the Creative Commons Attribution 4.0 License.
the Creative Commons Attribution 4.0 License.
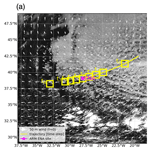
Evaluation of aerosol–cloud interactions in E3SM using a Lagrangian framework
Matthew W. Christensen
Po-Lun Ma
Adam C. Varble
Johannes Mülmenstädt
Jerome D. Fast
A Lagrangian framework is used to evaluate aerosol–cloud interactions in the U.S. Department of Energy's Energy Exascale Earth System Model (E3SM) version 1 (E3SMv1) for measurements taken at Graciosa Island in the Azores where a U.S. Department of Energy Atmosphere Radiation Measurement (ARM) site is located. This framework uses direct measurements of cloud condensation nuclei (CCN) concentration (instead of relying on satellite retrievals of aerosol optical depth) and incorporates a suite of ground-based ARM measurements, satellite retrievals, and meteorological reanalysis products that when applied to over a 1500 trajectories provides key insights into the evolution of low-level clouds and aerosol radiative forcing that is not feasible from a traditional Eulerian analysis framework. Significantly lower concentrations (40 %) of surface CCN concentration are measured when precipitation rates in 48 h back trajectories average above 1.2 mm d−1 in the Integrated Multi-satellitE Retrievals for Global Precipitation Measurement (IMERG) product. The depletion of CCN concentration when precipitation rates are elevated is nearly twice as large in the ARM observations compared to E3SMv1 simulations. The model CCN concentration bias remains significant despite modifying the autoconversion and accretion rates in warm clouds.
As the clouds in trajectories associated with larger surface-based CCN concentration advect away from Graciosa Island, they maintain higher values of droplet number concentrations (Nd) over multiple days in observations and E3SM simulations compared to trajectories that start with lower CCN concentrations. The response remains robust even after controlling for meteorological factors such as lower troposphere stability, the degree of cloud coupling with the surface, and island wake effects. E3SMv1 simulates a multi-day aerosol effect on clouds and a Twomey radiative effect that is within 30 % of the ARM and satellite observations. However, the mean cloud droplet concentration is more than 2–3 times larger than in the observations. While Twomey radiative effects are similar amongst autoconversion and accretion sensitivity experiments, the liquid water path and cloud fraction adjustments are positive when using a regression model as opposed to negative when using the present-day minus pre-industrial aerosol emissions approach. This result suggests that tuning the autoconversion and accretion alone is unlikely to produce the desired aerosol susceptibilities in E3SMv1.
- Article
(4875 KB) - Full-text XML
-
Supplement
(40690 KB) - BibTeX
- EndNote
Microphysical cloud properties, such as the size of cloud droplets and their concentrations, have been shown to change in response to an increase in cloud condensation nuclei (CCN; Twomey, 1974). Increases in CCN concentration lead to predictable increases in droplet number concentration (Nd) and smaller cloud droplet effective radii (Re) but only under no change in liquid water path (LWP) and cloud fraction (CF). While the Twomey effect is a useful theoretical construct, real-world cloud responses are not instantaneous in time because increased CCN concentration can affect LWP and CF through suppressing or enhancing precipitation and evaporation on timescales from hours to days (Yamaguchi et al., 2017). LWP and CF strongly influence cloud albedo (Stephens et al., 1991) and hence aerosol indirect radiative forcing, but LWP and CF radiative adjustments (to the Twomey effect) are considered highly uncertain due to the nature in which they co-evolve with precipitation, evaporation, and cloud lifetime (Bellouin et al., 2020). Links amongst cloud macrophysical variables to changes in aerosol processes are poorly constrained in general circulation models (GCMs), typically with unrealistically large LWP responses to increased aerosol concentration (Mülmenstädt et al., 2019; Gryspeerdt et al., 2020). Subtle changes to the tuning parameters of the warm-rain process in GCMs to close this gap with observations can result in significant departures in the global mean temperature response due to anthropogenic aerosol (Wang et al., 2012; Golaz et al., 2013, 2019; Mülmenstädt et al., 2020). We thus seek to apply a Lagrangian framework to determine whether macrophysical cloud variables vary over time and as a function of CCN concentration as a means to constrain the aerosol indirect radiative forcing in GCMs and other atmosphere models.
A Lagrangian framework has been demonstrated to be a useful tool to quantify the radiative budgets and impacts of meteorological and aerosol drivers on evolving cloud fields from large eddy simulations (LESs; Yamaguchi et al., 2017; Kazil et al., 2021), satellite observations (Pincus et al., 1997; Eastman et al., 2016; Christensen et al., 2020), and aircraft observations (Johnson et al., 2000; Mohrmann et al., 2019). Trajectory analysis is useful for determining aerosol source regions and for tracking cloud systems and rates of change in physical quantities needed to assess causality. This approach differs from the more common Eulerian perspective, which typically lacks key temporal connections between the current cloud state, its prior history, and future changes in precipitation and radiative properties over time. From an Eulerian perspective, aerosol–cloud relationships are typically inferred from a distribution of observations typically taken over many stages of the cloud life cycle. The extent to which there is a distinct advantage to using a Lagrangian framework to quantify aerosol–cloud radiation interactions and the impact of cumulative precipitation on aerosol CCN populations forms one of the key motivations for this research.
Routine ground-based observations, such as those taken from the Atmosphere Radiation Measurement (ARM) user facility, can complement satellite observations by providing (1) in situ CCN chamber measurements; (2) vertical profiles of aerosol layers through ground-based lidar observations; (3) vertical estimates of Re, vertical velocity, and turbulent kinetic energy from remote sensing instrumentation; and (4) vertical profiles of atmospheric state properties from radiosondes. These detailed aerosol, cloud, and thermodynamic measurements have been shown to be essential to validate satellite retrieval products (Liu and Li, 2014) and improve process-scale understanding of clouds (Wu et al., 2020) at several ARM sites. However, a key limitation is that ground-based measurements can provide only an Eulerian perspective. Another goal of this work is to combine these ground-based data sets within a Lagrangian framework to determine whether warm clouds evolve differently in satellite observations under varying levels of measured CCN concentration. This integrated approach is then used to make the same Lagrangian-framework-based comparisons with simulations from the Energy Exascale Earth System Model (E3SM) model.
Recently, Christensen et al. (2020) used geostationary satellite observations in a Lagrangian framework to show that above-median aerosol optical depth values (retrieved from confident clear-sky regions) enhance the longevity of marine stratocumulus by about 2 h along the classic stratus-to-cumulus transition zone. Similar aerosol effects on longevity have been observed and simulated in midlatitude boundary layer clouds (Goren et al., 2019). However, a key limitation of the multi-spectral imagery from polar orbiting and geostationary satellites is that retrievals are typically weighted towards the tops of clouds and thereby unable to inform processes deeper in the cloud (e.g., precipitation). Aerosol optical depth (AOD) or aerosol index (AI= Å τa, where Å is the Ångström exponent and τa is the AOD) is commonly used as a proxy for CCN concentration. Aerosol properties cannot be retrieved inside or below clouds using this satellite data, and thus retrievals in clear-sky regions are commonly extrapolated to the observations of nearby clouds. One disadvantage to this lack of co-location (between clouds and clear-sky regions) is that the clear-sky retrievals can be affected by variations in surface albedo, humidification by elevated relative humidity, and 3D radiative scattering off the sides of clouds that artificially illuminate the clear-sky region with the aerosol (Christensen et al., 2017). Furthermore, AOD and AI are vertically integrated quantities and thus may not represent the CCN concentration at cloud base (Quaas et al., 2020). The lack of vertical co-location between retrieved AOD or AI and cloud base CCN concentration typically leads to an underestimate in the Nd–CCN relationship (Costantino and Breon, 2010). Therefore, it may be prudent to use the CCN concentration (preferably at cloud base) to inform processes related to aerosol–cloud interactions and avoid retrieval issues and assumptions when using AOD or AI from satellite data. To the extent the vertical distribution of AOD is correlated to surface-based CCN concentration and whether it can be used as a suitable proxy for quantifying aerosol indirect radiative effects forms another key question of this research.
The outline of the paper is as follows: Sect. 2 describes the data sets used in this study, Sect. 3 provides an example of the Lagrangian framework methodology and its applicability to study aerosol–cloud interactions, Sect. 4 discusses the results, and a summary of the research is provided in Sect. 5.
We use a diversity of data sets containing ground-based measurements from ARM, satellite observations from geostationary and polar orbits, and GCM simulations from the E3SM version 1 (E3SMv1) global atmosphere model. The observational products used to sample the atmosphere upwind and downwind of Graciosa Island are depicted in Fig. 1 and summarized in Table 1.
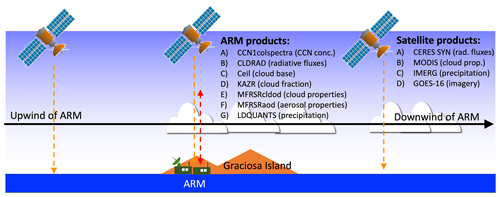
Figure 1Information graphic showing the satellite (dashed orange arrow) and surface ARM (dashed red arrow) data sets in trajectories passing over Graciosa Island.
Table 1Summary of observational data products analyzed for the period of 22 June 2016 through 28 October 2020.
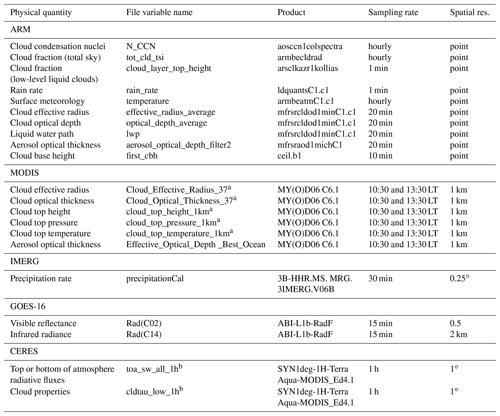
a Includes PCL variables. b Example of one variable name. LT stands for local time.
2.1 Ground-based observations from the ARM Eastern North Atlantic (ENA) site
The ARM ENA site is located on the northeastern side of Graciosa Island in the Azores archipelago. Ground-based observations began in 2013 after a successful ARM mobile facility (AMF) deployment during 2009–2010 (Wood et al., 2015). Graciosa Island resides on the boundary of the subtropics and the midlatitudes and experiences meteorological conditions in both regimes. Low clouds resembling typical open and closed cellular structures for stratocumulus are common in this location (Jensen et al., 2021) and are found to form under the influence of the Azores high-pressure system and behind frontal systems and cold-air outbreaks occurring during northwesterly flows. Low clouds over the Azores frequently produce precipitation, mostly in the form of drizzle. The atmosphere is moister and less stable during winter than during summer, resulting in thicker cloud layers with higher LWP and drizzle frequency (∼ 70 %) during winter months (Rémillard et al., 2012; Wood et al., 2015; Wu et al., 2020). Using a satellite cloud regime analysis, Rémillard and Tselioudis (2015) show that the variability in the cloud distribution over the Azores is similar to the global mean, thereby making it an ideal location to evaluate aerosol and warm-cloud properties and processes in climate model simulations.
CCN chamber measurements are provided using the Droplet Measurement Technologies (DMT) Model 1 CCN counter (Roberts and Nenes, 2005) at multiple set point supersaturations (0 %, 0.05 %, 0.1 %, 0.2 %, 0.3 %, 0.5 %, and 1.0 %). The counter completes one cycle through all supersaturations every 30 min. Data are provided from 22 June 2016–28 October 2020 in the Aerosol Observing System Cloud Condensation Nuclei Counter (single column) spectral data (AOSCCN1COLSPECTRA) product (Uin et al., 2016). The data in the value-added product (VAP) are filtered to improve the quality by providing output only when stable measurements occur in which fluctuations in the counter measurements remain below 2 standard deviations within a given saturation step.
We utilize the ARM best-estimate cloud radiation VAP data set (ARMBECLDRAD; Xie et al., 2010; Tang and Xie, 2020), which provides the total sky cloud fraction by the total sky imager in hourly intervals from 2014–2020 at the ENA site. Cloud top heights and low-level cloud fraction are estimated from the active remote sensing of clouds (ARSCL) product (O'Connor et al., 2004; Kollias et al., 2016) using Ka-band ARM zenith radar (KAZR) from the ARSCLKAZR1KOLLIAS product (Clothiaux et al., 2001). Ceilometer retrievals are used to obtain cloud base height using the CEIL VAP. Surface temperature and humidity measurements are used to calculate the lifted condensation level (LCL) provided from the ARM best-estimate atmospheric measurements (BEATM) VAP (Xie et al., 2010). These data are used to determine the degree of the coupling within the planetary boundary layer (PBL; see Sect. 4). Surface precipitation rate is obtained from a laser optical OTT Particle Size and Velocity (Parsivel) disdrometer, which measures the instantaneous rainfall rate of water flux from the number of drops in 32 size categories (0 to 25 mm) and 32 fall velocity categories (0.2 to 20 m s−1) falling to the surface. Precipitation rate from the laser disdrometer has a 6 % absolute bias with respect to reference gauges over a 1 min sampling interval (Tokay et al., 2014) as provided in the laser disdrometer quantities (LDQUANTS) VAP product (Hardin et al., 2020) and averaged into 1 h intervals to match the temporal sampling of our trajectories.
Layer mean cloud droplet effective radius is retrieved from cloud optical thickness using the multifilter rotating shadow band radiometer (MFRSR) for overcast single-layer liquid-only cloud layers. The retrieval is based on the algorithm developed by Min and Harrison (1996) of atmosphere radiative transfer at 415 nm. If the liquid water path is available from the microwave radiometer (MWR) then effective radius is also derived; otherwise, a default value of 8 µm is assumed in the MFRSRCLDOD VAP data set (Turner et al., 2021). However, we do not include the default values to ensure independent retrievals are used. These criteria occur less than 30 % of the time and ensure that the results are sensitive to the variations in changes in aerosol concentration. This follows from similar assessments of aerosol–cloud interactions using the MFRSR instrument (e.g., see Kim et al., 2003). AOD is obtained using the MFRSR product (Koontz et al., 2013) from the 550 nm wavelength by retrieving the total extinction of the direct and diffuse solar radiation.
2.2 Satellite observations
Geostationary Operational Environmental Satellite (GOES) Advanced Baseline Imager (ABI) data from the National Oceanic Atmospheric Adminstration (NOAA) GOES-R series satellite are used to track cloud systems across vast regions. The satellite imagery provides several channels spanning the visible, near-infrared, and far-infrared parts of the electromagnetic spectrum. At a distance of approximately 36 000 km, the imager views roughly 42 % of the Earth's surface (a full disk) in 10 min intervals (although with decreasing spatial resolution at higher latitudes). These observations have the remarkable capability to capture expansive continental-scale regions at spatial resolutions down to 0.5 km (for the 0.64 µm visible channel and 2 km for the 3.9 and 11 µm channels) at nadir making it useful for studying the development and decay of cloud fields.
