the Creative Commons Attribution 4.0 License.
the Creative Commons Attribution 4.0 License.
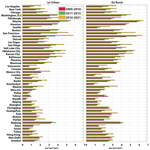
Insights into the long-term (2005–2021) spatiotemporal evolution of summer ozone production sensitivity in the Northern Hemisphere derived with the Ozone Monitoring Instrument (OMI)
Matthew S. Johnson
Sajeev Philip
Scott Meech
Rajesh Kumar
Meytar Sorek-Hamer
Yoichi P. Shiga
Jia Jung
Tropospheric ozone (O3) formation depends on the relative abundance of precursor species, nitrogen oxides (NOx), and volatile organic compounds (VOCs). Advancements in satellite retrievals of formaldehyde (HCHO) and nitrogen dioxide (NO2) vertical column densities (VCDs), and the corresponding ratios (FNRs), provide the opportunity to diagnose the spatiotemporal evolution of O3 production sensitivity regimes. This study investigates trends of Ozone Monitoring Instrument (OMI)-derived summertime VCD HCHO, NO2, and FNRs in the Northern Hemisphere from 2005 to 2021. FNR trends were analyzed for polluted regions, specifically for 46 highly populated cities, over the entire 17-year period and in 2020 when global anthropogenic emissions were reduced due to COVID-19 lockdown restrictions. It was determined that OMI-derived FNRs have increased on average by ∼ 65 % across cities in the Northern Hemisphere. Increasing OMI-derived FNRs indicates a general transition from radical-limited to NOx-limited regimes. The increasing trend is driven by reduced NO2 concentrations because of emission-control strategies of NOx. OMI FNR trends were compared to ground-based in situ measurements in US cities, and it was determined that they can capture the trends in increasing FNRs (R=0.91) and decreasing NO2 (R=0.98) occurring at the surface. OMI FNRs in urban areas were higher (∼ 20 %) in 2020 for most cities studied here compared to 2019 and 2021. In addition to studying the longest period of OMI FNRs across the Northern Hemisphere to date, the capabilities and challenges of using satellite VCD FNRs to study surface-level O3 production sensitivity regimes are discussed.
- Article
(8985 KB) - Full-text XML
-
Supplement
(3086 KB) - BibTeX
- EndNote
Tropospheric ozone (O3) is a harmful pollutant which has detrimental impacts on air quality, leading to adverse human health and premature mortality, and negative impacts on vegetation and agriculture (US EPA, 2006; Tai et al., 2014; GBD, 2020). A myriad of volatile organic compounds (VOCs) can be photochemically oxidized through a complex series of chemical reactions involving nitrogen oxides (), leading to tropospheric O3 formation (Haagen-Smit, 1952; Monks et al., 2015; Seinfeld and Pandis, 2016). The complex O3–NOx–VOC chemical relationship results in local nonlinear O3 formation, which is sensitive to the relative abundances of its precursor species (NOx and VOCs) that are generally categorized as “NOx-limited” versus “radical-limited” photochemical regimes (Sillman et al., 1990; Kleinman, 1994). In a NOx-limited regime, local O3 production increases/decreases with increased/reduced NOx emissions and concentrations, with no impact from VOC perturbations. Whereas, in a radical-limited regime (also known as “VOC-limited”, “hydrocarbon-limited”, or “NOx-saturated”), the formation of local O3 increases/decreases with increased/reduced VOC emissions and concentrations; however, it can also be impacted slightly by NOx emission and concentration changes. The accurate knowledge of regional and local O3 photochemical regimes is critical for developing emission-control strategies to reduce surface O3 concentrations. Overall, studying the spatiotemporal evolution of the nonlinear O3–NOx–VOC chemistry is critical to policy decision-making (National Research Council, 1991) and important as a fundamental scientific problem (Sillman, 1999).
Diagnosing regional and local O3 photochemical regimes has always been recognized as a challenging task. Measurements of proxy or indicator species (e.g., total reactive nitrogen, formaldehyde (HCHO), hydrogen peroxide, and nitric acid), and estimating the correlations of such species, are the observation-based or model-observation-synthesis approaches to detect O3-sensitivity regimes (Sillman, 1995; Jacob et al., 1995; Tonnesen and Dennis, 2000). These measurements and associated studies are typically limited to field campaign time periods and locations which hinder the spatiotemporal coverage of such data, posing an obstacle for investigating global and regional O3 production sensitivity over multi-year time periods. The response of O3 formation to changes in precursor emissions can also be assessed through modeling approaches such as source-apportionment studies (Li et al., 2012), forward-model sensitivity simulations (Wu et al., 2009), and simulations using adjoint model capabilities (Zhang et al., 2009). However, uncertainties inherent in model predictions of O3 physicochemical processes are a critical issue. Milford et al. (1994) and Sillman (1995) first introduced the concept of detecting photochemical regimes using the ratio of ambient concentrations of two “indicator species”, HCHO to NO2 (hereafter, indicator species refer to HCHO and NO2; the ratio of these two species is denoted FNR), which can be used to represent VOCs and NOx, which are directly involved in O3–NOx–VOC chemistry and are readily measured via in situ sampling and satellite remote-sensing techniques. These two indicator species are the most suitable candidates for tropospheric column and planetary boundary layer (PBL) O3-sensitivity analysis using satellites due to (1) the sensitivity of nadir-looking satellites to boundary-layer FNRs; (2) most other indicator species (e.g., hydrogen peroxide and VOCs other than HCHO) cannot be readily measured via satellites, and the retrievals of those species have less sensitivity to surface conditions (e.g., limb-scanning satellites); and (3) the short atmospheric lifetime of HCHO and NO2 allows these species to be suitable for proxies for surface emissions of NOx and VOCs.
Surface and PBL O3 production sensitivity diagnosed with the in situ measurements of FNRs (although sparse in spatial and temporal coverage) should be more accurate compared to satellite-based approaches of retrieving column-integrated concentrations (Schroeder et al., 2017); however, the spatiotemporal coverage of polar-orbiting satellites is a clear advantage over in situ techniques. The advancements in satellite remote sensing over the last 2 decades for retrieving HCHO and NO2 vertical column density (VCD) data (Burrows et al., 1999; González Abad et al., 2019) have emerged as a new observation-based tool to detect the spatiotemporal evolution of O3 sensitivity from a global to local scale (Martin et al., 2004; Jin et al., 2020). Martin et al. (2004) first demonstrated the capability of FNR VCDs from the Global Ozone Monitoring Experiment (GOME) satellite to detect photochemical regimes. Subsequently, this technique was adopted by more studies using other satellite instruments such as the Ozone Monitoring Instrument (OMI), GOME-2, and the TROPOspheric Monitoring Instrument (TROPOMI) (Duncan et al., 2010; Witte et al., 2011; Choi et al., 2012; Choi and Souri, 2015; Chang et al., 2016; Jin and Holloway, 2015; Souri et al., 2017; Jin et al., 2017, 2020; Wang et al., 2021; Tao et al., 2022; Johnson et al., 2023; Acdan et al., 2023) – up to the point that the results have been suggested to potentially be used to inform State Implementation Plans (SIPs) in the United States (US) (Jin et al., 2018). However, the accurate diagnosis of surface O3-sensitivity regimes is impeded by numerous uncertainty components which can be broadly classified into two major categories: (1) inherent uncertainties associated with the approach of relating indicator species to diagnose local O3 sensitivity at a location/time period and (2) uncertainties associated with satellite-retrieved column-integrated concentrations of indicator species to infer surface O3 sensitivity. The former uncertainty arises from numerous factors, including the difficulties in identifying accurate FNR “threshold” values (hereafter, threshold refers to threshold ratio values) separating different O3-sensitivity regimes over a location and time period (Schroeder et al., 2017; Jin et al., 2017); dependence of ambient O3 and its formation to factors other than precursor species such as water vapor, meteorology, deposition, transport, and aerosol interaction (e.g., Kleinman et al., 2005; Camalier et al., 2007); varying sensitivity of HCHO VCD data to anthropogenic VOCs (Jin et al., 2020); and dependence of NO2 in the production of ambient HCHO concentrations (Souri et al., 2020). These inherent uncertainty sources limit the utility of satellite-based data for diagnosing O3-sensitivity regimes. Fortunately, recent studies have investigated these discrepancies in the methodology of using satellite-derived FNRs to infer O3-sensitivity regimes using data from airborne campaign data and 0-D photochemical box models (e.g., Schroeder et al., 2017; Souri et al., 2020, 2023a).
This study investigates 17 years (2005–2021) of OMI satellite sensor data which provide consistent near-daily global coverage of VCD retrievals of HCHO and NO2 (Levelt et al., 2018) that are well suited to the investigation of the long-term spatiotemporal evolution of O3-sensitivity regimes. Numerous studies have used OMI VCD data up to the year 2016 to assess the trends in FNR values over specific regions, mostly over the US and East Asia (Mahajan et al., 2015; Jin and Holloway, 2015; Souri et al., 2017; Jin et al., 2017, 2020). Extending the OMI data set out to 2021 is novel and allows for the investigation of COVID-19 lockdown restrictions on FNRs throughout the Northern Hemisphere. In this study, we investigate the capability of VCD HCHO, NO2, and FNR data from OMI to reflect the trends in PBL and surface-level O3 production sensitivity regimes. We do not calculate actual magnitudes of surface HCHO, NO2, and FNRs derived with OMI VCD data as these proxy products are heavily reliant on chemical transport models (CTMs) and spatiotemporally sparse ancillary information, which are sources that have large uncertainties (discussed in Sect. 4). Satellite-retrieval errors can be reduced by averaging satellite data temporally (seasonal, annual, or multi-year means) and spatially (by averaging individual satellite pixels across tens to hundreds of kilometers), although such averaging approaches preclude the analysis of O3-sensitivity regimes at high spatiotemporal scales. Several studies have therefore focused on assessing O3 production sensitivity using spatially averaged satellite data aggregated to monthly, seasonal, or multi-year means over large areas (e.g., Jin et al., 2020). In this study, we investigate the long-term changes in summer mean (June, July, and August – JJA) VCD FNRs across numerous polluted cities (cities with high-NO2 VCDs) in the Northern Hemisphere. This paper is structured in the following way. Section 2 describes the OMI retrievals, surface concentration measurements, “bottom-up” emission inventories, and the approach to conducting spatiotemporal variability and trend analysis. In Sect. 3, we describe the comparison of satellite VCD FNRs to surface measurements and the analysis of OMI-derived FNR values over Northern Hemisphere cities. Section 4 discusses the capabilities and issues with applying satellite-derived FNRs for studying O3 production sensitivity, and concluding remarks are provided in Sect. 5.
2.1 OMI satellite sensor
The OMI sensor is a Dutch–Finnish-built payload on the NASA Earth Observing System Aura satellite. The Aura platform flies as part of the Afternoon Train (A-Train) satellite constellation along a sun-synchronous polar low-Earth orbit (Schoeberl et al., 2006). Aura passes through the sunlit part of the Earth 14 times a day, with a local overpass time of ∼ 13:45 LT (local time) at the Equator and with near-complete daily global coverage (Levelt et al., 2006). OMI is a nadir-viewing solar-backscatter-grating spectrograph which takes retrievals in the ultraviolet (264–311 nm [UV1] and 307–383 nm [UV2]) and visible (Vis) (349–504 nm) wavelengths (Levelt et al., 2006, 2018; Schenkeveld et al., 2017). The OMI instrument has a swath width of 2600 km (60 pixels across-track), with a near-nadir spatial resolution of 13 km (along-track) × 24 km (cross-track) and near-swath edge pixel size of 40 km×250 km. OMI has been widely used by the atmospheric science, air quality, and health impact assessment communities since its launch on 15 July 2004 (e.g., Levelt et al., 2018). The “row anomaly” appeared in May 2007, affecting the data quality of certain rows of OMI pixels (Dobber et al., 2008; Schenkeveld et al., 2017), and is avoided in the data products used in this study.
2.1.1 OMI HCHO
This study applies the NASA-released operational OMI HCHO version 3, collection 3 (OMHCHO), gridded-level 3 (L3) VCD data at a spatial resolution of 0.1°×0.1° latitude×longitude, using the Smithsonian Astrophysical Observatory (SAO) retrieval algorithm (González Abad et al., 2015). The OMHCHO retrieval applies a nonlinear fitting to the OMI-measured backscattered radiances in the UV2 spectral window and follows the basic optical absorption spectroscopy method (Chance, 1998) to get slant column densities (SCDs). The SCDs are then converted to VCDs by applying the air mass factor (AMF) formulation of Palmer et al. (2001), with scattering weights calculated using the Vector LInearized Discrete Ordinate Radiative Transfer (VLIDORT) version 2.4RT (VLIDORT) radiative transfer model (RTM) (Spurr, 2006) and with HCHO shape factors simulated using the GEOS-Chem global CTM at a spatial resolution of 2° × 2.5° latitude × longitude. The OMHCHO VCD product has a postprocessing bias correction (De Smedt et al., 2018) applied by comparing daily HCHO VCDs with background VCDs simulated with GEOS-Chem over a clean region in the Pacific Ocean (González Abad et al., 2015). González Abad et al. (2015) estimated the uncertainty in the OMHCHO product ranging from 45 % to 105 %, with relative contributions from the slant column retrievals (45 %–100 %) and AMF calculations (∼ 35 %). Model evaluation studies have shown that CTMs have errors and uncertainties in their predictions of HCHO in the clean regions of the Pacific Ocean which could also contribute to overall OMI HCHO bias/errors (Anderson et al., 2017). Validation of OMHCHO with aircraft-based observations indicates a high bias (66.1 %–112.1 %) for HCHO-poor environments and low bias (−44.5 % to −21.7 %) for HCHO-rich environments (Zhu et al., 2016, 2020; Johnson et al., 2023). The OMHCHO product has been used widely for estimating trends of VOC emissions (e.g., Marais et al., 2012; Shen et al., 2019) and inferring surface HCHO concentrations (Zhu et al., 2017a).
