the Creative Commons Attribution 4.0 License.
the Creative Commons Attribution 4.0 License.
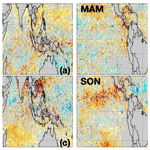
Opinion: Beyond global means – novel space-based approaches to indirectly constrain the concentrations of and trends and variations in the tropospheric hydroxyl radical (OH)
Bryan N. Duncan
Daniel C. Anderson
Arlene M. Fiore
Joanna Joiner
Nickolay A. Krotkov
Dylan B. Millet
Julie M. Nicely
Luke D. Oman
Jason M. St. Clair
Joshua D. Shutter
Amir H. Souri
Sarah A. Strode
Brad Weir
Glenn M. Wolfe
Helen M. Worden
Qindan Zhu
The hydroxyl radical (OH) plays a central role in tropospheric chemistry, as well as influencing the lifetimes of some greenhouse gases. Because of limitations in our ability to observe OH, we have historically relied on indirect methods to constrain its concentrations, trends, and variations but only as annual global or annual semi-hemispheric averages. Recent methods demonstrated the feasibility of indirectly constraining tropospheric OH on finer spatio-temporal scales using satellite observations as proxies for the photochemical drivers of OH (e.g., nitrogen dioxide, formaldehyde, isoprene, water vapor, ozone). We found that there are currently reasonable satellite proxies to constrain up to about 75 % of the global sources of tropospheric OH and up to about 50 % of the global sinks. With additional research and investment in observing various volatile organic compounds, there is potential to constrain an additional 10 % of the global sources and 30 % of the global sinks. We propose steps forward for the development of a comprehensive space-based observing strategy, which will improve our ability to indirectly constrain OH on much finer spatio-temporal scales than previously achieved. We discuss the strengths and limitations of such an observing strategy and potential improvements to current satellite instrument observing capabilities that would enable better constraint of OH. Suborbital observations (i.e., data collected from non-satellite platforms such as aircraft, balloons, and buildings) are required to collect information difficult to obtain from space and for validation of satellite-based OH estimates; therefore, they should be an integral part of a comprehensive observing strategy.
- Article
(3168 KB) - Full-text XML
- BibTeX
- EndNote
The hydroxyl radical (OH) has a pivotal role in atmospheric chemistry and controls the lifetimes of methane (CH4), hydrochlorofluorocarbons and hydrofluorocarbons (HCFCs and HFCs), carbon monoxide (CO), volatile organic compounds (VOCs), and numerous other gases relevant to climate and air quality. The relationship of OH to other atmospheric constituents is often complex and non-linear. For instance, the concentrations and lifetimes of ozone (O3) and OH are convolved, as the primary source of OH involves the destruction of O3, OH is integral in many tropospheric chemical reactions (e.g., initiating the oxidation of VOCs) that lead to O3 production, and the reaction of HO2 with NO is a secondary source of OH that can also lead to O3 production. The reader is referred to, for instance, Lelieveld et al. (2016) and Fiore et al. (2024) for comprehensive overviews of the importance of OH in tropospheric chemistry, as well as discussions of key uncertainties in its global chemical budgets and estimates of past global trends and variations in its atmospheric abundance.
In situ measurements provide information about tropospheric OH at a particular point in time and space. However, these observations are sparse, and OH is highly variable in space and time as its lifetime is < 1 s. Therefore, in situ observational networks are not a viable way to map the variability and trends in OH across the globe. In addition, there are few in situ instruments that can quantify OH at typical ambient concentrations of 105–107 molec. cm−3 (e.g., Stone et al., 2012). Generally, in situ measurement uncertainties are ∼ 30 % for integration times of 30–60 s (Brune et al., 2018). Comparing OH observations from multiple airborne missions to steady-state box model simulations, Miller and Brune (2022) concluded that “oxidation chemistry in most of the free troposphere is understood to as well as current measurements can determine.” This implies that for some parts of the atmosphere, further refinements in our understanding of tropospheric oxidation are limited by inherent measurement uncertainties for both OH and related species. Nevertheless, the suite of other trace gases and aerosols observed during campaigns, both with and without the sparse in situ OH data, have provided a detailed, albeit geospatially limited, view of tropospheric composition (e.g., Nicely et al., 2016).
A tropospheric OH data product derived from a space-borne instrument is not currently feasible, although it may become feasible with technological development. The total column of OH has been measured for decades during daytime with passive instruments at a few ground-based facilities (e.g., Cageao et al., 2001; Minschwaner et al., 2003), and new technology development could lead to an OH lidar (Pan et al., 2022). Studies would need to be performed to assess the suitability of these passive and active instruments for deployment on satellites. If it is feasible to deploy such instruments in space, a tropospheric column of OH could be inferred by subtracting the stratospheric column observations from the total column observations; space-based instruments that measure stratospheric-mesospheric OH, albeit not down to the tropopause, have already been demonstrated (e.g., the Microwave Limb Sounder, MLS; Wang et al., 2008).
There are a number of methods to indirectly constrain tropospheric OH on global scales, such as with suborbital observations (e.g., Lelieveld et al., 2006). Methods that use in situ data of methylchloroform or a combination of HCFC observations constrain only global or, possibly, hemispheric OH (e.g., Liang et al., 2017; Thompson et al., 2024). For methylchloroform, recent declines in tropospheric abundance limit its utility (Lelieveld et al., 2004; Liang et al., 2017). Nevertheless, multi-decadal methylchloroform observations have provided an important constraint on the long-term trends and variations in tropospheric OH (e.g., Patra et al., 2021, and references therein). Shortwave infrared (SWIR) observations of CH4 from the Japan Aerospace Exploration Agency (JAXA) Greenhouse gases Observing SATellite (GOSAT) have been proposed as a way to indirectly constrain global OH (Zhang et al., 2018), although additional surface and thermal infrared (TIR) observations may be required (Zhang et al., 2021).
In addition to the lack of data for spatio-temporal constraint, uncertainties in the atmospheric processes (e.g., trace gas and heterogenous chemistry, emissions) that influence tropospheric OH (e.g., as summarized in Table 2 of Fiore et al., 2024; Prather and Zhu, 2024) have resulted in a large range in simulated OH among chemical transport models (CTMs; e.g., Nicely et al., 2017, 2020; Zhao et al., 2019; Murray et al., 2021). Consequently, there is considerable uncertainty in the sink of CH4, which contributes significantly to the overall uncertainty in the budget of CH4 (Saunois et al., 2020).
Recent efforts have demonstrated satellite-based methods to indirectly constrain the spatio-temporal concentrations, trends, and variations in OH on local and regional scales (e.g., Valin et al., 2013, 2016; Wolfe et al., 2019; Wells et al., 2020; Pimlott et al., 2022; Zhu et al., 2022b; Anderson et al., 2023, 2024; Zhao et al., 2023; Souri et al., 2024; Shutter et al., 2024; Zhu et al., 2024). These methods use various satellite datasets as proxies for OH drivers (e.g., CH4, water vapor (H2O(v)), nitrogen dioxide (NO2), CO, formaldehyde (HCHO), isoprene, tropospheric O3). Thanks to contemporary multi-decadal data records, these approaches open new avenues for characterizing OH over long periods of time, which is important for understanding the impacts of both anthropogenic activities and natural phenomena on tropospheric OH.
The purpose of this paper is to make recommendations for developing a strategic and comprehensive observing strategy, which will improve our ability to indirectly constrain OH on much finer spatio-temporal scales than has previously been achieved. Our recommendations are intended to inform international efforts to prioritize observational needs. In Sect. 2, we describe the current space-based approaches to constrain tropospheric OH. In Sect. 3, we summarize suborbital observations (i.e., data collected from non-satellite platforms, such as aircraft, balloons, and buildings) that are required for information currently unobtainable from space (e.g., fine vertical resolution of satellite proxies) and for validation of satellite-based OH estimates. In Sect. 4, we discuss potential refinements to retrieval algorithms as well as to instrument capabilities that would improve satellite data products. Finally, in Sect. 5, we discuss potential improvements to current satellite observing capabilities that would better enable constraint of OH. These improvements include ones that are obtainable with current technologies (e.g., more observations, co-located observations) as well as ones requiring additional technology development (e.g., to obtain finer vertically resolved observations).
Here we provide a brief overview of the various space-based approaches that have been used to constrain tropospheric OH as partial and/or total vertical column densities (VCDs) or surface concentrations on monthly to seasonal timescales. Each of these approaches has strengths and limitations that need to be considered when being applied to constrain tropospheric OH.
2.1 Process-based approaches
These approaches exploit photochemical relationships between satellite-observable species and tropospheric OH, which aid understanding of the causes of trends and variations in inferred OH (e.g., Valin et al., 2016; Nicely et al., 2018; Baublitz et al., 2023).
-
Wolfe et al. (2019) found a linear relationship between OH and HCHO using in situ observations from NASA's Atmospheric Tomography (ATom) field campaign, which is consistent with the modeling work of Valin et al. (2016), who found that HCHO VCDs primarily depend on OH production rates at low OH concentrations. This indicates that HCHO VCDs, such as from the Ozone Monitoring Instrument (OMI) on NASA's Aura satellite, could be used to infer tropospheric OH VCDs on local scales. This approach is valid over remote oceanic environments, where CH4 oxidation is the primary HCHO source; there are broad regions of the troposphere that are characterized by this photochemical environment.
-
Pimlott et al. (2022) developed an approach that employs a simplified steady-state approximation with multiple trace gas observations (i.e., O3, CO, CH4, and H2O(v)) from the Infrared Atmospheric Sounding Interferometer (IASI) on the European Space Agency (ESA) MetOp-A satellite. This approach is most representative of OH from 600 to 700 hPa, although the authors argue that their approach could be reasonably applied from 400 to 800 hPa.
-
Shutter et al. (2024) developed an approach to constrain tropospheric OH trends and variability that uses the ratio of VCDs of isoprene from the NOAA Suomi NPP Cross-track Infrared Sounder (CrIS) and HCHO from the Ozone Mapping Profiler Suite (OMPS) over isoprene-emitting forest ecosystems. They take advantage of the fact that the ratio of the VCDs of isoprene and HCHO scales with in such environments (Wells et al., 2020).
2.2 Machine learning (ML) approaches
These approaches use a combination of an ML model, output from a CTM, and satellite observations. The utility of these approaches for constraining tropospheric OH depends on the accuracy of the training dataset. For example, there are known knowledge gaps in our understanding of the processes that influence OH (Fiore et al., 2024). In addition, these approaches reproduce OH only for photochemical environments that are represented in the training dataset. For example, the following two approaches were designed for polluted urban environments and cleaner tropical and subtropical ones, respectively.
-
Zhu et al. (2022b) developed an approach that takes advantage of the known response of surface OH to NOx (= NO2+ NO) concentrations to infer trends and variations in summertime surface OH over selected cities. Their six input parameters, on which their ML model was trained, explain 76 % of the variance in simulated surface OH. Two of the input parameters are satellite data (i.e., OMI NO2 and HCHO), although the other parameters are simulated by a CTM.
-
Anderson et al. (2023) developed a multi-satellite approach, testing the utility of datasets of numerous trace gases (i.e., Table 1 of Anderson et al., 2023) that influence OH through chemical, radiative and meteorological effects. They demonstrated the potential of their approach for inferring trends of tropospheric OH VCDs over tropical and subtropical oceans (Fig. 1), and their approach is being expanded over land and the extra-tropics. Preliminary results indicate that this approach, which uses tropospheric and total VCDs, may be used to infer OH for various layers of the troposphere; information on the vertical distribution of OH is important for estimating, for instance, the lifetime of CH4, given that CH4 loss is weighted toward the lower troposphere.
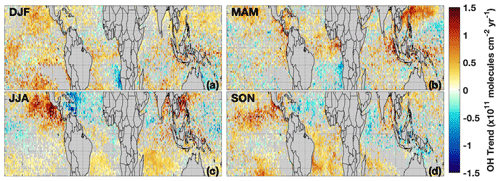
Figure 1There is significant seasonal and spatial variability in the trends in the tropospheric VCD of OH over tropical oceans from 2005 to 2019. We calculated the trends using the OH product described in Anderson et al. (2023) and a multiple linear regression described in Anderson et al. (2024). Colored areas indicate those grid boxes where the trend is statistically significant and with at least 10 years of data. This figure is an adaptation of Fig. 2 from Anderson et al. (2024).
2.3 Chemical data assimilation approaches
Historically, these approaches incorporate satellite observations of OH drivers into CTMs to constrain tropospheric OH. They use inverse modeling and/or chemical data assimilation methods (Bocquet et al., 2015) to reconcile modeled estimates with observations while relying on the underlying chemical mechanisms of the model to infer OH concentrations consistent with the assimilated observations. A notable benefit of data assimilation is that it provides an improved estimate of concentrations, and the resulting increments indicate where model parameterizations are deficient and/or require improvement. Overall, the benefit of data assimilation depends on the magnitude of the difference between the observed and simulated variable.
-
Miyazaki et al. (2012, 2020) and several other papers from the same group developed multi-species (notably NO2, O3, and CO) assimilation systems for estimating tropospheric composition and emissions. In particular, assimilation reduced the multi-model spread and annual biases of key OH drivers in four CTM frameworks. The multi-model spread for tropospheric mean OH was reduced by 24 %–58 % over polluted areas.
-
Gaubert et al. (2016, 2017) assimilated Measurements of Pollution in the Troposphere (MOPITT) CO observations into the Community Atmosphere Model with Chemistry (CAM-Chem) of the Community Earth System Model (CESM). They showed, for example, that increases in CO because of assimilation can lead to decreases in OH and finally to longer lifetimes of CH4. In contrast, decreases in CO can lead to increased OH and shorter CH4 lifetimes. Highlighting nonlinear effects, the study also demonstrated that assimilation led to changes in O3 and photochemical activity due to HOx recycling.
2.4 Simplified hybrid approaches
Several recent simplified approaches allow for the quantification of the impacts of constraining various OH drivers with satellite observations, which could inform decision-making (e.g., prioritization of observables) in inverse model and data assimilation efforts (Sect. 2.3).
-
Zhao et al. (2023) developed a method to post-process a CTM simulation to improve simulated tropospheric OH. Their method adjusts the simulated monthly averaged concentrations of OH drivers (CO, CH4, O3, HCHO, NO2, total column O3, and H2O(v)) with satellite observations for each model column and then uses a photochemical box model to recalculate the corresponding concentration of tropospheric OH.
-
Zhu et al. (2024) used an ML technique to develop an emulator of simulated tropospheric OH VCDs in the CESM Whole Atmosphere Community Climate Model (WACCM), which facilitated their assessment of the roles of satellite VCDs of NO2, HCHO, and CO on tropospheric OH concentrations, trends, and variations.
-
Souri et al. (2024) developed a Bayesian data fusion method that adjusts monthly averaged distributions of OH drivers from a CTM simulation using satellite observations. This method considers the quality of the datasets and the a priori used in the retrieval. The adjusted model fields are then used in a parameterization of OH (Anderson et al., 2022) in a CH4–CO–OH cycle model (ECCOH; Elshorbany et al., 2016), allowing for easy evaluation of the impact of adjustments on OH, CH4, and CO.
The robustness of constraining OH with these satellite-based approaches is not limited by, for instance, the ML or data assimilation methods at the present time but instead by the quality of the data used as input to these methods and limitations in our current understanding of the chemistry and emissions that influence tropospheric OH (e.g., Table 2 of Fiore et al., 2024). Therefore, the recommendations in the sections that follow focus on further development of the satellite-based approaches as well as improvement to the quality of satellite data and to independent validation products.
