the Creative Commons Attribution 4.0 License.
the Creative Commons Attribution 4.0 License.
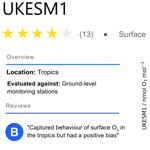
Performance evaluation of UKESM1 for surface ozone across the pan-tropics
Flossie Brown
Gerd Folberth
Stephen Sitch
Paulo Artaxo
Marijn Bauters
Pascal Boeckx
Alexander W. Cheesman
Matteo Detto
Ninong Komala
Luciana Rizzo
Nestor Rojas
Ines dos Santos Vieira
Steven Turnock
Hans Verbeeck
Alfonso Zambrano
Surface ozone monitoring sites in the tropics are limited, despite the risk that surface ozone poses to human health, tropical forest and crop productivity. Atmospheric chemistry models allow us to assess ozone exposure in unmonitored locations and evaluate the potential influence of changing policies and climate on air quality, human health and ecosystem integrity. Here, we utilise in situ ozone measurements from ground-based stations in the pan-tropics to evaluate ozone from the UK Earth system model, UKESM1, with a focus on remote sites. The study includes ozone data from areas with limited previous data, notably tropical South America, central Africa and tropical northern Australia. Evaluating UKESM1 against observations beginning in 1987 onwards, we show that UKESM1 is able to capture changes in surface ozone concentration at different temporal resolutions, albeit with a systematic high bias of 18.1 nmol mol−1 on average. We use the diurnal ozone range (DOR) as a metric for evaluation and find that UKESM1 captures the observed DOR (mean bias of 2.7 nmol mol−1 and RMSE of 7.1 nmol mol−1) and the trend in DOR with location and season. Results from this study reveal that hourly ozone concentrations from UKESM1 require bias correction before use for impact assessments based on human and ecosystem health. Indeed, hourly surface ozone data have been crucial to this study, and we encourage other modelling groups to include hourly surface ozone output as a default.
- Article
(2249 KB) - Full-text XML
-
Supplement
(3017 KB) - BibTeX
- EndNote
Surface level ozone is an air pollutant with detrimental effects on human and plant health (Ainsworth et al., 2012; Emberson, 2020), of which tropical forests are particularly important ecosystems that are vulnerable to climate change and anthropogenic disturbances (Artaxo et al., 2022; Andreae et al., 2015). Despite rising ozone precursor emissions across tropical cities (Sicard et al., 2023) and predicted damage to crop yields and tropical forest health (Kittipornkul et al., 2023; Hayes et al., 2020), measurements of surface ozone concentrations in tropical areas are sparse, and few pollution controls have been implemented. Models are therefore essential to provide information on ozone concentrations in areas with sparse observations and to understand the drivers of ozone formation. In addition, they are needed to evaluate intended and unintended impacts of pollution mitigation policies on air quality, human health and ecosystems and to produce assessments of future impacts. Comparison of model output to recent observations is essential to validating models and understanding biases or missing processes. Here, we focus on evaluating surface ozone concentrations from the UK Earth system model, UKESM1 (Archibald et al., 2020a; Mulcahy et al., 2020; Sellar et al., 2019; Williams et al., 2018), with emphasis on remote areas of the tropics.
Data from ground-level ozone monitoring stations are commonly used to evaluate modelled surface ozone, as they provide data at a high temporal frequency whilst remaining in a fixed location (Sofen et al., 2016a). This is compared to aircraft campaigns and ozonesondes, which are infrequent in time and sparse in space (Chang et al., 2020; Gaudel et al., 2024), and satellite products, which do not sample the lower troposphere well (Vieira et al., 2023). Although there are many ground-level stations in Europe, North America and east Asia, allowing for detailed analysis (Chang et al., 2017; Akimoto et al., 2015; Hickman et al., 2023), there has, until recently, only been a limited number of stations in South America and central Africa, leaving the tropical forests almost entirely unobserved (Sofen et al., 2016b). This presents challenges to performing impact analysis in these locations, for example in examining the extent that El Niño–Southern Oscillation (ENSO) signals play in driving surface ozone concentrations (Sofen et al., 2016b) and in understanding ozone effects on tropical forest health (Sitch et al., 2007). Furthermore, recent studies of ozone in the tropics find increasing concentrations in several South American cities (Seguel et al., 2024) and southeast Asia (Gaudel et al., 2024) but highlight a lack of information in several locations due to sporadic or missing monitoring as a limitation. Since 2019, several more monitoring stations have been set up, increasing the number of monitored tropical locations compared to previous evaluations by Young et al. (2018) and Gaudel et al. (2018) to include the Congo basin (Sibret et al., 2022); Panama; and the wet tropics bioregion of northeast Queensland, Australia.
Tropospheric ozone is not emitted into the atmosphere directly. Ozone is instead formed in situ from reactions involving precursors of nitrogen oxides (NOx) and volatile organic compounds (VOCs). The ozone production rate is controlled by the reaction and can therefore be considered NOx-limited or VOC-limited depending on the availability of these species (Archibald et al., 2020b; Wild and Palmer, 2008). However, the effect of changing NOx and VOC concentrations on ozone concentrations is non-linear. For example, in a VOC-limited regime, reducing NOx concentrations will not decrease the rate of ozone production (and instead would likely increase the rate). In some cases, ozone photochemistry can be suppressed by aerosols (Ivatt et al., 2022), creating an “aerosol-limited” regime. Whilst the remote tropics are mostly NOx-limited (Liu et al., 2022), cities can often be VOC-limited (e.g. Nakada and Urban, 2020; Dantas et al., 2020) and even include conditions under which “NOx titration” occurs, a process whereby large, local sources of nitric oxide (NO) react with and thereby remove ozone. Over Southeast Asia, an aerosol-inhibited regime may be the dominant process due to high levels of particle pollution (Ivatt et al., 2022). Tropical forests have high biogenic VOC emission rates, of which the most abundant is isoprene (Yáñez-Serrano et al., 2015), as well as more limited NOx sources, which include fires (Jaeglé et al., 2005; Pope et al., 2020), lightning (Bond et al., 2002; Verma et al., 2021) and soils (Weng et al., 2020). Many tropical locations show a strong seasonality in precursor emissions, with elevated NOx concentrations during months with high proximate biomass burning (van der A et al., 2008). The diversity of ozone regimes across the tropics and the mix of natural and anthropogenic sources of ozone precursors provide good study potential for a variety of model processes (Nascimento et al., 2022).
UKESM1 is considered to have a positive bias compared to ground-level observations in the tropics, overestimating the annual mean ozone concentrations by approximately a factor of 2 (Archibald et al., 2020a, Fig. 6). Health and impact studies therefore employ bias correction techniques to avoid overestimating risks (e.g. Turnock et al., 2023; Akriditis et al., 2024), so a thorough evaluation of biases is valuable for these assessments. A positive bias is not unique to UKESM1, with the latest evaluation of models contributing to the Coupled Model Intercomparison Project 6 (CMIP6), reporting a multi-model mean bias of 6 nmol mol−1 at the remote tropical site of Cape Matatula, American Samoa (14.2° S, 170.6° E) (Griffiths et al., 2021). This bias is partly attributed to the coarse spatial resolution of the models (Wild and Palmer, 2008), which is 1.875°×1.25° horizontally and 40 m vertically at the lowest model level in UKESM1. In reality, NOx emissions often occur as subgrid-scale plumes, and ozone concentrations decline sharply towards the surface, but the coarse resolution does not allow this to be resolved and tends to cause overestimation of ozone production (Jaffe and Wigder, 2012; Neal et al., 2017; Pfister et al., 2006), for example by reducing highly localised NOx titration. Some consequences of changing resolution are indirect; Wild and Prather (2006) showed that ozone deposition rates increased at higher resolution as ozone was redistributed to areas of lower boundary layer resistance. Further causes of bias may include missing processes, incorrect/incomplete parameterisations, or errors in simulation of small-scale transport and dynamics.
Although there are several model evaluations of how well Earth system models (ESMs) capture seasonality (Brown et al., 2022; Griffiths et al., 2021; Turnock et al., 2020; Young et al., 2018), there are limited studies on the ability of ESMs to replicate the diurnal cycle (Pacifico et al., 2015). Whilst seasonal cycles are important to determine average ozone concentrations, seasonal changes in ozone regime and trends over time, hourly or sub-daily resolution are key to assessing peak and duration exposure metrics for both human health and vegetation uptake (Lefohn et al., 2018). Given the pivotal role of sunlight in ozone formation and the short lifetime of ozone at the surface, ozone concentrations vary over the diurnal cycle, typically from lower values at night, to peak values in the early afternoon (Piikki et al., 2009). For plants, this diurnal cycle directly affects stomatal ozone uptake as leaf conductance also changes over the day (Felzer et al., 2007), with the highest stomatal conductance approximately coinciding with the highest daytime ozone concentrations. Thus, the ability of models to reproduce the observed diurnal cycle is critical to ecosystem impact assessments.
This study evaluates UKESM1 on its ability to reproduce hourly, daily, seasonal and annual cycles in ozone concentration across the tropics, with a focus on remote sites. By evaluating ozone concentrations from UKESM1 against different sites in the tropics over a range of time resolutions, we provide a starting point for further systematic evaluation of ozone-forming processes and their sensitivity in the tropics.
2.1 Station data
The monitoring station data used in this study comprise freely available data collected from the TOAR I database (Schröder, et al., 2021; Schultz et al., 2017). Further open-access data have been provided by the CongoFlux eddy-flux tower located in Yangambi, Democratic Republic of the Congo (Sibret et al., 2022); a canopy access crane at James Cook University's Daintree Rainforest Observatory, Australia (Liddell et al., 2007); and an eddy-flux tower station located on Barro Colorado Island, Panama (Detto and Pacala, 2022). Data from three urban stations in Darwin, Australia, are also publicly available from the Northern Territory Environment Protection Authority (NTEPA, 2023, http://ntepa.webhop.net/NTEPA/, last access: September 2023).
Monitoring stations (n=53, Table S1 in the Supplement) are aggregated into 13 distinct sites (Fig. 1) for model evaluation, of which 8 are remote. The sites are described in the Supplement. We use “station” to refer to an individual instrument dataset and “site” to refer to the collection of station data that are combined for comparison to UKESM1. Stations were discarded if a diurnal cycle was not available. The temporal range and completeness of the data within this range are shown in Fig. S1 in the Supplement. UKESM1 was evaluated at the grid cell level by comparing model output to the site, an average of all stations within the grid cell. Station networks that were geographically close but crossed adjacent grid cells were combined into one site and compared with the average of the corresponding grid cells: the urban air quality network in Bogotá spans three grid cells, São Paulo spans three grid cells and Jakarta spans two grid cells.
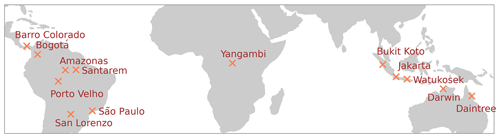
Figure 1Map showing locations of grid cells containing measurement sites (orange crosses) and the site names used in this paper.
Data have been cleaned to remove erroneously high and low values; the highest 20 hourly values from each station were checked and data points removed if there were sudden jumps between hours that were more than double the values for the preceding and succeeding hours. This was largely to remove extreme outliers because, for example, a random hourly measurement of 1000 nmol mol−1 where all other data are below 200 nmol mol−1 will affect the diurnal ozone range (DOR). Periods of 24 h or more with ozone values at 0–1 nmol mol−1 were also removed since this was an indication that the instrument was not working correctly. This occurred at the Daintree and Barro Colorado sites, which had known issues with their ozone monitors.
2.2 Model data
This study focuses on ozone concentrations produced by UKESM1 (UKESM1-0-LL). Hourly surface ozone concentrations were modelled by UKESM1 as part of the CMIP6 historical simulations (Tang et al., 2019), a core experiment of CMIP6, that covers the historical period from 1850 to 2014 including anthropogenic, solar and volcanic forcings (Eyring et al., 2016). One of the major purposes of the experiment was model evaluation.