2.1.2 OMI NO2
The NASA-released standard OMI NO2 (OMNO2) version 4, collection 3, gridded L3 high-resolution VCD data at the spatial resolution of 0.1°×0.1° latitude×longitude was applied in this study (Lamsal et al., 2015; Krotkov et al., 2017). The OMNO2 retrieval uses the differential optical absorption spectroscopy method (Marchenko et al., 2015) to derive tropospheric SCDs by spectrally fitting OMI-detected backscattered radiance in the visible wavelength window with a pseudo-reference spectrum (Chance and Spurr, 1997). The stratospheric contribution of the SCD is then subtracted, and the residual tropospheric SCDs are then converted to tropospheric VCDs by applying an AMF based on scattering weights calculated using the Total Ozone Mapping Spectrometer (TOMS) radiative transfer model (TOMRAD) (Dave, 1964) and shape factor profiles simulated using the Global Modeling Initiative (GMI) CTM at a spatial resolution of 1°×1.25° latitude×longitude (Krotkov et al., 2017). The uncertainty in the OMNO2 VCD product varies with cloudiness and pollution levels but is in the range of ∼ 20 %–60 % (Bucsela et al., 2013), with relative contributions from the spectral fitting (∼ 10 % over polluted regions; Boersma et al., 2011), stratospheric correction (< 5 %), and AMF calculations (10 %–20 %). The OMNO2 product has been used for a wide range of applications, including the estimation of spatiotemporal variability and trends of NOx emissions (e.g., Krotkov et al., 2016), NO2 surface concentrations (e.g., Kharol et al., 2015; Lamsal et al., 2015), information about atmospheric particulate organic matter (Philip et al., 2014), and surface O3-sensitivity regime detections (e.g., Duncan et al., 2010; Jin et al., 2017).
2.1.3 Calculation of VCD FNR values
The daily L3 OMHCHO and OMNO2 products were filtered and processed for calculating FNR values. Both the operational products were already filtered for daily VCDs with an effective cloud fraction > 30 % solar zenith angles of > 70 % (for HCHO) and > 85 % (for NO2), and pixels affected by row anomalies and L2 data-quality flags not designated as good were removed (see more details in the user's guides for OMNO2 (https://disc.gsfc.nasa.gov/datasets/OMNO2d_003/summary, last access: 11 December 2023) and OMHCHO (https://acdisc.gesdisc.eosdis.nasa.gov/data/Aura_OMI_Level3/OMHCHOd.003/doc/README_OMHCHOd_v003.pdf, last access: 11 December 2023). During this study, we avoided unrealistically large positive and negative values for both indicator species which occur due to uncertainties in slant column retrievals and the calculation of tropospheric VCDs. We followed Zhu et al. (2020) to filter out HCHO daily VCDs outside the range of to 7.6×1016 molec. cm−2. The OMNO2 L3 product already included an upper limit of 1×1017 molec. cm−2, and we applied a lower limit of molec. cm−2 below which NO2 VCD are assumed to be unrealistic in this study. After data filtering, OMI VCD FNRs are calculated by taking the ratio of HCHO:NO2 for each grid of the summer mean products.
2.2 Surface measurement data
To determine if OMI VCD FNRs can replicate the trends of PBL and surface-level FNRs, long-term trends in OMI-derived VCD HCHO and NO2 are compared to in situ measurement data from the United States Environmental Protection Agency's Air Quality System (US EPA AQS; https://www.epa.gov/aqs, last access: 16 June 2023). We focus this evaluation on the US due to the much denser in situ measurement networks compared to other global regions. Hourly data from the EPA AQS NO2 data were averaged daily from 13:00 to 15:00 LT to be consistent with the OMI overpass time. Since there is insufficient hourly data for HCHO from the EPA AQS network, we use 24 h average data for the HCHO evaluation which are provided by the EPA. AQS data for HCHO and NO2 from each site are only used for days on which both species are measured. Valid and continuous data points were then averaged to obtain seasonal summertime-mean (JJA) values from 2005 to 2019 to be intercompared with corresponding OMI VCD values.
The AQS NO2 data suffer from the potential interference of reactive nitrogen species while measuring NO converted from NO2 in molybdenum catalytic converters, since other reactive species also get converted to NO. We attempted to account for this interference by applying a model-simulated correction factor (CF; Eq. 1) to the raw AQS data, following the approach of previous studies (Lamsal et al., 2008, 2010; Cooper et al., 2020).
The CF is calculated using the MERRA2-GMI-simulated concentrations of NO2, HNO3, peroxyacetyl nitrate (PAN), and alkyl nitrates and applying an assumed effective conversion efficiency of 15 % for HNO3 and 95 % for PAN (see Lamsal et al., 2010). The AQS method for measuring HCHO is affected by interference from species such as O3 and NO2, and since there is insufficient information to correct those interferences, here we use uncorrected AQS HCHO data.
2.3 Surface emissions of NOx
To compare the long-term evolution of FNRs with human-induced changes in precursor emissions (anthropogenic emissions of NOx), we used the most recent Community Emissions Data System (CEDS v_2021_04_21) NOx bottom-up emission data set (McDuffie et al., 2020). As explained in Sect. 3 of this work, we focus our analysis on trends in NOx emissions instead of HCHO as it was determined that trends in NO2 concentrations clearly drive the global trends in FNRs. The CEDS data provide monthly anthropogenic NOx emissions at 0.5°×0.5° horizontal spatial scales from 1750–2019. For this study, we analyze trends in anthropogenic NOx emissions (source sectors include (1) agriculture; (2) energy; (3) industrial; (4) transportation; (5) residential, commercial, and other; (6) solvent production and application; (7) waste; and (8) international shipping) between 2005 and 2019 to overlap with OMI observations. We used mean emissions for summer months (JJA) for each year to intercompare with OMI-derived NO2 and FNR trends. Since our focus in this study was to assess the overall relationship of long-term changes in OMI-derived FNR values and corresponding changes in the anthropogenic NOx over a city/region, we do not consider other natural sources (e.g., biomass burning) contributing to ambient concentrations of urban NO2.
2.4 Spatiotemporal analysis of FNRs
The spatiotemporal analysis of OMI-derived VCD NO2 and HCHO values was conducted as follows. First, summer mean trends from 2005 to 2021 of HCHO and NO2 VCDs and FNR values were calculated at the native spatial resolution (0.1°×0.1°). Long-term trends were calculated for each grid of HCHO, NO2, and FNRs with an ordinary least squares linear regression (at various confidence levels calculated with the Mann–Kendall test), similar to past studies (e.g., Boys et al., 2014; Kharol et al., 2015; Geddes et al., 2016). To reduce retrieval random errors and improve precision, we focus on summer mean data for each year and multi-year means (3 multi-year means for 2005–2010, 2011–2015, and 2016–2021) around 46 cities across the Northern Hemisphere. The focus on the summer season was also chosen to utilize HCHO VCD retrievals with significantly better signal-to-noise ratios compared to the winter, spring, and fall months. High levels of surface HCHO concentrations over source regions form due to the higher-oxidant availability in summer (González Abad et al., 2015; Zhu et al., 2014, 2017a, b), which leads to better retrievals of HCHO VCDs. We restrict our analysis to the Northern Hemisphere, as most continental polluted regions exist there. We assessed the evolution of FNRs over urban and rural/suburban (hereinafter referred to just as rural) areas around cities. To define urban city regions, we used the hybrid data set, CGLC-MODIS-LCZ (Demuzere et al., 2023), which is based on the Copernicus Global Land Service Land Cover (CGLC) product resampled to the MODIS International Geosphere–Biosphere Programme (IGBP) classes (CGLC-MODIS) and the global map of local climate zones (LCZs) (Stewart and Oke, 2012) that describe the heterogeneous urban land surface. This data set was interpolated onto a 0.1°×0.1° grid to match the resolution of the L3 OMI satellite data. Urban classification is defined by the CGLC-MODIS-LCZ land-use categories 51–60, including a range of urban land use from sparsely built to compact high-rises and the heavy industry category. These land-use categories capture both urban and suburban landscapes. Rural grids are those not defined as urban and within 7×7 grid boxes of the city center. The CGLC-MODIS-LCZ urban and rural maps derived for this study are static and will not capture urban expansion which has occurred over the last 2 decades. However, since our urban classification includes both urban and suburban landscapes (including sparsely built-up areas), the transition from the suburban to urban landscapes will already be included in our urban map. The only thing not captured would be the transition from completely vegetative areas to more built-up landscapes, which are expected to have a minor impact on the results of this study.
In this study, we focus only on the spatiotemporal variability in the indicator ratios rather than the exact ozone-sensitivity regimes which can be inferred from these ratios. Although several previous studies assigned ratio values to certain O3 regimes (e.g., Jin et al., 2017; Souri et al., 2017) based on previous modeling and limited-observation studies, large uncertainty exists in the classification of O3 regimes using FNR values (Schroeder et al., 2017; Jin et al., 2020; Souri et al., 2023a). Nevertheless, whenever the ratio values were assessed over a city/region, we also presented the threshold ratio values (separating O3 regimes) suggested by Jin et al. (2020) for some cities in the US (Los Angeles, New York, Chicago, Washington, D.C., Pittsburgh, Atlanta, and Houston), suggested by Wang et al. (2021) for cities in China, and suggested by Duncan et al. (2010) for all other cities/regions. Note that the threshold FNR values (<1 as radical-limited versus >2 as NOx-limited) suggested by Duncan et al. (2010) is a crude approximation as opposed to more recent and observationally constrained threshold ratio values suggested by Jin et al. (2020) and Wang et al. (2021). We believe that an accurate classification of O3 regimes is still an ongoing research topic (Schroeder et al., 2017; Jin et al., 2020; Souri et al., 2021) which should be addressed in future studies.
3.1 Long-term mean OMI data
Figure 1 shows the long-term mean (2005–2021) maps of OMI-derived VCDs of HCHO and NO2 and the corresponding column FNR values. Formaldehyde enhancements reflect surface emissions of anthropogenic VOC (densely populated regions in China; Shen et al., 2019), biogenic isoprene (southeastern US; Millet et al., 2008), and biomass burning (South Asia; Mahajan et al., 2015). OMI VCD NO2 is abundant over urban areas, primarily due to fossil fuel combustion emissions from traffic (Duncan et al., 2010) and over regions with large industrial activities (Krotkov et al., 2016). The column FNRs clearly reveal lower values over cities (FNR<2), marginal values over rural/suburban regions surrounding large cities (FNR in the range of 2–5), and higher values elsewhere (FNR>5). The lower FNRs over cities suggest radical limited conditions, and larger FNR values in non-polluted background regions reflect NOx-limited conditions (Martin et al., 2004; Duncan et al., 2010; Jin et al., 2017, 2020; Wang et al., 2021). Lower FNR values retrieved by OMI are most noticeable in the highly populated regions of the US (e.g., Los Angeles, New York, and Chicago), Europe (e.g., London, Amsterdam, and Paris), East Asia (e.g., Beijing, Shanghai, and Jinan), and the Middle East (e.g., Dubai, Tehran, and Riyadh), where tropospheric column NO2 abundances are enhanced. The highest FNR values are observed in regions of the southeastern US and southern Asia (e.g., Malaysia), where there are no large cities, and enhanced tropospheric column HCHO abundances, primarily from biogenic emissions, are observed.
3.2 Capability of OMI VCD data to observe surface-level FNR trends
Before assessing VCD FNR trends, we compared trends in OMI NO2 and HCHO VCD data, and corresponding tropospheric column FNRs, to surface in situ measurements from EPA AQS in the US in order to determine whether OMI VCD information tracks trends occurring at the surface. Figure 2 shows the 15-year time series (2005–2019) comparison between the normalized time series of OMI VCD indicator species abundances and FNRs and AQS data over selected cities (US cities with continuous AQS data) and over all cities averaged across the continental US (373 separate sites). Table 1 shows the correlation between OMI VCD and AQS in situ NO2, HCHO, and FNR summer mean values in addition to the simple linear regression slope of normalized trends from OMI and AQS for both indicator species and FNRs. Figure 2 shows that both OMI VCD and in situ AQS data have relatively neutral trends in HCHO between 2005 and 2019 for most of the large urban cities of the US. While there is large interannual variability in HCHO concentrations, the long-term trends are relatively flat. On average, the normalized linear trends in surface HCHO in urban regions of the US were −0.05 yr−1, and OMI VCDs were +0.15 yr−1. OMI VCD HCHO data are unable to replicate the interannual variability and long-term trends of surface data displayed by the low-correlation values and opposing trends in multiple large cities in the US. The inability of OMI to reflect the variability in HCHO observed at the surface is likely due to the coarse spatial resolution of the OMI footprint, large noise in OMI HCHO retrievals (e.g., Johnson et al., 2023; Souri et al., 2023a), and complex vertical distributions of HCHO complicating satellite retrievals and the representation of surface values (e.g., Souri et al., 2023b).
