the Creative Commons Attribution 4.0 License.
the Creative Commons Attribution 4.0 License.
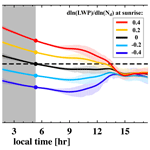
Cloud water adjustments to aerosol perturbations are buffered by solar heating in non-precipitating marine stratocumuli
Yao-Sheng Chen
Takanobu Yamaguchi
Graham Feingold
Marine low-level clouds are key to the Earth's energy budget due to their expansive coverage over global oceans and their high reflectance of incoming solar radiation. Their responses to anthropogenic aerosol perturbations remain the largest source of uncertainty in estimating the anthropogenic radiative forcing of climate. A major challenge is the quantification of the cloud water response to aerosol perturbations. In particular, the presence of feedbacks through microphysical, dynamical, and thermodynamical pathways at various spatial and temporal scales could augment or weaken the response. Central to this problem is the temporal evolution in cloud adjustment, governed by entangled feedback mechanisms. We apply an innovative conditional Monte Carlo subsampling approach to a large ensemble of diurnal large-eddy simulation of non-precipitating marine stratocumulus to study the role of solar heating in governing the evolution in the relationship between droplet number and cloud water. We find a persistent negative trend in this relationship at night, confirming that the role of microphysically enhanced cloud-top entrainment. After sunrise, the evolution in this relationship appears buffered and converges to in the late afternoon. This buffering effect is attributed to a strong dependence of cloud-layer shortwave absorption on cloud liquid water path. These diurnal cycle characteristics further demonstrate a tight connection between cloud brightening potential and the relationship between cloud water and droplet number at sunrise, which has implications for the impact of the timing of advertent aerosol perturbations.
- Article
(3097 KB) - Full-text XML
-
Supplement
(3174 KB) - BibTeX
- EndNote
Marine stratocumulus (Sc) clouds, found ubiquitously over subtropical oceans, are key to the Earth's radiation budget (Wood, 2012). They cool the Earth effectively through reflecting a considerable amount of incoming solar radiation (Bender et al., 2011; Stephens et al., 2012). The radiative effect of marine stratocumulus is governed by its macrophysical properties, such as areal coverage and liquid water path (LWP), and microphysical properties, such as cloud droplet number concentration (Nd) or drop size. Increases in atmospheric aerosol particles lead to an increase in smaller cloud droplets (Twomey, 1974, 1977); modulate the rate of warm cloud processes, e.g., collision–coalescence and entrainment mixing; and subsequently cause adjustments in cloud macrophysical properties (e.g., Albrecht, 1989; Bretherton et al., 2007; Wang et al., 2003; Xue and Feingold, 2006). The radiative forcing attributed to cloud adjustments in response to anthropogenic aerosol increases is currently poorly constrained, especially for marine boundary layer clouds, and remains the largest source of uncertainty in projections of the future climate (Boucher et al., 2013; Forster et al., 2021; Bellouin et al., 2020).
A key, yet uncertain, component of these cloud adjustments is the response of cloud water to aerosol perturbations. Constraining it is particularly challenging because the impact of aerosol on cloud LWP is bidirectional and regime-dependent (Chen et al., 2014; Gryspeerdt et al., 2019; Possner et al., 2020; Toll et al., 2019). For precipitating clouds, an increase in aerosol tends to increase LWP through precipitation suppression (Albrecht, 1989), whereas for non-precipitating clouds, LWP decreases through enhanced turbulent entrainment of dry, free-tropospheric (FT) air at cloud top, attributed to smaller droplets (Bretherton et al., 2007; Wang et al., 2003). Thus, the frequency of occurrence of different cloud states governs the overall response of cloud water to aerosol perturbations, which depends strongly on large-scale meteorological conditions (e.g., Zhang et al., 2022; Zhou et al., 2021; Zhang and Feingold, 2023).
Making the quantification of the LWP adjustment to aerosol perturbations even more challenging is the presence of feedbacks among system-wide microphysical, dynamical, and thermodynamical processes at different spatiotemporal scales, acting to buffer the system's response to perturbations (Stevens and Feingold, 2009). Quantifying aerosol effects on LWP in such a buffered system requires understanding not only of individual causal mechanisms but also of their timescales (Glassmeier et al., 2021; Fons et al., 2023; Gryspeerdt et al., 2022). Therefore, characterizing the temporal evolution of cloud adjustments is central to this problem, as it provides a way to assess the relative importance of individual mechanisms. Based on an ensemble of nocturnal large-eddy simulations (LESs) of marine stratocumulus, Glassmeier et al. (2021) suggested that the estimated cooling effect due to aerosol–cloud interactions derived from ship-track observations may be an overestimation if the temporal evolution in cloud water adjustment is not taken into account. Using satellite observations, Gryspeerdt et al. (2021) showed that the Nd–LWP relationship between ship tracks and their surroundings is indeed time-dependent and sensitive to the cloud and meteorological states under which the aerosol perturbation occurs. More generally, studies using geostationary satellites (e.g., Qiu et al., 2024; Christensen et al., 2023; Smalley et al., 2024) and polar-orbiting satellites (e.g., Diamond et al., 2020; Zhang and Feingold, 2023) have indicated diurnal variation in cloud adjustments to aerosol perturbations, such that LWP adjustments become more negative in the afternoon. Through extrapolating the Terra (late morning) and Aqua (early afternoon) difference, Gryspeerdt et al. (2022) demonstrated the importance of controlling initial cloud states to account for feedbacks in the system and found a negative, but weaker, Nd–LWP relationship when feedbacks are accounted for.
When it comes to the attribution of the diurnal variation in cloud adjustment to aerosol perturbations, an often overlooked, yet important, process is the shortwave (SW) absorption in the cloud layer. The balance between SW heating and longwave (LW) cooling plays a crucial role in governing the daytime evolution of cloud water in marine stratocumulus (e.g., Sandu et al., 2008; Chen et al., 2024). Since cloud SW absorption is a strong function of LWP and also dependent on Nd (Petters et al., 2012), it can potentially act as an important feedback (or “buffering”, in the case of a negative feedback) mechanism as cloud water changes throughout the sunlit hours.
In this study, we aim to characterize the diurnal evolution in cloud water adjustments to aerosol perturbations, with a particular focus on understanding the importance of SW absorption in affecting this evolution. We have performed a large ensemble of diurnal simulations of non-precipitating marine stratocumulus that represents conditions in the northeastern Pacific region, using an LES model that resolves aerosol–cloud interactions. By applying a novel subsampling approach (introduced in Sect. 2), we find that cloud SW absorption acts to flatten the Nd–LWP relationship (indicated by the regression slope) after sunrise, suggesting a buffered evolution in cloud water response to aerosol perturbations (Sect. 3.1). Enlightened by these results, we further use the subsampling approach to demonstrate a tight connection between the potential for cloud brightening and the cloud water–droplet number relationship at sunrise (Sect. 3.2). This has implications for the optimal timing of deliberate aerosol perturbations in the context of marine cloud brightening (MCB), one of the proposed climate intervention approaches (National Academies of Sciences, Engineering, and Medicine (NASEM) report, 2021; Latham and Smith, 1990; Latham et al., 2012), to the extent that they are constrained by the duration and the prescribed, time-invariant large-scale conditions of these simulations.
While process-model-based perturbation experiments help a great deal in understanding the causal mechanisms driving cloud adjustments to aerosol perturbations (e.g., Prabhakaran et al., 2023, 2024; Chun et al., 2023), these studies are typically limited in their ability to represent the range of boundary layer conditions observed in nature. A new approach in the most recent decade suggests that one can infer process-level understanding from the systematic behavior of simulation ensemble(s) that depict the evolution of cloud systems from a wide range of initial boundary layer conditions (e.g., Glassmeier et al., 2019, 2021; Hoffmann et al., 2020, 2023), as a way to bridge “Newtonian” (bottom-up) and “Darwinian” (top-down) approaches (Feingold et al., 2016; Mülmenstädt and Feingold, 2018). Following this methodology, we analyze a large ensemble of diurnal simulations of marine stratocumulus with an innovative subsampling approach, in which the large ensemble is sub-grouped into smaller ensembles as a means to investigate the impact of Nd on cloud water evolution and how it is mediated by SW heating.
2.1 Large-eddy simulation ensemble of marine stratocumulus
All simulations used in this study are carried out with the System for Atmospheric Modeling (SAM; Khairoutdinov and Randal, 2003). The model domain size is set to km3 with a horizontal and vertical grid spacing of 200 and 10 m, respectively. This setup allows for development of mesoscale organizations (Kazil et al., 2017) while keeping computational cost affordable for a large ensemble of simulations. All simulations are run for 24 h from 18:40 local time right after sunset at a time step of 1 s. Cloud microphysical processes are simulated with a two-moment, bin-emulating bulk microphysical scheme (Feingold et al., 1998) with prognostic total number concentration and total water content (Yamaguchi et al., 2019). Aerosol number concentration (Na) is prescribed to be initially uniform throughout the domain, and we assume a lognormal aerosol size distribution (ammonium sulfate) with a geometric-mean diameter of 0.2 µm and geometric standard deviation of 1.5 µm, following Feingold et al. (2016). Aerosol particles are lost to cloud and precipitation processing, such as collision–coalescence, scavenging, and wet deposition, and we apply a constant surface flux of aerosol of 70 cm−2 s−1 (Yamaguchi et al., 2017; Kazil et al., 2011) to mitigate depletion of aerosol. Radiative heating rates are calculated interactively every 10 s using the Rapid Radiative Transfer Model (RRTMG; Clough et al., 2005) with extended thermodynamic profiles above the domain top (2.5 km), following Yamaguchi et al. (2017). Surface sensible and latent heat fluxes are calculated interactively based on Monin–Obukhov similarity and initialized with climatological mean surface winds. We prescribe a constant sea surface temperature (SST) of 292.4 K, based on the ERA5-derived climatology of large-scale meteorological conditions associated with the stratocumulus deck off the coast of California (Hersbach et al., 2020) and a fixed large-scale divergence of s−1 (Ackerman et al., 2009) for all simulations. The reader is referred to Chen et al. (2024) for more technical details on the setup of the simulations.
