the Creative Commons Attribution 4.0 License.
the Creative Commons Attribution 4.0 License.
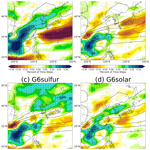
Future changes in atmospheric rivers over East Asia under stratospheric aerosol intervention
Ju Liang
Jim Haywood
Atmospheric rivers (ARs) are closely associated with historical extreme precipitation events over East Asia. The projected increase in such weather systems under global warming has been extensively discussed in previous studies, while the role of stratospheric aerosol, particularly for the implementation of stratospheric aerosol intervention (SAI), in such a change remains unknown. Based on an ensemble of the UK Earth System Model (UKESM1) simulations, here we investigate changes in the frequency of ARs and their associated mean and extreme precipitation under a range of climate forcing, including greenhouse gas emission scenarios of high (SSP5–8.5) and medium (SSP2–4.5) levels, the deployment of SAI geoengineering (G6sulfur), and solar dimming (G6solar). The result indicates a significant increase in AR frequency and AR-related precipitation over most of East Asia in a warmer climate, and the most pronounced changes are observed in southern China. Comparing G6solar and both the Shared Socioeconomic Pathway (SSP) scenarios, the G6sulfur simulations indicate that SAI is effective at partly ameliorating the increases in AR activity over the subtropical region; however, it may result in more pronounced increases in ARs and associated precipitation over the upper-midlatitude regions, particularly northeastern China. Such a response is associated with the further weakening of the subtropical westerly jet stream under SAI that favours the upper-midlatitude AR activity. This is driven by the decreased meridional gradient of thermal expansion in the mid–high troposphere associated with aerosol cooling across the tropical region, though SAI effectively ameliorates the widespread increase in thermal expansion under climate warming. Such a side effect of SAI over the populated region implies that caution must be taken when considering geoengineering approaches to mitigating hydrological risk under climate change.
- Article
(14990 KB) - Full-text XML
-
Supplement
(5762 KB) - BibTeX
- EndNote
East Asia is a populated region exposed to devastating and frequent hydrological extremes due to the influence of precipitating weather systems. One of these systems is that of atmospheric rivers (ARs) that feature elongated and intense moisture transport in the low troposphere. Numerous studies have suggested close linkages between ARs and extreme precipitation events over East Asia (Kamae et al., 2017a, b; Pan and Lu, 2019; Liang et al., 2020, 2022; Fu et al., 2021; Kim et al., 2021; Park et al., 2021; Liang and Yong, 2021, 2022). The AR–precipitation association stems from different dynamical factors. First, the main AR pathway connects the mid–high latitudes of East Asia with the subtropical moisture source regions, including the Arabian Sea, Bay of Bengal, and South China Sea (Pan and Lu, 2019). Second, despite the abundant moisture within the AR plumes, the adjacent environment of ARs, in terms of precipitation efficiency, exhibits a relatively high efficiency for depleting atmospheric moisture to generate precipitation (Liang and Yong, 2021). This is dependent on the active synoptic-scale transient eddies in the downstream area of ARs, which further transport moisture to the mid–high latitudes and trigger precipitation through various physical processes such as the release of low-level potential instability and orographic lifting (Gimeno et al., 2016; Kamae et al., 2021; Park et al., 2021). Given the AR–precipitation linkage, the response of ARs to future climate warming might have implications for the regional climate in East Asia, and this has attracted studies on projecting the possible future changes in ARs over this region. The first attempt to investigate the possible future changes in ARs over East Asia was reported by Espinoza et al. (2018) using an ensemble of coupled global climate models (GCMs), which projected a pronounced increase in the frequency of ARs affecting southern China under the high-level greenhouse gas (GHG) emission scenario of the Representative Concentration Pathway (RCP8.5). Under the same scenario, more recent studies based on a high-resolution GCM projected similar results (Kamae et al., 2021). These similarities suggest that there is growing recognition of the projected increase in AR activity over East Asia under climate warming, and such a change is not simply a function of the choice of methodology, although some studies, especially those based on the Tier 2 experiments of the Atmospheric River Tracking Method Intercomparison Project (ARTMIP; Payne et al., 2020; Collow et al., 2022; O'Brien et al., 2022), have suggested that the projected changes in ARs are susceptible to uncertainties in the algorithms used for detecting ARs, and such uncertainties outweigh those in climate models and reanalysis products.
ARs can cause significant economic losses by triggering hydrological disasters such as flooding (Dominguez et al., 2018; Corringham et al., 2019), landslides (Miller et al., 2018), and frozen precipitation (Guan et al., 2016; Liang and Sushama, 2019). In addition, ARs are associated with the melting of glaciers (Neff, 2018) and the weakening of ice shelf stability (Wille et al., 2022) due to the intense meridional transport of both sensible and latent heat within AR plumes (Shields et al., 2019; Liang and Yong, 2022). Hence, the projected increase in ARs by the current climate models, particularly over the populated regions in East Asia, implies more catastrophic hydrological extremes and irreversible changes to high-latitude ecosystems in future climate that are badly in need of mitigation and adaptation strategies. Despite the demands of enhancing early warning systems and climate adaptation planning to cope with the possible future changes in ARs, a better understanding of the effects of warming mitigation strategies, including the impacts of a reduction in GHG emissions and geoengineering on AR climatology, is required at present.
