the Creative Commons Attribution 4.0 License.
the Creative Commons Attribution 4.0 License.
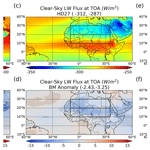
Modeling impacts of dust mineralogy on fast climate response
Qianqian Song
Paul Ginoux
María Gonçalves Ageitos
Ron L. Miller
Vincenzo Obiso
Carlos Pérez García-Pando
Mineralogical composition drives dust impacts on Earth's climate systems. However, most climate models still use homogeneous dust, without accounting for the temporal and spatial variation in mineralogy. To quantify the radiative impact of resolving dust mineralogy on Earth's climate, we implement and simulate the distribution of dust minerals (i.e., illite, kaolinite, smectite, hematite, calcite, feldspar, quartz, and gypsum) from Claquin et al. (1999) (C1999) and activate their interaction with radiation in the GFDL AM4.0 model. Resolving mineralogy reduces dust absorption compared to the homogeneous dust used in the standard GFDL AM4.0 model that assumes a globally uniform hematite volume content of 2.7 % (HD27). The reduction in dust absorption results in improved agreement with observation-based single-scattering albedo (SSA), radiative fluxes from CERES (the Clouds and the Earth's Radiant Energy System), and land surface temperature from the CRU (Climatic Research Unit) compared to the baseline HD27 model version. It also results in distinct radiative impacts on Earth's climate over North Africa. Over the 19-year (from 2001 to 2019) modeled period during JJA (June–July–August), the reduction in dust absorption in AM4.0 leads to a reduction of over 50 % in net downward radiation across the Sahara and approximately 20 % over the Sahel at the top of the atmosphere (TOA) compared to the baseline HD27 model version. The reduced dust absorption weakens the atmospheric warming effect of dust aerosols and leads to an alteration in land surface temperature, resulting in a decrease of 0.66 K over the Sahara and an increase of 0.7 K over the Sahel. The less warming in the atmosphere suppresses ascent and weakens the monsoon inflow from the Gulf of Guinea. This brings less moisture to the Sahel, which combined with decreased ascent induces a reduction of precipitation. To isolate the effect of reduced absorption compared to resolving spatial and temporal mineralogy, we carry out a simulation where the hematite volume content of homogeneous dust is reduced from 2.7 % to 0.9 % (HD09). The dust absorption (e.g., single-scattering albedo) of HD09 is comparable to that of the mineralogically speciated model on a global mean scale, albeit with a lower spatial variation that arises solely from particle size. Comparison of the two models indicates that the spatial inhomogeneity in dust absorption resulting from resolving mineralogy does not have significant impacts on Earth's radiation and climate, provided there is a similar level of dust absorption on a global mean scale before and after resolving dust mineralogy. However, uncertainties related to emission and distribution of minerals may blur the advantages of resolving minerals to study their impact on radiation, cloud properties, ocean biogeochemistry, air quality, and photochemistry. On the other hand, lumping together clay minerals (i.e., illite, kaolinite, and smectite), but excluding externally mixed hematite and gypsum, appears to provide both computational efficiency and relative accuracy. Nevertheless, for specific research, it may be necessary to fully resolve mineralogy to achieve accuracy.
- Article
(13679 KB) - Full-text XML
-
Supplement
(9921 KB) - BibTeX
- EndNote
Soil dust aerosols emitted from erodible land surfaces, hereafter referred to as dust, are the most abundant aerosol component in the atmosphere in terms of dry mass. Dust has significant impacts on the Earth's climate systems (atmosphere, ocean, cryosphere) due to its interaction with terrestrial and solar radiation (Sokolik and Toon, 1999), cloud microphysics (Guo et al., 2021), tropospheric chemistry (Bian and Zender, 2003; Paulot et al., 2016), and oceanic and terrestrial biogeochemistry (Mahowald, 2011; Evans et al., 2019; Dunne et al., 2020). In addition, dust particles deposited on snow and ice decrease surface reflectivity and accelerate snowmelt (Skiles et al., 2018; Réveillet et al., 2022). Dust can influence Earth's radiative energy budget through different pathways, including (1) directly by interacting with both solar and terrestrial radiation (i.e., the direct radiative effect, hereafter referred to as DRE), (2) by radiatively influencing the thermal dynamical structure of atmosphere and thereby clouds (i.e., semi-direct radiative effect), and (3) indirectly by altering cloud reflectivity (cloud albedo effect) and lifetime (cloud lifetime effect). Unfortunately, the quantitative estimate of dust DRE at the top of the atmosphere (TOA) is largely uncertain (Claquin et al., 1998; Miller et al., 2014; Kok et al., 2017; Song et al., 2022). A significant part of this uncertainty has been attributed to neglecting variations in dust mineralogical composition and its evolution during transport (Li et al., 2021).
The magnitude of dust impacts on the Earth's climate systems depends on its mineralogical composition, as has been shown in multiple studies. In the shortwave (SW), dust absorption depends on the iron oxide content. Sokolik and Toon (1999) suggested that a small quantity of iron oxides internally mixed with less absorptive minerals is able to reverse the sign of DRESW at TOA from negative (cooling effect) to positive (warming effect). Multiple studies have confirmed the importance of iron oxides to the dust DRESW (Balkanski et al., 2007; Li et al., 2021; Obiso et al., 2023). In the longwave (LW) spectrum, absorption and DRELW depend on the abundance of quartz, calcite, and clays in coarse and super-coarse modes (Di Biagio et al., 2017; Sokolik and Toon, 1999). As a result, resolving dust mineralogy allows better understanding the impact of dust DRE, such as the fast response of the land surface temperature, as opposed to the slow response of sea surface temperature that will not be studied here. This fast temperature response will affect precipitation and atmospheric circulation (Ming et al., 2010; Persad et al., 2014).
In addition, resolving dust mineralogy is also crucial for studying heterogeneous reactions of acid gases with dust aerosols. For example, the uptake of HNO3, , and N2O5 on dust particles is suggested to be limited by alkalinity that comprises calcium and magnesium carbonates (Song and Carmichael, 2001; Paulot et al., 2016). These reactions will modify the composition of dust particles and subsequently change their hygroscopicity, cloud condensation nucleation (CCN), and ice nucleation activities (Kelly et al., 2007), thereby further affecting precipitation (Rosenfeld et al., 2001). Moreover, heterogeneous reactions with mineral dust could significantly affect tropospheric photochemical oxidation cycles, causing up to 10 % reduction in O3 concentrations in dust source regions and nearby (Dentener et al., 1996). Among the different minerals, K-feldspar appears to dominate ice nucleation, despite being a minor component of aeolian dust (Atkinson et al., 2013; Harrison et al., 2019), although other minerals such as quartz may also contribute (Chatziparaschos et al., 2023). The key factor controlling the production and removal of pollutants as well as damage by acid rain is the pH of raindrops, which has been observed to increase due to its dependency on Ca-rich dust (Grider et al., 2023).
Despite the potential importance of resolving dust mineralogy in various respects, current climate models tend to use a fixed mineralogy without considering the temporal and spatial variations in dust mineralogical composition. To test the importance of resolving dust mineralogy for the fast climate response (e.g., surface temperature response over land, atmospheric circulation, and precipitation response) through its interactions with SW and LW radiation (i.e., through dust DRE), dust mineralogy has been implemented and simulated in the GFDL AM4.0 model (Zhao et al., 2018a, b), including its online interactions with radiation. Following the pioneering work of Claquin et al. (1999) (C1999), we consider the emission, transport, and interactions with radiation and deposition of eight minerals: illite, kaolinite, smectite, hematite, calcite, feldspar, quartz, and gypsum. Following the recent launch of the Earth Surface Mineral Dust Source Investigation (EMIT) instrument specifically designed to retrieve the global distribution of dust mineralogy over dust sources (Green et al., 2020), there have been coordinated efforts to represent dust mineralogy and investigate DRE of mineral-speciated dust in climate models, in particular in Li et al. (2021), Gonçalves Ageitos et al. (2023), and Obiso et al. (2023). However, to the best of our knowledge, there have been no studies investigating the fast climate impact of dust while accounting for its mineral speciation. Our work contributes to these efforts by incorporating dust mineralogy into the GFDL models, and it is distinguished by extending its investigation to the fast climate response of mineral-speciated dust. The impacts of dust mineralogy on other aspects, such as sea surface temperature and slow climate response, heterogeneous reactions, and ice nucleation ability, will be examined in future studies.
Section 2 provides the description of the GFDL AM4.0 model and dust mineralogy implementation. Section 3 describes our experimental design. In Sect. 4, we calculate mineral optical properties, activate the interaction of minerals with radiation in GFDL AM4.0, and compare modeled dust optical properties with observations. Section 5 presents the impacts of resolving dust mineralogy on Earth's radiation and climate with a focus on North Africa, as well as their evaluations. In Sect. 6, we investigate the influences of reducing the number of mineral tracers. Section 7 provides a summary of the study along with the main conclusions.
2.1 Model description
We conduct a series of experiments with GFDL AM4.0 (Zhao et al., 2018a, b) over the period 2001–2019. These experiments use the AMIP protocol, where sea surface temperature (SST) and sea ice are imposed based upon average monthly observations (see Gates, 1992, for details). Observed gridded SST and sea ice concentration boundary conditions are from the reconstructions of Taylor et al. (2000). Historical reconstructions of monthly solar spectral irradiances are from Matthes et al. (2017). For radiation calculations, global monthly mean concentrations of greenhouse gases (GHGs), including nitrous oxide (N2O), and ozone-depleting substances (ODSs, including CFC-11, CFC-12, CFC-113, and HCFC-22) are specified from Meinshausen et al. (2017). The solar irradiances and GHG databases are standard for CMIP6. Longwave (LW) scattering of aerosols is not accounted for in the model.
In AM4.0, dust emission is calculated interactively following the parameterization of Ginoux et al. (2001) with a threshold of wind erosion and global scaling factor of 3.5 m s−1 and 0.2 µg s2 m−5, respectively. Dust size is represented by five bins with diameter ranging from 0.2 to 20 µm (bin 1: 0.2–2, bin 2: 2–3.6, bin 3: 3.6–6, bin 4: 6–12, bin 5: 12–20 µm). The corresponding source fractions have been updated from 0.1, 0.225, 0.225, 0.225, and 0.225 to values of 0.04, 0.14, 0.19, 0.49, and 0.14 for the five bins. These updated source functions allocate a higher fraction to coarser size bins, following the suggested brittle fragmentation theory (BFT) as proposed by Kok (2011). Dust mineral composition in the standard AM4.0 is considered uniform, with no temporal and spatial variations; in other words, the dust refractive index (RI) is temporally and spatially homogeneous (the case referred to as homogeneous dust hereafter). The dust RI in the standard AM4.0 is taken from Balkanski et al. (2007), assuming a fixed hematite content of 2.7 % by volume (HD27), which was calculated for the internal mixture of hematite and five other minerals (calcite, quartz, illite, kaolinite, and montmorillonite) using the Maxwell–Garnett mixing rule (see details in Balkanski et al., 2007). The decision to fix the hematite content for dust particles at 2.7 % was made during the development of the previous GFDL Climate Model CM3 (Donner et al., 2011). This decision was prompted by the discovery that dust absorption was unrealistically high (by a factor 3) in CM2 (Delworth et al., 2006) compared to AERONET observations (Balkanski et al., 2007). In CM3, the conjunction of a sharp decrease in black carbon (strong aerosol absorber) with a new emission inventory and the switch to more scattering dust had a negative effect on precipitation bias and late 20th century warming (see Donner et al., 2011, for details). To mitigate this bias, the selection of 2.7 % hematite was adopted in CM3, as well as in the subsequent GFDL models. The control run conducted with the homogeneous dust in the standard AM4.0 model is labeled as HD27 as described in Table 2.
In addition, we conduct simulations assuming homogeneous dust with hematite content of 0.9 % by volume, with RI from Balkanski et al. (2007). Similar to HD27, this experiment, labeled as HD09 in Table 2, does not account for the temporal and spatial variations in dust mineralogy.
Table 1The list of minerals considered in this study and their importance to Earth's climate. Mineral-dependent mass densities are defined following Table 1 in Gonçalves Ageitos et al. (2023), in which the references of mineral densities are listed.
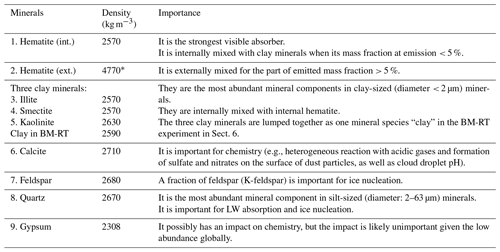
∗ We use the mean of hematite and goethite densities for hematite, as in Gonçalves Ageitos et al. (2023).
2.2 Dust mineralogy implementation
Claquin et al. (1999) (C1999) is the earliest study providing a soil mineralogy map oriented toward atmospheric and climate modeling. The soil map provides the mineral mass fractions present in the clay and silt size ranges for eight different minerals, namely illite, smectite, kaolinite, calcite, quartz, feldspars, gypsum, and hematite. In this study, we implement the eight minerals from the soil mineralogy map provided by C1999 in GFDL AM4.0 to resolve dust mineralogy. The soil map is based on soil analyses that are usually done after wet sieving, which disperse mineral aggregates into small particles. This dispersal is particularly relevant for the phyllosilicates, typically found in the form of aggregates in soils. They are detected in the atmosphere with higher proportions at coarser (silt) sizes than those reported in the soil maps (Perlwitz et al., 2015b; Perez Garcia-Pando et al., 2016). These recent studies also show that the brittle fragmentation theory (BFT; Kok, 2011) represents a practical framework to generate the emitted particle size distribution based on the dispersed soil PSD, which facilitates the utilization of soil mineralogy maps. In our simulations, we employ BFT to reconstruct the mineral aggregates emitted from the original undispersed soils, following the methods described in Gonçalves Ageitos et al. (2023). The mass densities of the eight minerals, along with a brief description of their importance to Earth's climate, are listed in Table 1. The density of minerals impacts their settling velocity, which is relevant to the removal of particles in the atmosphere. Goethite and hematite are the two major types of iron oxides present in soils. Goethite is less absorptive than hematite and is not resolved in C1999. So, iron oxides are represented by hematite in this study. Hematite has larger density than other minerals so that hematite deposits more quickly and is not able to be transported to remote regions when not aggregated or internally mixed with lighter clay minerals. Moreover, among the minerals considered here, hematite is the strongest absorber at ultraviolet (UV) and visible wavelengths, while it does not have noticeable absorption at infrared (IR) wavelengths compared to other minerals (Sokolik and Toon, 1999). As such, the correct representation of hematite content in dust aerosols is critical in improving the representation of dust interaction with SW radiation in climate models. All minerals are considered to be externally mixed, except for iron oxides. A large part of the emitted flux of iron oxides is considered to be internally mixed with other minerals, e.g., in the form of accretions in phyllosilicates, in line with observational evidence and previous modeling studies (Kandler et al., 2009; Perlwitz et al., 2015a; Zhang et al., 2015; Panta et al., 2023). As suggested by Gonçalves Ageitos et al. (2023), we define two different types of tracers for the iron oxides: one set of tracers carries the mass of the hematite that constitutes small accretions in clay minerals (i.e., internally mixed with clay minerals), which are allowed to be up to 5 % of the masses of their host minerals at emission (Perlwitz et al., 2015a; Gonçalves Ageitos et al., 2023). Given the low fractional mass of hematite compared to their host minerals, we assume that these accretions do not change the density of their host particles. These internally mixed accretions form the largest fraction of the emitted hematite. Another smaller set of tracers carries the mass of the remaining fraction of hematite, which is considered to be externally mixed with the other minerals, including the internal mixtures of hematite with clay.