Keeping the above model setup and large-scale forcings the same for all simulations, we vary the initial conditions for boundary layer (BL) thermodynamics in a six-parameter variable space to create ensemble members, using a maximin Latin hypercube sampling approach (Morris and Mitchell, 1995) to minimize correlations between parameters, as described in Feingold et al. (2016) and Glassmeier et al. (2019). The six parameters include BL liquid water potential temperature ( K), the BL total water mixing ratio ( g kg−1), the jumps of temperature and humidity between BL and FT ( K and g kg−1), the initial mixed-layer depth ( m), and the aerosol number concentration ( mg−1). Using the Latin hypercube sampling approach, we generate hundreds of initial thermodynamic profiles, from which we carry out simulations whenever a cloud layer is produced and when the lifting condensation level is between 225 and 1075 m and the FT θl and qt profiles are within the ERA5 climatology of the northeastern Pacific. This yields a total of 316 diurnal simulations. Since we focus on the non-precipitating marine stratocumulus system, we impose a threshold of 0.5 mm d−1 on the cloud base rain rate to screen for non-precipitating simulations (Wood, 2012). We further exclude simulations that generate a surface fog, cloud tops higher than 2 km, and a domain cloud fraction (fc) of less than 0.01 (full cloud dissipation) to ensure the robustness of our analysis when the subsampling is applied. Domain-mean 2-dimensional and 3-dimensional outputs are saved every 2 min and every hour, respectively. A total of 204 non-precipitating simulations are selected for analysis. We discard the first 4 h of all simulations as model spin-up and use a cloud optical depth (τ) threshold of 1 to identify clouds. A higher threshold of τ=5 was tested but did not change the conclusions qualitatively.
2.2 A conditional Monte Carlo sampling approach
Many recent studies (e.g., Gryspeerdt et al., 2016, 2019; Glassmeier et al., 2021; Zhang et al., 2022; Zhou et al., 2021; Smalley et al., 2024) have chosen to infer the impact of aerosol on cloud properties by examining the spatiotemporal correlation between cloud macrophysical properties and Nd, with Nd serving as an intermediate variable, in order to mitigate the influence of confounding factors on the causal relationship between aerosol and clouds and to avoid uncertainties in relating aerosol information, such as aerosol optical depth and aerosol index, to cloud condensation nuclei (CCN; Stier, 2016). Here, we adopt the same methodology and focus on the relationship between Nd and LWP, quantified as the slope of linear regressions (e.g., McComiskey and Feingold, 2012). Least-squares log regressions are used to alleviate the dependence of the regression slope on the absolute value of Nd (e.g., Feingold et al., 2003; Zhang et al., 2022).
Nevertheless, covarying meteorological and aerosol conditions can still confound the Nd–LWP relationship in observations (e.g., Gryspeerdt et al., 2019) and in model simulations (e.g., Mülmenstädt et al., 2024). Therefore, we introduce a subsampling approach that can be conditioned on prescribed relationships among Nd, LWP, and initial boundary layer conditions, following the Monte Carlo methodology (Hammersley and Handscomb, 1964), with modifications to enable selection of specified conditions. We term this subsampling approach “conditional Monte Carlo (cMC).” The fundamental idea of employing the Monte Carlo concept is to use repetitive, semi-random (i.e., conditional) samplings to capture systematic behaviors (deterministic in principle) of stochastically initialized realizations of marine Sc evolution. The purpose of the cMC approach in this work is threefold. First, it serves to help constrain the covariation between Nd and meteorological conditions under which the simulations are initialized, which could confound the effect of Nd on LWP. Second, it serves as a means to free ourselves from dealing with an initially positive (after spin-up) Nd–LWP relationship imposed purely by the Latin hypercube sampling used to construct the initial boundary layer conditions. Third, we use it to select Nd–LWP relationships and observe their temporal evolution. In this work, we use statistical regression slopes to indicate the relationship (not necessarily causal) between two variables (e.g., Nd and LWP). The application of the cMC method alleviates the concern of whether statistical slopes can indicate causal relationships, as we focus on the evolution rather than the absolute value of these slopes by selecting a range of slopes at sunrise.
The cMC approach is applied as follows. We first randomly draw 25 simulations from the 204 LES ensemble members (non-precipitating), using a random seed generator assuming a normal distribution. The “conditional” part of cMC is implemented such that a drawing is saved only when the following conditions are met: first, the covariation between Nd and three boundary layer conditions (abbreviated as MET hereafter) at the beginning of the simulation (4 h) is smaller than user-imposed thresholds (i.e., minimizing the correlation between Nd and MET after spin-up). These three variables are cloud-top height (zct; a measure of boundary layer depth), surface sensible heat flux (SHF), and 800 hPa relative humidity (RH800), and the thresholds are , , and . Second, the Nd–LWP regression slope is close enough, within uncertainty ranges, to a user-prescribed value – essentially prescribing a cloud water–droplet number relationship for the randomly drawn 25 simulations. In our first investigation (Sect. 3.1), we prescribe five values for the Nd–LWP slope () at sunrise, ±0.4 (±0.02), ±0.2 (±0.01), and 0 (±0.005), to examine the role of SW heating. In our second investigation (Sect. 3.2), we prescribe flat slopes for Nd–LWP and Nd–fc, i.e., and , to mimic the relationship between cloud micro- and macrophysical properties at the time of aerosol perturbation, representing a difference in the timescale between the “instantaneous” (order of minutes) microphysical response and the slower (order of hours) macrophysical adjustments. In order to maintain practical sampling efficiency of the cMC approach while approximating desired regression slopes, we impose arbitrary bounding values (or thresholds) around the desired slopes without any threshold on the correlation coefficient between Nd and LWP. We note that our approach is not designed to select a narrow, linear band of points in ln (LWP)–ln (Nd) space but rather relies on the correlation between Nd–LWP to infer the relationship between them, given the relatively large number of samples in each sub-ensemble.
Within each 25-member subgroup of simulations, we calculate the slope between Nd and LWP as at each time step. We focus on the temporal evolution in , in particular on the difference between nighttime and sunlit hours (Sect. 3.1) and the impact of Nd–LWP relationship at sunrise on time-integrated cloud radiative effect (Sect. 3.2), rather than the absolute value of , which we prescribe when subsampling. The drawing is repeated with the same pre-conditions but different random number seeds to produce 50 25-member subgroups, and the mean evolution (averaged over 50 repetitions) is shown in the results. We also tested other configurations of the cMC setup, varying the number of members within each draw, number of draws, and the user-imposed thresholds. Different configurations yield the same conclusions, qualitatively, and the choice of the current configuration is based on sampling efficiency.
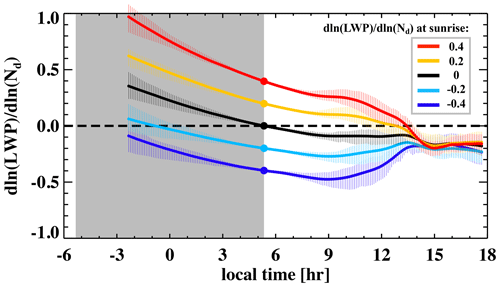
Figure 1Diurnal cycle of the Nd–LWP regression slope (). Solid lines indicate mean values of the 50 25-member cMC subsampling for individual groups, which are separated by colors representing different values at sunrise (large dots). Vertical bars indicate interquartile ranges for each group. A 1 h running mean is applied. Gray shading indicates nighttime hours.
3.1 The role of SW absorption in affecting diurnal evolution in the Nd–LWP relationship
3.1.1 A buffered evolution during the daytime
Besides the variations in Nd being a fundamental perturbation to the Sc system, the impact of solar heating on cloud water evolution starting from sunrise is another important perturbation to the system. During daytime, the sensitivity of radiation to cloud macro- and microphysical properties is critical to the evolution in the Nd–LWP slope. In particular, the dependence of cloud-layer LW cooling on LWP and Nd is only apparent in thin clouds and saturates at around 20 to 30 g m−2, whereas SW heating increases continuously as LWP and Nd increase, more pronouncedly with LWP (Petters et al., 2012). The different sensitivities of solar heating to LWP and Nd, which vary among LES ensemble members, are hypothesized to affect the daytime evolution in the Nd–LWP slope. In order to examine the effects of solar heating on the cloud water–droplet number relationship, using the cMC approach, we subsample five conditions where subsampled simulations possess prescribed Nd–LWP slopes at sunrise, ranging from −0.4 to 0.4 with an increment of 0.2 (Sect. 2.2). The diurnal evolution in the Nd–LWP slope (and correlation coefficient) of the five subgroups is shown in Fig. 1 (and Fig. S1 in the Supplement), with the red curve indicating the most positive (0.4) Nd–LWP slope at sunrise and the blue curve representing the most negative (−0.4) one. A persistent feature of the Nd–LWP slopes becoming more negative with time is observed at night, consistent with the findings in Glassmeier et al. (2021), regardless of the prescribed slopes at sunrise. This is attributed to the sensitivity of turbulent entrainment at cloud top to drop size, such that smaller drops (higher Nd) promote stronger entrainment. A sensitivity of the entrainment mechanism to LWP is also evident in the nighttime evolution where the decrease in the Nd–LWP slope for the group that starts with an initially positive Nd–LWP slope (higher Nd associated with higher LWP) is faster (from 1 to 0.4, red), compared to that in the group starting with a negative slope (from −0.1 to −0.4, blue; Fig. 1).