The Clouds and the Earth's Radiant Energy System Synoptic (SYN1deg-1Hour) edition 4.1 product (Doelling et al., 2016) provides Re, visible cloud optical thickness, LWP, and longwave and shortwave radiative fluxes at the top and bottom of the atmosphere. The data are gridded to 1∘ × 1∘ spatial resolution at hourly intervals from aggregated retrievals from a network of 16 geostationary satellites (e.g., GOES-R) that have multi-spectral imagery spanning key channels in the visible, near-infrared, and infrared. The NASA Goddard algorithm retrieves cloud properties following the methodology described in Nakajima and King (1989) and cross-calibrates the data with MODIS collection 5.1 to make a consistent comparison. Finally, an additional calibration step is carried out to ensure the radiative budget of the top of the atmosphere modeled with the Fu–Liou algorithm (Fu and Liou, 1992) is consistent with the retrieved CERES top-of-atmosphere radiative fluxes from the single-scanning radiometer. A recent analysis (Hinkelman and Marchand, 2020) has found that the solar and infrared flux estimates have strong seasonal and diurnal variations that still need to be addressed in the CERES SYN product, but despite these biases the relative differences between clean and polluted clouds are informative for studying aerosol–cloud interactions. We compare CERES SYN data with the MODIS collection 6 product at 1 km spatial resolution for the cloud product and aerosol optical thickness retrieved in 10 km2 clear-sky regions at 550 and 865 nm wavelengths.
Precipitation rates are provided on a half-hourly basis on a 0.1∘ grid using the Integrated Multi-satellitE Retrievals for Global Precipitation Measurement (IMERG) product (Huffman et al., 2015). This product integrates passive microwave precipitation estimates from low-Earth-orbiting satellites (Special Sensor Microwave Imager, Tropical Rainfall Measurement Mission, Advanced Microwave Scanning Radiometer, Global Precipitation Measurement) and infrared imagery in geostationary orbit (ABI and Spinning Enhanced Visible and InfraRed Imager), with the final result tuned to radar and rain gauge retrievals. This data set has weaker sensitivity to precipitation rates, typically less than 0.1 mm h−1, as compared to the active radar on CloudSat (Christensen et al., 2013; Skofronick-Jackson et al., 2018) but has the advantage of continuous spatiotemporal coverage that spaceborne radar does not provide. We recognize that light precipitation can be important for shaping the mesoscale structure of clouds (Savic-Jovcic and Stevens, 2008), and this limitation cannot currently be overcome with existing satellite observations. We thus use accumulated precipitation along trajectories spanning multiple days to examine broadscale changes in cloud and aerosol properties in a Lagrangian framework.
2.3 Meteorological data
Reanalysis data from the Modern-Era Retrospective analysis for Research and Applications version 2 (MERRA-2) (Gelaro et al., 2017) is used to drive the trajectory model (discussed in subsequent sections). Profiles of temperature, humidity, and wind speed are extracted along trajectories using bilinear interpolation in space and time. MERRA-2 data are spatially gridded at 0.5∘ resolution with 72 vertical levels and provided every 3 h. Aerosols are included through data assimilation of the bias-corrected aerosol optical depth (AOD) from the Advanced very-high-resolution radiometer MODIS and Multi-angle Imaging Spectroradiometer (MISR) AOD over bright surfaces and the Aerosol Robotic Network (AERONET) AOD. Lower-tropospheric stability (LTS=θ700–θsfc, where θ700 and θsfc are the potential temperatures at 700 hPa and the surface, respectively) and free-tropospheric humidity (FTH, the relative humidity at 850 hPa; the top of the PBL is found to reside below this level ∼90 % of the time) are shown to influence the liquid water path adjustment of clouds (Chen et al., 2014). We extract these meteorological quantities from MERRA-2 profiles of temperature and specific humidity as well the vertical velocity at 500 hPa.
We investigate whether there is a substantial island wake effect caused by wind blowing over the terrain and whether the downstream cloud properties can be isolated from aerosol impacts on clouds. Ebmeier et al. (2014) found that an increase in the Froude number increased cloud optical thickness in the vicinity of relatively tall and prominent volcanoes (Yasur and Piton de la Fournaise), but it had a limited effect on the systematic differences in cloud droplet effective radius between the upwind and downwind locations. We compute the Froude number, a dimensionless number defined by the ratio of the flow inertia to the external field, which is based on the speed–length ratio and can be written as , where h is the characteristic height of the mountains in the Azores (in this case h=1000 m to account for the elevation of surrounding nearby islands where the mountain tops can be significantly higher than Graciosa at 375 m); U is the perpendicular wind speed from the trajectory at the middle of the PBL impinging on the island; and N is the Brunt–Väisälä frequency, which can be written as , where g is the gravitational constant assumed to be 9.81 m s−2, θ is the potential temperature at the surface, and is the potential temperature difference over h. If the Froude number is low (<1), the flow is referred to as being subcritical. In these cases it is blocked by the island, meaning that the air will not make it over the top and will instead flow around the island, sometimes creating eddy-like structures and vortex shedding downstream. If the Froude number is high (>1), the flow is referred to as being supercritical and will flow freely over the island, while mountain waves will form mainly over the leeward side and propagate toward the region downstream.
2.4 Earth system modeling
The Energy Exascale Earth System Model (E3SM) version 1 (E3SMv1) (Golaz et al., 2019) is a long-term Earth system modeling effort sponsored by the U.S. Department of Energy. The E3SM Atmosphere Model (EAM) version 1 (EAMv1) (Rasch et al., 2019) uses a spectral-element dynamical core (Dennis et al., 2012; Taylor and Fournier, 2010); an updated two-moment cloud microphysics scheme (hereafter, MG2; Gettelman and Morrison, 2015); the Cloud Layers Unified By Binormals (CLUBB) for cloud macrophysics, turbulence, and shallow convection schemes (Golaz et al., 2002; Larson et al., 2002; Larson and Golaz, 2005; Bogenschutz et al., 2013); a deep convection parameterization (Zhang and McFarlane, 1995) with convective momentum transport (Richter and Rasch, 2008); a dilute plume treatment scheme (Neale et al., 2008); and a four-mode version of the Modal Aerosol Module (MAM4) (Liu et al., 2012, 2016; Wang et al., 2020). We run the EAMv1 model with a grid spacing of 1∘ × 1∘ with 72 vertical levels and nudge the atmospheric horizontal winds toward MERRA-2. Output is saved every hour over a geographic region spanning 20–60∘ N and 20∘ W–5∘ E in the vicinity of Graciosa Island. The Cloud Feedback Model Intercomparison Project (CFMIP) Observation Simulator Package (COSP) (Bodas-Salcedo et al., 2011; Swales et al., 2018) and MODIS simulator (Pincus et al., 2012) were used to ensure an apples-to-apples comparison between the EAMv1 model and the MODIS satellite retrievals.
In this study, we test the sensitivity of the autoconversion and accretion parameters that have been shown to have a large impact on cloud properties and the radiation budget (Golaz et al., 2013). The Khairoutdinov and Kogan (2000) (hereafter, KK2000) autoconversion scheme is part of MG2, but in E3SMv1 the coefficients have been changed to ensure better fidelity in the climate simulations. The coefficients (a, b, c) that define the autoconversion are expressed as , where qr is the rainwater mixing ratio, t is time, Qc cloud water content, and Nd is the cloud droplet number concentration. Accretion rate is expressed in KK2000 as , where Qr is the rainwater content, ρ is the air density, F1 is the sub-grid variability in Qc predicted by CLUBB, and F2 is the micro_mg_accre_enhan_fac, which varies by 50 % depending on the experiment (see Table 2). In the original KK2000 formulation, these parameters were derived by fitting a bin microphysics LES run on one marine stratocumulus case. In EAMv1, however, a variant of the KK2000 scheme is used (Rasch et al., 2019), and the parameterization is further adjusted in E3SMv2 (Ma et al., 2022; Golaz et al., 2022) because these parameters are subject to large uncertainty depending on the cloud regime (Wood, 2005; Kogan, 2013). We reduce the dependency of the autoconversion on Nd and increase its dependency on Qc. Four different simulations are performed to examine the parameter space of the effects of autoconversion and accretion on the aerosol indirect radiative effect. Table 2 lists the coefficient and exponents in the autoconversion parameterization for each experiment. In addition, we carry out simulations with pre-industrial emissions of aerosols and their precursors using the same configuration as our control run (labeled as “A0R0”) for present-day emissions. The differences between the two simulations reveal the effects of anthropogenic aerosols.
The Lagrangian framework is similar in scope to that described in Christensen et al. (2020), except for the following three notable exceptions that enhance confidence in our assessment of the indirect radiative effect of aerosols studied here: (1) detailed ground-based measurements of the aerosol, cloud, and meteorological state at the start time of the trajectory; (2) improved characterization of the polluted versus clean state through the use of actual CCN concentration measurements; and (3) initializing trajectories in more diverse meteorological conditions (i.e., when aerosol and cloud measurements coexist instead of using an AOD retrieval as the proxy for CCN concentration, which requires clear-sky conditions to be retrieved from satellite observations). The Hybrid Single-Particle Lagrangian Integrated Trajectory (HYSPLIT) (Stein et al., 2015) version 5 model is used to calculate 48 h back and forward trajectories using MERRA-2 reanalysis data. Trajectories are calculated from the middle of the planetary boundary layer (as computed using HYSPLIT from the profiles of temperature and humidity). Back trajectories are initialized at the Graciosa Island ARM site each day at 10:00 LT (local time) to coincide right before the Terra (morning at 10:30 LT) and Aqua (afternoon at 13:30 LT) MODIS overpass times. Forward trajectories are initialized 48 h later to coincide with the arriving air mass following the end of the back trajectory at Graciosa Island. These trajectories are stitched together to form a 96 h trajectory starting from the tail of the back trajectory and ending at the tail of the forward trajectory. This method ensures that the air mass moves through the ARM site and that the meteorological and cloud states would start and end in roughly the same phase of the diurnal cycle for each trajectory. While trajectories could be spawned randomly at different times of the day, starting all of them at the same local time reduces some of the complexity related to temporal changes in radiation and precipitation that are influenced by the diurnal cycle.
3.1 Product integration
Figure 2a shows the GOES-R visible image with a trajectory that intersects the ARM site at ENA. Locations along the trajectory are displayed at discrete times (, , , , t4=0, t5=0.5, t6=1, t7=1.6, t8=3.2 h) relative to the start of the trajectory (the time at which the trajectory intersects the ARM site). Satellite images of the Lagrangian trajectory at these discrete times are displayed in Fig. S1 in the Supplement. The trajectory tracks well with the boundary layer clouds as depicted in Movie S1. This particular case was selected to highlight the stratus-to-cumulus transitions that sometimes occur in this region. The large bank of closed-cell-type stratocumulus clouds to the northeast of Graciosa Island and lack of overlying high-level clouds as indicated by the relatively high values (greater than 273 K) of the 11 µm brightness temperature (an indicator for the presence of low-level clouds) make this a notable example (Fig. 2b). During the day the cloud bank continuously impinges on the island, producing precipitation with Ka-band radar reflectivities as large as 15 dBZ (Movie S2 as observed from an Eulerian perspective).
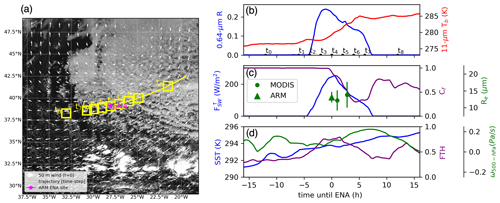
Figure 2(a) Lagrangian trajectory (yellow line) initialized at ENA on 27 October 2018 at 12:00 LT computed using HYSPLIT and MERRA-2 products is plotted over the GOES-16 visible image (0.64 µm reflectance). The trajectory spans two 16 h periods (backward and forward). (b) Visible reflectance and 11 µm brightness temperature from GOES-16; (c) CERES SYN top-of-atmosphere (TOA) outgoing shortwave flux (, blue) and cloud fraction (purple) with droplet effective radius from MODIS (green circle) and ARM (triangle; vertical lines denote 1 standard deviation in the retrievals); and (d) sea surface temperature (SST; blue), free-tropospheric relative humidity at 850 hPa (FTH; purple), and subsidence rate at 500 hPa (ω500 hPa; green) interpolated in time and space to the trajectory in 15 min intervals.
The Re retrieved using the 3.7 µm channel from MODIS is in good agreement (within the noise of the instruments and differences between a layer mean average and a retrieval near cloud top; in this case about 2 µm) with that retrieved from the ARM MFRSR instrument (Fig. 2c). This is further confirmed through examination of coincident ARM retrievals of Re from an Eulerian perspective (centered over the ARM site; Fig. S2). Figure S2 reveals a bias in the simulated Re from E3SMv1 using the COSP satellite (observation) simulator. The bias remains despite changing the autoconversion parameter values or from even turning off anthropogenic aerosol emissions (i.e., similar to a run based on pre-industrial data) altogether. For the first half of the trajectory the cloud fraction is 1.0 (Fig. 2c), but shortly after passing the ARM site the cloud fraction decreases in the wake of the island and then recovers to ∼ 0.6 about 6 h later. The Froude number was estimated to be 2.5 for this case, thus indicating that the flow was not blocked by the islands within the Azores, and a wake in the lee of the island is observable in Movie S1. The gradual increase in sea surface temperature over the trajectory (Fig. 2d) and cloud clearing possibly from the island wake result in larger observed brightness temperatures downstream from the island where warmer sea surface temperatures (SSTs) occur. Due to the potential impact of the island topography on the cloud properties, we stratify trajectories using a wide range of meteorological indices in this study, including Froude number.
3.2 Screening procedure
A total of 1589 (96 h) trajectories are calculated for the time period between 22 June 2016 and 28 October 2020 based on a once-per-day strategy. We use two separate screening approaches to (1) examine the effect of precipitation along back trajectories (Sect. 4.1) on measured and simulated CCN concentration and (2) quantify the aerosol indirect radiative effect and meteorological drivers on warm cloud at Graciosa Island in forward trajectories (Sect. 4.2–4.5). For the first analysis, we consider all precipitation types (shallow and deep convection) occurring in the air mass of all back trajectories and split trajectories into those with relatively low and high median rates of precipitation.