In addition to the similar roles of clay minerals in carrying iron oxides, the optical properties of the three clay minerals are very similar, and the optical properties of their external mixture are found to be almost identical to their internal mixture (see Sect. S1 in the Supplement). This finding suggests the use of a single mineral species to represent all three clay minerals in their interaction with radiation to reduce computational cost. Therefore, the optical properties of one single mineral (clay433) are used to represent the optical properties of all three clay minerals. The clay433 represents a mixed mineral comprising three clay minerals: illite, kaolinite, and smectite, with mass fractions of 40 %, 30 %, and 30 %, respectively (see detailed descriptions in Sect. S1). This simplification streamlines the calculations of optical properties for internal mixtures of hematite and the three clay minerals (illite, kaolinite, and smectite), reducing it from an internal mixture of four minerals (hematite, illite, kaolinite, and smectite) to an internal mixture of two minerals (hematite and clay433).
The optical properties of the internal mixture of hematite and clay433 are calculated using three mixing rules: volume-weighted average (VOL-mixing), Maxwell–Garnett mixing rule (MG-mixing), and Bruggeman mixing rule (BM-mixing). Generally, VOL-mixing is used for a quasi-homogeneous mixture: that is, when the components have a similar refractive index. For cases involving a dominant homogeneous host with small inclusions of contrasting composition, MG-mixing is appropriate. BM-mixing is suitable for mixtures in which the inclusions virtually occupy the entire volume of the particle, and the host disappears. A detailed discussion regarding the three mixing rules and their applications can be found in Liu and Daum (2008) and Markel (2016). The appropriate selection of mixing rules is important for the determination of the optical properties of the mixtures. Therefore, we incorporate all three mixing rules in this study. These calculations are performed for various volume mixing fractions of hematite with respect to clay433 to construct a lookup table (LUT) for each mixing rule. The optical properties of each mineral as well as the internal mixtures of hematite and clay433 are calculated offline using Mie code with a spherical shape assumption. As all other minerals have similar SW absorption, internal or external mixing does not change their absorption properties. So, we assume all other minerals to be externally mixed. More details about optical properties of minerals will be discussed in Sect. 4.
Overall, we implement nine types of mineral tracers: seven non-hematite minerals along with distinguished internal and external hematite, as listed in Table 1. Each type is distributed across five size bins. As a result, 45 mineral tracers have been incorporated in AM4.0 to account for dust mineralogy.
2.3 AERONET dust SSA
The AERONET Version 3 Level 2.0 Almucantar inversion retrievals (Giles et al., 2019; Sinyuk et al., 2020) from 2000 to 2020 are screened for dust events following the methodology in Gonçalves Ageitos et al. (2023) and Obiso et al. (2023). This screening process aims to select dust-dominated events and filter out the AERONET scenes contaminated by other absorbing aerosols. The criteria that are applied to AERONET retrievals to screen dust events are as follows: (1) hourly retrievals from AERONET are considered to represent dust when the fine volume fraction is small (below 15 %), (2) the SSA increases from 440 to 675 nm (a feature that distinguishes dust from other species, see Dubovik et al., 2002), and (3) the mean of the imaginary index at red and near-infrared wavelengths (675, 870, and 1020 nm) is lower than 0.0042 (as higher values would indicate the presence of absorbing black and brown carbon, following Schuster et al., 2016). We calculate AERONET SSA in the visible by averaging AERONET-retrieved SSA at two visible wavelengths (0.44 and 0.67 µm) weighted by the solar spectrum following Eq. (2).
2.4 Laboratory dust SSA
The lab-measured dust SSA at 550 nm is obtained from Di Biagio et al. (2019) (DB-2019 hereafter), in which dust SSA was directly retrieved from scattering and absorption measurements. We acknowledge the limits of laboratory measurements, where the dust samples are not aerosols present in the atmosphere, but instead are reemitted in the lab from soil samples collected from various source regions. Consequently, the laboratory measurements in DB-2019 do not account for dust aerosols transported from other regions to the regions of interest. In addition, in contrast to the modeled dust diameter range of 0.2 to 20 µm, DB-2019 measures dust particles with a diameter ranging from 0.2 to 10 µm.
2.5 CERES data
To compare modeled fluxes at TOA with observations, we use the Clouds and the Earth's Radiant Energy System (CERES) Energy Balanced and Filled (EBAF) Edition-4.2 data (Loeb et al., 2018). The standard CERES level-3 products provide clear-sky fluxes by averaging all CERES footprints within a region that are completely free of clouds. Therefore, there are many missing regions in monthly mean clear-sky TOA flux maps because completely cloud-free conditions are not always observed at the CERES footprint scale (∼ 20 km at nadir). In contrast to the standard CERES level-3 products, the CERES_EBAF product infers clear-sky fluxes from clear portions of partly cloudy CERES footprints, thereby producing a clear-sky TOA flux climatology free of any missing regions (details in Loeb et al., 2018). Starting from CERES_EBAF_Ed4.1, the product also provides clear-sky flux estimates for the total region (i.e., the total CERES footprints) by combining CERES observations and radiative transfer calculations, which represents clear-sky flux with clouds removed from the entire atmospheric column of CERES footprints. These clear-sky fluxes for the total region are defined in a way that is more consistent with how clear-sky fluxes are represented in climate models (for details see the CERES_EBAF_Ed4.1 Data Quality Summary). In this study, the monthly mean TOA “Clear-Sky Flux Estimate for Total Region” variables in the CERES_EBAF_Ed4.2 product, the most recent version of the product, are used to compare with modeled monthly mean clear-sky flux at TOA. The comparisons allow us to examine the agreement of modeled clear-sky fluxes from different experiments with observations. The comparison results are shown in Sect. 5.1.
2.6 CRU TS data
The CRU TS (Climatic Research Unit gridded Time Series) provides a high-resolution (0.5° latitude × 0.5° longitude) climate dataset over land except Antarctica. The dataset is based on extensive networks of weather stations going back to 1901 (Harris et al., 2020). This dataset has been widely used in various research areas since its first release in 2000. The mean 2 m temperature (TMP) and precipitation rate (PRE) variables from CRU TS v4.07 are used to evaluate our model simulations. The results are shown in Sect. 5.2 and 5.3.
We conduct a total of six experiments using the GFDL AM4.0 model, with each experiment's description provided in Table 2. Two of these experiments serve as control runs in which dust aerosols are represented with temporally and spatially fixed composition in the model. The first control run, referred to as HD27, represents how dust aerosols are implemented in the standard GFDL AM4.0 model (Zhao et al., 2018a). The second control run is the HD09, in which dust is more scattering than that in the standard AM4.0 model (i.e., HD27) due to its reduced hematite volume fraction from 2.7 % to 0.9 %.
The other three experiments, namely VOL, MG, and BM, resolve dust mineralogy and activate their interaction with radiation. These three experiments incorporate 45 mineral tracers for nine types of mineral tracers distributed over five size bins as described in Sect. 2.2. Additionally, we conduct the BM-RT experiments, which consist of three sub-experiments: BM-LC, BM-LCRH, and BM-LCRHRG. These experiments aim to explore the potential of reducing mineral tracers, which can improve the model computational efficiency. The results are discussed in Sect. 6.
Each of the experiments ran for 19 years from 2001 to 2019. We consider the 19-year runs of the experiment to be a group of simulations containing 19 members of a 1-year simulation. The two control runs (i.e., HD27 and HD09), combined with the three mineral-resolved experiments (i.e., VOL, MG, and BM), form a total of six contrasting pairs. In this study, for each contrasting pair, we define the anomaly as the group mean difference (based on 19-year mean) between the mineral-resolved experiment and control run. An anomaly is considered statistically significant if the p value, determined by the Student's t test between the two contrasting groups of simulations, is smaller than 0.05.
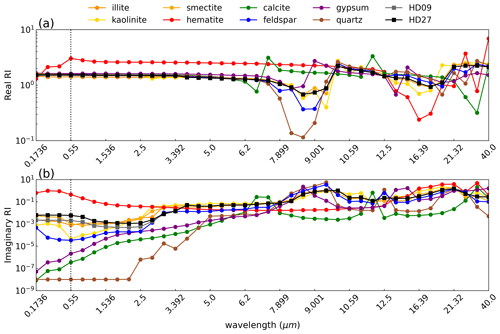
Figure 1Real part and imaginary part of complex refractive indices (RIs) of two homogeneous dust types (e.g., HD27 and HD09) and eight minerals (Scanza et al., 2015). The dotted lines represent the real part (a) and imaginary part (b) of dust or minerals for the corresponding wavelength. The black and grey dotted lines are for HD27 and HD09, respectively. The colorful dotted lines are for the eight minerals.
4.1 Dust optical properties
We use the refractive indices (RIs) of each mineral from Scanza et al. (2015) and the RIs of HD27 and HD09 from Balkanski et al. (2007) to calculate the LUT of optical properties. The spectral RIs of each mineral and homogeneous dust (e.g., HD27 and HD09) are shown in Fig. 1. The HD09 dust has a lower imaginary part of RI at 550 nm than HD27 dust, indicating its lower absorption in the visible band due to a reduced content of hematite.
Table 3The 19-year (2001–2019) averaged global dust emission and load, globally averaged visible band dust optical depth (), and single-scattering albedo () for each experiment in this study. We use each grid cell surface area as a weight for the global DAOD average. We use each grid cell surface area times DAOD in each grid cell as a weight for the global SSA average. In addition, we include the results from previous studies for the purpose of comparison. Note that the modeled DAOD and SSA in this study are averaged in the visible band (0.44–0.625 µm) of GFDL AM4.0, while they are averaged in the UV–Vis band (0.30–0.77 µm) of GISS ModelE2.1 in Obiso et al. (2023).
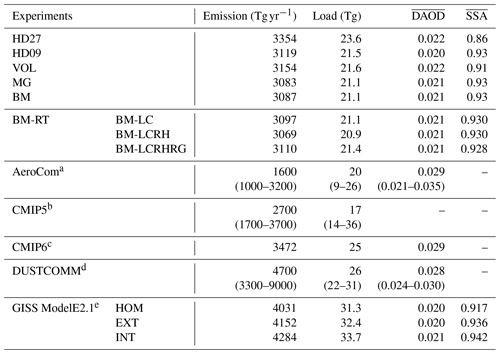
a Results are from AeroCom Phase I, which were taken from Table 3 in Huneeus et al. (2011), and the 1 standard error range was obtained by eliminating the two highest and lowest values. b Results are from Table 3 in Wu et al. (2020). c Results from Zhao et al. (2022). d Results are from Table 3 in Kok et al. (2021). e Results from Obiso et al. (2023).
After calculating the LUT of optical properties (details in Sect. 2.2), we incorporate the interaction of minerals with radiation into GFDL AM4.0. The modeled emission, load, deposition, and lifetime for each mineral are provided in Table S1 in the Supplement. Table 3 provides global total dust emission and load, globally averaged dust aerosol optical depth (DAOD), and SSA for each experiment listed in Table 2 and their comparisons with previous studies. DAOD and SSA from AM4.0 simulations are averaged in the visible band (0.44–0.625 µm) of GFDL AM4.0. Unless otherwise specified, DAOD and SSA in this study refer to the average in the visible band. Note that in our calculations, the domain-averaged DAOD is always weighted by the area of each grid cell. The domain-averaged SSA is always weighted by the area and DAOD of each grid cell. Additionally, the spectrally averaged DAOD and SSA are always weighted by the TOA solar radiation intensity at the corresponding wavelengths, peaking around 0.50 µm, as shown in Eqs. (1) and (2).
B(λ) describes the solar radiation energy intensity, which can be calculated by means of the Planck's function B(T,λ), using the temperature of the Sun (T = 5800 K).
The lowest SSA of HD27 in Table 3 suggests that HD27 dust, which has been used in the standard AM4.0 model, is the most absorptive among all experiments. The HD09 dust is much less absorptive, attributed to its smaller hematite content, as indicated by the lower imaginary part of RI in the visible range (Fig. 1). For the three mineral-resolved experiments, the lower global mean SSA () in VOL suggests that the VOL-mixing dust is more absorptive than MG-mixing and BM-mixing dust. This finding is consistent with previous studies that have suggested that VOL-mixing method, when applied to minerals to compute the bulk aerosol optical properties, may artificially enhance absorption relative to scattering and lead to a lower SSA for bulk dust aerosol (Zhang et al., 2015). We can see that the global mean SSA () of HD09 dust is comparable to the values obtained in cases where minerals are resolved (e.g., MG and BM). This implies that, from a global perspective, HD09 dust is as absorptive as mineral-resolved dust (e.g., MG and BM).
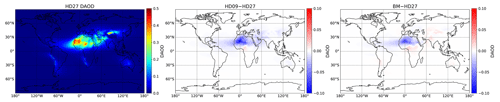
Figure 2The global distribution of dust optical depth (DAOD) for HD27 and the difference in DAOD between HD09 and BM compared to HD27. The global mean DAOD values () of each experiment are shown in Table 3.