Interesting evolution in the Nd–LWP slope appears a couple of hours after sunrise where groups starting from very different Nd–LWP slopes at sunrise begin to converge (Fig. 1). The group convergence shares features typical to buffered evolution, such that the groups starting with a negative slope become less negative, whereas the groups starting with a positive slope become less positive over time. We will show that the cause of such a buffered evolution during the day is the primary dependence of SW heating on cloud LWP, such that thicker clouds (higher LWP) experience stronger cloud thinning with stronger SW absorption, whereas thinner clouds thin more slowly with weaker SW absorption, leading to flattening of all Nd–LWP slopes, regardless of their values at sunrise. For this task, we will need to quantify the rate of change in LWP attributed to radiative processes. Hence, we performed a budget analysis of the LWP tendency, following Chen et al. (2024), to further illustrate this attribution in the following.
3.1.2 The sensitivity of LWP tendency to Nd
The impact of Nd perturbations on cloud LWP is through affecting the rates of processes that govern the budget of cloud water. Here, we focus on two terms in this budget that are known to be sensitive to cloud water and droplet number, namely entrainment and radiation processes, derived as below, following Chen et al. (2024). First, the total rate of change of cloud LWP is written as
where ℒ denotes LWP, ′ denotes time derivatives, zcb is the mean cloud base height, zinv is the mean inversion base height, and Γl is the liquid water adiabatic lapse rate. We then decompose 〈qt〉′ and 〈θl〉′ into individual budget terms grouped by processes (), e.g., radiation (RAD) and entrainment (ENT). 〈ϕ〉 is the volume mean of a scalar quantity that represents either qt or θl in our case. In particular, is straightforwardly calculated from the 3-dimensional, modeled radiative heating rates, and is approximated by the difference between the total tendency of 〈ϕ〉 in the boundary layer (BL) and the sum of contributions from all processes other than ENT, which can be directly estimated from the modeled fields. The reader is referred to Chen et al. (2024) for more details on the derivation and justification of assumptions for the LWP tendency budget analysis.
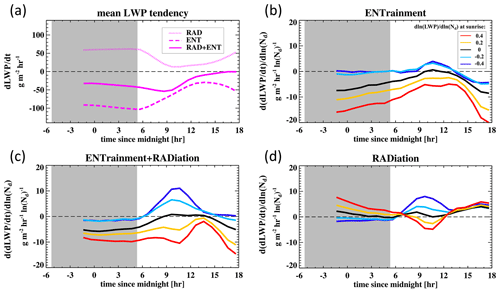
Figure 2Diurnal cycle of (a) mean LWP tendencies attributed to radiation (RAD), entrainment (ENT), and the sum of RAD and ENT (RAD + ENT) and (b–d) their sensitivity to Nd. Mean sensitivity evolution is shown for the five groups with different prescribed Nd–LWP relationships () at sunrise, whose evolution in is shown in Fig. 1. A 1 h running mean is applied. Gray shading indicates nighttime hours.
First, we show the mean evolution in LWP tendencies attributed to entrainment, radiation, and their net effect (Fig. 2a). remains constant throughout the night, consistent with the saturation of the dependence of LW cooling on LWP when clouds are still relatively thin. strengthens weakly as cloud thickens during the night. After sunrise, SW heating offsets LW cooling and weakens the entrainment mixing at cloud tops. During cloud recovery in the late afternoon, the impacts of radiation and entrainment on LWP tendency balance each other. We caution that during the late afternoon the difference between the ℒ′ from the budget analysis (i.e., Eq. 1) and the ℒ′ diagnosed directly from the simulations increases, and for this reason, we limit our interpretation of the LWP budget evolution to the hours before 15:00 local time.
Next, we investigate the sensitivity of LWP tendency to Nd (i.e., , , and , where the second ′ indicates derivatives with respect to ln (Nd); Fig. 2b–d), focusing on their role in governing the evolution in , as seen in Fig. 1. Different colors in Fig. 2b–d represent exactly the same subgroups conditioned on prescribed values of at sunrise, i.e., from −0.4 to 0.4. An important note to keep in mind is that these sensitivities to Nd inherently include sensitivities to LWP because we prescribed the Nd–LWP slope in these subgroups, such that high Nd is associated with high LWP when is positive (e.g., the red line), and high Nd is associated with low LWP for a negative (e.g., the blue line).
During the night, the net effect of entrainment and radiation on the LWP tendency (Fig. 2c) nicely explains the persistent decreasing trend in (Fig. 1). The negative values in (regardless of the prescribed values) suggest that clouds with higher Nd experience stronger LWP loss, resulting in the Nd–LWP slope becoming more negative with time. This effect is primarily driven by the term (Fig. 2b), consistent with the entrainment-enhancement mechanism due to more smaller droplets (Wang et al., 2003). When cloud water and droplet number are positively correlated (the red line), the sensitivity of the LWP tendency to Nd () is found to be the strongest (Fig. 2c), confirming the fastest decrease in in that subgroup (Fig. 1), as both higher Nd and higher LWP induce stronger entrainment.
After sunrise, a feature essential to explaining the buffered evolution in emerges, that is in subgroups with a negative at sunrise (i.e., blue and cyan) become positively correlated with Nd (Fig. 2c), indicating a reverse of the persistent nighttime negative trend in , which leads to the flattening of the negative Nd–LWP slopes (Fig. 1). Radiation, especially SW heating, plays a critical role here by dominating the contribution to the stratification feature observed in between 10:00 and 11:00 local time (Fig. 2d). This would not be the case if were to follow its trend during the nighttime as if there were no solar radiation. The dependence of solar heating on Nd and especially cloud water is key. Unlike LW cooling, whose dependence on LWP saturates when clouds are still relatively thin, the dependence of SW heating on LWP persists in thicker clouds (Petters et al., 2012), such that thick clouds absorb more SW than thin clouds – a positive slope between SW heating and LWP. This leads to a negative slope between and LWP, given that LW cooling still dominates the contribution of radiative processes to the LWP tendency in the daytime; i.e., is positive in the mean (Fig. 2a, red line). In other words, higher LWP induces more SW heating or stronger offsetting of the LW cooling, leading to a weaker LWP tendency due to radiation. Effectively, the inclusion of SW radiation reverses the slope between and Nd, regardless of the prescribed Nd–LWP slope (Fig. 2d). When a positive Nd–LWP slope is imposed at sunrise, this translates into a negative slope between and Nd (red line in Fig. 2d), whereas when Nd and LWP are negatively correlated, is positive (Fig. 2d, blue line). The fact that the dependence of on LWP is able to explain the observed evolution in suggests that the effect of Nd on the LWP tendency driven by radiative processes is secondary to the impact of LWP. In other words, if the counterhypothesis is true, that is the Nd impact is not secondary to the LWP impact (or comparable to the LWP impact), then should be skewed towards negative values after sunrise, as the LWP impact and the Nd impact offset (complement) each other in the case of a negative (positive) Nd–LWP slope. Therefore, we conclude that the buffered evolution observed in after sunrise (Fig. 1) can be attributed to the primary dependence of SW heating on cloud water.
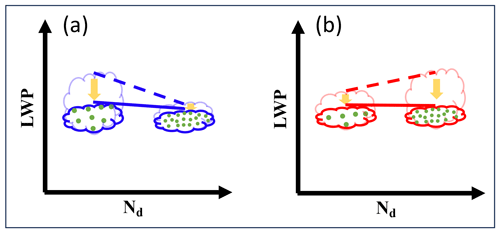
Figure 3A schematic illustrating the hypothesis for the cause of the buffered daytime evolution in Nd–LWP relationship – that is thicker clouds thin faster, whereas thinner clouds thin slower, resulting in flattened slopes (solid lines) regardless of the initial slope at sunrise (dashed lines). Blue (red) “clouds” represent the blue (red) group in Fig. 1.
To summarize, we have identified two features associated with the diurnal evolution of the cloud water–droplet number relationship for non-precipitating Sc: (1) the Nd–LWP slope becomes more negative with time at night, and (2) the Nd–LWP slope flattens (is buffered) after sunrise due to the strong dependence of SW heating on cloud LWP than on Nd. A schematic summarizing the latter point is shown in Fig. 3, where thicker clouds (higher LWP) experience stronger cloud thinning, resulting in flattening of the Nd–LWP slope. Keeping these two features in mind, we next explore the dependence of the cloud radiative effect, in the form of daytime-integrated SW reflection, on the relationship between cloud water and droplet number at sunrise.