For the second analysis, we restrict the selection criteria to only single-layer warm (cloud top temperature greater than 273 K), low-level (cloud top pressure greater than 500 hPa), and liquid-phase clouds along both the backward and forward trajectories. This screening criteria is essential to avoid uncertainties related to glaciation indirect effects involving aerosol–ice–cloud interactions (Lohmann, 2002). A time step in the trajectory is ignored if any mixed-phase or ice clouds are detected. If ice cloud is found to occur in more than 25 % of the time steps, the whole trajectory is removed from the analysis. Trajectories are split into two categories based on their initial value of CCN concentration. The peak of the CCN concentration distribution at the ARM Graciosa Island site is approximately 110 cm−3. Therefore, to ensure a similar number of samples between the distributions, a trajectory is considered clean if CCN < 110 cm−3 and polluted if CCN > 110 cm−3.
The spatial distribution of the trajectories of this study are shown in Fig. S3. Trajectories mostly originate from the southwest and translate to the northeast over time, which is consistent with the dominant wind flow pattern in this region (Wood et al., 2017).
4.1 Back trajectories: precipitation and CCN
Is the ARM measured CCN concentration affected by precipitation along back trajectories? The CCN budget is largely dictated by aerosol sinks (via dry deposition, wet deposition, and advection), surface sources (e.g., sea spray production, horizontal and vertical advection, or new particle formation and growth), and aerosol chemistry in this region (Wood et al., 2017). Figure 3 shows two time series of CCN concentrations from both ARM measurements and E3SM simulations. A seasonal cycle is displayed in both data sets, with larger values of CCN concentration seen in the summer and lower values seen in the winter. On average, ARM shows larger variations in hourly measurements of CCN concentration compared to the E3SM model, but these variations tend to decrease at longer averaging timescales (e.g., the 2-week running mean shows similar characteristics). The larger hourly variability may be caused by differences in the following properties: (1) spatial scales between point location and grid box mean values as suggested by Schutgens et al. (2016), (2) land area representation in E3SM, and (3) local-scale island emissions. The minima in winter months are likely associated with more frequent passages of frontal systems and cold air outbreaks producing more precipitation and cleaner atmospheric conditions (Wood et al., 2017).
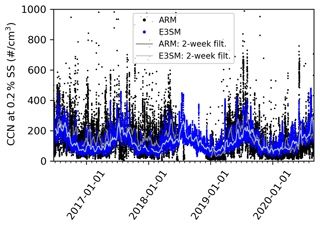
Figure 3(a) Cloud condensation nuclei concentration at 0.2 % supersaturation are measured from ARM (black) and simulated using E3SM (blue) at the ENA site over the period 21 June 2016–29 August 2020. A 2-week filter is applied to the hourly data for ARM (gray) and E3SM (light blue) data sets.
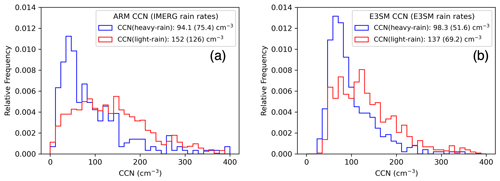
Figure 4(a) Relative frequency values in the distribution of CCN concentration measured at 0.2 % supersaturation observed by ARM at the ENA site are provided for trajectories with above (blue) and below (red) median accumulated IMERG precipitation values in the previous 48 h along the back trajectories. Panel (b) shows the same distributions from the same trajectories but using E3SM CCN concentration and precipitation rates instead. Mean and standard deviation values (shown in parentheses) are provided for each distribution.
Figure 4a shows a histogram of the CCN concentration for trajectories sorted by precipitation state. The average accumulated precipitation amount from the IMERG and E3SM data sets along 48 h back trajectories is 2.50±7.5 mm and 2.48±4.68 mm, respectively. The accumulation totals are about the same between observations and the model, but the standard deviation is larger in the IMERG data, which denotes a wider range of estimates than E3SM. Trajectories are sorted into relatively low and high values of the accumulated average precipitation rate with a threshold of 0.05 mm h−1 (depicted in Fig. S4). Figure 4a shows that there is a 40 % decrease in the mean CCN concentration at the ARM ENA site when above-median precipitation values are present 48 h prior to the measurement time. In addition, we find that CCN concentration is decreased when above-median precipitation occurs even in an Eulerian framework (Fig. S5) but with only a slightly less pronounced change than when using a Lagrangian perspective as compared to the results displayed in Fig. 4.
The extent to which wet deposition is responsible for the reduction in measured CCN concentration or that this difference in CCN can be explained by the advection of different air masses with different types of aerosols in the back trajectories is examined using the aerosol budgets from the MERRA-2 reanalysis data. Evidently, sea salt and sulfate make up the bulk of the AOD in this region (Fig. 5a). The change in total AOD over time along trajectories is slightly positive, but the changes are broadly similar for each aerosol species. In addition, Fig. S6 shows that the difference in AOD between clean and polluted composites is roughly in balance for each aerosol species over the course of the trajectory. This result may imply that the aerosol sinks and sources are also roughly in balance along the trajectories on average and that the changes associated with CCN concentration may be tied to local-scale disturbances caused by precipitation and not by the advection of changing aerosol concentrations along the trajectories. This is in general agreement with the CCN closure model used by Wood et al. (2017), which found that the CCN concentration is strongly tied to wet deposition of aerosol by the precipitation, particularly when clouds have high LWPs.
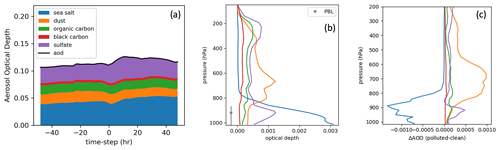
Figure 5(a) Aerosol optical depth decomposed into sea salt (blue), dust (orange), organic matter (green), black carbon (red), and sulfate (purple) from MERRA-2 averaged along backward and forward trajectories over the period 21 June 2016–29 August 2020. (b) Vertical profile of the mean aerosol optical thickness for each species in each of the 72 pressure levels at the location of the ARM site at ENA. The average PBL height plotted over 2 standard deviations is provided along the y axis. (c) Change in AOD between polluted and clean trajectories based on above- and below-median CCN concentration measured from ARM.
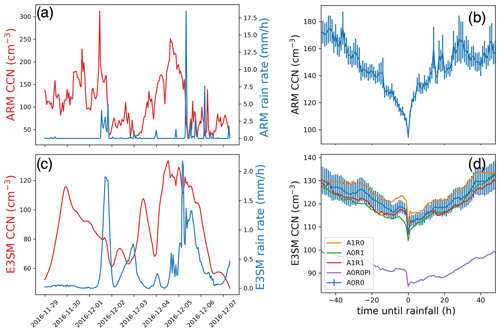
Figure 6(a) CCN concentration and rain rate from ARM hourly measurements. (b) CCN concentration measured at ARM averaged over the period 21 June 2016–29 August 2020 grouped by the amount of time until a rain event occurs with a threshold above 0.05 mm h−1. (c) The same as (a) except using simulations from the E3SM model A0R0 model run. (d) The same as (b) except using simulations from each E3SM experiment. Note that the range of CCN (y axes) values is larger in ARM compared to E3SM.
The CCN concentration typically decreases following the passage of precipitation over the ARM site. This effect is shown in an Eulerian framework using disdrometer precipitation measurements (Fig. 6a) and E3SM simulations (Fig. 6c). The CCN concentration is linked in time to the presence of precipitation. This example shows that CCN concentration can both gradually decrease in the E3SM simulations over a couple days following relatively large precipitation rates (30 November 2016 to 2 December 2016) and rapidly decrease after more intense rainfall (5 December 2016). Figure 6b shows that on average there is a precipitous decline in CCN concentration in the hours prior to the occurrence of precipitation, with the largest declines occurring at the time of the rainfall (all prior times have to have either no rainfall or rainfall less than 0.05 mm h−1). The observations show a much sharper decline in CCN concentrations as a function of time until rainfall (−63 % using the ARM disdrometer and −28 % using IMERG over 20 h) compared to the E3SM simulations (−9 %). At the time of rainfall, CCN concentration decreases as hourly rain rates increase (R) in the ARM observations ( cm−3 (mm h−1)−1), and this rate of change is more negative compared to the E3SM (A0R0) simulations ( cm−3 (mm h−1)−1) (Fig. S7).
The lack of a substantial difference in CCN concentration caused by precipitation between Eulerian and Lagrangian perspectives (i.e., differences between Figs. 4 and S5) in the observations may be due to insufficient sensitivity in the precipitation detection from the IMERG product and may be indicative of the weaker relationship found when examining this product in an Eulerian framework. Small differences between frameworks could also manifest if the CCN concentration is depleted rapidly in time with respect to the temporal time step of 1 h by precipitation. The precipitous drop in CCN concentration in the few hours within the time until a rain event occurs may indicate that precipitation is very effective at removing CCN from the atmosphere and could explain why Lagrangian and Eulerian frameworks have similar results for this set of particular variables in this location. However, we cannot entirely rule out the effects of shifting air masses, for example, the passage of cold fronts that cleanse the air mass before arrival at the ARM ENA site, which may also cause precipitous drops in CCN concentration in an Eulerian framework without detailed CCN measurements along trajectories.
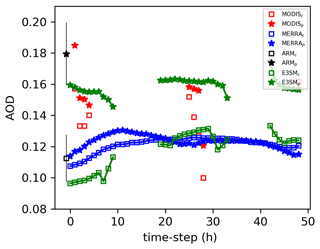
Figure 7Aerosol optical depth averaged along forward trajectories in MERRA-2, E3SM, MODIS, and ARM observations for trajectories with below-median (square) and above-median (star) initial CCN concentrations of 110 for MODIS, ARM, MERRA-2, and E3SM data sets.
A decrease in CCN concentration is simulated when the trajectories have precipitation rates greater than a threshold of 0.05 mm h−1 in the E3SM model (Fig. 4b), though the response in E3SM is only about half as large as in the observations (Fig. 4a). The lack of CCN removal in the model by precipitation may indicate that E3SM does not have strong enough wet deposition at this location for processing and removal of aerosol from the atmosphere. Increasing the accretion efficiency decreases the base state CCN concentration but not the rate of decline with respect to this timescale to precipitation occurrence (Fig. 6d). This inference is supported by similar CCN changes listed in Table 3. Interestingly, the change in CCN as a function of rain rate (Fig. S7) is similar amongst the various autoconversion and accretion experiments despite a 15 %–30 % stronger in-cloud wet deposition rate in the A1R0- and A1R1-based experiments (compared to the A0R0- and A0R0-based experiments) where the autoconversion rates are larger (Fig. S8 and Table S1). The fractional decrease in CCN concentration by precipitation is 2 times smaller in the pre-industrial atmosphere compared to the present day (Fig. S7). This result implies that precipitation is more efficient at removing aerosol from the atmosphere in present-day conditions, probably because there is simply more aerosol to remove. Unlike the observations that use IMERG, a Lagrangian perspective enhances the change in CCN concentration between precipitating composites compared to the Eulerian perspective in the E3SM model. Despite the enhancement in CCN removal by precipitation in the Lagrangian framework, a significant bias remains, and deeper investigation into the sources and sinks of the CCN concentration in the E3SM model is outside of the scope of this study but warranted for future releases of this version of the model.
Table 3Fractional change in cloud condensation nuclei (CCN) concentration at 0.2 % supersaturation based on trajectories sorted by above- and below-median precipitation rate (with a threshold of 0.05 mm h−1) for the IMERG and E3SM simulation experiments listed in Table 2. Precipitation is aggregated in both the Eulerian (EUL) and Lagrangian (LAG) frames of reference.
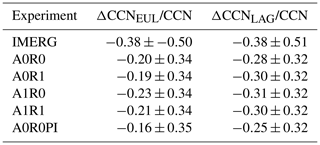
4.2 Forward trajectories: cloud radiative effects
Forward trajectories are used to quantify the cloud property perturbations to changes in aerosol concentration downstream from the ENA site. Trajectories are sorted by above- and below-median CCN concentrations (defined here as polluted and clean with a threshold of 110 cm−3), giving approximately 650 clean and polluted warm-cloud cases to quantify the aerosol response to warm clouds. Coincidentally, the two classes of trajectories flow in similar directions (Fig. S9), and as a consequence the meteorology is broadly consistent between them in both location (at the ENA site) (Fig. S10) and time along the trajectories (Fig. S11). While the meteorological means are within their respective ranges regarding the uncertainties between clean and polluted composites, even small changes in, for example, LTS (in this case being lower on average in the clean trajectories) could be relevant for the cloud properties (Klein and Hartmann, 1993), and this is why we bin by meteorological variable to examine the impact of aerosols in Sect. 4.4.
Figure 7 generally shows that higher AOD is associated with the more polluted trajectories with larger concentrations of CCN. Despite the relatively higher noise in MODIS, AOD remains elevated in the available hourly intervals along trajectories that are polluted (star) compared to clean (square) samples. While this difference is substantial (albeit less so in MERRA-2, which may suggest deeper investigation into MERRA-2's ability to represent the evolution of aerosols) the AOD differences tend to be largest at the start of the trajectory (i.e., at t=0) and gradually become smaller over the next 48 h. As a result, when higher concentrations of CCN are measured at the ARM site, CCN likely remains elevated in the atmosphere for multiple days, as inferred by the change in downstream AOD. As expected, there is a larger concentration of below-cloud Aitken, accumulation, and coarse-mode aerosol under present-day polluted conditions compared to present-day clean aerosol simulations and pre-industrial aerosol simulations in E3SMv1 (Fig. S12). The extent to which the elevated aerosol concentrations influence cloud properties and radiative budgets along trajectories is examined next.
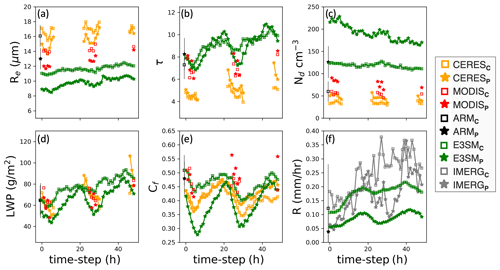
Figure 8Droplet effective radius (a), cloud optical thickness (b), droplet number concentration (c), liquid water path (d), liquid cloud fraction (e), and precipitation rate (f) averaged along the forward trajectories initialized from the ENA site where the air mass is considered polluted (star) and clean (square) for CERES (orange), ARM (black), MODIS (red), and E3SM (green).