UKESM1 is a combination of the physical climate model HadGEM-GC3.1 (Williams et al., 2018) with additional Earth system components including land and atmospheric chemistry (Sellar et al., 2019). The UK Chemistry and Aerosol scheme (UKCA) contains stratospheric and tropospheric chemistry (Archibald et al., 2020a), combined with the GLOMAP-mode aerosol microphysics scheme (Mulcahy et al., 2018, 2020). The lowest vertical model level of UKESM1 represents an altitude of 0–40 m with a layer midpoint height of 20 m above orography/ground, and the horizontal resolution is 1.25° latitude by 1.875° longitude (∼180 km in the tropics). UKCA includes 84 chemical species used to simulate chemical cycles of Ox, HOx and NOx, as well as oxidation reactions of CO, CH4 and NMVOCs (non-methane VOCs; isoprene, ethane, propane), described in detail by Archibald et al. (2020a). Monoterpenes are treated as a single lumped species that react with ozone, OH and NO3, resulting in formation of secondary organic aerosol.
Anthropogenic and biomass burning emissions, including the ozone precursors VOCs, NOx and CO, are prescribed at a monthly resolution (Hoesly et al., 2018; van Marle et al., 2017). Lightning NOx is calculated using the parameterisation of Price and Rind (1992), which calculates a lightning flash density based on cloud-top height and produces a global annual emission rate of 5.93 Tg N yr−1 over 2005 to 2014. Soil NO is prescribed as a spatially explicit model output according to Yienger and Levy (1995), scaled to give an annual flux of 12 Tg NO. CH4 is prescribed as an annual mean surface concentration based on observations over the historical period (Meinshausen et al., 2017). Emissions of isoprene and monoterpenes are generated by the interactive biogenic VOC (iBVOC) emission model (Pacifico et al., 2011), with annual mean emissions of 495.9 and 115.1 Tg C yr−1, respectively. Other biogenic emissions are prescribed as monthly mean climatologies based on the years 2001–2010 (Guenther et al., 2012; Sindelarova et al., 2014).
2.3 Analysis
This study looks in detail at remote sites (8 of 13) across the tropics (Table 1), with the rural site in Watukosek classed as remote for this study. Urban sites (5 of 13) are also included for annual mean calculations to identify differences between sites and to assess whether UKESM1 can capture spatial patterns. Where possible, years of data from UKESM1 are matched to the years of data measured at each site, and, where observations fall outside of the model time period, the years 2005–2014 are used (Table 1). As UKESM1 is free-running, i.e. it simulates its own weather and climate, the meteorology in each year does not necessarily reflect the conditions at the time but should reflect the variability and the average over the decade. Comparison to reanalysis shows UKESM1 overestimates annual mean surface temperature by an average of 0.7 K in the period 2005–2014 (Table S2 in the Supplement). Differences in observed climate at sites where the model and observation period are mismatched are given in Fig. S3 in the Supplement and show that the model period (2005–2014) differs from the observation period (2019–2022) by 0.5 K on average using reanalysis and that UKESM1 temperatures are closer to those observed in 2019–2022. Archibald et al. (2020b) show that the temperature sensitivity of ozone in the chemistry scheme of UKESM1 is on the order of 1 in the absence of feedbacks from the land surface, meaning climate trends are unlikely to cause a significant difference in ozone between the different periods. However, prescribed emissions are specific to the year, so where the modelled and observed periods do not match, there may be differences in ozone concentrations due to emissions changes. The major source of air pollution in the Darwin, Daintree and Yangambi sites is from biomass burning. Figure S3 shows that whilst regional biomass burning emissions are decreasing, emissions closest to the Yangambi and Darwin sites are increasing, so it is difficult to predict how precursor emissions at each site may differ between the model and observations. Standard deviations are calculated using interannual variability where possible and are intended to suggest how sensitive the model may be to changes in meteorology and emissions.
Table 1Information on ozone measurement stations and sites used in this study. The first eight rows are remote sites. Latitude and longitude refer to the grid cell centre. The locations of individual stations are given in Table S1.
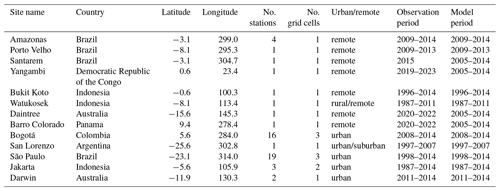
It is important to acknowledge that the lowest 40 m, represented by a single UKESM1 “surface level” grid cell, has a substantial ozone gradient that is not resolved by the model. However, without measurements of the form of this gradient at each site, no attempt is made to reconcile the model and measurement height. The rainforest canopy usually sits at 25–40 m above the ground, with the tallest trees reaching 60 m. Therefore, although above-canopy measurement stations are consistent with the model grid cell height (see Supplement), models do not include important characteristics of the canopy such as turbulent mixing regimes between below- and above-canopy layers and cannot resolve height-dependent processes within the 40 m. To further understand how successfully the site may represent the grid cell as a whole, we consider the homogeneity of the grid cells in terms of emissions and land cover. For example, all urban sites will contain inhomogeneous urban emissions sources. Many precursors are transported to remote sites by remote air masses, which are likely distributed fairly uniformly across the grid cell, although some remote sites may contain biomass burning emissions. Using reanalysis data from GFED4s (van der Werf et al., 2017), we confirm fire activity within the Daintree grid cell, in addition to some smaller and more infrequent burned areas at the Porto Velho and Yangambi sites. Furthermore, land cover in UKESM1 shows the Yangambi, Bukit Koto and Watukosek sites contain some agriculture, and Bukit Koto, Watukosek, Daintree and Barro Colorado are all adjacent to ocean. Based on this analysis, the Amazonas and Santarem sites are the most homogeneous grid cells and therefore may be best represented by the model.
To calculate ozone mean values, station data are converted to a monthly climatological data composite at each site before averaging all stations within the same grid cell. This avoids biases if some stations have a longer period of recording than other stations in the same grid cell or if some months have limited measurement data. To calculate year-to-year variability, data from all stations within a site are first averaged to create a monthly time series. For comparison to annual mean ozone, standard deviations are then taken using annual means for years with 11 or more months of data, regardless of the number of stations contributing to each month. For comparison of monthly mean ozone, standard deviations are taken for each month. Figure S2 in the Supplement shows the total number of days of data contributing to the analysis in each month. Diurnal cycle data from the TOAR I database are only available as a monthly mean climatology, so no standard deviation is available for the observations of the diurnal ozone range (DOR) for these sites. To evaluate how well UKESM1 captures differences among sites or months, we calculate a Pearson's coefficient of determination (r2) and/or root mean square error (RMSE).
This study applies the DOR metric to quantitatively evaluate the model's ability to capture the increase in ozone concentration during the day compared to the night at different locations and seasons (Piikki et al., 2009). The DOR is the difference between the minimum and maximum ozone concentrations measured over a diurnal cycle. Although the time that the minimum and maximum occur varies with season and latitude, the DOR is independent of the time of these minima and maxima. Studies in remote locations have shown that the DOR is related to the diurnal temperature range and is highest inland, lower to the ground and in valleys (Klingberg et al., 2012). For example, above the canopy, ozone concentrations tend to show less diurnal variation than closer to the canopy; downdrafts of ozone from the free troposphere occur throughout the night because of a contracted planetary boundary layer (PBL), and there are fewer deposition processes than within the canopy, leading to higher ozone concentrations overnight and therefore less diurnal variability. In polluted regions, the DOR can also depend on entrainment and circulation of ozone-rich air masses (Klumpp et al., 2006). The ability of UKESM1 to capture the DOR reflects the ability of the model to accurately reproduce the diurnal ozone cycle for varying environmental conditions.
To understand factors affecting ozone concentrations in UKESM1, monthly mean NOx concentration and ozone production rate at the lowest model level are also calculated for the period 2004–2015 for the eight remote sites. Additionally, tropospheric NO2 columns are produced by summing NO2 below the modelled tropopause height. These values are compared to tropospheric NO2 columns from the TROPOMI instrument on the Sentinel-5 satellite. We use daily tropospheric NO2 columns from 2004–2015 at an overpass time of 13:00 LT, converted to monthly means and regridded to the resolution of UKESM1.
3.1 Average ozone concentrations at each site
UKESM1 overestimates ozone concentrations at all sites by an average of 18.1 nmol mol−1, a factor of 2, but the bias varies from 8.8 to 33.2 nmol mol−1 across sites (Fig. 2). The positive bias indicates that UKESM1 overestimates ozone concentrations at surface level where it is relevant to human and ecosystem health.
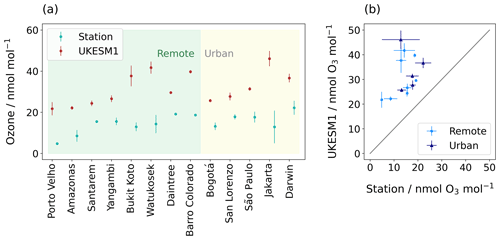
Figure 2Mean annual ozone concentration at each site compared to in the corresponding grid cell of UKESM1 (a) by site and (b) showing the correlation between the model and observations. Bars represent 1 standard deviation based on annual means and represent interannual variability. Missing bars in the observations are due to insufficient data.
Despite the positive bias, UKESM1 captures the relationship in average ozone concentrations between sites, except in Indonesia (Fig. 2b). Excluding Bukit Koto, Jakarta and Watukosek, the mean bias is 13.0 nmol mol−1 (r2=0.61, p=0.01). Grouping by region, the mean bias (and mean % bias) in South America is 12.1 nmol mol−1 (91 %), in Indonesia is 28.5 nmol mol−1 (213 %), in Australia is 12.4 nmol mol−1 (60 %) and in Africa is 11.1 nmol mol−1 (71 %). The limited number of sites in each region creates uncertainty in the regional pattern of the bias, but from the data available, there is high confidence that the bias in Indonesia is greater than at the other sites (p=0.0001 when using Student's t test to compare the bias for sites in Indonesia against all other sites). There are no differences between the magnitudes of the bias in remote compared to urban areas when groups are compared using Student's t test (p>0.05) and low confidence in a correlation between the magnitude of the ozone concentration and the bias. The observed annual means are within a range of 5 to 25 nmol mol−1, with 11 of the 13 sites within a range of 10 to 20 nmol mol−1, whereas annual means in UKESM1 range from 20 to 50 nmol mol−1.
3.2 The diurnal cycle of ozone at remote sites
Analysis of the diurnal cycle reveals that the positive bias in the annual mean is due to a systematic overestimation of ozone concentrations across all hours of the day, so UKESM1 performs similarly during day and night (Fig. S4 in the Supplement). As expected, UKESM1 predicts an increase in ozone concentrations at sunrise, a peak in the mid-afternoon and decline into the night, although the exact shape of the diurnal cycle varies at each site. At Daintree, for example, night-time ozone concentrations drop by only a few nmol mol−1, whereas at Watukosek the annual mean diurnal cycle ranges from 4.2 to 30.9 nmol mol−1 (Fig. S4). Qualitatively, UKESM1 captures the variation well over the diurnal cycle at the Amazon sites (Fig. S4a–c) and the Yangambi site (Fig. S4d) but performs less well at the Daintree site (Fig. S4g). The very shallow diurnal cycles modelled at Daintree and Barro Colorado are likely due to the coastal nature of the sites. The ozone deposition rate over ocean is low, which results in a diminished diurnal variation because ozone is not removed efficiently overnight.
To quantify whether the model is able to capture the magnitude of the changes in diurnal cycle, we examine the DOR at each site (Fig. 3). The observations show an average DOR of 17.1 nmol mol−1, with individual sites ranging from 2.2 nmol mol−1 at the Daintree site to 30.3 nmol mol−1 at the São Paulo site (Fig. 3a). Comparing the annual mean DOR between the model and observations, we confirm that UKESM1 is able to capture the DOR with reasonably well. The mean bias is 2.7 nmol mol−1, with a range of −11.6 to 15.1 nmol mol−1 across different sites, so, unlike the absolute ozone concentrations, UKESM1 does not exhibit a systematic high bias in the DOR (Fig. 3b). As a percentage, the mean bias across all sites is 16 %, and UKESM1 is able to capture the differences in the DOR between sites (r2=0.64, p=0.005). The overall RMSE of 7.1 nmol mol−1 over all sites is largely driven by urban sites Jakarta and Darwin, whereas selecting only remote sites gives an RMSE of 4.8 nmol mol−1.
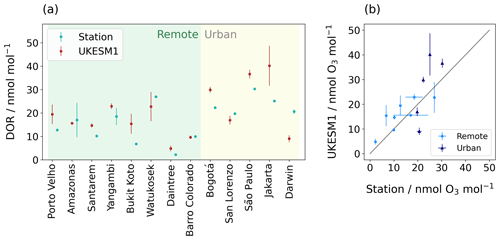
Figure 3Mean diurnal ozone range (DOR) at each site compared to the corresponding grid cell of UKESM1 (a) by site and (b) showing the correlation between the model and observations. Bars represent 1 standard deviations using annual means to indicate interannual variability. Missing bars in the observations are due to insufficient data.