In addition to the globally averaged dust properties listed in Table 3, we illustrate the distribution of global dust mass across five size bins (Fig. S3 in the Supplement) and the global distribution of DAOD (Fig. 2) for the three experiments: before (e.g., HM27 and HD09) and after (e.g., BM) resolving mineralogy. The global dust mass distribution across the five size bins remains largely unchanged across experiments. Besides the subtle difference (∼ 10 %) in global mean DAOD across the three experiments as listed in Table 3, the global distribution of DAOD responds differently in HD09 and BM. Compared to HD27, reducing hematite content to HD09 generally decreases DAOD, except over the Sahel region. In contrast, resolving mineralogy as in BM decreases DAOD over the Sahara region while increasing DAOD over the Sahel and Asia regions. The reduction in DAOD over the Sahara region further contributes to the decrease in dust absorption over the region, primarily attributed to the change in dust optical properties, such as the enhancement in dust SSA. The indistinct variation in DAOD across different experiments results from the feedback of dust interactions with radiation (Miller et al., 2004a, 2014; Pérez et al., 2006), which is influenced by the distinct scattering properties of dust aerosols in each experiment as shown in Table 3.
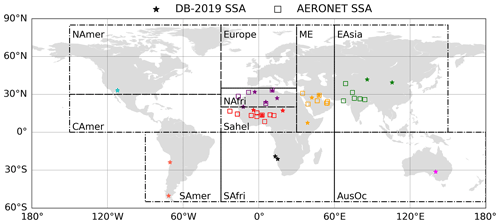
Figure 3Dust sample locations in Di Biagio et al. (2019) and AERONET stations selected by filtering dust events. AERONET stations for SSA retrievals are in North Africa (NAfri), the Sahel, the Middle East (ME), and East Asia (EAsia). Lab measurements by Di Biagio et al. (2017, 2019) expand dust sampling to include soils from North America (NAmer), South America (SAmer), southern Africa (SAfri), and Australia (AusOc).
4.2 Comparison of dust optical properties with observations
The iron oxide content of dust determines shortwave radiation absorption by dust: the higher the quantity of iron oxides, the lower the SSA. Following our calculations of dust optical properties in Sect. 4.1, we compare GFDL AM4.0 modeled dust SSA (averaged in the visible band 0.44–0.625 µm) against AERONET SSA retrievals (averaged at two visible wavelength: 0.44 and 0.67 µm) in Sect. 4.2.1 and laboratory measurements of SSA (at 0.55 µm) in Sect. 4.2.2. The modeled dust SSA is evaluated against observation-based results utilizing the following evaluation metrics: the mean SSA (mSSA) is calculated based on SSA for all locations displayed in Fig. 3. The standard deviation (σ), derived from SSA for all locations displayed in Fig. 3, is used as an indicator of dust SSA spatial variation. The normalized mean bias (nMB) and normalized root mean square error (nRMSE) are utilized to assess the mean bias and root mean square error, respectively, of modeled SSA in comparison to observed SSA. Definitions of nMB and nRMSE are provided in Sect. S3.
4.2.1 Comparison with AERONET retrievals
Figure 3 displays the AERONET stations selected by filtering dust events. The global distributions of modeled dust SSA and AERONET-retrieved SSA over the selected AERONET sites are shown in Fig. 4. There is a significant decrease in dust absorption from HD27 to HD09 globally due to the reduction in hematite content. HD09 and BM exhibit similar dust absorption on a global scale (e.g., the same global mean ), but the regional differences are evident. For instance, compared to HD09, resolving mineralogy (e.g., BM) decreases dust absorption over Iceland and Taklamakan regions, while it enhances dust absorption over the Southern Hemisphere, particularly over Australia. Additionally, there is a shift in dust absorption from the Sahara to the Sahel region after resolving mineralogy.
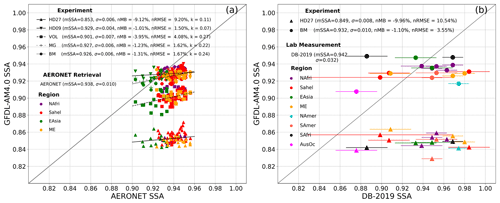
Figure 5GFDL AM4.0 modeled 19-year (2001–2019) averaged monthly dust SSA (average in 0.44–0.625 µm) versus (a) AERONET 21-year (2000–2020) averaged monthly SSA retrievals (average at two visible wavelengths: 0.44 and 0.67 µm) and (b) laboratory SSA measurements (at 0.55 µm) of dust particles with diameter ranging from 0.2 up to 10 µm obtained by Di Biagio et al. (2019) (DB-2019). The lab measurements were carried out in March 2015; horizontal error bars represent measurement uncertainties. Markers represent different experiments, and colors represent different regions. mSSA in the legend represents the mean SSA averaged over all locations indicated in Fig. 2 (squares for AERONET, stars for DB-2019). The standard deviation (σ), normalized mean bias (nMB), normalized root mean square error (nRMSE), and the slope of linear regression (k) are also indicated in the legend.
Figure 5a shows GFDL AM4.0 modeled 19-year (2001–2019) averaged dust SSA (average in 0.44–0.625 µm) versus AERONET SSA (average at 0.44 and 0.67 µm) retrievals. Compared to AERONET SSA retrievals, both HD27 and VOL overestimate dust absorption, as indicated by their relatively low SSA (Fig. 5a). HD27 dust is the most absorptive, indicating that the standard AM4.0 dust is overly absorptive. Dust SSA values in MG and BM are quite similar (i.e., mSSA: 0.926 versus 0.927) and show better agreement with AERONET measurements (nMB ≈ −1.3 % and nRMSE ≈ 1.6 %), and they exhibit stronger scattering (i.e., higher SSA) than HD27 and VOL. HD09 is almost as scattering as MG and BM, as indicated by the mSSA of 0.929 versus 0.926 and 0.927 in Fig. 5a, which is consistent with the global mean SSA () results shown in Table 3. Overall, both the fixed mineralogy dust HD09 and mineral-resolved MG and BM dust agree well with AERONET SSA retrievals, while HD27 and VOL are too absorptive. Therefore, we recommend using mixing ratios of MG or BM in calculating optical properties of internal mixtures of hematite and clay minerals. Unless otherwise specified, in the following part of the paper, we will refer to mineral-resolved experiments as MG or BM.
We further assess the SSA spatial variation (indicated by σ) for each experiment from AM4.0 by comparing it to observation-based results. SSA is generally determined by dust mineralogy, size, and shape. Various dust mineralogy leads to distinct dust SSA due to the different absorption properties of minerals (Fig. 1). Coarser dust generally tends to be more absorptive (i.e., has lower SSA) than finer dust when other factors are the same (Ryder et al., 2018). The spherical dust assumption tends to underestimate dust SSA (Huang et al., 2023). Given the uncertainty in dust shape, we assume dust particles to be spherical in this study, aligning with other model studies (e.g., Gliß et al., 2021). Consequently, in the mineral-resolved experiments of this study, namely VOL, MG, and BM, dust mineralogy and dust size are the two factors affecting the SSA spatial variation.
Conversely, in homogeneous dust experiments, specifically HD27 and HD09, SSA variation is solely attributed to variation in dust size, as dust mineralogy remains uniform globally. Interestingly, HD09 demonstrates smaller spatial variation (i.e., lower σ) in SSA compared to HD27 (Fig. 5a). To investigate the impact of dust size on SSA for different hematite content (e.g., HD27 and HD09), we perform a simple test in Sect. S4. Figures S4 and S5 illustrate that the variation of SSA due to the dust particle size being more pronounced with increasing absorption, i.e., from HD09 to HD27. This suggests that enhancement in dust scattering relative to dust absorption (i.e., an increase in SSA) mitigates the sensitivity of SSA to dust size.
The conclusions above provide an understanding of the SSA spatial variation (indicated by σ) before (i.e., HD27 and HD09) and after (i.e., VOL, MG, and BM) implementing dust mineralogy. The same σ (0.06) between HD27 and BM can be explained as follows: because mineral-resolved BM-mixing dust is overall more scattering than HD27 dust, resulting in a reduced sensitivity of SSA to size, the σ of SSA caused by dust size is reduced in BM relative to HD27. However, the incorporation of dust mineralogy in BM leads to an increase in σ. These contrasting effects compensate for each other, resulting in the same σ. In contrast, HD09 is overall as scattering as BM, as shown in Table 3 and Fig. 5a, suggesting a similar sensitivity of SSA to size. Therefore, the incorporation of dust mineralogy in BM results in a higher σ compared to HD09. Overall, while the enhancement in σ can be offset by the reduction in σ due to the reduced sensitivity to dust size, resolving dust mineralogy increases σ on its own, consequently enhancing the spatial variation in dust SSA.
It is worth mentioning that AERONET dust is quite scattering as shown in Fig. 5a; therefore, its SSA is less sensitive to dust size. The high σ (0.010) of AERONET SSA can be mainly due to spatial variations in dust mineral composition. Reducing dust hematite content (HD09) leads to better agreement in mean SSA with AERONET (i.e., more scattering dust) but results in very low σ (0.004), while implementing dust mineralogy (e.g., MG and BM) retains the agreement with AERONET in mean SSA and, at the same time, increases σ (0.006).
Besides the standard deviation (σ), the slopes (k) obtained from the statistics of modeled SSA versus AERONET SSA can also indicate the regional contrast of SSA. The regional contrast of AERONET SSA is well captured by the model when k is 1, underestimated when k is lower than 1, and overestimated when k is higher than 1. As such, the slopes in Fig. 5a show that the contrast in SSA from different regions (e.g., North Africa vs. East Asia) observed by AERONET is better captured by mineral-resolved experiments (e.g., VOL, MG, and BM with k ranging from 0.22 to 0.27) than homogeneous dust experiments (e.g., HD27 and HD09 with k ranging from 0.07 to 0.11). However, the modeled regional contrast of SSA in mineral-resolved experiments remains overly underestimated (i.e., k is much lower than 1). The significant underestimation of regional SSA contrast (k) and spatial variation (σ) in AM4.0, even after accounting for mineralogy, implies that something important is still missing in models. For instance, (1) the observed regional contrast in iron oxide content may be higher than that in the soil map used in this study, (2) the model may have underestimated regional contrasts in the dust aerosol size distributions and thus their contribution to SSA, and (3) there may be spatial variation of dust shape, which is not taken into account in the model.
4.2.2 Comparison with laboratory measurements
We further compare the GFDL AM4.0 modeled dust SSA (average in 0.44–0.625 µm) with DB-2019 laboratory measurements of SSA at 0.55 µm (Fig. 5b). Figure 3 shows the locations where dust samples were collected for the lab measurements. Considering that MG and BM are very similar in terms of dust absorption and agree the best with AERONET SSA, we select BM as a representative to compare with DB-2019 SSA. Moreover, to evaluate how dust absorption is represented in the standard AM4.0 relative to lab measurements, we also show the comparison of SSA between HD27 used in the standard AM4.0 and DB-2019 (Fig. 5b). Consistent with the comparison with AERONET, the comparison with lab measurements suggests that dust representation in the standard AM4.0 (i.e., HD27) is excessively absorptive. The smaller nMB and nRMSE values in BM suggest that SSA of BM agrees better with lab measurements.
Moreover, regarding spatial variation (σ), resolving dust mineralogy in BM increases σ from 0.008 for HD27 to 0.010 for BM, even though it is still lower than the σ (0.032) in DB-2019 lab measurements. Note that the variation for HD27 results from the high sensitivity of SSA to dust size due to its higher absorption (as discussed in Sect. S4). The inability to reproduce spatial variation observed in the lab measurements is likely attributed to two aspects. The first limitation is the fact that samples of DB-2019 are from soils rather than aeolian dust. Aeolian dust is expected to exhibit greater uniformity in mineralogy than soils because of the atmospheric mixing of dust emitted from various soil sources. The second one is associated with the under-representation of regional contrast in iron oxide content in our model. Observations from EMIT are therefore essential to constrain soil mineralogy in climate models.
Resolving dust mineralogy in climate models affects dust optical properties (as discussed in Sect. 4) and their spatial and temporal variability, thereby affecting their interactions with shortwave (SW) and longwave (LW) radiation. The variability in dust radiative interactions further induces the fast response of land surface temperature, circulation, and precipitation. To investigate the impacts of resolving dust mineralogy on climate, we need to compare modeled results in mineral-resolved experiments to the baseline homogeneous dust (i.e., non-resolved mineralogy) control run. As a result, the significance of the impacts depends on the selection of the baseline homogeneous dust experiment. In this section, we investigate the impacts of resolving dust mineralogy compared to two baseline homogeneous dust experiments. One baseline experiment is the homogeneous dust used in the standard GFDL AM4.0, in which dust mineralogy is assumed to be temporally and spatially uniform (i.e., non-resolved), with a volume fraction of 2.7 % hematite (HD27). The impacts of resolving dust mineralogy, compared to the baseline HD27, can be attributed to two factors: (1) the reduction in dust absorption after resolving dust mineralogy in comparison to HD27 as discussed in Sect. 4 and (2) spatial and temporal variations in dust scattering properties induced by inhomogeneity in dust mineralogy. The other baseline experiment is the homogeneous dust, with a volume fraction of 0.9 % hematite (HD09). Given the comparable global mean dust scattering properties (e.g., ) between the control run HD09 and mineral-resolved experiments (e.g., MG and BM), the impacts of resolving dust mineralogy, compared to baseline HD09, are solely attributed to the inhomogeneity in dust scattering properties induced by resolving dust mineralogy.
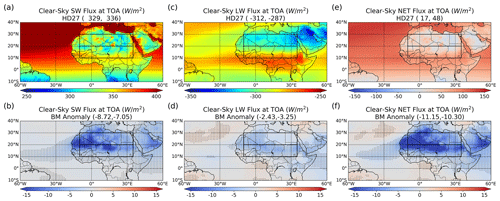
Figure 6Seasonal mean JJA climatology (2001–2019) clear-sky SW (a, b), LW (c, d), and net (e, f) radiative flux at TOA for the HD27 control run (a, c, e) and their anomalies resulting from resolving dust mineralogy in the Bruggeman mixing experiment (b, d, f). The downward direction is defined as positive. The dotted area denotes anomalies that are statistically significant. The two values in parentheses within the title of each figure are the domain average for the Sahara and Sahel regions.
5.1 Impacts on clear-sky radiative fluxes
We start our analysis by examining the impacts of resolving dust mineralogy on clear-sky radiative fluxes. By “clear sky”, we mean that our results do not consider the radiative effects of clouds. We use anomalies (ΔF) to evaluate the impacts of resolving mineralogy on clear-sky radiative fluxes, which is defined as , where represents the 19-year mean clear-sky upward radiative fluxes with resolved mineralogy, and represents the 19-year mean clear-sky upward radiative fluxes for homogeneous dust. Section S5 provides the clear-sky radiative fluxes anomalies at TOA and the surface (SFC) induced by resolving dust mineralogy over the global scale, we see much more significant anomalies over North Africa than other regions, which makes senses because that dust aerosol is the most dominant aerosol species in this area. The changes in dust aerosol optical properties have a greater potential to lead to significant impacts on radiation and climate over the region than in the others. Therefore, this section focuses on the North Africa region, where the Sahara, the largest dust source in the world, is located. The Sahara (20–30° N, 10° W–35° E) and the Sahel (10–20° N, 10° W–35° E) regions are studied separately. We specifically analyze the results for the June–July–August (JJA) season when dust loading is at its highest and the West African monsoon is the strongest.
