the Creative Commons Attribution 4.0 License.
the Creative Commons Attribution 4.0 License.
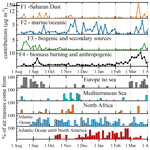
Chemical characterization of atmospheric aerosols at a high-altitude mountain site: a study of source apportionment
Elena Barbaro
Matteo Feltracco
Fabrizio De Blasi
Clara Turetta
Marta Radaelli
Warren Cairns
Giulio Cozzi
Giovanna Mazzi
Marco Casula
Jacopo Gabrieli
Carlo Barbante
Andrea Gambaro
The study of aerosols in high mountain regions is essential because particulate matter can play a role in altering the energy balance of high mountain regions, and aerosols can accelerate glacier melting in high mountain areas by darkening the ice surface, reducing its reflectivity (albedo). Studying aerosols in high mountain areas provides insights into long-range transport of pollutants, atmospheric dynamics, and climate change impacts. These regions can serve as valuable observatories for studying atmospheric processes.
The main aim of this paper is to define the main sources of aerosols over an entire year of sampling at the Col Margherita Atmospheric Observatory (MRG; 46°22′0.059′′ N, 11°47′30.911′′ E; 2543 m a.s.l.), a high-altitude background site in the eastern Italian Alps. Here, we discuss the potential origins of more than 100 chemical markers (major ions, water-soluble organic compounds, trace elements, and rare earth elements) using different approaches. Some diagnostic ratios were applied, but source apportionment using positive matrix factorization (PMF) was used to define the main inputs of PM10 collected at this high-altitude site, resulting in the identification of four factors: (1) Saharan dust events, (2) long-range marine/anthropogenic influence, (3) biogenic sources, and (4) biomass-burning and anthropogenic emissions. It can be inferred that, despite the distant location of the Col Margherita site, both regional pollution and long-range anthropogenic pollution have discernible effects on this area.
- Article
(4827 KB) - Full-text XML
-
Supplement
(4166 KB) - BibTeX
- EndNote
Atmospheric aerosols have strong impacts on the climate (Ren-Jian et al., 2012) because these particles can absorb and diffuse solar radiation, impacting the radiation budget. Their chemical composition can influence their hygroscopic proprieties and hence their ability to act as cloud condensation nuclei (CCN) (Hitzenberger et al., 1999). Aerosol particles can also impact the environment, influencing air pollution. The chemical composition of these particles can have specific impacts on human health, producing respiratory disorders, strokes, and pulmonary and cardiovascular diseases (Ren-Jian et al., 2012).
The aerosols depend on local and regional emission sources, but they can also be derived from atmospheric long-range transport. These particles can be directly emitted into the atmosphere by natural sources (i.e., sea salts or crustal particles) or by anthropogenic activity such as industry or vehicular traffic. Secondary aerosols are another important component of particulate matter because several gas-to-particle or particle-phase reactions can occur, and several aging or oxidative processes can modify their chemical proprieties.
High-altitude mountain stations are considered the best sites to investigate the background levels of trace gases and aerosols, due to their distance from anthropogenic emission sources. The data collected in these stations can be used to study long-range transport of dust or of anthropogenic and biomass-burning pollutants from emission regions. Okamoto and Tanimoto (2016) summarized the results from past and ongoing field measurements of atmospheric constituents at high-altitude stations across the globe with a particular focus on trace gases such as ozone. The authors identified 31 stations at high altitude above 1500 m a.s.l. that provide reliable observational data, which are available online or published in previous studies. Many stations are located in northern mid-latitudes, particularly in central Europe and western North America. Compared to the Northern Hemisphere, significantly fewer stations are in the Southern Hemisphere, and no high-altitude stations are present in the Oceania region. One of the final goals of this publication is to propose the Col Margherita Observatory as a high-altitude background observatory of atmospheric aerosols in the eastern Italian Alps.
The main aim of this paper is to characterize the different sources of atmospheric aerosols collected at the Col Margherita Atmospheric Observatory (MRG; 46°22′0.059′′ N, 11°47′30.911′′ E; 2543 m a.s.l.), a high-altitude background site in the eastern Italian Alps. The chemical composition was used to define specific sources or processes by combining chemometric singular specific diagnostic ratios. More than 100 chemical markers determined inorganic ions (Cl−, Br−, NO, SO, K+, Mg2+, Na+, NH, Ca2+), 12 organic acids (methanesulfonic acid and C2–C7 carboxylic acids), 7 monosaccharides (arabinose, fructose, galactose, glucose, mannose, ribose, and xylose), 8 sugar alcohols (arabitol, erythritol, mannitol, ribitol, sorbitol, xylitol, and maltitol), 3 anhydrosugars (levoglucosan, mannosan, and galactosan), sucrose, 11 phenolic compounds, 40 free L- and D-amino acids, 2 photo-oxidation products of α-pinene (cis-pinonic acid and pinic acid), 27 trace elements (TEs), and 15 rare earth elements (REEs).
The novelty of this paper is in the combination of a consistent number of chemical species to characterize the aerosol sources by considering different types of markers for biogenic, anthropogenic, crustal, and other types of sources at a high mountain site. Positive matrix factorization (PMF) was performed (1) to identify the sources and (2) to define which chemical species were characteristic for each source, because the sources of some compounds are still not well known.
2.1 Sampling and meteorological conditions
Atmospheric aerosols were sampled over the period of a year from 10 August 2021 to 22 July 2022 at the Col Margherita Atmospheric Observatory (MRG; 46°22′0.059′′ N, 11°47′30.911′′ E; 2543 m a.s.l.). Thanks to its location, MRG is an ideal site for the investigation of atmospheric circulation on a regional scale.
Samples were collected using a low-volume aerosol sampler (Tecora Skypost) equipped with a sequential sampling module (average flow rate of 38.3 L min−1) and a sequential sampling module for automatic filter changing. PM10 samples were collected on quartz fiber filters (QFFs; Filtros Anoia, Barcelona) previously decontaminated by a 4 h pre-combustion at 400 °C in a muffle furnace. Each sample was collected over 96 h with an average volume of 335±12 m3 (in ambient conditions) because this time resolution is demonstrated to be the best balance between quantifying the target species at trace levels and sampling resolution. Over the sampling periods, field blanks were taken at the beginning, during, and at the end by loading a filter into the filter holder of the sampler for 5 min with the vacuum pump turned off.
Meteorological sensors were installed on a compact aluminum tower at 3 m above ground level (ATW3, Campbell Scientific), close to the observatory. The measured parameters were air temperature (T) and relative humidity (RH), with an accuracy of ±0.1 °C and ±2 %, respectively (CS215, Campbell Scientific); atmospheric pressure (P), with an accuracy of ±0.3 hPa at +20 °C (PTB110 barometer, Vaisala); and snow depth (SnD), with an accuracy of ±1 cm (SR50A Sonic Ranging Sensor, Campbell Scientific). The sampling frequency was every 5 min, but hourly averages were used for data analysis.
During the considered period, we registered over 89 % of the hourly data. We used the procedure described by Carturan et al. (2019) to fill the gaps in the time series. The reference weather station for gap filling is the one located at Passo Valles, about 3.3 km away and 500 m lower. Figure S1 in the Supplement shows the average and extreme values for each meteorological parameter that we have investigated.
2.2 Mass concentration and chemical analysis
Blank and sample filters were weighed three times (%RSD 5–10 %) before and after sampling using a Sartorius CP225D balance (precision ±0.01 mg), placed inside a class 1000 clean room, at Ca' Foscari University. The balance and the filters were kept in a temperature- (25°) and humidity-controlled (RH 50 %) nitrogen glove box.
Half of the filter was broken into small pieces with a ceramic cutter and placed in a 15 mL vial (previously cleaned with ultrapure water in an ultrasonic bath for 30 min). The sample was spiked with internal standards and extracted with 5 mL of ultrapure water in an ultrasonic bath for 30 min at 10 °C to avoid volatilization of the analytes. The extract solution was filtered through a 0.45 µm PTFE filter to remove particles and quartz fiber traces before instrumental analysis. This extract was analyzed to determine inorganic ions and organic acids (methanesulfonic acid and C2–C7 carboxylic acids) (Barbaro et al., 2020), monosaccharides, sugar alcohols, levoglucosan and its isomers, sucrose (Barbaro et al., 2015b), free amino acids (Barbaro et al., 2015a), phenolic compounds (Zangrando et al., 2013), and photo-oxidation products of α-pinene (Feltracco et al., 2018).
Briefly, an ion chromatograph (IC; Thermo Scientific Dionex™ ICS-5000, Waltham, MA, USA) coupled with a single quadrupole mass spectrometer (MSQ Plus™, Thermo Scientific, Bremen, Germany) was used to analyze anionic compounds: Cl−, NO, SO, Br−, methanesulfonate, and carboxylic acids. The separation was carried out using a Dionex IonPac AS19 anion exchange column (2×250 mm) equipped with a Dionex IonPac AG19 guard column (2×50 mm) using sodium hydroxide (NaOH), produced by an eluent generator, as a mobile phase and with a flow of 0.25 mL min−1. Cationic species (Na+, NH, Mg2+, Ca2+, and K+) were determined using a capillary IC (Thermo Scientific Dionex ICS-5000) equipped with a capillary cation exchange column (Dionex IonPac CS19-4 µm, 0.4×250 mm) and a guard column (Dionex IonPac CG19-4 µm, 0.4×50 mm) and connected with a conductibility detector (Barbaro et al., 2020).
The determination of sugars (Barbaro et al., 2015b) in aerosol samples involved the utilization of the same ion chromatograph with a quadrupole mass spectrometer (ICS-5000 and MSQ Plus™, Thermo Scientific). The separation of seven monosaccharides (arabinose, fructose, galactose, glucose, mannose, ribose, and xylose), sucrose, and maltitol was achieved using a CarboPac PA10™ column (Thermo Scientific, 2×250 mm) equipped with a CarboPac PA10™ guard column (2×50 mm). Separation of seven sugar alcohols (arabitol, erythritol, mannitol, ribitol, sorbitol, xylitol, and galactitol) and anhydrosugars (levoglucosan, mannosan, and galactosan) was accomplished using a CarboPac MA1™ analytical column (Thermo Scientific, 2×250 mm) with an AminoTrap column (2×50 mm). In both methods, the injection volume was 50 µL and the flow rate of NaOH was 0.25 mL min−1. Compound detection was performed in the selected ion monitoring (SIM) acquisition mode in negative electrospray ionization (−ESI-MS).