We also validate whether the model captures the time of the maxima and minima in the diurnal cycles (Fig. 4). To the nearest hour, the minimum ozone concentration tends to occur slightly earlier in UKESM1 than observed, and the maximum occurs later. The Bukit Koto and Daintree sites have a diurnal cycle with a small amplitude (Fig. S4e and g), which causes the minimum hour to be misrepresented, but at all other sites the model and observations differ by 2 h or less (Fig. 4).
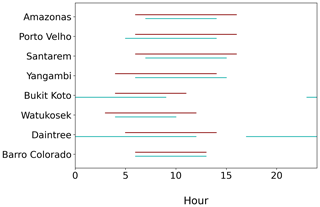
Figure 4The hours in the diurnal cycle during which ozone is increasing in the observations (solid blue line) and UKESM1 (solid red line); i.e. the line connects the minimum ozone concentration and the maximum ozone concentration. The minimum and maximum values at the Barro Colorado site are the same for both observations and UKESM1.
3.3 Daily ozone variation
Figure S5 in the Supplement shows histograms of the anomalies in daily mean ozone concentration compared to the monthly mean at remote sites. A broader distribution indicates higher variability in day-to-day ozone anomalies. Most remote sites show daily deviations of up to 10 nmol mol−1 from the monthly mean, and a few (Yangambi, Watukosek and Bukit Koto) show days with ca. 20 nmol mol−1 differences compared to the monthly mean (Fig. S5c, d and e). UKESM1 overestimates the frequency of these events at Bukit Koto and Watukosek, as well as in Porto Velho (Fig. S5b, d, and e).
Comparing the standard deviation, skew and kurtosis of the daily distribution plots, UKESM1 reproduces the variability in daily ozone concentration in several locations (Table S2). The model standard deviation is within 50 % of observations at 9 out of 13 sites but is overestimated at Bukit Koto, Watukosek, Porto Velho and São Paulo. Some patterns in the standard deviation between sites are captured, such as the broader distribution in Africa compared to South America, but, the overall relationship between different sites does not resemble observations (r2=0.34, p=0.05). The kurtosis describes the tailedness of the distribution; positive values indicate a higher number of days with large deviations from the monthly mean compared to a normal distribution. UKESM1 tends to overestimate the kurtosis of the distributions, exemplified at the Porto Velho site (Fig. S5b). The skew describes whether the distribution is shifted to one side relative to the zero value. The observational data show that a positive skew is present at all the sites except the Daintree site, indicating that events with substantially higher ozone than the monthly mean are more common than events with substantially lower ozone. UKESM1 displays a positive skew of a similar magnitude to that observed at all sites (a mean of 0.58 compared to 0.66 in observations) but cannot capture the relationship between sites (r2=0.07, p=0.39).
3.4 The seasonal ozone cycle at remote sites
As with the diurnal cycle, UKESM1 captures the seasonal cycle at several sites (Fig. 5) but overestimates ozone concentrations in absolute terms (RMSE=18.5 nmol mol−1). Often, the monthly means predicted by UKESM1 are greater than the daily maximum ozone recorded in each month (Fig. 5, blue dots).
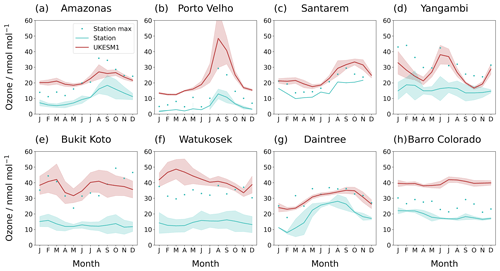
Figure 5Mean monthly ozone concentration at each site (solid blue line) compared to in the corresponding grid cell of UKESM1 (solid red line). Shading covers 1 standard deviation using monthly means to indicate interannual variability. The maximum ozone concentration recorded in each month using daily means is shown for observations (blue circles).
In South America (Fig. 5a–c), UKESM1 correctly captures an increase in ozone concentrations during biomass burning months July–October; however the increase is overestimated at the Porto Velho site. Porto Velho, in the arc of deforestation, records concentrations of less than 5 nmol mol−1 during the wet season (December–May), lower than other sites in South America, followed by an increase to 12.9 nmol mol−1 during the burning season. UKESM1 captures this seasonal pattern, but the magnitude of the increase in the burning season is 4 times larger than observed (Fig. 5b).
In Africa, the biomass burning season occurs in June–July in central Africa and December–February in northern Africa. The Yangambi site sits between these biomass burning areas, and ozone is expected to be transported to the site by seasonal circulation patterns. UKESM1 predicts much larger increases in ozone concentration during these months than is observed at the Yangambi site (Fig. 5d); the range of observed monthly means is 5.2 nmol mol−1 compared to 21.4 nmol mol−1 in the model. Surprisingly, the seasonal cycle predicted by UKESM1 is similar to the measured daily maximum ozone concentration in each month, which have a range of 20.1 nmol mol−1 (from 23.9 to 44.0 nmol mol−1; Fig. 5d, blue dots). This suggests that, although ozone concentrations can be much higher on specific days during biomass burning seasons compared to other months, these high-ozone events are not frequent enough to generate the large seasonal variation predicted by UKESM1. Similar features are seen at the Bukit Koto site in Indonesia (Fig. 5e); UKESM1 predicts a seasonal cycle that follows the variation in the observed daily maximum, which was 23.8 nmol mol−1 in May but reached 49.3 nmol mol−1 in October, rather than the monthly means, which are below 20 nmol mol−1.
The bias at the Bukit Koto, Yangambi and Porto Velho sites may be amended using a multiplicative linear correction rather than an additive correction because the modelled seasonal cycle has greater monthly variability in ozone than the observations. Applying a bias correction multiplier of 0.33, 0.55 and 0.25 for Bukit Koto, Yangambi and Porto Velho, respectively, brings the magnitude of the monthly means and the seasonal variation closer to observations (Fig. S6 in the Supplement); however it is not necessarily suitable for correcting daily or hourly biases. The seasonal variability at these sites is dominated by changes in biomass burning, suggesting that the model overestimates ozone formed from burning due to either incorrect emissions or process representation. At the other remote sites, the bias is consistent between months, and therefore the annual means in Fig. 2 represent the biases sufficiently well (or, in the case of Watukosek, the seasonal cycle is not represented well enough with either correction). In these cases, scaling the model output as in Fig. S6 removes the seasonality, suggesting a background bias that is not dependent on the local ozone concentration.
At Watukosek, the seasonal cycle from UKESM1 is completely different to observations (Fig. 5f), where monthly mean ozone concentrations are between 10 and 20 nmol mol−1 with only a small seasonal variation. Analysis of the surrounding area shows that the observed seasonal trend is captured by UKESM1 in adjacent ocean grid cells to the south (Fig. S7 in the Supplement). The grid cell chosen contains the measurement station but also the city of Surabaya, whereas the station may only be recording clean air outside of the city and therefore would be better represented by an adjacent grid cell.
Figure 6 shows the seasonal cycle in the DOR, which is captured more accurately at remote sites than the monthly mean ozone concentrations (r2=0.56, p=0.002 and RMSE=6.3 nmol mol−1), indicating that the relative change in ozone concentration over the day is well represented by UKESM1 across different seasons.
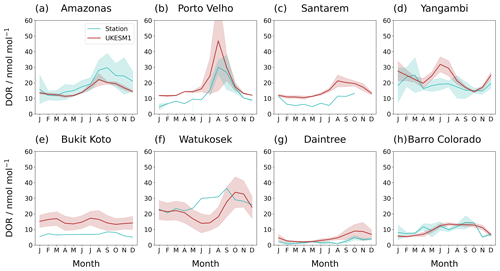
Figure 6Mean monthly diurnal ozone range (DOR) at each site (solid blue line) compared to in the corresponding grid cell of UKESM1 (solid red line). Shading covers 1 standard deviation except at the TOAR I sites (Santarem, Bukit Koto, Watukosek), where diurnal cycle data were only available as a monthly climatology.
At Porto Velho, the seasonal cycle in the DOR is captured substantially better than the monthly means (Fig. 5b), but UKESM1 still has a positive bias of 6.0 nmol mol−1, with the largest overestimation of 16.9 nmol mol−1 occurring in biomass burning months August and September (Fig. 6b). However, the standard deviation (shading) is large during August–September in the model and observations, so the site is clearly very sensitive to yearly variability.
Similarly, at the Yangambi site, the observed seasonal variation of 14.3 to 25.0 nmol mol−1 is still overestimated by UKESM1 during the June–July biomass burning months, giving a modelled seasonal variation of 14.3 to 31.9 nmol mol−1 (Fig. 6d). This is improved compared to the monthly mean concentrations but again highlights biomass burning months as periods with worse model performance.
At Bukit Koto, there is very little variation in the DOR by month, and UKESM1 overestimates it by 5.1 to 10.6 nmol mol−1 (Fig. 6e). As with the monthly means, the model fails to capture the seasonal cycle in the DOR at Watukosek, displaying a very different pattern in April–August compared to the observed DOR (Fig. 6f).
To examine possible reasons for (i) the bias in the monthly means and (ii) the worse performance of the DOR at some sites, we consider how these variables are related to NOx concentrations. At the majority of remote sites in the tropics, ozone production is controlled by NOx concentrations; i.e. with the exception of Watukosek, the sites are NOx-limited because ozone production rate increases with increasing NOx (Fig. 7a). Here, ozone production rate is defined as the rate of reaction , with NO controlling variability at NOx-limited sites. At Watukosek, the seasonality in ozone production rate is less clearly attributable to NOx concentrations, which indicates other factors (such as VOC concentration and meteorology) are involved.
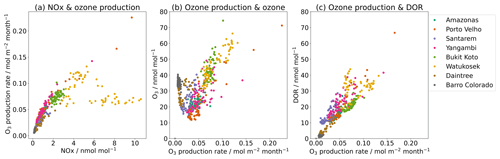
Figure 7Relationship between monthly mean ozone production rate at the surface at each remote site for (a) surface NOx concentration, (b) surface ozone concentration and (c) the diurnal ozone range (DOR).
However, seasonal patterns in mean ozone concentrations can differ from ozone production rate due to changes in chemical loss rate and non-chemical factors such as deposition and transport. Two sites that highlight this in Fig. 7b are Daintree and Barro Colorado; the low ozone production rates suggest that transport to these sites causes ozone concentrations to be high and unrelated to seasonality in ozone production. As coastal sites, they are likely to be strongly influenced by coastal weather phenomena, which may include thermally driven transport. However, the DOR, which shows different seasonality to mean ozone concentrations at these sites (cf. Figs. 5 and 6), is correlated with the seasonal changes in ozone production (Fig. 7c). This suggests that the DOR may be useful in understanding local processes, and in particular NOx concentration is likely a major factor affecting the DOR. In fact, even at Watukosek, the seasonal cycle in the DOR is correlated with NOx concentrations (r2=0.58; Fig. S8 in the Supplement). This perhaps suggests that NO availability, and its change with daily insolation, is the main factor affecting the DOR. Of course, loss processes must also play a role in the DOR magnitude; however it certainly seems that, at these rural sites, ozone production and the DOR have strong relationships to seasonal NOx concentration.
Therefore, sites where UKESM1 performs less well at reproducing the observed DOR may indicate poor representation of local NOx chemistry within UKESM1. These sites include Yangambi, Watukosek and Bukit Koto, so we compare their tropospheric NO2 columns in UKESM1 to OMI satellite products (Fig. S9 in the Supplement). Yangambi and Bukit Koto show different seasonality in the NO2 columns at the site compared to the satellite product. This indicates that there could be an issue with prescribed emissions of NO2 or that NOx processes are poorly represented at these sites. Additionally, we find that NO2 columns from UKESM1 are approximately 3 times higher than the satellite columns, which likely signifies that NOx concentrations are too high in the model, although not necessarily at the surface. Inefficient boundary layer mixing of surface-emitted species may contribute to the aggregation of NOx in near-surface model levels. Given the relationship between NOx and ozone production (Fig. 7a), decreasing NOx concentration in UKESM1 would likely result in a decrease in ozone concentrations. However, it may also decrease the DOR and therefore would not be the only cause of the differences between modelled and observed ozone. It is also worth noting that NO2 columns are sensitive to the algorithm used to calculate the columns, including the method used to separate tropospheric from stratospheric NO2. Therefore, although a systematic high background NO2 in the troposphere may be a cause of the systematic ozone bias, more observations of NOx are needed to confirm this, as well as to understand whether a bias is related to emissions, the physical model or chemistry. On the other hand, the representation of the NOx seasonal cycle at Yangambi and Watukosek does seem likely to contribute to poor model performance of ozone seasonality and the DOR in UKESM1. In four other Earth system models performing the same simulation, the seasonality at Watukosek is captured better, whereas all models overestimate the change in ozone during biomass burning months at Yangambi, with UKESM1 performing among the best on account of the smaller seasonal variation (Fig. S10 in the Supplement).