5.1.1 Impacts on clear-sky radiative fluxes relative to HD27
Before comparing mineral-resolved experiments (e.g., BM) with the HD27 control run, the dust has been used in the standard GFDL AM4.0 to understand its impacts on clear-sky radiative fluxes relative to HD27. It is worth recapping that the effects of resolving mineralogy relative to HD27 can be attributed to two factors: the reduction in dust absorption and the variation in dust scattering properties induced by the mineralogical inhomogeneity.
The first row in Fig. 6 illustrates the modeled clear-sky shortwave (SW), longwave (LW), and net (NET: the combination of SW and LW) radiative flux at TOA from the HD27 control run. Relative to HD27, mineral-resolved dust (e.g., BM-mixing dust) generally reflects more SW radiation back to space and induces negative SW flux anomalies at TOA (Fig. 6a and b; positive: downward). Relative to the HD27 control run, the LW flux anomaly at TOA resulting from resolving mineralogy is less substantial compared to the SW flux anomaly (Fig. 6c and d). After combining SW and LW, resolving mineralogy turns out to induce a substantial decrease in NET flux at TOA, with a more than 50 % negative anomaly over the Sahara and around a 20 % negative anomaly over the Sahel (see values in parentheses in Fig. 6e and f). Therefore, less NET radiation reaches the Earth at TOA in the mineral-resolved dust cases due to their lower absorptivity.
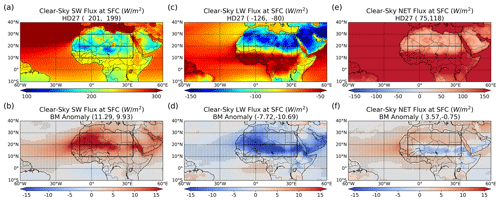
Figure 7As in Fig. 6, but for the surface.
At the surface (SFC) in Fig. 7, the enhanced scattering of mineral-resolved dust scatters more SW radiation toward Earth's surface, leading to a positive SW flux anomaly at SFC (positive: downward). In the LW, the cooling of the mineral-resolved dust layer, due to its low absorption, results in less LW radiation being emitted toward Earth's surface. This reduction in the downward LW emission outweighs the change in the upward LW emission from the Earth's surface, thereby causing a negative LW flux anomaly at SFC. The positive anomalies in SW radiation are approximately canceled out by the negative anomalies in LW radiation (Fig. 7). As a result, a similar amount of radiation reaches the Earth's surface in both the HD27 and mineral-resolved cases. Despite less NET radiation entering the Earth at TOA in mineral-resolved cases, the similar amount of NET radiation reaching the Earth's surface indicates that less NET radiation is absorbed in the atmosphere in mineral-resolved cases.
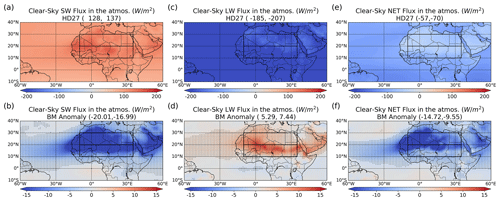
Figure 8As in Fig. 6, but for the radiative flux absorbed in the atmosphere.
In Fig. 8, the negative SW flux anomalies are partially offset by positive LW flux anomalies, resulting in negative NET flux anomalies in the atmosphere. These anomalies amount to approximately a 25 % reduction over the Sahara and 10 % reduction over the Sahel (see values in parentheses in Fig. 8b, d, and f).
5.1.2 Impacts on clear-sky radiative fluxes relative to HD09
Before comparing mineral-resolved experiments with the HD09 control run, where the homogeneous dust is as absorptive as mineral-resolved dust (e.g., MG and BM) from a global perspective, to understand its impacts on clear-sky radiative fluxes relative to HD09, it is worth recapping that the effects of resolving mineralogy relative to HD09 are primarily attributed to the variation in dust scattering properties induced by the mineralogical inhomogeneity.
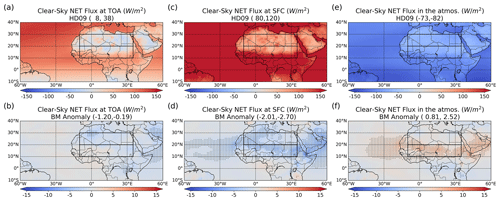
Figure 9As in the Fig. 6, but for the HD09 control run. In addition, SW and LW flux anomalies are not shown here. Clear-sky net fluxes at TOA (a, b), at the surface (c, d), and in the atmosphere (e, f) are shown in this figure.
Table 4Comparison of modeled clear-sky SW upward (SWup, first row), LW upward (LWup, second row), and NET downward (NETdn, third row) fluxes at TOA with CERES observation-based results over 2001–2019 JJA. The first column shows the clear-sky flux estimates at TOA from the CERES_EBAF_Ed4.2 product, which represents clear-sky flux with clouds removed from the atmospheric column. The following columns show the difference of modeled clear-sky flux at TOA in HD27 (second column), HD09 (third column), and BM (fourth column) experiments from CERES observations. The two values in parentheses represent the domain average for the Sahara and Sahel regions as indicated in the figures in Sect. 5.1.2. Specifically, the first column (CERES) is the domain-averaged flux, while the second (HD27–CERES), third (HD09–CERES), and fourth (BM–CERES) columns are the domain-averaged flux differences between model and CERES observation-based results.

Figure 9 shows the clear-sky fluxes anomalies with respect to HD09 over North Africa, and the anomalies over the global scale are shown in Figs. S7, S9, S11, and S13.
In contrast to the anomalies with respect to the HD27 control run, resolving dust mineralogy does not cause substantial anomalies (< 5 %) in clear-sky fluxes with respect to the HD09 control run. This can be attributed to their similarity in dust scattering properties from a global mean perspective, particularly SSA as shown in Fig. 5. The comparable effects of HD09 and mineral-resolved dust on radiation suggest that resolving dust mineralogy does not have significant impacts on clear-sky fluxes when homogeneous dust is as scattering as mineral-resolved dust aerosols on a global scale. However, the equivalence between HD09 and mineral-resolved dust in terms of their interactions with radiation may be related to the three limitations in the current model simulations. (1) Soil mineralogy: the limited soil mineralogy database fails to adequately capture the regional variation of iron content (or SSA) within the region. (2) Dust emission based on Ginoux et al. (2001) uses a continuous function of topography, which does not take into account geomorphological characteristics of the surface to differentiate soil properties of dust sources as done by others (Zender et al., 2003; Bullard et al., 2011). (3) Dust transport: excessive numerical diffusion may occur when solving the advection equation (Ginoux, 2003). Given all those limitations of our model simulations, this finding may differ with improved representation of dust sources and transport. Such improvement may come from a spaceborne soil mineralogy dataset (e.g., EMIT) that may accurately capture the regional contrasts in iron oxide content.
5.1.3 Compare clear-sky radiative fluxes with ceres observations
Furthermore, we conduct a comparison of modeled SW upward, LW upward, and NET downward flux at TOA with observation-based results from the CERES_EBAF_Ed4.2 product (see Table 4). The differences between the modeled flux and CERES observations are listed in parentheses within the title of each figure in Table 4. Compared to HD27, the more scattering HD09 and mineral-resolved BM achieve much better agreement with CERES observations in clear-sky flux (i.e., SWup, LWup, and NETdn) at TOA. This is evident in the smaller values of HD09–CERES (e.g., NETdn: 1.6 for the Sahara and 2.4 for the Sahel) and BM–CERES (e.g., NETdn: 0.4 for the Sahara and 2.1 for the Sahel) compared to HD07–CERES (e.g., NETdn: 11.3 for the Sahara and 12.4 for the Sahel), as shown by the values in parentheses in Table 4. Between HD09 and BM, BM tends to agree slightly better with CERES.
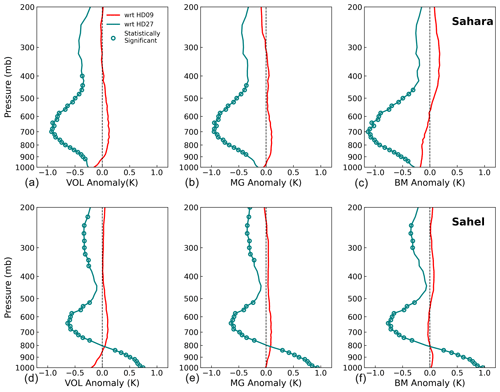
Figure 10Vertical profile of temperature anomalies induced by resolving dust mineralogy for the Sahara (a–c) and the Sahel (d–f) regions in the three mineral-resolved experiments (i.e., VOL, MG, BM). Green lines represent temperature anomalies with respect to the HD27 control run. Red lines are temperature anomalies with respect to the HD09 control run. The circles represent statistically significant temperature anomalies.
5.2 Impacts on land temperature
Here we explore the impacts on the temperature vertical profile and near-land surface temperature relative to HD27 and HD09, respectively. Compared to the HD27 control run, lower absorption of radiation in the atmosphere by mineral-resolved dust aerosols results in statistically significant negative temperature anomalies in the atmosphere ranging from 800 up to 500 mb where dust aerosols are mainly located (Fig. 10). In contrast, there is no statistically significant temperature anomaly for mineral-resolved dust cases compared to HD09, as illustrated by the red curves in Fig. 10. This finding aligns with the insubstantial anomalies (< 5 %) in clear-sky NET radiative fluxes discussed in Sect. 5.1.2. In the subsequent part of the section, we will delve into comparing a mineral-resolved experiment (using BM as an example) with the HD27 control run. This comparison will help us further understand the impact of dust aerosols with distinct absorption on land temperature.
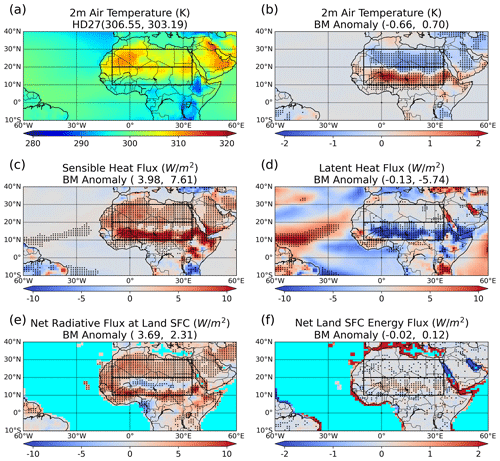
Figure 11Air temperature at 2 m from the HD27 control run (a), anomaly (b) induced by implementing Bruggeman mixing minerals in the BM experiment, surface sensible heat flux (c), latent heat flux (d), net radiative flux (e), and net energy flux (f) anomalies between BM and HD27. Upward flux is positive in (c) and (d), while downward flux is positive in (e) and (f). Net energy flux (f) is the subtraction of (c), (d), and downward ground flux from (e). Note that ground flux is not shown in the figure considering its relatively small magnitude, but it is included in the land surface net energy flux calculations in panel (f). The dotted area denotes anomalies that are statistically significant. The two values in parentheses within the title of each figure are domain averages for the Sahara and Sahel regions.
Figure 11a shows air temperature at 2 m from the HD27 control run over North Africa. Near the land surface, more scattering mineral-resolved dust induces a temperature decrease (i.e., negative temperature anomaly −0.66 K) over the Sahara and a temperature increase (i.e., positive temperature anomaly 0.70 K) over the Sahel as shown Fig. 11b. To understand this phenomenon, we further analyze the surface energy budget in Fig. 11c–f.
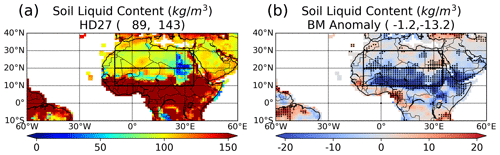
Figure 12Soil liquid content in the HD27 control run (a) and anomaly resulting from implementing Bruggeman mixing minerals in the BM experiment (b). The two values in parentheses within the title of each figure are domain averages for the Sahara and Sahel regions.
Radiative flux perturbation over land is quickly equilibrated by balancing surface radiative fluxes with sensible heat flux, latent heat flux, and ground flux (i.e., downward heat flux into the ground), which results in nearly zero net energy flux at the land surface as shown in Fig. 11f. Specifically, the radiative flux anomaly comprises two contributions: one is the instantaneous radiative forcing (IRF) caused by the change in dust mineralogy in the atmosphere, and the other one is the associated radiative feedbacks. For simplicity, we will not partition the radiative flux anomaly in our discussion here. Over the Sahel region, the positive net radiative flux anomaly at the land surface is balanced out by the increased sensible heat flux and the decreased latent heat flux as well as ground flux. Note that the ground flux is generally small in magnitude and not shown in Fig. 11, but we include it in calculating net surface energy flux in Fig. 11f. The decrease in latent heat flux over the Sahel in the BM case (Fig. 11d) is due to the depletion of soil moisture (and therefore evaporation) in the region as shown in Fig. 12. The depletion of soil moisture is caused by the decrease in moisture carried by onshore winds over the Sahel and the decrease in precipitation over the same region, as discussed in Sect. 5.3. Therefore, a large enhancement of sensible heat flux (∼ 7.6 W m−2) is needed (Fig. 11c) not only to compensate for the depletion in latent heat flux (∼ 5.7 W m−2 in d), but also to balance out the increased net radiative flux (∼ 2.3 W m−2 in Fig. 11e). As a result, higher land surface temperature with an anomaly around 0.7 K is needed in the region to achieve the required sensible heat flux enhancement.
Table 5The 19-year (2001–2019) JJA mean 2 m air temperature (T2 m, unit: K) and its standard deviation over the 19 years from CRU observations and modeled experiments over the Sahara and Sahel regions. The “Contrast” row indicates the T2 m regional contrast between the Sahara and the Sahel.
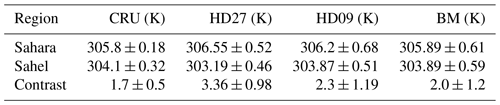
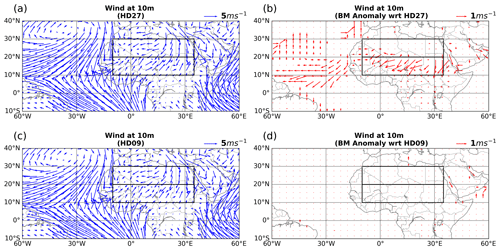
Figure 13Surface wind at 10 m from HD27 (a) and HD09 (c) control runs and their anomalies (b, d) resulting from implementing Bruggeman mixing minerals in the BM experiment. Statistically significant wind anomalies are highlighted by red arrows.