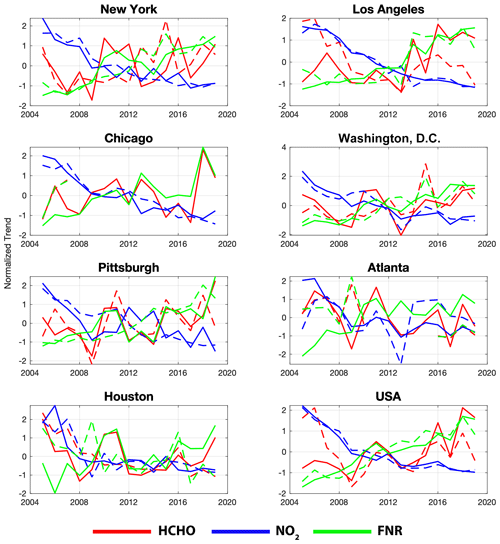
Figure 2Normalized time series (values from 2005 to 2019 normalized to the 2005–2019 mean) of summer mean OMI HCHO and NO2 VCDs and column FNRs (solid lines). The same information is shown for surface concentrations from the EPA AQS in situ observations (dashed lines) over selected cities and over all urban monitoring sites in the United States (bottom-right panel).
Table 1Statistics of the correlation of OMI and AQS (“Obs.”) normalized trends for HCHO, NO2, and FNRs for major cities in the US and the average of all cities in the US (US urban areas) between 2005 and 2019. Slopes of the trends for each species (units in yr−1) are also provided. The values in italics are linear regression slopes which are statistically significant at a 95 % confidence level (p≤0.05). NaN stands for not a number.
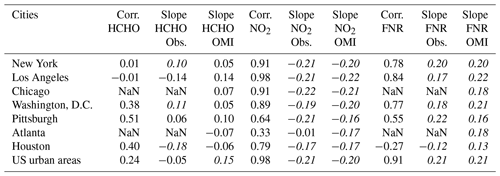
Correlation values are the correlation coefficient (R). NaN values indicate cities for which particular species data are not available for all years between 2005 and 2019.
OMI VCD and in situ AQS data of NO2 display a different story, where statistically significant reductions at a 95 % confidence level in NO2 concentrations are observed by both data sources. The normalized trends in NO2 from both measurement platforms are in strong agreement (see Fig. 2 and Table 1). Correlation between OMI VCD and AQS NO2 was near 1.0 (R=0.98), and both data sources had normalized linear regression slopes of ∼ −0.20. This suggests that OMI is able to observe the strong reduction in NO2 concentrations at the surface measured by AQS across the US. Both data sources suggest that NO2 reduced between 2005 and 2019 and that the strongest negative trends were in the large cities of the US such as New York, Chicago, and Los Angeles. The near-neutral trend in HCHO and large decreases in NO2 result in both OMI VCD and in situ data sources observing an increasing trend in FNR data in all major cities of the US (shown in Fig. 2). The normalized linear regression trend slopes of FNRs (0.21 from both OMI and AQS data) are all statistically significant at a 95 % confidence level and are nearly equal and opposite to NO2, suggesting the reduction in NO2 is the primary driver of FNR trends over time. It is encouraging that OMI VCD data are able to accurately reproduce the normalized trends in surface FNRs in the US. This agrees with the recent studies from Jin et al. (2017) and Souri et al. (2023a) which show that ratios of midday tropospheric VCD FNRs to PBL and surface-level concentrations are near unity. Since OMI VCD FNRs appear to be able to replicate the trends in surface FNRs, the rest of this study focuses on the trends of FNRs from OMI VCD data for the Northern Hemisphere.
3.3 17-year trend in OMI observations
This study investigates the 17-year trend of OMI VCD of HCHO, NO2, and FNR values between 2005 and 2021. Figure 3 shows the long-term trend in OMI VCD HCHO, NO2, and FNR values at an 85 % confidence level (p≤0.15) (Fig. S1 in the Supplement shows the same trend values at a 99 % [p≤0.01] confidence level and for all grid cells with OMI retrievals). The same information shown in Fig. 3 is displayed individually for North America, Europe, and Asia in the Supplement (see Fig. S2). Formaldehyde VCDs increased by ∼ 0.5 × 1014 molec. cm−2 yr−1 over most of the Northern Hemisphere, with reductions up to ∼ −0.5 ×1014 molec. cm−2 yr−1 over the southeastern US. This trend is consistent with previous studies documenting increases in multi-satellite (including OMI) summer mean HCHO over northern China (during 2005–2016, Shen et al., 2019, and 2005–2014, Souri et al., 2017) and increases in most regions in the US (during 2005–2014; Zhu et al., 2017b) due to increasing anthropogenic VOC emissions. Some near-neutral trends, to small even decreases, are seen in Fig. 3 in eastern China. The decrease in HCHO over southern China could be due to reductions in anthropogenic VOCs (Souri et al., 2017; Itahashi et al., 2022) or biogenic VOC emissions, as noted by Jin and Holloway (2015). The decreases in the summer mean OMI HCHO over the southeastern US were also documented in earlier studies (De Smedt et al., 2015; Zhu et al., 2017b). Note that the trend in HCHO calculated in this study is influenced by yearly variations in temperature, in contrast to Shen et al. (2019) and Zhu et al. (2017b), that corrected for the impact of varying temperature on HCHO VCDs.
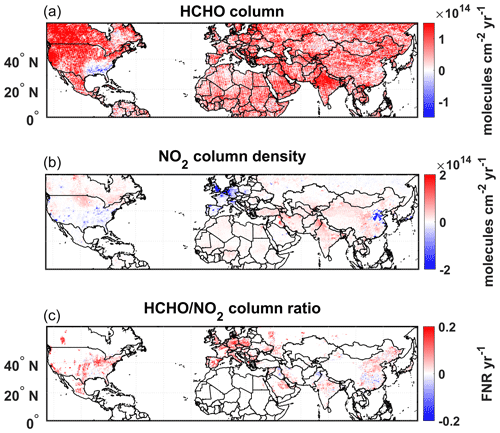
Figure 3OMI-derived trends in the summer mean (June–August) time series of HCHO (a) and NO2 (b) VCDs (units in molec. cm−2 yr−1) and corresponding FNR values (c; unitless yr−1) at the 0.1° × 0.1° latitude × longitude grid cells between 2005 and 2021. Values in panel (c) are displayed only for polluted regions (OMI NO2 molec. cm−2). The white color indicates data gaps or oceanic grid cells. All trend values that are displayed are at an 85 % confidence level (p ≤ 0.15) for better visualization of spatial trend variability. Figure S1 shows the trend values at 99 % confidence level and for all grid cells.
It should be noted that the NASA-released operational OMI HCHO version 3, collection 3, data product used in this study has been shown to have a positive drift due to instrument aging (e.g., Marais et al., 2012; Zhu et al., 2014, 2017b). This positive trend in OMI HCHO data (displayed in Fig. 3) is likely largely impacted by the artificial positive drift in the collection 3 OMI data. A new NASA OMI HCHO version 3, collection 4, product is in development and uses the SAO algorithm which has removed this positive drift in HCHO (Ayazpour et al., 2024; SAO HCHO algorithm team, personal communication, 2024). This new HCHO retrieval product shows that HCHO has a near-neutral trend across most of the populated cities in the Northern Hemisphere. These new collection 4 retrieval data are not yet peer-reviewed or available to the public; therefore, the data are not used here, and the remaining results in this study use OMI HCHO version 3, collection 3, data. However, to test the potential impact on the results of this study using an OMI VCD product with this average positive drift eliminated, we removed the mean annual Northern Hemispheric HCHO trend (∼ 0.004 DU yr−1) from the collection 3 data and evaluate the resulting FNR trends over 18 selected large cities in the Northern Hemisphere (discussed in Sect. 3.4).
The negative trend in NO2 OMI VCDs over populated regions of the US, Europe, and eastern China, and increases in the South Asia and Middle East regions (seen in Fig. 3), are consistent with several previous studies (e.g., Hilboll et al., 2013; Jin et al., 2017). The decreases in eastern China and Europe are as large as −2.0 ×1014 molec. cm−2 yr−1, while reductions in NO2 in the US are between −0.1 ×1014 and −1.0 ×1014 molec. cm−2 yr−1. The decreasing NO2 trend in eastern China could be due to recent reductions in anthropogenic NOx emissions after the year 2011 (e.g., Fan et al., 2021). It is well demonstrated that the tropospheric NO2 decreases in most Northern Hemisphere regions, particularly in urban regions, are due to reductions in anthropogenic NOx emissions implemented through national governmental policies (e.g., Duncan et al., 2010; Koplitz et al., 2021). Figure S3 shows the trends in CEDS anthropogenic NOx emissions between 2005 and 2019, which have nearly identical regions of reduction to those retrieved by OMI NO2. Overall, the summer mean trend in VCD NO2 estimated in this study is generally consistent with the reported satellite-based annual-mean surface NO2 trend estimated on a global scale (Geddes et al., 2016) and over the US (Kharol et al., 2015; Lamsal et al., 2015).
The most notable feature in Fig. 3 is the general increasing trend in OMI VCD FNR values over most of the polluted regions in the Northern Hemisphere. The increasing OMI column FNR values suggest a trend towards more NOx-limited regimes around cities in recent years, which has been noted by some previous studies (Jin et al., 2017, 2020; Souri et al., 2017). Increases in FNRs in the populated regions of China, Europe, and US reach values between 0.1 and 0.2 yr−1. The increases in FNRs are driven mostly by the reductions in NO2 rather than the small variations in HCHO, as evident in Figs. 2 and 3. The following sections focus on the assessment of the evolution of summer mean OMI-derived VCD FNRs over numerous selected cities in the Northern Hemisphere.
3.4 Evolution of OMI FNRs around populated cities in the Northern Hemisphere
Figure 4 shows the time series of summer mean OMI VCD FNRs from 2005 to 2021 over 18 selected large cities. The corresponding normalized time series trends of OMI-derived NO2 abundances and FNRs, and CEDS anthrophonic emissions of NOx over these cities, are displayed in Fig. 5. From Fig. 4 it can be seen that the largest positive trends in OMI FNRs during the 2005–2021 time period occurred over three mega-cities in the US, namely Los Angeles, New York, and Chicago. Time series of the actual magnitudes of OMI VCD NO2 and HCHO abundances over the selected 18 large cities are shown in Fig. S4. In addition to increases in FNRs in US cities, relatively large increases in FNRs are also evident in European (e.g., London) and Asian (e.g., Guangzhou) cities. To test whether the positive drift in the NASA OMI HCHO collection 3 data significantly impacted the results of the FNR trends over the 18 selected large cities in the Northern Hemisphere, we present these same results in Fig. S5 with the OMI data which have the annual average Northern Hemispheric HCHO trend removed (more representative of OMI HCHO version 3, collection 4, data), and Fig. S6 shows the spatial trends of HCHO, NO2, and FNRs over the Northern Hemisphere using these detrended HCHO data. Comparing Figs. S5 and 4, it is seen that while some of the FNR values are slightly lower in magnitude, the positive trends are very similar when using collection 3 HCHO retrievals and a data product with the positive drift removed. Throughout the Northern Hemisphere, HCHO trends now display both positive and negative values (see Fig. S6) instead of the constant positive trends from the OMI HCHO collection 3 product. Using the detrended OMI HCHO data does result in more negative FNR trends in remote regions outside of large urban regions; however, over urban areas, and rural regions surrounding large cities, the FNR trends are still positive, as displayed in Figs. S5 and S6. Overall, using the OMI HCHO version 3, collection 3, data product does not significantly impact the FNR results in large cities in the Northern Hemisphere that are focused on in this study. Future studies investigating FNRs conducted when the NASA OMI HCHO version 3, collection 4, data are available to the public should, however, use this new product to present more accurate results compared to those shown here using the NASA OMI HCHO version 3, collection 3, product.