4.1 How well does UKESM1 reproduce surface ozone in the tropics?
UKESM1 overestimates ozone in the tropics by a mean of 18.1 nmol mol−1 at 13 sites (Fig. 2), covering environments such as remote forests, urban areas and coastal locations (Fig. 1). In relative terms, we find that UKESM1 overestimates ozone concentrations by a factor of ca. 2, in agreement with Archibald et al. (2020a). The spatial differences in annual mean ozone concentrations among sites are captured reasonably well, although there is a large bias (+28.5 nmol mol−1) at the Indonesian sites (Watukosek, Jakarta, Bukit Koto). More promisingly, the diurnal ozone range (DOR) is reproduced with much smaller biases (RMSE=7.1 nmol mol−1) (Fig. 3), and seasonal cycles in the DOR are captured at most remote sites (Fig. 6). In conjunction with a good representation of the shape of the diurnal cycle (Figs. 4 and S4), the ability to model the DOR shows that UKESM1 can reproduce the increase in ozone concentration from its night-time minimum, including how it changes with season and location. This gives confidence in the ability of UKESM1 to represent the behaviour of surface ozone in the tropics.
This study shows that analysis of the DOR provides unique information on the seasonality in NOx concentrations and ozone production at these remote tropical sites. Seasonality in the DOR is strongly related to NOx concentrations, demonstrating that change in NO concentration over the day is an important contributor to the DOR. Overnight, absence of photolysis prevents the ozone-producing reaction as NO is locked up in NO2 and reservoir species, causing ozone concentrations to decline. During the day, NO is formed, and the maximum rate of ozone production is determined partly by the NOx concentration, allowing a greater diurnal increase in months with higher NOx. This is by no means the only process controlling the DOR but suggests that changes in local chemistry, such as NOx chemistry and subsequent ozone formation, seem to be captured by UKESM1.
Furthermore, the systematic ozone bias is present even in locations with low ozone production or where the ozone seasonal cycle is dominated by transport (e.g. Barro Colorado). Previous studies have indicated a bias at remote ocean sites of 10 ppb (Brown et al., 2022), indicating a background bias that likely extends across large parts of the tropics and does not necessarily originate at the site. From comparison of the seasonal cycle, we show that the bias at remote sites is largest during biomass burning seasons (Fig. 5), and bias correction at sites where the seasonal cycle is controlled by biomass burning was best applied using a multiplicative scaling factor, whereas at other sites, the bias is a constant value across all months (Figs. 5 and S6). Similarly, although UKESM1 reproduces the seasonal cycle in the DOR at remote sites (RMSE=6.3 nmol mol−1), performance is worse during months strongly impacted by biomass burning. This is true at both the Porto Velho and the Yangambi sites, possibly indicating that either the NOx chemistry, emission factors or the altitude of emission from biomass burning is incorrect in the model. Certainly, comparison to satellite tropospheric NO2 columns at Yangambi suggests NOx is a contributor to the differences in ozone behaviour between the model and observations (Fig. S9). Regardless, on the whole the magnitude of the seasonal cycle is captured better in the DOR than the absolute ozone concentrations. This suggests that there is a systematic error, for example, from an incorrect emission factor, a missing process or unresolved subgrid-scale processes, and further work is needed to identify the cause of the systematic bias. In Europe and North America, UKESM1 tends to produce an underestimation of surface ozone in December–February and a positive bias in July–August (Archibald et al., 2020a), similar to other models (Young et al., 2018). Turnock et al. (2020) found that recent Earth system models have improved the negative bias over the Northern Hemisphere, but a positive bias remains elsewhere. Whilst the negative bias is attributed to excessive NOx titration, the cause of the positive bias has not been conclusively determined. Causes of model bias are discussed in more detail in the following sections.
This bias is not unique to UKESM1; a positive bias is present in several Earth system models that took part in CMIP6, although it is larger than most in UKESM1 (Fig. S10). Crucially, however, the magnitude of the mean bias does not relate to the model's ability to capture the seasonal cycle, highlighting that bias in the mean state does not necessarily reflect the model representation of trends and variability. In this paper, we focus on UKESM1, confirming that there is a bias in the mean state in the tropics, yet also demonstrating the model has success in reproducing seasonality and the DOR at several sites. In this way, UKESM1 can be a useful tool to understand surface ozone processes and responses to changing forcings. With appropriate bias correction, UKESM1 has been used to assess health burdens in different scenarios (Turnock et al., 2023; Akriditis et al., 2024), and this study allows for further understanding of the bias in the tropics. This can reduce uncertainty in the assessments in this area.
4.2 Challenges to process representation as a result of resolution
The coarse resolution of UKESM1 provides a different type of information when comparing a grid cell average to only a few measurement stations. Station measurements may not be representative of the grid cell as a whole, especially if there is spatial heterogeneity in precursor sources or meteorological features (e.g. from mountains) within the grid cell (Young et al., 2018). Many sites in this study have a limited duration of measurements and contain only one measurement station (Table 1), exacerbating the discrepancy between the model and observations without necessarily indicating a model weakness (Schutgens et al., 2017). In several cases, observations were only available outside of the model time range, which ended in 2014. Although the meteorology is shown to be representative of the present day, prescribed emissions such as those from biomass burning are not identical between the model and observations (Fig. S3), which is especially important if there is a trend over time. Lack of long-term monitoring means that there is no clear idea of temporal trends in surface ozone concentrations in the tropics, although observations at American Samoa do not detect any significant change in background ozone over the period 1975 to 2014 (Griffiths et al., 2020). At the site level, local changes in emissions such as decreasing fire activity in the African savannah and increasing deforestation fires in African forest (van Marle et al., 2017) are likely to cause some differences between the model and observations. Continued monitoring and evaluation of ozone concentrations at recently established stations will be instrumental to furthering understanding of ozone in the tropics.
To demonstrate how ozone concentrations can vary considerably within a grid cell, we can use the Amazonas site as an example. This site contains four monitoring stations, among which the average ozone concentration differs from the grid cell average by 9 % to 23 %, and individual monthly mean values vary by up to 95 % from the grid cell monthly mean (Fig. S11 in the Supplement). These differences can come from the altitude of the measurement station as well as numerous other reasons including proximity to precursor emissions sources, prevailing air flow direction and surface type. At the Amazonas site, stations T2 and T3 are in clearings close to the ground and downwind of the city of Manaus, whereas T0z is above the canopy and upwind of Manaus. As ozone concentrations decrease rapidly within the canopy (Sörgel et al., 2020), measurement stations capturing air that has been depleted of ozone from in-canopy loss processes are incongruous with model data, which represent ozone concentrations above the canopy. In general, measurement stations are located above the canopy, although future studies may consider adjusting modelled concentrations to match the measurement height more precisely.
Several sites in this study are coastal (the model grid cell is split between ocean and land), namely Bukit Koto, Watukosek, Daintree and Yangambi. Due to a low deposition velocity of ozone over water (Sarwar et al., 2016; Luhar et al., 2018) and limited oceanic emission sources, concentrations of ozone over the ocean in UKESM1 are ∼20 nmol mol−1, and minimal diurnal variation is present. The grid cell chemistry and deposition velocities along coasts will be an average of the land and ocean, implying that the grid cell ozone concentration may not be representative of the site and the DOR is likely to be lower.
The resolution of UKESM1 can also introduce biases because emissions that, in reality, often occur as small, concentrated plumes are spread homogeneously across the whole grid cell volume. Of the remote sites included here, Daintree shows local fire emissions within the model grid cell that would be affected by this, possibly resulting in inaccurate representation of NOx concentrations and ozone formation. The formation of ozone depends critically on relative concentrations of precursors, so resolution can dramatically change rates of production and loss (e.g. Archibald et al., 2020b). Dilution of NOx spatially and temporally due to coarse resolution can increase its ozone production efficiency and alter its lifetime (Chatfield and Delany, 1990; Wild and Prather, 2006). In the horizontal, Wild and Prather (2006) show that diluting point sources of NOx over a large grid cell can bring NOx into contact with BVOCs more immediately as the separation of clean and polluted regions is unable to be resolved. In the vertical, coarse resolution can prevent build-up of NOx at the surface, which decreases surface deposition processes and NOx titration (Chatfield and Delany, 1990; Nassar et al., 2009). Both processes lead to increased ozone concentrations in source regions, so it is likely that coarse resolution partially contributes to the larger biases in biomass burning regions (Fig. 4b). In this simulation, NOx emissions (except from interactive lightning) are provided as monthly means, thereby also diluting emissions over time, which has been similarly shown to increase ozone production (Chatfield and Delany, 1990). Changes in temporal resolution may be more pronounced for emissions with high temporal variability such as biomass burning. On the other hand, prescribing monthly emissions of NOx did not seem to reduce the ability of UKESM1 to simulate the daily variability in ozone (Fig. S5, Table S2), which is governed by interactive processes including BVOC emissions, lightning NOx and meteorology.
4.3 Challenges to process representation due to knowledge gaps
To calculate ozone concentrations, chemistry models must necessarily parameterise and simplify the atmospheric chemistry and deposition processes that lead to ozone formation. This includes, among other simplifications, grouping VOCs by their size, reactivity or functional groups and parameterising stomatal and non-stomatal deposition (Archibald et al., 2020a; Hardacre et al., 2015). A semi-mechanistic process in UKESM1 determines biogenic emissions of isoprene and monoterpenes (Pacifico et al., 2012), which, although it agrees with the global average estimate (Sindelarova et al., 2022), is poorly constrained by observations. To lead to ozone formation, isoprene undergoes several other chemical reactions. This process has considerable uncertainty, especially with regards to recycling of OH and NOx (Fiore et al., 2005; Lee et al., 2013), and is challenging to validate (Horowitz et al., 2007; Schwantes et al., 2020). Furthermore, the reactions determining the fate of isoprene and other VOCs are too numerous, and the lack of detailed reaction-kinetic information is an obstacle to including them explicitly in the model (Archibald et al., 2010). However, studies which include a more detailed suite of organic oxidation products and explicit HOx recycling mechanisms have been shown to further increase the bias in UKESM1, especially over tropical forests, suggesting this bias is due to other processes (Archer-Nicholls et al., 2021; Weber et al., 2021).
As for NOx, it is possible that UKESM1 overestimates NOx concentrations in the boundary layer. Tropospheric NOx columns are 3 times higher in UKESM1 compared to the OMI satellite product (Fig. S9), but the complete reasons for this require further investigation. Previous studies have identified insufficient venting of chemical species out of the boundary layer (O'Connor et al., 2014). This suggests an issue with the physical model, which has not yet been solved. Injection height may also play a role in surface NOx concentrations; NOx is injected at the surface rather than at different vertical levels, with the exception of aircraft emissions (Archibald et al., 2020a). Leung et al. (2007) show that varying injection altitudes of biomass burning emissions resulted in increases in ozone further above the PBL and greater transport efficiency of NOx to remote regions. Compared to our study, ozonesondes at Watukosek record lower tropospheric ozone concentrations greater than 60 nmol mol−1 during biomass burning season (Adedeji et al., 2020; Komala et al., 1996), which is similar to the ozone concentrations predicted by UKESM1 but larger than the measurement station (10–20 nmol mol−1) (Fig. 5f). However, there are many other uncertainties associated with biomass burning, such as emission factors of both NOx and organic compounds (Schultz et al., 2008) and subsequent chemistry (Young et al., 2018), that may contribute to biases. The NOx emission factors for savannah and grassland fires used in CMIP6 (van Marle et al., 2017) are at the upper end of the range used by other inventories (Jin et al., 2021), and isoprene emission factors from C4 grasses are likely to have been overestimated in this setup of UKESM1 (Weber et al., 2023). An overestimation of either or both of these factors could contribute to model bias during biomass burning seasons, especially in the Congo, where most burning occurs in the savannah biome, and are likely to be adapted for CMIP7.