In the current context of technical capacity constraints and geopolitical factors causing increasing difficulties in achieving the target of the Paris 21st Conference of Parties, i.e. the global mean temperature targets of 1.5 and 2 ∘C above preindustrial levels (e.g. Millar et al., 2017; IPCC, 2018), dramatic increases in the frequency of very extreme precipitation have been observed in the recent period (Myhre et al., 2019). This calls for research into unconventional mitigation strategies (e.g. MacMartin et al., 2018) to mitigate the risks of future extreme precipitation changes. One of the most prominent approaches is the solar radiation management strategy via stratospheric aerosol intervention (SAI), i.e. injection of the precursor of scattering aerosols (sulfur dioxide) into the stratosphere where the atmosphere is relatively stable and has a longer lifetime compared to that in the troposphere so as to achieve cooling of the planet (Lawrence et al., 2018). Among the concerns regarding SAI deployment are impacts on Antarctic ozone recovery (e.g. Tilmes et al., 2008; Heckendorn et al., 2009), the termination effect (e.g. Jones et al., 2013), continued ocean acidification (e.g. Williamson and Turley, 2012), impacts on key modes of climate variability such as the North Atlantic Oscillation and quasi-biennial oscillation (Jones et al., 2022), and moral and ethical issues surrounding any deployment (e.g. Lawrence et al., 2018). However, climate model simulations have indicated that continuous SAI can effectively maintain the global surface temperature under the 2020 conditions (Tilmes et al., 2021) and lead to much more ameliorated hydrological extremes than in unmitigated climate change scenarios (e.g. Jones et al., 2018). To provide physical assessments of the impact of SAI, the Geoengineering Model Intercomparison Project (GeoMIP; Kravitz et al., 2011, 2015; Xia et al., 2017) has performed a set of model experiments to simulate climate scenarios with injections of sulfur into the stratosphere. In East Asia, the G4 experiments (i.e. future climate simulations under the RCP4.5 scenario with constantly injecting sulfur dioxide into the low-level equatorial stratosphere at a rate of 5 Tg yr−1; Kravitz et al., 2011) of GeoMIP have been used to assess the role of SAI in ameliorating the changes in precipitation (Liu et al., 2021). However, the G4 experiments are based on an idealised one-point injection of sulfur dioxide at the Equator with an abrupt beginning and termination that are the only represented potential deployment of geoengineering (Visioni et al., 2021).
As GeoMIP experiments progressed from G4 to G6, a new experiment (G6sulfur; Kravitz et al., 2015) considered the more recently developed GHG emission scenarios from the Shared Socioeconomic Pathways (SSPs; O'Neill et al., 2016) and more plausible SAI deployment, i.e. the injection of sulfur dioxide between 10∘ N and 10∘ S and between 18 and 20 km altitude. Similar SAI simulations have been proposed by the Stratospheric Aerosol Geoengineering Large Ensemble (GLENS; Tilmes et al., 2018) project. GeoMIP G6 also performed experiments of future climate simulations considering the reduction in the total incoming solar irradiance (G6solar; Visioni et al., 2021), and examining differences between G6sulfur and G6solar helps us understand the role of aerosol–climate interaction and, in particular, the impacts that aerosol-induced stratospheric heating may have on atmospheric dynamics under SAI and the uncertainties in its simulation using GCMs. Among others, GeoMIP experiments have investigated changes in precipitating weather systems, including tropical cyclones (Jones et al., 2017; Wang et al., 2018) and extratropical cyclones (Gertler et al., 2020). For ARs, the recent study based on the GLENS experiments found that SAI may lead to decreases in extreme rainfall events from ARs affecting western North America and increases in moderate rainfall events by the end of the century (Shields et al., 2022). However, to date, little research has focused on the impact of SAI on the future changes in weather systems over East Asia and the difference between G6sulfur and G6solar simulations, which can provide more insights into underlying mechanisms of SAI.
This study aims to assess the possible impact of SAI geoengineering on the characteristics of ARs, in terms of the frequency of occurrence and precipitation, in the populated regions of East Asia (e.g. China, Japan, and the Korean Peninsula) by using an ensemble of the G6sulfur and G6solar simulations from a state-of-the-art GCM. We also investigate the effect of SAI on the large-scale circulations related to AR activity to understand the environmental mechanisms driving the changes in ARs over East Asia. This study helps to achieve a better understanding of the AR–aerosol interactions and inform the outcome of SAI in terms of the changes in mean and extreme precipitation from ARs. Following this section, Sect. 2 describes the climate data from the GCM and climate reanalysis datasets and the identification method for detecting AR features in the used data. Section 3 presents the results, and Sect. 4 summarises and discusses the findings.
2.1 The G6 experiments based on UKESM1
In this study, the G6sulfur and G6solar experiments in the sixth phase of GeoMIP are applied for analysing the AR characteristics under SAI. The G6sulfur experiment simulates future climate under the SSP5–8.5 scenario with stratospheric SO2 injection aiming to reduce the global mean air temperature to the level under the SSP2–4.5 scenario (Kravitz et al., 2015; Jones et al., 2021, 2022). The SAI is continuous and applied between 10∘ N and 10∘ S along the Greenwich meridian at 18–20 km altitude. Such a tropical injection strategy has a minor impact on polar ozone (Tilmes et al., 2022), though it can overcool the injection sites relative to high latitudes and suppress global monsoon precipitation in contrast to the polar injection strategy (Sun et al., 2020). The injection rate is adjusted every 10 years to achieve a simulated decadal global mean temperature within ±0.2 K of that under the SSP2–4.5 medium forcing scenario. The G6solar experiment has the same goal but achieves it through the idealised gradual reduction in the solar constant (Kravitz et al., 2015; Visioni et al., 2021). Although such a solar dimming scenario is highly idealised compared to the SAI strategy prescribed by G6sulfur, it provides an important reference of a cooled future climate excluding the SAI-related aerosol–climate interaction; hence, its comparison with G6sulfur helps us understand the effect of SAI.