3.2 The role of the Nd–LWP relationship at sunrise in governing the daytime cloud radiative effect
When we assess the radiative effect at the top of the atmosphere (TOA) due to aerosol–cloud interactions (ACIs), the reflectance from the entire Sc scene matters. In other words, the all-sky SW albedo of the cloud field is governed by its areal coverage (fc) and the optical thickness of the cloud, which is a function of its LWP and Nd (i.e., , based on the adiabatic assumption; Boers and Mitchell, 1994). Using a two-stream approximation to relate changes in cloud albedo (Ac) to changes in τ (Platnick and Twomey, 1994), one can show that the sensitivity of Ac to Nd perturbations (S) follows the form of
Clearly, one sees that the subject of this study – the cloud water–droplet number relationship () – is central to this equation, in the sense that close to −0.4 it could determine the sign of S, i.e., cloud brightening or darkening. As demonstrated in the previous section, diurnal evolution in is sensitive to its value at sunrise. This motivates us to further investigate the effect of the Nd–LWP slope at sunrise on the daytime cloud radiative effect due to Nd perturbations. Given the persistent decreasing trend in during the night (Fig. 1), assuming unchanged large-scale meteorological conditions throughout the day, one can relate the sunrise value of to the elapsed time since the perturbation in Nd was introduced. This is because at the time when an aerosol perturbation is applied to a Sc system, we know that Nd responds to the addition of aerosol much more quickly than the amount of cloud water and its horizontal extent (i.e., cloud fraction) adjust to the new microphysical state of the cloud, resulting in a flat slope between cloud micro- and macrophysical properties. As a result, the earlier the Nd perturbation is applied, the more negative will be at sunrise, as persistently decreases during the night.
We use the cMC method to subsample conditions where a 25-member subset of the LES ensemble has near-zero Nd–LWP and Nd–fc slopes, to mimic flat slopes between cloud micro- and macrophysical properties, in addition to the constraint on Nd–MET covariations. (See Sect. 2.2 for the threshold values used to impose these constraints.) We vary the time at which we impose these near-zero slopes, ranging from 22:40 to 05:40 (∼ sunrise) local time with an increment of 1 h, yielding eight subsampled groups whose diurnal evolution in the slope between cloud properties (LWP, fc, Ac, and SW reflection) and Nd is further examined. Although our opportunistic sampling strategy based on background aerosol conditions does not fully represent deliberate aerosol seeding, such as MCB, which will likely inject larger and more hygroscopic particles than we assumed in these simulations, it does provide insights into the qualitative relationship between MCB efficacy and seeding time.
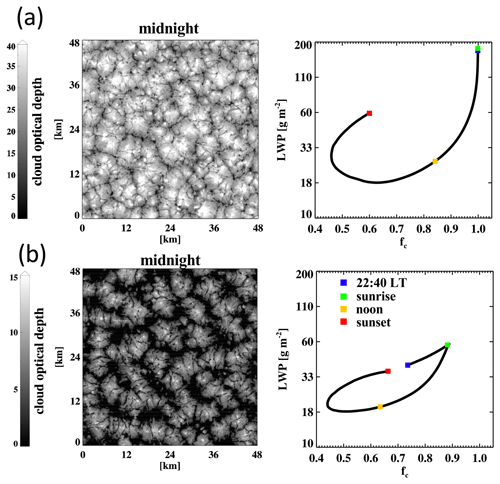
Figure 4Left column: example 2D snapshots of cloud optical depth at local midnight (hour 6 of the simulation time) and right column: mean diurnal cycle of LWP and cloud fraction (fc) of the simulated closed-cell and (non-precipitating) open–cell Sc. Sunrise, sunset, noon, and 22:40 LT (end of spin-up) are indicated on the diurnal cycle.
A subtlety here is the interpretation of Nd–fc relationships (quantified as ), as the diurnal evolution in fc between open-cell (non-precipitating) Sc and closed-cell Sc is distinct from each other (e.g., Fig. 4). Besides, open-cell Sc clouds can have quite different cloud-top entrainment characteristics, compared to closed-cell clouds (e.g., Abel et al., 2020). For these reasons, we further classify the 204 non-precipitating cases into (1) overcast closed-cell Sc and (2) non-precipitating open-cell Sc, based on fc values at night. A total of 114 simulations where fc remains 1 from ∼ 22:40 (local time; after spin-up) to sunrise are classified into (1), and the rest (90 runs) are classified into (2). Figure 4 shows example snapshots of the cloud field at midnight and the mean cloud behaviors of these two classes. For overcast closed-cell Sc, clouds thin first while maintaining the overcast state before they start to break up at ∼100 g m−2 (Fig. 4a). For non-precipitating open-cell Sc, clouds thicken and widen at the same time before sunrise, and, in a similar manner, they thin and shrink after sunrise, creating a loop-like diurnal cycle in the LWP–fc variable space (Fig. 4b). Both classes of clouds begin to recover LWP and fc after noon, except that the non-precipitating open-cell class recovers fc faster.
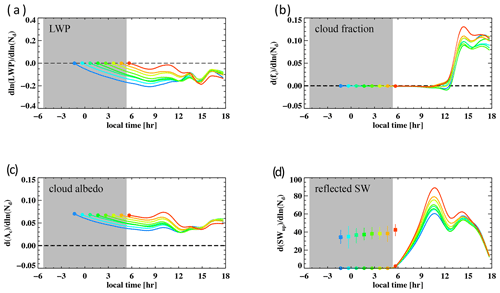
Figure 5Diurnal cycle of (a) , (b) , (c) , and (d) . Colors separate groups mimicking “aerosol perturbation” at different times when and are set to ∼0. Mean values averaged over 50 repeated cMC samplings of each group are shown. Relationships between Nd and diurnally integrated reflected SW (i.e., ) for different perturbation times are shown as filled squares with interquartile ranges using the same color scheme. A 1 h running mean is applied. Gray shading indicates nighttime hours.
3.2.1 Overcast closed-cell Sc
Figure 5a–d show the evolution of slopes between Nd and cloud properties, including LWP, fc, cloud albedo (Ac), and upwelling SW radiation at TOA (SWup; a measure of reflected SW radiation by the entire cloud scene) for the eight cMC-subsampled groups (separated by colors). The Nd–LWP slope in all subgroups trends negatively with time during the night, and its evolution appears buffered after sunrise (Fig. 5a), consistent with the results shown in Sect. 3.1 (Fig. 1). The Nd–Ac slope is positive despite the negative Nd–LWP slope (Fig. 5c), in agreement with the critical Nd–LWP slope of −0.4 for the LWP adjustment to overcome the Twomey effect (Eq. 2). The evolution in the Nd–Ac slope closely tracks that in the Nd–LWP slope, suggesting a strong control of over S. The clouds remain overcast throughout the night until late morning, when the thinnest clouds break up earliest, resulting in a slight negative Nd–fc slope, owing to the negative slope between Nd and LWP at sunrise, but only when is strongly negative (e.g., blue line in Fig. 5). This is also evident in the relationships between Nd and the cloud breakup time (), where only the two groups with the earliest perturbation time (thereby more negative at sunrise) do not show a delayed breakup (Fig. 6, black) under high-Nd conditions. After noon, the Nd–fc slope becomes positive for all groups (Fig. 5b), attributed to a generally delayed diurnal cycle in both LWP and fc (Fig. 6), meaning cloud thinning and breakup occur later in high-Nd clouds due to weaker LWP and fc tendencies when Nd and LWP are negatively correlated at sunrise (Figs. 5a and S2).
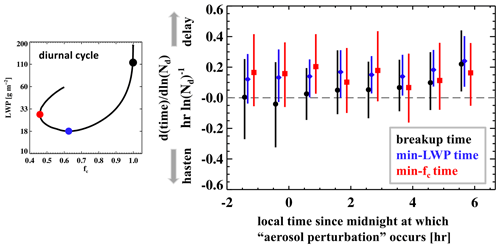
Figure 6Relationships between Nd and overcast closed-cell Sc diurnal cycle critical times, i.e., , which include the time when cloud breaks up (fc<0.95; black), reaches minimum LWP (blue), and reaches minimum fc (red), for different “aerosol perturbation” times. Mean values and interquartile ranges are shown. The left-hand-side diagram is the same as that in Fig. 5a, for the illustration of critical times in the diurnal cycle.
When we combine the effects of Twomey, LWP, and fc adjustments, it comes as no surprise that higher Nd leads to more reflected SW at TOA throughout the day (Fig. 5d), given that the negative is not strong enough to overcome the Twomey effect (Fig. 5c) and that is mostly positive. Clearly, SWup has the strongest sensitivity to Nd perturbations in the group with the latest “aerosol perturbation” (at sunrise; red line in Fig. 5d), which produces the greatest increase in the temporally integrated SWup per unit increase in ln (Nd) (Fig. 5, filled squares). A critical difference between these groups is the Nd–LWP relationship at sunrise, which is important for daytime cloud tendencies and strongly tied to the time of “aerosol perturbation” in this setup.