Uncertainty in emissions is especially high for peat fires (Christian et al., 2003; Nassar et al., 2009), which can make up the majority of fire emissions in southeast Asia (Gaveau et al., 2014), but is poorly represented in the model. In fact, UKESM1 did not perform well in Indonesia against most metrics used in this study. Further analysis of the surrounding grid cells showed large variation in the magnitude and pattern of the seasonal cycle between adjacent grid cells (Fig. S7). In Watukosek, for example, the measured seasonal cycle shows a similar pattern to the cycle over the ocean grid cells, which is quite different from the pattern over land. It is likely that the station is exposed to ocean air masses, whereas the grid cell responds to anthropogenic emissions that change the seasonal pattern. Since other models with the same prescribed emissions display a seasonal cycle at Watukosek that looks closer to observations (Fig. S10f), the poor performance of UKESM1 likely relates to transport of anthropogenic emissions and their chemistry within UKESM1 rather than the emissions themselves. Indonesia is a mosaic of agriculture, forest and dense megacities, in addition to having a complex meteorology affected by summer monsoon circulation, ENSO and outflow from continental Asia (biomass burning in February–April) (Permadi and Oanh, 2021). A more detailed analysis of these separate processes and their representation in UKESM1 is needed to understand the cause of the model errors.
4.4 Improving understanding of surface ozone in the tropics
For this evaluation, we have synthesised real-world tropical surface ozone concentrations from more sites than previously available in the literature. Whilst modelled ozone concentrations are necessary for a range of applications, the existing observational data already reveal that surface ozone in the tropics may already be crossing safe thresholds for vegetation and human health. Although annual mean ozone concentrations are below 20 nmol mol−1 at all sites (Fig. 2), we show that there is large variability in ozone concentrations that can exceed 40 nmol mol−1 at times, even in remote tropical forests (Fig. 5). Daily mean ozone concentrations can vary by up to 20 nmol mol−1 from the monthly mean at the Indonesian sites and in Yangambi (Fig. S5), with the highest daily mean values being more than double the monthly mean at the Amazonas, Yangambi, Bukit Koto and Watukosek sites (Fig. 5). In absolute terms, daily means greater than 30 nmol mol−1 were recorded at all sites except Santarem and the annual mean diurnal cycle peaks at over 30 nmol mol−1 on average at Watukosek (Fig. S4), Jakarta and Bogotá (not shown). Furthermore, over the course of a day, ozone concentrations vary by over 20 nmol mol−1 on average at the Watukosek, Jakarta, São Paulo and Bogotá sites (Figs. 3 and S4), meaning that ozone concentrations during daylight hours are higher than annual, monthly or daily means represent. This increases the risk to ecosystems, demonstrated by Cheesman et al. (2023), who have shown that using hourly data instead of monthly means can increase modelled stomatal ozone uptake by 40 %. Since our study focuses on remote sites, further research is needed to evaluate the human health impacts at urban sites. Recently Gaudel et al. (2024) have shown that long-term, continuous monitoring is required in several tropical regions to reliably detect ozone trends, and the ground-based measurements used here are mostly too limited in duration for trend analysis at the present time. Clearly, more studies and greater monitoring are needed to evaluate the human and ecosystem impacts in these globally important regions, with emphasis on maintaining existing sites for trend analysis and more robust datasets.
To increase understanding of ozone in the tropics, we identify a gap in monitoring of the savannah/grassland regions such as northern and central Africa and Cerrado, the savannah region in Brazil. In general, Africa is under-represented by monitoring stations, despite large variation across the continent from growing cities and seasonal variation in biomass burning and circulation. Increased monitoring of ozone over areas with large populations and of ecological importance would significantly help in assessing the environmental risk factors to human and plant health.
Areas with poor model performance, large intermodel spread and high uncertainty in future trends could also benefit from increased observational data to help constrain model predictions. In this study, UKESM1 performed worst over Indonesia, an area previously identified as having a high intermodel standard deviation in ozone concentration (Young et al., 2013). Previous studies have also identified southern Africa as an area of high future uncertainty due to intermodel variation of surface ozone changes in response to climate change (Brown et al., 2022) and precursor emissions (Turnock et al., 2020).
We show that UKESM1 can capture observed variability in surface ozone concentrations across the tropics such as increased ozone over the diurnal cycle and during biomass burning seasons. However, UKESM1 overestimates surface ozone concentrations by a factor of 2 on average. The mean bias is 18.1 nmol mol−1, but this varies with location and season, with the largest positive bias of 28.5 nmol mol−1 occurring in Indonesia. In other locations, biases are generally largest during biomass burning seasons, which suggests emission factors from fires may need to be revised. Coarse resolution may affect the processes being represented, which can lead to biases; future studies should aim to quantify the effect of increasing resolution in order to better identify model deficiencies. Biases are substantially smaller in the diurnal ozone range (DOR); UKESM1 reproduces the DOR, which represents the change in ozone concentration over the diurnal cycle, with a mean bias of 2.7 nmol mol−1 (15.9 %), including how it varies seasonally (RMSE=6.3 nmol mol−1). Analysis of the DOR allows local-scale responses to be considered separately to the systematic bias and may be a useful diagnostic for other researchers to consider. Overall, our results suggest that UKESM1 can be useful for understanding ozone responses to forcings, but hourly data should not be used “off the shelf” for health and ecosystem impact assessments. Bias correction is an option to avoid overestimation of the risks, but users should be aware that monthly mean concentrations may require multiplicative bias correction in biomass burning regions and that grid cells containing non-homogeneous emission sources or land cover types may be impacted by the negative effects of coarse model resolution more than pristine regions. The magnitude of the bias in different regions and seasons and its dependence on factors such as distance from emissions sources remain to be quantified. For this, more in situ monitoring is instrumental.
Furthermore, the observed ozone concentrations show that tropical ozone concentrations are highly variable in space and time. Ozone concentrations on individual days can be double the monthly mean concentration, in addition to DORs that are regularly greater than 20 nmol mol−1 at both urban and remote sites. Further studies on human and ecosystem risks in the tropics are required, and we encourage the inclusion of hourly surface ozone output from all models as a default option.
Data are open-access. Processed data used to make the figures are available at https://doi.org/10.5281/zenodo.11583524 (Brown, 2024).
Data from UKESM1 (Tang et al., 2019) are available from ESGF (https://esgf-node.llnl.gov/search/cmip6/, last access: September 2023).
Please see individual data repositories for data use statements.
Daily data for Barro Colorado, Yangambi and Daintree are available at https://doi.org/10.5281/zenodo.10252774 (Brown, 2024).
Data for the São Paulo, Bukit Koto, Watukosek, Jakarta, Amazonas, Bogotá, Santarem and San Lorenzo sites are available on the TOAR I database (https://doi.org/10.34730/4d9a287dec0b42f1aa6d244de8f19eb3, Schröder et al., 2021).
Data for Darwin are available at NTEPA (NTEPA, 2023; http://ntepa.webhop.net/NTEPA/, last access: September 2023).
The supplement related to this article is available online at: https://doi.org/10.5194/acp-24-12537-2024-supplement.
Conceptualisation: FB. Data curation: PA, LR, AWC, IdSV, HV, PB, MB, MD, AZ, NR, NK. Resources: PA, LR, AWC, IdSV, HV, PB, MB, MD, AZ, NR, NK, ST. Supervision: GF, SS, AWC. Analysis: FB. Visualisation: FB. Writing (original draft): FB. Writing (review and editing): all authors.
The contact author has declared that none of the authors has any competing interests.
Publisher's note: Copernicus Publications remains neutral with regard to jurisdictional claims made in the text, published maps, institutional affiliations, or any other geographical representation in this paper. While Copernicus Publications makes every effort to include appropriate place names, the final responsibility lies with the authors.
This article is part of the special issue “Tropospheric Ozone Assessment Report Phase II (TOAR-II) Community Special Issue (ACP/AMT/BG/GMD inter-journal SI)”. It is not associated with a conference.
Stephen Sitch and Alexander W. Cheesman were supported by NERC funding (grant no. NE/R001812/1). Gerd A. Folberth was supported by the Met Office Hadley Centre Climate Programme, funded by BEIS and by the EU Horizon 2020 Research Programme CRESCENDO project (grant no. 641816). Inês Dos Santos Vieira was funded by the Fonds Wetenschappelijk Onderzoek Flanders (FWO; grant no. G018319N). The contributions of Steven Turnock were funded by the Met Office Climate Science for Service Partnership (CSSP) China project under the International Science Partnerships Fund (ISPF). The authors are grateful to the field and chemistry analytical technicians who operate the stations and help to provide reliable data. Further thanks go to editors and reviewers for their comments, patience and support with this paper.
This research has been supported by UK Research and Innovation (grant no. NE/S007504/1).
This paper was edited by Tim Butler and reviewed by two anonymous referees.
Adedeji, A. R., Dagar, L., Petra, M. I., and Silva, L. C. D.: Sensitivity of WRF-Chem model resolution in simulating tropospheric ozone in Southeast Asia, IOP C. Ser. Earth Env., 489, 012030, https://doi.org/10.1088/1755-1315/489/1/012030, 2020.
Ainsworth, E. A., Yendrek, C. R., Sitch, S., Collins, W. J., and Emberson, L. D.: The effects of tropospheric ozone on net primary productivity and implications for climate change, Annu. Rev. Plant Biol., 63, 637–661, https://doi.org/10.1146/annurev-arplant-042110-103829, 2012.
Akimoto, H., Mori, Y., Sasaki, K., Nakanishi, H., Ohizumi, T., and Itano, Y.: Analysis of monitoring data of ground-level ozone in Japan for long-term trend during 1990–2010: Causes of temporal and spatial variation, Atmos. Environ., 102, 302–310, https://doi.org/10.1016/j.atmosenv.2014.12.001, 2015.
Archibald, A. T., Jenkin, M. E., and Shallcross, D. E.: An isoprene mechanism intercomparison, Atmos. Environ., 44, 5356–5364, https://doi.org/10.1016/j.atmosenv.2009.09.016, 2010.
Archibald, A. T., O'Connor, F. M., Abraham, N. L., Archer-Nicholls, S., Chipperfield, M. P., Dalvi, M., Folberth, G. A., Dennison, F., Dhomse, S. S., Griffiths, P. T., Hardacre, C., Hewitt, A. J., Hill, R. S., Johnson, C. E., Keeble, J., Köhler, M. O., Morgenstern, O., Mulcahy, J. P., Ordóñez, C., Pope, R. J., Rumbold, S. T., Russo, M. R., Savage, N. H., Sellar, A., Stringer, M., Turnock, S. T., Wild, O., and Zeng, G.: Description and evaluation of the UKCA stratosphere–troposphere chemistry scheme (StratTrop vn 1.0) implemented in UKESM1, Geosci. Model Dev., 13, 1223–1266, https://doi.org/10.5194/gmd-13-1223-2020, 2020a.
Archibald, A. T., Turnock, S. T., Griffiths, P. T., Cox, T., Derwent, R. G., Knote, C., and Shin, M.: On the changes in surface ozone over the twenty-first century: sensitivity to changes in surface temperature and chemical mechanisms: 21st century changes in surface ozone, Philos. T. Roy. Soc. A, 378, https://doi.org/10.1098/rsta.2019.0329, 2020b.
Artaxo, P., Hansson, H. C., Machado, L. A. T., and Rizzo, L. V.: Tropical forests are crucial in regulating the climate on Earth, PLOS Climate, 1, e0000054, https://doi.org/10.1371/journal.pclm.0000054, 2022.
Bond, D. W., Steiger, S., Zhang, R., Tie, X., and Orville, R. E.: The importance of NOx production by lightning in the tropics, Atmos. Environ., 36, 1509–1519, https://doi.org/10.1016/S1352-2310(01)00553-2, 2002.
Brown, F., Folberth, G. A., Sitch, S., Bauer, S., Bauters, M., Boeckx, P., Cheesman, A. W., Deushi, M., Dos Santos Vieira, I., Galy-Lacaux, C., Haywood, J., Keeble, J., Mercado, L. M., O'Connor, F. M., Oshima, N., Tsigaridis, K., and Verbeeck, H.: The ozone–climate penalty over South America and Africa by 2100, Atmos. Chem. Phys., 22, 12331–12352, https://doi.org/10.5194/acp-22-12331-2022, 2022.