The G6 experiment outputs are from the UK Earth System Model (UKESM1; Sellar et al., 2019), a contributing model to the current Coupled Model Intercomparison Project Phase 6 (CMIP6; Eyring et al., 2016). The atmospheric component of UKESM1 is at a spatial resolution of and 85 hybrid levels extending up to 85 km. The model applies the Even Newer Dynamics for General atmospheric modelling of the environment (ENDGame) dynamical core, a semi-implicit semi-Lagrangian scheme to solve the non-hydrostatic, fully compressible Navier–Stokes equations (Wood et al., 2014; Walters et al., 2019; Mulcahy et al., 2018). The atmospheric component is coupled to the NEMO (Nucleus for European Modelling of the Ocean) v3.6 ocean model with a spatial resolution of ∼1∘ and 75 levels (Storkey et al., 2018). The land component applies the Joint UK Land Environment Simulator (JULES; Best et al., 2011). The atmospheric chemistry model in UKESM1 applies the United Kingdom Chemistry and Aerosols (UKCA) modelling framework and considers a stratospheric–tropospheric scheme with aerosol chemistry and online photolysis (Morgenstern et al., 2009; Mann et al., 2010; Archibald et al., 2020). The model also simulates the marine carbon cycle using a biogeochemical model described by Yool et al. (2013). For the experiments considered in this study, UKESM1 is run with three ensemble members (identifier index of r1i1p1f2, r4i1p1f2, and r8i1p1f2) corresponding to different realisation set-ups. The atmospheric river detection tool (ARDT) is first applied to simulations by each ensemble member. Then, the future changes in AR characteristics are analysed by calculating the ensemble mean differences between those identified in the future climate simulations (SSP5–8.5, SSP2–4.5, G6sulfur, and G6solar) for the period 2071–2100 and those identified during the baseline period 1981–2010 using the UKESM1 historical simulations for CMIP6. An evaluation of the performance of UKESM1 in simulating the climatology of the detected ARs and associated environmental fields and precipitation is given in the Supplement. Although some biases are noted, UKESM1 displays some consistency in the diagnosed AR properties with those identified in the historical climate reanalysis ERA5 (Hersbach et al., 2020) and observed precipitation (Hamada et al., 2011; Yatagai et al., 2014). For the ability to capture the modulation of ARs by the large-scale circulation, particularly for the East Asian jet stream (EAJS), comparisons of the correlation between AR frequency and the jet intensity are made between ERA5 and UKESM1 in the Supplement. It is found that UKESM1 reasonably captures the negative correlations between AR frequency to the north of 35∘ N and the strength of westerly jet stream in terms of the East Asian jet stream index (EAJSI; Lu et al., 2011) compared to the ERA5 reanalysis dataset (Fig. S4a and b). Hence, the model can provide useful insight into the impact of SAI on ARs and its environmental drivers. Other information on the performance of UKESM1, e.g. biases in simulating the global distributions of temperature, precipitation, and aerosol optical depth, can be found in Sellar et al. (2019). In addition, at present, UKESM1 is the only model that provides outputs of pressure-level winds and specific humidity data every 6 h that satisfy the requirement of the used ARDT; hence, only the UKESM1 simulations are considered in this study, and other GCM ensembles conducted by the G6 experiments of GeoMIP (e.g. Jones et al., 2021, 2022; Visioni et al., 2021) are not used.
2.2 AR identification
The analyses of AR characteristics in gridded climate data rely on a variety of ARDTs. According to the current ARTMIP project (Shields et al., 2018; Rutz et al., 2019; O'Brien et al., 2022), the diversity of ARDTs is a major cause of the uncertainties in the diagnoses of AR metrics; hence, the use of ARDTs should be carefully selected and tuned for the specific research objectives. This study is based on the ARDT named Atmospheric River Identification Algorithm for the Asian monsoon region (ARIA-Asia). A detailed description of the algorithm procedures and their schematic can be found in Liang et al. (2022). In general, this ARDT comprises three steps, i.e. feature isolation, geometric analysis, and computation of diagnostic fields. As shown in Liang and Yong (2022), the first step calculates fields of vertically integrated water vapour transport (IVT) every 6 h and isolates the continuous regions that are likely AR plumes for each time step using fixed or spatially varying IVT thresholds (the latter is used in this study). The second step is to identify the axes of the detected features using the skeletonisation method for a binary image (Wick et al., 2013) and filter out the non-AR features (e.g. tropical cyclones and monsoonal flows) via the geometric criteria, i.e. the length of axis must be longer than 2000 km, and the equivalent width (the area–length ratio) must be less than 750 km. The third step is to calculate the diagnostic fields that quantify AR characteristics, including fields of AR frequency and AR-associated precipitation. This algorithm applies a filter of tropical moisture filaments, as seen in Liang et al. (2022) and Liang and Yong (2022); however, in contrast to these studies using an absolute (fixed) IVT threshold for the isolation of AR plumes, here we apply a relative thresholding method. First, following the methodology of ARDTs used in East Asia (e.g. Pan and Lu, 2019, 2020; Park et al., 2021; Kim et al., 2021), the algorithm uses spatially varying thresholds of IVT in terms of the 85th percentile of IVT averaged over a 5-month moving time window (Park et al., 2021) during the targeted 30-year periods. Second, as per Pan and Lu (2019, 2020), the Gaussian filter with a bandwidth of 6∘ is applied to the IVT threshold fields. The lower limit of the thresholds (i.e. the lowest boundary value of the isolated AR plumes) is determined by the 80th percentile of IVT over the region from 40∘ E to 120∘ W and 20∘ S to 60∘ N. These help to discern the AR plumes from the large-scale background with relatively weak IVT and avoid the influence of high-frequency noise in IVT fields so that more coherent features can be obtained. In addition, the relative thresholding method scales the thresholds, respectively, for the historical and future climate simulations, which helps remove influences of the large-scale thermodynamic factors that are solely governed by the Clausius–Clapeyron relationship so that the dynamic changes in ARs due to different external climate forcings are focused.
3.1 Changes in AR-associated environments
Before analysing the impacts of SAI on the identified AR characteristics, such as the AR frequency and AR-related precipitation, we first assess the changes in AR-associated large-scale environmental fields and discuss their potential influences on ARs. Figure 1 shows the ensemble mean of absolute changes in the low-level, environmental fields averaged over the main AR season (May to September, MJJAS) for the future period (2071–2100) relative to the historical baseline period (1981–2010). The mean 850 hPa geopotential height during the historical baseline period shows the domination of the western Pacific subtropical high (WPSH), an important monsoon system that determines the spatial distribution of ARs (Pan and Lu, 2020; Park et al., 2021). Simulations under the SSP5–8.5 scenario project significant increases in geopotential height to the south of 25∘ N (maximum change centred around 20∘ N, 85∘ E; coloured in Fig. 1a) accompanied with an enhancement of low-level convergence across 30–45∘ N. Such changes are associated with an increase in the meridional land–sea contrast in terms of the surface temperatures (Fig. S5). These drive an intensification of the southwesterly monsoon across about 20∘ N and lead to stronger stationary moisture transport from the moisture source region over the Bay of Bengal, which implies a more favourable environment for the upstream AR activities. Similar patterns are noted under SSP2–4.5 (Fig. 1b), while the magnitude of increases in the 850 hPa geopotential height is reduced by about one-third compared to SSP5–8.5. Both G6sulfur (Fig. 1c) and G6solar (Fig. 1d) show ameliorated changes with respect to SSP5–8.5. Although the SSP2–4.5, G6sulfur, and G6solar experiments present similar magnitudes of changes, there are noticeable differences that are investigated further.