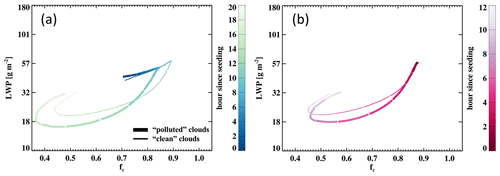
Figure 8Diurnal cycle of cloud evolution in LWP–fc space, for (a) the earliest and (b) the latest (at sunrise) “aerosol perturbation” groups. Thick lines represent the mean evolution of the highest 20 % of the members in Nd (“polluted” clouds, high-Nd), whereas the thinner lines indicate the lowest 20 % in Nd (“clean” clouds, low-Nd). Lines are colored and separated at every hour since the “aerosol perturbation”.
3.2.2 Non-precipitating open-cell Sc
Similar evolution in Nd–LWP and Nd–Ac slopes is found in non-precipitating open-cell Sc (Fig. 7a and c). In contrast to the evolution in Nd–fc slope for the overcast closed-cell Sc, where different groups track each other quite closely throughout the day, the Nd–fc slope after sunrise stratifies by both the Nd–LWP and the Nd–fc slopes at sunrise in the non-precipitating open-cell Sc (Fig. 7b). This is consistent with the characteristic diurnal cycle of LWP and fc (Fig. 4b) such that they increase (or decrease) coherently with time, leading to a similarly buffered evolution in (Fig. 7b). Worth noting is that the buffering effect drives a sign change in after noon for the groups with the latest “aerosol perturbation” (orange and red lines). A comparison between the earliest and the latest (at sunrise) “aerosol perturbation” groups (Fig. 8) reveals that for groups starting with already pronounced negative Nd–LWP and Nd–fc slopes at sunrise (Fig. 8a), the effect of increasing Nd is to shift the diurnal cycle towards lower LWP and lower fc in general. However, for groups where LWP and fc remain similar between high- and low-Nd clouds at sunrise (Fig. 8b), the addition of smaller cloud droplets reduces LWP gradually, a process that can be attributed to the enhanced cloud-top entrainment, while similar fc is maintained. For both cases, cloud recovery is noted to be slighted hastened under high-Nd conditions (Fig. S3), which is likely facilitated by weaker SW heating due to reduced LWP. In the case of sunrise “perturbation” where fc is only subtly adjusted, hastened fc recovery leads to a positive in the afternoon (Figs. 7b and 8).
This stratification in Nd–fc slopes complements the radiative impact due to Nd–LWP stratification alone (Fig. 7a), leading to an even more pronounced stratification in evolution. As a result, the dependence of on “aerosol perturbation” time is more pronounced than that in the overcast closed-cell Sc (Fig. 7d, filled squares).
Despite the wide usage of the statistical regression method to derive aerosol–cloud relationships from which process understanding is inferred, the extent to which these statistical relationships equate to a causal response, thereby representing cloud adjustments, has been a nagging concern of studies of this kind. More recently, there is evidence showing that the negative branch of the observed inverted-V shape in the Nd–LWP relationship (e.g., Gryspeerdt et al., 2019) overestimates the true causal effect of Nd on LWP (e.g., Arola et al., 2022; Fons et al., 2023). Using general circulation models (GCMs), Mülmenstädt et al. (2024) demonstrate the possibility that the sign of the cloud adjustment inferred from the Nd–LWP relationship derived from internal variabilities can even be misleading, which they attribute to the confounding effect of the covariation between Nd and meteorological conditions.
We wish to note that the way we investigate the relationship between cloud water and droplet number, i.e., by subsampling conditions where a subsample of the large simulation ensemble has a predetermined Nd–LWP relationship and by focusing on its evolution rather than its absolute value, alleviates reliance on the interpretation of the Nd–LWP relationships as causal relationships. In other words, the SW-heating-driven feedback (or buffering) mechanism we have uncovered in this work is a robust feature of the Sc system and does not depend on the actual (prescribed) value of in the cMC experiments or in the real world. From this perspective, we discuss the role that these results, in particular this feedback mechanism, play in the aerosol–cloud interactions that we observe in nature, where the Nd–LWP relationship is not predetermined and often confounded by other cloud controlling factors. In fact, a number of satellite-based studies have suggested that this relationship in nature is strongly dependent on cloud regime, boundary layer characteristics, and the spatial scale of one's investigation (e.g., Gryspeerdt et al., 2019; Possner et al., 2020; Toll et al., 2019; Zhou and Feingold, 2023). The essence of this radiation buffering is the dependence of LWP tendency attributed to radiation processes (SW absorption in particular) on cloud LWP, meaning thicker clouds thin faster and thinner clouds thin more slowly (Fig. 3), flattening whatever slope Nd and LWP may have had before sunrise, depending on the large-scale meteorological conditions the clouds have experienced, no matter whether the Nd–LWP relationship is causal or not.
Although many aspects of the boundary layer thermodynamic structure are varied to construct the large ensemble, two large-scale conditions, namely SST and free-troposphere subsidence, are fixed among ensemble members. The cMC approach is designed to effectively limit the role that the variability in these large-scale conditions can play in driving the evolution in the Nd–LWP relationship, by subsampling simulations with flat slopes between Nd and other cloud controlling factors at the beginning of the simulations. Although such a variability in the prescribed large-scale conditions can cause subtle differences in the exact timing and strength of the “buffered” feature, the finding of the feature itself remains robust based on a sensitivity test with variable SST simulations (not shown). Once again, the concept of using a large ensemble with cMC sampling is not to provide a reference value for the Nd–LWP relationship, which may still be weakly dependent on the prescribed SST and subsidence even after applying cMC, but to explore features of the Sc system that are robust even in the context of (co-)varying large-scale conditions, e.g., in the real world.
Moreover, one of the strengths of this novel subsampling approach is by design to minimize the confounding effects from the initial boundary layer conditions in this large ensemble of simulations and to address some of the aforementioned concerns. Therefore, although our emphasis is not on quantifying actual cloud adjustments, we aim to advance our understanding of the temporal evolution in adjustments. Consider the marine cloud brightening (MCB) idea, one of the proposed climate intervention approaches, as an example. When we think about how we might maximize the total amount of sunlight reflected over a day if we were to seed non-precipitating Sc clouds to increase their reflectivity of solar radiation, we want neither a negative LWP adjustment to start with nor seeding after the sun is up. Given the persistent negative trend in the nighttime evolution of (Fig. 1 and Sect. 3.1), it is logical to propose that seeding at sunrise would be the most effective brightening strategy, which our results in Sect. 3.2 have validated. This is attributed to the critical role of sunrise values of cloud water, Nd, and their correlation in governing the daytime evolution of cloud fraction and LWP.
There are, of course, caveats to this implication. For one, we focus only on non-precipitating Sc systems, whereas studies have shown that precipitation can modulate the impact of cloud-top entrainment on the LWP adjustment (Smalley et al., 2024; Stevens et al., 1998). Furthermore, suppressing or even preventing precipitation in Sc systems can potentially generate larger radiative impacts, compared to brightening the non-precipitating systems (e.g., Wang and Feingold, 2009; Prabhakaran et al., 2023, 2024; Chun et al., 2023). Moreover, given the typical lifetime of aerosol in the marine boundary layer (a few days; Wood, 2012, 2021), our integration over one diurnal cycle may seem short in terms of representing the full extent of the radiative impact due to seeding. Extending the analysis to three diurnal cycles by re-using the 24 h simulations for cMC subsampling results in similar conclusions with respect to the persistent nighttime negative trend in the Nd–LWP slope and the daytime buffering due to SW absorption, which essentially makes the Nd–LWP slope oscillate between −0.1 and −0.4 after convergence during the first afternoon (Fig. S4). That said, these non-precipitating Sc clouds tend to be advected by the prevailing winds in the region and experience pronounced large-scale forcing changes, e.g., warming SST and deepening marine boundary layer, which lead to transition into a more cumulus regime, during the course of 3 to 5 d over subtropical ocean basins (Bretherton and Wyant, 1997; Yamaguchi et al., 2017). Studies deploying large ensemble of multi-day Lagrangian simulations are warranted to further address this issue. While the implications of this particular exemplary application (i.e., MCB) are limited, the great potential of applying this cMC approach to simulation ensembles is demonstrated.
A novel conditional Monte Carlo (cMC) subsampling approach is applied to a large ensemble of diurnal LESs, in order to explore the role of solar heating in affecting the temporal evolution and timescale of cloud water adjustment to aerosol perturbations in non-precipitating marine stratocumulus. We find evidence supporting an important negative feedback (or buffering) mechanism in the daytime evolution of the Nd–LWP relationship such that a persistent decreasing trend at night is buffered (Nd–LWP slope becomes flattened) after sunrise, regardless of the actual value of . Using a budget analysis of the LWP tendency, we separate and quantify the contributions from individual processes to this tendency, including entrainment and radiation. This enables us to attribute this buffering effect to the primary dependence of SW heating on LWP. This result emphasizes the dominant role of cloud LWP in governing daytime cloud tendencies, especially those related to SW absorption. The impacts of Nd perturbations appear to be only secondary.
This SW-LWP buffering has important implications for the temporal evolution in cloud adjustments to aerosol perturbations and the timescale of adjustments. Among various feedback mechanisms through microphysical processes, such as evaporation and sedimentation, surface fluxes, and/or large-scale circulation adjustments (e.g., Wang et al., 2003; Bretherton et al., 2007; Chun et al., 2023; Dagan et al., 2023), the role of SW heating has received the least attention. The implications for aerosol–cloud radiative forcing of climate are yet to be fully evaluated.