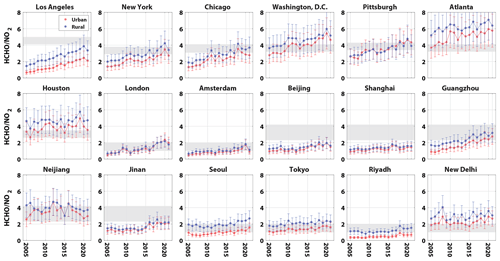
Figure 4Time series of OMI-derived summer mean (June–August) FNR VCD values for 18 selected cities across the Northern Hemisphere from 2005 to 2021. The different colors illustrate mean FNR values for urban (red) and rural areas around each city (blue). Grey shaded areas represent the transition zone of the ozone production sensitivity regime threshold values as suggested by Jin et al. (2020) (cities in the United States include Los Angeles, New York, Chicago, Washington, D.C., Pittsburgh, Atlanta, and Houston), Wang et al. (2021) (cities in China include Beijing, Shanghai, Guangzhou, Neijiang, and Jinan), and Duncan et al. (2010) (other cities). For interpretation, FNR values that are less than the transition zone have O3 production which is VOC-limited, and FNR values larger than the transition zone have O3 production which is NOx-limited.
Figure 5 reveals that the increases in OMI FNR values over the selected 18 mega-cities are linked with decreases in NO2 abundances due to reductions in anthropogenic NOx emissions. The spatial map of trends in the CEDS anthrophonic emissions of NOx across the Northern Hemisphere between 2005 and 2019 are shown in Fig. S3 (time series of CEDS NOx emission magnitudes for the selected 18 mega-cities shown in Fig. S7). Based on the O3 production sensitivity regime thresholds suggested by Jin et al. (2020) (note that these thresholds are applicable for VCD data), all of the US cities shown in Fig. 4 that were VOC-limited in the early 2000s show a clear transition towards NOx-limited and transitional regimes in recent years. Major cities in Europe such as London and Amsterdam have also experienced increasing FNRs moving from VOC-limited regimes to transitional, or even NOx-limited, regimes in recent years (based on thresholds from Duncan et al., 2010). Increases in the magnitudes of FNRs were generally smaller in large cities of Asia; however, only Neijiang does not display some noticeable increases in FNRs in recent years. In Neijiang, CEDS anthropogenic NOx emissions are decreasing after 2012; however, OMI does not retrieve decreasing NO2 abundances, leading to the near-neutral trend in FNR values. Based on the O3 production sensitivity regime thresholds defined by Wang et al. (2021) and Duncan et al. (2010), major cities in Asia have FNR values which are in the transitional or NOx-limited regimes in recent years, except for Beijing, Shanghai, Jinan, and Riyadh (surrounding rural region is in the transitional regimes) (see Fig. 4). Figure 5 shows that these large Asian cities, except for Riyadh, implemented NOx emission-control strategies in ∼ 2012 and have recent negative trends in OMI NO2; however, based on Wang et al. (2021), these urban regions have O3 production which is still limited by VOCs. Overall, it is difficult to conclude if these major cities in the Northern Hemisphere have in fact transitioned to NOx-limited and transitional regimes due to the large uncertainties in the exact threshold FNR values which separate these chemical regimes.
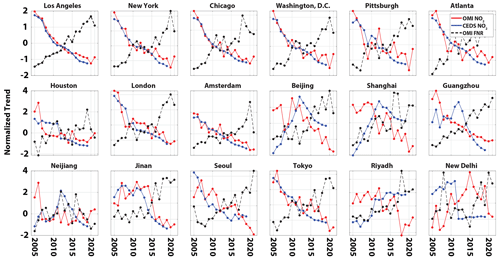
Figure 5Time series of normalized OMI-derived summer mean (June–August) VCD NO2 and FNR trend values and corresponding trends in anthropogenic emission of NOx from the CEDS bottom-up inventory over the selected 18 cities across the Northern Hemisphere from 2005 to 2021. CEDS emission data are only displayed until 2019 due to this being the most recent year of availability.
In the vast majority of cities, OMI retrieved larger FNR values in the rural regions surrounding urban regions in the Northern Hemisphere compared to the urban city centers between 2005 and 2021. Figure 6 shows spatial maps of OMI-derived VCD FNRs around the selected 18 cities discussed above for two 6-year averages, 2005–2010 and 2015–2021, reflecting the earliest and most recent years of OMI data studied here. The spatial maps of OMI-derived HCHO and NO2 VCD values for these same time periods over the 18 cities are displayed in Figs. S8 and S9, respectively. Figure 6 shows that OMI is able to retrieve the differences in FNRs in urban and rural regions surrounding large cities in the Northern Hemisphere (Fig. S10 shows the same information in Fig. 6, except with the CGLC-MODIS-LCZ urban grids used to separate urban and rural values). In the urban areas of cities, where emission sources of NOx are largest, OMI FNRs tend to be lower, indicating more tendency towards VOC-sensitive O3 production regimes compared to the surrounding rural regions. This figure also displays the decadal-scale changes (2016–2021 versus 2005–2010) in the OMI FNRs over the Northern Hemisphere urban regions and surrounding rural regions. In all 18 cities, FNR values increase in both rural and urban areas with noticeable increases in the spatial coverage of potentially NOx-limited O3 production regimes. These spatial distributions of increasing FNR values retrieved by OMI are clearly correlated with decreasing tropospheric NO2 over the vast majority of cities displayed in Fig. S9. Large cities in the US show the clearest increase in the spatial coverage of potentially NOx-limited O3 production regimes; however, European and Asian cities also follow a similar pattern with a smaller increase in the FNR magnitude overall. Recent studies have also noted that NOx-limited regimes have expanded spatially into the city centers, on a decadal-scale, throughout the Northern Hemisphere (Jin et al., 2017) and in the US (Jin et al., 2020). This has large implications for O3-sensitivity analysis and the development of future emission-control strategies for improving air quality.
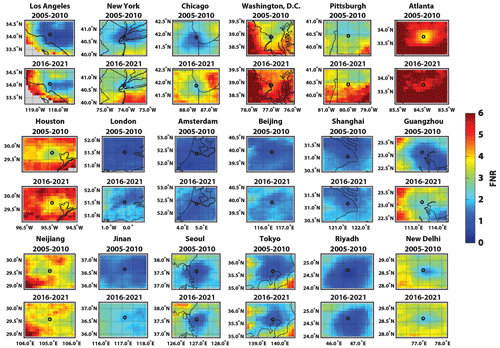
Figure 6OMI-derived summer mean (June–August) FNR VCD values for 18 selected cities across the Northern Hemisphere during 2005–2010 and 2016–2021. The black circle represents each city center. CGLC-MODIS-LCZ urban grids for each city are displayed in Fig. S10. Grey coloring indicates data gaps or oceanic grid cells.
Figure 7 shows the changes in OMI FNRs (multi-year-averaged values for 2005–2010, 2011–2015, and 2016–2021) over 46 cities in the Northern Hemisphere. The vast majority of urban regions in the Northern Hemisphere (44 of the 46 selected cities) experienced increasing FNRs between 2005 and 2010 and 2016–2021. OMI FNRs for Tehran, Iran, and Neijiang, China, were two selected cities which did not display increasing values. On average, FNRs in urban areas of the selected cities increased by ∼ 65 % between 2005–2010 and 2016–2021. Similar to urban regions, the vast majority of rural regions surrounding urban areas in the Northern Hemisphere (44 of the 46 selected cities) experienced increasing FNRs between 2005–2010 and 2016–2021. The average increase in FNRs in the rural regions increased slightly less (∼ 38 %) compared to urban areas. In agreement with the results discussed above, FNR values in rural regions are larger compared to city centers. However, OMI VCD FNR differences between rural and urban regions were reduced by ∼ 15 % on average over the 17-year time period. This suggests that the urban–rural interface of FNRs is becoming less drastic, and NOx-limited O3 production regimes that were predominantly observed in rural regions in the past have expanded into the urban regions of larger cities. More accurate assessment of the actual threshold ratio values separating the different O3 production regimes would allow for the determination of exactly what extent of each city has in fact transitioned to NOx-limited regimes. Overall, Fig. 7 demonstrates that the long-term record of OMI observations can observe the impact of global emission reduction strategies on air quality and O3-sensitivity regimes throughout the Northern Hemisphere.
3.5 Impact of the COVID-19 lockdown on FNRs in the Northern Hemisphere
The global impacts of the COVID-19 lockdown in 2020 on atmospheric pollution, such as the reduction in tropospheric NO2, has been well documented to have impacted O3-sensitivity regimes in the PBL and mid-troposphere to upper troposphere (e.g., Goldberg et al., 2020; He et al., 2020; Cooper et al., 2022; Nussbaumer et al., 2022). Here we studied, for the first time, OMI-derived VCD FNRs to evaluate the impact of the COVID-19 lockdown on summer mean FNRs in 2020 throughout the Northern Hemisphere compared to the years before (2019) and after (2021). Figure 8 shows the changes in OMI FNRs before, during, and after (2019, 2020, and 2021) the COVID-19 lockdown over the 46 selected cities discussed in this study. Out of the 46 selected cities, 32 of the urban regions (∼ 70 %) experienced higher FNRs in 2020 compared to 2019. On average, the cities that experienced increased FNRs in 2020 had values which were ∼ 19 % higher compared to 2019. Similarly, 26 of the urban regions (∼ 57 %) experienced higher FNRs in 2020 compared to 2021, and these city centers had FNR values that were ∼ 18 % larger. OMI also retrieved increased FNR values in rural regions surrounding city centers throughout the Northern Hemisphere during the COVID-19 lockdown period of 2020 (see Fig. 8). As observed for urban areas, a similar number of rural areas experienced increased FNRs in 2020 compared to 2019 and 2021. The increases in FNRs for rural regions in 2020 compared to 2019 and 2021 were 16 % and 13 %. The OMI data evaluated here suggest that the majority of cities in the Northern Hemisphere, and surrounding rural regions, tended to have O3 production which was more sensitive to NOx emissions/concentrations in 2020 compared to the years before and after. Cooper et al. (2022) demonstrated that in 2020 NO2 concentrations were on average ∼ 30 % lower during the COVID-19 lockdown periods, and these reductions were from decreased anthropogenic emissions and cannot be explained by meteorological differences. The degree of reduction in NO2 determined in Cooper et al. (2022) agrees well with the OMI VCD FNR increases determined during our study of ∼ 20 %.
3.6 Comparison of OMI and TROPOMI FNR spatiotemporal variability in US cities
To expand upon previous studies which investigated OMI FNR trends published prior to the availability of TROPOMI retrievals (e.g., Mahajan et al., 2015; Jin and Holloway, 2015; Souri et al., 2017; Jin et al., 2017, 2020), here we compare the ability of OMI and TROPOMI to reproduced inter-city and interannual FNR variability in the US measured by EPA AQS sites in the seven major US cities illustrated in Fig. 2. For this purpose, we applied the TROPOMI operational Royal Belgian Institute for Space Aeronomy (BIRA) L2 HCHO version 2.4.1 and the Dutch OMI NO2 data products of the Royal Netherlands Meteorological Institute (KNMI) for OMI (DOMINO) NO2 version 2.4 retrievals interpolated to a standardized 0.1°×0.1° grid format. Figure 9 shows the normalized summer mean FNRs (city-specific annual FNR values normalized by the seven-city FNR mean) for the seven selected US cities for 2018 and 2019. For both years, TROPOMI was able to reproduce the inter-city variability in normalized AQS FNRs with better agreement compared to OMI for five of the seven US cities. This is further emphasized by the fact that TROPOMI reproduced 48 % and 93 % of the inter-city FNR variance (R2) measured by AQS data for 2018 and 2019, respectively, while OMI only reproduced ∼ 30 % of the FNR variability measured in both years. Furthermore, TROPOMI more closely reproduced the direction of change in AQS measured FNRs between 2018 and 2019 for six of the seven cities (85 %), while OMI was only able to reproduce the FNR differences for three of the seven cities (43 %). The improved capability of TROPOMI to capture spatiotemporal FNR variability compared to OMI is to be expected, as recent studies have demonstrated improved HCHO and NO2 retrievals from the newer- and higher-spatial-resolution sensor (e.g., Souri et al., 2023a; Johnson et al., 2023), and OMI is far past the expected lifetime of the sensor. Future studies should intercompare both of the sensor retrievals of FNRs for the entire lifetime of TROPOMI, which overlaps with OMI (2018–present), to fully understand the improvements when applying TROPOMI.
The OMI satellite sensor offers a continuous data record across the globe, with a sufficient spatiotemporal resolution to assess the tropospheric O3 production sensitivity which cannot be achieved with in situ observations. The analysis in this study demonstrated that the 17-year record of OMI-retrieved HCHO and NO2 data offered an unprecedented opportunity to assess the long-term evolution of VCD, and likely surface-level FNR values, with potential future applications in linking these ratio changes with the changes in surface O3 regimes. Here we show that the OMI VCD data of FNRs replicate the trends observed with surface in situ information. In order to produce actual satellite-derived surface values of HCHO, NO2, and FNRs using VCD retrievals requires algorithms which largely depend on CTM-predicted vertical distributions of these trace gases (e.g., Zhu et al., 2017a; Jin et al., 2017; Cooper et al., 2020). Surface-based and aircraft in situ observations are also used for this purpose; however, these observations have minimal observational coverage due to being very spatiotemporally limited (e.g., Souri et al., 2023a). Using model simulations or in situ data to convert satellite VCD information to surface-level HCHO, NO2, and FNRs concentrations is inhibited by errors. In situ observations are too sparse, and CTMs have system-specific errors/biases and differ largely in their prediction of HCHO and NO2 vertical distributions (Lamsal et al., 2008; Geddes et al., 2016; Souri et al., 2023b). This results in large uncertainties in surface-level FNRs when convolving satellite VCDs. Our study does not address the conversion of OMI VCDs to surface-level values but clearly shows that this spaceborne sensor can capture the trends in surface-level FNRs. The ability of VCD information from low-Earth orbit satellites to capture midday surface-level FNR trends might be due to studies showing that ratios of VCD FNRs to PBL/surface values are near unity (Jin et al., 2017; Souri et al., 2023a). However, during times where HCHO and NO2 vertical profiles in the troposphere are not similar to the climatological averages, models, and satellites may be challenged to accurately assess the conversion ratios of VCD FNRs to PBL/surface values (e.g., Souri et al., 2023b). Overall, if methods are improved to reduce the uncertainty in the conversion of satellite VCD retrievals of HCHO and NO2 to surface-level concentrations, then satellite-derived surface-level FNRs will be able to be applied in higher confidence for air-quality research and potentially policy decisions.