The utility of any space-based OH estimate (Sect. 2) hinges on an adequate understanding of how OH responds to its drivers. Therefore, long-term measurements of key atmospheric properties, supplemented with occasional air- and ground-based intensive campaigns, would build confidence far beyond what has been achieved with previous intermittent efforts, such as the NASA Atmospheric Tomography Mission (AToM) that provided a comprehensive suite of observations, primarily in remote oceanic environments. In addition, a comprehensive suite of co-located observations, both in situ and remotely sensed, is desired for validating CTMs, developing process-based diagnostics (Sect. 5.3), interpreting trends and variations in inferred OH, and improving satellite retrievals (Sect. 4).
We recommend the development of a long-term, robust suborbital observing network composed of instruments at stationary sites as well as ones deployed in field campaigns. The establishment of OH “supersite” observatories would allow for the collection of a comprehensive suite of co-located measurements of key atmospheric constituents (discussed below) to constrain tropospheric OH production and loss (Sect. 5.2.1). Focusing first on augmenting existing efforts (e.g., NOAA; In-service Aircraft for a Global Observing System, IAGOS; Network for the Detection of Atmospheric Composition Change, NDACC; and Advanced Global Atmospheric Gases Experiment, AGAGE) would keep early-development efforts feasible by leveraging existing facility infrastructure. In addition, some existing efforts (e.g., four NOAA baseline observatories, the IAGOS program) already observe many of the variables that reasonably constrain OH, as discussed below. Periodic field campaigns would supplement the data collected at OH supersites, especially vertical composition profiles and in photochemical environments not represented by the ground and routine aircraft networks. Continuous and co-located surface and VCD observations would be ideal, especially over a range of photochemical environments (e.g., remote to urban) and seasons. Planetary boundary layer height data would be useful for interpreting these observations. The placement and density of OH supersites and locations/timing of field campaigns would benefit from observing system simulation experiments (OSSEs) in order to maximize the value of the suborbital data for constraining OH. For instance, a simpler strategy would likely be satisfactory over the relatively homogeneous remote oceans (e.g., fewer stations and observations of individual VOCs) as compared to more complex land environments. Therefore, the OSSE studies would also need to consider the required suite of observations at individual sites.
We recommend a focus on the collection of suborbital observations of OH drivers, given the lower uncertainties typically associated with these measurements as compared to those for OH (Sect. 1). Such observations would allow for the constraint of OH within photochemical box models, although OH budget closure remains an active area of research (Stone et al., 2012), and it is unclear whether existing in situ observations can adequately explain OH in all cases (e.g., Fuchs et al., 2017; Lew et al., 2020; Hansen et al., 2021; Yang et al., 2022; Bottorff et al., 2023; Yang et al., 2024).
The following variables reasonably constrain tropospheric OH in photochemical box models and should be priority measurements: H2O(v), NO, NO2, CO, O3, actinic flux (or filter radiometers for wavelength ranges relevant to NO2 and O(1D)), HCHO, and temperature. In regions closer to strong emissions sources (over and near land), chemical controls on OH are more complex and variable and a more comprehensive measurement suite (e.g., total OH reactivity, VOC composition, nitrous acid (HONO), and peroxy radicals (HOx, ROx)) (e.g., Lelieveld et al., 2016; Yang et al., 2016; Murray et al., 2021) may be needed to constrain all drivers. In remote marine environments, information on VOC speciation and reactivity is less critical but not unimportant (Travis et al., 2020; Baublitz et al., 2023), as the background atmosphere is (relatively) uniform, and large-scale OH variability primarily reflects variability in OH production from photolysis and HOx cycling (Wolfe et al., 2019; Brune et al., 2020).
Occasional deployment of in situ instruments that observe tropospheric OH at OH supersite observatories and in field campaigns would allow for an assessment of the consistency between observed OH and OH inferred from the satellite-based approaches (Sect. 2). As mentioned above, there are few in situ instruments, and technology development is necessary before such observations could become routine.
Here, we discuss potential refinements to retrieval algorithms, as well as to instrument capabilities, that would improve data products for the satellite-based approaches (Sect. 2).
4.1 Ultraviolet/visible (UV/Vis) data
The quality of UV/Vis satellite-based data products is determined by a number of issues, including signal-to-noise ratio (SNR), spectral fitting uncertainties, systematic and random measurement errors, and uncertainties associated with creating VCDs (e.g., Duncan et al., 2014; Lorente et al., 2017). For example, to extract the spectral signature of HCHO used in retrievals, several interfering geophysical processes need to be accounted for, including absorption by O3, NO2, bromine monoxide (BrO), and oxygen dimers (O2–O2), as well as rotational Raman scattering (Ring effect; e.g., González Abad et al., 2016). Coupled with its small atmospheric loading, especially over remote oceanic areas, the HCHO VCDs are “noisy”, requiring temporal and/or spatial averaging to reduce random errors so that the geophysical signal becomes clear (e.g., Liao et al., 2024). As another example, NO2 VCDs are dominated by the stratospheric component over remote areas, leading to relatively large uncertainties in the corresponding tropospheric portion of VCDs (e.g., Lamsal et al., 2021). Improvements in data quality, whether through data product refinement (below), oversampling techniques, or enhancements in instrument capabilities (Sect. 5), will be required to use the satellite-based approaches (Sect. 2) at finer spatial and temporal resolutions than are achievable with current instruments.
Work is ongoing to improve the accuracy and precision of satellite data products through retrieval algorithm refinement and also using, for example, data-driven ML data analysis methods that show promise for reducing noise in data products when the SNR is low and/or the trace gas is a weak absorber (e.g., Li et al., 2022; Joiner et al., 2023). For instance, Joiner et al. (2024) have tested their technique on NASA Tropospheric Emissions: Monitoring of Pollution (TEMPO) HCHO retrievals. Preliminary results (Fig. 2) show visibly less noise while keeping overall consistency with the original noisy data, and there is a reduction in the number of pixels with negative slant column density (SCD) values in the noise-reduced data.
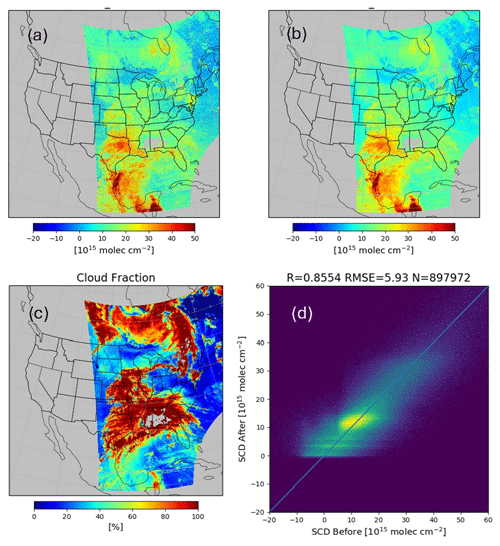
Figure 2Preliminary results of noise reduction applied to HCHO-retrieved SCD from version 3 TEMPO data on 5 May 2024 scan 5: (a) original data before noise reduction used as training, (b) after noise reduction was applied, (c) effective cloud fraction supplied in the L2 data, and (d) the scatter diagram showing overall agreement between noise-reduced and original SCDs relative to the 1:1 line with fit statistics. R is the correlation, RMSE is the root-mean-square error, and N is the number of pixels used in the comparison.
Additionally, different retrieval methods applied to the same instrument often produce large differences in data products, which impact inferred OH (e.g., Anderson et al., 2023). Discrepancies created by retrieval algorithm assumptions could be resolved with more robust suborbital data than is currently available for validation (Sect. 3), especially for a priori profiles used in retrievals.
Additional potential areas of retrieval algorithm refinement include aerosol correction and stratosphere–troposphere separation that will benefit from improvements in radiative transfer forward model simulations as implemented in level 2 (L2) retrieval algorithms. For example, to reduce computation time, many retrieval algorithms use pre-computed lookup tables and do not explicitly represent important geophysical processes such as aerosol scattering/absorption. With sufficient acceleration, radiative transfer models or ML proxies can be used directly in L2 algorithms, reducing interpolation errors and providing greater flexibility to use input data that are more representative of the atmospheric state (e.g., the aerosol profiles from MERRA-2 reanalysis used in OMI NO2 retrievals; Vasilkov et al., 2021); satellite data processing levels indicate the degree of data processing of satellite data, where L2 indicates that a geophysical parameter has been derived.
4.2 Thermal infrared (TIR) data
Trace gas measurements in the TIR spectrum often target species with relatively weak absorption, and low instrument noise is thus a key requirement for useful retrievals (Fu et al., 2019). High spectral resolution is also pivotal for resolving relevant spectral features and distinguishing them from atmospheric or surface interferences (Clarisse et al., 2011). In addition to providing spectral coverage encompassing the chemical species of interest, future TIR sounders aiming to constrain atmospheric composition therefore need to prioritize these instrumental characteristics.
New retrieval developments employing ML and species detection based on a hyperspectral range index (Walker et al., 2011) have led to increased sensitivity and expanded the suite of species that can be measured in the TIR range (e.g., acetic acid, acetone, ethane, ethene, ethyne, formic acid, glycoaldehyde, hydrogen cyanide, isoprene, methanol, peroxyacetyl nitrate (PAN)) ( e.g., Wells et al., 2020; Franco et al., 2022, 2024; Brewer et al., 2024). Since TIR observations are based on the thermal contrast between the Earth surface and the absorber, uncertainties increase when that temperature difference is small. For the same reason, profile shape uncertainties can be an important error source in TIR retrievals. Validation data are often limited for species targeted in this spectral range, and future space-based measurements would benefit from an expanded portfolio of surface and airborne data to validate retrievals and refine profile shape assumptions. Observational errors can also arise from uncertainties in the absorption cross section and surface emissivity datasets employed in retrievals; advancing the fidelity of these resources would therefore benefit the reliability of TIR trace gas products.
4.3 Short-wave infrared (SWIR) data
Measurements of trace gases in the SWIR rely on reflected solar radiance and can provide a true total column observation through the atmosphere under cloud-free conditions and when the atmospheric pathlength is characterized. Here we assume the SWIR range includes wavelengths from approximately 0.7 to 3 µm. This range is especially useful for measuring total columns (surface to top of atmosphere) of CO2 (Greenhouse gases Observing SATellite, GOSAT, and Orbiting Carbon Observatory-2, OCO-2), CH4 (GOSAT and TROPOspheric Monitoring Instrument, TROPOMI) and CO (MOPITT, GOSAT-2, and TROPOMI). In many cases, the O2 A-band around 0.76 mm is used as a reference observation of the atmospheric path and cloud detection due to the constant and well-known concentration of molecular oxygen. SWIR observations of total column CO and CH4 are especially important for quantifying surface source emissions that have a significant contribution to OH sinks (Sect. 5.2).
4.4 Enhancing vertical resolution
As is the case for suborbital observation needs (Sect. 3), vertically resolved data of OH drivers are a priority for future satellite instruments to improve retrieval algorithms, especially for a priori profiles. In addition, they will advance the ability of the satellite-based approaches (Sect. 2) to constrain tropospheric OH at a finer vertical resolution than a tropospheric VCD measurement can provide. Such data are also useful for validating CTMs, developing process-based diagnostics (Sect. 5.3), and interpreting the causes of trends and variations in inferred OH throughout the tropospheric column (e.g., Baublitz et al., 2023). For example, the efficiency and quality of the ML approaches (Sect. 2.2) would benefit from vertically resolved input variables.
We recommend that studies be done to maximize the vertical information of the observations of OH drivers, which may be achieved through a number of approaches, including developing satellite instrument technology (e.g., multi-angle and/or multispectral instruments) and investing in the development of multispectral data products that use radiances from one or more instruments (e.g., CH4; Zhang et al., 2018). Multispectral data products were developed for O3 (Tropospheric Emission Spectrometer, TES/OMI; Fu et al., 2013; Colombi et al., 2021; Atmospheric Infrared Sounder (AIRS)/OMI, Fu et al., 2018; TROPOMI/Cross-track Infrared Sounder (CrIS), Mettig et al., 2022) and CO (MOPITT NIR/TIR, Worden et al., 2010; Gaubert et al., 2017; CrIS/TROPOMI, Fu et al., 2016); development of these and other products is occurring through the NASA TRopospheric Ozone and Precursors from Earth System Sounding (TROPESS) project.
In addition to gaining vertical information through the development of satellite instrument technology and multispectral algorithms, one may combine multiple data products of an OH driver from instruments that observe different tropospheric vertical layers. As an example, Oman et al. (2013) constrained the response of O3 to ENSO using vertically resolved data from the NASA TES and MLS, both on the NASA Aura satellite. In this analysis, MLS and TES data were treated separately and not merged (TES operations ended in 2018 and MLS operations are predicted to end in mid-2026, with no follow-on instruments currently planned).
In this section, we discuss considerations and make recommendations for an observing strategy that would advance our ability to quantify the concentrations, trends, and variations in tropospheric OH using atmospheric remote-sensing observations of its drivers.
5.1 Optimizing an observing strategy through trade-space analyses
As discussed in Sect. 2, there are a number of approaches in the literature to indirectly constrain OH with satellite proxies for OH drivers. Each of the approaches applies to specific regions and/or photochemical environments or was devised for specific applications, and each of them has its strengths and limitations that should be considered. Therefore, some combination of these approaches, as well as the development of new approaches for specific photochemical environments, may be required to maximize spatio-temporal coverage of tropospheric OH around the globe for individual applications (as discussed below).
The design process of a comprehensive, multi-satellite observing strategy would benefit from trade-space analyses (i.e., assessing the impact of changing one or more variables while simultaneously changing one or more different variables in the opposite direction). For example, such analyses of spatial, temporal, and spectral resolutions for specific instruments would inform design decision making. The design process would also benefit from cost–benefit analyses. For instance, what observations are achievable using more cost-effective suborbital instruments (Sect. 3) than satellite instruments?
5.1.1 Continuity
The fidelity of a sustained, long-term record of inferred tropospheric OH from satellite-based approaches (Sect. 2) depends on the continuity of satellite proxies for OH drivers. Therefore, a priority for a robust observing strategy is for the continuity of satellite instruments with similar or enhanced capabilities, as well as inter-agency coordination, to maintain self-consistent and trend-quality level 1B (L1B) radiances for the generation of multi-instrument data records. L1B data, which are used in scientific algorithms to derive geophysical parameters, have been calibrated and geolocated. The transition from one instrument to its successor instrument ideally would include a period of observational overlap (e.g., 2–5 years) so that self-consistent, multi-instrument data records may be achieved. As an example, near-daily global coverage of the NO2 VCD is currently observed by OMI (since 2004) and its successor, TROPOMI (since 2017) as well as OMPS on Suomi NPP (since 2011), NOAA-20 (since 2017), and NOAA-21 (since 2022). All five instruments have overpass times near 13:30 local time. However, the OMPS instruments are missing visible wavelengths (e.g., < 380 nm for Suomi NPP) as compared to OMI or TROPOMI and have lower spectral resolutions (∼ 1 nm), which result in lower quality NO2 data products than those from either OMI or TROPOMI. The planned successor to TROPOMI, Sentinel-5, will have similar capabilities as TROPOMI but will have an Equator overpass time near 09:30 local time. Therefore, there will be discontinuities in the long-term, record given that NOx emissions, NOx partitioning between NO and NO2, and the vertical distribution of NO2 within the VCD vary throughout the day. Depending on TROPOMI's lifespan, potential successor instruments with overpass times near 13:30 local time, are the NOAA series of OMPS instruments being planned for the Joint Polar Satellite System (JPSS)-3 and JPSS-4 satellites. JPSS-3 and JPSS-4 OMPS will observe additional visible wavelengths relative to those observed by earlier OMPS instruments, but they will not have comparable instrument capabilities (e.g., SNR, spectral coverage) to OMI or TROPOMI. Therefore, the data quality of a self-consistent, multi-instrument data record for NO2 at an overpass time near 13:30 local time from OMI, TROPOMI, and OMPS will be determined by the instrument parameters (e.g., spectral resolution, wavelength range) of the least capable OMPS instrument.