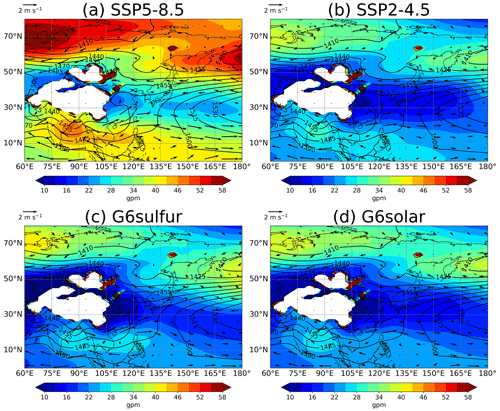
Figure 1Absolute changes in MJJAS mean geopotential height (shaded; unit in geopotential metres or gpm) and wind (vectors) at 850 hPa for the future period of 2071–2100 relative to the historical baseline during 1981–2010. Black contours show the MJJAS mean 850 hPa geopotential height during the historical baseline period. Areas with a surface pressure below the 850 hPa level are shown in white.
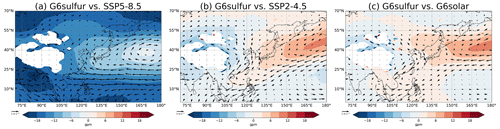
Figure 2Differences in MJJAS mean geopotential height (shaded) and wind (vectors) at 850 hPa for the future period of 2071–2100 between G6sulfur and SSP5–8.5 (a) and SSP2–4.5 (b) and G6solar (c).
Figure 2 shows comparisons of the simulated future low-level circulations between G6sulfur and other experiments. Compared with SSP5–8.5 (Fig. 2a), the simulated SAI by G6sulfur leads to smaller increases in the geopotential height at 850 hPa over most of the study region, despite the North Pacific (near 40∘ N, 165∘ E), where an anticyclonic anomaly (in terms of the 850 hPa wind field) is observed, and this favours the northeastward moisture transport of ARs across 40–55∘ N. Compared to SSP2–4.5, G6sulfur shows negative anomalies of the geopotential height over the continent (Fig. 2b). Such a change corresponds with a more pronounced land–sea thermal contrast, with a significant warming (p value < 0.05) of the surface temperature to the north of 30∘ N and cooling to the south (Fig. 3b). These changes drive an intensification of the southwesterly monsoon flow and the related cyclonic shear across the upstream of the main AR-active region (25–30∘ N, 105–120∘ E). In addition, the low-level anticyclonic response over the North Pacific is corresponding with a significant warming of the sea surface temperature (SST) by up to 1.5 K. These changes may favour the AR-associated blocking at upper midlatitudes (Mullen, 1989; Pohl et al., 2021). Similar patterns of circulation differences are presented when comparing G6sulfur with G6solar (Figs. 2c and 3c). Therefore, although G6sulfur simulates ameliorated changes in the low-level circulation compared to SSP5–8.5, the low-level environments are more favourable for AR activity for G6sulfur compared to SSP2–4.5 and G6solar. Thus, although the simulated SAI strategy is successful at reducing many of the changes apparent under SSP5–8.5, some differences are evident between G6sulfur, G6solar, and SSP2–4.5 (which all have the same global mean temperature), which is due to the existence of the aerosol–climate feedback under SAI.
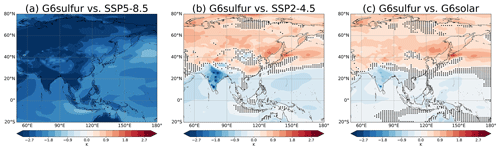
Figure 3As in Fig. 2 but for the MJJAS mean surface temperature. Stippling indicates changes that are statistically insignificant (p value > 0.05 based on Student's t test).
The meridional displacement of ARs is related to various high-tropospheric systems, including the westerly jet streams and the associated upper-level high pressure (Payne and Magnusdottir, 2015; Liang et al., 2022). Following the analyses of Kamae et al. (2017b) and Liang et al. (2022), Fig. 4 assesses changes in the AR-related upper-level environments, including the EAJS, in terms of the 200 hPa wind field and the upper-level thermal expansion quantified by 200–500 hPa geopotential thickness. For the warming scenario under SSP5–8.5 (Fig. 3a), significant increases (p value < 0.05) in the thermal expansion are observed over the study region, with a maximum increase of more than 230 gpm near northeastern China (130∘ E, 50∘ N) and to the south of the WPSH centre (170∘ W, 20∘ N). The increased thermal expansion to the north of 45∘ N leads to a decreased meridional thickness gradient and consequently drives a significant weakening (by up to 5 m s−1) of the EAJS across 30–45∘ N. Similar changes in the thermal expansion (Kamae et al., 2014) and EAJS (Endo et al., 2018) related to the increase in the meridional land–sea thermal contrast have been found under the radiative forcing of the 4×CO2 experiments, while opposite changes have been observed under only the forcing of the SST warming. The projected weakening of the EAJS favours a northward shift in ARs, according to the correlation analyses by Liang et al. (2022) and those discussed later (Fig. S4; discussed in Sect. 3.2). Smaller magnitudes of changes (by about 90 gpm) in the geopotential thickness are shown over most of the region under SSP2–4.5 (Fig. 4b), and this also results in a less apparent weakening of the EAJS. However, G6sulfur projects the most pronounced weakening of the EAJS (by up to 8 m s−1) due to the significant decrease in the thickness gradient between 30–45∘ N, which is associated with the reduced increases in the geopotential thickness to the south of 30∘ N. The further weakening of the EAJS is also related to the presence of the increased maximum to the north (near 130∘ E, 50∘ N), though the magnitude is smaller (by about 70 gpm) than that in SSP5–8.5. In contrast, G6solar (Fig. 4d) projects similar patterns and magnitudes of the changes in the upper-level thermal expansion and winds compared to SSP2–4.5. To further present the effect of SAI on the AR-related upper-level environments, the absolute differences, of 200–500 hPa geopotential thickness and 200 hPa winds, between G6sulfur and other future climate simulations are displayed (Fig. 5). The comparisons confirm that SAI can lead to a further weakening of the EAJS in a warmer climate, even though it effectively ameliorates the increase in the upper-level thermal expansion of the high-emission scenario (shown by the negative red contours in Fig. 5a). Compared with SSP2–4.5 and G6solar, the injected sulfur dioxide simulated by G6sulfur leads to further decreases in the intensity of the EAJS, and these are driven by the reduction in the meridional thickness gradient across 30–45∘ N (Fig. 5b and c).