OMI VCD FNR and NO2 retrievals display a high correlation with surface in situ data trends (see Table 1). However, this satellite demonstrated a lesser capability to replicate the trends of surface-level observations of HCHO. The vertical structure of HCHO can be complex, which complicates the relationship between VCDs and surface-level values (Souri et al., 2023b); however, OMI has also been shown to have large systematic and random biases in HCHO retrievals, which drive the overall errors in OMI-derived VCD FNRs (Johnson et al., 2023; Souri et al., 2023a). However, since decreasing NO2 emissions/concentrations driven by NOx emission-control strategies is the primary reason for the increasing trends of FNRs at the surface, while HCHO has near-neutral trends, and OMI NO2 VCDs have much lower errors compared to HCHO (Johnson et al., 2023; Souri et al., 2023a), this study shows that the OMI VCD data of indicator species can still replicate surface-level trends of FNRs. Emission-control strategies for VOCs have also been shown to have caused regional reductions in the concentrations of these compounds; however, it is challenging to derive and assess the impact of VOC emission-control strategies as there are thousands of different VOC compounds all with a different chemical reactivity (Pei et al., 2022). Furthermore, a large fraction of VOCs is emitted from biogenic sources which cannot be controlled through changes in human activities (Guenther et al., 1995). In addition to retrieval errors, the coarse spatial resolution of OMI and other spaceborne sensors results in representation errors when compared to point-source surface observations (Souri et al., 2022). This also likely contributes to the challenge of satellite-derived HCHO, NO2, and FNRs to replicate the trends and variability determined from in situ measurements located in city centers.
Apart from the southeastern US and some small areas of eastern China, OMI HCHO version 3, collection 3, data result in mostly positive trends between 2005 and 2021. A portion of this positive trend could be due to the OMI instrument drift which has been identified in past studies (e.g., Marais et al., 2012; Zhu et al., 2016, 2017b). Currently, there is an OMI HCHO version 3, collection 4, product in development using the SAO algorithm; however, it has yet to be published and therefore could not be applied in this study. A major difference between the collection 3 and 4 data products is how the level 1b (L1b) data are produced, as described in Kleipool et al. (2022). Furthermore, changes in molecular absorption cross sections and input parameters for SCD calculations, AMF calculations, and latitudinal bias corrections are applied in collection 4 OMI HCHO data. Preliminary analysis of the differences in OMI VCD HCHO using the collections 3 and 4 data demonstrates changes in the magnitudes and trends of this species (the SAO OMI HCHO algorithm team, personal communication, 2024). These differences could have an impact on the analysis of trends in global FNRs such as the ones conducted in this study. Once the OMI HCHO version 3, collection 4, product becomes available to the public, the analysis in this study should be redone with the updated HCHO data. Overall, we do not expect that the results in FNR trends will be impacted much due to observed trends in OMI VCD and surface-level NO2 being much larger than that in HCHO (see Sect. 3.2).
Due to the inadequacy of our current quantitative understanding of exact threshold FNR values marking the O3 photochemical regime transitions (e.g., Schroeder et al., 2017), this study avoided explicitly linking OMI FNRs with exact chemical regimes. An accurate diagnosis of the surface O3 sensitivity requires more in-depth analysis of satellite FNRs at a higher spatiotemporal resolution and accurately relating these FNRs to O3 regimes by estimating the threshold ratio values applicable to specific regions and time periods. Global CTM simulations (Jin et al., 2017), photochemical box modeling utilizing measurement data from airborne field campaigns (Schroeder et al., 2017; Souri et al., 2020), and observation-based methods linking ratio values with surface O3 concentrations (Jin et al., 2020; Wang et al., 2021) could lead to the derivation of more accurate regime threshold ratio values. Nevertheless, the OMI-derived VCD FNR values investigated in this study revealed many aspects of O3 sensitivity to NOx versus VOCs. In general, OMI-derived summer FNR values indicated radical-limited regimes within many cities in the Northern Hemisphere (FNRs<2) and NOx-limited regimes over the rural regions around those cities (FNRs>3). The analysis of the multi-year summer mean OMI HCHO and NO2 values revealed a positive trend in FNRs, indicating a transition from radical-limited to NOx-limited regimes, especially during more recent years. The positive trend in OMI FNRs over most cities is mainly due to decreases in NO2 resulting from the decrease in anthropogenic NOx emissions and mixed variations in biogenic VOC sources. This study evaluated FNR trends for 46 large Northern Hemisphere cities and expands on other recent studies which evaluated O3 production sensitivity regimes around smaller numbers of cities (e.g., Jin et al., 2017). Due to the majority of highly populated Northern Hemisphere cities outside of the tropics that appear in developed nations, the increasing trend in FNRs due to anthropogenic emission reductions holds true for the vast majority of the 46 cities studied here.
In the earliest years of studying satellite-derived FNRs, it was hoped that this data source could potentially be used for policy decisions and developing emission-control strategies (Martin et al., 2004). However, more recent studies starting with Duncan et al. (2010) suggested that satellite retrievals may have errors too large for applying FNRs for air-quality regulations. Furthermore, satellite data typically have to be temporally averaged to reduce the noise in the retrievals, which may mask out important O3 exceedance events and the indicator species characteristics on these days (Schroeder et al., 2017). The recent study by Souri et al. (2023a) compiled a comprehensive error budget for using satellite retrievals to assess surface-level FNRs. This study showed that total relative error in satellite FNRs over large cities tends to be ∼ 50 %, whereas, over rural regions, there are much larger errors (> 100 %). The majority of this error comes from noise in satellite retrievals (40 %–90 %), especially from HCHO retrievals, and the rest of this error is associated with the ability of indicator species to accurately describe complex O3 chemistry (∼ 20 %), VCD-to-surface translation (∼ 19 %), and spatial representation (∼ 13 %). These total relative errors are likely too large to apply satellite FNRs for air-quality regulation purposes; however, they still provide a useful scientific research product for investigating long-term and short-term events (e.g., meteorological variations, droughts/floods, wildfires,and socioeconomic events) and impacts of emissions on O3 production sensitivity regimes.
This study applied the 17-year data record of the OMI satellite sensor's summer mean VCD HCHO, NO2, and FNRs between 2005 and 2021 over the Northern Hemisphere to understand the long-term evolution of O3 photochemical regimes. This expands the global OMI record of VCD FNRs out to 2021 – further than previous studies (e.g., Jin et al., 2020). The long-term trends for 46 highly populated cities in the Northern Hemisphere agree with past work, which has shown that FNRs are primarily increasing due to reductions in the emissions/concentrations of NOx (Duncan et al., 2010; Jin et al., 2017, 2020). OMI VCD NO2 data are decreasing in most urban regions of the Northern Hemisphere, while HCHO data are near-neutral or slightly increasing, resulting in the increasing FNR trends. The extension of OMI FNR data out to 2021 suggests a continuing trend towards more NOx-limited O3 production sensitivity regimes within and around cities throughout the Northern Hemisphere.
Another unique finding in our study is the extension of OMI FNR data out to 2021, covering the impact of the COVID-19 lockdown of 2020. Out of the 46 selected cities, ∼ 70 % of urban regions experienced higher FNRs in 2020 compared to 2019, and ∼ 57 % had higher FNRs in 2020 compared to 2021. OMI FNRs were 18 %–19 % higher in 2020 compared to the years before and after the COVID-19 lockdown in 2020. We studied the summer mean FNRs in this study; however, COVID-19 lockdown restrictions were largest in the spring of 2020. Thus, the full impact of COVID-19 lockdown restrictions on VCD FNRs was likely larger than that experienced in the summer. A similar percentage of rural areas around the 46 selected cities experienced higher FNRs in 2020 compared to 2019 and 2021; however, the increases in FNRs were smaller (13 %–16 %) compared to urban areas. The OMI data evaluated here suggest that the majority of cities in the Northern Hemisphere, and the surrounding rural regions, tended to have O3 production which was more sensitive to NOx emissions/concentrations in 2020 compared to the years before and after.
Past studies have shown that midday FNR VCDs are similar to those observed at the surface (Jin et al., 2017; Souri et al., 2023a). However, during times where HCHO and NO2 vertical profiles in the troposphere are complex, models and satellites may be challenged to accurately assess conversion ratios of VCD FNRs to PBL/surface values (e.g., Souri et al., 2023b). This study shows that, on average, the long-term trends of OMI VCD FNRs agree well with observations at the surface in cities distributed around the US, suggesting that this satellite is capable of assessing the long-term trends of surface-level O3 production sensitivity regimes. However, the magnitudes of both indicator species calculated with satellite VCDs using scaling factors derived with CTMs and/or in situ observations are highly uncertain. Both OMI VCD and surface in situ data of HCHO, NO2, and FNRs emphasize that the increasing trend in FNRs is driven by the reduced emissions/concentrations of NO2, while HCHO has a near-neutral trend. While OMI VCD HCHO trends and variability do not agree well with surface in situ observations, OMI does replicate the strong decreasing trend of NO2 observed at the surface, resulting in the agreement between OMI and surface data of FNR trends.
Higher spatiotemporal retrievals from newer low-Earth orbit (e.g., TROPOMI) and geostationary (e.g., Tropospheric Emissions: Monitoring of Pollution [TEMPO] and Geostationary Environment Monitoring Spectrometer [GEMS]) satellite sensors provide more insight into the short-term (daily and diurnal) recent (2017–present) evolution of O3 photochemical regimes. Compared to OMI, TROPOMI was shown to retrieve VCD FNR values with more accuracy and better precision compared to OMI observations, primarily due to improvements in the HCHO product performance (e.g., Johnson et al., 2023). Johnson et al. (2023) demonstrated that TROPOMI can retrieve spatiotemporal HCHO variability with uncertainties low enough to capture FNR variability on a daily basis, while OMI was not. The current study compared the capability of OMI and TROPOMI to capture inter-city and interannual FNR variability measured by ground-based AQS data (using normalized FNR values) for seven selected US cities. Here we quantitatively showed that TROPOMI was able to reproduce the spatiotemporal variability in observed FNRs more accurately compared to OMI for the reasons mentioned above. Future improvements in satellite HCHO retrievals will allow for more accurate retrievals of FNRs on a daily to monthly scale. TEMPO and GEMS provide HCHO and NO2 VCD information at 1 to 3 h temporal resolution and a higher spatial resolution compared to both OMI and TROPOMI, which will allow for the assessment of diurnal FNR variability on a regional scale. This has not yet been possible as TEMPO and GEMS are the first UV-Vis spectrometers on geostationary platforms with a spatial resolution high enough to retrieve air-quality-relevant HCHO and NO2 VCD data. The new diurnal and high-spatial-resolution information from these geostationary satellites is expected to greatly improve the understanding of FNRs. As OMI is set to be decommissioned in the coming years, it is critical to merge TROPOMI HCHO and NO2 VCD data with OMI in order to continue the long-term data set from 2005–present. Furthermore, combining retrieved information from geostationary satellites with once-a-day low-Earth orbit data will provide a vast wealth of information about global daily to hourly variability in FNRs.
While recent studies have shown that OMI FNR retrieval errors are likely too large to apply in air-quality regulation and for deriving emission-control strategies to reduce surface-level O3 concentrations, this long-term satellite product provides a useful scientific research product for investigating atmospheric O3 chemistry and investigating the qualitative impacts of emission changes on O3 production sensitivity regimes. This is especially true for regions of the globe outside of the US and Europe that have limited long-term surface in situ observation networks able to measure HCHO and NO2 concentrations. Improvements in HCHO and NO2 VCD retrieval algorithms and methods to derive VCD-to-surface/PBL conversion factors for these indicator species would greatly improve the ability to apply OMI and other satellite products to study surface air quality. Data assimilation and inverse models have been combined with satellite retrievals of HCHO and NO2 data to constrain the predictions of NOx and VOC emissions and the resulting O3 chemistry (e.g., Souri et al., 2020). These satellite-data-constrained models can then be used to assess trends and variability in FNRs, indicator species emissions, and O3 photochemistry regimes. These improvements in satellite retrieval algorithms, CTMs, data assimilation, and inverse modeling techniques, along with studies to better define the actual O3 production sensitivity regime thresholds, will allow for a more confident investigation of long-term air quality and the impacts of NOx and VOC emission changes on O3 production sensitivity.