5.1.2 Accuracy/precision and signal-to-noise ratio (SNR)
As discussed in the scientific papers describing the satellite-based approaches (Sect. 2), additional improvements to accuracy/precision and SNR of current observing capabilities are required to advance our ability to constrain OH drivers. For instance, the design of new instruments should emphasize capabilities for in-flight instrument calibration and monitoring, such as solar diffusers and internal light sources. During the manufacturing and integration phase, adequate time and resources should be allocated to allow for thorough pre-flight calibration and characterization. As another example, technology improvements, such as better thermal design and detector cooling, can lead to reduced noise and enhancements in SNR.
5.1.3 Spatio-temporal coverage
In addition to enhanced instrument capabilities, improvements to current spatio-temporal coverage of satellite proxies for OH drivers could be achieved with additional satellites. For instance, the datasets used in the satellite-based approaches (Sect. 2) are from satellites in low-Earth orbit (LEO), so most global locations where OH levels are highest (i.e., tropics and subtropics) are observed daily or every few days. Therefore, a suite of instruments could be placed on multiple satellites, providing observations at various times throughout daylight hours. This strategy would also have the benefit of providing more opportunities to observe non-cloudy conditions (Sect. 5.4), especially if the instruments have finer spatial resolutions than current satellite instruments to allow more observations between clouds.
Instruments on geostationary (GEO) satellites provide an opportunity to assess the value of hourly data to constrain OH. For instance, several new UV/Vis instruments such as the Korean Geostationary Environment Monitoring Spectrometer (GEMS; Kim et al., 2020) and NASA's Tropospheric Emissions: Monitoring of Pollution (TEMPO; Zoogman et al., 2017) observe VCDs of NO2, HCHO, and ozone over East Asia and North America, respectively. ESA's Sentinel-4 GEO satellite with the Ultraviolet–Visible–Near-Infrared (UVN) instrument will make similar observations over Europe and northern Africa (Courrèges-Lacoste et al., 2017). The spatial coverages of these instruments are primarily limited to the midlatitudes and subtropics of the Northern Hemisphere. For hyperspectral TIR instruments on GEO satellites, the Chinese Fengyun Geostationary Interferometric Infrared Sounder already exists (GIIRS; Yang et al., 2017); similar instruments are tentatively planned by space agencies in Japan (Okamoto et al., 2020), Europe (Holmlund et al., 2021), and the US (Lindsey et al., 2024). The spatial coverages of these instruments are more comprehensive, covering most latitudes (i.e., below ∼ 60°) of both hemispheres, as compared to the coverages of the UV/Vis instruments. We recommend coordinated deployment of UV, Vis, NIR, and TIR instruments on GEO satellites to provide co-located observations of satellite proxies for key OH drivers (Sect. 5.2).
5.1.4 Case studies
Ultimately, the design of a space-based observing strategy to constrain tropospheric OH depends on the specific science need or needs to be addressed. For instance, is there a need to constrain OH concentrations, trends, and/or variations, which could require different designs? For what regions and seasons would this be necessary? For illustrative purposes, we provide design considerations for two different potential applications.
In case 1, we wish to constrain the spatio-temporal variations in methane's loss by reaction with OH, which occurs primarily in the lower troposphere of the tropics and subtropics. Given methane's long lifetime, one might think that the spatial and temporal resolutions of inferred tropospheric OH could be low (e.g., several degrees latitude by longitude, seasonal in photochemically homogeneous environments). However, one issue that requires consideration is that the number of pixels with sufficiently low cloud contamination would decrease as the horizontal resolution of observations degrades in cloud-prone areas (e.g., in much of the tropics and subtropics; Sect. 5.4). Therefore, an observational strategy for this case would require finer spatial resolutions for measurements of OH drivers than are required for the spatial resolution of inferred OH. In addition, much of the loss of CH4 occurs over relatively remote ocean environments, so improving the SNR of OH drivers is particularly important, especially since the signals for some important species (e.g., NO2, HCHO) are on par with noise for current instruments (e.g., Zhu et al., 2020). Potentially, new satellite missions can be designed to take advantage of sun glint, where specular reflection over water bodies can enhance the SNR. Over land in the tropics and subtropics, observations of additional VOC constituents, especially isoprene (e.g., Fu et al., 2019; Shutter et al., 2024), are desired to constrain tropospheric OH (Sect. 5.2). Given the strong spatial gradients of OH drivers, spatial resolution should also be prioritized over land as well as over ocean affected by offshore flow. Satellite observations of dynamical variables (e.g., meteorological, climate indices) may also be useful for this case as they can influence OH (Sect. 5.3). For this case, design objectives may be met by multiple instruments with different design objectives (e.g., fine spatial resolution for over land vs. improved SNR for over ocean) or instruments with multiple sampling modes.
In case 2, we wish to constrain tropospheric OH in polluted urban areas. This environment is typically heterogeneous and complicated, requiring fine spatio-temporal resolutions (e.g., kilometers, hourly, or daily) to capture spatial gradients in OH drivers. Additional VOC constituents are highly desired as the urban environment has thousands of VOCs; therefore, observations of VOCs that represent classes of VOCs (e.g., isoprene, fast-reacting alkenes, alkanes, aromatics) are desired and will require multispectral satellite observations (i.e., TIR, NIR, UV/Vis) for the development of these data products (Sect. 5.2). Depending on the timescale of interest, photochemical variables may be sufficient to constrain OH, which can be assumed to be in a photostationary state given its short lifetime (< 1 s). Nevertheless, meteorological variables that influence OH (e.g., temperature, convection) may be desired for studying the photochemical evolution of the urban plume by hour, for instance. For this case, design objectives may be met by observations of UV/Vis (e.g., NO2, HCHO) and TIR (VOCs) wavelengths. Instruments on GEO satellites would provide fine temporal resolution, as opposed to the ones on LEO satellites.
5.2 Assessment of current satellite data to constrain chemical processes that influence OH
We recommend studies to determine the optimal combination of input variables/satellite observables for the satellite-based approaches (Sect. 2) to maximize the interpretability (in a process-based sense) of the causes of trends and variations in tropospheric OH. ML approaches (Sect. 2.2) are able to reproduce OH quite well from the training datasets using various satellite observations; however, the utility of the approaches for attribution studies is determined by the choice of input variables. That is, the suite of input variables must adequately represent the chemical processes of interest that determine OH. For instance, attribution of OH variations and trends to the influence of CH4 is not possible in an ML study if CH4 concentration is not included as an input variable (e.g., Anderson et al., 2024). In addition, ML techniques may not reproduce OH in a process-based sense if the input variables to the ML model are not independent of one another, which is an inherent characteristic of atmospheric chemistry problems (e.g., O3 and NO2, temperature-dependence of most species' lifetimes, co-emitted species such as CH4 and VOCs, and tropospheric species impacted by the same meteorology). Therefore, the causes of trends and variations in tropospheric OH inferred from ML approaches should be carefully interpreted. This is also true for process-based approaches (Sect. 2.1). For example, Pimlott et al. (2022) concluded that their approach “suggests that O3 and CO were the key drivers of variability in the production and loss of OH” for their study period. However, their approach uses input variables observed from a single satellite instrument that does not collect data on NO2, which is responsible for a substantial portion of the variations in tropospheric OH (e.g., Zhu et al., 2022a; Anderson et al., 2023; Baublitz et al., 2023).
5.2.1 A thought experiment
A key question is as follows: what chemical processes are not adequately represented in the current suite of satellite observations that are used in the satellite-based approaches (Sect. 2) to indirectly constrain local and regional concentrations of and trends and variations in tropospheric OH? To answer this question, it is instructive to assess our current ability to constrain individual sources and sinks for tropospheric OH (Table 1). For this thought experiment, we use the annual tropospheric OH budget presented in Table 1 of Lelieveld et al. (2016), noting that the relative importance of the sources and sinks for various regions and seasons can vary substantially from the annual global distribution. In addition, we acknowledge that OH budgets can vary significantly from one CTM to another (Sect. 1). As we will show, the results of our thought experiment, as summarized in Table 1, reveal that the ideal observing strategy for the indirect constraint of the chemical processes that control OH requires co-located observations of the UV/Vis, NIR, and TIR wavelengths.
The first and second sources (i.e., O(1D) + H2O(v) and NO + HO2; Table 1) account for 63 % of the total source in Lelieveld et al. (2016) and are represented relatively well with current satellite datasets. For the second source, satellite observations of NO2 can be used to estimate NO using a CTM; this conversion is a function of a number of variables such as ozone concentrations and photolysis (e.g., de Foy et al., 2015). Lightning is an important source of NOx in the middle and upper troposphere (Allen et al., 2021), which modulates OH there (e.g., Fiore et al., 2006). Satellite observations of lightning flash counts (e.g., over the Americas from the Geostationary Lightning Mapper, GLM, aboard the NOAA Geostationary Operational Environmental Satellite-16, GOES-16 and over Europe, Africa, and the Middle East from EUMETSAT Meteosat Third Generation – Imager 1 (MTG-I1) Lightning Imager) may be useful to constrain the vertical distribution of NO2 using ML or a CTM to relate flash counts to NO2 concentrations. While we do have estimates of tropospheric O3 VCDs, representation of the third source (i.e., O3+ HO2) would benefit from an accurate separation of the stratospheric and tropospheric portions of the total O3 VCD (e.g., Ziemke et al., 2006; Orfanoz-Cheuquelaf et al., 2024) in addition to information on the vertical distribution of tropospheric O3 (Sect. 4.4). We are unaware of satellite proxies for tropospheric HO2, which occurs in both the second and third sources and has a lifetime that is slightly longer than the lifetime of OH. Field campaign data from remote ocean regions indicate that HO2 variation does modulate the rates of the second and third OH source reactions (Fig. 3a, b), but whether possible proxies for HO2 (Fig. 3c) are robust in more complex photochemical regimes is a question that requires further research. A proxy for HO2 may not be necessary for the ML approaches (Sect. 2.2), given that OH and HO2 (i.e., HOx family) share many of the same chemical drivers. There are satellite observations from the Atmospheric Chemistry Experiment (ACE) for H2O2, the fourth source (i.e., H2O2+hv), in the middle to upper troposphere, although this source primarily occurs in the lower troposphere (e.g., Spivakovsky et al., 2000); these observations are sparse, so multi-year averages are required to obtain seasonal zonally averaged distributions (Allen et al., 2013). For satellite proxies that are not yet well supported by current satellite instruments, such as H2O2 and many VOCs (discussed below), simulated distributions may be used in the satellite-based approaches (Sect. 2), especially the distributions that have been well validated with suborbital datasets (Sect. 3). Similar to the argument for HO2, OH and H2O2 share many of the same chemical drivers, so we may not need a satellite proxy for H2O2 in ML approaches (Sect. 2.2). We will discuss the fifth source, which involves VOCs, below. We added the source of OH from the photolysis of nitrous acid (HONO; HONO + hv) to Table 1, although it is not included in Table 1 of Lelieveld et al. (2016). The overall importance of this source on a global scale is uncertain (e.g., Wu et al., 2022; Ha et al., 2023; Zhang et al., 2023), but is thought to be an important source in urban and agricultural environments. Satellite observations of HONO (e.g., TROPOMI, IASI) are primarily limited to intense wildfire plumes (e.g., Theys et al., 2020; Fredrickson et al., 2023; Franco et al., 2024).
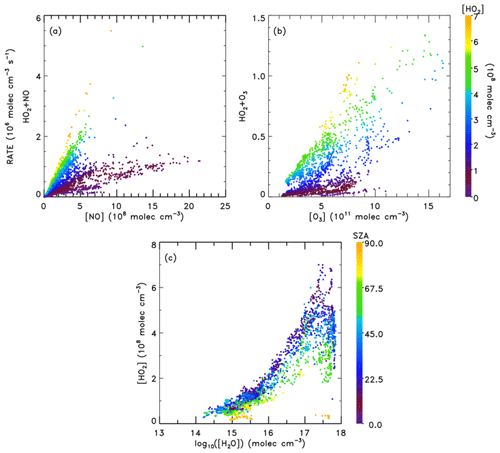
Figure 3Data shown are for tropical over-ocean samples from the ATom-1 field campaign. Box-modeled rates are for the HO2+ NO reaction plotted against the NO concentration (panel a) and the HO2+ O3 reaction vs. the O3 concentration (b), both colored by concentration of HO2. Panel (c) shows the HO2 concentration plotted against log10 of water vapor concentration and colored by solar zenith angle.
The sinks that have straightforward satellite proxies are the second and third sinks (i.e., OH + CH4; OH + CO; e.g., Gaubert et al., 2017; Nguyen et al., 2020; Jacob et al., 2022; Worden et al., 2022), which together represent 51 % of the total tropospheric OH sink from Lelieveld et al. (2016). CH4 is relatively well mixed throughout the troposphere; therefore we could assume a tropospheric distribution informed by in situ observations. For the first sink (i.e., OH + HOy, where HOy= H2, O3, H2O2, radical–radical reaction), there are satellite data for tropospheric O3 and limited data for H2O2, as discussed above. In addition, H2 is relatively well mixed throughout the troposphere; therefore we could assume a tropospheric distribution informed by in situ observations. We will discuss the fourth and fifth sinks, which involve VOCs, below.
Overall, we currently have reasonable satellite proxies to constrain up to 77 % of the global source and up to 51 % of the sink (Table 1) in the annual tropospheric OH budget of Lelieveld et al. (2016). With the development of new VOC data products (e.g., De Longueville et al., 2021; Franco et al., 2022; Brewer et al., 2024; Wells et al., 2024), which may require new technology development, we may well be able to represent a larger portion of the total sources (up to 90 %) and sinks (up to 80 %). To do this would require that we leverage numerous spectral regions (UV/Vis, NIR, TIR) to maximize our ability to represent key source and sink reactions for tropospheric OH with satellite proxy data. An important caveat is that the satellite data products in Table 1 are not of equal quality and require additional research and/or technological development to improve their utility for indirectly constraining OH. A quantitative assessment of each data product's quality should be performed using independent suborbital observations when available (Sect. 3). Such an assessment would benefit from inter-comparable uncertainty characterization between the different data products; however, this is not currently done. Therefore, we recommend the use of a common set of reporting standards to be applied for uncertainty characterization of satellite data products (e.g., von Clarmann et al., 2020), which will allow for a more inter-comparable assessment of the utility of each satellite data product for indirectly constraining tropospheric OH.
We recommend that additional research be a priority to explore the potential of these observable VOCs to constrain tropospheric OH. In Table 1, there is one source (i.e., OVOCs, ROOH + hv; 13 % of total source) and two sinks (i.e., OH + other C1 VOC and OH + C2+ VOC; 29 % of total sink) that would benefit from the development of space-based observations of VOCs, including oxygenated VOCs (OVOCs). Several VOCs are currently observable from space, including in the UV/Vis (HCHO, glyoxal) and TIR (acetic acid, acetone, ethane, ethene, ethyne, formic acid, glycoaldehyde, hydrogen cyanide, isoprene, methanol, and peroxyacetyl nitrate (PAN); e.g., Franco et al., 2018, 2019, 2020, 2022; Wells et al., 2024). Given that it is not feasible to observe the hundreds of individual VOCs in the troposphere and potentially thousands in the polluted urban atmosphere, we recommend that observable VOCs be identified that could represent (1) important classes of compounds (e.g., alkanes, alkenes, aromatics) that contribute to total OH reactivity and (2) markers of various VOC emission sources (e.g., biogenic, anthropogenic, pyrogenic), which have characteristic VOC mixes. Additional constraints of these sources and sinks may benefit from other satellite proxies such as CO (e.g., Baublitz et al., 2024) that covary with VOCs under certain conditions.