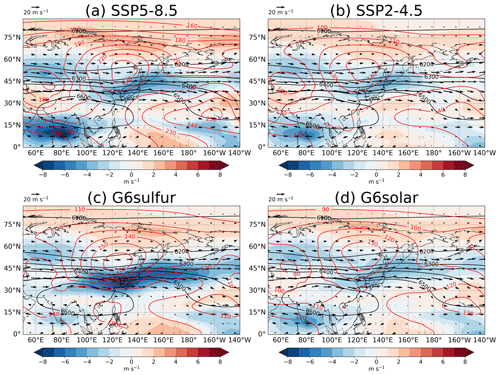
Figure 4Absolute changes in MJJAS mean geopotential thickness between 200 and 500 hPa (red contours; unit is gpm) and 200 hPa wind speeds (shaded) for the future period of 2071–2100 relative to the historical baseline during 1981–2010. Black contours (unit in gpm) show the MJJAS mean 200–500 hPa geopotential thickness, and vectors show the MJJAS mean 200 hPa wind fields during the historical baseline period.
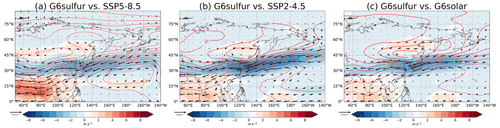
Figure 5Differences in MJJAS mean geopotential thickness between 200 and 500 hPa (red contours) and 200 hPa winds (shaded for velocity and vectors for direction) for the future period of 2071–2100 between G6sulfur and SSP5–8.5 (a) and SSP2–4.5 (b) and G6solar (c).
As the variation in AR frequency over East Asia is closely associated with the North Pacific storm track (Ryu et al., 2021), the absolute differences in the root mean square field of 2–6 d bandpass-filtered mean sea level pressure (MSLP) between G6sulfur and other future climate simulations are analysed as a proxy for eddy kinetic energy and storm tracks (Fig. 6). Such an analysis is based on the Lanczos bandpass filter, following Harvey et al. (2020). For the main AR-active season (MJJAS), all the future climate simulations capture a notable reduction in the midlatitude storm tracks (Fig. S6), with the weakening of the EAJS in a warmer climate, a result consistent with the study of Harvey et al. (2020). Compared with SSP5–8.5, the injected sulfur dioxide simulated by G6sulfur shows no apparent amelioration of the decreases in the storm tracks (Fig. 6a), particularly to the south of Japan. It also presents further decreases across most of the North Pacific compared to SSP2–4.5 (Fig. 6b) and G6solar (Fig. 6c), and these changes can be explained by the weakened baroclinicity under the decreased meridional gradient of surface temperature across 20–50∘ N (Fig. 3). Thus, the changes in storm tracks under the simulated SAI may further inhibit the transient AR features over the downstream region.
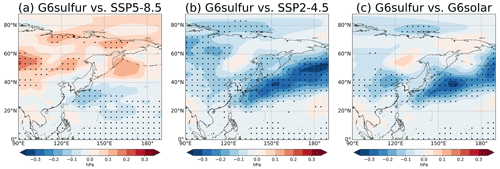
Figure 6As in Fig. 5 but for the root mean square of 2–6 d bandpass-filtered MSLP (proxy for eddy kinetic energy and storm tracks; unit in hPa) during MJJAS. Stippling indicates changes that are statistically significant (p value < 0.05 based on Student's t test).
In summary, the UKESM1 simulations under the SSP5–8.5 high-emission scenario project a strengthening of the upstream monsoon flows and the low-level convergence that are favourable for AR activity in most of East Asia. In addition, a weakening of the EAJS driven by the increased upper-level thermal expansion at high latitudes is displayed. The experiments SSP2–4.5, G6sulfur, and G6solar show ameliorated changes in the low-level circulation associated with ARs; however, compared to SSP2–4.5 and G6solar, the simulated deployment of SAI in G6sulfur exacerbates the weakening of the upper-level westerly jet, which is linked to the cooling effect of the injected aerosol precursor, concentrated across the lower latitudes. This is evident through the comparisons between G6sulfur and G6solar in terms of the global distributions of aerosol optical depth determined at 550 nm and the surface air temperature according to the study of Jones et al. (2021). Moreover, the simulated SAI by G6sulfur shows a limited effect on reducing the general decrease in the storm track across the North Pacific, which creates a less favourable environment for the downstream AR activity.
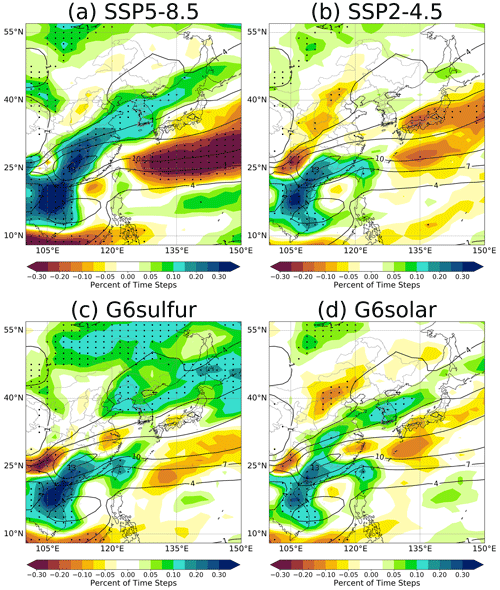
Figure 7Absolute changes (shaded) in annual mean AR frequency (percent of time steps every 6 h) for the future period of 2071–2100 relative to the historical baseline during 1981–2010. Black contours show the annual mean AR frequency during the historical baseline period. Stippling indicates changes that are statistically significant at a confidence level of >95 % (p value < 0.05 based on Student's t test).