The determination of free L- and D-amino acids was conducted using an Agilent 1100 Series HPLC System (Waldbronn, Germany) featuring a binary pump, a vacuum degasser, and an autosampler. This system was coupled with an API 4000 triple quadrupole mass spectrometer (Applied Biosystems/MDS SCIEX, Concord, Ontario, Canada) employing a Turbo V electrospray source in positive mode with multiple reaction monitoring (MRM). The separation of free L- and D-amino acids utilized a chiral 2.1×250 mm CHIROBIOTIC TAG column (Advanced Separation Technologies Inc, USA) with ultrapure water containing 0.1 % formic acid (eluent A) and methanol with 0.1 % formic acid (eluent B) as mobile phases. The binary elution program took place at a flow rate of 0.15 mL min−1, and the injection volume was 100 µL (Barbaro et al., 2015a).
For the determination of phenolic compounds (vanillic acid (VA), isovanillic acid (IVA), homovanillic acid (HA), syringic acid (SyA), p-coumaric acid (PA), ferulic acid (FA), vanillin (VAN), syringaldehyde (SyAH), coniferyl aldehyde (CAH), acetosyringone (SyAC), and acetovanillone (VAC)) and photo-oxidation products of α-pinene (cis-pinonic acid and pinic acid), the same HPLC-MS/MS system as used for amino acid determination was employed. Both separations used a ZORBAX Extend-C18 column (150 mm × 4.6 mm, 3.5 µm, Agilent) with a 0.01 % formic acid aqueous solution (eluent A) and a solution of methanol / acetonitrile 80 / 20 (eluent B) as mobile phases and a flow rate of 0.5 mL min−1. The injection volume was 100 µL, and the ESI source operated in negative mode. The binary elution programs for phenolic compounds, pinonic acids, and pinic acids were reported in Zangrando et al. (2013) and Feltracco et al. (2018).
The other half of the filter was microwave-digested (ETHOS-1, Milestone), with 6 mL HNO3, 3 mL H2O2, and 1 mL HF (ROMIL-UpA) in PFA high-pressure digestion vessels. The digestion temperature program consisted of a ramp from room temperature to 190 °C in 25 min, after which this value was maintained for 15 min. One or two blank samples per digestion batch containing the same amount of the individual acids were processed during sample digestion. The analysis of 27 TEs (Li, Be, Mg, K, Ca, V, Cr, Mn, Fe, Co, Ni, Cu, Zn, Ga, As, Y, Rb, Sr, Ag, Cd, Cs, Ba, Tl, Pb, Bi, U, and Th) and 14 REEs (La, Ce, Pr, Nd, Sm, Eu, Gd, Tb, Dy, Ho, Er, Tm, Yb, and Lu) was performed by ICP-SFMS (Element XR, Thermo Scientific, Bremen) following the method described by Turetta et al. (2021)
2.3 Source apportionment using positive matrix factorization (PMF)
To gain a comprehensive understanding of the complete dataset and acquire insights from the high number of species investigated in this paper, we employed the positive matrix factorization (PMF) method developed by Paatero and Tapper (1994). The primary objective of utilizing the PMF technique was to determine the count of underlying factors or sources, their chemical compositions, and the corresponding mass contributions. These factors/sources played a role in shaping the observed PM concentrations. For this study, we utilized the EPA PMF 5.0 code, which applies the Multilinear Engine (ME-2) for its implementation.
In this study, we examined a dataset comprising the concentrations of identified chemical species (n=37), PM10 mass concentration, and 85 aerosol samples. The number of species was reduced, considering that some species have the same source: for example, the sum of free L-amino acids, D- amino acids, and photodegradation products of α-pinene (pinic acid and pinonic acid) was considered for the biogenic sources; the sum of phenolic compounds described the biomass-burning input; the sum of carboxylic acids described the secondary organic aerosols; some sugars were included; and only some TEs and REEs were included in the PMF to avoid introducing noise in the model. Magnesium and sodium were included as ionic and total species because these elements have different sources. These ranges were treated as independent samples of the same species (, where n represents each chemical species and m represents the number of cases or samples). The uncertainties of each sample concentration were defined using the relative standard deviations for each variable during the validation process and using method detection limits obtained by standard deviations of field blanks. To calculate the uncertainty matrix, the formula reported in the PMF 5.0 guide, an additional 10 % modeling uncertainty was applied during the elaboration. All variables are considered “strong” except for photodegradation products of α-pinene, which are considered “weak” because their signal-to-noise ratios were lower than 2.
To determine the uncertainties in the PMF outcomes, the bootstrap method was employed. The classification of the chemical species was carried out using the signal-to-noise criteria () as outlined by Paatero and Hopke (2003). Moreover, we also utilized the percentage of data surpassing the detection limit as a supplementary criterion, following the approach introduced by Amato et al. (2016). This combined analysis underscored the robust nature of all the identified chemical species.
2.4 Air mass back trajectories
To understand the long-range transport pattern of air masses recorded at the sampling site, we conducted a new back trajectory analysis every 6 h over a 7 d period. The elevation setup of the starting location was regarded as the elevation of the site (2543 m a.s.l), plus an extra 1000 m to avoid problems from the surrounding orography. The HYSPLIT model from the NOAA ARL was applied, using the GDAS 1-degree meteorological database (Draxler, 1998). Based on the backward particle release simulation, the cluster aggregation was displayed for each 4 d sampling period, considering the total spatial variance.
3.1 Seasonal trend of PM10 at the alpine site of Col Margherita
Mass concentrations of PM10 for a full year were evaluated at the high-altitude alpine site of Col Margherita (Fig. 1). The concentrations ranged between 0.5 and 180 µg m−3, with a median value of 6 µg m−3. A huge concentration spike of 180 µg m−3 (sample between 15 and 19 March) was found due to the intrusion of an air mass containing Saharan dust. The 5 d back trajectories (Fig. S2) show the air masses came from the Sahara and crossed Spain and crossed France at low altitude, before arriving at the MRG sampling site.
Higher mean concentrations were found during astronomical summer 2021 (7±4 µg m−3), spring 2022 (9±5 µg m−3), and summer 2022 (12±6 µg m−3) than those during fall 2021 and winter 2021–2022, where the mean concentrations were 3±3 µg m−3 and 5±3 µg m−3, respectively. This astronomical seasonal trend with higher values during the spring and summer seasons was also found in more than 20 years of measurements at the Jungfraujoch Research Station (Bukowiecki et al., 2016) in the western Swiss Alps (3580 m a.s.l.) and during 1998–2011 at the Mt. Cimone observatory (Tositti et al., 2013) in the Italian northern Apennines (2165 m a.s.l.).
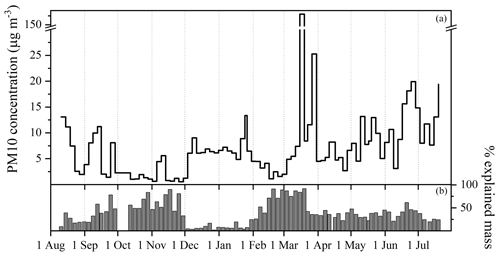
Figure 1(a) Annual trend of PM10 mass concentrations at MRG from August 2021 to July 2022. (b) Percentages of explained mass obtained by the comparison of the sum of analytes determined in filters with the mass concentration.
This well-known seasonal trend was confirmed in our year of measurements at MRG. It is due to a combination of several atmospheric processes: thermal convection, a mountain–valley breeze regime, and upward motion due to mixed-layer expansion. The effect of valley breezes was evaluated in our recent publication (Feltracco et al., 2022), demonstrating that wind typically arises in the late morning of warm-season days (July and August) and then is channeled upward to the MRG site, interacting with the local up-valley flow. This effect is much less present during the cold season. However, at Jungfraujoch (Bukowiecki et al., 2016), vertical transport of the planet boundary layer (PBL) was found to be mostly responsible for this seasonality, thanks to observations of in situ aerosol parameters and of the aerosol optical depth (Ingold et al., 2001). The impact of the PBL was evaluated at MRG using ERA5 (Vardè et al., 2022) during different seasons in 2018–2019, finding a diurnal variability with the lowest PBL height in winter.
Figure 1b underlines the percentage of PM10 mass concentration explained through the analysis of all species considered in this study. During the spring, around 50 % of the PM10 is explained, while the PM10 is well characterized in February–March with values close to 100%. In December and January, the percentages of explained mass were found to be between 4 % and 25 %, and in the next section a cationic deficit is demonstrated, probably due to the presence of hydrogen cations and then an acidic atmosphere.
3.2 Chemical composition
This paper shows for the first time an extended chemical characterization with 100 species of atmospheric aerosols at a high-altitude sampling site.
Sodium, calcium, and magnesium are three elements determined with two different analytical methods: the data obtained by ion chromatography coupled with a conductivity detector reflect the concentration of the ionic species of the element in the water-soluble fraction, while the concentrations of the same elements determined from samples extracted in acid media and analyzed using ICP-SFMS describes the total concentration of these elements, including a portion of the insoluble fraction. In general, the mean percentages of ionic sodium, magnesium, and calcium are 54±45 %, 29±27 %, and 65±45 %, respectively, compared to the total elements (Fig. S3). This explains why the concentrations obtained by ICP-SFMS are noticeably higher than the ionic fractions. The crustal particles are dissolved with acids, and any fine particles present below an aerodynamic diameter of 8 µm act as a solution aerosol in the spray chamber and plasma and are atomized in the plasma of ICP-SFMS (Goodall et al., 1993; Tong and Guo, 2019).
A comparison of the chemical compositions of each season is reported in Fig. 2 by considering the total Na, Ca, and Mg, as analyzed with ICP-SFMS, and excluding the samples contaminated by the Saharan dust event (SDE). Sulfate and nitrate are the most abundant species in the alpine aerosols that were collected each season, and other crustal elements, such as Al, Ca, Na, Mg, and Fe, were also abundant and represented together with the anions mentioned above, comprising the majority of the chemical species in the aerosols collected. These abundances are consistent with the chemical composition of aerosols described at Jungfraujoch station (Cozic et al., 2008), although for a much smaller number of species (mainly major ions, organic material, and black carbon). As shown in Fig. 2, some trace elements such as Fe and Al, which appear in crustal sources together with Ca, are especially abundant components of these aerosols during the seasons without snow cover.