Brown, F., Cheesman, A., Boeckx, P., Rojas, N., Artaxo, P., Dos Santos Vieira, I., Verbeeck, H., Bauters, M., Rizzo, L., Detto, M., zambrano, A., Mbifo, J., Sitch, S., Folberth, G., Komala, N., and Turnock, S.: Empirical data for: Performance evaluation of UKESM1 for surface ozone across the pan-tropics, Zenodo [data set], https://doi.org/10.5281/zenodo.11583524, 2024.
Chang, K.-L., Petropavlovskikh, I., Cooper, O. R., Schultz, M. G., and Wang, T.: Regional trend analysis of surface ozone observations from monitoring networks in eastern North America, Europe and East Asia, Elem. Sci. Anthr., 5, 50, https://doi.org/10.1525/elementa.243, 2017.
Chang, K.-L., Cooper, O. R., Gaudel, A., Petropavlovskikh, I., and Thouret, V.: Statistical regularization for trend detection: an integrated approach for detecting long-term trends from sparse tropospheric ozone profiles, Atmos. Chem. Phys., 20, 9915–9938, https://doi.org/10.5194/acp-20-9915-2020, 2020.
Chatfield, R. B. and Delany, A. C.: Convection links biomass burning to increased tropical ozone: However, models will tend to overpredict O3, J. Geophys. Res.-Atmos., 95, 18473–18488, https://doi.org/10.1029/JD095iD11p18473, 1990.
Cheesman, A. W., Brown, F., Farha, M. N., Rosan, T. M., Folberth, G. A., Hayes, F., Moura, B. B., Paoletti, E., Hoshika, Y., Osborne, C. P., Cernusak, L. A., Ribeiro, R. V., and Sitch, S.: Impacts of ground-level ozone on sugarcane production, Sci. Total Environ., 904, 166817, https://doi.org/10.1016/j.scitotenv.2023.166817, 2023.
Christian, T. J., Kleiss, B., Yokelson, R. J., Holzinger, R., Crutzen, P. J., Hao, W. M., Saharjo, B. H., and Ward, D. E.: Comprehensive laboratory measurements of biomass-burning emissions: 1. Emissions from Indonesian, African, and other fuels, J. Geophys. Res.-Atmos., 108, 4719, https://doi.org/10.1029/2003JD003704, 2003.
Dantas, G., Siciliano, B., França, B. B., da Silva, C. M., and Arbilla, G.: The impact of COVID-19 partial lockdown on the air quality of the city of Rio de Janeiro, Brazil, Sci. Total Environ., 729, 139085, https://doi.org/10.1016/j.scitotenv.2020.139085, 2020.
Detto, M. and Pacala, S. W.: Plant hydraulics, stomatal control, and the response of a tropical forest to water stress over multiple temporal scales, Global Change Biol., 28, 4359–4376, https://doi.org/10.1111/gcb.16179, 2022.
Emberson, L.: Effects of ozone on agriculture, forests and grasslands, Philos. T. Roy. Soc., 378, 2831, https://doi.org/10.1098/rsta.2019.0327, 2020.
Eyring, V., Bony, S., Meehl, G. A., Senior, C. A., Stevens, B., Stouffer, R. J., and Taylor, K. E.: Overview of the Coupled Model Intercomparison Project Phase 6 (CMIP6) experimental design and organization, Geosci. Model Dev., 9, 1937–1958, https://doi.org/10.5194/gmd-9-1937-2016, 2016.
Felzer, B. S., Cronin, T., Reilly, J. M., Melillo, J. M., and Wang, X.: Impacts of ozone on trees and crops, C. R. Geosci., 339, 784–798, https://doi.org/10.1016/j.crte.2007.08.008, 2007.
Fiore, A. M., Horowitz, L. W., Purves, D. W., Levy II, H., Evans, M. J., Wang, Y., Li, Q., and Yantosca, R. M.: Evaluating the contribution of changes in isoprene emissions to surface ozone trends over the eastern United States, J. Geophys. Res.-Atmos., 110, D12, https://doi.org/10.1029/2004JD005485, 2005.
Gaudel, A., Cooper, O. R., Ancellet, G., Barret, B., Boynard, A., Burrows, J. P., Clerbaux, C., Coheur, P.-F., Cuesta, J., Cuevas, E., Doniki, S., Dufour, G., Ebojie, F., Foret, G., Garcia, O., Granados-Muñoz, M. J., Hannigan, J. W., Hase, F., Hassler, B., Huang, G., Hurtmans, D., Jaffe, D., Jones, N., Kalabokas, P., Kerridge, B., Kulawik, S., Latter, B., Leblanc, T., Le Flochmoën, E., Lin, W., Liu, J., Liu, X., Mahieu, E., McClure-Begley, A., Neu, J. L., Osman, M., Palm, M., Petetin, H., Petropavlovskikh, I., Querel, R., Rahpoe, N., Rozanov, A., Schultz, M. G., Schwab, J., Siddans, R., Smale, D., Steinbacher, M., Tanimoto, H., Tarasick, D. W., Thouret, V., Thompson, A. M., Trickl, T., Weatherhead, E., Wespes, C., Worden, H. M., Vigouroux, C., Xu, X., Zeng, G., and Ziemke, J.: Tropospheric Ozone Assessment Report: Present-day distribution and trends of tropospheric ozone relevant to climate and global atmospheric chemistry model evaluation, Elem. Sci. Anthr., 6, 39, https://doi.org/10.1525/elementa.291, 2018.
Gaveau, D. L. A., Salim, M. A., Hergoualc'h, K., Locatelli, B., Sloan, S., Wooster, M., Marlier, M. E., Molidena, E., Yaen, H., DeFries, R., Verchot, L., Murdiyarso, D., Nasi, R., Holmgren, P., and Sheil, D.: Major atmospheric emissions from peat fires in Southeast Asia during non-drought years: evidence from the 2013 Sumatran fires, Sci. Rep.-UK, 4, 6112, https://doi.org/10.1038/srep06112, 2014.
Griffiths, P. T., Murray, L. T., Zeng, G., Shin, Y. M., Abraham, N. L., Archibald, A. T., Deushi, M., Emmons, L. K., Galbally, I. E., Hassler, B., Horowitz, L. W., Keeble, J., Liu, J., Moeini, O., Naik, V., O'Connor, F. M., Oshima, N., Tarasick, D., Tilmes, S., Turnock, S. T., Wild, O., Young, P. J., and Zanis, P.: Tropospheric ozone in CMIP6 simulations, Atmos. Chem. Phys., 21, 4187–4218, https://doi.org/10.5194/acp-21-4187-2021, 2021.
Guenther, A. B., Jiang, X., Heald, C. L., Sakulyanontvittaya, T., Duhl, T., Emmons, L. K., and Wang, X.: The Model of Emissions of Gases and Aerosols from Nature version 2.1 (MEGAN2.1): an extended and updated framework for modeling biogenic emissions, Geosci. Model Dev., 5, 1471–1492, https://doi.org/10.5194/gmd-5-1471-2012, 2012.
Hardacre, C., Wild, O., and Emberson, L.: An evaluation of ozone dry deposition in global scale chemistry climate models, Atmos. Chem. Phys., 15, 6419–6436, https://doi.org/10.5194/acp-15-6419-2015, 2015.
Hayes, F., Harmens, H., Sharps, K., and Radbourne, A.: Ozone dose-response relationships for tropical crops reveal potential threat to legume and wheat production, but not to millets, Sci. African, 9, e00482, https://doi.org/10.1016/j.sciaf.2020.e00482, 2020.
Hoesly, R. M., Smith, S. J., Feng, L., Klimont, Z., Janssens-Maenhout, G., Pitkanen, T., Seibert, J. J., Vu, L., Andres, R. J., Bolt, R. M., Bond, T. C., Dawidowski, L., Kholod, N., Kurokawa, J.-I., Li, M., Liu, L., Lu, Z., Moura, M. C. P., O'Rourke, P. R., and Zhang, Q.: Historical (1750–2014) anthropogenic emissions of reactive gases and aerosols from the Community Emissions Data System (CEDS), Geosci. Model Dev., 11, 369–408, https://doi.org/10.5194/gmd-11-369-2018, 2018.
Horowitz, L. W., Fiore, A. M., Milly, G. P., Cohen, R. C., Perring, A., Wooldridge, P. J., Hess, P. G., Emmons, L. K., and Lamarque, J.-F.: Observational constraints on the chemistry of isoprene nitrates over the eastern United States, J. Geophys. Res.-Atmos., 112, D12, https://doi.org/10.1029/2006JD007747, 2007.
Ivatt, P. D., Evans, M. J., and Lewis, A. C.: Suppression of surface ozone by an aerosol-inhibited photochemical ozone regime, Nat. Geosci., 15, 536–540, https://doi.org/10.1038/s41561-022-00972-9, 2022.
Jaeglé, L., Steinberger, L., V. Martin, R., and Chance, K.: Global partitioning of NO x sources using satellite observations: Relative roles of fossil fuel combustion, biomass burning and soil emissions, Faraday Discuss., 130, 407–423, https://doi.org/10.1039/B502128F, 2005.
Jaffe, D. A. and Wigder, N. L.: Ozone production from wildfires: A critical review, Atmos. Environ., 51, 1–10, https://doi.org/10.1016/J.ATMOSENV.2011.11.063, 2012.
Jin, X., Zhu, Q., and Cohen, R. C.: Direct estimates of biomass burning NOx emissions and lifetimes using daily observations from TROPOMI, Atmos. Chem. Phys., 21, 15569–15587, https://doi.org/10.5194/acp-21-15569-2021, 2021.
Kittipornkul, P., Thiravetyan, P., Hoshika, Y., Sorrentino, B., Popa, I., Leca, S., Sicard, P., Paoletti, E., and De Marco, A.: Surface ozone risk to human health and vegetation in tropical region: The case of Thailand, Environ. Res., 234, 116566, https://doi.org/10.1016/j.envres.2023.116566, 2023.
Klingberg, J., Karlsson, P. E., Pihl Karlsson, G., Hu, Y., Chen, D., and Pleijel, H.: Variation in ozone exposure in the landscape of southern Sweden with consideration of topography and coastal climate, Atmos. Environ., 47, 252–260, https://doi.org/10.1016/j.atmosenv.2011.11.004, 2012.
Klumpp, A., Ansel, W., Klumpp, G., Calatayud, V., Pierre Garrec, J., He, S., Peñuelas, J., Ribas, À., Ro-Poulsen, H., Rasmussen, S., Sanz, M. J., and Vergne, P.: Ozone pollution and ozone biomonitoring in European cities. Part I: Ozone concentrations and cumulative exposure indices at urban and suburban sites, Atmos. Environ., 40, 7963–7974, https://doi.org/10.1016/j.atmosenv.2006.07.017, 2006.
Komala, N., Saraspriya, S., Kita, K., and Ogawa, T.: Tropospheric ozone behavior observed in Indonesia, Atmos. Environ., 30, 1851–1856, https://doi.org/10.1016/1352-2310(95)00382-7, 1996.
Lee, L. A., Pringle, K. J., Reddington, C. L., Mann, G. W., Stier, P., Spracklen, D. V., Pierce, J. R., and Carslaw, K. S.: The magnitude and causes of uncertainty in global model simulations of cloud condensation nuclei, Atmos. Chem. Phys., 13, 8879–8914, https://doi.org/10.5194/acp-13-8879-2013, 2013.
Lefohn, A. S., Malley, C. S., Smith, L., Wells, B., Hazucha, M., Simon, H., Naik, V., Mills, G., Schultz, M. G., Paoletti, E., De Marco, A., Xu, X., Zhang, L., Wang, T., Neufeld, H. S., Musselman, R. C., Tarasick, D., Brauer, M., Feng, Z., Tang, H., Kobayashi, K., Sicard, P., Solberg, S., and Gerosa, G.: Tropospheric ozone assessment report: Global ozone metrics for climate change, human health, and crop/ecosystem research, Elem. Sci. Anthr., 6, 27, https://doi.org/10.1525/elementa.279, 2018.
Leung, F.-Y. T., Logan, J. A., Park, R., Hyer, E., Kasischke, E., Streets, D., and Yurganov, L.: Impacts of enhanced biomass burning in the boreal forests in 1998 on tropospheric chemistry and the sensitivity of model results to the injection height of emissions, J. Geophys. Res.-Atmos., 112, D10, https://doi.org/10.1029/2006JD008132, 2007.