We have satellite proxies for the dependence of reactions on temperature and sunlight. The temperature dependence of chemical reactions is represented by satellite observations of temperature (TIR), and continuity is assured with CrIS, for example. The stratospheric O3 VCD (UV/Vis) may serve as a proxy for sunlight (hv), where photochemically important wavelengths are modulated by the thickness of the stratospheric O3 layer. Continuity is assured with several current and upcoming UV/Vis instruments (e.g., TROPOMI, OMPS). Aerosols also modulate the amount of sunlight in the troposphere, affecting tropospheric oxidants (e.g., Martin et al., 2003; Mok et al., 2016; Madronich et al., 2024). There are several potential proxies, such as total and absorption aerosol optical depth and single scattering albedo, that are observed by a number of satellite instruments (e.g., on ESA's Earth Cloud Aerosol and Radiation Explorer (EarthCARE) satellite and NASA's Plankton, Aerosol, Cloud, ocean Ecosystem (PACE) satellite). Additional research is required to develop a combined satellite proxy for spectral solar planar and scalar (i.e., actinic flux) irradiances (e.g., Vasilkov et al., 2022).
In summary, the results of our thought experiment, as summarized in Table 1, reveal that the ideal observing strategy for the indirect constraint of the chemical processes that control OH would require co-located observations of multiple wavelengths in the UV/Vis, NIR, and TIR spectra, which could be integrated into a broader, comprehensive observation strategy for tropospheric chemistry and climate (Millet et al., 2024).
5.2.2 Looking backward
This paper is primarily focused on looking forward – i.e., what is required/desired to improve our ability to constrain tropospheric OH with satellite observations? However, a natural question is how far back in time can we adequately constrain tropospheric OH. Addressing this question and comparing estimates of past OH abundance would increase confidence in our ability to project OH into the future. Answering this question depends on the level of explanatory power that is desired, as discussed above. For instance, one of the first satellite proxies listed in Table 1 is the stratospheric O3 VCD. As shown by Rohrer and Berresheim (2006), tropospheric OH correlates linearly with solar UV irradiance, which is modulated by stratospheric O3. Therefore, one could partially constrain tropospheric OH back to 1979, when satellite measurements of stratospheric O3 became routine (e.g., Stolarski et al., 1986; Weber et al., 2018). As another example, discussion surrounding the continuity of the NO2 VCD (Sect. 5.1.1) started with OMI (launched in 2004), although such observations actually began in 1996 with ESA's Global Ozone Monitoring Experiment (GOME, 1995–2011; Burrows et al., 1999), which was followed by SCanning Imaging Absorption SpectroMeter for Atmospheric CHartographY (SCIAMACHY, 2002–2012), GOME-2 instruments on the Meteorological Operational (METOP) satellites (METOP-A, 2006–2021; B, since 2012; and C, since 2018). All these ESA instruments were/are in morning orbits. Therefore, depending on one's tolerance for data that have relatively coarser resolution, poorer SNR, or a longer time period to obtain global coverage, partial constraint of tropospheric OH with satellite data of NO2 from a morning orbit could extend back to 1996 (e.g., Boersma et al., 2018). Continuity of the morning orbit is planned with Sentinel-5 (anticipated launch in 2025).
Continuity is assured for most satellite proxies (Table 1), and we currently have the ability to adequately or partially constrain most of the sources and sinks of tropospheric OH (Table 1) with the satellite-based approaches (Sect. 2) from the late 1990s/early 2000s to the present. As discussed in Sect. 5.2.1, the primary exception is related to VOCs (e.g., isoprene, which only became available in 2012).
Table 1Satellite proxies for tropospheric source and sink fluxes of OH.
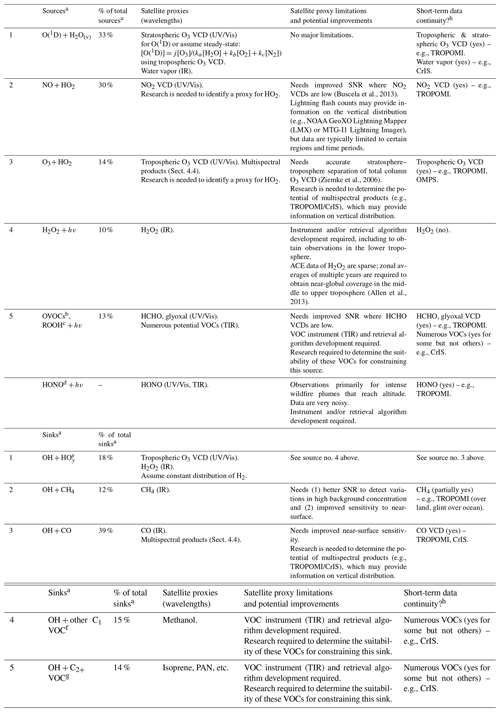
a Reproduced from Table 1 of Lelieveld et al. (2016), except neglecting two minor sinks. b OVOCs = oxygenated VOCs, such as acetone and acetaldehyde. c ROOH = organic peroxides, such as CH3OOH. d HONO is not explicitly listed as a source of OH in Lelieveld et al. (2016), although it is an important source in some environments (Theys et al., 2020; Fredrickson et al., 2023). e HOy= H2, O3, H2O2, radical–radical reaction. f VOC with one C atom (excluding methane), including methanol and C1-reaction products. g VOC with ≥ 2 C atoms, C2+ reaction products. h Defined in Sect. 5.1.1. We focus on current satellite instruments that provide near-complete spatial coverage of the troposphere, neglecting instruments that provide data of, for instance, the upper troposphere (e.g., MLS) and geostationary orbits. We do not list potential future instruments given that space agency priorities and budgets change and because of the history of satellites failing to reach orbit during launch.
5.3 Influence of tropospheric dynamics on OH
We recommend additional research to determine the full potential of using satellite-observable meteorology (e.g., temperature) and climate variables in space-based approaches (Sect. 2) to better understand the dependence of tropospheric OH on dynamics. For instance, climate indices, such as the Multivariate El Niño–Southern Oscillation (ENSO) Index (MEI) and indicators of drought, correlate well with long-term (e.g., monthly, seasonal) variations in tropospheric constituents (e.g., CO, NO2, O3, isoprene, water vapor) that influence OH (e.g., Oman et al., 2011, 2013; Turner et al., 2018; Wells et al., 2020; Anderson et al., 2021, 2023; Shutter et al., 2024), as well as OH itself. Anderson et al. (2021) estimated that ∼ 30 % of simulated variability in global OH in boreal winter is associated with ENSO alone. However, additional proxies may be required to fully capture the complex impact of meteorological variations on the tropospheric constituents that influence OH, including weather-dependent emissions (e.g., CH4, isoprene, lightning NOx) and anthropogenic activities (e.g., Shutter et al., 2024). For instance, Field et al. (2016) reported a nonlinear sensitivity of CO abundance to emissions from fires in Indonesia during El Niño-induced drought, when many fires escape or are intentionally set to clear land on areas with degraded peat. Building on this research, the locations of peat deposits convolved with satellite observations of fire-counts could be used to estimate pollution emitted from these fires. Alternatively, a total sum of pollutant emission estimates (e.g., Global Fire Emissions Database, GFED; van Wees et al., 2022) from Indonesia or the preceding dry season total rainfall in conjunction with the locations of degraded peat could be used to capture the impact of ENSO variations on tropospheric OH.
We recommend additional research to develop process-based diagnostics using satellite and suborbital observations to improve the representation of key atmospheric processes in CTMs that influence OH. Although many of the current knowledge gaps in CTMs (e.g., Table 2 of Fiore et al., 2024) require new laboratory studies, enhancements to current satellite capabilities as well as a more comprehensive suite of suborbital datasets (Sect. 3; e.g., Murray et al., 2021) could be used to develop process-based diagnostics to better constrain the factors that influence OH drivers, such as constraining the response of O3 to ENSO (Oman et al., 2013). Such diagnostics would inform development priorities of CTMs with the goals of improving the simulation of tropospheric OH and aiding interpretation of inferred OH from satellite-based approaches (Sect. 2).
5.4 Addressing the “clear-sky” bias of inferred OH from satellite-based approaches
The presence of clouds limits a satellite's ability to observe tropospheric composition, especially in seasonally cloudy regions. King et al. (2013) used MODIS cloud fraction data to estimate that ∼ 67 % of Earth's surface is covered by clouds at any given time. Consequently, temporal averaging (e.g., over a season) of the data is often employed to achieve statistical significance, although the averages are biased toward clear-sky/low-cloud conditions. We found that these biases in simulated OH (from one CTM) relative to all-sky conditions are substantial (e.g., ±25 % in the tropospheric OH VCDs in the tropics and higher elsewhere; Fig. 4). One way to reduce these biases is to improve the spatial resolutions of satellite instruments, which would increase the chances of collecting observations between broken clouds and, thus, minimize the number of cloud-contaminated pixels (e.g., Frankenberg et al., 2024). There may be viable ways to infer some of the OH drivers over clouds (e.g., NO2; Marais et al., 2018, 2021; CO, Landgraf et al., 2016), but further study is needed.
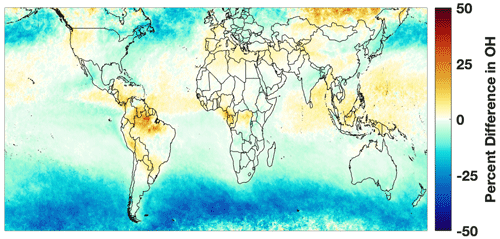
Figure 4Simulated percent difference in tropospheric column OH between low-cloud (cloud fraction less than 30 %) and all-sky conditions. Red indicates higher OH abundance in near-clear-sky conditions; blue indicates lower OH abundance under such conditions. Simulated data are from the MERRA-2 GMI simulation and averaged over 2005–2019 for October.
We recommend additional research to identify ways to gap fill OH inferred from the satellite-based approaches (Sect. 2). Potential ways are to (1) use simulated OH, which would benefit from model development priorities informed by satellite-constrained, process-based diagnostics (Sect. 5.3); (2) scale inferred OH by the ratio of observed OH in clear and cloudy conditions from tropospheric composition field campaigns (Sect. 3); and (3) use a photolysis model with satellite-observable cloud properties (e.g., cloud optical depth, cloud top pressure, and cloud fraction) to scale inferred OH. The problem with the second way is that cloudy regions are typically avoided during field campaigns. Therefore, a priority of future field campaigns is to collect observations of OH and its drivers in both clear and cloudy environments. Finally, there is the potential to develop an ML model using simulated tropospheric OH with meteorological variables and climate indices (Sect. 5.3), which are observable from space, to understand the relationships of OH concentrations, trends, and variations in cloudy and mostly cloudy environments. All of these potential methods to gap fill inferred OH require further study.
The satellite-based approaches (Sect. 2) offer the promise that we may achieve constraint of tropospheric OH on spatio-temporal scales that has been previously unobtainable. Given this promise, we make recommendations to facilitate the design of a robust observing strategy of satellite and suborbital observations of atmospheric constituents and dynamical variables to indirectly constrain concentrations of and trends and variations in tropospheric OH. The increased spatio-temporal information about tropospheric OH that could be gained by following these recommendations would advance our ability to determine the causes of trends and variations in the concentrations of atmospheric constituents that are influenced by OH. For instance, Turner et al. (2016) concluded that “the current surface observing system does not allow unambiguous attribution of the decadal trends in methane without robust constraints on OH variability, which currently rely purely on methyl chloroform data and its uncertain emissions estimate.”
We recommend the development of a comprehensive satellite-based approach to maximize the indirect constraint of tropospheric OH that may be composed of a combination of existing satellite-based approaches (Sect. 2) and new satellite-based approaches developed for specific photochemical environments. Studies are needed to determine the optimal combination of input variables/satellite observables for some of the satellite-based approaches to maximize the interpretability (in a process-based sense) of the causes of trends and variations in tropospheric OH. The design process would benefit from trade-space and cost–benefit analyses, including consideration of incorporating more cost-effective suborbital observations where feasible.
Priorities (in no particular order) for this observing strategy include the following:
-
continuity of satellite instruments with similar or enhanced capabilities;
-
improvements to accuracy/precision and SNR of current observing capabilities through instrument design;
-
additional LEO and GEO satellites to obtain greater spatio-temporal coverage;
-
development of a robust suborbital observing network composed of instruments at stationary sites (OH supersites) as well as ones deployed in field campaigns to collect suborbital observations of OH drivers, including in cloudy environments;
-
a common set of reporting standards to be applied for uncertainty characterization of satellite data products, which will allow for a more robust assessment of the utility of each satellite data product for indirectly constraining tropospheric OH; and
-
coordinated deployment of UV, Vis, NIR, and TIR instruments to provide co-located observations of satellite proxies for key OH drivers.
We also recommend additional investment in research (in no particular order) to
-
maximize the vertical information of the observations of OH drivers (e.g., with multispectral data products);
-
maintain self-consistent and trend-quality L1B radiances for the generation of multi-agency, multi-instrument data records;
-
determine the full potential of using satellite-observable meteorology (e.g., temperature, clouds, aerosols) and climate variables in space-based approaches to better understand the dependence of OH concentrations, trends, and variations on dynamics;
-
develop process-based diagnostics using satellite and suborbital observations to improve the representation of key atmospheric processes in CTMs that influence OH;
-
explore the potential of observable VOCs to aid constraint of tropospheric OH; and
-
address the clear-sky bias of inferred OH from satellite-based approaches.
Finally, we recommend additional investment in technology development that may lead to passive and/or active space-based instruments that directly observe OH.
Figure 1 – data are available upon request from Daniel C. Anderson (daniel.c.anderson@nasa.gov).
Figure 2 – satellite retrievals for TEMPO HCHO V3 data can be found at https://doi.org/10.5067/IS-40e/TEMPO/HCHO_L2.003 and the radiance v3 data at https://doi.org/10.5067/IS-40e/TEMPO/HCHO_L2.003 (NASA/LARC/SD/ASDC, 2024a), and the irradiance v3 data at https://doi.org/10.5067/IS-40e/TEMPO/IRR_L1.003 (NASA/LARC/SD/ASDC, 2024b; González Abad et al., 2024).
Figure 3 – data from the ATom campaign are located at https://doi.org/10.3334/ORNLDAAC/1925 (Wofsy et al., 2021).
Figure 4 – output from the MERRA-2 GMI simulation is publicly available at https://acd-ext.gsfc.nasa.gov/Projects/GEOSCCM/MERRA2GMI/ (NASA Goddard Space Flight Center, 2024).
BND initiated the opinion piece and wrote the first draft. All co-authors contributed to the overall content and direction of the paper through many collaborative discussions. Many co-authors contributed text and all figures. BW contributed Sect. 2.3. JMSC and GMW contributed heavily to Sect. 3. CL, JJ, and NAK contributed Sect. 4.1. DBM contributed Sect. 4.2. HMW contributed Sect. 4.3. DCA contributed Figs. 1 and 4. JJ contributed Figure 2. JMN contributed Fig. 3, including performing the associated analysis.
At least one of the (co-)authors is a member of the editorial board of Atmospheric Chemistry and Physics. The peer-review process was guided by an independent editor, and the authors also have no other competing interests to declare.
Publisher’s note: Copernicus Publications remains neutral with regard to jurisdictional claims made in the text, published maps, institutional affiliations, or any other geographical representation in this paper. While Copernicus Publications makes every effort to include appropriate place names, the final responsibility lies with the authors.
We would like to thank the NASA Goddard Space Flight Center's Earth Sciences Division for supporting NASA personnel for this effort with Strategic Science funding.
This paper was edited by Barbara Ervens and Andreas Hofzumahaus and reviewed by Maarten Krol and one anonymous referee.