The methodology applied to the large simulation ensemble (i.e., subsampling) differs from previous studies in which the whole ensemble is used at once to map emergent properties, such as the cloud radiative effect (Glassmeier et al., 2019) and their flow field, from a wide range of initial conditions (e.g., Glassmeier et al., 2021; Hoffmann et al., 2020). This work demonstrates the substantial potential in the application of this cMC approach. It can enhance the usefulness of any large ensemble of simulations by generating numerous sub-ensembles, whose potential in scientific applications is well beyond that of the original ensemble, without the need to increase the size of the original ensemble.
The cMC subsampling approach presents a new pathway to explore systematic behaviors in cloud evolution from a large number of simulated realizations or observations while avoiding spurious covariations among cloud controlling factors that are related to either the seemingly random initializations or meteorological confounding factors. This alleviates the need to assume that spatiotemporal correlations can be used to infer causal relationships. Moreover, it enables one to select conditions where hypothesis-driven constraints can be prescribed and tested.
The SW-LWP buffering mechanism and its important role in governing the diurnal evolution in cloud water response to droplet number perturbations also have implications for the assessment of the viability of MCB. The robust decreasing trend in the Nd–LWP relationship at night motivates an MCB-oriented thinking on how one might maximize the sunlight reflected by a cloud scene. Using the cMC subsampling approach as a way to mimic the timing of the aerosol perturbation, we make the case that seeding at sunrise presents the highest potential for brightening. This statement is by no means an endorsement of MCB as a viable climate intervention method. Much more solid research is needed at this stage to determine the viability of MCB and to quantify the potential risks associated with it (Feingold et al., 2024).
The System for Atmospheric Modeling (SAM) code is graciously provided by Marat Khairoutdinov and is publicly available at http://rossby.msrc.sunysb.edu/SAM (Khairoutdinov, 2024). Input files for reproducing the simulation data are available from the NOAA Chemical Sciences Laboratory's Clouds, Aerosol, & Climate program at https://csl.noaa.gov/groups/csl9/datasets/data/2024-Zhang-etal/ (Zhang, 2024).
The supplement related to this article is available online at: https://doi.org/10.5194/acp-24-10425-2024-supplement.
JZ carried out the data analysis and wrote the manuscript with input from all authors. YSC and TY ran the simulation ensemble. All authors contributed to the design of the study and the interpretation of the results.
At least one of the (co-)authors is a member of the editorial board of Atmospheric Chemistry and Physics. The peer-review process was guided by an independent editor, and the authors also have no other competing interests to declare.
Publisher's note: Copernicus Publications remains neutral with regard to jurisdictional claims made in the text, published maps, institutional affiliations, or any other geographical representation in this paper. While Copernicus Publications makes every effort to include appropriate place names, the final responsibility lies with the authors.
We thank Franziska Glassmeier and Fabian Hoffmann for insightful comments and discussion. We thank the two anonymous reviewers for their insights and suggestions for improving our manuscript. We thank the NOAA Earth's Radiation Budget (ERB) for supporting this research.
This research has been supported in part by the U.S. Department of Energy, Office of Science, Atmospheric System Research Program Interagency Award 89243023SSC000114, the U.S. Department of Commerce Earth's Radiation Budget program (NOAA CPO Climate & CI no. 03-01-07-001), and the NOAA Cooperative Agreement with CIRES (grant no. NA22OAR4320151).
This paper was edited by Toshihiko Takemura and reviewed by two anonymous referees.
Abel, S. J., Barrett, P. A., Zuidema, P., Zhang, J., Christensen, M., Peers, F., Taylor, J. W., Crawford, I., Bower, K. N., and Flynn, M.: Open cells exhibit weaker entrainment of free-tropospheric biomass burning aerosol into the south-east Atlantic boundary layer, Atmos. Chem. Phys., 20, 4059–4084, https://doi.org/10.5194/acp-20-4059-2020, 2020. a
Ackerman, A. S., vanZanten, M. C., Stevens, B., Savic-Jovcic, V., Bretherton, C. S., Chlond, A., Golaz, J.-C., Jiang, H., Khairoutdinov, M., Krueger, S. K., Lewellen, D. C., Lock, A., Moeng, C.-H., Nakamura, K., Petters, M. D., Snider, J. R., Weinbrecht, S., and Zulauf, M.: Large-Eddy Simulations of a Drizzling, Stratocumulus-Topped Marine Boundary Layer, Mon. Weather Rev., 137, 1083–1110, https://doi.org/10.1175/2008MWR2582.1, 2009. a
Albrecht, B. A.: Aerosols, Cloud Microphysics, and Fractional Cloudiness, Science, 245, 1227–1230, https://doi.org/10.1126/science.245.4923.1227, 1989. a, b
Arola, A., Lipponen, A., Kolmonen, P., Virtanen, T. H., Bellouin, N., Grosvenor, D. P., Gryspeerdt, E., Quaas, J., and Kokkola, H.: Aerosol effects on clouds are concealed by natural cloud heterogeneity and satellite retrieval errors, Nat. Commun., 13, 7357, https://doi.org/10.1038/s41467-022-34948-5, 2022. a
Bellouin, N., Quaas, J., Gryspeerdt, E., Kinne, S., Stier, P., Watson-Parris, D., Boucher, O., Carslaw, K., Christensen, M., Daniau, A.-L., Dufresne, J.-L., Feingold, G., Fiedler, S., Forster, P., Gettelman, A., Haywood, J., Lohmann, U., Malavelle, F., Mauritsen, T., and Stevens, B.: Bounding global aerosol radiative forcing of climate change, Rev. Geophys., 58, e2019RG000660, https://doi.org/10.1029/2019RG000660, 2020. a
Bender, F. A.-M., Charlson, R. J., Ekman, A. M. L., and Leahy, L. V.: Quantification of Monthly Mean Regional Scale Albedo of Marine Stratiform Clouds in Satellite Observations and GCMs, J. Appl. Meteor. Climatol., 50, 2139–2148, https://doi.org/10.1175/JAMC-D-11-049.1, 2011. a
Boers, R. and Mitchell, R. M.: Absorption feedback in stratocumulus clouds Influence on cloud top albedo, Tellus A, 46, 229–241, https://doi.org/10.1034/j.1600-0870.1994.00001.x, 1994. a
Boucher, O., Randall, D., Artaxo, P., Bretherton, C., Feingold, G., Forster, P., Kerminen, V.-M., Kondo, Y., Liao, H., Lohmann, U., Rasch, P., Satheesh, S., Sherwood, S., Stevens, B., and Zhang, X.: Clouds and Aerosols, in: Climate Change 2013: The Physical Science Basis. Contribution of Working Group I to the Fifth Assessment Report of the Intergovernmental Panel on Climate Change, edited by: Stocker, T., Qin, D., Plattner, G.-K., Tignor, M., Allen, S., Boschung, J., Nauels, A., Xia, Y., Bex, V., and Midgley, P., pp. 571–658, Cambridge University Press, Cambridge, United Kingdom and New York, NY, USA, 2013. a
Bretherton, C. S. and Wyant, M. C.: Moisture Transport, Lower-Tropospheric Stability, and Decoupling of Cloud-Topped Boundary Layers, J. Atmos. Sci., 54, 148–167, https://doi.org/10.1175/1520-0469(1997)054<0148:MTLTSA>2.0.CO;2, 1997. a
Bretherton, C. S., Blossey, P. N., and Uchida, J.: Cloud droplet sedimentation, entrainment efficiency, and subtropical stratocumulus albedo, Geophys. Res. Lett., 34, L03813, https://doi.org/10.1029/2006GL027648, 2007. a, b, c
Chen, Y.-C., Christensen, M., Stephens, G. L., and Seinfeld, J. H.: Satellite-based estimate of global aerosol–cloud radiative forcing by marine warm clouds, Nat. Geosci., 7, 643–646, https://doi.org/10.1038/ngeo2214, 2014. a