3.2 Changes in AR features
Now we analyse the future changes in AR features. Under the SSP5–8.5 scenario (Fig. 7a), the ensemble mean of UKESM1 projects a significant increase (p value < 0.05) in the AR frequency over most of regions from southern China to the Korean Peninsula. The greatest magnitude of increase (by above 0.3 %) is seen in southern China. Significant decreases in frequency are shown over the western Pacific, which is possibly associated with the domination of the stable warm high-pressure within WPSH (Fig. 1a) and the less active storm tracks (Fig. S6a). The significant increase in the AR frequency is consistent with the more favourable dynamical condition in the low troposphere, as displayed in Fig. 1a, including the intensification of the southwesterly monsoon flow and the midlatitude low-level convergence. Under SSP2–4.5, a similar pattern of the frequency change to SSP5–8.5 is projected but with smaller magnitudes (Fig. 7b). The experiments of G6sulfur (Fig. 7c) and G6solar (Fig. 7d) show generally ameliorated changes compared to SSP5–8.5. However, generally, any amelioration of AR frequency is the lowest under G6sulfur, and G6sulfur shows opposite changes (increase by up to 0.2 %) to the north of the main AR-active region, implying a pronounced northward shift in ARs under SAI. This change is linked to the further weakening of the EAJS (Fig. 5c) given the negative correlation between AR frequency and EAJSI to the north of 35∘ N ,as presented by both the ERA5 reanalysis dataset (Fig. S4a) and UKESM1 (Fig. S4b); however, underestimation of such a correlation in UKESM1 is noted to the east of 135∘ E (Fig. S4c), and this bias is partly related to the underestimated AR frequency over the downstream region (Fig. S1c). Similar negative correlations between high-level jets and the upper-midlatitude AR frequency have been found by Zhang and Villarini (2018) and Liang et al. (2022). The mechanism behind the enhanced upper-midlatitude ARs by the weakening of EAJS remains elusive, though one of the possible causes is the anomalous low-level convergence to the northwest of the jet core and divergence to the northeast, according to the four-quadrant strait jet model (Uccellini and Johnson, 1979). This consequently favours the AR-associated northeastward transport of warm moist air across the northern flank of the EAJS. It is also noted that the further decreases in the storm track activity under the simulated SAI do not explain the increases in upper-midlatitude ARs over land, as these systems are mainly controlled by the anticyclonic quasi-stationary circulation (Park et al., 2021).
Figure 8 further illustrates how SAI influences the distribution of the AR frequency in terms of differences between G6sulfur and other experiments. Figure 8a shows that SAI ameliorates some changes to the south of 40∘ N under SSP5–8.5, while it induces significant increases (by up to 0.15 %) in the AR frequency over northeastern China. Compared to SSP2–4.5 and G6solar, G6sulfur demonstrates further frequency increases over most of northeastern China and northern Japan (Fig. 8b and c), which are partly linked to the more pronounced intensification of upstream monsoonal flow and low-level cyclonic shear (Fig. 2b and c).
In summary, the SAI strategy simulated by the G6sulfur experiments can partly mitigate the changes in AR features in East Asia, particularly for the increase in AR frequency over southern China and decreases at the lower latitudes. However, SAI could potentially induce increases in AR activity across northeastern China and Japan as it further weakens the EAJS intensity that is negatively correlated to the upper-midlatitude AR activity. The presented AR–SAI connections could possibly lead to changes in the mean and extreme precipitation of the region, and this will be examined in the following section.
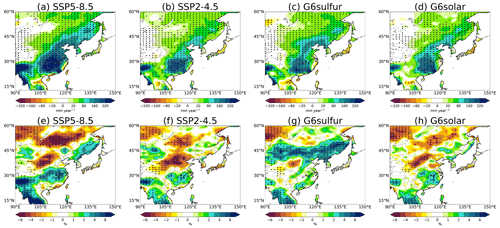
Figure 9Absolute changes in the annual accumulation of AR-related precipitation (a–d) and the fractional contribution of ARs to annual total precipitation amount (e–h) for the future period (2071–2100) relative to the historical baseline (1981–2010). Stippling indicates changes that are statistically significant at a confidence level of >95 % (p value < 0.05 based on Student's t test).
3.3 Changes in AR-associated precipitation
Previous sections have discussed the impact of SAI on the distribution of AR frequency and the associated large-scale environments in East Asia. This section further examines the responses of AR-associated mean and extreme precipitation under the future changes in ARs. Here, precipitation at a given location is considered to be AR-associated if an AR axis is within 350 km. For the SSP5–8.5 scenario (2071–2100) relative to the historical baseline period (1981–2010), the ensemble mean of UKESM1 projects a significant increase (p value < 0.05) in the annual mean accumulation of AR-associated precipitation across the Korean Peninsula and most of China, with magnitudes of up to above 320 mm yr−1 (Fig. 9a). Similar changes with smaller magnitudes are seen under the SSP2–4.5 scenario (Fig. 9b). Resembling the changes in AR frequency, both G6sulfur (Fig. 9c) and G6solar (Fig. 9d) show similar magnitudes of increases across 30∘ N compared to SSP2–4.5. For the changes in the fractional contribution of ARs to annual total precipitation, ARs tend to contribute more precipitation across central and eastern China (by up to 9 %) and North Korea (up to 5 %) under SSP5–8.5 (Fig. 9e). Decreases in the fraction are seen over northeastern China due to the pronounced increases in non-AR precipitation, which is not the focus of this paper. SSP2–4.5 (Fig. 9f), G6sulfur (Fig. 9g), and G6solar (Fig. 9h) project less apparent increases in the fraction to the south of 30∘ N compared to SSP5–8.5. However, significant increases in the fraction by up to 6 % are seen in northeastern China and North Korea under the simulated deployment of SAI in G6sulfur.
For the ensemble mean of the simulated heavy rain events associated with ARs (which is the number of days accumulated when the daily precipitation amount is greater than 40 mm d−1 at a given location and an AR axis is within 350 km), the patterns of changes shown in Fig. 10a–d resemble those for precipitation accumulation (Fig. 9a–d). It is noted that G6sulfur (Fig. 10c) shows significant increases in AR-associated extreme precipitation in Japan with a magnitude similar to that under SSP5–8.5 (Fig. 10a). Also, G6sulfur generally projects a larger magnitude of increases in the fraction of heavy rain events over most of northeastern China (Fig. 10g) compared to other future climate simulations (Fig. 10e, f, and h). These changes imply pronounced side effects of SAI on hydrological extremes from ARs in the above-mentioned regions.