4.3 Aerosol influence on cloud properties
Figure 8a shows that smaller Re manifests in more polluted conditions (trajectories with higher CCN concentration). This result is consistent with other studies using ARM (Dong et al., 2015), satellite (Christensen et al., 2020), and modeling (Yamaguchi et al., 2017) data. Furthermore, the Lagrangian framework reveals that Re remains smaller in polluted clouds even 20–30 h after being sampled at the ARM site. This result is consistent with the elevated AOD over the trajectory and generally agrees with the central premise of the Twomey effect in which a larger concentration of CCN produces a larger concentration of smaller cloud droplets (the computation of Nd is described in Sect. 4.5). Interestingly, the CERES SYN retrievals of Re remain smaller in polluted clouds even at night (not shown) when the retrievals rely exclusively on near-infrared brightness temperature and are thus much less trustworthy. On average, cloud optical thickness and Nd (Fig. 8b, c) are larger in clouds (although Nd has a much larger difference than optical depth due to the LWP differences that are opposite) that are polluted compared to those that are unpolluted. LWP and CF (Fig. 8d, e) exhibit average decreases at elevated CCN concentration in most data sets (except at nighttime in CERES data where the retrievals are less trustworthy and in MODIS on the second day along the trajectory). These estimates are also provided in Tables 5 and 7. The decrease in liquid water path and cloud fraction occur despite the significant suppression of precipitation in polluted conditions on average (Fig. 8f), which is a response that would increase LWP rather than decrease it. A possible explanation for the polluted clouds that lose water despite moistening by drizzle suppression is that the evaporation may be more vigorous due to differing meteorological factors compared to the clean clouds with more liquid water (Chen et al., 2014).
Table 4List of radiative effects decomposed into contributions from the Twomey effect and liquid water path and cloud fraction adjustments averaged over the daylight period (roughly from 09:00 to 15:00 LT) of the first day of each trajectory.
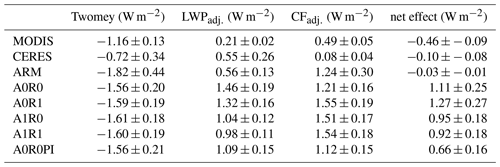
Table 5List of quantities used to compute the aerosol indirect radiative effect averaged over the daylight period (roughly from 09:00 to 15:00 LT) of the first day of each trajectory.
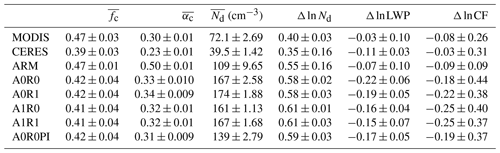
Table 6List of radiative effects decomposed into contributions from the Twomey effect and liquid water path and cloud fraction adjustments averaged over the daylight period (roughly from 09:00 to 15:00 LT) of the second day of each trajectory.
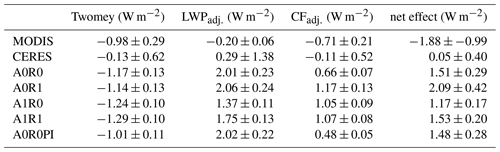
4.4 Meteorological factors
We seek to understand why the LWP and CF decrease at elevated CCN concentration by examining the aerosol response to several key geophysical variables: LTS, FTH, 500 hPa free-tropospheric vertical velocity, free-tropospheric vertical velocity ω500, degree of cloud coupling to surface moisture, fraction of the aerosol residing in the PBL (compared to the free troposphere), precipitation state, and Froude number. We compute the degree of decoupling based on the difference in ceilometer cloud base height and lifted condensation level (computed using radiosonde observations with a parcel height level of approximately 100 m above the surface at 10:00 LT). If the distance is less than 300 m, we consider the PBL to be well mixed with a higher likelihood of surface aerosol mixing with overlying clouds according to Comstock et al. (2005). An example of the method is displayed in Fig. S13. We find that the selection of warm clouds at ENA are more often decoupled to the surface moisture supply (about 54 %); Rémillard et al. (2012) found that despite a high frequency of stratocumulus clouds in the Azores, the PBL is almost never well mixed but is often in a meteorological state where the cumulus clouds are coupled to the surface moisture supply.
Does the vertical distribution of AOD and its location in the vertical influence cloud properties? Since aerosol can sometimes reside above the cloud tops and be poorly co-located with the clouds, we extracted the vertical profile of AOD along trajectories using the MERRA-2 data and computed it separately for the PBL and free troposphere using lookup tables for the dry mass extinction coefficients for each aerosol species (see Supplement Table S1 in Randles et al., 2017). The mean PBL height as determined from MERRA-2 is 912 hPa at the ENA ARM site. We estimate that about 55 % of the daily 12:00 LT column-integrated AOD is located within the PBL (Fig. 5b). Because an appreciable amount of the aerosol loading can reside above the PBL, we quantify cloud property changes in response to changes in CCN concentration separately for cases in which a majority of the aerosol resides in the PBL versus times when it does not as determined by MERRA-2.
Does the island create a geophysical wake and influence the cloud properties in Lagrangian trajectories (thereby obscuring the aerosol–cloud relationship)? The interactions of the incoming winds and high mountainous areas induces a wind-sheltered area and wake effect in the lee of the island. Figure S14 clearly shows a strong decrease in cloud fraction on average in the wake of the Madeira archipelago – this is consistent with observations from Azevedo et al. (2021). It is also evident that LWP decreases in the wake of Madeira and that cloud droplet concentration remains constant. On the other hand, we do not see strong signatures of an island wake effect on the clouds downstream from ENA. Instead we observe local anomalies in the cloud properties at ENA, but these are on relatively short timescales (less than 30 min) and likely a retrieval artifact caused by surface inhomogeneities resulting from the enhanced reflectance over land compared to the ocean surface (Fig. S14b). Cloud properties are also examined as a function of Froude number (Fig. S15); however, changing the Froude number does not strongly influence the cloud properties above the statistical noise in the region, a result that broadly agrees with taller non-volcanic (control) islands of Ebmeier et al. (2014). Therefore, we conclude that while the wake generated by Graciosa and nearby islands in the Azores may influence clouds on specific days (e.g., Fig. 2a), it does not significantly influence the cloud properties in a multi-year average.
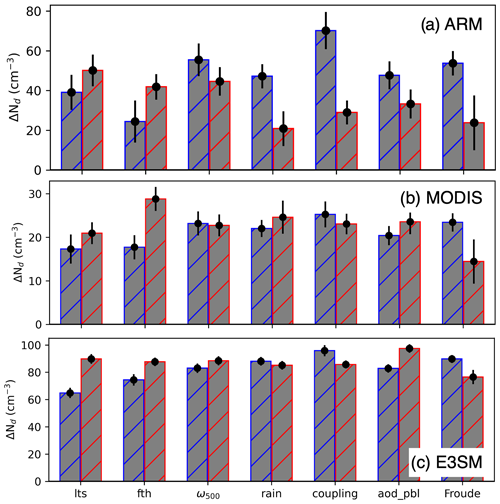
Figure 9Difference in cloud droplet number concentration (ΔNd) between polluted (CCN > 110 cm−3) and clean (CCN < 110 cm−3) trajectories within 6 h of the CCN measurement for (a) ARM, (b) MODIS, and (c) E3SM. Data are stratified by below (blue) and above (red) median lower tropospheric stability (LTS), free-tropospheric humidity (FTH), vertical velocity at 500 hPa (ω_500), rain rate (non-raining and raining), coupling strength (coupled and non-coupled), amount of aerosol in the PBL (aod_pbl), and Froude number (values 0–1 and 1–2). Error bars represent the 95 % confidence interval computed from a two-tailed t test. Hatching denotes statistically significant differences. Note that the range in ΔNd varies for each data set.
Taken together, Fig. 9 shows the average difference in Nd between clean and polluted clouds that have been stratified by low and high value thresholds of a variety of different meteorological composites. In general, Nd is larger in trajectories with higher CCN (i.e., a positive ΔNd value), and the differences amongst meteorological composites are not statistically significant outside of some notable exceptions. Higher Nd tends to be measured in polluted clouds when the atmospheric conditions tend to be more stable (larger ΔNd when the LTS is larger than the median conditions) and moist (using FTH). The variations in ΔNd across meteorological composites are slightly larger for ARM than for MODIS. ARM measurements indicate that significantly less ΔNd is measured in polluted clouds that are precipitating, but these retrievals may be affected by sub-cloud rain influencing the cloud retrieval (Wu et al., 2020), whereas rain contamination on the passive near-infrared cloud top retrievals from MODIS is smaller (Christensen et al., 2013). Overall, we observe broad agreement on the sign of ΔNd amongst MODIS, ARM, and E3SM, but the strength and sensitivity to environmental factors can vary significantly between them.
The difference in LWP (Fig. S16) and CF (Fig. S17) is more likely to become positive if the free troposphere is moist, the clouds are precipitating, or the Froude number is large, as indicated by the MODIS results. These results generally agree with the hypothesis that enhanced entrainment of dry air into polluted clouds causes them to lose more LWP (Chen et al., 2014) and that these responses are more likely to occur during summer months (not shown) when the atmosphere is drier compared to winter months (Dong et al., 2015; Wood et al., 2015). Strong positive ΔLWP in raining clouds is also in agreement with the CloudSat observations from Chen et al. (2014) and is hypothesized to result from the suppression of precipitation, which acts as a cloud moisture sink. There is also some evidence that greater losses in LWP and CF may occur when the clouds become polluted if clouds are less coupled to surface moisture or more of the aerosol is in the free troposphere. Decoupled clouds have been shown to be more susceptible to greater losses in LWP when they become polluted because there is less ability to transport moisture from near the surface and replenish the cloud (Zheng et al., 2022). While the signs of the responses from ARM are sometimes different from MODIS, the relative change between high and low for each of the meteorological composites is in broad agreement with MODIS and (to a lesser extent) E3SM simulations (e.g., ΔLWP and ΔCFliq are mostly negative across meteorological composites in E3SM but have much bigger variations in ARM and MODIS by comparison).
4.5 Effective radiative forcing by aerosol–cloud interactions (ERFaci)
The radiative effect due to changes in aerosol concentration is decomposed into contributions from the Twomey effect and adjustments caused by changes in LWP and CF. We use a bivariate statistics approach and split the data into “clean” and “polluted” states based on two methods: (1) using present-day composites of the data separated by CCN concentration and (2) using present-day (polluted) and pre-industrial (clean) conditions. Obviously, we can only assess ERFaci from satellite observations from the first approach, whereas the GCM is able to compute ERFaci using both approaches. Running a pre-industrial simulation also provides the needed constraint for determining annual mean incoming TOA shortwave radiation and anthropogenic aerosol fraction (to be used in approach 1).
4.5.1 ERFaci derivation
The change in reflected solar radiation caused by a change in the planetary albedo and Nd can be written as
where is the annual mean top of atmosphere (TOA) incoming solar radiation, taking a value of 329 W m−2 (is the daily mean annually averaged value at Graciosa Island), α is the planetary albedo, and is the change in droplet concentration due to anthropogenic activities, which can be approximated from the change in aerosol optical thickness (Quaas et al., 2008) from pre-industrial to present-day levels via , where ΔNd and Δτa are the changes in droplet concentration and aerosol optical thickness between clean and polluted conditions, respectively. Because pre-industrial aerosols cannot be obtained from satellite observations, we use E3SM to represent the aerosol change between present-day and pre-industrial aerosols (), which has a mean value of 0.392 for ERFaci estimates in both observations and models. α can be expanded into contributions from the surface and clouds following
where ϕatm is the transfer function that accounts for the average albedo of the air above the surface and clouds and takes an average value of 0.7 (Diamond et al., 2020). αc can be estimated using the two-stream delta-Eddington approximation assuming the surface albedo beneath the cloud is zero as follows:
where g is the asymmetry parameter and takes a value of 0.85 for liquid clouds and τc is the cloud optical thickness, which is approximated using an adiabatic assumption as L⅚ , where kg m, L is the LWP, which is approximated as (Stephens, 1978) using the density of water (ρw), cloud droplet effective radius (re), and cloud droplet concentration (Nd). We use the equivalent form of , where m−0.5 to compute cloud droplet number concentration from cloud effective radius and optical depth variables retrieved from ARM and satellites (MODIS and CERES) and obtained using COSP in E3SM simulations. Grosvenor et al. (2018) can be consulted for a comprehensive assessment of the Nd uncertainties. Taking the derivative of α with respect to Nd gives
where cloud-free conditions give . The chain rule expansion of can be solved by the following two derivatives: (1) and (2) . Combining with Eq. (3) gives the following equation:
which is used to compute the aerosol indirect shortwave radiative effect. Here, single-directional difference quotients () are represented as a linear relationship; however, they depend upon meteorological conditions and the background aerosol conditions (Glassmeier et al., 2019).
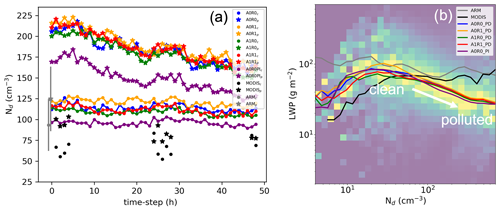
Figure 10(a) Cloud droplet concentration for clean (circle) and polluted (asterisks) air masses averaged along forward trajectories from each E3SM experiment. (b) Joint histogram of the liquid water path (LWP) and cloud droplet number concentration for E3SM. Median LWP is plotted over the joint histogram for each observational and simulation-based data set.
4.5.2 Present-day ERFaci based on CCN subsets (first approach)
The Δ terms represent differences in cloud properties between the clean and polluted state based on measured concentrations of CCN at the ARM site. The differences are computed between the clean and polluted forward trajectories at each time step. The first term in brackets is commonly referred to as the Twomey effect, denoting the change in shortwave reflection assuming liquid water path and cloud fraction terms (the following two terms) are zero. Liquid water path and cloud fraction changes are commonly referred to as radiative adjustments of the Twomey effect (IPCC, 2013).
Radiative effect estimates from trajectories intersecting the ENA site are displayed in Table 4, and associated cloud quantities are listed in Table 5. The Twomey radiative effect is negative in all data sets and spans the range from −0.72 to −1.82 W m−2. This is a wider range of estimates compared with global observation-based and model-based estimates (e.g., see Quaas et al., 2008; Lebsock et al., 2008; Christensen et al., 2017) and may be due to sampling a particular cloud environmental regime that is more susceptible to aerosol perturbations than the aggregated global mean value; further analysis contrasting other sites and regions will be examined in follow-up work.
The Twomey effect is more negative in the E3SM model and ARM observations (averaged over the first day of the Lagrangian trajectory) in part because these data sets exhibit larger increases in Δln Nd under polluted conditions (i.e., larger values of Δln Nd) compared to the retrievals from the satellite observations. The Twomey effect is also inversely proportional to Nd; thus, larger values of Nd result in smaller radiative effects. Part of the reason why the Twomey effect in ARM data is much more negative than the satellite-estimated values is because the cloud albedo and Nd are nearly 2 times larger. Similarly, the mean Nd in the E3SM model is significantly larger than in the observations. Despite the relatively large Nd in ARM, E3SM remains an outlier with respect to the observations of Nd and provides estimates of the Twomey radiative effect to within 30 % of the observations.