Allen, D., Pickering, K. E., Bucsela, E., Van Geffen, J., Lapierre, J., Koshak, W., and Eskes, H.: Observations of lightning NOx production from Tropospheric Monitoring Instrument case studies over the United States, J. Geophys. Res.-Atmos., 126, e2020JD034174, https://doi.org/10.1029/2020JD034174, 2021.
Allen, N. C. C., Abad, G. G., Bernath, P. F., and Boone, C. D.: Satellite observations of the global distribution of hydrogen dioxide (H2O2) from ACE, J. Quant. Spectrosc. Ra., 115, January 2013, 66–77, https://doi.org/10.1016/j.jqsrt.2012.09.008, 2013.
Anderson, D. C., Duncan, B. N., Fiore, A. M., Baublitz, C. B., Follette-Cook, M. B., Nicely, J. M., and Wolfe, G. M.: Spatial and temporal variability in the hydroxyl (OH) radical: understanding the role of large-scale climate features and their influence on OH through its dynamical and photochemical drivers, Atmos. Chem. Phys., 21, 6481–6508, https://doi.org/10.5194/acp-21-6481-2021, 2021.
Anderson, D. C., Follette-Cook, M. B., Strode, S. A., Nicely, J. M., Liu, J., Ivatt, P. D., and Duncan, B. N.: A machine learning methodology for the generation of a parameterization of the hydroxyl radical, Geosci. Model Dev., 15, 6341–6358, https://doi.org/10.5194/gmd-15-6341-2022, 2022.
Anderson, D. C., Duncan, B. N., Nicely, J. M., Liu, J., Strode, S. A., and Follette-Cook, M. B.: Technical note: Constraining the hydroxyl (OH) radical in the tropics with satellite observations of its drivers – first steps toward assessing the feasibility of a global observation strategy, Atmos. Chem. Phys., 23, 6319–6338, https://doi.org/10.5194/acp-23-6319-2023, 2023.
Anderson, D. C., Duncan, B. N., Liu, J., Nicely, J. M., Strode, S. A., Follette-Cook, M. B., Souri, A. H., Ziemke, J.R., Gonzalez-Abad, G., and Ayazpour, Z.: Trends and interannual variability of the hydroxyl radical in the remote tropics during boreal autumn inferred from satellite proxy data, Geophys. Res. Lett., 51, e2024GL108531, https://doi.org/10.1029/2024GL108531, 2024.
Baublitz, C. B., Fiore, A. M., Ludwig, S. M., Nicely, J. M., Wolfe, G. M., Murray, L. T. Commane, R., Prather, M. J., Anderson, D. C. Correa, G. Duncan, B. N., Follette-Cook, M., Westervelt, D. M., Bourgeois, I., Brune, W. H., Bui, T. P. DiGangi, J. P., Diskin, G. S., Hall, S. R., McKain, K., Miller, D. O., Peischl, J., Thames, A. B., Thompson, C. R., Ullmann, K., and Wofsy, S. C.: An observation-based, reduced-form model for oxidation in the remote marine troposphere, P. Natl. Acad. Sci. USA, 120, e2209735120, https://doi.org/10.1073/pnas.2209735120, 2023.
Bocquet, M., Elbern, H., Eskes, H., Hirtl, M., Žabkar, R., Carmichael, G. R., Flemming, J., Inness, A., Pagowski, M., Pérez Camaño, J. L., Saide, P. E., San Jose, R., Sofiev, M., Vira, J., Baklanov, A., Carnevale, C., Grell, G., and Seigneur, C.: Data assimilation in atmospheric chemistry models: current status and future prospects for coupled chemistry meteorology models, Atmos. Chem. Phys., 15, 5325–5358, https://doi.org/10.5194/acp-15-5325-2015, 2015.
Boersma, K. F., Eskes, H. J., Richter, A., De Smedt, I., Lorente, A., Beirle, S., van Geffen, J. H. G. M., Zara, M., Peters, E., Van Roozendael, M., Wagner, T., Maasakkers, J. D., van der A, R. J., Nightingale, J., De Rudder, A., Irie, H., Pinardi, G., Lambert, J.-C., and Compernolle, S. C.: Improving algorithms and uncertainty estimates for satellite NO2 retrievals: results from the quality assurance for the essential climate variables (QA4ECV) project, Atmos. Meas. Tech., 11, 6651–6678, https://doi.org/10.5194/amt-11-6651-2018, 2018.
Bottorff, B., Lew, M. M., Woo, Y., Rickly, P., Rollings, M. D., Deming, B., Anderson, D. C., Wood, E., Alwe, H. D., Millet, D. B., Weinheimer, A., Tyndall, G., Ortega, J., Dusanter, S., Leonardis, T., Flynn, J., Erickson, M., Alvarez, S., Rivera-Rios, J. C., Shutter, J. D., Keutsch, F., Helmig, D., Wang, W., Allen, H. M., Slade, J. H., Shepson, P. B., Bertman, S., and Stevens, P. S.: OH, HO2, and RO2 radical chemistry in a rural forest environment: measurements, model comparisons, and evidence of a missing radical sink, Atmos. Chem. Phys., 23, 10287–10311, https://doi.org/10.5194/acp-23-10287-2023, 2023.
Brewer, J. F., Millet, D. B., Wells, K. C., Payne, V. H., Kulawik, S., Vigouroux, C., Cady-Pereira, K. E., Pernak, R., and Zhou, M.: Space-based observations of tropospheric ethane map emissions from fossil fuel extraction, Nat. Commun., 15, 7829, https://doi.org/10.1038/s41467-024-52247-z, 2024.
Brune, W. H., Ren, X., Zhang, L., Mao, J., Miller, D. O., Anderson, B. E., Blake, D. R., Cohen, R. C., Diskin, G. S., Hall, S. R., Hanisco, T. F., Huey, L. G., Nault, B. A., Peischl, J., Pollack, I., Ryerson, T. B., Shingler, T., Sorooshian, A., Ullmann, K., Wisthaler, A., and Wooldridge, P. J.: Atmospheric oxidation in the presence of clouds during the Deep Convective Clouds and Chemistry (DC3) study, Atmos. Chem. Phys., 18, 14493–14510, https://doi.org/10.5194/acp-18-14493-2018, 2018.
Brune, W. H., Miller, D. O., Thames, A. B., Allen, H. M., Apel, E. C., Blake, D. R., Bui, T. P., Commane, R., Crounse, J. D., Daube, B. C., Diskin, G. S., DiGangi, J. P., Elkins, J. W., Hall, S. R., Hanisco, T. F., Hannun, R. A., Hintsa, E. J., Hornbrook, R. S., Kim, M. J., McKain, K., Moore, F. L., Neuman, J. A., Nicely, J. M., Peischl, J., Ryerson, T. B., St. Claire, J. M., Sweeney, C., Teng, A. P., Thompson, C., Ullmann, K., Veres, P. R., Wennberg, P. O., and Wolfe, G. M.: Exploring oxidation in the remote free troposphere: Insights from Atmospheric Tomography (ATom), J. Geophys. Res.-Atmos., 125, 1–17, https://doi.org/10.1029/2019JD031685, 2020.
Bucsela, E. J., Krotkov, N. A., Celarier, E. A., Lamsal, L. N., Swartz, W. H., Bhartia, P. K., Boersma, K. F., Veefkind, J. P., Gleason, J. F., and Pickering, K. E.: A new stratospheric and tropospheric NO2 retrieval algorithm for nadir-viewing satellite instruments: applications to OMI, Atmos. Meas. Tech., 6, 2607–2626, https://doi.org/10.5194/amt-6-2607-2013, 2013.
Burrows, J. P., Weber, M., Buchwitz, M., Rozanov, V., Ladstätter-Weißenmayer, A., Richter, A., DeBeek., R., Hoogen, R., Bramstedt, K., Eichmann, K. U., Eisinger, M., and Perner, D.: The Global Ozone Monitoring Experiment (GOME): Mission Concept and First Scientific Results, J. Atmos. Sci., 56, 151–175, https://doi.org/10.1175/1520-0469(1999)056<0151:TGOMEG>2.0.CO;2, 1999.
Cageao, R. P., Blavier, J.-F., McGuire, J. P., Jiang, Y., Nemtchinov, V., Mills, F. P., and Sander, S. P.: High-resolution Fourier-transform ultraviolet-visible spectrometer for the measurement of atmospheric trace species: Application to OH, Appl. Opt., 40, 2024–2030, https://doi.org/10.1364/AO.40.002024, 2001.
Clarisse, L., R'Honi, Y., Coheur, P.-F., Hurtmans, D., and Clerbaux, C.: Thermal infrared nadir observations of 24 atmospheric gases, Geophys. Res. Lett., 38, L10802, https://doi.org/10.1029/2011GL047271, 2011.
Colombi, N., Miyazaki, K., Bowman, K. W., Neu, J. L., and Jacob, D. J.: A new methodology for inferring surface ozone from multispectral satellite measurements, Environ. Res. Lett., 16, 105005, https://doi.org/10.1088/1748-9326/ac243d, 2021.
Courrèges-Lacoste, G. B., Sallusti, M., Bulsa, G., Bagnasco, G., Veihelmann, B., Riedl, S., Smith, D., J., and Maurer, R.: The Copernicus Sentinel 4 mission: A geostationary imaging UVN spectrometer for air quality monitoring, Proc. SPIE, 10423, 1042307, https://doi.org/10.1117/12.2282158, 2017.
de Foy, B., Lu, Z., Streets, D. G., Lamsal, L., and Duncan, B. N.: Estimates of power plant NOx emissions and lifetimes from OMI NO2 satellite retrievals, Atmos. Environ., 116, 1–11, https://doi.org/10.1016/j.atmosenv.2015.05.056, 2015.
De Longueville, H., Clarisse, L., Whitburn, S., Franco, B., Bauduin, S., Clerbaux, C., Camy-Peyret, C., and Coheur, P.-F.: Identification of short and long-lived atmospheric trace gases from IASI space observations, Geophys. Res. Lett., 48, e2020GL091742, https://doi.org/10.1029/2020GL091742, 2021.
Duncan, B. N., Prados, A. I., Lamsal, L., Liu, Y., Streets, D., Gupta, P., Hilsenrath, E., Kahn, R. A., Nielsen, J. E., Beyersdorf, A., Burton, S., Fiore, A., Fishman, J., Henze, D., Hostetler, C., Krotkov, N. A., Lee, P., Lin, M., Pawson, S., Pfister, G., Pickering, K. E., Pierce, R. B., Yoshida, Y., and Ziemba, L.: Satellite data of atmospheric pollution for U.S. air quality applications: Examples of applications, summary of data end-user resources, answers to FAQs, and common mistakes to avoid, Atmos. Environ., 94, 647–662, https://doi.org/10.1016/j.atmosenv.2014.05.061, 2014.
Elshorbany, Y. F., Duncan, B. N., Strode, S. A., Wang, J. S., and Kouatchou, J.: The description and validation of the computationally Efficient CH4–CO–OH (ECCOHv1.01) chemistry module for 3-D model applications, Geosci. Model Dev., 9, 799–822, https://doi.org/10.5194/gmd-9-799-2016, 2016.
Field, R. D., van der Werf, G.R., Fanin, T., Fetzer, E. J., Fuller, R., Jethva, H., Levy, R., Livesey, N. J., Luo, M., Torres, O., and Worden, H.: Indonesian fire activity and smoke pollution in 2015 show persistent nonlinear sensitivity to El Niño-induced drought, P. Natl. Acad. Sci. USA, 113, 9204-9209, https://doi.org/10.1073/pnas.1524888113, 2016.
Fiore, A., Mickley, M., Zhu, Q., and Baublitz, C.: Climate and Tropospheric Oxidizing Capacity, Ann. Rev. EPS, 52, 321–349, https://doi.org/10.1146/annurev-earth-032320-090307, 2024.
Fiore, A. M., Horowitz, L. W., Dlugokencky, E. J., and West, J. J.: Impact of meteorology and emissions on methane trends, 1990–2004, Geophys. Res. Lett., 33, L12809, https://doi.org/10.1029/2006GL026199, 2006.
Franco, B., Clarisse, L., Stavrakou, T., Müller, J. -F, Van Damme, M., Whitburn, S., Hadji-Lazaro, J., Hurtmans, D., Taraborrelli, D., Clerbaux, C., and Coheur, P.-F.: A general framework for global retrievals of trace gases from IASI: Application to methanol, formic acid, and PAN, J. Geophys. Res., 123, 13963–13984, https://doi.org/10.1029/2018JD029633, 2018.
Franco, B., Clarisse, L., Stavrakou, T., Müller, J. -F., Pozzer, A., Hadji-Lazaro, J., Hurtmans, D., Clerbaux, C., and Coheur, P.-F.: Acetone atmospheric distribution retrieved from space, Geophys. Res. Lett., 46, 2884–2893, https://doi.org/10.1029/2019GL082052, 2019.
Franco, B., Clarisse, L., Stavrakou, T., Müller, J. -F., Taraborrelli, D., Hadji-Lazaro, J., Hannigan, J. W., Hase, F., Hurtmans, D., Jones, N., Lutsch, E., Mahieu, E., Ortega, I., Schneider, M., Strong, K., Vigouroux, C., Clerbaux, C., and Coheur, P.-F.: Spaceborne measurements of formic and acetic acids: A global view of the regional sources, Geophys. Res. Lett., 47, e2019GL086239, https://doi.org/10.1029/2019GL086239, 2020.
Franco, B., Clarisse, L., Van Damme, M., Hadji-Lazaro, J., Clerbaux, C., and Coheur, P.-F.: Ethylene industrial emitters seen from space, Nat. Commun., 13, 6452, https://doi.org/10.1038/s41467-022-34098-8, 2022.
Franco, B., Clarisse, L., Theys, N., Hadji-Lazaro, J., Clerbaux, C., and Coheur, P.: Pyrogenic HONO seen from space: insights from global IASI observations, Atmos. Chem. Phys., 24, 4973–5007, https://doi.org/10.5194/acp-24-4973-2024, 2024.
Frankenberg, C., Bar-On, Y. M., Yin, Y., Wennberg, P. O., Jacob, D. J., and Michalak, A. M.: Data drought in the humid tropics: How to overcome the cloud barrier in greenhouse gas remote sensing, Geophys. Res. Lett., 51, e2024GL108791, https://doi.org/10.1029/2024GL108791, 2024.
Fredrickson, C. D., Theys, N., and Thornton, J. A.: Satellite evidence of HONO/NO2 increase with fire radiative power, Geophys. Res. Lett., 50, e2023GL103836, https://doi.org/10.1029/2023GL103836, 2023.
Fu, D., Worden, J. R., Liu, X., Kulawik, S. S., Bowman, K. W., and Natraj, V.: Characterization of ozone profiles derived from Aura TES and OMI radiances, Atmos. Chem. Phys., 13, 3445–3462, https://doi.org/10.5194/acp-13-3445-2013, 2013.
Fu, D., Bowman, K. W., Worden, H. M., Natraj, V., Worden, J. R., Yu, S., Veefkind, P., Aben, I., Landgraf, J., Strow, L., and Han, Y.: High-resolution tropospheric carbon monoxide profiles retrieved from CrIS and TROPOMI, Atmos. Meas. Tech., 9, 2567–2579, https://doi.org/10.5194/amt-9-2567-2016, 2016.
Fu, D., Kulawik, S. S., Miyazaki, K., Bowman, K. W., Worden, J. R., Eldering, A., Livesey, N. J., Teixeira, J., Irion, F. W., Herman, R. L., Osterman, G. B., Liu, X., Levelt, P. F., Thompson, A. M., and Luo, M.: Retrievals of tropospheric ozone profiles from the synergism of AIRS and OMI: methodology and validation, Atmos. Meas. Tech., 11, 5587–5605, https://doi.org/10.5194/amt-11-5587-2018, 2018.