Chen, Y.-S., Zhang, J., Hoffmann, F., Yamaguchi, T., Glassmeier, F., Zhou, X., and Feingold, G.: Diurnal evolution of non-precipitating marine stratocumuli in an LES ensemble, EGUsphere [preprint], https://doi.org/10.5194/egusphere-2024-1033, 2024. a, b, c, d, e
Christensen, M. W., Ma, P.-L., Wu, P., Varble, A. C., Mülmenstädt, J., and Fast, J. D.: Evaluation of aerosol–cloud interactions in E3SM using a Lagrangian framework, Atmos. Chem. Phys., 23, 2789–2812, https://doi.org/10.5194/acp-23-2789-2023, 2023. a
Chun, J.-Y., Wood, R., Blossey, P., and Doherty, S. J.: Microphysical, macrophysical, and radiative responses of subtropical marine clouds to aerosol injections, Atmos. Chem. Phys., 23, 1345–1368, https://doi.org/10.5194/acp-23-1345-2023, 2023. a, b, c
Clough, S. A., Shephard, M. W., Mlawer, E. J., Delamere, J. S., Iacono, M. J., Cady-Pereira, K., Boukabara, S., and Brown, P. D.: Atmospheric radiative transfer modeling: A summary of the AER codes, J. Quant. Spectrosc. Ra., 91, 233–244, https://doi.org/10.1016/j.jqsrt.2004.05.058, 2005. a
Dagan, G., Yeheskel, N., and Williams, A. I. L.: Radiative forcing from aerosol–cloud interactions enhanced by large-scale circulation adjustments, Nat. Commun., 16, 1092–1098, https://doi.org/10.1038/s41561-023-01319-8, 2023. a
Diamond, M. S., Director, H. M., Eastman, R., Possner, A., and Wood, R.: Substantial Cloud Brightening From Shipping in Subtropical Low Clouds, AGU Adv., 1, e2019AV000111, https://doi.org/10.1029/2019AV000111, 2020. a
Feingold, G., Walko, R., Stevens, B., and Cotton, W.: Simulations of marine stratocumulus using a new microphysical parameterization scheme, Atmos. Res., 47–48, 505–528, https://doi.org/10.1016/S0169-8095(98)00058-1, 1998. a
Feingold, G., Eberhard, W. L., Veron, D. E., and Previdi, M.: First measurements of the Twomey indirect effect using ground-based remote sensors, Geophys. Res. Lett., 30, 1287, https://doi.org/10.1029/2002GL016633, 2003. a
Feingold, G., McComiskey, A., Yamaguchi, T., Johnson, J. S., Carslaw, K. S., and Schmidt, K. S.: New approaches to quantifying aerosol influence on the cloud radiative effect, P. Natl. Acad. Sci. USA, 113, 5812–5819, https://doi.org/10.1073/pnas.1514035112, 2016. a, b, c
Feingold, G., Ghate, V. P., Russell, L. M., Blossey, P., Cantrell, W., Christensen, M. W., Diamond, M. S., Gettelman, A., Glassmeier, F., Gryspeerdt, E., Haywood, J., Hoffmann, F., Kaul, C. M., Lebsock, M., McComiskey, A. C., McCoy, D. T., Ming, Y., Mülmenstädt, J., Possner, A., Prabhakaran, P., Quinn, P. K., Schmidt, K. S., Shaw, R. A., Singer, C. E., Sorooshian, A., Toll, V., Wan, J. S., Wood, R., Yang, F., Zhang, J., and Zheng, X.: Physical science research needed to evaluate the viability and risks of marine cloud brightening, Sci. Adv., 10, eadi8594, https://doi.org/10.1126/sciadv.adi8594, 2024. a
Fons, E., Runge, J., Neubauer, D., and Lohmann, U.: Stratocumulus adjustments to aerosol perturbations disentangled with a causal approach, npj Clim. Atmos. Sci., 6, 130, https://doi.org/10.1038/s41612-023-00452-w, 2023. a, b
Forster, P., Storelvmo, T., Armour, K., Collins, W., Dufresne, J.-L., Frame, D., Lunt, D. J., Mauritsen, T., Palmer, M. D., Watanabe, M., Wild, M., and Zhang, H.: The Earth’s Energy Budget, Climate Feedbacks, and Climate Sensitivity, in: Climate Change 2021: The Physical Science Basis, Contribution of Working Group I to the Sixth Assessment Report of the Intergovernmental Panel on Climate Change, edited by: Masson-Delmotte, V., Zhai, P., Pirani, A., Connors, S. L., Péan, C., Berger, S., Caud, N., Chen, Y., Goldfarb, L., Gomis, M. I., Huang, M., Leitzell, K., Lonnoy, E., Matthews, J. B. R., Maycock, T. K., Waterfield, T., Yelekçi, O., Yu, R., and Zhou, B., pp. 923–1054, Cambridge University Press, Cambridge, United Kingdom and New York, NY, USA, 2021. a
Glassmeier, F., Hoffmann, F., Johnson, J. S., Yamaguchi, T., Carslaw, K. S., and Feingold, G.: An emulator approach to stratocumulus susceptibility, Atmos. Chem. Phys., 19, 10191–10203, https://doi.org/10.5194/acp-19-10191-2019, 2019. a, b, c
Glassmeier, F., Hoffmann, F., Johnson, J. S., Yamaguchi, T., Carslaw, K. S., and Feingold, G.: Aerosol-cloud-climate cooling overestimated by ship-track data, Science, 371, 485–489, https://doi.org/10.1126/science.abd3980, 2021. a, b, c, d, e, f
Gryspeerdt, E., Quaas, J., and Bellouin, N.: Constraining the aerosol influence on cloud fraction, J. Geophys. Res.-Atmos., 121, 3566–3583, https://doi.org/10.1002/2015JD023744, 2016. a
Gryspeerdt, E., Goren, T., Sourdeval, O., Quaas, J., Mülmenstädt, J., Dipu, S., Unglaub, C., Gettelman, A., and Christensen, M.: Constraining the aerosol influence on cloud liquid water path, Atmos. Chem. Phys., 19, 5331–5347, https://doi.org/10.5194/acp-19-5331-2019, 2019. a, b, c, d, e
Gryspeerdt, E., Goren, T., and Smith, T. W. P.: Observing the timescales of aerosol–cloud interactions in snapshot satellite images, Atmos. Chem. Phys., 21, 6093–6109, https://doi.org/10.5194/acp-21-6093-2021, 2021. a
Gryspeerdt, E., Glassmeier, F., Feingold, G., Hoffmann, F., and Murray-Watson, R. J.: Observing short-timescale cloud development to constrain aerosol–cloud interactions, Atmos. Chem. Phys., 22, 11727–11738, https://doi.org/10.5194/acp-22-11727-2022, 2022. a, b
Hammersley, J. M. and Handscomb, D. C.: Monte Carlo Methods, Springer Dordrecht, Netherlands, ISBN 978-94-009-5821-0, 1964. a
Hersbach, H., Bell, B., Berrisford, P., Hirahara, S., Horányi, A., Muñoz-Sabater, J., Nicolas, J., Peubey, C., Radu, R., Schepers, D., Simmons, A., Soci, C., Abdalla, S., Abellan, X., Balsamo, G., Bechtold, P., Biavati, G., Bidlot, J., Bonavita, M., De Chiara, G., Dahlgren, P., Dee, D., Diamantakis, M., Dragani, R., Flemming, J., Forbes, R., Fuentes, M., Geer, A., Haimberger, L., Healy, S., Hogan, R. J., Hólm, E., Janisková, M., Keeley, S., Laloyaux, P., Lopez, P., Lupu, C., Radnoti, G., de Rosnay, P., Rozum, I., Vamborg, F., Villaume, S., and Thépaut, J.-N.: The ERA5 global reanalysis, Q. J. Roy. Meteor. Soc., 146, 1999–2049, https://doi.org/10.1002/qj.3803, 2020. a
Hoffmann, F., Glassmeier, F., Yamaguchi, T., and Feingold, G.: Liquid Water Path Steady States in Stratocumulus: Insights from Process-Level Emulation and Mixed-Layer Theory, J. Atmos. Sci., 77, 2203–2215, https://doi.org/10.1175/JAS-D-19-0241.1, 2020. a, b
Hoffmann, F., Glassmeier, F., Yamaguchi, T., and Feingold, G.: On the Roles of Precipitation and Entrainment in Stratocumulus Transitions between Mesoscale States, J. Atmos. Sci, 80, 2791–2803, https://doi.org/10.1175/JAS-D-22-0268.1, 2023. a
Kazil, J., Wang, H., Feingold, G., Clarke, A. D., Snider, J. R., and Bandy, A. R.: Modeling chemical and aerosol processes in the transition from closed to open cells during VOCALS-REx, Atmos. Chem. Phys., 11, 7491–7514, https://doi.org/10.5194/acp-11-7491-2011, 2011. a
Kazil, J., Yamaguchi, T., and Feingold, G.: Mesoscale organization, entrainment, and the properties of a closed-cell stratocumulus cloud, J. Adv. Model. Earth Sy., 9, 2214–2229, https://doi.org/10.1002/2017MS001072, 2017. a
Khairoutdinov, M.: SAM source code, Stony Brook repository [code], http://rossby.msrc.sunysb.edu/SAM, last access: 16 September 2024. a
Khairoutdinov, M. F. and Randal, D. A.: Cloud Resolving Modeling of the ARM Summer 1997 IOP: Model Formulation Results Uncertainties and Sensitivities, J. Atmos. Sci, 60, 607–625, https://doi.org/10.1175/1520-0469(2003)060<0607:CRMOTA>2.0.CO;2, 2003. a
Latham, J. and Smith, M. H.: Effect on global warming of wind-dependent aerosol generation at the ocean surface, Nature, 347, 372–373, https://doi.org/10.1038/347372a0, 1990. a
