: Ship-based measurements of ice nuclei concentrations over the Arctic, Atlantic, Pacific and Southern oceans, Atmos. Chem. Phys., 20, 15191–15206, https://doi.org/10.5194/acp-20-15191-2020, 2020.
Wilson, T. W., Ladino, L. A., Alpert, P. A., Breckels, M. N., Brooks, I. M., Browse, J., Burrows, S. M., Carslaw, K. S., Huffman, J. A., Judd, C., Kilthau, W. P., Mason, R. H., McFiggans, G., Miller, L. A., Nájera, J. J., Polishchuk, E., Rae, S., Schiller, C. L., Si, M., Temprado, J. V., Whale, T. F., Wong, J. P. S., Wurl, O., Yakobi-Hancock, J. D., Abbatt, J. P. D., Aller, J. Y., Bertram, A. K., Knopf, D. A., and Murray, B. J.: A marine biogenic source of atmospheric ice-nucleating particles, Nature, 525, 234–238, https://doi.org/10.1038/nature14986, 2015.
Xiao, H. S., Dong, J. L., Wang, L. Y., Zhao, L. J., Wang, F., and Zhang, Y. H.: Spatially resolved micro-Raman observation on the phase separation of effloresced sea salt droplets, Environ. Sci. Technol., 42, 8698–8702, https://doi.org/10.1021/es801181f, 2008.
Yakobi-Hancock, J. D., Ladino, L. A., and Abbatt, J. P. D.: Feldspar minerals as efficient deposition ice nuclei, Atmos. Chem. Phys., 13, 11175–11185, https://doi.org/10.5194/acp-13-11175-2013, 2013.
Yan, J., Jung, J., Zhang, M., Bianchi, F., Tham, Y. J., Xu, S., Lin, Q., Zhao, S., Li, L., and Chen, L.: Uptake selectivity of methanesulfonic acid (MSA) on fine particles over polynya regions of the Ross Sea, Antarctica, Atmos. Chem. Phys., 20, 3259–3271, https://doi.org/10.5194/acp-20-3259-2020, 2020.
Yao, Y., Curtis, J. H., Ching, J., Zheng, Z., and Riemer, N.: Quantifying the effects of mixing state on aerosol optical properties, Atmos. Chem. Phys., 22, 9265–9282, https://doi.org/10.5194/acp-22-9265-2022, 2022.
Zhang, M., Chen, L., Xu, G., Lin, Q., and Liang, M.: Linking phytoplankton activity in polynyas and sulfur aerosols over Zhongshan Station, East Antarctica, J. Atmos. Sci., 72, 4629–4642, https://doi.org/10.1175/JAS-D-15-0094.1, 2015.