Fu, D., Millet, D.B., Wells, K.C., Payne, V. H., Yu, S., Guenter, A., and Eldering, A.: Direct retrieval of isoprene from satellite-based infrared measurements, Nat. Commun., 10, 3811, https://doi.org/10.1038/s41467-019-11835-0, 2019.
Fuchs, H., Tan, Z., Lu, K., Bohn, B., Broch, S., Brown, S. S., Dong, H., Gomm, S., Häseler, R., He, L., Hofzumahaus, A., Holland, F., Li, X., Liu, Y., Lu, S., Min, K.-E., Rohrer, F., Shao, M., Wang, B., Wang, M., Wu, Y., Zeng, L., Zhang, Y., Wahner, A., and Zhang, Y.: OH reactivity at a rural site (Wangdu) in the North China Plain: contributions from OH reactants and experimental OH budget, Atmos. Chem. Phys., 17, 645–661, https://doi.org/10.5194/acp-17-645-2017, 2017.
Gaubert, B., Arellano, A. F., Barré, J., Worden, H. M., Emmons, L. K., Tilmes, S., Buchholz, R. R., Vitt, F., Raeder, K., Collins, N., Anderson, J. L., Wiedinmyer, C., Martinez Alonso, S., Hannigan, J. W., Petri, C., Strong, K., and Jones, N.: Toward a chemical reanalysis in a coupled chemistry climate model: An evaluation of MOPITT CO assimilation and its impact on tropospheric composition, J. Geophys. Res.-Atmos., 121, 7310–7343, https://doi.org/10.1002/2016JD024863, 2016.
Gaubert, B., Worden, H. M., Arellano, A. F. J., Emmons, L. K., Tilmes, S., Barré, J., Martinez Alonso, S., Vitt, F., Anderson, J. L., Alkemade, F., Houweling, S., and Edwards, D. P.: Chemical feedback from decreasing carbon monoxide emissions, Geophys. Res. Lett., 44, 9985–9995, https://doi.org/10.1002/2017GL074987, 2017.
González Abad, G., Vasilkov, A., Seftor, C., Liu, X., and Chance, K.: Smithsonian Astrophysical Observatory Ozone Mapping and Profiler Suite (SAO OMPS) formaldehyde retrieval, Atmos. Meas. Tech., 9, 2797–2812, https://doi.org/10.5194/amt-9-2797-2016, 2016.
González Abad, G., Nowlan, C., Wang, H. Chong, H., Houck, J., Liu, X., and Chance, K.: Tropospheric Emissions: Monitoring Of Pollution (TEMPO) Project Trace Gas and Cloud Level 2 and 3 Data Products: User Guide [data set], https://asdc.larc.nasa.gov/documents/tempo/guide/TEMPO_Level-2-3_trace_gas_clouds_user_guide_V1.0.pdf (last access: 10 July 2024), 2024.
Ha, P. T. M., Kanaya, Y., Taketani, F., Andrés Hernández, M. D., Schreiner, B., Pfeilsticker, K., and Sudo, K.: Implementation of HONO into the chemistry–climate model CHASER (V4.0): roles in tropospheric chemistry, Geosci. Model Dev., 16, 927–960, https://doi.org/10.5194/gmd-16-927-2023, 2023.
Hansen, R. F., Griffith, S. M., Dusanter, S., Gilman, J. B., Graus, M., Kuster, W. C., Veres, P. R., de Gouw, J. A., Warneke, C., Washenfelder, R. A., Young, C. J., Brown S. S., Alvarez, S. L., Flynn, J. H., Grossberg, N. E., Lefer, B., Rappenglueck, B., and Stevens, P. S.: Measurements of total OH reactivity during CalNex-LA, J. Geophys. Res.-Atmos., 126, e2020JD032988, https://doi.org/10.1029/2020JD032988, 2021.
Holmlund, K., Grandell, J., Schmetz, J., Stuhlmann, R., Bojkov, B., Munro, R., Lekouara, M., Coppens, D., Viticchie, B., August, T., Theodore, B., Watts, P., Dobber, M., Fowler, G., Bojinski, S., Schmid, A., Salonen, K., Tjemkes, S., Aminou, D., and Blythe, P.: Meteosat Third Generation (MTG): Continuation and innovation of observations from geostationary orbit, B. Am. Meteorol. Soc., 102, E990–E1015, https://doi.org/10.1175/BAMS-D-19-0304.1, 2021.
Jacob, D. J., Varon, D. J., Cusworth, D. H., Dennison, P. E., Frankenberg, C., Gautam, R., Guanter, L., Kelley, J., McKeever, J., Ott, L. E., Poulter, B., Qu, Z., Thorpe, A. K., Worden, J. R., and Duren, R. M.: Quantifying methane emissions from the global scale down to point sources using satellite observations of atmospheric methane, Atmos. Chem. Phys., 22, 9617–9646, https://doi.org/10.5194/acp-22-9617-2022, 2022.
Joiner, J., Marchenko, S., Fasnacht, Z., Lamsal, L., Li, C., Vasilkov, A., and Krotkov, N.: Use of machine learning and principal component analysis to retrieve nitrogen dioxide (NO2) with hyperspectral imagers and reduce noise in spectral fitting, Atmos. Meas. Tech., 16, 481–500, https://doi.org/10.5194/amt-16-481-2023, 2023.
Joiner, J., Yoshida, Y., Guanter, L., Lamsal, L., Li, C., Fasnacht, Z., Kohler, P., Frankenberg, C., Sun, Y., and Parazoo, N.: Noise reduction for solar-induced fluorescence retrievals using machine learning and principal component analysis: simulations and applications to GOME-2 satellite retrievals, Artificial Intelligence for the Earth Systems, https://doi.org/10.1175/aies-d-23-0085.1, 2024.
Kim, J., Jeong, U., Ahn, M., Kim, J., Park, R., Lee, H., Song, C., Choi, Y., Lee, K., Yoo, J., Jeong, M., Park, S., Lee, K., Song, C., Kim, S., Kim, Y., Kim, S., Kim, M., Go, S., Liu, X., Chance, K., Chan Miller, C., Al-Saadi, J., Veihelmann, B., Bhartia, P., Torres, O., Abad, G., Haffner, D., Ko, D., Lee, S., Woo, J., Chong, H., Park, S., Nicks, D., Choi, W., Moon, K., Cho, A., Yoon, J., Kim, S., Hong, H., Lee, K., Lee, H., Lee, S., Choi, M., Veefkind, P., Levelt, P., Edwards, D., Kang, M., Eo, M., Bak, J., Baek, K., Kwon, H., Yang, J., Park, J., Han, K., Kim, B., Shin, H., Choi, H., Lee, E., Chong, J., Cha, Y., Koo, J., Irie, H., Hayashida, S., Kasai, Y., Kanaya, Y., Liu, C., Lin, J., Crawford, J., Carmichael, G., Newchurch, M., Lefer, B., Herman, J., Swap, R., Lau, A., Kurosu, T., Jaross, G., Ahlers, B., Dobber, M., McElroy, C., and Choi, Y.: New era of air quality monitoring from space: Geostationary Environment Monitoring Spectrometer (GEMS), B. Am. Meteorol. Soc., 101, E1–E22, https://doi.org/10.1175/BAMS-D-18-0013.1, 2020.
King, M. D., Platnick, S. E., Menzel, W. P., Ackerman, S. A., and Hubanks, P. A.: Spatial and temporal distribution of clouds observed by MODIS onboard the Terra and Aqua satellites, IEEE T. Geosci. Remote, 51, 3826–3852, https://doi.org/10.1109/TGRS.2012.2227333, 2013.
Lamsal, L. N., Krotkov, N. A., Vasilkov, A., Marchenko, S., Qin, W., Yang, E.-S., Fasnacht, Z., Joiner, J., Choi, S., Haffner, D., Swartz, W. H., Fisher, B., and Bucsela, E.: Ozone Monitoring Instrument (OMI) Aura nitrogen dioxide standard product version 4.0 with improved surface and cloud treatments, Atmos. Meas. Tech., 14, 455–479, https://doi.org/10.5194/amt-14-455-2021, 2021.
Landgraf, J., aan de Brugh, J., Scheepmaker, R., Borsdorff, T., Hu, H., Houweling, S., Butz, A., Aben, I., and Hasekamp, O.: Carbon monoxide total column retrievals from TROPOMI shortwave infrared measurements, Atmos. Meas. Tech., 9, 4955–4975, https://doi.org/10.5194/amt-9-4955-2016, 2016.
Lelieveld, J., Dentener, F. J., Peters, W., and Krol, M. C.: On the role of hydroxyl radicals in the self-cleansing capacity of the troposphere, Atmos. Chem. Phys., 4, 2337–2344, https://doi.org/10.5194/acp-4-2337-2004, 2004.
Lelieveld, J., Brenninkmeijer, C., Joeckel, P., Isaksen, I., Krol, M., Mak, J., Dlugokencky, E., Montzka, S., Novelli, P., and Peters, W.: New Directions: Watching over tropospheric hydroxyl (OH), Atmos. Environ., 40, 5741–5743, https://doi.org/10.1016/j.atmosenv.2006.04.008, 2006.
Lelieveld, J., Gromov, S., Pozzer, A., and Taraborrelli, D.: Global tropospheric hydroxyl distribution, budget and reactivity, Atmos. Chem. Phys., 16, 12477–12493, https://doi.org/10.5194/acp-16-12477-2016, 2016.
Lew, M. M., Rickly, P. S., Bottorff, B. P., Reidy, E., Sklaveniti, S., Léonardis, T., Locoge, N., Dusanter, S., Kundu, S., Wood, E., and Stevens, P. S.: OH and HO2 radical chemistry in a midlatitude forest: measurements and model comparisons, Atmos. Chem. Phys., 20, 9209–9230, https://doi.org/10.5194/acp-20-9209-2020, 2020.
Li, C., Joiner, J., Liu, F., Krotkov, N. A., Fioletov, V., and McLinden, C.: A new machine-learning-based analysis for improving satellite-retrieved atmospheric composition data: OMI SO2 as an example, Atmos. Meas. Tech., 15, 5497–5514, https://doi.org/10.5194/amt-15-5497-2022, 2022.
Liang, Q., Chipperfield, M. P., Fleming, E. L., Abraham, N. L., Braesicke, P., Burkholder, J. B., Daniel, J. S., Dhomse, S., Fraser, P. J., Hardiman, S., Jackman, C. H., Kinnison, D. E., Krummel, P. A. B., Montzka, S. A., Morgenstern, O., McCulloch, A., Muhle, J., Newman, p. A., Orkin, V. L., Pitari, G., Prinn, R. G., Rigby, M., Rozanov, E., Stenke, A., Tummon, F., Velders, G. J. M., Visioni, D., and Weiss, R. F.: Deriving global OH abundance and atmospheric lifetimes for long-lived gases: A search for CH3CCl3 alternatives, J. Geophys. Res.-Atmos., 122, 11914–11933, https://doi.org/10.1002/2017JD026926, 2017.
Liao, J., Wolfe, G. M., Kotsakis, A. E., Nicely, J. M., St. Clair, J. M., Hanisco, T. F., Gonzalez Abad, G., Nowlan, C. R., Ayazpour, Z., De Smedt, I., Apel, E. C., and Hornbrook, R. S.: Validation of formaldehyde products from three satellite retrievals (OMI SAO, OMPS-NPP SAO, and OMI BIRA) in the marine atmosphere with four seasons of ATom aircraft observations, Atmos. Meas. Tech. Discuss. [preprint], https://doi.org/10.5194/amt-2024-72, in review, 2024.
Lindsey, D. T., Heidinger, A. K., Sullivan, P. C., McCorkel, J., Schmit, T. J., Tomlinson, M., Vandermeulen, R., Frost, G. J., Kondragunta, S., and Rudlosky, S.: GeoXO: NOAA's Future Geostationary Satellite System, B. Am. Meteorol. Soc., 105, E660–E679, https://doi.org/10.1175/BAMS-D-23-0048.1, 2024.
Lorente, A., Folkert Boersma, K., Yu, H., Dörner, S., Hilboll, A., Richter, A., Liu, M., Lamsal, L. N., Barkley, M., De Smedt, I., Van Roozendael, M., Wang, Y., Wagner, T., Beirle, S., Lin, J.-T., Krotkov, N., Stammes, P., Wang, P., Eskes, H. J., and Krol, M.: Structural uncertainty in air mass factor calculation for NO2 and HCHO satellite retrievals, Atmos. Meas. Tech., 10, 759–782, https://doi.org/10.5194/amt-10-759-2017, 2017.
Madronich, S., Bernhard, G. H., Neale. R. E., Neale, P. J., Heikkilä, A., Sulbæk Andersen, M. P., Andrady, A. L., Aucamp, P. J., Bais, A. F., Banaszak, A. T., Barnes, P. J., Bornman, J. F., Bruckman, L. S., Busquets, R., Chiodo, G., Häder, D.-P., Hanson, M. L., Hylander, S., Jansen, M. A. K., Lucas, R. M., Mackenzie Calderon, R., Olsen, C., Ossola, R., Pandey, K., Petropavlovskikh, I., Revell, L. E., Rhodes, L. E., Robinson, S. A., Robson, T. M., Rose, K. C., Schikowski, T., Solomon, K. R., Sulzberger, B., Wallington, T. J., Wang, Q.-W., Wängberg, S.-Å., White, C. C. Wilson, S. R., and Zhu, L.: Continuing benefits of the Montreal Protocol and protection of the stratospheric ozone layer for human health and the environment, Photochem. Photobio. S., https://doi.org/10.1007/s43630-024-00577-8, 2024.
Marais, E. A., Jacob, D. J., Choi, S., Joiner, J., Belmonte-Rivas, M., Cohen, R. C., Beirle, S., Murray, L. T., Schiferl, L. D., Shah, V., and Jaeglé, L.: Nitrogen oxides in the global upper troposphere: interpreting cloud-sliced NO2 observations from the OMI satellite instrument, Atmos. Chem. Phys., 18, 17017–17027, https://doi.org/10.5194/acp-18-17017-2018, 2018.
Marais, E. A., Roberts, J. F., Ryan, R. G., Eskes, H., Boersma, K. F., Choi, S., Joiner, J., Abuhassan, N., Redondas, A., Grutter, M., Cede, A., Gomez, L., and Navarro-Comas, M.: New observations of NO2 in the upper troposphere from TROPOMI, Atmos. Meas. Tech., 14, 2389–2408, https://doi.org/10.5194/amt-14-2389-2021, 2021.
Martin, R. V., Jacob, D. J. Yantosca, R. M., Chin, M., and Ginoux, P.: Global and regional decreases in tropospheric oxidants from photochemical effects of aerosols, J. Geophys. Res., 108, 4097, https://doi.org/10.1029/2002JD002622, 2003.
Mettig, N., Weber, M., Rozanov, A., Burrows, J. P., Veefkind, P., Thompson, A. M., Stauffer, R. M., Leblanc, T., Ancellet, G., Newchurch, M. J., Kuang, S., Kivi, R., Tully, M. B., Van Malderen, R., Piters, A., Kois, B., Stübi, R., and Skrivankova, P.: Combined UV and IR ozone profile retrieval from TROPOMI and CrIS measurements, Atmos. Meas. Tech., 15, 2955–2978, https://doi.org/10.5194/amt-15-2955-2022, 2022.
Miller, D. O. and Brune, W. H.: Investigating the understanding of oxidation chemistry using 20 years of airborne OH and HO2 observations, J. Geophys. Res.-Atmos., 127, e2021JD035368, https://doi.org/10.1029/2021JD035368, 2022.
Millet, D. B., Palmer, P. I., Levelt, P. F., Gallardo, L., and Shikwambana, L.: Coordinated geostationary, multispectral satellite observations are critical for climate and air quality progress, AGU Advances, 5, e2024AV001322, https://doi.org/10.1029/2024AV001322, 2024.