Over the Sahara region, latent heat flux does not change from the HD27 case to BM case; therefore, the increased net radiative flux (∼ 3.69 W m−2) in BM compared to HD27 is mainly balanced out by the enhanced sensible heat flux (∼ 3.98 W m−2), which requires a larger temperature gradient between the surface and atmosphere. However, there is a very strong negative temperature anomaly (around −1 K) in the atmosphere near 700 hPa due to less dust absorption in BM as we discuss in Fig. 10. The strong negative temperature anomaly in the lower atmosphere effectively increases the vertical temperature gradient. As such, it is not necessary for the land surface temperature to increase; in fact, it may need to decrease by approximately 0.66 K to achieve the desired enhancement in sensible heat flux and reach equilibrium.
Additionally, to assess the effectiveness of various dust scattering properties (e.g., HD27, HD09, and BM) in matching observations of near-surface temperature, we compare the modeled near-surface temperature (T2 m) with CRU TS observations, which is described in Sect. 2.6, over the Sahara and Sahel regions (Table 5). Considering the relatively large inter-model spread of regional surface air temperature, we compare the Sahara–Sahel regional contrast in surface air temperature to the CRU rather than comparing their absolute values. Table 5 shows that HD09 and BM improve the agreement with CRU in Sahara–Sahel temperature contrast compared to HD27, and BM exhibits the closest agreement with CRU.
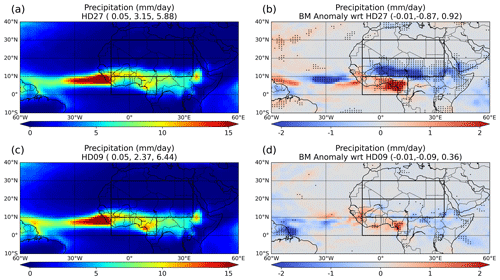
Figure 14The 19-year (2001–2019) JJA mean precipitation from HD27 (a) and HD09 (c) control runs and anomalies resulting from implementing Bruggeman mixing minerals with respect to HD27 (b) and with respect to HD09 (d). The three values in the parenthesis are domain-averaged values for the Sahara, Sahel, and GC regions.
5.3 Impacts on winds and precipitation
To understand the fast circulation and hydrological response resulting from resolving dust mineralogy, we examine surface wind speed anomalies (Fig. 13) and precipitation anomalies (Fig. 14) induced by mineral-resolved dust. We compare mineral-resolved experiments (using BM as an example) with HD27 and HD09, respectively, to investigate the effects of resolving dust mineralogy.
Global precipitation is higher by 0.017 mm d−1 for the BM mineral-speciated case compared to the HD27 experiment. This is consistent with the lower SW absorption in the former, given the global compensation between latent heating associated with precipitation and net radiative cooling (e.g., Allen and Ingram, 2002; Samset, 2022). (Global radiative cooling is also compensated for, although to a smaller extent, by the sensible heat flux.)
However, within the Sahel, precipitation for BM is reduced compared to HD27 (Fig. 14b), with weaker onshore flow during the summer monsoon (Fig. 13b), displacing West African precipitation toward the Guinea coast. This reduction is consistent with several previous calculations of the fast response calculated with fixed SST (e.g., Stephens et al., 2004; Miller et al., 2004b; Lau et al., 2009; Jin et al., 2016; Jordan et al., 2018).
Dust absorbs radiation and redistributes heating from the surface to within the dust layer (Miller and Tegen, 1999; Strong et al., 2015). The heating of the air warms the lower to middle troposphere, thereby enhancing upward motion. The rising warm air spawns a large-scale onshore flow, carrying the low-level moist air from the Atlantic to the Sahel, thus enhancing precipitation over this region (Balkanski et al., 2021). The more scattering mineral-resolved BM and MG dust absorbs less radiation and causes less warming of the atmosphere (Sect. 4.1), reducing adiabatic cooling through ascent and Sahel precipitation. The suppressed ascent in BM compared to HD27 is associated with a reduction in both the wind divergence aloft and convergence at the surface (Fig. 13b). The reduction in convergence results in northeast wind anomalies at 10 m over the Sahel (Fig. 13b), which are opposite in direction to the southwest onshore winds of the West African monsoon (Fig. 13a). The inhibition of onshore winds, bringing less moisture to the Sahel, is consistent with the reduction of ascent and precipitation over this region (Fig. 14a and b).
Besides the Sahel, there is a statistically significant positive anomaly (0.92 mm d−1) of precipitation over the region to the south of the Sahel in BM relative to HD27 (Fig. 14b). We will call this region the Guinea coast (GC: 0–10° N, 10° W–15° E). One possible reason for the increase in precipitation over the GC is that the region is located to the south of the Saharan dust layer, where the suppression of ascent over the Sahel in BM suppresses the subsidence in the GC region and, therefore, enhances the precipitation (Guo et al., 2021). Alternatively, the moist onshore flow that is weakened in BM is subject to greater dilution by the dry desert air, resulting in reduced moist static energy and buoyancy, limiting convection to the coastal region.
These changes in precipitation have non-negligible effects on soil moisture content in North Africa due to its moisture-starved environment. The decrease in precipitation over the Sahel in BM leads to a reduction in soil moisture content. Conversely, the increase in precipitation over the GC leads to increases in soil moisture (Fig. 12). The change in soil moisture content further affects the partitioning of surface energy fluxes and the efficiency of the latent heat flux, thereby affecting land surface temperature, as illustrated by Fig. 11.
Table 6Comparison of modeled precipitation rate (PRE, unit: mm d−1) with observations from the CRU TS dataset over 2001–2019 JJA. The CRU column represents the 19-year (2001–2019) JJA mean PRE over the region as well as the 19-year standard deviation (SD). The HD27–CRU column shows the 19-year mean PRE difference between the HD27 control run and CRU observations, along with the corresponding SD of this 19-year difference. Similar for HD09–CRU and BM–CRU.
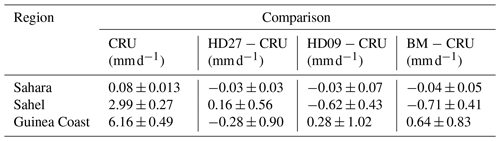
So far, we have been focusing on discussing the impacts of resolving dust mineralogy on winds and precipitation relative to the HD27 control run. The large discrepancy in optical properties between HD27 and mineral-resolved dust allows us to better understand how distinct dust absorption impacts our climate through its distinct radiative effects.
As discussed in Sect. 4.1, HD09 dust is nearly as scattering as mineral-resolved dust but exhibits smaller regional variability. Section 5.1.2 shows that resolving dust mineralogy does not lead to statistically significant anomalies of radiation relative to HD09. Consistently, there are no further statistically significant impacts on winds (Fig. 13c and d) and precipitation (Fig. 13c and d).
To investigate the effectiveness of various dust scattering properties (e.g., HD27, HD09, BM) in matching observations of precipitation rate, we compare the modeled precipitation with CRU TS observations over the Sahara, Sahel, and GC regions (Table 6). The greater difference between HD09, BM, and CRU (i.e., HD09–CRU and BM–CRU) indicates that more scattering HD09 and BM lead to a larger discrepancy between the modeled precipitation and CRU observations. In contrast, Balkanski et al. (2021) describe the same balance of increased dust absorption and Sahel precipitation but find improved agreement with Global Precipitation Climatology Project (GPCP) data by assuming homogeneous dust containing 3 % iron oxides by volume. Contrasts between that study and ours result from differences between the GPCP and CRU datasets, contrasts in dust absorption (related to contrasts in the dust size distribution or assumed index of refraction), non-dust model biases in precipitation, or differences between the slow response computed by Balkanski et al. (2021) and the fast response that we calculate. The fast and slow responses even exhibit differences in the sign of the calculated precipitation anomaly within some regions of the WAM (Miller and Tegen, 1998; Jordan et al., 2018).
Thus far in this study, we have been using 45 mineral tracers in mineral-resolved experiments (i.e., VOL, MG, and BM). However, it is important to investigate the potential of reducing the number of mineral tracers in climate models to lower computational costs. In this section, we take BM as a reference for providing the best comparisons with CRU temperature and CERES flux observations and conduct an experiment named BM-RT to assess the possibility of reducing mineral tracers in BM. The BM-RT experiment consists of three sub-experiments, namely, BM-LC, BM-LCRH, and BM-LCRHRG. In each of the three sub-experiments, the number of mineral tracers is progressively reduced, allowing for an examination of the relative impacts of different minerals on climate compared to the reference BM.
As discussed in Sect. 4.1, the three clay minerals (i.e., illite, kaolinite, smectite) exhibit similar optical properties and perform similar functions in climate by hosting hematite. Hence, they can be combined in their interaction with radiation without significant impacts on climate. In addition, by lumping the three clay minerals together, the number of mineral tracers can be reduced from 45 in BM (9 types of minerals × 5 size bins) to 35 (7 types of minerals × 5 size bins). Therefore, in the first sub-experiment BM-LC (where “LC” represents “lump clay minerals”), we lump together the three clay minerals as one mineral species “clay433”.
Based upon the C1999 soil mineral composition that we use, externally mixed hematite is mainly concentrated over the Sahel region (Ginoux et al., 2024) and cannot be transported to remote regions due to its high mass density. Obiso et al. (2023) show that visible extinction due to externally mixed hematite is negligible compared to other mineral components including hematite internally mixed with other minerals. Thus, we further remove external hematite tracers in the second sub-experiment BM-LCRH (where “RH” indicates “remove externally mixed hematite”). The mass fraction of external hematite is combined with internal hematite to ensure that the total mineral fraction at emission remains equal to 1. In this sub-experiment, the number of mineral tracers is reduced from 35 in BM-LC to 30 in BM-LCRH.
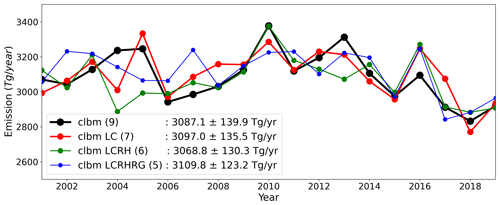
Figure 15Time series of total dust mineral emission from 2001 to 2019 before and after reducing the number of mineral tracers. The legend displays the following information. “Experiment name (number of mineral tracers): 19-year averaged total mineral emission ± 19-year standard deviation of total mineral emission”.
Since there are no known specific impacts of gypsum on climate, we conducted the third sub-experiment, BM-LCRHRG (“RG” indicates “remove gypsum”), where gypsum was removed. The mass fraction previously attributed to gypsum at emission, which is very low at the global scale, was proportionally redistributed among all other minerals. The number of mineral tracers is finally reduced from 30 in BM-LCRH to 25 in BM-LCRHRG.
We analyze the 19-year (2001–2019) time series of total dust mineral emission before and after reducing mineral tracers in Fig. 15. We observe subtle differences in total mineral emission between experiments, which arises from the feedback of mineral radiative interactions. However, these differences are numerically small, and the Student's t test suggests that the time series of the four experiments are not statistically different. Additionally, the globally averaged DAOD and SSA of each sub-experiment remain highly similar to those of the reference experiment BM, as listed in Table 3.
Given the highly similar optical properties of minerals before and after reducing mineral tracers, we further investigate their impact on climate. Firstly, we examine the clear-sky flux anomaly of each of the three sub-experiments at TOA and surface relative to the reference experiment BM, as shown in Fig. S14 for TOA and Fig. S15 for the surface. We only observe a few statistically significant (p value < 0.05) anomalies over the North Africa, suggesting that the reduction of mineral tracers in the three sub-experiments has a weak impact on radiation.
Furthermore, we investigate the anomaly in temperature profile, surface winds, and precipitation of each of the three sub-experiments relative to the reference experiment BM. The results are presented in Figs. S16–S18. No statistically significant (p value < 0.05) temperature anomalies (Fig. S16) and surface wind anomalies (Fig. S17) are observed. Only a few statistically significant anomalies in precipitation are observed in Fig. S18. These results suggest that the reduction of mineral tracers in the three sub-experiments has a weak impact on climate.
The results from the BM-RT experiment suggest combining clay minerals and excluding the externally mixed hematite and gypsum to simulate dust impact on radiation. This does not preclude a similar conclusion for other impacts of dust on the Earth's climate systems. The removal of mineral tracers reduces the number of mineral tracers from 45 to 25, effectively lowering computational costs without causing statistically significant impacts on simulating climate.
We simulate the distribution of dust mineralogy (i.e., illite, kaolinite, smectite, hematite, calcite, feldspar, quartz, and gypsum) and activate its interaction with radiation in the GFDL AM4.0 model. Our investigation focuses on the radiative impacts of resolving dust mineralogy on Earth's atmosphere and its fast response of land temperature, surface winds, and precipitation.
We set up two baseline homogeneous dust control runs: HD27 and HD09, in which dust mineralogy is considered to be temporally and spatially uniform, the former following the standard configuration for the dust optical properties in GFDL AM4.0 and the latter including a more scattering dust. Three experiments with resolved mineralogy are also conducted, VOL, MG, and BG, using three different mixing rules for the internal mixture between hematite and clay minerals (i.e., volume-weighted mean, Maxwell–Garnett, and Bruggeman). The comparison of dust absorption properties (e.g., SSA) with observation-based results suggests that the homogeneous dust used in the standard GFDL AM4.0 (i.e., HD27) is overly absorptive and that the Maxwell–Garnett and Bruggeman mixing rules are more appropriate than the volume-weighted mixing rule in calculating optical properties of internal mixtures of hematite and clays. Compared to HD27, the homogeneous dust with reduced hematite content (HD09) and mineral-resolved dust (i.e., MG and BM) exhibit much better agreement with AERONET retrievals and laboratory measurements in terms of dust absorption properties (i.e., SSA). Additionally, resolving dust mineralogy enhances regional variability in dust SSA compared to homogenous dust and further improves the agreement with AERONET, even though it remains lower than the observed variability.