Liddell, M. J., Nieullet, N., Campoe, O. C., and Freiberg, M.: Assessing the above-ground biomass of a complex tropical rainforest using a canopy crane, Austral Ecol., 32, 43–58, https://doi.org/10.1111/j.1442-9993.2007.01736.x, 2007.
Liu, Z., Doherty, R. M., Wild, O., O'Connor, F. M., and Turnock, S. T.: Tropospheric ozone changes and ozone sensitivity from the present day to the future under shared socio-economic pathways, Atmos. Chem. Phys., 22, 1209–1227, https://doi.org/10.5194/acp-22-1209-2022, 2022.
Luhar, A. K., Woodhouse, M. T., and Galbally, I. E.: A revised global ozone dry deposition estimate based on a new two-layer parameterisation for air–sea exchange and the multi-year MACC composition reanalysis, Atmos. Chem. Phys., 18, 4329–4348, https://doi.org/10.5194/acp-18-4329-2018, 2018.
Meinshausen, M., Vogel, E., Nauels, A., Lorbacher, K., Meinshausen, N., Etheridge, D. M., Fraser, P. J., Montzka, S. A., Rayner, P. J., Trudinger, C. M., Krummel, P. B., Beyerle, U., Canadell, J. G., Daniel, J. S., Enting, I. G., Law, R. M., Lunder, C. R., O'Doherty, S., Prinn, R. G., Reimann, S., Rubino, M., Velders, G. J. M., Vollmer, M. K., Wang, R. H. J., and Weiss, R.: Historical greenhouse gas concentrations for climate modelling (CMIP6), Geosci. Model Dev., 10, 2057–2116, https://doi.org/10.5194/gmd-10-2057-2017, 2017.
Mulcahy, J. P., Jones, C., Sellar, A., Johnson, B., Boutle, I. A., Jones, A., Andrews, T., Rumbold, S. T., Mollard, J., Bellouin, N., Johnson, C. E., Williams, K. D., Grosvenor, D. P., and Mccoy, D. T.: Improved Aerosol Processes and Effective Radiative Forcing in HadGEM3 and UKESM1, J. Adv. Model. Earth Sy., 10, 2786–2805, https://doi.org/10.1029/2018MS001464, 2018.
Mulcahy, J. P., Johnson, C., Jones, C. G., Povey, A. C., Scott, C. E., Sellar, A., Turnock, S. T., Woodhouse, M. T., Abraham, N. L., Andrews, M. B., Bellouin, N., Browse, J., Carslaw, K. S., Dalvi, M., Folberth, G. A., Glover, M., Grosvenor, D. P., Hardacre, C., Hill, R., Johnson, B., Jones, A., Kipling, Z., Mann, G., Mollard, J., O'Connor, F. M., Palmiéri, J., Reddington, C., Rumbold, S. T., Richardson, M., Schutgens, N. A. J., Stier, P., Stringer, M., Tang, Y., Walton, J., Woodward, S., and Yool, A.: Description and evaluation of aerosol in UKESM1 and HadGEM3-GC3.1 CMIP6 historical simulations, Geosci. Model Dev., 13, 6383–6423, https://doi.org/10.5194/gmd-13-6383-2020, 2020.
Nakada, L. Y. K. and Urban, R. C.: COVID-19 pandemic: Impacts on the air quality during the partial lockdown in São Paulo state, Brazil, Sci. Total Environ., 730, 139087, https://doi.org/10.1016/j.scitotenv.2020.139087, 2020.
Nascimento, J. P., Barbosa, H. M. J., Banducci, A. L., Rizzo, L. V., Vara-Vela, A. L., Meller, B. B., Gomes, H., Cezar, A., Franco, M. A., Ponczek, M., Wolff, S., Bela, M. M., and Artaxo, P.: Major Regional-Scale Production of O3 and Secondary Organic Aerosol in Remote Amazon Regions from the Dynamics and Photochemistry of Urban and Forest Emissions, Environ. Sci. Technol., 56, 9924–9935, https://doi.org/10.1021/acs.est.2c01358, 2022.
Nassar, R., Logan, J. A., Megretskaia, I. A., Murray, L. T., Zhang, L., and Jones, D. B. A.: Analysis of tropical tropospheric ozone, carbon monoxide, and water vapor during the 2006 El Niño using TES observations and the GEOS-Chem model, J. Geophys. Res.-Atmos., 114, 2009JD011760, https://doi.org/10.1029/2009JD011760, 2009.
Neal, L. S., Dalvi, M., Folberth, G., McInnes, R. N., Agnew, P., O'Connor, F. M., Savage, N. H., and Tilbee, M.: A description and evaluation of an air quality model nested within global and regional composition-climate models using MetUM, Geosci. Model Dev., 10, 3941–3962, https://doi.org/10.5194/gmd-10-3941-2017, 2017.
NTEPA: Northern Territory Enviroment Protection Authority, Station Report [data set] http://ntepa.webhop.net/NTEPA/ (last access: September 2023), 2021.
O'Connor, F. M., Johnson, C. E., Morgenstern, O., Abraham, N. L., Braesicke, P., Dalvi, M., Folberth, G. A., Sanderson, M. G., Telford, P. J., Voulgarakis, A., Young, P. J., Zeng, G., Collins, W. J., and Pyle, J. A.: Evaluation of the new UKCA climate-composition model – Part 2: The Troposphere, Geosci. Model Dev., 7, 41–91, https://doi.org/10.5194/gmd-7-41-2014, 2014.
Pacifico, F., Harrison, S. P., Jones, C. D., Arneth, A., Sitch, S., Weedon, G. P., Barkley, M. P., Palmer, P. I., Serça, D., Potosnak, M., Fu, T.-M., Goldstein, A., Bai, J., and Schurgers, G.: Evaluation of a photosynthesis-based biogenic isoprene emission scheme in JULES and simulation of isoprene emissions under present-day climate conditions, Atmos. Chem. Phys., 11, 4371–4389, https://doi.org/10.5194/acp-11-4371-2011, 2011.
Pacifico, F., Folberth, G. A., Jones, C. D., Harrison, S. P., and Collins, W. J.: Sensitivity of biogenic isoprene emissions to past, present, and future environmental conditions and implications for atmospheric chemistry, J. Geophys. Res.-Atmos., 117, D22302, https://doi.org/10.1029/2012JD018276, 2012.
Pacifico, F., Folberth, G. A., Sitch, S., Haywood, J. M., Rizzo, L. V., Malavelle, F. F., and Artaxo, P.: Biomass burning related ozone damage on vegetation over the Amazon forest: a model sensitivity study, Atmos. Chem. Phys., 15, 2791–2804, https://doi.org/10.5194/acp-15-2791-2015, 2015.
Permadi, D. A. and Oanh, N. T. K.: Evaluation of regional simulation of surface ozone over Southeast Asia using ground-based observation at two existing Global Atmospheric Watch stations, IOP C. Ser. Earth Env., 880, 012006, https://doi.org/10.1088/1755-1315/880/1/012006, 2021.
Pfister, G. G., Emmons, L. K., Hess, P. G., Honrath, R., Lamarque, J.-F., Val Martin, M., Owen, R. C., Avery, M. A., Browell, E. V., Holloway, J. S., Nedelec, P., Purvis, R., Ryerson, T. B., Sachse, G. W., and Schlager, H.: Ozone production from the 2004 North American boreal fires, J. Geophys. Res.-Atmos., 111, D24S07, https://doi.org/10.1029/2006JD007695, 2006.
Piikki, K., Klingberg, J., Pihl Karlsson, G., Karlsson, P. E., and Pleijel, H.: Estimates of AOT ozone indices from time-integrated ozone data and hourly air temperature measurements in southwest Sweden, Environ. Pollut., 157, 3051–3058, https://doi.org/10.1016/j.envpol.2009.05.038, 2009.
Pope, R. J., Arnold, S. R., Chipperfield, M. P., Reddington, C. L. S., Butt, E. W., Keslake, T. D., Feng, W., Latter, B. G., Kerridge, B. J., Siddans, R., Rizzo, L., Artaxo, P., Sadiq, M., and Tai, A. P. K.: Substantial Increases in Eastern Amazon and Cerrado Biomass Burning-Sourced Tropospheric Ozone, Geophys. Res. Lett., 47, e2019GL084143, https://doi.org/10.1029/2019GL084143, 2020.
Price, C. and Rind, D.: A Simple Lightning Parameterization for Calculating Global Lightning Distributions, J. Geophys. Res., 97, 9919–9933, https://doi.org/10.1029/92JD00719, 1992.
Sarwar, G., Kang, D., Foley, K., Schwede, D., Gantt, B., and Mathur, R.: Technical note: Examining ozone deposition over seawater, Atmos. Environ., 141, 255–262, https://doi.org/10.1016/j.atmosenv.2016.06.072, 2016.
Schröder, S., Schultz, M. G., Selke, N., Sun, J., Ahring, J., Mozaffari, A. Romberg, M., Epp, E., Lensing, M., Apweiler, S., Leufen, L. H., Betancourt, C., Hagemeier, B., and Rajveer, S.: TOAR Data Infrastructure, FZ-Juelich B2SHARE, UEDAT [data set], https://doi.org/10.34730/4D9A287DEC0B42F1AA6D244, 2021.
Schultz, M. G., Heil, A., Hoelzemann, J. J., Spessa, A., Thonicke, K., Goldammer, J. G., Held, A. C., Pereira, J. M. C., and van het Bolscher, M.: Global wildland fire emissions from 1960 to 2000, Global Biogeochem. Cy., 22, 2, https://doi.org/10.1029/2007GB003031, 2008
Schultz, M. G., Schröder, S., Lyapina, O., Cooper, O. R., Galbally, I., Petropavlovskikh, I., von Schneidemesser, E., Tanimoto, H., Elshorbany, Y., Naja, M., Seguel, R. J., Dauert, U., Eckhardt, P., Feigenspan, S., Fiebig, M., Hjellbrekke, A.-G., Hong, Y.-D., Christian Kjeld, P., Koide, H., Lear, G., Tarasick, D., Ueno, M., Wallasch, M., Baumgardner, D., Chuang, M.-T., Gillett, R., Lee, M., Molloy, S., Moolla, R., Wang, T., Sharps, K., Adame, J. A., Ancellet, G., Apadula, F., Artaxo, P., Barlasina, M. E., Bogucka, M., Bonasoni, P., Chang, L., Colomb, A., Cuevas, E., Parrish, D., Read, K. A., Reid, N., Ries, L., Saxena, P., Schwab, J. J., Scorgie, Y., Senik, I., Simmonds, P., Sinha, V., Skorokhod, A. I., Spain, G., Spangl, W., Spoor, R., Springston, S. R., Steer, K., Steinbacher, M., Suharguniyawan, E., Torre, P., Trickl, T., Weili, L., Weller, R., Xiaobin, X., Xue, L., Zhiqiang, M., and Henriques, D.: Tropospheric Ozone Assessment Report: Database and metrics data of global surface ozone observations, Elem. Sci. Anthr., 34, 43, https://doi.org/10.1525/elementa.244, 2017.
Schutgens, N., Tsyro, S., Gryspeerdt, E., Goto, D., Weigum, N., Schulz, M., and Stier, P.: On the spatio-temporal representativeness of observations, Atmos. Chem. Phys., 17, 9761–9780, https://doi.org/10.5194/acp-17-9761-2017, 2017.
Schwantes, R. H., Emmons, L. K., Orlando, J. J., Barth, M. C., Tyndall, G. S., Hall, S. R., Ullmann, K., St. Clair, J. M., Blake, D. R., Wisthaler, A., and Bui, T. P. V.: Comprehensive isoprene and terpene gas-phase chemistry improves simulated surface ozone in the southeastern US, Atmos. Chem. Phys., 20, 3739–3776, https://doi.org/10.5194/acp-20-3739-2020, 2020.
Seguel, R. J., Castillo, L., Opazo, C., Rojas, N. Y., Nogueira, T., Cazorla, M., Gavidia-Calderón, M., Gallardo, L., Garreaud, R., Carrasco-Escaff, T., and Elshorbany, Y.: Changes in South American surface ozone trends: exploring the influences of precursors and extreme events, Atmos. Chem. Phys., 24, 8225–8242, https://doi.org/10.5194/acp-24-8225-2024, 2024.