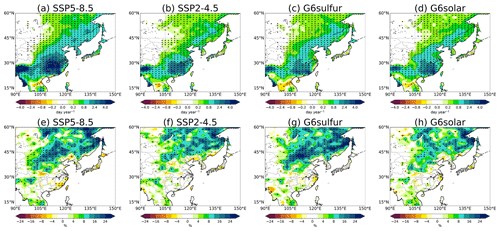
Figure 10As in Fig. 9 but for the occurrence of AR-related events (a–d) of heavy rain (daily precipitation > 40 mm) and the contribution of ARs to the annual total heavy rain events (e–h).
We now compare the ensemble mean of the AR-associated precipitation patterns between G6sulfur and other experiments. As expected, G6sulfur effectively ameliorates the increase in AR precipitation across 30∘ N compared to SSP5–8.5 (Fig. 11a). Figure 11b shows the comparison between G6sulfur and SSP2–4.5, which indicates a higher amount of AR precipitation for most of the upper midlatitudes, particularly central–northern China (by up to 160 mm yr−1). Likewise, the comparison between G6sulfur and G6solar (Fig. 11c) indicates greater AR precipitation (by up to 100 mm yr−1) for the same regions. For the AR-related heavy rain events, although ameliorated increases are shown in central and eastern China when comparing G6sulfur with SSP5–8.5 (Fig. 11d), the simulated SAI strategy exhibits amplified increases in the events in central–northern China and the Korean Peninsula with respect to SSP2–4.5 (Fig. 11e) and G6solar (Fig. 11f), implying a considerable side effect of SAI on extreme precipitation.
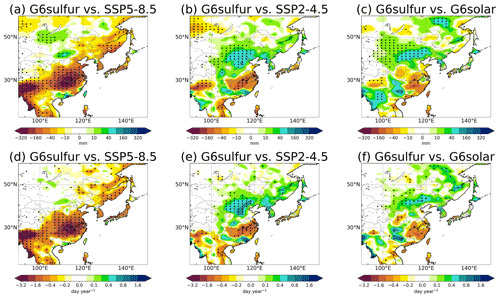
Figure 11Differences in annual accumulation of AR-related precipitation (a–c) and heavy rain events (d–f) for the future period of 2071–2100 between G6sulfur and SSP5–8.5 (a, d) and SSP2–4.5 (b, e) and G6solar (c, f). Stippling indicates differences that are statistically significant at a confidence level > 95 % (p value < 0.05 based on Student's t test).
In this study, the future changes in ARs and associated precipitation over East Asia by the end of the 21st century are examined using the historical (1981–2010) and future climate (2071–2100) simulations based on UKESM1 under different external climate forcings. The effect of SAI on ARs is assessed by comparing the experiment G6sulfur with the idealised solar dimming condition (G6solar) and high (SSP5–8.5) and medium (SSP2–4.5) levels of GHG emissions. The conclusions of the paper are summarised as follows:
-
Under both the SSP2–4.5 and SSP5–8.5 scenarios, a strengthening of the upstream monsoon flows and low-level convergence is projected, which creates a more favourable environment for AR activity in most of East Asia. Consequently, an increase in AR frequency and AR-associated precipitation relative to the historical baseline period is projected in the study region under SSP5-8.5. In addition, a weakening of the EAJS driven by the increase in high-tropospheric thermal expansion at upper midlatitudes is projected. Given the negative correlation between the strength of EAJS and the local AR frequency at higher latitudes, northward shifts in AR activity under the weakening of EAJS leads to significant increases in AR-associated mean and extreme precipitation at upper midlatitudes, particularly in northeastern China.
-
The comparison of the identified AR features among the different experiments shows that the simulated SAI strategy is effective at partly mitigating the projected future increase in AR activity under SSP5–8.5 over the study region, particularly in southern China; however, such a strategy is generally less effective than that of the GHG emission reductions considered by SSP2–4.5.
-
The simulated SAI strategy in G6sulfur exacerbates the weakening of the EAJS due to the concentrated cooling effect of the injected aerosol precursors across the lower latitudes. It also leads to stronger land–sea thermal contrast that favours ARs with respect to SSP2–4.5 and G6solar. As a result, a side effect of SAI exacerbating the increases in the activity of upper-midlatitude ARs and associated mean and extreme precipitation is observed, particularly over northeastern China and the Korean Peninsula.
The presented future changes in AR activity over East Asia, particularly the increase in AR frequency over southern China, agree well with previous AR projection studies (Espinoza et al., 2018; Kamae et al., 2021). These similarities imply additional confidence in the reported future AR projection in this study. However, any presented changes should be carefully interpreted, especially considering the bias of UKESM1 in simulating the spatial distributions of AR frequency and AR precipitation (as shown in the Supplement). Particularly, the underestimation of the downstream AR frequency for UKESM1 compared to the ERA5 reanalysis dataset is possibly related to the use of relatively coarse horizontal resolution, according to Liang and Yong (2022). Moreover, the presented future changes in ARs are based on the ensemble mean of three different realisation set-ups of UKESM1, which is limited to exclude model uncertainties and impacts from the internal variability. These should be addressed by future study depending on the potential improvement in the data availability for GeoMIP GCMs. Nevertheless, over the Far East, the opposite effects of the radiative forcing of the CO2 concentration and the warming of the SST on the land–sea thermal contrast and the associated monsoon circulation have been observed (Kamae et al., 2014), and such opposite effects are associated with the limited confidence in the projected changes in the Asian monsoon systems (Voigt and Shaw, 2015); hence, investigations of these effects under SAI should be supplemented in a future study to help assess the uncertainty in the simulation of the SAI–AR connection across the study region.
Another limitation is the lack of analyses of the uncertainties associated with the choice of ARDTs due to the limited resources, although some configuration and the performance of the used ARDT have demonstrated some similarities compared to other ARDTs in the ARTMIP protocol (Liang et al., 2022). Also, as the chosen ARDT rescales the IVT thresholds for each month, it partly excludes the signal of AR seasonality; thus, the projected change in AR seasonality is not investigated. This should be addressed in future work by changing the current thresholding set-up, such as the use of a fixed IVT threshold (Liang et al., 2022). Also, given the close linkage between rainfall extremes and tropical cyclones over the study region (e.g. Kim et al., 2012; Wang et al., 2020), future work should incorporate the analyses of the detected ARs with the identified tropical cyclones in the model simulation to help understand the dynamics behind the projected changes in extreme precipitation controlled by the changes in different weather systems.