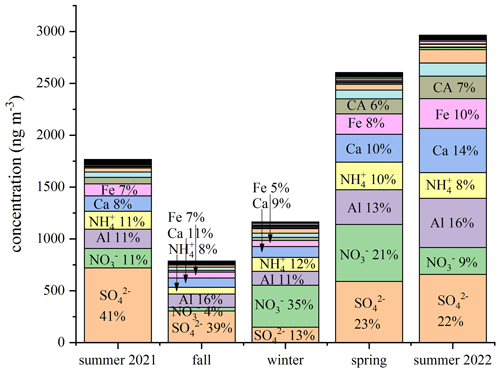
Figure 2Comparison of the chemical compositions of aerosols collected from August 2021 to July 2022 at the Col Margherita Observatory in different seasons.
3.2.1 Ionic composition and ionic balance
Ionic species represent a major percentage of the total chemical composition (Fig. 2). The highest concentrations are found in spring and summer, while low ion concentrations are detected during the snow season. Sulfate, ammonium, and nitrate are the most abundant species in the aerosol samples collected in the Eastern Alps at MRG, as also found at the Jungfraujoch (Western Alps) (Bukowiecki et al., 2016). As demonstrated by Cozic et al. (2008), sulfate and nitrate are neutralized by ammonia. Figure 3 reports the equivalent concentrations of each ion over the entire year and the equivalent sum of the analyzed cations / anions ratio. From November to February, the total anion concentration exceeded the total cation concentration (neutral situation with a ratio of 1), meaning the aerosols were probably acidic due to a significant (unmeasured) concentration of hydrogen cations present in the aqueous solution associated with the anions in the aerosols. Aerosol acidity depends on a strong acid content, mainly from sulfuric and nitric acids, whose precursors occur in both gaseous and aqueous phases (Squizzato et al., 2013). On the contrary, during the spring–summer period, an anion deficit in our measurements is recorded, plausibly due to the presence of carbonate (an unmeasured anion) emitted in the atmosphere by the erosion process of Dolomite rocks (likely CaMg(CO3)2).
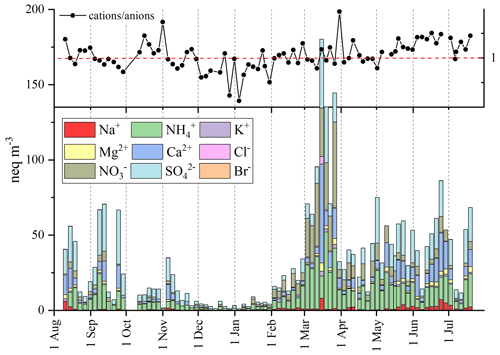
Figure 3Ion concentrations (expressed in neq m−3) determined in the annual aerosol sampling from August 2021 to the end of July 2022 at the Col Margherita Observatory.
Sulfate is one of the most concentrated ionic species in MRG aerosols for the entire sampling campaign with concentrations between 31 ng m−3 and 2 µg m−3. Although sulfate can be emitted into the atmosphere by sea salt or produced by the oxidation of dimethyl sulfide (DMS) released from marine algae (Gondwe et al., 2003), the anthropogenic input from the oxidation of SO2 has already been confirmed as the main source of sulfate in atmospheric aerosols collected at MRG (Barbaro et al., 2020). The mean anthropogenic contribution ex SO, obtained by excluding sea-salt sulfate and mineral dust sulfate contributions (Schwikowski et al., 1999), was 92±6 %, demonstrating that the other sources are minor but present. For example, biogenic sources were confirmed by the significant correlation (R person 0.61, p value < 0.05) between non-sea-salt SO and methanesulfonate (MSA) and by the seasonal trends (Fig. S4) and higher concentrations during the summer period. The reasons for this are ascribed to the presence of biogenic inputs (Els et al., 2020) and to the valley breeze and PBL impacts on the PM10 concentrations (as previously discussed).
Nitrate is another very abundant ion in the MRG aerosol samples. The nitrate-to-sulfate ratio (r) in equivalents is comparable (Fig. 4) with other data in different environments. At MRG, the r average value is 0.3±0.2, except for the period between 16 January and 26 March when the mean value was 2±1 (Fig. 4). This ratio is strongly linked to distance from emission sources. Henning et al. (2003) compared the r value at Jungfraujoch (r=0.2) with r=0.7 at an urban site in Zurich (Hueglin et al., 2005). Other r values reported are those of r=1.1 in Milan (Putaud et al., 2002) and r=1.6 for a polluted coastal area of England (Yeatman et al., 2001). In general, remote areas or high mountain sites (Huebert et al., 1998; Preunkert et al., 2002; Shrestha et al., 1997) show values similar to those found at MRG, except for during January–March. These low values are likely due to a faster oxidation of NOx than SO2, leading to r values that are higher close to the sources. The higher values found at MRG mainly in the winter period could plausibly be due to domestic heating and to local emissions of NOx from increased road traffic linked to tourism.
3.2.2 Secondary aerosols: carboxylic acids and the photo-oxidation products of α-pinene
The annual trend of 12 organic acids (C2-acetic, C2-glycolic, C2-oxalic, C3-malonic, C4-succinic, cis-C4-maleic, trans-C4-fumaric, hC4-malic, C5-glutaric, C6-adipic, pinonic, and pinic acids) showed that the concentrations in spring (150±107 ng m−3) and in summer (70±61 ng m−3 for 2021 and 222±124 ng m−3 for 2022) were higher than in fall (22±19 ng m−3) and in winter (32±41 ng m−3), probably due to an improvement of solar radiation and then of atmospheric photo-reactivity. Many organic acids are not found in the cold period, while only pinonic and pinic acids, the photo-oxidation products of α-pinene, are found in these seasons (Fig. S5). The concentrations of the spring and summer periods are consistent with those previously found at MRG during 2018 (Barbaro et al., 2020) and with the summer mean concentrations found at Sonnblick (Austria; 30 ng m−3) and at Vallot (French Alps; 67–82 ng m−3) (Legrand et al., 2007). During winter, the reduced concentrations of total C2–C5 acids were also found by Legrand et al. (2007) at six sites along a west–east transect of 4000 km across Europe, considering rural and mountain sites. They found higher concentrations in winter when the surrounding local and regional sources were dominant, while the summer concentrations came from larger-scale emissions.
The smaller organic acids are the most abundant: C2-oxalic acid shows a mean percentage of 36 % all year round, followed by C3-malonic (15 %), C2-glycolic (14 %), C2-formic (10 %), and hC4-malic (7 %) acids. The other carboxylic acids were found with percentage abundances of <5 %.
Carboxylic acids can be emitted by primary sources, such as biomass burning (Falkovich et al., 2005), fossil fuel combustion (Wang et al., 2006), vehicular exhaust (Kawamura and Kaplan, 1987), and marine input (Rinaldi et al., 2011), but these species are also produced by oxidation reactions in the atmosphere (Kawamura et al., 1996). Instead, pinonic and pinic acids are secondary products of α-pinene, the most important monoterpene released by biogenic sources. Kawamura et al. (2016) reviewed the main sources and transformations, suggesting the significance of atmospheric photochemical oxidation processing with a similar temporal trend with solar irradiation and ambient temperatures. It is confirmed by our results where the higher concentrations are found during the spring and summer seasons, while during winter the lowest concentrations are found (Fig. S5).
Some diagnostic ratios of dicarboxylic acids can be used to define the photochemical aging of air masses. For example, the C3-malonic acid / C4-succinic acid ratio is commonly used because C4-succinic acid can be degraded to C3-malonic acid by decarboxylation reactions activated by OH radicals (Fu et al., 2013). At MRG, we found the C3-to-C4 ratio ranged between 1 and 52, thus excluding primary emissions as the main sources. Typical values observed for vehicular emissions ranged between 0.6 and 2.9 (Kawamura and Ikushima, 1993), while values obtained during an intense biomass-burning period were always below 1 (Kundu et al., 2010). These data at MRG are consistent with previous values found during the 2018 spring–summer campaign, where we hypothesized a photochemical production of these species at this mountain site (Barbaro et al., 2020).
Another diagnostic ratio is the cis-C4-maleic acid / trans-C4-fumaric acid ratio because ambient photochemical processes can affect their conversion. In our data, cis-C4-maleic acid concentrations are always higher than those of trans-C4-fumaric acid, with a mean ratio of 3 (range 0.2–12), suggesting low photo-isomerization from cis-C4-maleic acid to trans-C4-fumaric acid and then low aerosol aging (Kawamura and Sakaguchi, 1999).
Oxidative processes are also involved in the formation of pinonic and pinic acids. These species showed a higher total concentration during spring (6 ± 3 ng m−3) and summer (8 ± 2 ng m−3 for 2021 and 7 ± 2 ng m−3 for 2022) with respect to fall (5 ± 4 ng m−3) and winter (4 ± 2 ng m−3). These species reflect the vicinity of conifers as sources (Haque et al., 2016) and are linked to the photo-oxidation processes in the atmosphere which are more active during the summer period (Librando and Tringali, 2005), as is also found for the other carboxylic acids.
3.2.3 Biomass-burning tracers: anhydrosugars and phenolic compounds
Biomass burning is an important primary source of many organic compounds and soot particulate matter. Several emission sources can produce a biomass-burning signal: wildfires, use of specific plant species as fuels, fossil fuel utilization, anthropogenic urban and industrial emissions from food preparation, and domestic heating (Simoneit, 2002). Each tree species is constituted of cellulose, lignin, and fillers. Cellulose is a long-chain linear polymer of glucose, and its burning specifically produces levoglucosan and its isomers (mannosan and galactosan). Hemicelluloses are a mixture of polysaccharides derived mainly from glucose, mannose, galactose, xylose, and arabinose, but the sugar composition varies widely among different tree species. Finally, lignin is a biopolymer with vanillyl, syringyl, and p-coumaryl moieties. The study of the composition of phenolic compounds derived from lignin can be used to differentiate between the different types of vegetation burned (Kuo et al., 2011).