Minschwaner, K., Canty, T., and Burnett, C. R.: Hydroxyl column abundance measurements: PEPSIOS instrumentation at the Fritz Peak Observatory and data analysis techniques, J. Atmos. Sol.-Terr. Phy., 65, 335–334, https://doi.org/10.1016/S1364-6826(02)00297-3, 2003.
Miyazaki, K., Eskes, H. J., Sudo, K., Takigawa, M., van Weele, M., and Boersma, K. F.: Simultaneous assimilation of satellite NO2, O3, CO, and HNO3 data for the analysis of tropospheric chemical composition and emissions, Atmos. Chem. Phys., 12, 9545–9579, https://doi.org/10.5194/acp-12-9545-2012, 2012.
Miyazaki, K., Bowman, K. W., Yumimoto, K., Walker, T., and Sudo, K.: Evaluation of a multi-model, multi-constituent assimilation framework for tropospheric chemical reanalysis, Atmos. Chem. Phys., 20, 931–967, https://doi.org/10.5194/acp-20-931-2020, 2020.
Mok, J., Krotkov, N. A., Arola, A., Torres, O., Jethva, H., Andrade, M., Labow, G., Eck, T., Li, Z., Dickerson, R., Stenchikov, G. L., Osipov, S., and Ren, X.: Impacts of atmospheric brown carbon on surface UV and ozone in the Amazon Basin, Sci. Rep., 6, 36940, https://doi.org/10.1038/srep36940, 2016.
Murray, L. T., Fiore, A. M., Shindell, D. T., Naik, V., and Horowitz, L. W.: Large uncertainties in global hydroxyl projections tied to fate of reactive nitrogen and carbon, P. Natl. Acad. Sci. USA., 118, e2115204118, https://doi.org/10.1073/pnas.2115204118, 2021.
NASA/LARC/SD/ASDC: TEMPO formaldehyde total column V03 (BETA), Atmospheric Science Data Center [data set], https://doi.org/10.5067/IS-40e/TEMPO/HCHO_L2.003, 2024a.
NASA/LARC/SD/ASDC: TEMPO solar irradiance V03 (BETA), Atmospheric Science Data Center [data set], https://doi.org/10.5067/IS-40e/TEMPO/IRR_L1.003, 2024b.
NASA Goddard Space Flight Center: MERRA2 GMI, NASA [data set], https://acd-ext.gsfc.nasa.gov/Projects/GEOSCCM/MERRA2GMI/, last access: 10 July 2024.
Nicely, J. M., Anderson, D. C., Canty, T. P., Salawitch, R. J., Wolfe, G. M., Apel, E. C., Arnold, S. R., Atlas, E. L., Blake, N. J., Bresch, J. F., Campos, T. L., Dickerson, R. R., Duncan, B., Emmons, L. K., Evans, M. J., Fernandez, R. P., Flemming, J., Hall, S. R., Hanisco, T. F., Honomichl, S. B., Hornbrook, R. S., Huijnen, V., Kaser, L., Kinnison, D.E., Lamarque, J., Mao, J., Monks, S. A., Montzka, D. D., Pan, L. L., Riemer, D. D., Saiz-Lopez, A., Steenrod, S. D., Stell, M. H., Tilmes, S., Turquety, S., Ullmann, K., and Weinheimer, A. J.: An observationally constrained evaluation of the oxidative capacity in the tropical western Pacific troposphere, J. Geophys. Res. Atmos., 121, 7461–7488, https://doi.org/10.1002/2016JD025067, 2016.
Nicely, J. M., Salawitch, R. J., Canty, T., Manyin, M., Oman, L. D., Salawitch, R. J., Steenrod, S. D., Strahan, S. E., and Strode, S. A.: Quantifying the Causes of Differences in Tropospheric OH within Global Models, J. Geophys. Res.-Atmos., 122, 1983–2007, https://doi.org/10.1002/2016jd026239, 2017.
Nicely, J. M., Canty, T. P., Manyin, M., Oman, L. D., Salawitch, R. J., Steenrod, S. D., Strahan, S. E., and Strode, S. A.: Changes in global tropospheric OH expected as a result of climate change over the last several decades, J. Geophys. Res.-Atmos., 123, 10,774–10,795, https://doi.org/10.1029/2018JD028388, 2018.
Nicely, J. M., Duncan, B. N., Hanisco, T. F., Wolfe, G. M., Salawitch, R. J., Deushi, M., Haslerud, A. S., Jöckel, P., Josse, B., Kinnison, D. E., Klekociuk, A., Manyin, M. E., Marécal, V., Morgenstern, O., Murray, L. T., Myhre, G., Oman, L. D., Pitari, G., Pozzer, A., Quaglia, I., Revell, L. E., Rozanov, E., Stenke, A., Stone, K., Strahan, S., Tilmes, S., Tost, H., Westervelt, D. M., and Zeng, G.: A machine learning examination of hydroxyl radical differences among model simulations for CCMI-1, Atmos. Chem. Phys., 20, 1341–1361, https://doi.org/10.5194/acp-20-1341-2020, 2020.
Nguyen, N. H., Turner, A. J., Yin, Y., Prather, M. J., and Frankenberg, C.: Effects of chemical feedbacks on decadal methane emissions estimates, Geophys. Res. Lett., 47, e2019GL085706, https://doi.org/10.1029/2019GL085706, 2020.
Okamoto, K., Owada, H., Fujita, T., Kazumore, M., Otsuka, M., Seko, H., Ota, Y., Uekiyo, N., Ishimoto, H., Hayashi, M., Ishida, H., Ando, A., Takahashi, M., Bessho, K., and Yokota, H.: Assessment of the potential impact of a hyper-spectral infrared sounder on the Himawari follow-on geostationary satellite, SOLA, 16, 162–168, https://doi.org/10.2151/sola.2020-028, 2020.
Oman, L. D., Ziemke, J. R. Douglass, A. R., Waugh, D. W., Lang, C. Rodriguez, J. M., and Nielsen, J. E.: The response of tropical tropospheric ozone to ENSO, Geophys. Res. Lett., 38, L13706, https://doi.org/10.1029/2011GL047865, 2011.
Oman, L. D., Douglass, A. R., Ziemke, J. R., Rodriguez, J. M., Waugh, D. W., and Nielsen, J. E.: The ozone response to ENSO in Aura satellite measurements and a chemistry-climate simulation, J. Geophys. Res., 118, 965–976, https://doi.org/10.1029/2012JD018546, 2013.
Orfanoz-Cheuquelaf, A., Arosio, C., Rozanov, A., Weber, M., Ladstätter-Weißenmayer, A., Burrows, J. P., Thompson, A. M., Stauffer, R. M., and Kollonige, D. E.: Tropospheric ozone column dataset from OMPS-LP/OMPS-NM limb–nadir matching, Atmos. Meas. Tech., 17, 1791–1809, https://doi.org/10.5194/amt-17-1791-2024, 2024.
Pan, L., Geng, J., Hanisco, T. F., and Jiang, S.: Single frequency tunable UV laser at 308 nm based on all-fiberized master oscillator power amplifiers, Opt. Lett., 47, 5845–5848, https://doi.org/10.1364/OL.472559, 2022.
Patra, P. K., Krol, M. C., Prinn, R. G., Takigawa, M., Mühle, J., Montzka, S. A., Lal, S., Yamashita, Y., Naus, S., Chandra, N., Weiss, R. F., Krummel, P. B., Fraser, P. J., O'Doherty, S., and Elkins, J. W.: Methyl chloroform continues to constrain the hydroxyl (OH) variability in the troposphere, J. Geophys. Res.-Atmos., 126, e2020JD033862, https://doi.org/10.1029/2020JD033862, 2021.
Pimlott, M. A., Pope, R. J., Kerridge, B. J., Latter, B. G., Knappett, D. S., Heard, D. E., Ventress, L. J., Siddans, R., Feng, W., and Chipperfield, M. P.: Investigating the global OH radical distribution using steady-state approximations and satellite data, Atmos. Chem. Phys., 22, 10467–10488, https://doi.org/10.5194/acp-22-10467-2022, 2022.
Prather, M. J. and Zhu, L.: Resetting tropospheric OH and CH4 lifetime with ultraviolet H2O absorption, Science, 385, 201–204, https://doi.org/10.1126/science.adn0415, 2024.
Rohrer, F. and Berresheim, H.: Strong correlation between levels of tropospheric hydroxyl radicals and solar ultraviolet radiation, Nature, 442, 184–187, https://doi.org/10.1038/nature04924, 2006.
Saunois, M., Stavert, A. R., Poulter, B., Bousquet, P., Canadell, J. G., Jackson, R. B., Raymond, P. A., Dlugokencky, E. J., Houweling, S., Patra, P. K., Ciais, P., Arora, V. K., Bastviken, D., Bergamaschi, P., Blake, D. R., Brailsford, G., Bruhwiler, L., Carlson, K. M., Carrol, M., Castaldi, S., Chandra, N., Crevoisier, C., Crill, P. M., Covey, K., Curry, C. L., Etiope, G., Frankenberg, C., Gedney, N., Hegglin, M. I., Höglund-Isaksson, L., Hugelius, G., Ishizawa, M., Ito, A., Janssens-Maenhout, G., Jensen, K. M., Joos, F., Kleinen, T., Krummel, P. B., Langenfelds, R. L., Laruelle, G. G., Liu, L., Machida, T., Maksyutov, S., McDonald, K. C., McNorton, J., Miller, P. A., Melton, J. R., Morino, I., Müller, J., Murguia-Flores, F., Naik, V., Niwa, Y., Noce, S., O'Doherty, S., Parker, R. J., Peng, C., Peng, S., Peters, G. P., Prigent, C., Prinn, R., Ramonet, M., Regnier, P., Riley, W. J., Rosentreter, J. A., Segers, A., Simpson, I. J., Shi, H., Smith, S. J., Steele, L. P., Thornton, B. F., Tian, H., Tohjima, Y., Tubiello, F. N., Tsuruta, A., Viovy, N., Voulgarakis, A., Weber, T. S., van Weele, M., van der Werf, G. R., Weiss, R. F., Worthy, D., Wunch, D., Yin, Y., Yoshida, Y., Zhang, W., Zhang, Z., Zhao, Y., Zheng, B., Zhu, Q., Zhu, Q., and Zhuang, Q.: The Global Methane Budget 2000–2017, Earth Syst. Sci. Data, 12, 1561–1623, https://doi.org/10.5194/essd-12-1561-2020, 2020.
Shutter, J. D., Millet, D. B., Wells, K. C., Payne, V. H., Nowlan, C. R., and Gonzalez Abad, G.: Interannual changes in atmospheric oxidation over forests determined from space, Science Advances, 10, 20, https://doi.org/10.1126/sciadv.adn1115, 2024.
Souri, A. H., Duncan, B. N., Strode, S. A., Anderson, D. C., Manyin, M. E., Liu, J., Oman, L. D., Zhang, Z., and Weir, B.: Enhancing long-term trend simulation of the global tropospheric hydroxyl (TOH) and its drivers from 2005 to 2019: a synergistic integration of model simulations and satellite observations, Atmos. Chem. Phys., 24, 8677–8701, https://doi.org/10.5194/acp-24-8677-2024, 2024.
Spivakovsky, C. M., Logan, J. A., Montzka, S. A., Balkanski, Y. J., Foreman-Fowler, M., Jones, D. B. A., Horowitz, L. W., Fusco, A. C., Brenninkmeijer, C. A. M., Prather, M. J., Wofsy, S. C., and McElroy, M. B.: Three-dimensional climatological distribution of tropospheric OH: Update and evaluation, J. Geophys. Res., 105, 8931–8980, https://doi.org/10.1029/1999JD901006, 2000.
Stolarski, R. S., Krueger, A. J., Schoeberl, M. R., McPeters, R. D., Newman, P. A., and Alpert, J. C.: Nimbus 7 satellite measurements of the springtime Antarctic ozone decrease, Nature, 322, 808–811, https://doi.org/10.1038/322808a0, 1986.
Stone, D., Whalley, L. K., and Heard, D. E.: Tropospheric OH and HO2 radicals: field measurements and model comparisons, Chem. Soc. Rev., 41, 6348–6404, https://doi.org/10.1039/C2CS35140D, 2012.
Theys, N., Volkamer, R., Müller, J.-F., Zarzana, K. J., Kille, N., Clarisse, L., De Smedt, I., Lerot, C., Finkenzeller, H., Hendrick, F., Koenig, T. K., Lee, C. F., Knote, C., Yu, H., and Van Roozendael, M.: Global nitrous acid emissions and levels of regional oxidants enhanced by wildfires, Nat. Geosci., 13, 681–686, https://doi.org/10.1038/s41561-020-0637-7, 2020.
Thompson, R. L., Montzka, S. A., Vollmer, M. K., Arduini, J., Crotwell, M., Krummel, P. B., Lunder, C., Mühle, J., O'Doherty, S., Prinn, R. G., Reimann, S., Vimont, I., Wang, H., Weiss, R. F., and Young, D.: Estimation of the atmospheric hydroxyl radical oxidative capacity using multiple hydrofluorocarbons (HFCs), Atmos. Chem. Phys., 24, 1415–1427, https://doi.org/10.5194/acp-24-1415-2024, 2024.
Travis, K. R., Heald, C. L., Allen, H. M., Apel, E. C., Arnold, S. R., Blake, D. R., Brune, W. H., Chen, X., Commane, R., Crounse, J. D., Daube, B. C., Diskin, G. S., Elkins, J. W., Evans, M. J., Hall, S. R., Hintsa, E. J., Hornbrook, R. S., Kasibhatla, P. S., Kim, M. J., Luo, G., McKain, K., Millet, D. B., Moore, F. L., Peischl, J., Ryerson, T. B., Sherwen, T., Thames, A. B., Ullmann, K., Wang, X., Wennberg, P. O., Wolfe, G. M., and Yu, F.: Constraining remote oxidation capacity with ATom observations, Atmos. Chem. Phys., 20, 7753–7781, https://doi.org/10.5194/acp-20-7753-2020, 2020.
Turner, A. J., Frankenberg, C., Wennberg, P. O., and Jacob, D. J.: Ambiguity in the causes for decadal trends in atmospheric methane and hydroxyl, P. Natl. Acad. Sci. USA, 114, 5367–5372, https://doi.org/10.1073/pnas.1616020114, 2016.
Turner, A. J., Fung, I., Naik, V., Horowitz, L. W., and Cohen, R. C.: Modulation of hydroxyl variability by ENSO in the absence of external forcing, Nature, 115, 8931–8936, https://doi.org/10.1073/pnas.1807532115, 2018.
Valin, L. C., Russell, A. R., and Cohen, R. C.: Variations of OH radical in an urban plume inferred from NO2 column measurements, Geophys. Res. Lett., 40, 1856–1860, https://doi.org/10.1002/grl.50267, 2013.
Valin, L. C., Fiore, A. M., Chance, K., and González Abad, G.: The role of OH production in interpreting the variability of CH2O columns in the southeast U.S., J. Geophys. Res.-Atmos., 121, 478–493, https://doi.org/10.1002/2015JD024012, 2016.
van Wees, D., van der Werf, G. R., Randerson, J. T., Rogers, B. M., Chen, Y., Veraverbeke, S., Giglio, L., and Morton, D. C.: Global biomass burning fuel consumption and emissions at 500 m spatial resolution based on the Global Fire Emissions Database (GFED), Geosci. Model Dev., 15, 8411–8437, https://doi.org/10.5194/gmd-15-8411-2022, 2022.