The two homogeneous dust control runs, HD27 and HD09, with distinct dust absorption properties, allow us to investigate the impact of dust mineralogy on Earth's radiation and fast climate response relative to distinct baseline homogeneous dust. In comparison to HD27, resolving mineralogy reduces dust absorption. During JJA, the reduced dust absorption results in a reduction of over 50 % in NET downward radiation across the Sahara and approximately 20 % over the Sahel at TOA. Additionally, there is a reduction of around 25 % in the atmospheric absorption of radiation over the Sahara and around 10 % over the Sahel in the atmosphere. The reduced surface absorption of radiation by mineral-resolved dust leads to a temperature decrease of 0.66 K at the land surface across the Sahara and an increase of 0.7 K over the Sahel. The reduced NET downward radiation at TOA, attributed to the less absorption of radiation by mineral-resolved dust, suppresses ascent and weakens the monsoon inflow from the Gulf of Guinea. This brings less moisture to the Sahel, which combined with decreased ascent induces a reduction of precipitation. On the other hand, compared to HD09, resolving dust mineralogy results in dust absorption comparable to that of HD09 on a global scale. However, when resolving mineralogy, there is an increase in spatial variation of dust absorption. Additionally, we observe a noticeable change in the global distribution of dust absorption, with more dust absorption distributed in the Southern Hemisphere and lower dust absorption over the Iceland and Taklamakan regions. Nevertheless, the higher spatial variation in dust absorption does not lead to statistically significant changes in any of the climate aspects mentioned above. The models with reduced absorption (HD09 and fully resolved mineralogy) improve the comparison with observations of CERES fluxes and CRU land surface temperature. We see slightly better agreement with observations for fully resolved mineralogy than HD09; however, it is not statistically significant. As such, when using fixed mineralogical composition, we recommend using a 0.9 % hematite content in volume, which represents the lowest of the three hematite mixing volumes considered by Balkanski et al. (2007).
Historically, climate models have relied on the fixed refractive index to consider dust radiative forcing starting (IPCC, 2001) with a strongly absorptive value based on dust samples in the Sahara (Patterson et al., 1977) transitioning to more scattering values after dust absorption could be inferred from satellite and surface observations (e.g., Sinyuk et al., 2003; Balkanski et al., 2007). With the launch of EMIT in July 2022 and the expected delivery of a high-resolution map of soil mineralogy in source areas, dust interactions with radiation in climate models will be calculated directly from the simulated mineralogical composition (Li et al., 2021). Still, the additional burden of simulating a dozen minerals may be too prohibitive for large ensemble climate model simulations. In such cases, our analysis suggests the use of a fixed value providing similar radiative effects as the comprehensive representation of minerals. However, our recommendation is directed toward the GFDL AM4.0 model with all its uncertainties related to mineral distribution, emission sources, and aerosol transport. Moreover, incorporating dust mineralogy in models is likely to be important in other respects, such as cloud properties, ocean biogeochemistry, air quality, and photochemistry. For studies with resolved mineralogy, we show that the number of mineral tracers can be reduced from 45 to 25 without losing the quality of comparison with observations of CERES fluxes and CRU surface temperature. Such reduction can be achieved by lumping together clay minerals and removing external hematite and gypsum. For specific research such as biogeochemistry, it may be necessary to fully resolve mineralogy to achieve accuracy.
This study has some limitations. First, the soil mineralogy map from C1999 is based on extensive extrapolation and limited observations. In terms of the need to improve knowledge of soil mineralogy in dust source regions, the EMIT instrument operating from the International Space Station will provide mineral identifications of dust sources using hyperspectral measurements (Green et al., 2020). The EMIT soil map measurements will improve resolving dust mineralogy in climate models and advance our understanding of dust's effects in the Earth system. Second, hematite and goethite are the most common iron oxides present in soils. However, goethite is not considered in this study because it is not included in the soil mineralogy map used. Previous studies suggest that goethite is generally more abundant than hematite, but it is less absorptive than hematite in the visible spectrum (Formenti et al., 2014). Therefore, the abundance of iron oxides may be underestimated in this study, which may lead to underestimation of dust absorption in the SW. A more recent database by Journet et al. (2014) (J2014) includes the distribution of goethite but it shares many limitations with C1999 (e.g., extensive extrapolation) and has other major disadvantages, such as missing soil fractions of some minerals at some locations. Third, the refractive index of hematite used in our study is close to the upper range of the values available in the literature (Zhang et al., 2015). Hence, the last two limitations, underestimation of iron oxide content and overestimation of absorption by hematite, may have compensating effects.
This study, by prescribing SST, calculates only the fast response to the dust DRE, without including the slow response by the sea surface temperature. This avoids the need to spin up the model for decades before reaching new equilibrium but may overestimate the eventual response, as shown by Miller and Tegen (1998) and Balkanski et al. (2021). This complicates model evaluation because the observations include the slow response to dust. Variables like precipitation are especially sensitive to the inclusion of the slow response because prescribed SST experiments omit the surface energy balance over the ocean. Thus, the surface DRE beneath the aerosol layer, which is generally negative, is not fully balanced by a fast reduction of evaporation (Miller et al., 2004a). The addition of the surface balance in the slow response can reverse the sign of the fast precipitation anomaly (Miller and Tegen, 1998; Jordan et al., 2018). In this study, the increase in dust scattering (e.g., through consideration of dust mineral composition) generally reduces model biases for all variables except precipitation. Future works may include satellite-based inventory of soil mineralogy using fully coupled Earth system components.
The GFDL AM4.0 code is publicly available at https://github.com/NOAA-GFDL/AM4 (last access: 16 June 2024; Zhao et al., 2018a, b).
The AERONET Version 3 Level 2.0 Almucantar inversion retrieval data are available at https://aeronet.gsfc.nasa.gov/new_web/download_all_v3_inversions.html (Giles et al., 2019; Sinyuk et al., 2020). The lab-measured dust SSA at 550 nm is available at https://data.eurochamp.org/data-access/optical-properties/#/ (Di Biagio et al., 2019). CERES EBAF Ed 4.2 data were obtained from the NASA Langley Research Center CERES ordering tool at https://ceres.larc.nasa.gov/data/ (Loeb et al., 2018). The CRU TS data are available at https://crudata.uea.ac.uk/cru/data/hrg/cru_ts_4.07/observation.v4.07/ (Harris et al., 2020).
The supplement related to this article is available online at: https://doi.org/10.5194/acp-24-7421-2024-supplement.
QS: conceptualization, formal analysis, investigation, methodology, validation, visualization, resources, writing (original draft preparation). PG: conceptualization, investigation, methodology, validation, resources, supervision, writing (review and editing). MGA, VO, RLM, and CPGP: conceptualization, methodology, resources, writing (review and editing).
The contact author has declared that none of the authors has any competing interests.
Publisher's note: Copernicus Publications remains neutral with regard to jurisdictional claims made in the text, published maps, institutional affiliations, or any other geographical representation in this paper. While Copernicus Publications makes every effort to include appropriate place names, the final responsibility lies with the authors.
The authors are grateful to the AERONET PI(s) and their staff for establishing and maintaining the sites and data used in this investigation. We acknowledge the CERES EBAF Ed 4.2 data, which were obtained from the NASA Langley Research Center CERES ordering tool at https://ceres.larc.nasa.gov/data/ (last access: 16 June 2024). We also thank the National Aeronautics and Space Administration (NASA) EMIT project, which is supported by the NASA Earth Venture Instrument program under the Earth Science Division of the Science Mission Directorate.
This research was supported by a collaboration between Princeton University and the NOAA GFDL Cooperative Institute for Modeling the Earth System (CIMES). A portion of this work is funded by the Earth Surface Mineral Dust Source Investigation (EMIT), a NASA Earth Ventures-Instrument (EVI-4) Mission. Carlos Pérez García-Pando and María Gonçalves Ageitos were supported by the European Research Council (ERC) under the EU Horizon 2020 research and innovation program through the ERC Consolidator Grant FRAGMENT (grant agreement no. 773051), the AXA Research Fund through the AXA Chair on Sand and Dust Storms at the Barcelona Supercomputing Center (BSC), the European Union’s Horizon 2020 research and innovation program under grant agreement no. 821205 (FORCeS), and the Department of Research and Universities of the Government of Catalonia via the Research Group Atmospheric Composition (code 2021 SGR 01550). Vincenzo Obiso was supported by the NASA Postdoctoral Program at the NASA Goddard Institute for Space Studies administered by Oak Ridge Associated Universities under contract with NASA (80HQTR21CA005).
This paper was edited by Yves Balkanski and reviewed by two anonymous referees.
Allen, M. R. and Ingram, W. J.: Constrains on future changes in climate and the hydrologic cycle, Nature, 419, 224–232, 2002.
Atkinson, J. D., Murray, B. J., Woodhouse, M. T., Whale, T. F., Baustian, K. J., Carslaw, K. S., Dobbie, S., O'Sullivan, D., and Malkin, T. L.: The importance of feldspar for ice nucleation by mineral dust in mixed-phase clouds, Nature, 498, 355–358, https://doi.org/10.1038/nature12278, 2013.
Balkanski, Y., Schulz, M., Claquin, T., and Guibert, S.: Reevaluation of Mineral aerosol radiative forcings suggests a better agreement with satellite and AERONET data, Atmos. Chem. Phys., 7, 81–95, https://doi.org/10.5194/acp-7-81-2007, 2007.
Balkanski, Y., Bonnet, R., Boucher, O., Checa-Garcia, R., and Servonnat, J.: Better representation of dust can improve climate models with too weak an African monsoon, Atmos. Chem. Phys., 21, 11423–11435, https://doi.org/10.5194/acp-21-11423-2021, 2021.
Bian, H. and Zender, C. S.: Mineral dust and global tropospheric chemistry: Relative roles of photolysis and heterogeneous uptake, J. Geophys. Res.-Atmos., 108, D21, https://doi.org/10.1029/2002jd003143, 2003.
Bullard, J. E., Harrison, S. P., Baddock, M. C., Drake, N., Gill, T. E., McTainsh, G., and Sun, Y.: Preferential dust sources: A geomorphological classification designed for use in global dust-cycle models, J. Geophys. Res.-Earth, 116, F4, https://doi.org/10.1029/2011JF002061, 2011.
Chatziparaschos, M., Daskalakis, N., Myriokefalitakis, S., Kalivitis, N., Nenes, A., Gonçalves Ageitos, M., Costa-Surós, M., Pérez García-Pando, C., Zanoli, M., Vrekoussis, M., and Kanakidou, M.: Role of K-feldspar and quartz in global ice nucleation by mineral dust in mixed-phase clouds, Atmos. Chem. Phys., 23, 1785–1801, https://doi.org/10.5194/acp-23-1785-2023, 2023.
Claquin, T., Schulz, M., Balkanski, Y., and Boucher, O.: Uncertainties in assessing radiative forcing by mineral dust, Tellus B, 50, 491–505, https://doi.org/10.1034/j.1600-0889.1998.t01-2-00007.x, 1998.
Claquin, T., Schulz, M., and Balkanski, Y. J.: Modeling the mineralogy of atmospheric dust sources, J. Geophys. Res.-Atmos., 104, 22243–22256, https://doi.org/10.1029/1999JD900416, 1999.
Delworth, T. L., Broccoli, A. J., Rosati, A., Stouffer, R. J., Balaji, V., Beesley, J. A., Cooke, W. F., Dixon, K. W., Dunne, J., Dunne, K. A., Durachta, J. W., Findell, K. L., Ginoux, P., Gnanadesikan, A., Gordon, C. T., Griffies, S. M., Gudgel, R., Harrison, M. J., Held, I. M., Hemler, R. S., Horowitz, L. W., Klein, S. A., Knutson, T. R., Kushner, P. J., Langenhorst, A. R., Lee, H. C., Lin, S. J., Lu, J., Malyshev, S. L., Milly, P. C. D., Ramaswamy, V., Russell, J., Schwarzkopf, M. D., Shevliakova, E., Sirutis, J. J., Spelman, M. J., Stern, W. F., Winton, M., Wittenberg, A. T., Wyman, B., Zeng, F., and Zhang, R.: GFDL's CM2 global coupled climate models. Part I: Formulation and simulation characteristics, J. Climate, 19, 643–674, https://doi.org/10.1175/JCLI3629.1, 2006.
Dentener, F. J., Carmichael, G. R., Zhang, Y., Lelieveld, J., and Crutzen, P. J.: Role of mineral aerosol as a reactive surface in the global troposphere, J. Geophys. Res.-Atmos., 101, 22869–22889, https://doi.org/10.1029/96jd01818, 1996.
Di Biagio, C., Formenti, P., Balkanski, Y., Caponi, L., Cazaunau, M., Pangui, E., Journet, E., Nowak, S., Caquineau, S., Andreae, M. O., Kandler, K., Saeed, T., Piketh, S., Seibert, D., Williams, E., and Doussin, J.-F.: Global scale variability of the mineral dust long-wave refractive index: a new dataset of in situ measurements for climate modeling and remote sensing, Atmos. Chem. Phys., 17, 1901–1929, https://doi.org/10.5194/acp-17-1901-2017, 2017.
Di Biagio, C., Formenti, P., Balkanski, Y., Caponi, L., Cazaunau, M., Pangui, E., Journet, E., Nowak, S., Andreae, M. O., Kandler, K., Saeed, T., Piketh, S., Seibert, D., Williams, E., and Doussin, J.-F.: Complex refractive indices and single-scattering albedo of global dust aerosols in the shortwave spectrum and relationship to size and iron content, Atmos. Chem. Phys., 19, 15503–15531, https://doi.org/10.5194/acp-19-15503-2019, 2019 (data available at: https://data.eurochamp.org/data-access/optical-properties/#/, last access: 16 June 2024).
Donner, L. J., Wyman, B. L., Hemler, R. S., Horowitz, L. W., Ming, Y., Zhao, M., Golaz, J. C., Ginoux, P., Lin, S. J., Schwarzkopf, M. D., Austin, J., Alaka, G., Cooke, W. F., Delworth, T. L., Freidenreich, S. M., Gordon, C. T., Griffies, S. M., Held, I. M., Hurlin, W. J., Klein, S. A., Knutson, T. R., Langenhorst, A. R., Lee, H. C., Lin, Y., Magi, B. I., Malyshev, S. L., Milly, P. C. D., Naik, V., Nath, M. J., Pincus, R., Ploshay, J. J., Ramaswamy, V., Seman, C. J., Shevliakova, E., Sirutis, J. J., Stern, W. F., Stouffer, R. J., Wilson, R. J., Winton, M., Wittenberg, A. T., and Zeng, F.: The dynamical core, physical parameterizations, and basic simulation characteristics of the atmospheric component AM3 of the GFDL global coupled model CM3, J. Climate, 24, 3484–3519, https://doi.org/10.1175/2011JCLI3955.1, 2011.