Sellar, A. A., Jones, C. G., Mulcahy, J. P., Tang, Y., Yool, A., Wiltshire, A., O'Conner, F. M., Stringer, M., Hill, R., Palmieri, J., Woodward, S., de Mora, L., Kuhlbrodt, T., Rumbold, S. T., Kelley, D. I., Ellis, R., Johnson, C. E., Walton, J., Luke Abraham, N., Andrews, M. B., Andrews, T., Archibald, A. T., Berthou, S., Burke, E., Blockley, E., Carslaw, K., Dalvi, M., Edwards, J., Folberth, G. A., Gedney, N., Griffiths, P. T., Harper, A. B., Hendry, M. A., Hewitt, A. J., Johnson, B., Jones, A., Jones, C. D., Keeble, J., Liddicoat, S., Morgenstern, O., Parker, R. J., Predoi, V., Robertson, E., Siahaan, A., Smith, R. S., Swaminathan, R., Woodhouse, M. T., Zeng, G., and Zerroukat, M.: UKESM1: Description and Evaluation of the U. K. Earth System Model Special Section: The UK Earth System Models for CMIP6, J. Adv. Model. Earth Sy., 11, 4513–4558, https://doi.org/10.1029/2019MS001739, 2019.
Sibret, T., Bauters, M., Bulonza, E., Lefevre, L., Cerutti, P. O., Lokonda, M., Mbifo, J., Michel, B., Verbeeck, H., and Boeckx, P.: CongoFlux: the first eddy covariance flux tower in the Congo Basin, Front. Soil Sci., 2, 883236, https://doi.org/10.3389/fsoil.2022.883236, 2022.
Sicard, P., Agathokleous, E., Anenberg, S. C., De Marco, A., Paoletti, E., and Calatayud, V.: Trends in urban air pollution over the last two decades: A global perspective, Sci. Total Environ., 858, 160064, https://doi.org/10.1016/j.scitotenv.2022.160064, 2023.
Sindelarova, K., Granier, C., Bouarar, I., Guenther, A., Tilmes, S., Stavrakou, T., Müller, J.-F., Kuhn, U., Stefani, P., and Knorr, W.: Global data set of biogenic VOC emissions calculated by the MEGAN model over the last 30 years, Atmos. Chem. Phys., 14, 9317–9341, https://doi.org/10.5194/acp-14-9317-2014, 2014.
Sindelarova, K., Markova, J., Simpson, D., Huszar, P., Karlicky, J., Darras, S., and Granier, C.: High-resolution biogenic global emission inventory for the time period 2000–2019 for air quality modelling, Earth Syst. Sci. Data, 14, 251–270, https://doi.org/10.5194/essd-14-251-2022, 2022.
Sitch, S., Cox, P. M., Collins, W. J., and Huntingford, C.: Indirect radiative forcing of climate change through ozone effects on the land-carbon sink, Nature, 448, 791–794, https://doi.org/10.1038/nature06059, 2007.
Sofen, E. D., Bowdalo, D., Evans, M. J., Apadula, F., Bonasoni, P., Cupeiro, M., Ellul, R., Galbally, I. E., Girgzdiene, R., Luppo, S., Mimouni, M., Nahas, A. C., Saliba, M., and Tørseth, K.: Gridded global surface ozone metrics for atmospheric chemistry model evaluation, Earth Syst. Sci. Data, 8, 41–59, https://doi.org/10.5194/essd-8-41-2016, 2016a.
Sofen, E. D., Bowdalo, D., and Evans, M. J.: How to most effectively expand the global surface ozone observing network, Atmos. Chem. Phys., 16, 1445–1457, https://doi.org/10.5194/acp-16-1445-2016, 2016b.
Sörgel, M., Tsokankunku, A., Wolff, S., Araùjo, A., Assis, P., Harder, H., Martins, G., Sá, M., Souza, R., Williams, J., and Zannoni, N.: Quantifying deposition pathways of Ozone at a rainforest site (ATTO) in the central Amazon basin, EGU General Assembly 2020, Online, 4–8 May 2020, EGU2020-19455, https://doi.org/10.5194/egusphere-egu2020-19455, 2020.
Tang, Y., Rumbold, S., Ellis, R., Kelley, D., Mulcahy, J., Sellar, A., Walton, J., and Jones, C.: MOHC UKESM1.0-LL model output prepared for CMIP6 CMIP, ESGF [data set], https://doi.org/10.22033/ESGF/CMIP6.1569, 2019.
Turnock, S. T., Allen, R. J., Andrews, M., Bauer, S. E., Deushi, M., Emmons, L., Good, P., Horowitz, L., John, J. G., Michou, M., Nabat, P., Naik, V., Neubauer, D., O'Connor, F. M., Olivié, D., Oshima, N., Schulz, M., Sellar, A., Shim, S., Takemura, T., Tilmes, S., Tsigaridis, K., Wu, T., and Zhang, J.: Historical and future changes in air pollutants from CMIP6 models, Atmos. Chem. Phys., 20, 14547–14579, https://doi.org/10.5194/acp-20-14547-2020, 2020.
Turnock, S. T., Reddington, C. L., West, J. J., and O'Connor, F. M. The Air Pollution Human Health Burden in Different Future Scenarios That Involve the Mitigation of Near‐Term Climate Forcers, Climate and Land‐Use, GeoHealth, 7, e2023GH000812, https://doi.org/10.1029/2023GH000812, 2023.
van der A, R. J., Eskes, H. J., Boersma, K. F., van Noije, T. P. C., Van Roozendael, M., De Smedt, I., Peters, D. H. M. U., and Meijer, E. W.: Trends, seasonal variability and dominant NOx source derived from a ten year record of NO2 measured from space, J. Geophys. Res.-Atmos., 113, D04302, https://doi.org/10.1029/2007JD009021, 2008.
van der Werf, G. R., Randerson, J. T., Giglio, L., van Leeuwen, T. T., Chen, Y., Rogers, B. M., Mu, M., van Marle, M. J. E., Morton, D. C., Collatz, G. J., Yokelson, R. J., and Kasibhatla, P. S.: Global fire emissions estimates during 1997–2016, Earth Syst. Sci. Data, 9, 697–720, https://doi.org/10.5194/essd-9-697-2017, 2017.
van Marle, M. J. E., Kloster, S., Magi, B. I., Marlon, J. R., Daniau, A.-L., Field, R. D., Arneth, A., Forrest, M., Hantson, S., Kehrwald, N. M., Knorr, W., Lasslop, G., Li, F., Mangeon, S., Yue, C., Kaiser, J. W., and van der Werf, G. R.: Historic global biomass burning emissions for CMIP6 (BB4CMIP) based on merging satellite observations with proxies and fire models (1750–2015), Geosci. Model Dev., 10, 3329–3357, https://doi.org/10.5194/gmd-10-3329-2017, 2017.
Verma, S., Yadava, P. K., Lal, D. M., Mall, R. K., Kumar, H., and Payra, S.: Role of Lightning NOx in Ozone Formation: A Review, Pure Appl. Geophys., 178, 1425–1443, https://doi.org/10.1007/s00024-021-02710-5, 2021.
Vieira, I., Verbeeck, H., Meunier, F., Peaucelle, M., Sibret, T., Lefevre, L., Cheesman, A. W., Brown, F., Sitch, S., Mbifo, J., Boeckx, P., and Bauters, M.: Global reanalysis products cannot reproduce seasonal and diurnal cycles of tropospheric ozone in the Congo Basin, Atmos. Environ., 304, 119773, https://doi.org/10.1016/j.atmosenv.2023.119773, 2023.
Weber, J., Archer-Nicholls, S., Abraham, N. L., Shin, Y. M., Bannan, T. J., Percival, C. J., Bacak, A., Artaxo, P., Jenkin, M., Khan, M. A. H., Shallcross, D. E., Schwantes, R. H., Williams, J., and Archibald, A. T.: Improvements to the representation of BVOC chemistry–climate interactions in UKCA (v11.5) with the CRI-Strat 2 mechanism: incorporation and evaluation, Geosci. Model Dev., 14, 5239–5268, https://doi.org/10.5194/gmd-14-5239-2021, 2021.
Weber, J., King, J. A., Sindelarova, K., and Val Martin, M.: Updated isoprene and terpene emission factors for the Interactive BVOC (iBVOC) emission scheme in the United Kingdom Earth System Model (UKESM1.0), Geosci. Model Dev., 16, 3083–3101, https://doi.org/10.5194/gmd-16-3083-2023, 2023.
Weng, H., Lin, J., Martin, R., Millet, D. B., Jaeglé, L., Ridley, D., Keller, C., Li, C., Du, M., and Meng, J.: Global high-resolution emissions of soil NOx, sea salt aerosols, and biogenic volatile organic compounds, Sci. Data, 7, 148, https://doi.org/10.1038/s41597-020-0488-5, 2020.
Wild, O. and Palmer, P. I.: How sensitive is tropospheric oxidation to anthropogenic emissions?, Geophys. Res. Lett., 35, 22, https://doi.org/10.1029/2008GL035718, 2008.
Wild, O. and Prather, M. J.: Global tropospheric ozone modeling: Quantifying errors due to grid resolution, J. Geophys. Res.-Atmos., 111, D11, https://doi.org/10.1029/2005JD006605, 2006.
Williams, K. D., Copsey, D., Blockley, E. W., Bodas-Salcedo, A., Calvert, D., Comer, R., Davis, P., Graham, T., Hewitt, H. T., Hill, R., Hyder, P., Ineson, S., Johns, T. C., Keen, A. B., Lee, R. W., Megann, A., Milton, S. F., Rae, J. G. L., Roberts, M. J., Scaife, A. A., Schiemann, R., Storkey, D., Thorpe, L., Watterson, I. G., Walters, D. N., West, A., Wood, R. A., Woollings, T., and Xavier, P. K.: The Met Office Global Coupled Model 3.0 and 3.1 (GC3.0 and GC3.1) Configurations, J. Adv. Model. Earth Sy., 10, 357–380, https://doi.org/10.1002/2017MS001115, 2018.
Yáñez-Serrano, A. M., Nölscher, A. C., Williams, J., Wolff, S., Alves, E., Martins, G. A., Bourtsoukidis, E., Brito, J., Jardine, K., Artaxo, P., and Kesselmeier, J.: Diel and seasonal changes of biogenic volatile organic compounds within and above an Amazonian rainforest, Atmos. Chem. Phys., 15, 3359–3378, https://doi.org/10.5194/acp-15-3359-2015, 2015.
Yienger, J. J. and Levy II, H.: Empirical model of global soil-biogenic NOχ emissions, J. Geophys. Res.-Atmos., 100, 11447–11464, https://doi.org/10.1029/95JD00370, 1995.
Young, P. J., Archibald, A. T., Bowman, K. W., Lamarque, J.-F., Naik, V., Stevenson, D. S., Tilmes, S., Voulgarakis, A., Wild, O., Bergmann, D., Cameron-Smith, P., Cionni, I., Collins, W. J., Dalsøren, S. B., Doherty, R. M., Eyring, V., Faluvegi, G., Horowitz, L. W., Josse, B., Lee, Y. H., MacKenzie, I. A., Nagashima, T., Plummer, D. A., Righi, M., Rumbold, S. T., Skeie, R. B., Shindell, D. T., Strode, S. A., Sudo, K., Szopa, S., and Zeng, G.: Pre-industrial to end 21st century projections of tropospheric ozone from the Atmospheric Chemistry and Climate Model Intercomparison Project (ACCMIP), Atmos. Chem. Phys., 13, 2063–2090, https://doi.org/10.5194/acp-13-2063-2013, 2013.
Young, P. J., Naik, V., Fiore, A. M., Gaudel, A., Guo, J., Lin, M. Y., Neu, J. L., Parrish, D. D., Rieder, H. E., Schnell, J. L., Tilmes, S., Wild, O., Zhang, L., Ziemke, J., Brandt, J., Delcloo, A., Doherty, R. M., Geels, C., Hegglin, M. I., Hu, L., Im, U., Kumar, R., Luhar, A., Murray, L., Plummer, D., Rodriguez, J., Saiz-Lopez, A., Schultz, M. G., Woodhouse, M. T., and Zeng, G.: Tropospheric Ozone Assessment Report: Assessment of global-scale model performance for global and regional ozone distributions, variability, and trends, Elem. Sci. Anthr., 6, 10, https://doi.org/10.1525/elementa.265, 2018.