The modification of the autoconversion and accretion parameterizations does not significantly influence the Twomey radiative effect (Tables 4 and 6) or base state cloud variables (i.e., cloud fraction, cloud albedo, and droplet number concentration; Tables 5 and 7). However, the LWP radiative adjustment becomes smaller for the stronger autoconversion experiments (i.e., A1 compared to A0), and this is consistent with stronger precipitation suppression when the dependence of precipitation is greater with larger Nd (also shown in prior studies; Gettelman et al., 2021). Overall, the modification of these autoconversion and accretion factors has a small influence on the time series of the CCN concentration (not shown) and Nd (Fig. 10a) and the aerosol indirect radiative effect (Table 4) over this parameter space. A much larger and more pronounced effect on these variables occurs when the anthropogenic aerosol emissions are turned off in the model (A0R0PI simulation). This result implies that, despite recent upgrades to the E3SM code base (Wang et al., 2020), there remains a relatively weak connection between the warm-rain process, specifically autoconversion and accretion, and cloud radiative effects in the model.
The ENA site shows a negative LWP response to increasing aerosol concentrations in E3SM and observational data sets. This leads to a positive radiative effect despite the large increase in ΔNd (Fig. 10a) and outweighs the cooling caused by the Twomey radiative effect. The negative adjustments are likely driven by the strong descending branch in the LWP–Nd relationship (Fig. 10b). This effect is robust in the pre-industrial experiments and all present-day autoconversion and accretion experiments. The descending branch may be attributed to enhanced entrainment drying on clouds as they become increasingly polluted (Gryspeerdt et al., 2019). While the mechanism for entrainment drying is included in E3SMv1 via the connection between aerosols and droplet sedimentation (Bretherton et al., 2007), we speculate that the droplet population may not have enough time to separate vertically over the integration timescale (Karset et al., 2020), and therefore we do not expect the model to simulate negative slopes for LWP as a function of Nd (and yet it does). Thus, further developments on improving the biases with respect to CCN concentration, precipitation rate, and Nd may be needed to bring radiative forcing estimates into better agreement with the observations.
The net indirect radiative effect on the first day along the trajectories takes on a positive value in E3SM simulations despite the negative contributions from the Twomey effect. The positive warming effect manifests from the decrease in both LWP and CF as CCN levels increase. These adjustments are nearly as large in magnitude as the Twomey effect in the E3SM model. As a consequence, the net indirect radiative effect takes on a positive value after these contributions are added to the Twomey effect. The satellite and ground-based measurements also show positive LWP and CF adjustments; however, their contributions are much smaller by comparison to the Twomey effect. As a result, the net indirect radiative effect remains negative in the observational data sets.
Cloud responses to changes in CCN concentration also change between days 1 and 2 along the trajectories (Tables 5 and 7). While the Δln Nd remains positive on day 2, it decreased by 32 % in MODIS observations and 15 % in E3SM simulations. The LWP and CF adjustments are less consistent in their change between days. MODIS observations show such a reversal of the sign on the second day that polluted clouds become associated with larger cloud fraction and liquid water paths. This result could imply that microphysical changes associated with increased aerosol loading are less affected over time compared to macrophysical cloud properties such as LWP and CF that may be more affected by meteorology, precipitation, and evaporation processes where Nd may be less impacted because it is considered a mediating variable that is less directly influenced by relative humidity (Gryspeerdt et al., 2016). Another potential caveat is the influence of the diurnal cycle on the cloud properties. LWPs decreased more in polluted clouds during the afternoon and evening hours, a result that broadly agrees with observations of polluted cloud tracks (Rahu et al., 2022). The cloud fraction is decreased in polluted clouds the most during the night into early morning hours in the E3SM model.
4.5.3 Present-day minus pre-industrial ERFaci (second approach)
Here, the Δ terms in Eq. (5) represent differences in cloud properties between the present-day and pre-industrial atmosphere from E3SM. Table 8 shows some notable differences between using the bivariate statistics regression approach using ΔCCN (first approach) and Δ(PD-PI) in computing ERFaci. Firstly, Nd is significantly larger, Re is significantly smaller, and cloud optical thickness is larger with present-day emissions. This agrees with our first approach and the general Twomey hypothesis. Interestingly, LWP increases from the pre-industrial period to present-day conditions, and thus it does not decrease as shown using the bivariate statistics of ΔCCN. As a consequence, a stronger negative net radiative cooling effect can be seen. Similarly, CF increases, thereby leading to additional cooling (not warming as seen before).
(Ghan, 2013)Table 8List of cloud and radiative effects from aerosol perturbations based on present-day values relative to pre-industrial aerosol simulations. ERFaci estimates are provided separately for the decomposition by Twomey, LWPadj, and CFadj, in addition to those computed using the method described in Ghan (2013).
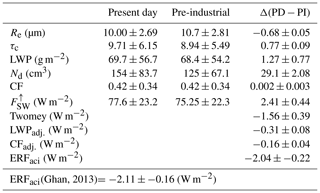
The discrepancy between the bivariate statistical approaches may result from a variety of factors (e.g., see Ghan et al., 2016; Bellouin et al., 2020) that can be broadly summarized here as follows: (1) co-variability between meteorology and the cloud variables may affect the sensitivities in and using the ΔCCN approach; (2) assumptions in Eq. (5) (ϕatm=0.7, g=0.85, and the adiabatic approximation for estimating LWP and Nd, to name a few) may not be reasonable for certain types of clouds, such as those where the cloud fraction is low (Coakley et al., 2005), decoupled from the surface, or precipitating; (3) the estimate of the pre-industrial aerosol state is poorly constrained and uncertain (Carslaw et al., 2013); and (4) shifts in cloud properties in the pre-industrial simulation have smaller Nd but with decreased LWP and CF effects relative to Twomey (see Table 4). We compute a residual term in the calculation of ERFaci of 0.37 ± 0.18 W m2 as determined from the difference between ERFaci and ΔF in Table 8. The size of the residual suggests that this approach captures most of the variability and that the decomposition of ERFaci is accurate to within 85 %. Based on this level of accuracy, we are confident in the estimates of the Twomey effect but less confident that the changes in LWP and CF are statistically different from zero. We aim to determine which of these factors is most responsible for the computed residual and the differences between the bivariate statistics for ΔCCN and Δ(PD-PI) in computing ERFaci in a follow-up study.
As a final check, we have estimated ERFaci using the same approach as that described in Ghan (2013). This method uses the difference in cloud radiative forcing between present-day and pre-industrial aerosol emissions, i.e., ), where Fclean is the top of model net radiative flux, neglecting the scattering and absorption of solar radiation by all of the aerosol, and Fclear is the flux calculated as a diagnostic with clouds neglected. Using this method we estimate ERFaci to be −2.011 W m2, which is nearly identical in strength to the combined Twomey, LWPadj, and CFadj radiative effects discussed previously (Table 8).
This study utilizes a Lagrangian framework to characterize the radiative effect of aerosols on clouds passing by the ARM site at Graciosa Island. This framework is applied to over 1500 trajectories that track warm boundary layer clouds at distances of several hundred kilometers in both satellite observations and GCM simulations. This approach utilizes ground-based measurements of CCN concentration instead of satellite-retrieved AOD, thus bypassing the need to rely exclusively on clear-sky conditions to initialize trajectories. Below we summarize answers to the key scientific questions of this study.
-
Is the Lagrangian framework advantageous for quantifying the relationship between CCN concentration and precipitation occurrence? In agreement with Wood et al. (2017), we show that CCN concentrations precipitously decline in association with precipitation occurring along back trajectories. We find increased sensitivity in the E3SM model when using a Lagrangian framework, but this relationship was relatively unchanged by the autoconversion or accretion experiments conducted in the E3SM model. An analysis using an Eulerian framework found similar results but with only slightly lower sensitivity than in the satellite observations, possibly due to sensors that are not able to capture light precipitation.
-
Do cloud properties evolve differently under varying levels of measured CCN? Yes, our analysis shows that clouds tend to have higher Nd at higher starting concentrations of CCN and that Nd and other cloud properties remain perturbed downstream from ENA for several days, albeit with decreasing strength over time. E3SMv1 is able to capture the temporal response in ΔNd, but the decrease over time is weaker than the observations would otherwise suggest. These perturbations result in aerosol indirect radiative effects. While the microphysical changes in the cloud lead to a cooling Twomey radiative effect, we also find substantial warming radiative effects using decreases in LWP and CF that are observed in satellite observations and simulated with larger estimates in the E3SM model. The positive LWP and CF adjustments may be the result of the bivariate statistical sampling of clean and polluted samples occurring on the descending branch of the LWP–Nd relationship (Fig. 10b). E3SM simulations show that LWP and CF actually increase from the pre-industrial to present-day aerosol emissions, which causes even more radiative cooling using the PD–PI approaches described here and using the Ghan (2013) method. The lack of agreement between the bivariate regression and PD–PI sampling approaches is large, with a complete reversal of ERFaci suggesting that further assessments of the Twomey, LWP, and CF decomposition calculations, which many other studies utilize (Quaas et al., 2008; Goren and Rosenfeld, 2015; Bellouin et al., 2013; Christensen et al., 2017; Toll et al., 2019; Christensen et al., 2020), are needed to assess factors that may violate assumptions using these approaches.
-
Is the Lagrangian flow affected by Graciosa Island? We do not find strong evidence of the flow affecting the statistical relationships between CCN concentration and cloud properties when averaged across multiple years. Although an island wake effect can be observed in individual case studies (e.g., Fig. 2), analysis of the Froude number suggests leeward wakes capable of strongly influencing cloud properties are small on average (Figs. S14 and S15).
-
Is the vertical distribution of AOD correlated with surface-based CCN concentration? We observe increased Nd in overlying clouds for larger values of surface-measured CCN regardless of the degree of surface-to-cloud coupling. Nd remained elevated despite conditions when the bulk of the aerosol resided above the planetary boundary layer (Fig. 5b) as determined from the MERRA-2 reanalysis product. These responses are robust across a multitude of meteorological parameters and factors that are influenced by the island wake effect.
In general, E3SM simulates positive LWP and CF radiative adjustments (using ΔCCN as a proxy for bivariate regression method 1 statistics) regardless of the meteorological state. While the differences between E3SM and the observations are notable, the lack of consistency between ARM, MODIS, and CERES makes it difficult to constrain cloud system behavior based on different meteorological states in the simulations. This challenge was also identified in Neubauer et al. (2017), in which the sign of the LWP response from MODIS and the Advanced Along-track Scanning Radiometer to changing aerosol optical thickness did not agree as a function of different meteorological states. Diamond et al. (2020) demonstrate that at least 5 years of observational data are needed to detect a sufficient signal-to-noise ratio response in cloud properties to a change in aerosol (where aerosol sources are known, in this case a shipping corridor). Thus, this study may be at the limits of having enough observational data collected to be a useful constraint for aerosol–cloud interactions after subdivision of the data into meteorological composites. However, these smaller but detailed ground-based data sets are essential for model evaluation when the methods applied to nudged models by reanalysis data and observations are consistent.
One targeted improvement to the observations might be to incorporate ground-based retrievals of precipitation using a scanning radar. This would improve detection of drizzle along trajectories; however, these types of data are sparse in the region, and the horizontal range of a typical X-band radar cannot cover a typical multi-day trajectory that spans hundreds to thousands of kilometers. Complete coverage via other ocean-based platforms or spaceborne retrievals of precipitation at geostationary orbit are not feasible or possible in the near-term future. Combining the retrievals from CloudSat with those from other passive radars has been shown to be useful for increasing the detection of light precipitation (Eastman et al., 2019). In addition, the satellite-retrieved Re and cloud optical thickness data that are used to estimate Nd have large uncertainties from passive remote sensors (upwards of 80 % for Grosvenor et al., 2018) due to invoking a variety of assumptions on the cloud state (one being cloud adiabaticity Merk et al., 2016) in the retrieval calculation and instrument limitations described in Grosvenor et al. (2018) and may be better constrained when combined with detailed ground-based ARM retrievals.
The E3SMv1 model showed that there is less efficient CCN concentration loss by precipitation compared to ARM observations. This may be due to the lack of mesoscale cloud systems, which cannot be properly simulated at coarse 1∘ scales. GCMs with higher resolution (typically 25–50 km scales) generally show improvement in the simulation precipitation frequency and intensity (Haarsma et al., 2016) unless the parameterization schemes are not suited to resolution (Xie et al., 2018). In addition, a lack of strong aerosol perturbations caused by local-scale aerosol sources (e.g., airports) may not be represented well at these coarse scales and potentially affect aerosol–cloud susceptibility. Lastly, the choice of the solubility factor and scavenging coefficient in the aerosol wet deposition scheme in EAMv1 (based on Wang et al., 2013) for improving long-range transport of aerosols could contribute to the lower scavenging efficiency compared to the point measurements. In future work we plan to examine these impacts from a regionally refined mesh (Tang et al., 2019) where precipitation and highly concentrated aerosol plumes may not be overly smoothed by the coarse grid and compare better to observations.
Finally, a series of autoconversion and accretion experiments were carried out to determine whether the sensitivity of the warm-rain process is tied to the microphysical and macrophysical properties of the clouds. In general, we find only small changes in the Twomey effect amongst experiments but larger impacts on the LWP and CF radiative forcing adjustments (with an unexpected positive sign). While the radiative forcing estimates are in fair agreement with the satellite observations, E3SM simulations suggest that aerosol activation, warm-rain processes, and or turbulence parameterizations may require further modification (such as including improved parameterizations of entrainment and wet deposition) to achieve better agreement with base state variables (such as cloud droplet concentration) and ERFaci compared to satellite and ARM observations.
All ARM products listed in Table 1 are available at https://www.arm.gov/data/ (last access: 17 August 2022). The CERES SYN Ed4a 4 product is available at https://ceres.larc.nasa.gov (last access: 17 August 2022). The MODIS collection 6 MYD08 D3 product is available at https://earthdata.nasa.gov (last access: 17 August 2022). IMERG data are available from the NASA Goddard Space Flight Center at https://pmm.nasa.gov (last access: 17 August 2022). MERRA-2 data were obtained from https://goldsmr4.gesdisc.eosdis.nasa.gov/data/MERRA2/ (last access: 17 August 2022; NASA, 2023). The HYSPLIT trajectory code is available at https://www.ready.noaa.gov/HYSPLIT.php (last access: 17 August 2022; NOAA, 2023). All data and code availability websites were last accessed on 17 August 2022.
Movies S1 and S2 related to this article are available in the Supplement. The supplement related to this article is available online at: https://doi.org/10.5194/acp-23-2789-2023-supplement.
MWC wrote the manuscript and developed the Lagrangian trajectory approach and analysis. PLM guided the implementation of the E3SMv1 simulations. Research and development ideas, as well as writing and editing, were contributed to by PLM, PW, ACV, JM, and JDF.