Latham, J., Bower, K., Choularton, T., Coe, H., Connolly, P., Cooper, G., Craft, T., Foster, J., Gadian, A., Galbraith, L., Iacovides, H., Johnston, D., Launder, B., Leslie, B., Meyer, J., Neukermans, A., Ormond, B., Parkes, B., Rasch, P., Rush, J., Salter, S., Stevenson, T., Wang, H., Wang, Q., and Wood, R.: Marine cloud brightening, Philos. trans., Math. Phys. Eng. Sci., 370, 4217–4262, https://doi.org/10.1098/rsta.2012.0086, 2012. a
McComiskey, A. and Feingold, G.: The scale problem in quantifying aerosol indirect effects, Atmos. Chem. Phys., 12, 1031–1049, https://doi.org/10.5194/acp-12-1031-2012, 2012. a
Morris, M. D. and Mitchell, T. J.: Exploratory designs for computational experiments, J. Stat. Plan. Inf., 43, 381–402, https://doi.org/10.1016/0378-3758(94)00035-T, 1995. a
Mülmenstädt, J. and Feingold, G.: The Radiative Forcing of Aerosol–Cloud Interactions in Liquid Clouds: Wrestling and Embracing Uncertainty, Curr. Clim. Change Rep., 4, 23–40, https://doi.org/10.1007/s40641-018-0089-y, 2018. a
Mülmenstädt, J., Gryspeerdt, E., Dipu, S., Quaas, J., Ackerman, A. S., Fridlind, A. M., Tornow, F., Bauer, S. E., Gettelman, A., Ming, Y., Zheng, Y., Ma, P.-L., Wang, H., Zhang, K., Christensen, M. W., Varble, A. C., Leung, L. R., Liu, X., Neubauer, D., Partridge, D. G., Stier, P., and Takemura, T.: General circulation models simulate negative liquid water path–droplet number correlations, but anthropogenic aerosols still increase simulated liquid water path, Atmos. Chem. Phys., 24, 7331–7345, https://doi.org/10.5194/acp-24-7331-2024, 2024. a, b
National Academies of Sciences, Engineering, and Medicine (NASEM) report: Reflecting Sunlight: Recommendations for Solar Geoengineering Research and Research Governance, The National Academies Press, Washington, DC, USA, https://doi.org/10.17226/25762, 2021. a
Petters, J. L., Harrington, J. Y., and Clothiaux, E. E.: Radiative–Dynamical Feedbacks in Low Liquid Water Path Stratiform Clouds, J. Atmos. Sci, 69, 1498–1512, https://doi.org/10.1175/JAS-D-11-0169.1, 2012. a, b, c
Platnick, S. and Twomey, S.: Determining the susceptibility of cloud albedo to changes in droplet concentration with the advanced very high resolution radiometer, J. Appl. Meteorol., 33, 334–347, https://doi.org/10.1175/1520-0450(1994)033<0334:DTSOCA>2.0.CO;2, 1994. a
Possner, A., Eastman, R., Bender, F., and Glassmeier, F.: Deconvolution of boundary layer depth and aerosol constraints on cloud water path in subtropical stratocumulus decks, Atmos. Chem. Phys., 20, 3609–3621, https://doi.org/10.5194/acp-20-3609-2020, 2020. a, b
Prabhakaran, P., Hoffmann, F., and Feingold, G.: Evaluation of Pulse Aerosol Forcing on Marine Stratocumulus Clouds in the Context of Marine Cloud Brightening, J. Atmos. Sci, 80, 1585–1604, https://doi.org/10.1175/JAS-D-22-0207.1, 2023. a, b
Prabhakaran, P., Hoffmann, F., and Feingold, G.: Effects of intermittent aerosol forcing on the stratocumulus-to-cumulus transition, Atmos. Chem. Phys., 24, 1919–1937, https://doi.org/10.5194/acp-24-1919-2024, 2024. a, b
Qiu, S., Zheng, X., Painemal, D., Terai, C. R., and Zhou, X.: Daytime variation in the aerosol indirect effect for warm marine boundary layer clouds in the eastern North Atlantic, Atmos. Chem. Phys., 24, 2913–2935, https://doi.org/10.5194/acp-24-2913-2024, 2024. a
Sandu, I., Brenguier, J.-L., Geoffroy, O., Thouron, O., and Masson, V.: Aerosol Impacts on the Diurnal Cycle of Marine Stratocumulus, J. Atmos. Sci., 65, 2705–2718, https://doi.org/10.1175/2008JAS2451.1, 2008. a
Smalley, K. M., Lebsock, M. D., and Eastman, R.: Diurnal Patterns in the Observed Cloud Liquid Water Path Response to Droplet Number Perturbations, Geophys. Res. Lett., 51, e2023GL107323, https://doi.org/10.1029/2023GL107323, 2024. a, b, c
Stephens, G. L., Li, J., Wild, M., Clayson, C. A., Loeb, N., Kato, S., L'Ecuyer, T., Stackhouse, P. W., Lebsock, M., and Andrews, T.: An update on Earth's energy balance in light of the latest global observations, Nat. Geosci., 5, 691–696, https://doi.org/10.1038/ngeo1580, 2012. a
Stevens, B. and Feingold, G.: Untangling aerosol effects on clouds and precipitation in a buffered system, Nature, 461, 607–613, https://doi.org/10.1038/nature08281, 2009. a
Stevens, B., Cotton, W. R., Feingold, G., and Moeng, C.-H.: Large Eddy Simulations of Strongly Precipitating, Shallow, Stratocumulus-Topped Boundary Layers, J. Atmos. Sci, 55, 3616–3638, https://doi.org/10.1175/1520-0469(1998)055<3616:LESOSP>2.0.CO;2, 1998. a
Stier, P.: Limitations of passive remote sensing to constrain global cloud condensation nuclei, Atmos. Chem. Phys., 16, 6595–6607, https://doi.org/10.5194/acp-16-6595-2016, 2016. a
Toll, V., Christensen, M., Quaas, J., and Bellouin, N.: Weak average liquid-cloud-water response to anthropogenic aerosols, Nature, 572, 51–55, https://doi.org/10.1038/s41586-019-1423-9, 2019. a, b
Twomey, S.: Pollution and the planetary albedo, Atmos. Environ., 8, 1251–1256, https://doi.org/10.1016/0004-6981(74)90004-3, 1974. a
Twomey, S.: The Influence of Pollution on the Shortwave Albedo of Clouds, J. Atmos. Sci., 34, 1149–1152, https://doi.org/10.1175/1520-0469(1977)034<1149:TIOPOT>2.0.CO;2, 1977. a
Wang, H. and Feingold, G.: Modeling Mesoscale Cellular Structures and Drizzle in Marine Stratocumulus Part I: Impact of Drizzle on the Formation and Evolution of Open Cells, J. Atmos. Sci, 66, 3237–3256, https://doi.org/10.1175/2009JAS3022.1, 2009. a
Wang, S., Wang, Q., and Feingold, G.: Turbulence, Condensation, and Liquid Water Transport in Numerically Simulated Nonprecipitating Stratocumulus Clouds, J. Atmos. Sci, 60, 262–278, https://doi.org/10.1175/1520-0469(2003)060<0262:TCALWT>2.0.CO;2, 2003. a, b, c, d
Wood, R.: Stratocumulus Clouds, Mon. Weather Rev., 140, 2373–2423, https://doi.org/10.1175/MWR-D-11-00121.1, 2012. a, b, c
Wood, R.: Assessing the potential efficacy of marine cloud brightening for cooling Earth using a simple heuristic model, Atmos. Chem. Phys., 21, 14507–14533, https://doi.org/10.5194/acp-21-14507-2021, 2021. a
Xue, H. and Feingold, G.: Large-Eddy Simulations of Trade Wind Cumuli: Investigation of Aerosol Indirect Effects, J. Atmos. Sci., 63, 1605–1622, https://doi.org/10.1175/JAS3706.1, 2006. a
Yamaguchi, T., Feingold, G., and Kazil, J.: Stratocumulus to Cumulus Transition by Drizzle, J. Adv. Model. Earth Sy., 9, 2333–2349, https://doi.org/10.1002/2017MS001104, 2017. a, b, c
Yamaguchi, T., Feingold, G., and Kazil, J.: Aerosol-Cloud Interactions in Trade Wind Cumulus Clouds and the Role of Vertical Wind Shear, J. Geophys. Res.-Atmos., 124, 12244–12261, https://doi.org/10.1029/2019JD031073, 2019. a
Zhang, J.: Input files, NOAA Chemical Sciences Laboratory (CSL), NOAA [data set], https://csl.noaa.gov/groups/csl9/datasets/data/2024-Zhang-etal/, last access: 26 March 2024. a
Zhang, J. and Feingold, G.: Distinct regional meteorological influences on low-cloud albedo susceptibility over global marine stratocumulus regions, Atmos. Chem. Phys., 23, 1073–1090, https://doi.org/10.5194/acp-23-1073-2023, 2023. a, b
Zhang, J., Zhou, X., Goren, T., and Feingold, G.: Albedo susceptibility of northeastern Pacific stratocumulus: the role of covarying meteorological conditions, Atmos. Chem. Phys., 22, 861–880, https://doi.org/10.5194/acp-22-861-2022, 2022. a, b, c
Zhou, X. and Feingold, G.: Impacts of Mesoscale Cloud Organization on Aerosol-Induced Cloud Water Adjustment and Cloud Brightness, Geophys. Res. Lett., 50, e2023GL103417, https://doi.org/10.1029/2023GL103417, 2023. a
Zhou, X., Zhang, J., and Feingold, G.: On the Importance of Sea Surface Temperature for Aerosol-Induced Brightening of Marine Clouds and Implications for Cloud Feedback in a Future Warmer Climate, Geophys. Res. Lett., 48, e2021GL095896, https://doi.org/10.1029/2021GL095896, 2021. a, b