Vasilkov, A., Krotkov, N., Yang, E.-S., Lamsal, L., Joiner, J., Castellanos, P., Fasnacht, Z., and Spurr, R.: Explicit and consistent aerosol correction for visible wavelength satellite cloud and nitrogen dioxide retrievals based on optical properties from a global aerosol analysis, Atmos. Meas. Tech., 14, 2857–2871, https://doi.org/10.5194/amt-14-2857-2021, 2021.
Vasilkov, A., Krotkov, N., Haffner, D., Fasnacht, Z., and Joiner, J.: Estimates of Hyperspectral Surface and Underwater UV Planar and Scalar Irradiances from OMI Measurements and Radiative Transfer Computations, Remote Sens., 14, 2278, https://doi.org/10.3390/rs14092278, 2022.
von Clarmann, T., Degenstein, D. A., Livesey, N. J., Bender, S., Braverman, A., Butz, A., Compernolle, S., Damadeo, R., Dueck, S., Eriksson, P., Funke, B., Johnson, M. C., Kasai, Y., Keppens, A., Kleinert, A., Kramarova, N. A., Laeng, A., Langerock, B., Payne, V. H., Rozanov, A., Sato, T. O., Schneider, M., Sheese, P., Sofieva, V., Stiller, G. P., von Savigny, C., and Zawada, D.: Overview: Estimating and reporting uncertainties in remotely sensed atmospheric composition and temperature, Atmos. Meas. Tech., 13, 4393–4436, https://doi.org/10.5194/amt-13-4393-2020, 2020.
Walker, J. C., Dudhia, A., and Carboni, E.: An effective method for the detection of trace species demonstrated using the MetOp Infrared Atmospheric Sounding Interferometer, Atmos. Meas. Tech., 4, 1567–1580, https://doi.org/10.5194/amt-4-1567-2011, 2011.
Wang, S., Pickett, H. M., Pongetti, T. J., Cheung, R., Yung, Y. L., C. Shim, Li, Q., Canty, T., Salawitch, R. J., Jucks, K. W., Drouin, B., and Sander, S. P.: Validation of Aura Microwave Limb Sounder OH measurements with Fourier Transform Ultra-Violet Spectrometer total OH column measurements at Table Mountain, California, J. Geophys. Res., 113, D22301, https://doi.org/10.1029/2008JD009883, 2008.
Weber, M., Coldewey-Egbers, M., Fioletov, V. E., Frith, S. M., Wild, J. D., Burrows, J. P., Long, C. S., and Loyola, D.: Total ozone trends from 1979 to 2016 derived from five merged observational datasets – the emergence into ozone recovery, Atmos. Chem. Phys., 18, 2097–2117, https://doi.org/10.5194/acp-18-2097-2018, 2018.
Wells, K.C., Millet, D. B., Payne, V. H., Deventer, M. J., Bates, K. H., de Gouw, J. A., Graus, M., Warneke, C., Wisthaler, A., and Fuentes, J. D.: Satellite isoprene retrievals constrain emissions and atmospheric oxidation, Nature, 585, 225–233, https://doi.org/10.1038/s41586-020-2664-3, 2020.
Wells, K., Millet, D., Brewer, J., Payne, V., Cady-Pereira, K., Pernak, R., Kulawik, S., Vigouroux, C., Jones, N., Mahieu, E., Makarova, M., Nagahama, T., Ortega, I., Palm, M., Strong, K., Schneider, M., Smale, D., Sussmann, R., and Zhou, M.: Long-term global measurements of methanol, ethene, ethyne, and HCN from the Cross-track Infrared Sounder, EGUsphere [preprint], https://doi.org/10.5194/egusphere-2024-1551, 2024.
Wofsy, S. C., Afshar, S., Allen, H. M., Apel, E. C., Asher, E. C., Barletta, B., Bent, J., Bian, H., Biggs, B. C., Blake, D. R., Blake, N., Bourgeois, I., Brock, C. A., Brune,W. H., Budney, J. W., Bui, T. P., Butler, A., Campuzano-Jost, P., Chang, C. S., Chin, M., Commane, R., Correa, G., Crounse, J. D., Cullis, P. D., Daube, B. C., Day, D. A., Dean-Day, J. M., Dibb, J. E., DiGangi, J. P., Diskin, G. S., Dollner, M., Elkins, J. W., Erdesz, F., Fiore, A. M., Flynn, C. M., Froyd, K. D., Gesler, D. W., Hall, S. R., Hanisco, T. F., Hannun, R. A., Hills, A. J., Hintsa, E. J., Hoffman, A., Hornbrook, R. S., Huey, L. G., Hughes, S., Jimenez, J. L., Johnson, B. J., Katich, J. M., Keeling, R. F., Kim, M. J., Kupc, A., Lait, L. R., McKain, K., McLaughlin, R. J., Meinardi, S., Miller, D. O., Montzka, S. A., Moore, F. L., Morgan, E. J., Murphy, D. M., Murray, L. T., Nault, B. A., Neuman, J. A., Newman, P. A., Nicely, J. M., Pan, X., Paplawsky, W., Peischl, J., Prather, M. J., Price, D. J., Ray, E. A., Reeves, J. M., Richardson, M., Rollins, A. W., Rosenlof, K. H., Ryerson, T. B., Scheuer, E., Schill, G. P., Schroder, J. C., Schwarz, J. P., St.Clair, J. M., Steenrod, S. D., Stephens, B. B., Strode, S. A., Sweeney, C., Tanner, D., Teng, A. P., Thames, A. B., Thompson, C. R., Ullmann, K., Veres, P. R., Wagner, N. L., Watt, A., Weber, R., Weinzierl, B. B., Wennberg, P. O., Williamson, C. J., Wilson, J. C., Wolfe, G. M., Woods, C. T., Zeng, L. H., and Vieznor, N.: ATom: Merged Atmospheric Chemistry, Trace Gases, and Aerosols, Version 2, ORNL Distributed Active Archive Center [data set], https://doi.org/10.3334/ORNLDAAC/1925, 2021.
Wolfe, G. M., Nicely, J. M., St. Clair, J. M., Hanisco, T. F., Liao, J., Oman, L. D., Brune, W. B., Miller, D., Thames, A., González Abad, G., Ryerson, T. B., Thompson, C. R., Peischl, J., McKain, K., Sweeney, C., Wennberg, P. O., Kim, M., Crounse, J. D., Hall, S. R., Ullmann, K., Diskin, G., Bui, P., Chang, C., and Dean-Day, J.: Mapping hydroxyl variability throughout the global remote troposphere via synthesis of airborne and satellite formaldehyde observations, P. Natl. Acad. Sci. USA, 116, 11171–11180, https://doi.org/10.1073/pnas.1821661116, 2019.
Worden, H. M., Deeter, M. N., Edwards, D. P., Gille, J. C., Drummond, J. R., and Nédélec, P.: Observations of near-surface carbon monoxide from space using MOPITT multispectral retrievals, J. Geophys. Res., 115, D18314, https://doi.org/10.1029/2010JD014242, 2010.
Worden, J. R., Cusworth, D. H., Qu, Z., Yin, Y., Zhang, Y., Bloom, A. A., Ma, S., Byrne, B. K., Scarpelli, T., Maasakkers, J. D., Crisp, D., Duren, R., and Jacob, D. J.: The 2019 methane budget and uncertainties at 1° resolution and each country through Bayesian integration Of GOSAT total column methane data and a priori inventory estimates, Atmos. Chem. Phys., 22, 6811–6841, https://doi.org/10.5194/acp-22-6811-2022, 2022.
Wu, D., Zhang, J., Wang, M., An, J., Wang, R., Haider, H., Ri, Z., Huang, Y., Zhang, Q., Zhou, F., Tian, H., Zhang, X., Deng, L., Pan, Y., Chen, X., Yu, Y., Hu, C., Wang, R., Song, Y., Gao, Z., Wang, Y., Hou, L., and Liu, M.: Global and regional patterns of soil nitrous acid emissions and their acceleration of rural photochemical reactions, J. Geophys. Res.-Atmos., 127, e2021JD036379, https://doi.org/10.1029/2021JD036379, 2022.
Yang, J., Zhang, Z., Wei, C., Lu, F., and Guo, Q.: Introducing the new generation of Chinese geostationary weather satellites, Fengyun-4, B. Am. Meteorol. Soc., 98, 1637–1658, https://doi.org/10.1175/BAMS-D-16-0065.1, 2017.
Yang, X., Lu, K., Ma, X., Gao, Y., Tan, Z., Wang, H., Chen, X., Li, X., Huang, X., He, L., Tang, M., Zhu, B., Chen, S., Dong, H., Zeng, L., and Zhang, Y.: Radical chemistry in the Pearl River Delta: observations and modeling of OH and HO2 radicals in Shenzhen in 2018, Atmos. Chem. Phys., 22, 12525–12542, https://doi.org/10.5194/acp-22-12525-2022, 2022.
Yang, X., Wang, H., Lu, K., Ma, X., Tan, Z., Long, B., Chen, X., Li, C., Zhai, T., Li, Y., Qu, K., Xia, Y., Zhang, Y., Li, X., Chen, S., Dong, H., Zeng, L., and Zhang, Y.: Reactive aldehyde chemistry explains the missing source of hydroxyl radicals, Nat. Commun., 15, 1648, https://doi.org/10.1038/s41467-024-45885-w, 2024.
Yang, Y., Shao, M., Wang, X., Nölscher, A. C., Kessel, S., Guenther, A., and Williams, J.: Towards a quantitative understanding of total OH reactivity: A review, Atmos. Environ., 134, 147–161, https://doi.org/10.1016/j.atmosenv.2016.03.010, 2016.
Zhang, Y., Jacob, D. J., Maasakkers, J. D., Sulprizio, M. P., Sheng, J.-X., Gautam, R., and Worden, J.: Monitoring global tropospheric OH concentrations using satellite observations of atmospheric methane, Atmos. Chem. Phys., 18, 15959–15973, https://doi.org/10.5194/acp-18-15959-2018, 2018.
Zhang, Y., Jacob, D. J., Lu, X., Maasakkers, J. D., Scarpelli, T. R., Sheng, J.-X., Shen, L., Qu, Z., Sulprizio, M. P., Chang, J., Bloom, A. A., Ma, S., Worden, J., Parker, R. J., and Boesch, H.: Attribution of the accelerating increase in atmospheric methane during 2010–2018 by inverse analysis of GOSAT observations, Atmos. Chem. Phys., 21, 3643–3666, https://doi.org/10.5194/acp-21-3643-2021, 2021.
Zhang, Q., Liu, P., Wang, Y., George, C., Chen, T., Ma, S., Ren, Y., Mu, Y., Song, M., Herrmann, H., Mellouki, A., Chen, J., Yue, Y., Zhao, X., Wang, S., and Zeng, Y.: Unveiling the underestimated direct emissions of nitrous acid (HONO), P. Natl. Acad. Sci. USA, 120, e2302048120, https://doi.org/10.1073/pnas.2302048120, 2023.
Zhao, Y., Saunois, M., Bousquet, P., Lin, X., Berchet, A., Hegglin, M. I., Canadell, J. G., Jackson, R. B., Hauglustaine, D. A., Szopa, S., Stavert, A. R., Abraham, N. L., Archibald, A. T., Bekki, S., Deushi, M., Jöckel, P., Josse, B., Kinnison, D., Kirner, O., Marécal, V., O'Connor, F. M., Plummer, D. A., Revell, L. E., Rozanov, E., Stenke, A., Strode, S., Tilmes, S., Dlugokencky, E. J., and Zheng, B.: Inter-model comparison of global hydroxyl radical (OH) distributions and their impact on atmospheric methane over the 2000–2016 period, Atmos. Chem. Phys., 19, 13701–13723, https://doi.org/10.5194/acp-19-13701-2019, 2019.
Zhao, Y., Saunois, M., Bousquet, P., Lin, X., Hegglin, M. I., Canadell, J. G., Jackson, R. B., and Zheng, B.: Reconciling the bottom-up and top-down estimates of the methane chemical sink using multiple observations, Atmos. Chem. Phys., 23, 789–807, https://doi.org/10.5194/acp-23-789-2023, 2023.
Zhu, L., González Abad, G., Nowlan, C. R., Chan Miller, C., Chance, K., Apel, E. C., DiGangi, J. P., Fried, A., Hanisco, T. F., Hornbrook, R. S., Hu, L., Kaiser, J., Keutsch, F. N., Permar, W., St. Clair, J. M., and Wolfe, G. M.: Validation of satellite formaldehyde (HCHO) retrievals using observations from 12 aircraft campaigns, Atmos. Chem. Phys., 20, 12329–12345, https://doi.org/10.5194/acp-20-12329-2020, 2020.
Zhu, Q., Laughner, J. L., and Cohen, R. C.: Estimate of OH trends over one decade in North American cities, P. Natl. Acad. Sci. USA, 119, e2117399119, https://doi.org/10.1073/pnas.2117399119, 2022a.
Zhu, Q., Laughner, J. L., and Cohen, R. C.: Combining Machine Learning and Satellite Observations to Predict Spatial and Temporal Variation of near Surface OH in North American Cities, Environ. Sci. Technol., 56, 11, https://doi.org/10.1021/acs.est.1c05636, 2022b.
Zhu, Q., Fiore, A. M., Correa, G., Lamarque, J. F., and Worden, H.: The impact of internal climate variability on OH trends between 2005 and 2014, Environ. Res. Lett., 19, 064032, https://doi.org/10.1088/1748-9326/ad4b47, 2024.
Ziemke, J. R., Chandra, S., Duncan, B.N. Froidevaux, L., Bhartia, P. K., Levelt, P. F., and Waters, J. W.: Tropospheric ozone determined from Aura OMI and MLS: Evaluation of measurements and comparison with the Global Modeling Initiative's Chemical Transport Model, J. Geophys. Res., 111, D19303, https://doi.org/10.1029/2006JD007089, 2006.
Zoogman, P., Liu, X., Suleiman, R. M., Pennington, W. F., Flittner, D. E., Al-Saadi, J., Hilton, B. B., Nicks, D. K., Newchurch, M., Carr, J. L., Janz, S., Andraschko, M., Arola, A., Baker, B. D., Canova, B. P., Miller, C. C., Cohen, R. C., Davis, J. E., Dussault, M. E., Edwards, D., Fishman, J., Ghulam, A., Abad, G. G., Grutter, M., Herman, J. R., Houck, J., Jacob, D. J., Joiner, J., Kerridge, B. J., Kim, J., Krotkov, N., Lamsal, L. N., Li, C., Lindfors, A., Martin, R. V., McElroy, C. T., McLinden, C., Natraj, V., Neil, D. O., Nowlan, C. R., O'Sullivan, E. J., Palmer, P. I., Pierce, R. B., Pippin, M. R., Saiz-Lopez, A., Spurr, R. J. D., Szykman, J., Torres, O., Veefkind, J. P., Veihelmann, B., Wang, H., Wang, J., and Chance, K.: Tropospheric emissions: Monitoring of pollution (TEMPO), J. Quant. Spectrosc. Ra., 186, 17–39, https://doi.org/10.1016/j.jqsrt.2016.05.008, 2017.
- Abstract
- Introduction
- Current approaches to indirectly constrain local and regional concentrations of and trends and variations in tropospheric OH with satellite observations
- Recommendations for suborbital needs
- Recommendations for retrieval algorithm refinement
- Recommendations for satellite needs
- Summary of recommendations
- Data availability
- Author contributions
- Competing interests
- Disclaimer
- Acknowledgements
- Review statement
- References
- Abstract
- Introduction
- Current approaches to indirectly constrain local and regional concentrations of and trends and variations in tropospheric OH with satellite observations
- Recommendations for suborbital needs
- Recommendations for retrieval algorithm refinement
- Recommendations for satellite needs
- Summary of recommendations
- Data availability
- Author contributions
- Competing interests
- Disclaimer
- Acknowledgements
- Review statement
- References