Levoglucosan is one of the key tracers when investigating biomass-burning contributions to aerosols (Simoneit, 1999). At MRG, its concentration ranges between 0.1 and 48 ng m−3 with an annual mean value of 2 ng m−3. As shown in Fig. 5, the annual trends of all the biomass-burning tracers are deeply impacted by the Saharan dust event recorded in March. At MRG, excluding the samples affected by Saharan dust, the mean values were 1.2±0.4 ng m−3 and 1.3±0.9 ng m−3 for summer 2021 and 2022, respectively; 1.2±0.9 ng m−3 for fall 2021; 1.6±0.7 ng m−3 for winter; and ng m−3 for spring 2022. Values below 10 ng m−3 are consistent with high-level mountain sites such as Sonnblick (Austria; 3105 m a.s.l.), with an annual mean concentration of 7.8 ng m−3 (Puxbaum et al., 2007), or Alpe San Colombano (Italy; 2250 m a.s.l.), where the values range between 5 and 10 ng m−3 (Perrone et al., 2012). Considering the ratio between winter and summer concentrations of levoglucosan, Puxbaum et al. (2007) found a relationship between this ratio and different sites, with values of around 40 for continental rural sites and a value of 3 for a maritime background site. At MRG, the winter-to-summer ratio of 1.3 is aligned with elevated sites because low ratios can be explained by valley breeze effects, although it can be assumed that there is considerable consumption of biomass fuels in the mountains. During winter, the mixing height in alpine valleys is low, and therefore the atmosphere at mountain peaks is effectively decoupled from the lower sites. In summer, elevated sites receive air masses from lower levels, and the elevational gradient becomes less steep.
Levoglucosan and its isomers are usually major water-soluble organic components in atmospheric aerosols impacted by wood smoke, but smoke aerosols can also be strongly enriched (by factors of 2–5) in the monosaccharides: glucose, arabinose, galactose, and mannose (Medeiros et al., 2006). In particular, glucose is present at higher levels because it is present in vascular plants, explaining the enrichment of those sugars in smoke-impacted aerosols (Cowie and Hedges, 1984). The samples collected at MRG in fall and winter are clearly impacted by biomass burning, as shown in the panel related to the glucose-to-levoglucosan ratio in Fig. 4. In fact, Medeiros et al. (2006) reported a glucose / levoglucosan ratio of about 4.5 for smoke-free samples and a considerably lower value of 0.9 for smoke samples. On the contrary, during spring and summer the ratio went beyond the smoke-free threshold, indicating a natural input as the most probable source. It is plausible that this is also due to domestic heating with wood in the mountain areas.
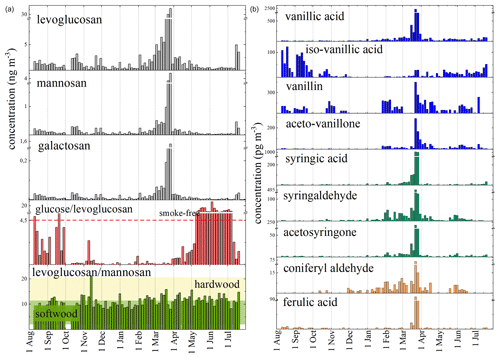
Figure 4Seasonal trend of concentrations of levoglucosan and its isomers and phenolic compounds. Two discriminator ratios are reported in the panels: glucose to levoglucosan with the threshold of smoke-free samples (Medeiros et al., 2006) and levoglucosan to mannosan with the range of different biomass fuels (Fabbri et al., 2009).
The diagnostic ratio between levoglucosan and mannosan can also help to define the type of vegetation burned. Fabbri et al. (2009) proposed this discrimination ratio after analyzing different types of plants and their levoglucosan-to-mannosan ratios. Ratios of 13.8–22 and 0.6–13.8 are indicative of hardwood and softwood combustion, respectively, while values > 24 indicate grass fires. Figure 5 shows that at MRG softwood is the main combustion fuel. This is also confirmed by the presence of mainly vanillyl species such as vanillic acid, vanillin, isovanillic acid, and acetovanillone, typical compounds present in softwood (Fig. 4). An increasing concentration of all types of phenolic compounds is found in the samples affected by Saharan dust, suggesting a mix of burned vegetation. The increase in all biomass-burning tracers in the Saharan dust particles is due to atmospheric long-range transport (Fig. S2) of air masses over Spain and France, where domestic heating with wood (and coal) in small private stoves can produce this type of signal. The only phenolic compound without a peak related to Saharan dust is isovanillic acid, and its trend is similar to those of the organic acids (Fig. 4). This suggests a photochemical source for this compound that is not correlated with biomass-burning sources.
3.2.4 Biogenic aerosols: saccharides, sugar alcohols, and free amino acids
Primary biological aerosol particles (PBAPs) can be defined as solid airborne particles that are directly emitted by the biosphere into the atmosphere (Després et al., 2012). PBAPs are likely to be a major source of proteinaceous materials in the atmosphere (Matos et al., 2016). They can be emitted as biological aerosols such as viruses, algae, fungi, bacteria, spores and pollen, and fragments of plants and insects, and they also can be associated with anthropogenic sources such as industry, agricultural practices, and wastewater treatment plants (Lazaridis, 2008).
Free amino acids and saccharides have been traditionally used for the identification of particles of biological origin (Bauer et al., 2008; Matos et al., 2016; Ruiz-Jimenez et al., 2021). A total of 17 free L- and D-amino acids (L-Ala, D-Ala, L-Arg, L-Asn, L-Asp, D-Asp, Gly, L-Glu, L-Leu, L-Hys, L-Hyp, L-Phe, D-Phe, L-Pro, L-Thr, L-Tyr, and L-Val) were found in the MRG samples (Fig. 6). The total mean concentrations were 4±2 and 5±1 ng m−3 in summer 2021 and 2022, respectively; 0.9±0.7 ng m−3 in fall; 0.6±0.8 ng m−3 in winter; and 3±1 ng m−3 in spring. As also shown in Fig. 5, higher concentrations of free L- and D-amino acids were found in summer, and, while only free L-amino acids were abundant in the spring, D-amino acids increased in concentration in the late spring. The concentration values are consistent with those found in PM10 in the Arctic (Feltracco et al., 2021a; Scalabrin et al., 2012) and in the Antarctic (Barbaro et al., 2015a; Feltracco et al., 2023) but are lower than typical values found at rural (Mace, 2003) or urban sites (Di Filippo et al., 2014; Zangrando et al., 2016).
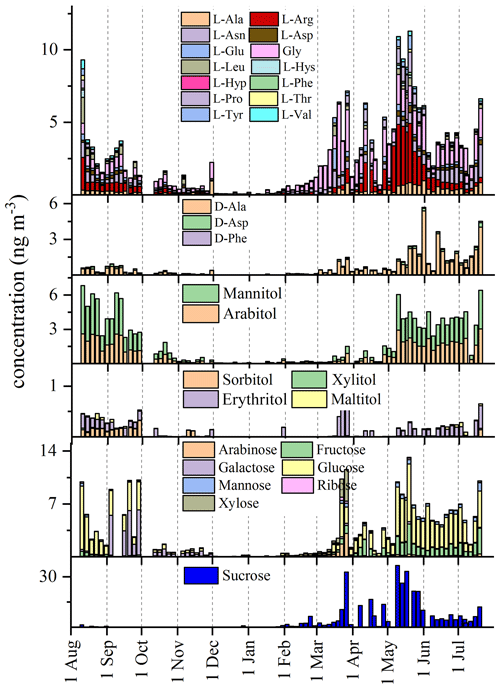
Figure 5Seasonal variations in free L- and D-amino acids and sugars in the aerosols collected at MRG from August 2021 to the end of July 2022.
A total of seven monosaccharides (arabinose, fructose, galactose, glucose, mannose, ribose, and xylose); six sugar alcohols (arabitol, mannitol, ribitol, iso-erythritol, sorbitol, and xylitol); and one disaccharide, sucrose, were found in the MRG aerosols from August 2021 to the end of July 2022. The highest concentrations were determined in spring and summer (Fig. 5), with the total mean concentrations of sugars at 9±4 ng m−3 and 14±8 ng m−3 for the summers of 2021 and 2022, respectively, and 17±14 ng m−3 for spring, while concentrations fell to 2±3 ng m−3 and 1±2 ng m−3 in fall and winter, respectively. Mannitol and arabitol are shown in another panel of Fig. 5 because these species are specific tracers of fungal spores (Bauer et al., 2008), and they seem to have another trend with significant concentrations from May to October. Glucose and other monosaccharides seem to be weakly affected by the Saharan dust, while other biogenic species (free amino acids and sugars; Fig. 5) are not affected by the Saharan dust event at all, suggesting that long-range transport should be a negligible source compared to local emissions in the mountain environment.
3.2.5 Elemental composition and possible tracer of aerosol sources
This study employs trace-element enrichment factors (EFs) to assess the contribution of non-natural or anthropogenic sources to elemental concentrations. A fuller and more detailed explanation is given in the Supplement. The enrichment factor (EFi) has been calculated using the ratio of an element i to a reference element j in the atmosphere and is compared to the same ratio in the upper crust. Marine enrichment factors (MEFs) are also calculated relative to seawater concentrations. An EF of > 1 indicates non-crustal origin, with higher values signifying greater enrichment. The dominant sources for various elements are assessed based on EF values, revealing geogenic origins for several elements, while others show non-geogenic sources, especially during certain seasons.
The research explores multiple sources of aerosols at a high-elevation station. Principal sources include local crustal contributions; long-range transport of Saharan dust; sea-salt spray from the Mediterranean; biomass combustion (fires or domestic heating); and, to a lesser extent, traffic, refinery emissions, and oil combustion. Ternary diagrams of rare earth elements in the Supplement help us to identify different sources in various seasons. The Mg distribution is analyzed to distinguish between crustal and sea-salt spray origins, with peaks indicating marine or Saharan events. Other elements like Ti, Mn, and Mo further support the evidence of North African contributions.
This study also considers biomass burning, distinguishing between types of fires using rare earth elements Eu, Ho, and Yb. Ternary diagrams involving V, La, and Ce help us recognize aerosols from anthropogenic sources such as traffic/refinery and oil combustion processes. The analysis of the MRG aerosol samples suggests a predominant crustal source for V, but distinct groups of samples indicate potential contributions from different anthropogenic sources, specifically traffic/refinery and oil combustion processes.