The contact author has declared that none of the authors has any competing interests.
Publisher’s note: Copernicus Publications remains neutral with regard to jurisdictional claims in published maps and institutional affiliations.
We would like to thank the anonymous reviewers for their insightful and helpful feedback. This research was supported by the “Enabling Aerosol cloud interactions at GLobal convection-permitting scalES (EAGLES)” project (74358), funded by the U.S. Department of Energy, Office of Science, Office of Biological and Environmental Research, Earth System Model Development program area. This research used resources of the National Energy Research Scientific Computing Center (NERSC), a U.S. Department of Energy Office of Science User Facility located at Lawrence Berkeley National Laboratory, operated under contract no. DE-AC02-05CH11231 using NERSC awards ALCC-ERCAP0016315, BER-ERCAP0015329, BER-ERCAP0018473, and BER-ERCAP0020990. The Pacific Northwest National Laboratory is operated for the U.S. Department of Energy by Battelle Memorial Institute under contract no. DE-AC05-76RL01830.
This study was funded by the U.S. Department of Energy, Office of Science, Office of Biological and Environmental Research, Earth System Model Development (ESMD) program area (project no. 74358). The Pacific Northwest National Laboratory is operated for the U.S. Department of Energy by Battelle Memorial Institute (contract no. DE-AC05-76RL01830).
This paper was edited by Yuan Wang and reviewed by three anonymous referees.
Azevedo, C. C., Camargo, C. M. L., Alves, J., and Caldeira, R. M. A.: Convection and Heat Transfer in Island (Warm) Wakes, J. Phys. Oceanogr., 51, 1187–1203, https://doi.org/10.1175/Jpo-D-20-0103.1, 2021. a
Bellouin, N., Quaas, J., Morcrette, J.-J., and Boucher, O.: Estimates of aerosol radiative forcing from the MACC re-analysis, Atmos. Chem. Phys., 13, 2045–2062, https://doi.org/10.5194/acp-13-2045-2013, 2013. a
Bellouin, N., Quaas, J., Gryspeerdt, E., Kinne, S., Stier, P., Watson-Parris, D., Boucher, O., Carslaw, K. S., Christensen, M., Daniau, A.-L., Dufresne, J.-L., Feingold, G., Fiedler, S., Forster, P., Gettelman, A., Haywood, J. M., Lohmann, U., Malavelle, F., Mauritsen, T., McCoy, D. T., Myhre, G., Mülmenstädt, J., Neubauer, D., Possner, A., Rugenstein, M., Sato, Y., Schulz, M., Schwartz, S. E., Sourdeval, O., Storelvmo, T., Toll, V., Winker, D., and Stevens, B.: Bounding Global Aerosol Radiative Forcing of Climate Change, Rev. Geophys., 58, e2019RG000660, https://doi.org/10.1029/2019RG000660, 2020. a, b
Bodas-Salcedo, A., Webb, M. J., Bony, S., Chepfer, H., Dufresne, J.-L., Klein, S. A., Zhang, Y., Marchand, R., Haynes, J. M., Pincus, R., and John, V. O.: COSP: Satellite simulation software for model assessment, B. Am. Meteorol. Soc., 92, 1023–1043, https://doi.org/10.1175/2011BAMS2856.1, 2011. a
Bogenschutz, P. A., Gettelman, A., Morrison, H., Larson, V. E., Craig, C., and Schanen, D. P.: Higher-Order Turbulence Closure and Its Impact on Climate Simulations in the Community Atmosphere Model, J. Climate, 26, 9655–9676, https://doi.org/10.1175/JCLI-D-13-00075.1, 2013. a
Bretherton, C. S., Blossey, P. N., and Uchida, J.: Cloud Droplet Sedimentation, Entrainment Efficiency, and Subtropical Stratocumulus Albedo, Geophys. Res. Lett., 34, L03813, https://doi.org/10.1029/2006gl027648, 2007. a
Carslaw, K. S., Lee, L. A., Reddington, C. L., Pringle, K. J., Rap, A., Forster, P. M., Mann, G. W., Spracklen, D. V., Woodhouse, M. T., Regayre, L. A., and Pierce, J. R.: Large contribution of natural aerosols to uncertainty in indirect forcing, Nature, 503, 67–71, https://doi.org/10.1038/nature12674, 2013. a
Chen, Y.-C., Christensen, M. W., Stephens, G. L., and Seinfeld, J. H.: Satellite-based estimate of global aerosol-cloud radiative forcing by marine warm clouds, Nat. Geosci., 7, 643–646, https://doi.org/10.1038/ngeo2214, 2014. a, b, c, d
Christensen, M. W., Stephens, G. L., and Lebsock, M. D.: Exposing biases in retrieved low cloud properties from CloudSat: A guide for evaluating observations and climate data, J. Geophys. Res.-Atmos., 118, 12120–12131, https://doi.org/10.1002/2013JD020224, 2013. a, b
Christensen, M. W., Neubauer, D., Poulsen, C. A., Thomas, G. E., McGarragh, G. R., Povey, A. C., Proud, S. R., and Grainger, R. G.: Unveiling aerosol–cloud interactions – Part 1: Cloud contamination in satellite products enhances the aerosol indirect forcing estimate, Atmos. Chem. Phys., 17, 13151–13164, https://doi.org/10.5194/acp-17-13151-2017, 2017. a, b, c
Christensen, M. W., Jones, W. K., and Stier, P.: Aerosols Enhance Cloud Lifetime and Brightness along the Stratus-to-Cumulus Transition, P. Natl. Acad. Sci. USA, 117, https://doi.org/10.1073/pnas.1921231117, 2020. a, b, c, d, e
Clothiaux, E. E., Miller, M. A., Perez, R. C., Turner, D. D., Moran, K. P., Martner, B. E., Ackerman, T. P., Mace, G. G., Marchand, R. T., Widener, K. B., Rodriguez, D. J., Uttal, T., Mather, J. H., Flynn, C. J., Gaustad, K. L., and Ermold, B.: The ARM Millimeter Wave Cloud Radars (MMCRs) and the Active Remote Sensing of Clouds (ARSCL) Value Added Product (VAP), NA, https://doi.org/10.2172/1808567, 2001. a
Coakley, J. A., Friedman, M. A., and Tahnk, W. R.: Retrieval of Cloud Properties for Partly Cloudy Imager Pixels, J. Atmos. Ocean. Tech., 22, 3–17, https://doi.org/10.1175/JTECH-1681.1, 2005. a
Comstock, K. K., Bretherton, C. S., and Yuter, S. E.: Mesoscale variability and drizzle in southeast Pacific stratocumulus, J. Atmos. Sci., 62, 3792–3807, https://doi.org/10.1175/JAS3567.1, 2005. a
Costantino, L. and Breon, F. M.: Analysis of aerosol-cloud interaction from multi-sensor satellite observations, Geophys. Res. Lett., 37, L11801, https://doi.org/10.1029/2009gl041828, 2010. a
Dennis, J. M., Edwards, J., Evans, K. J., Guba, O., Lauritzen, P. H., Mirin, A. A., St-Cyr, A., Taylor, M. A., and Worley, P. H.: CAM-SE: A scalable spectral element dynamical core for the Community Atmosphere Model, The Int. J. High Perform. Comput. Appl., 26, 74–89, https://doi.org/10.1177/1094342011428142, 2012. a
Diamond, M. S., Director, H. M., Eastman, R., Possner, A., and Wood, R.: Substantial Cloud Brightening From Shipping in Subtropical Low Clouds, AGU Advances, 1, e2019AV000111, https://doi.org/10.1029/2019AV000111, 2020. a, b
Doelling, D. R., Sun, M., Nguyen, L. T., Nordeen, M. L., Haney, C. O., Keyes, D. F., and Mlynczak, P. E.: Advances in Geostationary-Derived Longwave Fluxes for the CERES Synoptic (SYN1deg) Product, J. Atmos. Ocean. Technol., 33, 503–521, https://doi.org/10.1175/JTECH-D-15-0147.1, 2016. a
Dong, X., Schwantes, A. C., Xi, B., and Wu, P.: Investigation of the marine boundary layer cloud and CCN properties under coupled and decoupled conditions over the Azores, J. Geophys. Res.-Atmos., 120, 6179–6191, https://doi.org/10.1002/2014JD022939, 2015. a, b
Eastman, R., Wood, R., and Bretherton, C. S.: Time Scales of Clouds and Cloud-Controlling Variables in Subtropical Stratocumulus from a Lagrangian Perspective, J. Atmos. Sci., 73, 3079–3091, https://doi.org/10.1175/JAS-D-16-0050.1, 2016. a
Eastman, R., Lebsock, M., and Wood, R.: Warm Rain Rates from AMSR-E 89-GHz Brightness Temperatures Trained Using CloudSat Rain-Rate Observations, J. Atmos. Ocean. Tech., 36, 1033–1051, https://doi.org/10.1175/JTECH-D-18-0185.1, 2019. a
Ebmeier, S. K., Sayer, A. M., Grainger, R. G., Mather, T. A., and Carboni, E.: Systematic satellite observations of the impact of aerosols from passive volcanic degassing on local cloud properties, Atmos. Chem. Phys., 14, 10601–10618, https://doi.org/10.5194/acp-14-10601-2014, 2014. a, b
Fu, Q. and Liou, K. N.: On the Correlated k-Distribution Method for Radiative Transfer in Nonhomogenous Atmospheres, J. Atmos. Sci., 49, 2139–2156, 1992. a
Gelaro, R., McCarty, W., Suárez, M. J., Todling, R., Molod, A., Takacs, L., Randles, C. A., Darmenov, A., Bosilovich, M. G., Reichle, R., Wargan, K., Coy, L., Cullather, R., Draper, C., Akella, S., Buchard, V., Conaty, A., da Silva, A. M., Gu, W., Kim, G.-K., Koster, R., Lucchesi, R., Merkova, D., Nielsen, J. E., Partyka, G., Pawson, S., Putman, W., Rienecker, M., Schubert, S. D., Sienkiewicz, M., and Zhao, B.: The Modern-Era Retrospective Analysis for Research and Applications, Version 2 (MERRA-2), J. Climate, 30, 5419–5454, https://doi.org/10.1175/JCLI-D-16-0758.1, 2017. a
Gettelman, A. and Morrison, H.: Advanced Two-Moment Bulk Microphysics for Global Models. Part I: Off-Line Tests and Comparison with Other Schemes, J. Climate, 28, 1268–1287, https://doi.org/10.1175/JCLI-D-14-00102.1, 2015. a
Gettelman, A., Gagne, D. J., Chen, C.-C., Christensen, M. W., Lebo, Z. J., Morrison, H., and Gantos, G.: Machine Learning the Warm Rain Process, J. Adv. Model. Earth Syst., 13, e2020MS002268, https://doi.org/10.1029/2020MS002268, 2021. a
Ghan, S., Wang, M., Zhang, S., Ferrachat, S., Gettelman, A., Griesfeller, J., Kipling, Z., Lohmann, U., Morrison, H., Neubauer, D., Partridge, D. G., Stier, P., Takemura, T., Wang, H., and Zhang, K.: Challenges in constraining anthropogenic aerosol effects on cloud radiative forcing using present-day spatiotemporal variability, P. Natl. Acad. Sci. USA, 113, 5804–5811, https://doi.org/10.1073/pnas.1514036113, 2016. a
Ghan, S. J.: Technical Note: Estimating aerosol effects on cloud radiative forcing, Atmos. Chem. Phys., 13, 9971–9974, https://doi.org/10.5194/acp-13-9971-2013, 2013. a, b, c, d
Glassmeier, F., Hoffmann, F., Johnson, J. S., Yamaguchi, T., Carslaw, K. S., and Feingold, G.: An emulator approach to stratocumulus susceptibility, Atmos. Chem. Phys., 19, 10191–10203, https://doi.org/10.5194/acp-19-10191-2019, 2019. a
Golaz, J.-C., Larson, V. E., and Cotton, W. R.: A PDF-Based Model for Boundary Layer Clouds. Part I: Method and Model Description, J. Atmos. Sci., 59, 3540–3551, https://doi.org/10.1175/1520-0469(2002)059<3540:APBMFB>2.0.CO;2, 2002. a
Golaz, J.-C., Horowitz, L. W., and Levy, H.: Cloud tuning in a coupled climate model: Impact on 20th century warming, Geophys. Res. Lett., 40, 2246–2251, https://doi.org/10.1002/grl.50232, 2013. a, b
Golaz, J. C., Caldwell, P. M., Van Roekel, L. P., Petersen, M. R., Tang, Q., Wolfe, J. D., Abeshu, G., Anantharaj, V., Asay-Davis, X. S., Bader, D. C., Baldwin, S. A., Bisht, G., Bogenschutz, P. A., Branstetter, M., Brunke, M. A., Brus, S. R., Burrows, S. M., Cameron-Smith, P. J., Donahue, A. S., Deakin, M., Easter, R. C., Evans, K. J., Feng, Y., Flanner, M., Foucar, J. G., Fyke, J. G., Griffin, B. M., Hannay, C., Harrop, B. E., Hoffman, M. J., Hunke, E. C., Jacob, R. L., Jacobsen, D. W., Jeffery, N., Jones, P. W., Keen, N. D., Klein, S. A., Larson, V. E., Leung, L. R., Li, H. Y., Lin, W. Y., Lipscomb, W. H., Ma, P. L., Mahajan, S., Maltrud, M. E., Mametjanov, A., McClean, J. L., McCoy, R. B., Neale, R. B., Price, S. F., Qian, Y., Rasch, P. J., Eyre, J. E. J. R., Riley, W. J., Ringler, T. D., Roberts, A. F., Roesler, E. L., Salinger, A. G., Shaheen, Z., Shi, X. Y., Singh, B., Tang, J. Y., Taylor, M. A., Thornton, P. E., Turner, A. K., Veneziani, M., Wan, H., Wang, H. L., Wang, S. L., Williams, D. N., Wolfram, P. J., Worley, P. H., Xie, S. C., Yang, Y., Yoon, J. H., Zelinka, M. D., Zender, C. S., Zeng, X. B., Zhang, C. Z., Zhang, K., Zhang, Y., Zheng, X., Zhou, T., and Zhu, Q.: The DOE E3SM Coupled Model Version 1: Overview and Evaluation at Standard Resolution, J. Adv. Model. Earth Sy., 11, 2089–2129, https://doi.org/10.1029/2018ms001603, 2019. a, b
Golaz, J.-C., Van Roekel, L. P., Zheng, X. et al.: The DOE E3SM Model Version 2: Overview of the physical model, Earth and Space Science Open Archive, p. 61, https://doi.org/10.1002/essoar.10511174.1, 2022. a