The OMI HCHO L3 data used in this paper are publicly available at https://doi.org/10.5067/Aura/OMI/DATA3010 (Chance, 2019). The OMI NO2 High-Resolution L3 data are also available from a public data repository at https://doi.org/10.5067/Aura/OMI/DATA2017 (Krotkov et al., 2019). The CEDS emission inventory used in this work is also publicly available at https://doi.org/10.5281/zenodo.12803197 (Hoesly et al., 2019). EPA AQS data of HCHO and NO2 can be downloaded from https://www.epa.gov/outdoor-air-quality-data (US Environmental Protection Agency, 2023).
The supplement related to this article is available online at: https://doi.org/10.5194/acp-24-10363-2024-supplement.
MSJ and SP obtained the funding for this project. MSJ, SP, and RK played fundamental roles in developing the investigation strategy of this study. MSJ, SP, SM, MSH, and YPS conducted the analysis which produce the results presented in this work. Finally, MSJ and SP were the primary authors who wrote the text of the paper.
The contact author has declared that none of the authors has any competing interests.
The views, opinions and findings of this paper are those of the authors and should not be construed as an official NASA or United States Government position, policy, or decision.
Publisher's note: Copernicus Publications remains neutral with regard to jurisdictional claims made in the text, published maps, institutional affiliations, or any other geographical representation in this paper. While Copernicus Publications makes every effort to include appropriate place names, the final responsibility lies with the authors.
Computational resources were provided by the NASA High-End Computing Program through the NASA Advanced Supercomputing Division at NASA Ames Research Center. We acknowledge the United States Environmental Protection Agency for the free availability of in situ data.
Matthew S. Johnson, Sajeev Philip, Scott Meech, Rajesh Kumar, Meytar Sorek-Hamer, and Yoichi P. Shiga have been supported by the National Aeronautics and Space Administration (grant no. NNH19ZDA001N-AURAST) as part of the Upper Atmosphere Research Program (UARP). Part of this material is based upon work supported by the National Science Foundation (NSF) National Center for Atmospheric Research, which is a major facility sponsored by the U.S. NSF under Cooperative Atmospheric Research (grant no. 1852977).
This paper was edited by Bryan N. Duncan and reviewed by two anonymous referees.
Acdan, J. J. M., Pierce, R. B., Dickens, A. F., Adelman, Z., and Nergui, T.: Examining TROPOMI formaldehyde to nitrogen dioxide ratios in the Lake Michigan region: implications for ozone exceedances, Atmos. Chem. Phys., 23, 7867–7885, https://doi.org/10.5194/acp-23-7867-2023, 2023.
Anderson, D. C., Nicely, J. M., Wolfe, G. M., Hanisco, T. F., Salawitch, R. J., Canty, T. P., Dickerson, R. R., Apel, E. C., Baidar, S., Bannan, T. J., Blake, N. J., Chen, D., Dix, B., Fernandez, R. P., Hall, S. R., Hornbrook, R. S., Gregory Huey, L., Josse, B., Jöckel, P., Kinnison, D. E., Koenig, T. K., Le Breton, M., Marécal, V., Morgenstern, O., Oman, L. D., Pan, L. L., Percival, C., Plummer, D., Revell, L. E., Rozanov, E., Saiz-Lopez, A., Stenke, A., Sudo, K., Tilmes, S., Ullmann, K., Volkamer, R., Weinheimer, A. J., and Zeng, G.: Formaldehyde in the Tropical Western Pacific: Chemical Sources and Sinks, Convective Transport, and Representation in CAM-Chem and the CCMI Models, J. Geophys. Res.-Atmos., 122, 11201–11226, https://doi.org/10.1002/2016JD026121, 2017.
Ayazpour, Z., Abad, G. G., Nowlan, C. R., Sun, K., Kwon, H. A., Miller, C. C., Chong, H., Wang, H., Liu, X., Chance, K. V., O'Sullivan, E., Zhu, L., Vigouroux, C., De Smedt, I., Stremme, W., Hannigan, J. W., Notholt, J., Sun, X., Palm, M., Petri, C., Strong, K., Rohling, A. N., Mahieu, E., Smale, D., Te, Y., Morino, I., Murata, I., Nagahama, T., Kivi, R., Makarova, M., Jones, M. B., and Sussmann, R.: Aura Ozone Monitoring Instrument (OMI) Collection 4 Formaldehyde Product, ESS Open Archive [preprint], https://doi.org/10.22541/essoar.171804891.19520982/v1, 10 June 2024.
Boersma, K. F., Eskes, H. J., Dirksen, R. J., van der A, R. J., Veefkind, J. P., Stammes, P., Huijnen, V., Kleipool, Q. L., Sneep, M., Claas, J., Leitão, J., Richter, A., Zhou, Y., and Brunner, D.: An improved tropospheric NO2 column retrieval algorithm for the Ozone Monitoring Instrument, Atmos. Meas. Tech., 4, 1905–1928, https://doi.org/10.5194/amt-4-1905-2011, 2011.
Boys, B., Martin, R., van Donkelaar, A., MacDonell, R., Hsu, C., Cooper, M., Yantosca, R., Lu, Z., Streets, D. G., Zhang, Q., and Wang, S.: Fifteen-year global time series of satellite-derived fine particulate matter, Environ. Sci. Technol., 48, 11109–11118, 2014.
Bucsela, E. J., Krotkov, N. A., Celarier, E. A., Lamsal, L. N., Swartz, W. H., Bhartia, P. K., Boersma, K. F., Veefkind, J. P., Gleason, J. F., and Pickering, K. E.: A new stratospheric and tropospheric NO2 retrieval algorithm for nadir-viewing satellite instruments: applications to OMI, Atmos. Meas. Tech., 6, 2607–2626, https://doi.org/10.5194/amt-6-2607-2013, 2013.
Burrows, J. P., Weber, M., Buchwitz, M., Rozanov, V., Ladstätter-Weißenmayer, A., Richter, A., DeBeek, R., Hoogen, R., Bramstedt, K., Eichmann, K.-U., Eisingera, M., and Pernerb, D.: The global ozone monitoring experiment (GOME): Mission concept and first scientific results, J. Atmos. Sci., 56, 151–175, 1999.
Camalier, L., Cox, W., and Dolwick, P.: The effects of meteorology on ozone in urban areas and their use in assessing ozone trends, Atmos. Environ., 41, 7127–7137, https://doi.org/10.1016/j.atmosenv.2007.04.061, 2007.
Chance, K.: Analysis of BrO measurements from the Global Ozone Monitoring Experiment, Geophys. Res. Lett., 25, 3335–3338, https://doi.org/10.1029/98GL52359, 1998.
Chance, K.: OMI/Aura Formaldehyde (HCHO) Total Column Daily L3 Weighted Mean Global 0.1deg Lat/Lon Grid V003, Goddard Earth Sciences Data and Information Services Center (GES DISC) [data set], Greenbelt, MD, USA, https://doi.org/10.5067/Aura/OMI/DATA3010, 2019.
Chance, K. V. and Spurr, R. J. D.: Ring effect studies: Rayleigh scattering, including molecular parameters for rotational Raman scattering, and the Fraunhofer spectrum, Appl. Optics, 36, 5224–5230, https://doi.org/10.1364/AO.36.005224, 1997.
Chang, C.-Y., Faust, E., Hou, X., Lee, P., Kim, H. C., Hedquist, B. C., and Liao, K.-J.: Investigating ambient ozone formation regimes in neighboring cities of shale plays in the northeast United States using photochemical modeling and satellite retrievals, Atmos. Environ., 142, 152–170, https://doi.org/10.1016/j.atmosenv.2016.06.058, 2016.
Choi, Y. and Souri, A.: Chemical condition and surface ozone in large cities of Texas during the last decade: observational evidence from OMI, CAMS, and Model Analysis, Remote Sens. Environ., 168, 90–101, https://doi.org/10.1016/j.rse.2015.06.026, 2015.
Choi, Y., Kim, H., Tong, D., and Lee, P.: Summertime weekly cycles of observed and modeled NOx and O3 concentrations as a function of satellite-derived ozone production sensitivity and land use types over the Continental United States, Atmos. Chem. Phys., 12, 6291–6307, https://doi.org/10.5194/acp-12-6291-2012, 2012.
Cooper, M. J., Martin, R. V., McLinden, C. A., and Brook, J. R.: Inferring ground-level nitrogen dioxide concentrations at fine spatial resolution applied to the TROPOMI satellite instrument, Environ. Res. Lett., 15, 104013, https://doi.org/10.1088/1748-9326/aba3a5, 2020.
Cooper, M. J., Martin, R. V., Hammer, M. S., Levelt, P. F., Veefkind, P., Lamsal, L. N., Krotkov, N. A., Brook, J. R., and McLinden, C. A.: Global fine-scale changes in ambient NO2 during COVID-19 lockdowns, Nature, 601, 380–387, https://doi.org/10.1038/s41586-021-04229-0, 2022.
Dave, J. V.: Meaning of successive iteration of the auxiliary equation in the theory of radiative transfer, Astrophys. J., 140, 1292–1303, 1964.
De Smedt, I., Stavrakou, T., Hendrick, F., Danckaert, T., Vlemmix, T., Pinardi, G., Theys, N., Lerot, C., Gielen, C., Vigouroux, C., Hermans, C., Fayt, C., Veefkind, P., Müller, J.-F., and Van Roozendael, M.: Diurnal, seasonal and long-term variations of global formaldehyde columns inferred from combined OMI and GOME-2 observations, Atmos. Chem. Phys., 15, 12519–12545, https://doi.org/10.5194/acp-15-12519-2015, 2015.
De Smedt, I., Theys, N., Yu, H., Danckaert, T., Lerot, C., Compernolle, S., Van Roozendael, M., Richter, A., Hilboll, A., Peters, E., Pedergnana, M., Loyola, D., Beirle, S., Wagner, T., Eskes, H., van Geffen, J., Boersma, K. F., and Veefkind, P.: Algorithm theoretical baseline for formaldehyde retrievals from S5P TROPOMI and from the QA4ECV project, Atmos. Meas. Tech., 11, 2395–2426, https://doi.org/10.5194/amt-11-2395-2018, 2018.
Demuzere, M., He, C., Martilli, A., and Zonato, A.: Technical documentation for the hybrid 100 m global land cover dataset with Local Climate Zones for WRF, https://doi.org/10.5281/zenodo.7670791, 2023.
Dobber, M., Kleipool, Q., Dirksen, R., Levelt, P., Jaross, G., Taylor, S., Kelly, T., and Flynn, L.: Validation of ozone monitoring instrument level-1b data products, J. Geophys. Res., 113, D15S06, https://doi.org/10.1029/2007JD008665, 2008.
Duncan, B., Yoshida, Y., Olson, J., Sillman, S., Martin, R., Lamsal, L., Hu, Y., Pickering, K., Retscher, D., Allen, D., and Crawford, J.: Application of OMI observations to a space-based indicator of NOx and VOC controls on surface ozone formation, Atmos. Environ., 44, 2213–2223, https://doi.org/10.1016/j.atmosenv.2010.03.010, 2010.
Fan, C., Li, Z., Li, Y., Dong, J., van der A, R., and de Leeuw, G.: Variability of NO2 concentrations over China and effect on air quality derived from satellite and ground-based observations, Atmos. Chem. Phys., 21, 7723–7748, https://doi.org/10.5194/acp-21-7723-2021, 2021.
GBD 2019 Risk Factor Collaborators: Global burden of 87 risk factors in 204 countries and territories, 1990–2019: a systematic analysis for the Global Burden of Disease Study 2019, Lancet, 396, 1223–1249, https://doi.org/10.1016/s0140-6736(20)30752-2, 2020.
Geddes, J. A., Martin, R. V., Boys, B. L., and van Donkelaar, A.: Long term trends worldwide in ambient NO2 concentrations inferred from satellite observations, Environ. Health Persp., 124, 281–289, https://doi.org/10.1289/ehp.1409567, 2016.
Goldberg, D. L., Anenberg, S. C., Griffin, D., McLinden, C. A., Lu, Z., and Streets, D. G.: Disentangling the Impact of the COVID-19 Lockdowns on Urban NO2 From Natural Variability, Geophys. Res. Lett., 47, e2020GL089269, https://doi.org/10.1029/2020GL089269, 2020.
González Abad, G., Liu, X., Chance, K., Wang, H., Kurosu, T. P., and Suleiman, R.: Updated Smithsonian Astrophysical Observatory Ozone Monitoring Instrument (SAO OMI) formaldehyde retrieval, Atmos. Meas. Tech., 8, 19–32, https://doi.org/10.5194/amt-8-19-2015, 2015.
González Abad, G., Souri, A. H., Bak, J., Chance, K., Flynn, L. E., Krotkov, N. A., Lamsal, L., Li, C., Liu, X., Miller, C. C., Nowlan, C. R., Suleiman, R., and Wang, H.: Five decades observing Earth's atmospheric trace gases using ultraviolet and visible backscatter solar radiation from space, J. Quant. Spectrosc. Ra., 238, 106478, https://doi.org/10.1016/j.jqsrt.2019.04.030, 2019.