3.3 Source apportionment
A source apportionment approach using positive matrix factorization yielded a reasonable resolution employing four factors. To ascertain the most suitable number of factors possessing meaningful physical implications, an evaluation was conducted using parameters IM (representing the maximum individual column mean) and IS (indicating the maximum individual column standard deviation), both derived from the scaled residual matrix. This analysis was supplemented by considering Q values (a parameter assessing the goodness of fit) (Viana et al., 2008). The analysis of the scaled residual is symmetrically distributed for almost all variables, meaning that the model is able to fit each chemical species quite well. Considering the plotting of modeled data obtained by PMF and the observed data, R2 values above 0.9 were often obtained for the considered species (Table S1 in the Supplement). Some exceptions are recognized: for example, levoglucosan, the key tracer of biomass burning, is well reconstructed during the entire sampling period, but its high concentration during the intense Saharan dust event of March is not recognized, probably because this concentration is not correlated with the Saharan source but with transport over contaminated areas (Spain and France). In general, the model is able to reasonably reconstruct the observed concentrations with a slope of 0.977 and an R2 of 0.97 considering the entire dataset, but the concentrations related to the intense Saharan dust event of March forced the linearity. Considering the dataset without this point, the linearity is preserved with a slope of 0.897 and an R2 of 0.84 (Fig. S12).
In Fig. 6 the profiles of each factor are reported in terms of absolute and relative concentrations, with the relative contribution of the factors. The error bars represent the standard deviations of the bootstrap runs. Figure S12 reports the contribution in terms of concentrations of each factor considering the entire sampling period. Moreover, to define the source of these contributions, a comparison with air mass back trajectories is also shown in terms of percentage calculated by cluster means obtained for each sample (Fig. S13).
The first factor describes 41 % of the PM10, and it is mainly characterized by crustal elements, such as Mg and Ca, but also by some trace elements and rare earth elements (Fig. 6). This attribution is also confirmed by the presence of air masses coming from North Africa, also defined using back trajectories. Although the most intense Saharan dust event is recognized in the sample from 15–19 March, zooming into the factor contributions, by putting a break in the y axes, reveals the other Saharan dust events that were detectable at the Col Margherita Observatory.
The second factor accounted for only 11 % of the total PM10 concentration, and it mainly loads with Mo and U, although there are also some rare earth elements (Ho and Yb) typically associated with atmospheric long-range transport, comprising above 25 % of the total PM10 concentration (Fig. 6). Considering the back trajectories (Figs. 7 and S13), the annual trend of contributions of this factor seems to be related to the intrusion of air masses coming from the Atlantic Ocean and from the Atlantic Ocean across to North America. Coupling this evidence with our knowledge about Mo and U possible origins (Aciego et al., 2015; Flett et al., 2021; Masson et al., 2015; Qi et al., 2016; Wong et al., 2021, 2020b) and with the EF's indications, we can hypothesize long-range transport from polluted areas with a further enrichment when oceanic air masses pass over the industrialized areas of central northern Europe.
The third factor (Fig. 6), accounting for 29 % of the PM10 concentration, was identified as biogenic sources because it is characterized by the major ions of saline components and water-soluble organic compounds, such as carboxylic acids (CA), free L- and D-amino acids (L-FAA and D-FAA), and mannitol, which are key tracers of fungal spores, glucose, and sugars with a typical biogenic input. Moreover, the presence of CA suggested that in this factor the contribution of secondary organic aerosols is also included. The seasonal trend of this factor (Fig. 7) showed higher concentrations during the warm season, linked to an increase in biogenic activities but also to seasonal effects due to (1) valley breeze and (2) planetary boundary layer (PBL) changes. Previous studies at MRG already demonstrated the increase in PM10 and mercury concentrations during summer (Feltracco et al., 2022; Vardè et al., 2022).
Finally, the fourth factor, which describes 19 % of PM10, is mainly defined by levoglucosan and phenolic compounds, suggesting biomass-burning sources, and some heavy metals such as Pb, V, and Ag, adding an anthropogenic source (Fig. 6). This factor showed the highest concentrations between February and April when the air masses mainly come from Europe without any marine input. The correspondence of contributions of this factor with air masses clearly suggests the impact of urban areas on air quality. Although the evaluation of ternary diagrams involving V, La, and Ce (see the Supplement) also suggested the presence of anthropogenic sources such as traffic/refinery and oil combustion processes, the PMF model skipped these sources, probably because it is negligible compared to the other described sources.
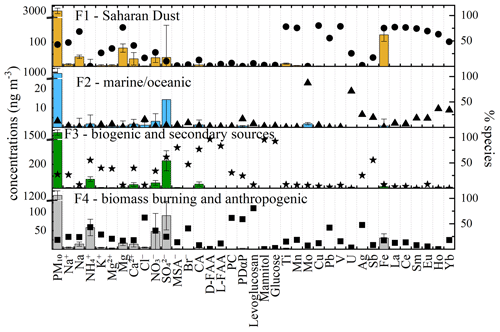
Figure 6Source profiles obtained with PMF. The bars identify the species in terms of concentrations that mainly characterize each factor profile. Error bars were obtained with the bootstrap method. The points describe the species in terms of relative concentrations. PC is phenolic compounds, CA is carboxylic acids, FAA is free amino acids, and PDαP is photodegradation products of α-pinene.
A study of the chemical composition of atmospheric aerosols from an entire year (August 2021–July 2022) was carried out at the Col Margherita Atmospheric Observatory, a high-altitude background site in the eastern Italian Alps. For the first time, more than 100 chemical markers from a whole year's worth of samples were determined, including major ions, organic acids, sugars, free amino acids, trace elements, and rare earth elements.
The main aims of this study were to define (i) the main sources of aerosols arriving at this remote site and (ii) background values for the chemical markers when describing the aerosol inputs to this remote area. To the best of our knowledge, information on high-mountain aerosols is limited to major ions, some trace elements, or some organic compounds.
For the first time, intrusions of air masses impacted by Saharan dust events are demonstrated and chemically characterized. Each class of analytes is discussed, considering these chemical species as specific markers of sources or processes. Sulfate and nitrate are the most abundant species for the entire sampling period, but the crustal elements (Ca, Mg, and Fe) are an important component of alpine aerosols. Using specific diagnostic ratios, the anthropogenic inputs of sulfate and nitrate were demonstrated. Photochemical processes are also recognized using carboxylic acids and photo-oxidation products of α-pinene, which showed high concentrations when irradiation was higher.
Levoglucosan and phenolic compounds have allowed us to define the different types of biomass burning that occurred, distinguishing the contribution coming from domestic heating in European cities and that coming from air masses transported though Saharan dust events. The biogenic input was characterized by using some water-soluble organic compounds, such as free amino acids and sugars. For example, the presence of specific tracers of fungal spores such as mannitol and arabitol is mainly recognized during spring and summer, as clearly expected.
Enrichment factors (EFs) for trace elements have proven invaluable in emphasizing the impact of sources beyond natural origins on elemental concentrations.
The dominant origin for elements like K, Na, Cs, Sr, Ti, Ca, V, Ba, Li, Mn, Rb, Co, Tl, Fe, Mg, and light REE is geogenic, except during the fall and winter seasons when a moderate increase in these elements is observable. On the other hand, rare earth elements and U exhibit significant fluctuations between geogenic and non-geogenic sources, particularly from fall 2021 to spring 2022. Elements such as Cr, Cu, Pb, Zn, Ni, Sb, and Cd primarily stem from non-geogenic sources across all seasons, although with occasional deviations, notably during both summer periods and winter phases. Ag and Mo consistently indicate a clear non-geogenic origin. In summary, our research employs various enrichment factors and elemental analyses to identify and differentiate sources of aerosols in the studied region, considering both natural and anthropogenic origins. The findings highlight the complexity of aerosol composition, with variations across seasons and contributions from multiple sources.
A source apportionment using positive matrix factorization was performed, obtaining four factors: (1) Saharan dust events, (2) long-range marine/anthropogenic input, (3) biogenic sources, and (4) biomass-burning and anthropogenic emissions.
We can conclude that, despite the remoteness of the Col Margherita site, anthropogenic pollution, from both regional and long-range transport, impacts this area.
The data that support the findings of this study are available from the corresponding author upon request.
The supplement related to this article is available online at: https://doi.org/10.5194/acp-24-2821-2024-supplement.
EB: conceptualization, investigation, formal analysis, data curation, writing (original draft), writing (review and editing). MF: conceptualization, investigation, formal analysis, data curation, writing (original draft). FDB: investigation, data curation, writing (original draft). CT: investigation, formal analysis, data curation, writing (original draft), writing (review and editing). MR: formal analysis, writing (review and editing). WC: investigation, writing (review and editing). GC: investigation, writing (review and editing). GM: investigation, writing (review and editing). MR: formal analysis, writing (review and editing). JG: investigation, supervision, writing (review and editing). CB: investigation, supervision, writing (review and editing). AG: investigation, supervision, writing (review and editing).
The contact author has declared that none of the authors has any competing interests.
Publisher’s note: Copernicus Publications remains neutral with regard to jurisdictional claims made in the text, published maps, institutional affiliations, or any other geographical representation in this paper. While Copernicus Publications makes every effort to include appropriate place names, the final responsibility lies with the authors.
This study was carried out within the “Interconnected Nord-Est Innovation Ecosystem (iNEST)” project and received funding from the European Union NextGenerationEU National Recovery and Resilience Plan (NRRP), Mission 4, Component 2, investment no. ECS00000043, CUP no. H43C22000540006. This article reflects only the authors' views and opinions, and neither the European Union nor the European Commission can be considered responsible for them.
This work has benefited from the infrastructural support of the Centre for Trace Analysis (CeTrA) of Ca' Foscari University through the project IR0000032, Italian Integrated Environmental Research Infrastructures System (ITINERIS), funded by the EU: NextGenerationEU, PNRR, Mission 4 (“Education and Research”), Component 2 (“From research to business”), Investment 3.1 (“Fund for the realization of an integrated system of research and innovation infrastructures”).
The results are also produced in the framework DECIPHER – Disentangling mechanisms controlling atmospheric transport and mixing processes over mountain areas at different space- and timescales (grant no. 2022NEWP4J) funded by PRIN MUR.
The authors thank ELGA LabWater, High Wycombe, UK, for supplying the pure water systems used in this study. A special thanks goes to the personnel of the Ski Area San Pellegrino: in particular, the director Renzo Minella, the head of the cable car service, and the technicians for their invaluable help and cooperation during the sampling activities at the observatory.