Goren, T. and Rosenfeld, D.: Extensive Closed Cell Marine Stratocumulus Downwind of Europe – A Large Aerosol Cloud Mediated Radiative Effect or Forcing?, J. Geophys. Res-.Atmos., 120, 6098–6116, https://doi.org/10.1002/2015JD023176, 2015. a
Goren, T., Kazil, J., Hoffmann, F., Yamaguchi, T., and Feingold, G.: Anthropogenic Air Pollution Delays Marine Stratocumulus Break-up to Open-Cells, Geophys. Res. Lett., 46, 14,135–14,144, https://doi.org/10.1029/2019gl085412, 2019. a
Grosvenor, D. P., Sourdeval, O., Zuidema, P., Ackerman, A., Alexandrov, M. D., Bennartz, R., Boers, R., Cairns, B., Chiu, J. C., Christensen, M., Deneke, H., Diamond, M., Feingold, G., Fridlind, A., Hunerbein, A., Knist, C., Kollias, P., Marshak, A., McCoy, D., Merk, D., Painemal, D., Rausch, J., Rosenfeld, D., Russchenberg, H., Seifert, P., Sinclair, K., Stier, P., van Diedenhoven, B., Wendisch, M., Werner, F., Wood, R., Zhang, Z., and Quaas, J.: Remote Sensing of Droplet Number Concentration in Warm Clouds: A Review of the Current State of Knowledge and Perspectives, Rev. Geophys., 56, 409–453, https://doi.org/10.1029/2017RG000593, 2018. a, b, c
Gryspeerdt, E., Quaas, J., and Bellouin, N.: Constraining the aerosol influence on cloud fraction, J. Geophys. Res.-Atmos., 121, 3566–3583, https://doi.org/10.1002/2015JD023744, 2016. a
Gryspeerdt, E., Goren, T., Sourdeval, O., Quaas, J., Mülmenstädt, J., Dipu, S., Unglaub, C., Gettelman, A., and Christensen, M.: Constraining the aerosol influence on cloud liquid water path, Atmos. Chem. Phys., 19, 5331–5347, https://doi.org/10.5194/acp-19-5331-2019, 2019. a
Gryspeerdt, E., Mülmenstädt, J., Gettelman, A., Malavelle, F. F., Morrison, H., Neubauer, D., Partridge, D. G., Stier, P., Takemura, T., Wang, H., Wang, M., and Zhang, K.: Surprising similarities in model and observational aerosol radiative forcing estimates, Atmos. Chem. Phys., 20, 613–623, https://doi.org/10.5194/acp-20-613-2020, 2020. a
Haarsma, R. J., Roberts, M. J., Vidale, P. L., Senior, C. A., Bellucci, A., Bao, Q., Chang, P., Corti, S., Fučkar, N. S., Guemas, V., von Hardenberg, J., Hazeleger, W., Kodama, C., Koenigk, T., Leung, L. R., Lu, J., Luo, J.-J., Mao, J., Mizielinski, M. S., Mizuta, R., Nobre, P., Satoh, M., Scoccimarro, E., Semmler, T., Small, J., and von Storch, J.-S.: High Resolution Model Intercomparison Project (HighResMIP v1.0) for CMIP6, Geosci. Model Dev., 9, 4185–4208, https://doi.org/10.5194/gmd-9-4185-2016, 2016. a
Hardin, J., Giangrande, S. E., and Zhou, A.: Laser Disdrometer Quantities (LDQUANTS) and Video Disdrometer Quantities (VDISQUANTS) Value-Added Products Report, OSTI.GOV, https://doi.org/10.2172/1808573, 2020. a
Hinkelman, L. M. and Marchand, R.: Evaluation of CERES and CloudSat Surface Radiative Fluxes Over Macquarie Island, the Southern Ocean, Earth Space Sci., 7, e2020EA001224, https://doi.org/10.1029/2020EA001224, 2020. a
Huffman, G., Bolvin, D., Braithwaite, D., Hsu, K., Joyce, R., and Xie, P.: Integrated Multi-satellitE Retrievals for GPM (IMERG), version 4.4. NASA's Precipitation Processing Center, NA, ftp://arthurhou.pps.eosdis.nasa.gov/gpmdata/, last access: 31 March, 2015. a
IPCC: Summary for policymakers, in Climate Change 2013: The Physical Science Basis, Contribution of Working Group I to the Fifth Assessment Report of the Intergovernmental Panel on Climate Change, edited by: Stocker, T. F., Qin, D., and Plattner, G., Cambridge University Press, Cambridge, United Kingdom and New York, NY, USA, Chapter 7, 2013. a
Jensen, M. P., Ghate, V. P., Wang, D., Apoznanski, D. K., Bartholomew, M. J., Giangrande, S. E., Johnson, K. L., and Thieman, M. M.: Contrasting characteristics of open- and closed-cellular stratocumulus cloud in the eastern North Atlantic, Atmos. Chem. Phys., 21, 14557–14571, https://doi.org/10.5194/acp-21-14557-2021, 2021. a
Johnson, D. W., Osborne, S., Wood, R., Suhre, K., Johnson, R., Businger, S., Quinn, P. K., Wiedensohler, A., Durkee, P. A., Russell, L. M., Andreae, M. O., O’Dowd, C., Noone, K. J., Bandy, B., Rudolph, J., and Rapsomanikis, S.: An overview of the Lagrangian experiments undertaken during the North Atlantic regional Aerosol Characterisation Experiment (ACE-2), Tellus B, 52, 290–320, https://doi.org/10.3402/tellusb.v52i2.16110, 2000. a
Karset, I. H. H., Gettelman, A., Storelvmo, T., Alterskjær, K., and Berntsen, T. K.: Exploring Impacts of Size-Dependent Evaporation and Entrainment in a Global Model, J. Geophys. Res.-Atmos., 125, e2019JD031817, https://doi.org/10.1029/2019JD031817, 2020. a
Kazil, J., Christensen, M. W., Abel, S. J., Yamaguchi, T., and Feingold, G.: Realism of Lagrangian Large Eddy Simulations Driven by Reanalysis Meteorology: Tracking a Pocket of Open Cells Under a Biomass Burning Aerosol Layer, J. Adv. Model. Earth Syst., 13, e2021MS002664, https://doi.org/10.1029/2021MS002664, 2021. a
Khairoutdinov, M. and Kogan, Y.: A New Cloud Physics Parameterization in a Large-Eddy Simulation Model of Marine Stratocumulus, Mon. Weather Rev., 128, 229–243, https://doi.org/10.1175/1520-0493(2000)128<0229:ANCPPI>2.0.CO;2, 2000. a
Kim, B.-G., Schwartz, S. E., Miller, M. A., and Min, Q.: Effective radius of cloud droplets by ground-based remote sensing: Relationship to aerosol, J. Geophys. Res.-Atmos., 108, D23, https://doi.org/10.1029/2003JD003721, 2003. a
Klein, S. A. and Hartmann, D. L.: The seasonal cycle of low stratiform clouds, Climate, 5, 1281–1304, 1993. a
Kogan, Y.: A Cumulus Cloud Microphysics Parameterization for Cloud-Resolving Models, J. Atmos. Sci., 70, 1423–1436, https://doi.org/10.1175/JAS-D-12-0183.1, 2013. a
Kollias, P., Clothiaux, E. E., Ackerman, T. P., Albrecht, B. A., Widener, K. B., Moran, K. P., Luke, E. P., Johnson, K. L., Bharadwaj, N., Mead, J. B., Miller, M. A., Verlinde, J., Marchand, R. T., and Mace, G. G.: Development and Applications of ARM Millimeter-Wavelength Cloud Radars, Meteor. Mon., 57, 17.1–17.19, https://doi.org/10.1175/AMSMONOGRAPHS-D-15-0037.1, 2016. a
Koontz, A., Flynn, C., Hodges, G., Michalsky, J., and Barnard, J.: Atmospheric Radiation Measurement (ARM) user facility, 2013: Aerosol Optical Depth Value-Added Product (MFRSRAOD1MICH): An ARM Value-Added Product. 2016-01-01 to 2020-01-25, Eastern North Atlantic (ENA) Graciosa Island, Azores, Portugal [data set] (C1), https://www.arm.gov/capabilities/vaps/aod-mfrsr (last access: 19 January 2022), 2013. a
Larson, V. E. and Golaz, J.-C.: Using Probability Density Functions to Derive Consistent Closure Relationships among Higher-Order Moments, Mon. Weather Rev., 133, 1023–1042, https://doi.org/10.1175/MWR2902.1, 2005. a
Larson, V. E., Golaz, J.-C., and Cotton, W. R.: Small-Scale and Mesoscale Variability in Cloudy Boundary Layers: Joint Probability Density Functions, J. Atmos. Sci., 59, 3519–3539, https://doi.org/10.1175/1520-0469(2002)059<3519:SSAMVI>2.0.CO;2, 2002. a
Lebsock, M. D., Stephens, G. L., and Kummerow, C.: Multisensor satellite observations of aerosol effects on warm clouds, J. Geophys. Res.-Atmos., 113, D15, https://doi.org/10.1029/2008JD009876, 2008. a
Liu, J. and Li, Z.: Estimation of cloud condensation nuclei concentration from aerosol optical quantities: influential factors and uncertainties, Atmos. Chem. Phys., 14, 471–483, https://doi.org/10.5194/acp-14-471-2014, 2014. a
Liu, X., Easter, R. C., Ghan, S. J., Zaveri, R., Rasch, P., Shi, X., Lamarque, J.-F., Gettelman, A., Morrison, H., Vitt, F., Conley, A., Park, S., Neale, R., Hannay, C., Ekman, A. M. L., Hess, P., Mahowald, N., Collins, W., Iacono, M. J., Bretherton, C. S., Flanner, M. G., and Mitchell, D.: Toward a minimal representation of aerosols in climate models: description and evaluation in the Community Atmosphere Model CAM5, Geosci. Model Dev., 5, 709–739, https://doi.org/10.5194/gmd-5-709-2012, 2012. a
Liu, X., Ma, P.-L., Wang, H., Tilmes, S., Singh, B., Easter, R. C., Ghan, S. J., and Rasch, P. J.: Description and evaluation of a new four-mode version of the Modal Aerosol Module (MAM4) within version 5.3 of the Community Atmosphere Model, Geosci. Model Dev., 9, 505–522, https://doi.org/10.5194/gmd-9-505-2016, 2016. a
Lohmann, U.: A glaciation indirect aerosol effect caused by soot aerosols, Geophys. Res. Lett., 29, 11-1–11-4, https://doi.org/10.1029/2001GL014357, 2002. a
Ma, P.-L., Harrop, B. E., Larson, V. E., Neale, R. B., Gettelman, A., Morrison, H., Wang, H., Zhang, K., Klein, S. A., Zelinka, M. D., Zhang, Y., Qian, Y., Yoon, J.-H., Jones, C. R., Huang, M., Tai, S.-L., Singh, B., Bogenschutz, P. A., Zheng, X., Lin, W., Quaas, J., Chepfer, H., Brunke, M. A., Zeng, X., Mülmenstädt, J., Hagos, S., Zhang, Z., Song, H., Liu, X., Pritchard, M. S., Wan, H., Wang, J., Tang, Q., Caldwell, P. M., Fan, J., Berg, L. K., Fast, J. D., Taylor, M. A., Golaz, J.-C., Xie, S., Rasch, P. J., and Leung, L. R.: Better calibration of cloud parameterizations and subgrid effects increases the fidelity of the E3SM Atmosphere Model version 1, Geosci. Model Dev., 15, 2881–2916, https://doi.org/10.5194/gmd-15-2881-2022, 2022. a
Merk, D., Deneke, H., Pospichal, B., and Seifert, P.: Investigation of the adiabatic assumption for estimating cloud micro- and macrophysical properties from satellite and ground observations, Atmos. Chem. Phys., 16, 933–952, https://doi.org/10.5194/acp-16-933-2016, 2016. a
Min, Q. and Harrison, L. C.: Cloud properties derived from surface MFRSR measurements and comparison with GOES results at the ARM SGP Site, Geophys. Res. Lett., 23, 1641–1644, https://doi.org/10.1029/96GL01488, 1996. a
Mohrmann, J., Bretherton, C. S., McCoy, I. L., McGibbon, J., Wood, R., Ghate, V., Albrecht, B., Sarkar, M., Zuidema, P., and Palikonda, R.: Lagrangian Evolution of the Northeast Pacific Marine Boundary Layer Structure and Cloud during CSET, Mon. Weather Rev., 147, 4681–4700, https://doi.org/10.1175/MWR-D-19-0053.1, 2019. a
Mülmenstädt, J., Gryspeerdt, E., Salzmann, M., Ma, P.-L., Dipu, S., and Quaas, J.: Separating radiative forcing by aerosol–cloud interactions and rapid cloud adjustments in the ECHAM–HAMMOZ aerosol–climate model using the method of partial radiative perturbations, Atmos. Chem. Phys., 19, 15415–15429, https://doi.org/10.5194/acp-19-15415-2019, 2019. a
Mülmenstädt, J., Nam, C., Salzmann, M., Kretzschmar, J., L'Ecuyer, T. S., Lohmann, U., Ma, P.-L., Myhre, G., Neubauer, D., Stier, P., Suzuki, K., Wang, M., and Quaas, J.: Reducing the aerosol forcing uncertainty using observational constraints on warm rain processes, Sci. Adv., 6, eaaz6433, https://doi.org/10.1126/sciadv.aaz6433, 2020. a
Nakajima, T. and King, M. D.: Determination of the Optical Thickness and Effective Particle Radius of Clouds from Reflected Solar Radiation Measurements. Part I: Theory, J. Atmos. Sci., 47, 1878–1893, https://doi.org/10.1175/1520-0469(1990)047<1878:DOTOTA>2.0.CO;2, 1989. a
NASA: goldsmr4.gesdisc.eosdis.nasa.gov, GES DISC [data set], https://goldsmr4.gesdisc.eosdis.nasa.gov/data/MERRA2/, last access: 23 February 2023.
Neale, R. B., Richter, J. H., and Jochum, M.: The Impact of Convection on ENSO: From a Delayed Oscillator to a Series of Events, J. Climate, 21, 5904–5924, https://doi.org/10.1175/2008JCLI2244.1, 2008. a
Neubauer, D., Christensen, M. W., Poulsen, C. A., and Lohmann, U.: Unveiling aerosol–cloud interactions – Part 2: Minimising the effects of aerosol swelling and wet scavenging in ECHAM6-HAM2 for comparison to satellite data, Atmos. Chem. Phys., 17, 13165–13185, https://doi.org/10.5194/acp-17-13165-2017, 2017. a
NOAA: Hysplit, NOAA [data set], https://www.ready.noaa.gov/HYSPLIT.php, last access: 23 February 2023.