Guenther, A., Hewitt, N., Erickson, D., Fall, R., Geron, C., Graedel, T., Harley, P., Klinger, L., Lerdau, M., McKay, W., Pierce, T., Scholes, B., Steinbrecher, R., Tallamraju, R., Taylor, J., and Zimmerman, P.: A global model of natural volatile organic compound emissions, J. Geophys. Res., 100, 8873–8892, 1995.
Haagen-Smit, A.: Chemistry and physiology of Los Angeles smog, Industrial & Engineering Chemistry, 44, 1342–1346, https://doi.org/10.1021/ie50510a045, 1952.
He, G., Pan, Y. and Tanaka, T.: The short-term impacts of COVID-19 lockdown on urban air pollution in China, Nature Sustainability, 3, 1005–1011, https://doi.org/10.1038/s41893-020-0581-y, 2020.
Hilboll, A., Richter, A., and Burrows, J. P.: Long-term changes of tropospheric NO2 over megacities derived from multiple satellite instruments, Atmos. Chem. Phys., 13, 4145–4169, https://doi.org/10.5194/acp-13-4145-2013, 2013.
Hoesly, R., O’Rourke, P., Braun, C., Feng, L., Smith, S. J., Pitkanen, T., Seibert, J. J., Vu, L., Muwan, P., Bolt, R., Goldstein, B., and Kholod, N.: Community Emissions Data System, Version 2021-04-21, Zenodo [data set], https://doi.org/10.5281/zenodo.12803197, 2019.
Itahashi, S., Irie, H., Shimadera, H., and Chatani, S.: Fifteen-Year Trends (2005–2019) in the Satellite-Derived Ozone-Sensitive Regime in East Asia: A Gradual Shift from VOC-Sensitive to NOx-Sensitive, Remote Sens.-Basel, 14, 4512, https://doi.org/10.3390/rs14184512, 2022.
Jacob, D. J., Horowitz, L. W., Munger, J. W., Heikes, B. G., Dickerson, R. R., Artz, R. S., and Keene, W. C.: Seasonal transition from NOx- to hydrocarbon-limited conditions for ozone production over the eastern United States in September, J. Geophys. Res.-Atmos., 100, 9315–9324, https://doi.org/10.1029/94JD03125, 1995.
Jin, X. and Holloway, T.: Spatial and temporal variability of ozone sensitivity over China observed from the Ozone Monitoring Instrument, J. Geophys. Res.-Atmos., 120, 7229–7246, https://doi.org/10.1002/2015JD023250, 2015.
Jin, X., Fiore, A. M., Murray, L. T., Valin, L. C., Lamsal, L. N., Duncan, B., Boersma, K. F., De Smedt, I., Abad, G. G., Chance, K., and Tonnesen, G. S.: Evaluating a Space-Based Indicator of Surface Ozone-NOx-VOC Sensitivity Over Midlatitude Source Regions and Application to Decadal Trends, J. Geophys. Res.-Atmos., 122, 10439–10461, https://doi.org/10.1002/2017JD026720, 2017.
Jin, X., Fiore, A. M., and Geigert, M.: Using satellite observed formaldehyde (HCHO) and nitrogen dioxide (NO2) as an indicator of ozone sensitivity in a SIP, HAQAST Tech. Guid. Doc. no. 1, https://doi.org/10.7916/D8M34C7V, 2018.
Jin, X., Fiore, A., Boersma, K. F., De Smedt, I., and Valin, L.: Inferring Changes in Summertime Surface Ozone–NOx–VOC Chemistry over U. S. Urban Areas from Two Decades of Satellite and Ground-Based Observations, Environ. Sci. Technol., 54, 6518–6529, https://doi.org/10.1021/acs.est.9b07785, 2020.
Johnson, M. S., Souri, A. H., Philip, S., Kumar, R., Naeger, A., Geddes, J., Judd, L., Janz, S., Chong, H., and Sullivan, J.: Satellite remote-sensing capability to assess tropospheric-column ratios of formaldehyde and nitrogen dioxide: case study during the Long Island Sound Tropospheric Ozone Study 2018 (LISTOS 2018) field campaign, Atmos. Meas. Tech., 16, 2431–2454, https://doi.org/10.5194/amt-16-2431-2023, 2023.
Kharol, S. K., Martin, R. V., Philip, S., Boys, B., Lamsal, L. N., Jerrett, M., Brauer, M., Crouse, D. L., McLinden, C., and Burnett, R. T.: Assessment of the magnitude and recent trends in satellite-derived ground-level nitrogen dioxide over North America, Atmos. Environ., 118, 236–245, https://doi.org/10.1016/j.atmosenv.2015.08.011, 2015.
Kleinman, L. I.: Low and high NOx tropospheric photochemistry, J. Geophys. Res., 99, 16831–16838, 1994.
Kleinman, L. I., Daum, P. H., Lee, Y. N., Nunnermacker, L. J., Springston, S. R., Weinstein-Lloyd, J., and Rudolph, J.: A comparative study of ozone production in five US metropolitan areas, J. Geophys. Res.-Atmos., 110, D02301, https://doi.org/10.1029/2004JD005096, 2005.
Kleipool, Q., Rozemeijer, N., van Hoek, M., Leloux, J., Loots, E., Ludewig, A., van der Plas, E., Adrichem, D., Harel, R., Spronk, S., ter Linden, M., Jaross, G., Haffner, D., Veefkind, P., and Levelt, P. F.: Ozone Monitoring Instrument (OMI) collection 4: establishing a 17 year-long series of detrended level-1b data, Atmos. Meas. Tech., 15, 3527–3553, https://doi.org/10.5194/amt-15-3527-2022, 2022.
Koplitz, S., Simon, H., Henderson, B., Liljegren, J., Tonnesen, G., Whitehill, A., and Wells, B.: Changes in Ozone Chemical Sensitivity in the United States from 2007 to 2016, ACS Environ. Au, 2, 206–222, https://doi.org/10.1021/ACSENVIRONAU.1C00029, 2021.
Krotkov, N. A., McLinden, C. A., Li, C., Lamsal, L. N., Celarier, E. A., Marchenko, S. V., Swartz, W. H., Bucsela, E. J., Joiner, J., Duncan, B. N., Boersma, K. F., Veefkind, J. P., Levelt, P. F., Fioletov, V. E., Dickerson, R. R., He, H., Lu, Z., and Streets, D. G.: Aura OMI observations of regional SO2 and NO2 pollution changes from 2005 to 2015, Atmos. Chem. Phys., 16, 4605–4629, https://doi.org/10.5194/acp-16-4605-2016, 2016.
Krotkov, N. A., Lamsal, L. N., Celarier, E. A., Swartz, W. H., Marchenko, S. V., Bucsela, E. J., Chan, K. L., Wenig, M., and Zara, M.: The version 3 OMI NO2 standard product, Atmos. Meas. Tech., 10, 3133–3149, https://doi.org/10.5194/amt-10-3133-2017, 2017.
Krotkov, N. A., Lamsal, L. N., Marchenko, S. V., Bucsela, E. J., Swartz, W. H., Joiner, J., and the OMI core team: OMI/Aura Nitrogen Dioxide (NO2) Total and Tropospheric Column 1-orbit L2 Swath 13x24 km V003, Goddard Earth Sciences Data and Information Services Center (GES DISC) [data set], Greenbelt, MD, USA, https://doi.org/10.5067/Aura/OMI/DATA2017, 2019.
Lamsal, L. N., Martin, R. V., Steinbacher, M., Celarier, E. A., Bucsela, E., Dunlea, E. J., and Pinto, J.: Ground level nitrogen dioxide concentrations inferred from the satellite-borne Ozone Monitoring Instrument, J. Geophys. Res., 113, D16308, https://doi.org/10.1029/2007JD009235, 2008.
Lamsal, L. N., Martin, R. V., van Donkelaar, A., Celarier, E. A., Bucsela, E. J., Boersma, K. F., Dirksen, R., Luo, C., and Wang, Y.: Indirect validation of tropospheric nitrogen dioxide retrieved from the OMI satellite instrument: Insight into the seasonal variation of nitrogen oxides at northern midlatitudes, J. Geophys. Res., 115, D05302, https://doi.org/10.1029/2009JD013351, 2010.
Lamsal, L. N., Duncan, B. N., Yoshida, Y., Krotkov, N. A., Pickering, K. E., Streets, D. G., and Lu, Z.: U. S. NO2 trends (2005–2013): EPA Air Quality System (AQS) data versus improved observations from the Ozone Monitoring Instrument (OMI), Atmos. Environ., 110, 130–143, https://doi.org/10.1016/j.atmosenv.2015.03.055, 2015.
Levelt, P. F., Hilsenrath, E., Leppelmeier, G. W., Van Den Oord, G. H. J., Bhartia, P. K., Tamminen, J., De Haan, J. F., and Veefkind, J. P.: Science Objectives of the Ozone Monitoring Instrument, IEEE T. Geosci. Remote, 44, 1199–1208, 2006.
Levelt, P. F., Joiner, J., Tamminen, J., Veefkind, J. P., Bhartia, P. K., Stein Zweers, D. C., Duncan, B. N., Streets, D. G., Eskes, H., van der A, R., McLinden, C., Fioletov, V., Carn, S., de Laat, J., DeLand, M., Marchenko, S., McPeters, R., Ziemke, J., Fu, D., Liu, X., Pickering, K., Apituley, A., González Abad, G., Arola, A., Boersma, F., Chan Miller, C., Chance, K., de Graaf, M., Hakkarainen, J., Hassinen, S., Ialongo, I., Kleipool, Q., Krotkov, N., Li, C., Lamsal, L., Newman, P., Nowlan, C., Suleiman, R., Tilstra, L. G., Torres, O., Wang, H., and Wargan, K.: The Ozone Monitoring Instrument: overview of 14 years in space, Atmos. Chem. Phys., 18, 5699–5745, https://doi.org/10.5194/acp-18-5699-2018, 2018.
Li, Y., Lau, A. K. H., Fung, J. C. H., Zheng, J. Y., Zhong, L. J., and Louie, P. K. K.: Ozone source apportionment (OSAT) to differentiate local regional and super-regional source contributions in the Pearl River Delta region, China, J. Geophys. Res.-Atmos., 117, 1–18, https://doi.org/10.1029/2011JD017340, 2012.
Mahajan, A. S., De Smedt, I., Biswas, M. S., Ghude, S., Fadnavis, S., Roy, C., and van Roozendael, M.: Inter-annual variations in satellite observations of nitrogen dioxide and formaldehyde over India, Atmos. Environ., 116, 194–201, https://doi.org/10.1016/j.atmosenv.2015.06.004, 2015.
Marais, E. A., Jacob, D. J., Kurosu, T. P., Chance, K., Murphy, J. G., Reeves, C., Mills, G., Casadio, S., Millet, D. B., Barkley, M. P., Paulot, F., and Mao, J.: Isoprene emissions in Africa inferred from OMI observations of formaldehyde columns, Atmos. Chem. Phys., 12, 6219–6235, https://doi.org/10.5194/acp-12-6219-2012, 2012.
Marchenko, S., Krotkov, N., Lamsal, L., Celarier, E., Swartz, W., and Bucsela, E.: Revising the slant column density retrieval of nitrogen dioxide observed by the Ozone Monitoring Instrument, J. Geophys. Res., 120, 5670–5692, https://doi.org/10.1002/2014JD022913, 2015.
Martin, R. V., Fiore, A. M., and Van Donkelaar, A.: Space-based diagnosis of surface ozone sensitivity to anthropogenic emissions, Geophys. Res. Lett., 31, L06120, https://doi.org/10.1029/2004GL019416, 2004.
McDuffie, E. E., Smith, S. J., O'Rourke, P., Tibrewal, K., Venkataraman, C., Marais, E. A., Zheng, B., Crippa, M., Brauer, M., and Martin, R. V.: A global anthropogenic emission inventory of atmospheric pollutants from sector- and fuel-specific sources (1970–2017): an application of the Community Emissions Data System (CEDS), Earth Syst. Sci. Data, 12, 3413–3442, https://doi.org/10.5194/essd-12-3413-2020, 2020.
Milford, J. B., Gao, D. F., Sillman, S., Blossey, P., and Russell, A. G.: Total reactive nitraogen (NOy) as an indicator of the sensitivity of ozone to reductions in hydrocarbon and NOx emissions, J. Geophys. Res.-Atmos., 99, 3533–3542, https://doi.org/10.1029/93jd03224, 1994.
Millet, D. B., Jacob, D. J., Boersma, K. F., Fu, T.-M., Kurosu, T. P., Chance, K., Heald, C. L., and Guenther, A.: Spatial distribution of isoprene emissions from North America derived from formaldehyde column measurements by the OMI satellite sensor, J. Geophys. Res., 113, D02307, https://doi.org/10.1029/2007JD008950, 2008.