This publication was supported by the European Union - Next Generation EU (project no. ECS000043) – Innovation Ecosystem Program “Interconnected Northeast Innovation Ecosystem (iNEST)”. This work has also benefited from the infrastructural support of the Centre for Trace Analysis (CeTrA) of Ca' Foscari University (project no. IR0000032) – ITINERIS, Italian Integrated Environmental Research Infrastructures System, funded by EU – Next Generation EU, PNRR – Mission 4 “Education and Research” – Component 2: “From research to business” – Investment 3.1: “Fund for the realisation of an integrated system of research and innovation infrastructures”. Finally, the study was also supported by the Ministero dell'Università e della Ricerca by PRIN (project no. 2022NEWP4J) “Disentangling mechanisms controlling atmospheric transport and mixing processes over mountain areas at different space- and timescales” (DECIPHER).
This paper was edited by Radovan Krejci and reviewed by two anonymous referees.
Aciego, S. M., Aarons, S. M., and Sims, K. W. W.: The uranium-isotopic composition of Saharan dust collected over the central Atlantic Ocean, Aeol. Res., 17, 61–66, https://doi.org/10.1016/j.aeolia.2015.01.003, 2015.
Amato, F., Alastuey, A., Karanasiou, A., Lucarelli, F., Nava, S., Calzolai, G., Severi, M., Becagli, S., Gianelle, V. L., Colombi, C., Alves, C., Custódio, D., Nunes, T., Cerqueira, M., Pio, C., Eleftheriadis, K., Diapouli, E., Reche, C., Minguillón, M. C., Manousakas, M.-I., Maggos, T., Vratolis, S., Harrison, R. M., and Querol, X.: AIRUSE-LIFE+: a harmonized PM speciation and source apportionment in five southern European cities, Atmos. Chem. Phys., 16, 3289–3309, https://doi.org/10.5194/acp-16-3289-2016, 2016.
Arsene, C., Olariu, R. I., Zarmpas, P., Kanakidou, M., and Mihalopoulos, N.: Ion composition of coarse and fine particles in Iasi, north-eastern Romania: Implications for aerosols chemistry in the area, Atmos. Environ., 45, 906–916, https://doi.org/10.1016/j.atmosenv.2010.11.013, 2011.
Barbaro, E., Zangrando, R., Vecchiato, M., Piazza, R., Cairns, W. R. L., Capodaglio, G., Barbante, C., and Gambaro, A.: Free amino acids in Antarctic aerosol: potential markers for the evolution and fate of marine aerosol, Atmos. Chem. Phys., 15, 5457–5469, https://doi.org/10.5194/acp-15-5457-2015, 2015a.
Barbaro, E., Kirchgeorg, T., Zangrando, R., Vecchiato, M., Piazza, R., Barbante, C., and Garnbaro, A.: Sugars in Antarctic aerosol, Atmos. Environ., 118, 135–144, https://doi.org/10.1016/j.atmosenv.2015.07.047, 2015b.
Barbaro, E., Morabito, E., Gregoris, E., Feltracco, M., Gabrieli, J., Vardè, M., Cairns, W. R. L., Dallo, F., De Blasi, F., Zangrando, R., Barbante, C., and Gambaro, A.: Col Margherita Observatory: A background site in the Eastern Italian Alps for investigating the chemical composition of atmospheric aerosols, Atmos. Environ., 221, ID117071, https://doi.org/10.1016/j.atmosenv.2019.117071, 2020.
Bauer, H., Claeys, M., Vermeylen, R., Schueller, E., Weinke, G., Berger, A., and Puxbaum, H.: Arabitol and mannitol as tracers for the quantification of airborne fungal spores, Atmos. Environ., 42, 588–593, https://doi.org/10.1016/j.atmosenv.2007.10.013, 2008.
Bukowiecki, N., Weingartner, E., Gysel, M., Coen, M. C., Zieger, P., Herrmann, E., Steinbacher, M., Ga, H. W., and Baltensperger, U.: A review of more than 20 years of aerosol observation at the high altitude research station Jungfraujoch, Switzerland (3580 m asl), Aerosol and Air Quality Research, 16, 764–788, 2016.
Carturan, L., De Blasi, F., Cazorzi, F., Zoccatelli, D., Bonato, P., Borga, M., and Dalla Fontana, G.: Relevance and Scale Dependence of Hydrological Changes in Glacierized Catchments: Insights from Historical Data Series in the Eastern Italian Alps, Water, 11, 89, https://doi.org/10.3390/w11010089, 2019.
Cowie, G. L. and Hedges, J. I.: Carbohydrate sources in a coastal marine environment, Geochim. Cosmochim. Acta, 48, 2075–2087, https://doi.org/10.1016/0016-7037(84)90388-0, 1984.
Cozic, J., Verheggen, B., Weingartner, E., Crosier, J., Bower, K. N., Flynn, M., Coe, H., Henning, S., Steinbacher, M., Henne, S., Collaud Coen, M., Petzold, A., and Baltensperger, U.: Chemical composition of free tropospheric aerosol for PM1 and coarse mode at the high alpine site Jungfraujoch, Atmos. Chem. Phys., 8, 407–423, https://doi.org/10.5194/acp-8-407-2008, 2008.
Després, V. R., Huffman, J. A., Burrows, S. M., Hoose, C., Safatov, A. S., Buryak, G., Fröhlich-Nowoisky, J., Elbert, W., Andreae, M. O., Pöschl, U., and Jaenicke, R.: Primary biological aerosol particles in the atmosphere: a review, Tellus B, 64, 15598, https://doi.org/10.3402/tellusb.v64i0.15598, 2012.
Di Filippo, P., Pomata, D., Riccardi, C., Buiarelli, F., Gallo, V., and Quaranta, A.: Free and combined amino acids in size-segregated atmospheric aerosol samples, Atmos. Environ., 98, 179–189, https://doi.org/10.1016/j.atmosenv.2014.08.069, 2014.
Draxler, R. R.: An overview of the HYSPLIT_4 modelling system for trajectories, dispersion and deposition, Aust. Meteorol. Mag., 47, 295–308, 1998.
Dukes, D., Gonzales, H. B., Ravi, S., Grandstaff, D. E., Van Pelt, R. S., Li, J., Wang, G., and Sankey, J. B.: Quantifying Postfire Aeolian Sediment Transport Using Rare Earth Element Tracers, J. Geophys. Res.-Biogeosci., 123, 288–299, https://doi.org/10.1002/2017JG004284, 2018.
Els, N., Greilinger, M., Reisecker, M., Tignat-Perrier, R., Baumann-Stanzer, K., Kasper-Giebl, A., Sattler, B., and Larose, C.: Comparison of Bacterial and Fungal Composition and Their Chemical Interaction in Free Tropospheric Air and Snow Over an Entire Winter Season at Mount Sonnblick, Austria, Front. Microbiol., 11, 1–18, https://doi.org/10.3389/fmicb.2020.00980, 2020.
Fabbri, D., Torri, C., Simoneit, B. R. T., Marynowski, L., Rushdi, A. I., and Fabiańska, M. J.: Levoglucosan and other cellulose and lignin markers in emissions from burning of Miocene lignites, Atmos. Environ., 43, 2286–2295, https://doi.org/10.1016/j.atmosenv.2009.01.030, 2009.
Falkovich, A. H., Graber, E. R., Schkolnik, G., Rudich, Y., Maenhaut, W., and Artaxo, P.: Low molecular weight organic acids in aerosol particles from Rondônia, Brazil, during the biomass-burning, transition and wet periods, Atmos. Chem. Phys., 5, 781–797, https://doi.org/10.5194/acp-5-781-2005, 2005.
Feltracco, M., Barbaro, E., Contini, D., Zangrando, R., Toscano, G., Battistel, D., Barbante, C., and Gambaro, A.: Photo-oxidation products of α -pinene in coarse, fine and ultra fine aerosol: A new high sensitive HPLC-MS/MS method, Atmos. Environ., 180, 149–155, https://doi.org/10.1016/j.atmosenv.2018.02.052, 2018.
Feltracco, M., Barbaro, E., Hoppe, C. J. M., Wolf, K. K. E., Spolaor, A., Layton, R., Keuschnig, C., Barbante, C., Gambaro, A., and Larose, C.: Airborne bacteria and particulate chemistry capture Phytoplankton bloom dynamics in an Arctic fjord, Atmos. Environ., 256, 118458, https://doi.org/10.1016/j.atmosenv.2021.118458, 2021a.
Feltracco, M., Barbaro, E., Spolaor, A., Vecchiato, M., Callegaro, A., Burgay, F., Vardè, M., Maffezzoli, N., Dallo, F., Scoto, F., Zangrando, R., Barbante, C., and Gambaro, A.: Year-round measurements of size-segregated low molecular weight organic acids in Arctic aerosol, Sci. Total Environ., 763, 10, https://doi.org/10.1016/j.scitotenv.2020.142954, 2021b.
Feltracco, M., Barbaro, E., Maule, F., Bortolini, M., Gabrieli, J., De Blasi, F., Cairns, W. R., Dallo, F., Zangrando, R., and Barbante, C.: Airborne polar pesticides in rural and mountain sites of North-Eastern Italy: An emerging air quality issue, Environ. Pollut., 308, 119657, 2022.
Feltracco, M., Zangrando, R., Barbaro, E., Becagli, S., Park, K.-T., Vecchiato, M., Caiazzo, L., Traversi, R., Severi, M., Barbante, C., and Gambaro, A.: Characterization of free L- and D-amino acids in size-segregated background aerosols over the Ross Sea, Antarctica, Sci. Total Environ., 879, 163070, https://doi.org/10.1016/j.scitotenv.2023.163070, 2023.
Flett, L., McLeod, C. L., McCarty, J. L., Shaulis, B. J., Fain, J. J., and Krekeler, M. P. S.: Monitoring uranium mine pollution on Native American lands: Insights from tree bark particulate matter on the Spokane Reservation, Washington, USA, Environ. Res., 194, 110619, https://doi.org/10.1016/j.envres.2020.110619, 2021.