Dubovik, O., Holben, B., Eck, T. F., Smirnov, A., Kaufman, Y. J., King, M. D., Tanre, D., and Slutsker, I.: Variability of absorption and optical properties of key aerosol types observed in worldwide locations, J. Atmos. Sci., 59, 590–608, https://doi.org/10.1175/1520-0469(2002)059<0590:Voaaop>2.0.Co;2, 2002.
Dunne, J. P., Horowitz, L. W., Adcroft, A. J., Ginoux, P., Held, I. M., John, J. G., Krasting, J. P., Malyshev, S., Naik, V., Paulot, F., Shevliakova, E., Stock, C. A., Zadeh, N., Balaji, V., Blanton, C., Dunne, K. A., Dupuis, C., Durachta, J., Dussin, R., Gauthier, P. P. G., Griffies, S. M., Guo, H., Hallberg, R. W., Harrison, M., He, J., Hurlin, W., McHugh, C., Menzel, R., Milly, P. C. D., Nikonov, S., Paynter, D. J., Ploshay, J., Radhakrishnan, A., Rand, K., Reichl, B. G., Robinson, T., Schwarzkopf, D. M., Sentman, L. T., Underwood, S., Vahlenkamp, H., Winton, M., Wittenberg, A. T., Wyman, B., Zeng, Y., and Zhao, M.: The GFDL Earth System Model Version 4.1 (GFDL-ESM 4.1): Overall Coupled Model Description and Simulation Characteristics, J. Adv. Model. Earth Sy., 12, e2019MS002015, https://doi.org/10.1029/2019MS002015, 2020.
Evans, S., Malyshev, S., Ginoux, P., and Shevliakova, E.: The Impacts of the Dust Radiative Effect on Vegetation Growth in the Sahel, Global Biogeochem. Cy., 33, 1582–1593, https://doi.org/10.1029/2018GB006128, 2019.
Formenti, P., Caquineau, S., Chevaillier, S., Klaver, A., Desboeufs, K., Rajot, J. L., Belin, S., and Briois, V.: Dominance of goethite over hematite in iron oxides of mineral dust from Western Africa: Quantitative partitioning by X-ray absorption spectroscopy, J. Geophys. Res.-Atmos., 119, 12740–12754, https://doi.org/10.1002/2014JD021668, 2014.
Gates, W. L.: AMIP: the Atmospheric Model Intercomparison Project, B. Am. Meteorol. Soc., 73, 1962–1970, https://doi.org/10.1175/1520-0477(1992)073<1962:ATAMIP>2.0.CO;2, 1992.
Giles, D. M., Sinyuk, A., Sorokin, M. G., Schafer, J. S., Smirnov, A., Slutsker, I., Eck, T. F., Holben, B. N., Lewis, J. R., Campbell, J. R., Welton, E. J., Korkin, S. V., and Lyapustin, A. I.: Advancements in the Aerosol Robotic Network (AERONET) Version 3 database – automated near-real-time quality control algorithm with improved cloud screening for Sun photometer aerosol optical depth (AOD) measurements, Atmos. Meas. Tech., 12, 169–209, https://doi.org/10.5194/amt-12-169-2019, 2019 (data available at: https://aeronet.gsfc.nasa.gov/new_web/download_all_v3_inversions.html, last access: 16 June 2024).
Ginoux, P.: Effects of nonsphericity on mineral dust modeling, J. Geophys. Res.-Atmos., 108, D2, https://doi.org/10.1029/2002jd002516, 2003.
Ginoux, P., Chin, M., Tegen, I., Prospero, J. M., Holben, B., Dubovik, O., and Lin, S. J.: Sources and distributions of dust aerosols simulated with the GOCART model, J. Geophys. Res., 106, 20255–20273, https://doi.org/10.1029/2000jd000053, 2001.
Ginoux, P., Gonçalves Ageitos, M., Song, Q., Miller, R. L., Obiso, V., and Pérez García-Pando, C.: Implementation of dust mineralogy in the GFDL AM4.0 climate model, in preparation, 2024.
Gliß, J., Mortier, A., Schulz, M., Andrews, E., Balkanski, Y., Bauer, S. E., Benedictow, A. M. K., Bian, H., Checa-Garcia, R., Chin, M., Ginoux, P., Griesfeller, J. J., Heckel, A., Kipling, Z., Kirkevåg, A., Kokkola, H., Laj, P., Le Sager, P., Lund, M. T., Lund Myhre, C., Matsui, H., Myhre, G., Neubauer, D., van Noije, T., North, P., Olivié, D. J. L., Rémy, S., Sogacheva, L., Takemura, T., Tsigaridis, K., and Tsyro, S. G.: AeroCom phase III multi-model evaluation of the aerosol life cycle and optical properties using ground- and space-based remote sensing as well as surface in situ observations, Atmos. Chem. Phys., 21, 87–128, https://doi.org/10.5194/acp-21-87-2021, 2021.
Gonçalves Ageitos, M., Obiso, V., Miller, R. L., Jorba, O., Klose, M., Dawson, M., Balkanski, Y., Perlwitz, J., Basart, S., Di Tomaso, E., Escribano, J., Macchia, F., Montané, G., Mahowald, N. M., Green, R. O., Thompson, D. R., and Pérez García-Pando, C.: Modeling dust mineralogical composition: sensitivity to soil mineralogy atlases and their expected climate impacts, Atmos. Chem. Phys., 23, 8623–8657, https://doi.org/10.5194/acp-23-8623-2023, 2023.
Green, R. O., Mahowald, N., Ung, C., Thompson, D. R., Bator, L., Bennet, M., Bernas, M., Blackway, N., Bradley, C., Cha, J., Clark, P., Clark, R., Cloud, D., Diaz, E., Ben Dor, E., Duren, R., Eastwood, M., Ehlmann, B. L., Fuentes, L., Ginoux, P., Gross, J., He, Y., Kalashnikova, O., Kert, W., Keymeulen, D., Klimesh, M., Ku, D., Kwong-Fu, H., Liggett, E., Li, L., Lundeen, S., Makowski, M. D., Mazer, A., Miller, R., Mouroulis, P., Oaida, B., Okin, G. S., Ortega, A., Oyake, A., Nguyen, H., Pace, T., Painter, T. H., Pempejian, J., Garcia-Pando, C. P., Pham, T., Phillips, B., Pollock, R., Purcell, R., Realmuto, V., Schoolcraft, J., Sen, A., Shin, S., Shaw, L., Soriano, M., Swayze, G., Thingvold, E., Vaid, A., and Zan, J.: The Earth Surface Mineral Dust Source Investigation: An Earth Science Imaging Spectroscopy Mission, in: 2020 IEEE Aerospace Conference, 7 March 2020, 1–15, https://doi.org/10.1109/AERO47225.2020.9172731, 2020.
Grider, A., Ponette-González, A., and Heindel, R.: Calcium and ammonium now control the pH of wet and bulk deposition in Ohio, U. S., Atmos. Environ., 310, 119986, https://doi.org/10.1016/j.atmosenv.2023.119986, 2023.
Guo, H., Ming, Y., Fan, S., Zhou, L., Harris, L., and Zhao, M.: Two-Moment Bulk Cloud Microphysics With Prognostic Precipitation in GFDL's Atmosphere Model AM4.0: Configuration and Performance, J. Adv. Model. Earth Sy., 13, e2020MS002453, https://doi.org/10.1029/2020MS002453, 2021.
Harris, I., Osborn, T. J., Jones, P., and Lister, D.: Version 4 of the CRU TS monthly high-resolution gridded multivariate climate dataset, Sci. Data, 7, 109, https://doi.org/10.1038/s41597-020-0453-3, 2020 (data available at: https://crudata.uea.ac.uk/cru/data/hrg/cru_ts_4.07/observation.v4.07/, last access: 16 June 2024).
Harrison, A. D., Lever, K., Sanchez-Marroquin, A., Holden, M. A., Whale, T. F., Tarn, M. D., McQuaid, J. B., and Murray, B. J.: The ice-nucleating ability of quartz immersed in water and its atmospheric importance compared to K-feldspar, Atmos. Chem. Phys., 19, 11343–11361, https://doi.org/10.5194/acp-19-11343-2019, 2019.
Huang, Y., Kok, J. F., Saito, M., and Muñoz, O.: Single-scattering properties of ellipsoidal dust aerosols constrained by measured dust shape distributions, Atmos. Chem. Phys., 23, 2557–2577, https://doi.org/10.5194/acp-23-2557-2023, 2023.
Huneeus, N., Schulz, M., Balkanski, Y., Griesfeller, J., Prospero, J., Kinne, S., Bauer, S., Boucher, O., Chin, M., Dentener, F., Diehl, T., Easter, R., Fillmore, D., Ghan, S., Ginoux, P., Grini, A., Horowitz, L., Koch, D., Krol, M. C., Landing, W., Liu, X., Mahowald, N., Miller, R., Morcrette, J.-J., Myhre, G., Penner, J., Perlwitz, J., Stier, P., Takemura, T., and Zender, C. S.: Global dust model intercomparison in AeroCom phase I, Atmos. Chem. Phys., 11, 7781–7816, https://doi.org/10.5194/acp-11-7781-2011, 2011.
IPCC: Climate Change 2001: The Scientific Basis, Summary for Policy Makers and Technical Summary of the Working Group 1 Report, edited by: Houghton, J. T., Ding, Y., Griggs, D. J., Noguer, M., van der Linden, P. J., and Xiaosu, D., Intergovernmental Panel on Climate Change, Cambridge University Press, Cambridge, UK, 2001.
Jin, Q., Yang, Z. L., and Wei, J.: High sensitivity of Indian summer monsoon to Middle East dust absorptive properties, Sci. Rep.-UK, 6, 30690, https://doi.org/10.1038/srep30690, 2016.
Jordan, A. K., Gnanadesikan, A., and Zaitchik, B.: Simulated dust aerosol impacts on western sahelian rainfall: Importance of ocean coupling, J. Climate, 31, 9107–9124, https://doi.org/10.1175/JCLI-D-17-0819.1, 2018.
Journet, E., Balkanski, Y., and Harrison, S. P.: A new data set of soil mineralogy for dust-cycle modeling, Atmos. Chem. Phys., 14, 3801–3816, https://doi.org/10.5194/acp-14-3801-2014, 2014.
Kandler, K., Schutz, L., Deutscher, C., Ebert, M., Hofmann, H., Jackel, S., Jaenicke, R., Knippertz, P., Lieke, K., Massling, A., Petzold, A., Schladitz, A., Weinzierl, B., Wiedensohler, A., Zorn, S., and Weinbruch, S.: Size distribution, mass concentration, chemical and mineralogical composition and derived optical parameters of the boundary layer aerosol at Tinfou, Morocco, during SAMUM 2006, Tellus B, 61, 32–50, https://doi.org/10.1111/j.1600-0889.2008.00385.x, 2009.
Kelly, J. T., Chuang, C. C., and Wexler, A. S.: Influence of dust composition on cloud droplet formation, Atmos. Environ., 41, 2904–2916, https://doi.org/10.1016/j.atmosenv.2006.12.008, 2007.
Kok, J. F.: A scaling theory for the size distribution of emitted dust aerosols suggests climate models underestimate the size of the global dust cycle, P. Natl. Acad. Sci. USA, 108, 1016–1021, https://doi.org/10.1073/pnas.1014798108, 2011.
Kok, J. F., Ridley, D. A., Zhou, Q., Miller, R. L., Zhao, C., Heald, C. L., Ward, D. S., Albani, S., and Haustein, K.: Smaller desert dust cooling effect estimated from analysis of dust size and abundance, Nat. Geosci., 10, 274–278, https://doi.org/10.1038/Ngeo2912, 2017.
Kok, J. F., Adebiyi, A. A., Albani, S., Balkanski, Y., Checa-Garcia, R., Chin, M., Colarco, P. R., Hamilton, D. S., Huang, Y., Ito, A., Klose, M., Leung, D. M., Li, L., Mahowald, N. M., Miller, R. L., Obiso, V., Pérez García-Pando, C., Rocha-Lima, A., Wan, J. S., and Whicker, C. A.: Improved representation of the global dust cycle using observational constraints on dust properties and abundance, Atmos. Chem. Phys., 21, 8127–8167, https://doi.org/10.5194/acp-21-8127-2021, 2021.
Lau, K. M., Kim, K. M., Sud, Y. C., and Walker, G. K.: A GCM study of the response of the atmospheric water cycle of West Africa and the Atlantic to Saharan dust radiative forcing, Ann. Geophys., 27, 4023–4037, https://doi.org/10.5194/angeo-27-4023-2009, 2009.
Li, L., Mahowald, N. M., Miller, R. L., Pérez García-Pando, C., Klose, M., Hamilton, D. S., Gonçalves Ageitos, M., Ginoux, P., Balkanski, Y., Green, R. O., Kalashnikova, O., Kok, J. F., Obiso, V., Paynter, D., and Thompson, D. R.: Quantifying the range of the dust direct radiative effect due to source mineralogy uncertainty, Atmos. Chem. Phys., 21, 3973–4005, https://doi.org/10.5194/acp-21-3973-2021, 2021.
Liu, Y. and Daum, P. H.: Relationship of refractive index to mass density and self-consistency of mixing rules for multicomponent mixtures like ambient aerosols, J. Aerosol Sci., 39, 974–986, https://doi.org/10.1016/j.jaerosci.2008.06.006, 2008.
Loeb, N. G., Doelling, D. R., Wang, H., Su, W., Nguyen, C., Corbett, J. G., Liang, L., Mitrescu, C., Rose, F. G., and Kato, S.: Clouds and the Earth'S Radiant Energy System (CERES) Energy Balanced and Filled (EBAF) top-of-atmosphere (TOA) edition-4.0 data product, J. Climate, 31, 895–918, https://doi.org/10.1175/JCLI-D-17-0208.1, 2018 (data available at: https://ceres.larc.nasa.gov/data/, last access: 16 June 2024).
Mahowald, N.: Aerosol indirect effect on biogeochemical cycles and climate, Science (80-), 334, 794–796, https://doi.org/10.1126/science.1207374, 2011.
Markel, V. A.: Introduction to the Maxwell Garnett approximation: tutorial, J. Opt. Soc. Am. A, 33, 1244, https://doi.org/10.1364/josaa.33.001244, 2016.