Monks, P. S., Archibald, A. T., Colette, A., Cooper, O., Coyle, M., Derwent, R., Fowler, D., Granier, C., Law, K. S., Mills, G. E., Stevenson, D. S., Tarasova, O., Thouret, V., von Schneidemesser, E., Sommariva, R., Wild, O., and Williams, M. L.: Tropospheric ozone and its precursors from the urban to the global scale from air quality to short-lived climate forcer, Atmos. Chem. Phys., 15, 8889–8973, https://doi.org/10.5194/acp-15-8889-2015, 2015.
National Research Council: Rethinking the Ozone Problem in Urban and Regional Air Pollution, The National Academies Press, Washington, DC, 524 pp., https://doi.org/10.17226/1889, 1991.
Nussbaumer, C. M., Pozzer, A., Tadic, I., Röder, L., Obersteiner, F., Harder, H., Lelieveld, J., and Fischer, H.: Tropospheric ozone production and chemical regime analysis during the COVID-19 lockdown over Europe, Atmos. Chem. Phys., 22, 6151–6165, https://doi.org/10.5194/acp-22-6151-2022, 2022.
Palmer, P. I., Jacob, D. J., Chance, K., Martin, R. V., Spurr, R. J. D., Kurosu, T. P., Bey, I., Yantosca, R., Fiore, A., and Li, Q.: Air mass factor formulation for spectroscopic measurements from satellites: Application to formaldehyde retrievals from the Global Ozone Monitoring Experiment, J. Geophys. Res.-Atmos., 106, 14539–14550, https://doi.org/10.1029/2000JD900772, 2001.
Pei, C. L., Yang, W. Q., Zhang, Y. L., Song, W., Xiao, S. X., Wang, J., Zhang, J. P., Zhang, T., Chen, D. H., Wang, Y. J., Chen, Y. N., and Wang, X. M.: Decrease in ambient volatile organic compounds during the COVID-19 lockdown period in the Pearl River Delta region, South China, Sci. Total Environ., 823, 153720, https://doi.org/10.1016/j.scitotenv.2022.153720, 2022.
Philip, S., Martin, R. V., van Donkelaar, A., Lo, J. W., Wang, Y., Chen, D., Zhang, L., Kasibhatla, P. S., Wang, S. W., Zhang, Q., Lu, Z., Streets, D. G., Bittman, S., and Macdonald, D. J.: Global chemical composition of ambient fine particulate matter for exposure assessment, Environ. Sci. Technol., 48, 13060–13068, https://doi.org/10.1021/es502965b, 2014.
Schenkeveld, V. M. E., Jaross, G., Marchenko, S., Haffner, D., Kleipool, Q. L., Rozemeijer, N. C., Veefkind, J. P., and Levelt, P. F.: In-flight performance of the Ozone Monitoring Instrument, Atmos. Meas. Tech., 10, 1957–1986, https://doi.org/10.5194/amt-10-1957-2017, 2017.
Schoeberl, M. R., Douglass, A. R., Hilsenrath, E., Bhartia, P. K., Beer, R., Waters, J. W., Gunson, M. R., Froidevaux, L., Gille, J. C., Barnett, J. J., Levelt, P. F., and DeCola, P.: Overview of the EOS aura mission, IEEE T. Geosci. Remote, 44, 1066–1072, https://doi.org/10.1109/TGRS.2005.861950, 2006.
Schroeder, J. R., Crawford, J. H., Fried, A., Walega, J., Weinheimer, A., Wisthaler, A., Müller, M., Mikoviny, T., Chen, G., Shook, M., Blake, D. R., and Tonnesen, G. S.: New insights into the column CH2ONO2 ratio as an indicator of near-surface ozone sensitivity, J. Geophys. Res.-Atmos., 122, 8885–8907, https://doi.org/10.1002/2017JD026781, 2017.
Seinfeld, J. H. and Pandis, S. N.: Atmospheric Chemistry and Physics: From Air Pollution to Climate Change, John Wiley and Sons, Hoboken, ISBN: 978-1-118-94740-1, 2016.
Shen, L., Jacob, D. J., Liu, X., Huang, G., Li, K., Liao, H., and Wang, T.: An evaluation of the ability of the Ozone Monitoring Instrument (OMI) to observe boundary layer ozone pollution across China: application to 2005–2017 ozone trends, Atmos. Chem. Phys., 19, 6551–6560, https://doi.org/10.5194/acp-19-6551-2019, 2019.
Sillman, S.: The use of NOy, H2O2, and HNO3 as indicators for O3-NOx-hydrocarbon sensitivity in urban locations, J. Geophys. Res.-Atmos., 100, 14175–14188, https://doi.org/10.1029/94JD02953, 1995.
Sillman, S.: The relation between ozone, NOx, and hydrocarbons in urban and polluted rural environments, Atmos. Environ., 33, 1821–1845, 1999.
Sillman, S., Logan, J. A., and Wofsy, S. C.: The sensitivity of ozone to nitrogen oxides and hydrocarbons in regional ozone episodes, J. Geophys. Res., 95, 1837–1851, https://doi.org/10.1029/JD095iD02p01837, 1990.
Souri, A. H., Choi, Y., Jeon, W., Woo, J.-H., Zhang, Q., and Kurokawa J.-I.: Remote sensing evidence of decadal changes in major tropospheric ozone precursors over East Asia, J. Geophys. Res.-Atmos., 122, 2474–2492, https://doi.org/10.1002/2016JD025663, 2017.
Souri, A. H., Nowlan, C. R., Wolfe, G. M., Lamsal, L. N., Chan Miller, C. E., Abad, G. G., Janz, S. J., Fried, A., Blake, D. R., Weinheimer, A. J., Diskin, G. S., Liu, X., and Chance, K.: Revisiting the effectiveness of ratios for inferring ozone sensitivity to its precursors using high resolution airborne remote sensing observations in a high ozone episode during the KORUS-AQ campaign, Atmos. Environ., 224, 117341, https://doi.org/10.1016/j.atmosenv.2020.117341, 2020.
Souri, A. H., Chance, K., Sun, K., Liu, X., and Johnson, M. S.: Dealing with spatial heterogeneity in pointwise-to-gridded-data comparisons, Atmos. Meas. Tech., 15, 41–59, https://doi.org/10.5194/amt-15-41-2022, 2022.
Souri, A. H., Johnson, M. S., Wolfe, G. M., Crawford, J. H., Fried, A., Wisthaler, A., Brune, W. H., Blake, D. R., Weinheimer, A. J., Verhoelst, T., Compernolle, S., Pinardi, G., Vigouroux, C., Langerock, B., Choi, S., Lamsal, L., Zhu, L., Sun, S., Cohen, R. C., Min, K.-E., Cho, C., Philip, S., Liu, X., and Chance, K.: Characterization of errors in satellite-based tropospheric column ratios with respect to chemistry, column-to-PBL translation, spatial representation, and retrieval uncertainties, Atmos. Chem. Phys., 23, 1963–1986, https://doi.org/10.5194/acp-23-1963-2023, 2023a.
Souri, A. H., Kumar, R., Chong H., Golbazi, M., Knowland, K. E., Geddes, J., and Johnson, M. S.: Decoupling in the vertical shape of HCHO during a sea breeze event: The effect on trace gas satellite retrievals and column-to-surface translation, Atmos. Environ., 309, 119929, https://doi.org/10.1016/j.atmosenv.2023.119929, 2023b.
Spurr, R. J. D.: VLIDORT: a linearized pseudo-spherical vector discrete ordinate radiative transfer code for forward model and retrieval studies in multilayer multiple scattering media, J. Quant. Spectrosc. Ra., 102, 316–421, https://doi.org/10.1016/j.jqsrt.2006.05.005, 2006.
Stewart, I. D. and Oke, T. R.: Local Climate Zones for Urban Temperature Studies, B. Am. Meteorol. Soc., 93, 1879–1900, https://doi.org/10.1175/BAMS-D-11-00019.1, 2012.
Tai, A., Martin, M., and Heald, C.: Threat to future global food security from climate change and ozone air pollution, Nat. Clim. Change, 4, 817–821, https://doi.org/10.1038/nclimate2317, 2014.
Tao, M., Fiore, A. M., Jin, X., Schiferl, L. D., Commane, R., Judd, L. M., Janz, S., Sullivan, J. T., Miller, P. J., Karambelas, A., Davis, S., Tzortziou, M., Valin, L., Whitehill, A., Civerolo, K., and Tian, Y.: Investigating changes in ozone formation chemistry during summertime pollution vents over the northeastern United States, Environ. Sci. Technol., 56, 15312–15327, https://doi.org/10.1021/acs.est.2c02972, 2022.
Tonnesen, G. S. and Dennis, R. L.: Analysis of radical propagation efficiency to assess O3 sensitivity to hydrocarbons and NOx: 2. Long-lived species as indicators of O3 concentration sensitivity, J. Geophys. Res.-Atmos., 105, 9227–9241, https://doi.org/10.1029/1999JD900372, 2000.
US Environmental Protection Agency (US EPA): Air Quality Criteria for Ozone and Related Photochemical Oxidants (2006 Final Report), U. S. Environmental Protection Agency, Washington, DC, EPA/600/R-05/004aF-cF, 2006.
US Environmental Protection Agency: Air Quality System Data Mart [internet database], https://www.epa.gov/outdoor-air-quality-data, last access: 3 August 2023.
Wang, W., van der A, R., Ding, J., van Weele, M., and Cheng, T.: Spatial and temporal changes of the ozone sensitivity in China based on satellite and ground-based observations, Atmos. Chem. Phys., 21, 7253–7269, https://doi.org/10.5194/acp-21-7253-2021, 2021.
Witte, J., Duncan, B., Douglass, A., Kurosu, T., Chance, K., and Retscher, C.: The unique OMI HCHO NO2 feature during the 2008 Beijing Olympics: Implications for ozone production sensitivity, Atmos. Environ., 45, 3103–3111, https://doi.org/10.1016/j.atmosenv.2011.03.015, 2011.
Wu, S., Duncan, B. N., Jacob, D. J., Fiore, A. M., and Wild, O.: Chemical nonlinearities in relating intercontinental ozone pollution to anthropogenic emissions, Geophys. Res. Lett., 36, L05806, https://doi.org/10.1029/2008GL036607, 2009.
Zhang, L., Jacob, D. J., Kopacz, M., Henze, D. K., Singh, K., and Jaffe, D. A.: Intercontinental source attribution of ozone pollution at western U. S. sites using an adjoint method, Geophys. Res. Lett., 36, L11810, https://doi.org/10.1029/2009GL037950, 2009.
Zhu, L., Jacob, D. J., Mickley, L. J., Marais, E. A., Cohan, D. S., Yoshida, Y., Duncan, B. N., González Abad, G., and Chance, K. V.: Anthropogenic emissions of highly reactive volatile organic compounds in eastern Texas inferred from oversampling of satellite (OMI) measurements of HCHO columns, Environ. Res. Lett., 9, 114004, https://doi.org/10.1088/1748-9326/9/11/114004, 2014.
Zhu, L., Jacob, D. J., Kim, P. S., Fisher, J. A., Yu, K., Travis, K. R., Mickley, L. J., Yantosca, R. M., Sulprizio, M. P., De Smedt, I., González Abad, G., Chance, K., Li, C., Ferrare, R., Fried, A., Hair, J. W., Hanisco, T. F., Richter, D., Jo Scarino, A., Walega, J., Weibring, P., and Wolfe, G. M.: Observing atmospheric formaldehyde (HCHO) from space: validation and intercomparison of six retrievals from four satellites (OMI, GOME2A, GOME2B, OMPS) with SEAC4RS aircraft observations over the southeast US, Atmos. Chem. Phys., 16, 13477–13490, https://doi.org/10.5194/acp-16-13477-2016, 2016.
Zhu, L., Jacob, D. J., Keutsch, F. N., Mickley, L. J., Scheffe, R., Strum, M., González Abad, G., Chance, K., Yang, K., Rappenglück, B., Millet, D. B., and Baasandorj, M.: Formaldehyde (HCHO) as a Hazardous Air Pollutant: Mapping surface air concentrations from satellite and inferring cancer risks in the United States, Environ. Sci. Technol., 51, 5650–5657, https://doi.org/10.1021/acs.est.7b01356, 2017a.
Zhu, L., Mickley, L. J., Jacob, D. J., Marais, E. A., Sheng, J., Hu, L., González Abad, G., and Chance, K.: Long-term (2005–2014) trends in formaldehyde (HCHO) columns across North America as seen by the OMI satellite instrument: Evidence of changing emissions of volatile organic compounds, Geophys. Res. Lett., 44, 7079–7086, https://doi.org/10.1002/2017GL073859, 2017b.
Zhu, L., González Abad, G., Nowlan, C. R., Chan Miller, C., Chance, K., Apel, E. C., DiGangi, J. P., Fried, A., Hanisco, T. F., Hornbrook, R. S., Hu, L., Kaiser, J., Keutsch, F. N., Permar, W., St. Clair, J. M., and Wolfe, G. M.: Validation of satellite formaldehyde (HCHO) retrievals using observations from 12 aircraft campaigns, Atmos. Chem. Phys., 20, 12329–12345, https://doi.org/10.5194/acp-20-12329-2020, 2020.