Flood, M. T.: Elemental Analysis of Species Specific Wood Ash: A Pyrogenic Factor in Soil Formation and Forest Succession for a Mixed Hardwood Forest of Northern New Jersey, Theses, Dissertations and Culminating Projects, Montclair State University, 277, https://digitalcommons.montclair.edu/etd/277 (last access: 1 March 2024), 2019.
Fu, P., Kawamura, K., Usukura, K., and Miura, K.: Dicarboxylic acids, ketocarboxylic acids and glyoxal in the marine aerosols collected during a round-the-world cruise, Mar. Chem., 148, 22–32, https://doi.org/10.1016/j.marchem.2012.11.002, 2013.
Gao, Y., Arimoto, R., Duce, R. A., Lee, D. S., and Zhou, M. Y.: Input of atmospheric trace elements and mineral matter to the Yellow Sea during the spring of a low-dust year, J. Geophys. Res.-Atmos., 97, 3767–3777, https://doi.org/10.1029/91JD02686, 1992.
Gondwe, M., Krol, M., Gieskes, W., Klaassen, W., and de Baar, H.: The contribution of ocean-leaving DMS to the global atmospheric burdens, Global Biogeochem. Cy., 17, 1056, https://doi.org/10.1029/2002GB001937, 2003.
Goodall, P., Foulkes, M. E., and Ebdon, L.: Slurry nebulization inductively coupled plasma spectrometry-the fundamental parameters discussed, Spectrochim. Acta B, 48, 1563–1577, https://doi.org/10.1016/0584-8547(93)80143-I, 1993.
Haque, M. M., Kawamura, K., and Kim, Y.: Seasonal variations of biogenic secondary organic aerosol tracers in ambient aerosols from Alaska, Atmos. Environ., 130, 95–104, https://doi.org/10.1016/j.atmosenv.2015.09.075, 2016.
Henning, S., Weingartner, E., and Schwikowski, M.: Seasonal variation of water-soluble ions of the aerosol at the high-alpine site Jungfraujoch (3580 m asl), J. Geophys. Res., 108, 1–10, https://doi.org/10.1029/2002jd002439, 2003.
Hitzenberger, R., Berner, A., Giebl, H., Kromp, R., Larson, S. M., Rouc, A., Koch, A., Marischka, S., and Puxbaum, H.: Contribution of carbonaceous material to cloud condensation nuclei concentrations in European background (Mt. Sonnblick) and urban (Vienna) aerosols, Atmos. Environ., 33, 2647–2659, https://doi.org/10.1016/S1352-2310(98)00391-4, 1999.
Huang, X., Qiu, R., Chan, C. K., and Ravi Kant, P.: Evidence of high PM2.5 strong acidity in ammonia-rich atmosphere of Guangzhou, China: Transition in pathways of ambient ammonia to form aerosol ammonium at [NH4+]/[SO42–]=1.5, Atmos. Res., 99, 488–495, https://doi.org/10.1016/j.atmosres.2010.11.021, 2011.
Huebert, B. J., Howell, S. G., Zhuang, L., Heath, J. A., Litchy, M. R., Wylie, D. J., Kreidler-Moss, J. L., Cöppicus, S., and Pfeiffer, J. E.: Filter and impactor measurements of anions and cations during the first aerosol characterization experiment (ACE 1), J. Geophys. Res.-Atmos., 103, 16493–16509, https://doi.org/10.1029/98JD00770, 1998.
Hueglin, C., Gehrig, R., Baltensperger, U., Gysel, M., Monn, C., and Vonmont, H.: Chemical characterisation of PM2.5, PM10 and coarse particles at urban, near-city and rural sites in Switzerland, Atmos. Environ., 39, 637–651, https://doi.org/10.1016/j.atmosenv.2004.10.027, 2005.
Ingold, T., Mätzler, C., Kämpfer, N., and Heimo, A.: Aerosol optical depth measurements by means of a Sun photometer network in Switzerland, J. Geophys. Res.-Atmos., 106, 27537–27554, 2001.
Kawamura, K. and Bikkina, S.: A review of dicarboxylic acids and related compounds in atmospheric aerosols: Molecular distributions, sources and transformation, Atmos. Res., 170, 140–160, https://doi.org/10.1016/j.atmosres.2015.11.018, 2016.
Kawamura, K. and Ikushima, K.: Seasonal Changes in the Distribution of Dicarboxylic Acids in the Urban Atmosphere, Environ. Sci. Technol., 27, 2227–2235, https://doi.org/10.1021/es00047a033, 1993.
Kawamura, K. and Sakaguchi, F.: Molecular distributions of water soluble dicarboxylic acids in marine aerosols over the Pacific Ocean including tropics, J. Geophys. Res.-Atmos., 104, 3501–3509, https://doi.org/10.1029/1998jd100041, 1999.
Kawamura, K., Kasukabe, H., and Barrie, L. A.: Source and reaction pathways of dicarboxylic acids, ketoacids and dicarbonyls in arctic aerosols: One year of observations, Atmos. Environ., 30, 1709–1722, https://doi.org/10.1016/1352-2310(95)00395-9, 1996.
Kawamura, K. and Kaplan, I. R.: Motor exhaust emissions as a primary source for dicarboxylic acids in Los Angeles ambient air, Environ. Sci. Technol., 21, 105–110, https://doi.org/10.1021/es00155a014, 1987.
Kundu, S., Kawamura, K., Andreae, T. W., Hoffer, A., and Andreae, M. O.: Molecular distributions of dicarboxylic acids, ketocarboxylic acids and α-dicarbonyls in biomass burning aerosols: implications for photochemical production and degradation in smoke layers, Atmos. Chem. Phys., 10, 2209–2225, https://doi.org/10.5194/acp-10-2209-2010, 2010.
Kuo, L. J., Louchouarn, P., and Herbert, B. E.: Influence of combustion conditions on yields of solvent-extractable anhydrosugars and lignin phenols in chars: Implications for characterizations of biomass combustion residues, Chemosphere, 85, 797–805, https://doi.org/10.1016/j.chemosphere.2011.06.074, 2011.
Lazaridis, M.: Organic Aerosols, in: Environmental Chemistry of Aerosols, John Wiley & Sons, Ltd, 91–115, https://doi.org/10.1002/9781444305388.ch4, 2008.
Legrand, M., Preunkert, S., Oliveira, T., Pio, C. A., Hammer, S., Gelencsér, A., Kasper-Giebl, A., and Laj, P.: Origin of C2-C5 dicarboxylic acids in the European atmosphere inferred from year-round aerosol study conducted at a west-east transect, J. Geophys. Res.-Atmos., 112, D23, https://doi.org/10.1029/2006JD008019, 2007.
Librando, V. and Tringali, G.: Atmospheric fate of OH initiated oxidation of terpenes. Reaction mechanism of α-pinene degradation and secondary organic aerosol formation, J. Environ. Manage., 75, 275–282, https://doi.org/10.1016/j.jenvman.2005.01.001, 2005.
Mace, K. A.: Organic nitrogen in rain and aerosol in the eastern Mediterranean atmosphere: An association with atmospheric dust, J. Geophys. Res., 108, 4320, https://doi.org/10.1029/2002JD002997, 2003.
Masson, O., Pourcelot, L., Boulet, B., Cagnat, X., and Videau, G.: Environmental releases from fuel cycle facility: part 1: radionuclide resuspension vs. stack releases on ambient airborne uranium and thorium levels, J. Environ. Radio., 141, 146–152, https://doi.org/10.1016/j.jenvrad.2014.12.009, 2015.
Matos, J. T. V., Duarte, R., and Duarte, A. C.: Challenges in the identification and characterization of free amino acids and proteinaceous compounds in atmospheric aerosols: A critical review, Trac-Trend. Anal. Chem., 75, 97–107, https://doi.org/10.1016/j.trac.2015.08.004, 2016.
Medeiros, P. M., Conte, M. H., Weber, J. C., and Simoneit, B. R. T.: Sugars as source indicators of biogenic organic carbon in aerosols collected above the Howland Experimental Forest, Maine, Atmos. Environ., 40, 1694–1705, https://doi.org/10.1016/j.atmosenv.2005.11.001, 2006.
Moreno, T., Querol, X., Castillo, S., Alastuey, A., Cuevas, E., Herrmann, L., Mounkaila, M., Elvira, J., and Gibbons, W.: Geochemical variations in aeolian mineral particles from the Sahara–Sahel Dust Corridor, Chemosphere, 65, 261–270, https://doi.org/10.1016/j.chemosphere.2006.02.052, 2006.
Moreno, T., Querol, X., Alastuey, A., and Gibbons, W.: Identification of FCC refinery atmospheric pollution events using lanthanoid- and vanadium-bearing aerosols, Atmos. Environ., 42, 7851–7861, https://doi.org/10.1016/j.atmosenv.2008.07.013, 2008.
Nozaki, Y.: “Elemental distribution: overview”, Elements of Physical Oceanography: A derivative of the Encyclopedia of Ocean Sciences: 7. Compiled by Turekian, edited by: Steele, J. H., Thorpe, S. A., and Turekian, K. K., Academic Press, 16 December 2009 – 647 pp., ISBN 9780123785565, 2009.
Okamoto, S. and Tanimoto, H.: A review of atmospheric chemistry observations at mountain sites, Progr. Earth Planet. Sci., 3, 34, https://doi.org/10.1186/s40645-016-0109-2, 2016.
Paatero, P. and Hopke, P. K.: Discarding or downweighting high-noise variables in factor analytic models, Anal. Chim. Acta, 490, 277–289, https://doi.org/10.1016/S0003-2670(02)01643-4, 2003.
Paatero, P. and Unto T.: Positive matrix factorization: A non‐negative factor model with optimal utilization of error estimates of data values, Environmetrics, 5.2, 111–126, 1994.
Pathak, R. K., Wu, W. S., and Wang, T.: Summertime PM2.5 ionic species in four major cities of China: nitrate formation in an ammonia-deficient atmosphere, Atmos. Chem. Phys., 9, 1711–1722, https://doi.org/10.5194/acp-9-1711-2009, 2009.
Perämäki, S. E., Tiihonen, A. J., and Väisänen, A. O.: Occurrence and recovery potential of rare earth elements in Finnish peat and biomass combustion fly ash, J. Geochem. Explor., 201, 71–78, https://doi.org/10.1016/j.gexplo.2019.03.002, 2019.