Matthes, K., Funke, B., Andersson, M. E., Barnard, L., Beer, J., Charbonneau, P., Clilverd, M. A., Dudok de Wit, T., Haberreiter, M., Hendry, A., Jackman, C. H., Kretzschmar, M., Kruschke, T., Kunze, M., Langematz, U., Marsh, D. R., Maycock, A. C., Misios, S., Rodger, C. J., Scaife, A. A., Seppälä, A., Shangguan, M., Sinnhuber, M., Tourpali, K., Usoskin, I., van de Kamp, M., Verronen, P. T., and Versick, S.: Solar forcing for CMIP6 (v3.2), Geosci. Model Dev., 10, 2247–2302, https://doi.org/10.5194/gmd-10-2247-2017, 2017.
Meinshausen, M., Vogel, E., Nauels, A., Lorbacher, K., Meinshausen, N., Etheridge, D. M., Fraser, P. J., Montzka, S. A., Rayner, P. J., Trudinger, C. M., Krummel, P. B., Beyerle, U., Canadell, J. G., Daniel, J. S., Enting, I. G., Law, R. M., Lunder, C. R., O'Doherty, S., Prinn, R. G., Reimann, S., Rubino, M., Velders, G. J. M., Vollmer, M. K., Wang, R. H. J., and Weiss, R.: Historical greenhouse gas concentrations for climate modelling (CMIP6), Geosci. Model Dev., 10, 2057–2116, https://doi.org/10.5194/gmd-10-2057-2017, 2017.
Miller, R. L. and Tegen, I.: Climate response to soil dust aerosols, J. Climate, 11, 3247–3267, https://doi.org/10.1175/1520-0442(1998)011<3247:CRTSDA>2.0.CO;2, 1998.
Miller, R. L. and Tegen, I.: Radiative Forcing of a Tropical Direct Circulation by Soil Dust Aerosols, J. Atmos. Sci., 56, 2403–2433, https://doi.org/10.1175/1520-0469(1999)056<2403:RFOATD>2.0.CO;2, 1999.
Miller, R. L., Perlwitz, J., and Tegen, I.: Feedback upon dust emission by dust radiative forcing through the planetary boundary layer, J. Geophys. Res.-Atmos., 109, 1–17, https://doi.org/10.1029/2004JD004912, 2004a.
Miller, R. L., Perlwitz, J., and Tegen, I.: Modeling Arabian dust mobilization during the Asian summer monsoon: The effect of prescribed versus calculated SST, Geophys. Res. Lett., 31, 1–4, https://doi.org/10.1029/2004GL020669, 2004b.
Miller, R. L., Knippertz, P., Pérez García-Pando, C., Perlwitz, J. P., and Tegen, I.: Impact of dust radiative forcing upon climate, in: Mineral Dust: A Key Player in the Earth System, Springer Netherlands, 327–357, https://doi.org/10.1007/978-94-017-8978-3_13, 2014.
Ming, Y., Ramaswamy, V., and Persad, G.: Two opposing effects of absorbing aerosols on global-mean precipitation, Geophys. Res. Lett., 37, https://doi.org/10.1029/2010GL042895, 2010.
Obiso, V., Gonçalves Ageitos, M., Pérez García-Pando, C., Schuster, G. L., Bauer, S. E., Di Biagio, C., Formenti, P., Perlwitz, J. P., Tsigaridis, K., and Miller, R. L.: Observationally constrained regional variations of shortwave absorption by iron oxides emphasize the cooling effect of dust, EGUsphere [preprint], https://doi.org/10.5194/egusphere-2023-1166, 2023.
Panta, A., Kandler, K., Alastuey, A., González-Flórez, C., González-Romero, A., Klose, M., Querol, X., Reche, C., Yus-Díez, J., and Pérez García-Pando, C.: Insights into the single-particle composition, size, mixing state, and aspect ratio of freshly emitted mineral dust from field measurements in the Moroccan Sahara using electron microscopy, Atmos. Chem. Phys., 23, 3861–3885, https://doi.org/10.5194/acp-23-3861-2023, 2023.
Patterson, E. M., Gillette, D. A., and Stockton, B. H.: Complex index of refraction between 300 and 700 nm for Saharan aerosols, J. Geophys. Res., 82, 3153–3160, https://doi.org/10.1029/jc082i021p03153, 1977.
Paulot, F., Ginoux, P., Cooke, W. F., Donner, L. J., Fan, S., Lin, M.-Y., Mao, J., Naik, V., and Horowitz, L. W.: Sensitivity of nitrate aerosols to ammonia emissions and to nitrate chemistry: implications for present and future nitrate optical depth, Atmos. Chem. Phys., 16, 1459–1477, https://doi.org/10.5194/acp-16-1459-2016, 2016.
Pérez, C., Nickovic, S., Pejanovic, G., Baldasano, J. M., and Özsoy, E.: Interactive dust-radiation modeling: A step to improve weather forecasts, J. Geophys. Res.-Atmos., 111, D16, https://doi.org/10.1029/2005JD006717, 2006.
Perez Garcia-Pando, C., Miller, R. L., Perlwitz, J. P., Rodríguez, S., and Prospero, J. M.: Predicting the mineral composition of dust aerosols: Insights from elemental composition measured at the Izaña Observatory, Geophys. Res. Lett., 43, 10520–10529, https://doi.org/10.1002/2016GL069873, 2016.
Perlwitz, J. P., Pérez García-Pando, C., and Miller, R. L.: Predicting the mineral composition of dust aerosols – Part 1: Representing key processes, Atmos. Chem. Phys., 15, 11593–11627, https://doi.org/10.5194/acp-15-11593-2015, 2015a.
Perlwitz, J. P., Pérez García-Pando, C., and Miller, R. L.: Predicting the mineral composition of dust aerosols – Part 2: Model evaluation and identification of key processes with observations, Atmos. Chem. Phys., 15, 11629–11652, https://doi.org/10.5194/acp-15-11629-2015, 2015b.
Persad, G. G., Ming, Y., and Ramaswamy, V.: The role of aerosol absorption in driving clear-sky solar dimming over East Asia, J. Geophys. Res., 119, 10410–10424, https://doi.org/10.1002/2014JD021577, 2014.
Réveillet, M., Dumont, M., Gascoin, S., Lafaysse, M., Nabat, P., Ribes, A., Nheili, R., Tuzet, F., Ménégoz, M., Morin, S., Picard, G., and Ginoux, P.: Black carbon and dust alter the response of mountain snow cover under climate change, Nat. Commun., 13, 5279, https://doi.org/10.1038/s41467-022-32501-y, 2022.
Rosenfeld, D., Rudich, Y., and Lahav, R.: Desert dust suppressing precipitation: A possible desertification feedback loop, P. Natl. Acad. Sci. USA, 98, 5975–5980, https://doi.org/10.1073/pnas.101122798, 2001.
Ryder, C. L., Marenco, F., Brooke, J. K., Estelles, V., Cotton, R., Formenti, P., McQuaid, J. B., Price, H. C., Liu, D., Ausset, P., Rosenberg, P. D., Taylor, J. W., Choularton, T., Bower, K., Coe, H., Gallagher, M., Crosier, J., Lloyd, G., Highwood, E. J., and Murray, B. J.: Coarse-mode mineral dust size distributions, composition and optical properties from AER-D aircraft measurements over the tropical eastern Atlantic, Atmos. Chem. Phys., 18, 17225–17257, https://doi.org/10.5194/acp-18-17225-2018, 2018.
Samset, B. H.: Aerosol absorption has an underappreciated role in historical precipitation change, Commun. Earth Environ., 3, 242, https://doi.org/10.1038/s43247-022-00576-6, 2022.
Scanza, R. A., Mahowald, N., Ghan, S., Zender, C. S., Kok, J. F., Liu, X., Zhang, Y., and Albani, S.: Modeling dust as component minerals in the Community Atmosphere Model: development of framework and impact on radiative forcing, Atmos. Chem. Phys., 15, 537–561, https://doi.org/10.5194/acp-15-537-2015, 2015.
Schuster, G. L., Dubovik, O., and Arola, A.: Remote sensing of soot carbon – Part 1: Distinguishing different absorbing aerosol species, Atmos. Chem. Phys., 16, 1565–1585, https://doi.org/10.5194/acp-16-1565-2016, 2016.
Sinyuk, A., Torres, O., and Dubovik, O.: Combined use of satellite and surface observations to infer the imaginary part of refractive index of Saharan dust, Geophys. Res. Lett., 30, https://doi.org/10.1029/2002GL016189, 2003.
Sinyuk, A., Holben, B. N., Eck, T. F., Giles, D. M., Slutsker, I., Korkin, S., Schafer, J. S., Smirnov, A., Sorokin, M., and Lyapustin, A.: The AERONET Version 3 aerosol retrieval algorithm, associated uncertainties and comparisons to Version 2, Atmos. Meas. Tech., 13, 3375–3411, https://doi.org/10.5194/amt-13-3375-2020, 2020.
Skiles, S. M. K., Flanner, M., Cook, J. M., Dumont, M., and Painter, T. H.: Radiative forcing by light-absorbing particles in snow, Nat. Clim. Change, 8, 964–971, https://doi.org/10.1038/s41558-018-0296-5, 2018.
Sokolik, I. N. and Toon, O. B.: Incorporation of mineralogical composition into models of the radiative properties of mineral aerosol from UV to IR wavelengths, J. Geophys. Res., 104, 9423–9444, https://doi.org/10.1029/1998jd200048, 1999.
Song, C. H. and Carmichael, G. R.: Gas-particle partitioning of nitric acid modulated by alkaline aerosol, J. Atmos. Chem., 40, 1–22, https://doi.org/10.1023/A:1010657929716, 2001.
Song, Q., Zhang, Z., Yu, H., Kok, J. F., Di Biagio, C., Albani, S., Zheng, J., and Ding, J.: Size-resolved dust direct radiative effect efficiency derived from satellite observations, Atmos. Chem. Phys., 22, 13115–13135, https://doi.org/10.5194/acp-22-13115-2022, 2022.
Stephens, G. L., Wood, N. B., and Pakula, L. A.: On the radiative effects of dust on tropical convection, Geophys. Res. Lett., 31, 1–4, https://doi.org/10.1029/2004GL021342, 2004.
Strong, J. D. O., Vecchi, G. A., and Ginoux, P.: The response of the tropical Atlantic and West African climate to Saharan dust in a fully coupled GCM, J. Climate, 28, 7071–7092, https://doi.org/10.1175/JCLI-D-14-00797.1, 2015.
Taylor, K. E., Williamson, D., and Zwiers, F.: The sea surface temperature and sea-ice concentration boundary conditions for AMIP II simulations, Program for Climate Model Diagnosis and Intercomparison, Lawrence Livermore, https://pcmdi.llnl.gov/report/pdf/60.pdf (last acces: 24 June 2024), 2000.
Wu, C., Lin, Z., and Liu, X.: The global dust cycle and uncertainty in CMIP5 (Coupled Model Intercomparison Project phase 5) models, Atmos. Chem. Phys., 20, 10401–10425, https://doi.org/10.5194/acp-20-10401-2020, 2020.
Zender, C. S., Newman, D., and Torres, O.: Spatial heterogeneity in aeolian erodibility: Uniform, topographic, geomorphic, and hydrologic hypotheses, J. Geophys. Res.-Atmos., 108, D17, https://doi.org/10.1029/2002jd003039, 2003.
Zhang, X. L., Wu, G. J., Zhang, C. L., Xu, T. L., and Zhou, Q. Q.: What is the real role of iron oxides in the optical properties of dust aerosols?, Atmos. Chem. Phys., 15, 12159–12177, https://doi.org/10.5194/acp-15-12159-2015, 2015.
Zhao, A., Ryder, C. L., and Wilcox, L. J.: How well do the CMIP6 models simulate dust aerosols?, Atmos. Chem. Phys., 22, 2095–2119, https://doi.org/10.5194/acp-22-2095-2022, 2022.
Zhao, M., Golaz, J. C., Held, I. M., Guo, H., Balaji, V., Benson, R., Chen, J. H., Chen, X., Donner, L. J., Dunne, J. P., Dunne, K., Durachta, J., Fan, S. M., Freidenreich, S. M., Garner, S. T., Ginoux, P., Harris, L. M., Horowitz, L. W., Krasting, J. P., Langenhorst, A. R., Liang, Z., Lin, P., Lin, S. J., Malyshev, S. L., Mason, E., Milly, P. C. D., Ming, Y., Naik, V., Paulot, F., Paynter, D., Phillipps, P., Radhakrishnan, A., Ramaswamy, V., Robinson, T., Schwarzkopf, D., Seman, C. J., Shevliakova, E., Shen, Z., Shin, H., Silvers, L. G., Wilson, J. R., Winton, M., Wittenberg, A. T., Wyman, B., and Xiang, B.: The GFDL Global Atmosphere and Land Model AM4.0/LM4.0: 1. Simulation Characteristics With Prescribed SSTs, J. Adv. Model. Earth Sy., 10, 691–734, https://doi.org/10.1002/2017MS001208, 2018a (code available at: https://github.com/NOAA-GFDL/AM4, last access: 16 June 2024).
Zhao, M., Golaz, J. C., Held, I. M., Guo, H., Balaji, V., Benson, R., Chen, J. H., Chen, X., Donner, L. J., Dunne, J. P., Dunne, K., Durachta, J., Fan, S. M., Freidenreich, S. M., Garner, S. T., Ginoux, P., Harris, L. M., Horowitz, L. W., Krasting, J. P., Langenhorst, A. R., Liang, Z., Lin, P., Lin, S. J., Malyshev, S. L., Mason, E., Milly, P. C. D., Ming, Y., Naik, V., Paulot, F., Paynter, D., Phillipps, P., Radhakrishnan, A., Ramaswamy, V., Robinson, T., Schwarzkopf, D., Seman, C. J., Shevliakova, E., Shen, Z., Shin, H., Silvers, L. G., Wilson, J. R., Winton, M., Wittenberg, A. T., Wyman, B., and Xiang, B.: The GFDL Global Atmosphere and Land Model AM4.0/LM4.0: 2. Model Description, Sensitivity Studies, and Tuning Strategies, J. Adv. Model. Earth Sy., 10, 735–769, https://doi.org/10.1002/2017MS001209, 2018b.
- Abstract
- Introduction
- Model and data
- Experimental design
- Optical properties
- Impacts of dust mineralogy on climate
- Potential for reducing mineral tracers
- Conclusions
- Code availability
- Data availability
- Author contributions
- Competing interests
- Disclaimer
- Acknowledgements
- Financial support
- Review statement
- References
- Supplement
- Abstract
- Introduction
- Model and data
- Experimental design
- Optical properties
- Impacts of dust mineralogy on climate
- Potential for reducing mineral tracers
- Conclusions
- Code availability
- Data availability
- Author contributions
- Competing interests
- Disclaimer
- Acknowledgements
- Financial support
- Review statement
- References
- Supplement