O'Connor, E. J., Illingworth, A. J., and Hogan, R. J.: A Technique for Autocalibration of Cloud Lidar, J. Atmos. Ocean. Technol., 21, 777–786, https://doi.org/10.1175/1520-0426(2004)021<0777:ATFAOC>2.0.CO;2, 2004. a
Pincus, R., Baker, M. B., and Bretherton, C. S.: What Controls Stratocumulus Radiative Properties? Lagrangian Observations of Cloud Evolution, J. Atmos. Sci., 54, 2215–2236, https://doi.org/10.1175/1520-0469(1997)054<2215:WCSRPL>2.0.CO;2, 1997. a
Pincus, R., Platnick, S., Ackerman, S. A., Hemler, R. S., and Hofmann, R. J. P.: Reconciling Simulated and Observed Views of Clouds: MODIS, ISCCP, and the Limits of Instrument Simulators, J. Climate, 25, 4699–4720, https://doi.org/10.1175/JCLI-D-11-00267.1, 2012. a
Quaas, J., Boucher, O., Bellouin, N., and Kinne, S.: Satellite-Based Estimate of the Direct and Indirect Aerosol Climate Forcing, J. Geophys. Res.-Atmos., 113, D05204, https://doi.org/10.1029/2007jd008962, 2008. a, b, c
Quaas, J., Arola, A., Cairns, B., Christensen, M., Deneke, H., Ekman, A. M. L., Feingold, G., Fridlind, A., Gryspeerdt, E., Hasekamp, O., Li, Z., Lipponen, A., Ma, P.-L., Mülmenstädt, J., Nenes, A., Penner, J. E., Rosenfeld, D., Schrödner, R., Sinclair, K., Sourdeval, O., Stier, P., Tesche, M., van Diedenhoven, B., and Wendisch, M.: Constraining the Twomey effect from satellite observations: issues and perspectives, Atmos. Chem. Phys., 20, 15079–15099, https://doi.org/10.5194/acp-20-15079-2020, 2020. a
Rahu, J., Trofimov, H., Post, P., and Toll, V.: Diurnal Evolution of Cloud Water Responses to Aerosols, J. Geophys. Re.-Atmos., 127, e2021JD035091, https://doi.org/10.1029/2021JD035091, 2022. a
Randles, C. A., da Silva, A. M., Buchard, V., Colarco, P. R., Darmenov, A., Govindaraju, R., Smirnov, A., Holben, B., Ferrare, R., Hair, J., Shinozuka, Y., and Flynn, C. J.: The MERRA-2 Aerosol Reanalysis, 1980 Onward. Part I: System Description and Data Assimilation Evaluation, J. Climate, 30, 6823–6850, https://doi.org/10.1175/JCLI-D-16-0609.1, 2017. a
Rasch, P. J., Xie, S., Ma, P.-L., Lin, W., Wang, H., Tang, Q., Burrows, S. M., Caldwell, P., Zhang, K., Easter, R. C., Cameron-Smith, P., Singh, B., Wan, H., Golaz, J.-C., Harrop, B. E., Roesler, E., Bacmeister, J., Larson, V. E., Evans, K. J., Qian, Y., Taylor, M., Leung, L. R., Zhang, Y., Brent, L., Branstetter, M., Hannay, C., Mahajan, S., Mametjanov, A., Neale, R., Richter, J. H., Yoon, J.-H., Zender, C. S., Bader, D., Flanner, M., Foucar, J. G., Jacob, R., Keen, N., Klein, S. A., Liu, X., Salinger, A., Shrivastava, M., and Yang, Y.: An Overview of the Atmospheric Component of the Energy Exascale Earth System Model, J. Adv. Model. Earth Syst., 11, 2377–2411, https://doi.org/10.1029/2019MS001629, 2019. a, b
Richter, J. H. and Rasch, P. J.: Effects of Convective Momentum Transport on the Atmospheric Circulation in the Community Atmosphere Model, Version 3, J. Climate, 21, 1487–1499, https://doi.org/10.1175/2007JCLI1789.1, 2008. a
Roberts, G. C. and Nenes, A.: A continuous-flow streamwise thermal-gradient CCN chamber for atmospheric measurements, Aerosol Sci. Tech., 39, 206–221, https://doi.org/10.1080/027868290913988, 2005. a
Rémillard, J. and Tselioudis, G.: Cloud Regime Variability over the Azores and Its Application to Climate Model Evaluation, J. Climate, 28, 9707–9720, https://doi.org/10.1175/JCLI-D-15-0066.1, 2015. a
Rémillard, J., Kollias, P., Luke, E., and Wood, R.: Marine Boundary Layer Cloud Observations in the Azores, J. Climate, 25, 7381–7398, https://doi.org/10.1175/JCLI-D-11-00610.1, 2012. a, b
Savic-Jovcic, V. and Stevens, B.: The Structure and Mesoscale Organization of Precipitating Stratocumulus, J. Atmos. Sci., 65, 1587–1605, https://doi.org/10.1175/2007JAS2456.1, 2008. a
Schutgens, N. A. J., Gryspeerdt, E., Weigum, N., Tsyro, S., Goto, D., Schulz, M., and Stier, P.: Will a perfect model agree with perfect observations? The impact of spatial sampling, Atmos. Chem. Phys., 16, 6335–6353, https://doi.org/10.5194/acp-16-6335-2016, 2016. a
Skofronick-Jackson, G., Kirschbaum, D., Petersen, W., Huffman, G., Kidd, C., Stocker, E., and Kakar, R.: The Global Precipitation Measurement (GPM) mission's scientific achievements and societal contributions: reviewing four years of advanced rain and snow observations, Q. J. Roy. Meteorol. Soc., 144, 27–48, https://doi.org/10.1002/qj.3313, 2018. a
Stein, A. F., Draxler, R. R., Rolph, G. D., Stunder, B. J. B., Cohen, M. D., and Ngan, F.: NOAA’s HYSPLIT Atmospheric Transport and Dispersion Modeling System, B. Am. Meteorol. Soc., 96, 2059–2077, https://doi.org/10.1175/BAMS-D-14-00110.1, 2015. a
Stephens, G. L.: Radiation profiles in extended water clouds. II: Parameterization schemes, J. Atmos. Sci., 35, 2123–2132, 1978. a
Stephens, G. L., Gabriel, P. M., and Tsay, S. C.: Statistical radiative transport in one-dimensional media and its application to the terrestrial atmosphere, Transp. Theory Stat. Phys., 20, 139–175, https://doi.org/10.1080/00411459108203900, 1991. a
Swales, D. J., Pincus, R., and Bodas-Salcedo, A.: The Cloud Feedback Model Intercomparison Project Observational Simulator Package: Version 2, Geosci. Model Dev., 11, 77–81, https://doi.org/10.5194/gmd-11-77-2018, 2018. a
Tang, Q., Klein, S. A., Xie, S., Lin, W., Golaz, J.-C., Roesler, E. L., Taylor, M. A., Rasch, P. J., Bader, D. C., Berg, L. K., Caldwell, P., Giangrande, S. E., Neale, R. B., Qian, Y., Riihimaki, L. D., Zender, C. S., Zhang, Y., and Zheng, X.: Regionally refined test bed in E3SM atmosphere model version 1 (EAMv1) and applications for high-resolution modeling, Geosci. Model Dev., 12, 2679–2706, https://doi.org/10.5194/gmd-12-2679-2019, 2019. a
Tang, S. and Xie, S.: Atmospheric Radiation Measurement (ARM) user facility, 2020: ARM Best Estimate Cloud Radiation (ARMBECLDRAD). 2014-01-01 to 2020-12-31, Eastern North Atlantic (ENA) Graciosa Island, Azores, Portugal (C1), https://doi.org/10.5439/1333228, 2020. a
Taylor, M. A. and Fournier, A.: A compatible and conservative spectral element method on unstructured grids, J. Comput. Phys., 229, 5879–5895, https://doi.org/10.1016/j.jcp.2010.04.008, 2010. a
Tokay, A., Wolff, D. B., and Petersen, W. A.: Evaluation of the New Version of the Laser-Optical Disdrometer, OTT Parsivel2, J. Atmos. Ocean. Technol., 31, 1276–1288, https://doi.org/10.1175/JTECH-D-13-00174.1, 2014. a
Toll, V., Christensen, M., Quaas, J., and Bellouin, N.: Weak average liquid-cloud-water response to anthropogenic aerosols, Nature, 572, 51–55, https://doi.org/10.1038/s41586-019-1423-9, 2019. a
Turner, D., Q, C. L., Zhang, M. D., and Gaustad, K.: Atmospheric Radiation Measurement (ARM) user facility, 2021: Cloud Optical Properties from the Multifilter Shadowband Radiometer (MFRSRCLDOD): An ARM Value-Added Product. 2014-06-01 to 2019-10-27, Eastern North Atlantic (ENA) Graciosa Island, Azores, Portugal (C1), https://www.arm.gov/capabilities/vaps/mfrsrcldod (last access: 19 January 2022), 2021. a
Twomey, S.: Pollution and the Planetary Albedo, Atmos. Environ., 8, 1251–1256, 1974. a
Uin, J., Senum, G., Salwen, C., and Koontz, A.: Atmospheric Radiation Measurement (ARM) user facility, 2016: Cloud Condensation Nuclei Particle Counter (AOSCCN1COLSPECTRA). 2016-06-22 to 2020-10-28, Eastern North Atlantic (ENA) Graciosa Island, Azores, Portugal (C1), https://doi.org/10.5439/1342134, 2016. a
Wang, H., Easter, R. C., Rasch, P. J., Wang, M., Liu, X., Ghan, S. J., Qian, Y., Yoon, J.-H., Ma, P.-L., and Vinoj, V.: Sensitivity of remote aerosol distributions to representation of cloud–aerosol interactions in a global climate model, Geosci. Model Dev., 6, 765–782, https://doi.org/10.5194/gmd-6-765-2013, 2013. a
Wang, H., Easter, R. C., Zhang, R., Ma, P.-L., Singh, B., Zhang, K., Ganguly, D., Rasch, P. J., Burrows, S. M., Ghan, S. J., Lou, S., Qian, Y., Yang, Y., Feng, Y., Flanner, M., Leung, L. R., Liu, X., Shrivastava, M., Sun, J., Tang, Q., Xie, S., and Yoon, J.-H.: Aerosols in the E3SM Version 1: New Developments and Their Impacts on Radiative Forcing, J. Adv. Model. Earth Syst., 12, e2019MS001851, https://doi.org/10.1029/2019MS001851, 2020. a, b
Wang, M., Ghan, S., Liu, X., L'Ecuyer, T. S., Zhang, K., Morrison, H., Ovchinnikov, M., Easter, R., Marchand, R., Chand, D., Qian, Y., and Penner, J. E.: Constraining cloud lifetime effects of aerosols using A-Train satellite observations, Geophys. Res. Lett., 39, 20, https://doi.org/10.1029/2012GL052204, 2012. a
Wood, R.: Drizzle in Stratiform Boundary Layer Clouds. Part I: Vertical and Horizontal Structure, J. Atmos. Sci., 62, 3011–3033, https://doi.org/10.1175/JAS3529.1, 2005. a
Wood, R., Wyant, M., Bretherton, C. S., Rémillard, J., Kollias, P., Fletcher, J., Stemmler, J., de Szoeke, S., Yuter, S., Miller, M., Mechem, D., Tselioudis, G., Chiu, J. C., Mann, J. A. L., O’Connor, E. J., Hogan, R. J., Dong, X., Miller, M., Ghate, V., Jefferson, A., Min, Q., Minnis, P., Palikonda, R., Albrecht, B., Luke, E., Hannay, C., and Lin, Y.: CLOUDS, AEROSOLS, AND PRECIPITATION IN THE MARINE BOUNDARY LAYER: An ARM Mobile Facility Deployment, B. Am. Meteorol. Soc., 96, 419–440, http://www.jstor.org/stable/26219584, 2015. a, b, c
Wood, R., Stemmler, J. D., Remillard, J., and Jefferson, A.: Low-CCN concentration air masses over the eastern North Atlantic: Seasonality, meteorology, and drivers, J. Geophys. Res.-Atmos., 122, 1203–1223, https://doi.org/10.1002/2016jd025557, 2017. a, b, c, d, e
Wu, P., Dong, X. Q., Xi, B. K., Tian, J. J., and Ward, D. M.: Profiles of MBL Cloud and Drizzle Microphysical Properties Retrieved From Ground-Based Observations and Validated by Aircraft In Situ Measurements Over the Azores, J. Geophys. Res.-Atmos., 125, e2019JD032205, https://doi.org/10.1029/2019JD032205, 2020. a, b, c
Xie, S., McCoy, R. B., Klein, S. A., Cederwall, R. T., Wiscombe, W. J., Jensen, M. P., Johnson, K. L., Clothiaux, E. E., Gaustad, K. L., Long, C. N., Mather, J. H., McFarlane, S. A., Shi, Y., Golaz, J.-C., Lin, Y., Hall, S. D., McCord, R. A., Palanisamy, G., and Turner, D. D.: CLOUDS AND MORE: ARM Climate Modeling Best Estimate Data: A New Data Product for Climate Studies, B. Am. Meteorol. Soc., 91, 13–20, https://doi.org/10.1175/2009BAMS2891.1, 2010. a, b
Xie, S., Lin, W., Rasch, P. J., Ma, P.-L., Neale, R., Larson, V. E., Qian, Y., Bogenschutz, P. A., Caldwell, P., Cameron-Smith, P., Golaz, J.-C., Mahajan, S., Singh, B., Tang, Q., Wang, H., Yoon, J.-H., Zhang, K., and Zhang, Y.: Understanding Cloud and Convective Characteristics in Version 1 of the E3SM Atmosphere Model, J. Adv. Model. Earth Syst., 10, 2618–2644, https://doi.org/10.1029/2018MS001350, 2018. a
Yamaguchi, T., Feingold, G., and Kazil, J.: Stratocumulus to Cumulus Transition by Drizzle, J. Adv. Model. Earth Syst., 9, 2333–2349, https://doi.org/10.1002/2017MS001104, 2017. a, b, c
Zhang, G. and McFarlane, N. A.: Sensitivity of climate simulations to the parameterization of cumulus convection in the Canadian climate centre general circulation model, Atmos.-Ocean, 33, 407–446, https://doi.org/10.1080/07055900.1995.9649539, 1995. a
Zheng, X., Xi, B., Dong, X., Wu, P., Logan, T., and Wang, Y.: Environmental effects on aerosol–cloud interaction in non-precipitating marine boundary layer (MBL) clouds over the eastern North Atlantic, Atmos. Chem. Phys., 22, 335–354, https://doi.org/10.5194/acp-22-335-2022, 2022. a