Perrino, C., Canepari, S., Cardarelli, E., Catrambone, M., and Sargolini, T.: Inorganic constituents of urban air pollution in the Lazio region (Central Italy), Environ. Monit. Assess., 136, 69–86, https://doi.org/10.1007/s10661-007-9718-y, 2008.
Perrone, M. G., Larsen, B. R., Ferrero, L., Sangiorgi, G., De Gennaro, G., Udisti, R., Zangrando, R., Gambaro, A., and Bolzacchini, E.: Sources of high PM2.5 concentrations in Milan, Northern Italy: Molecular marker data and CMB modelling, Sci. Total Environ., 414, 343–355, https://doi.org/10.1016/j.scitotenv.2011.11.026, 2012.
Preunkert, S., Wagenbach, D., and Legrand, M.: Improvement and characterization of an automatic aerosol sampler for remote (glacier) sites, Atmos. Environ., 36, 1221–1232, https://doi.org/10.1016/S1352-2310(01)00371-5, 2002.
Putaud, J. P., Van Dingenen, R., and Raes, F.: Submicron aerosol mass balance at urban and semirural sites in the Milan area (Italy), J. Geophys. Res.-Atmos., 107, 1–11, https://doi.org/10.1029/2000JD000111, 2002.
Puxbaum, H., Caseiro, A., Sánchez-Ochoa, A., Kasper-Giebl, A., Claeys, M., Gelencsér, A., Legrand, M., Preunkert, S., and Pio, C. A.: Levoglucosan levels at background sites in Europe for assessing the impact of biomass combustion on the European aerosol background, J. Geophys. Res.-Atmos., 112, 1–11, https://doi.org/10.1029/2006JD008114, 2007.
Qi, L., Chen, M., Ge, X., Zhang, Y., and Guo, B.: Seasonal Variations and Sources of 17 Aerosol Metal Elements in Suburban Nanjing, China, Atmosphere, 7, 153, https://doi.org/10.3390/atmos7120153, 2016.
Ren-Jian, Z., Kin-Fai, H., and Zhen-Xing, S.: The Role of Aerosol in Climate Change, the Environment, and Human Health, Atmos. Ocean. Sci. Lett., 5, 156–161, https://doi.org/10.1080/16742834.2012.11446983, 2012.
Rinaldi, M., Decesari, S., Carbone, C., Finessi, E., Fuzzi, S., Ceburnis, D., Dowd, C. D. O., Sciare, J., Burrows, J. P., Vrekoussis, M., Ervens, B., Tsigaridis, K., and Facchini, M. C.: Evidence of a natural marine source of oxalic acid and a possible link to glyoxal, J. Geophys. Res., 116, 1–12, https://doi.org/10.1029/2011JD015659, 2011.
Ruiz-Jimenez, J., Okuljar, M., Sietiö, O.-M., Demaria, G., Liangsupree, T., Zagatti, E., Aalto, J., Hartonen, K., Heinonsalo, J., Bäck, J., Petäjä, T., and Riekkola, M.-L.: Determination of free amino acids, saccharides, and selected microbes in biogenic atmospheric aerosols – seasonal variations, particle size distribution, chemical and microbial relations, Atmos. Chem. Phys., 21, 8775–8790, https://doi.org/10.5194/acp-21-8775-2021, 2021.
Scalabrin, E., Zangrando, R., Barbaro, E., Kehrwald, N. M., Gabrieli, J., Barbante, C., and Gambaro, A.: Amino acids in Arctic aerosols, Atmos. Chem. Phys., 12, 10453–10463, https://doi.org/10.5194/acp-12-10453-2012, 2012.
Schwikowski, M., Döscher, A., Gäggeler, H. W., and Schotterer, U.: Anthropogenic versus natural sources of atmospheric sulphate from an Alpine ice core, Tellus B, 51, 938–951, https://doi.org/10.1034/j.1600-0889.1999.t01-4-00006.x, 1999.
Shrestha, A. B., Wake, C. P., and Dibb, J. E.: Chemical composition of aerosol and snow in the high Himalaya during the summer monsoon season, Atmos. Environ., 31, 2815–2826, https://doi.org/10.1016/S1352-2310(97)00047-2, 1997.
Simoneit, B. R. T.: A review of biomarker compounds as source indicators and tracers for air pollution, Environ. Sci. Pollut. Res., 6, 159–169, https://doi.org/10.1007/bf02987621, 1999.
Simoneit, B. R. T.: Biomass burning - A review of organic tracers for smoke from incomplete combustion, Appl. Geochem., 17, 129–162, https://doi.org/10.1016/s0883-2927(01)00061-0, 2002.
Squizzato, S., Masiol, M., Brunelli, A., Pistollato, S., Tarabotti, E., Rampazzo, G., and Pavoni, B.: Factors determining the formation of secondary inorganic aerosol: a case study in the Po Valley (Italy), Atmos. Chem. Phys., 13, 1927–1939, https://doi.org/10.5194/acp-13-1927-2013, 2013.
Stockwell, W. R., Watson, J. G., Robinson, N. F., Steiner, W., and Sylte, W. W.: The ammonium nitrate particle equivalent of NOx emissions for wintertime conditions in Central California's San Joaquin Valley, Atmos. Environ., 34, 4711–4717, https://doi.org/10.1016/S1352-2310(00)00148-5, 2000.
Tong, R. and Guo, W.: Slurry nebulisation ICP-MS direct determination of high field strength elements (Nb, Ta, Zr, and Hf) in silicate rocks, RSC Adv., 9, 32435–32440, https://doi.org/10.1039/C9RA06610A, 2019.
Tositti, L., Riccio, A., Sandrini, S., Brattich, E., Baldacci, D., Parmeggiani, S., Cristofanelli, P., and Bonasoni, P.: Short-term climatology of PM10 at a high altitude background station in southern Europe, Atmos. Environ., 65, 142–152, https://doi.org/10.1016/j.atmosenv.2012.10.051, 2013.
Turetta, C., Feltracco, M., Barbaro, E., Spolaor, A., Barbante, C., and Gambaro, A.: A year-round measurement of water-soluble trace and rare earth elements in arctic aerosol: Possible inorganic tracers of specific events, Atmosphere, 12, 694, https://doi.org/10.3390/atmos12060694, 2021.
Vardè, M., Barbante, C., Barbaro, E., Becherini, F., Bonasoni, P., Busetto, M., Calzolari, F., Cozzi, G., Cristofanelli, P., Dallo, F., De Blasi, F., Feltracco, M., Gabrieli, J., Gambaro, A., Maffezzoli, N., Morabito, E., Putero, D., Spolaor, A., and Cairns, W. R. L.: Characterization of atmospheric total gaseous mercury at a remote high-elevation site (Col Margherita Observatory, 2543 m a.s.l.) in the Italian Alps, Atmos. Environ., 271, 118917, https://doi.org/10.1016/j.atmosenv.2021.118917, 2022.
Vassilev, S. V. and Vassileva, C. G.: Contents and associations of rare earth elements and yttrium in biomass ashes, Fuel, 262, 116525, https://doi.org/10.1016/j.fuel.2019.116525, 2020.
Viana, M., Pandolfi, M., Minguillon, M. C., Querol, X., Alastuey, A., Monfort, E., and Celades, I.: Inter-comparison of receptor models for PM source apportionment: Case study in an industrial area, Atmos. Environ., 42, 3820–3832, https://doi.org/10.1016/j.atmosenv.2007.12.056, 2008.
Wang, H. B., Kawamura, K., and Yamazaki, K.: Water-soluble dicarboxylic acids, ketoacids and dicarbonyls in the atmospheric aerosols over the Southern Ocean and western Pacific Ocean, J. Atmos. Chem., 53, 43–61, https://doi.org/10.1007/s10874-006-1479-4, 2006.
Wedepohl, K. H.: The composition of the continental crust, Goechim. Cosmochim. Acta, 59, 1217–1232, 1995.
Wong, M. Y., Mahowald, N. M., Marino, R., Williams, E. R., Chellam, S., and Howarth, R. W.: Natural atmospheric deposition of molybdenum: a global model and implications for tropical forests, Biogeochemistry, 149, 159–174, https://doi.org/10.1007/s10533-020-00671-w, 2020a.
Wong, M. Y., Mahowald, N. M., Marino, R., Williams, E. R., Chellam, S., and Howarth, R. W.: Natural atmospheric deposition of molybdenum: a global model and implications for tropical forests, Biogeochemistry, 149, 159–174, https://doi.org/10.1007/s10533-020-00671-w, 2020b.
Wong, M. Y., Rathod, S. D., Marino, R., Li, L., Howarth, R. W., Alastuey, A., Alaimo, M. G., Barraza, F., Carneiro, M. C., Chellam, S., Chen, Y.-C., Cohen, D. D., Connelly, D., Dongarra, G., Gómez, D., Hand, J., Harrison, R. M., Hopke, P. K., Hueglin, C., Kuang, Y., Lambert, F., Liang, J., Losno, R., Maenhaut, W., Milando, C., Monteiro, M. I. C., Morera-Gómez, Y., Querol, X., Rodríguez, S., Smichowski, P., Varrica, D., Xiao, Y., Xu, Y., and Mahowald, N. M.: Anthropogenic Perturbations to the Atmospheric Molybdenum Cycle, Global Biogeochem. Cy., 35, e2020GB006787, https://doi.org/10.1029/2020GB006787, 2021.
Yeatman, S. G., Spokes, L. J., and Jickells, T. D.: Comparisons of coarse-mode aerosol nitrate and ammonium at two polluted coastal sites, Atmos. Environ., 35, 1321–1335, https://doi.org/10.1016/S1352-2310(00)00452-0, 2001.
Zangrando, R., Barbaro, E., Zennaro, P., Rossi, S., Kehrwald, N. M., Gabrieli, J., Barbante, C., and Gambaro, A.: Molecular Markers of Biomass Burning in Arctic Aerosols, Environ. Sci. Technol., 47, 8565–8574, https://doi.org/10.1021/es400125r, 2013.
Zangrando, R., Barbaro, E., Kirchgeorg, T., Vecchiato, M., Scalabrin, E., Radaelli, M., Đorđević, D., Barbante, C., and Gambaro, A.: Five primary sources of organic aerosols in the urban atmosphere of Belgrade (Serbia), Sci. Total Environ., 571, https://doi.org/10.1016/j.scitotenv.2016.06.188, 2016.