The side effect of SAI manifested by the thermal response of the upper tropospheric circulation and its control of the local AR activity implies that any deployment of SAI should be evaluated with caution, given the existence of the links/teleconnections between the regional climate over the populated regions of East Asia and the large-scale circulation that is sensitive to the injected aerosol precursor. Another example of the side effect of SAI on precipitation, i.e. exacerbating precipitation deficit over the Mediterranean, is presented by Jones et al. (2022). Further studies are required to understand the potential hydrological impacts of SAI. This includes the use of hydrological modelling tools to simulate hydrological extremes associated with ARs at the watershed level (e.g. Dettinger et al., 2011; Chen et al., 2019), with climate inputs from the GeoMIP experiments. Furthermore, the presented side effects should be incorporated into the dissemination of climate change information for decision-makers involved with adaptation strategies in the populated regions. It also implies the necessity to optimise the potential deployment of SAI, including adjustments of the injection location and considering different candidates of the injected material that are more effective at increasing the outgoing radiation with less absorption (Jones et al., 2016). Overall, this paper calls for better awareness of the consequences brought by any practical deployment of SAI geoengineering from a perspective of high-impact weather systems and their association with extreme climate events.
All model data used in this work are available from the Earth System Grid Federation (https://esgf-node.llnl.gov/projects/cmip6; WCRP, 2022). The used ERA5 reanalysis dataset has been downloaded from the Copernicus Climate Data Store (https://cds.climate.copernicus.eu/cdsapp#!/dataset/reanalysis-era5-pressure-levels?tab=overview/; Copernicus, 2023). The APHRODITE data have been downloaded from their official website, which is managed by the Data Integration and Analysis System (https://search.diasjp.net/en/dataset/APHRO_MA; DIAS, 2022).
The supplement related to this article is available online at: https://doi.org/10.5194/acp-23-1687-2023-supplement.
JL and JH led the analysis and wrote the paper. Funding acquisition and the computational facility and resources for this research were contributed by JH.
The contact author has declared that neither of the authors has any competing interests.
Publisher's note: Copernicus Publications remains neutral with regard to jurisdictional claims in published maps and institutional affiliations.
Ju Liang and Jim Haywood would like to acknowledge support from the NERC-funded SASSO standard grant (grant no. NE/S00212X/1). Jim Haywood has been supported by the Met Office Hadley Centre Climate Programme funded by BEIS. Jim Haywood would also like to acknowledge support from the NERC-funded EXTEND project (grant no. NE/W003880/1) and from SilverLining through its Safe Climate Research Initiative. The authors thank Andy Jones, for help with the UKESM1 outputs.
This research has been supported by the Natural Environment Research Council (grant nos. NE/S00212X/1 and NE/W003880/1), the Met Office Hadley Centre Climate Programme funded by the Department of Business, Energy and Industrial Strategy (BEIS), and SilverLining's Safe Climate Research Initiative.
This paper was edited by Geraint Vaughan and reviewed by two anonymous referees.
Archibald, A. T., O'Connor, F. M., Abraham, N. L., Archer-Nicholls, S., Chipperfield, M. P., Dalvi, M., Folberth, G. A., Dennison, F., Dhomse, S. S., Griffiths, P. T., Hardacre, C., Hewitt, A. J., Hill, R. S., Johnson, C. E., Keeble, J., Köhler, M. O., Morgenstern, O., Mulcahy, J. P., Ordóñez, C., Pope, R. J., Rumbold, S. T., Russo, M. R., Savage, N. H., Sellar, A., Stringer, M., Turnock, S. T., Wild, O., and Zeng, G.: Description and evaluation of the UKCA stratosphere–troposphere chemistry scheme (StratTrop vn 1.0) implemented in UKESM1, Geosci. Model Dev., 13, 1223–1266, https://doi.org/10.5194/gmd-13-1223-2020, 2020.
Best, M. J., Pryor, M., Clark, D. B., Rooney, G. G., Essery, R. L. H., Ménard, C. B., Edwards, J. M., Hendry, M. A., Porson, A., Gedney, N., Mercado, L. M., Sitch, S., Blyth, E., Boucher, O., Cox, P. M., Grimmond, C. S. B., and Harding, R. J.: The Joint UK Land Environment Simulator (JULES), model description – Part 1: Energy and water fluxes, Geosci. Model Dev., 4, 677–699, https://doi.org/10.5194/gmd-4-677-2011, 2011.
Chen, X., Leung, L. R., Wigmosta, M., and Richmond, M.: Impact of atmospheric rivers on surface hydrological processes in western U.S. watersheds, J. Geophys. Res.-Atmos., 124, 8896–8916, https://doi.org/10.1029/2019JD030468, 2019.
Collow, A. B. M., Shields, C. A., Guan, B., Kim, S., Lora, J. M., McClenny, E. E., Nardi, K., Payne, A., Reid, K., Shearer, E. J., Tomé, R., Wille, J. D., Ramos, A. M., Gorodetskaya, I. V., Leung, L. R., O'Brien, T. A., Ralph, F. M., Rutz, J., Ullrich, P. A., and Wehner, M.: An Overview of ARTMIP’s Tier 2 Reanalysis Intercomparison: Uncertainty in the Detection of Atmospheric Rivers and Their Associated Precipitation, J. Geophys. Res.-Atmos., 127, e2021JD036155, https://doi.org/10.1029/2021JD036155, 2022.
Copernicus: ERA5 hourly data on pressure levels from 1959 to present, Climate Data Store [data set], https://cds.climate.copernicus.eu/cdsapp#!/dataset/reanalysis-era5-pressure-levels?tab=overview/, last access: 5 January 2023.
Corringham, T. W., Martin Ralph, F., Gershunov, A., Cayan, D. R., and Talbot, C. A.: Atmospheric rivers drive flood damages in the western United States, Sci. Adv., 5, 1–7, https://doi.org/10.1126/sciadv.aax4631, 2019.
Dettinger, M. D., Ralph, F. M., Das, T., Neiman, P. J., and Cayan, D. R.: Atmospheric rivers, floods and the water resources of California, Water, 3, 445–478, https://doi.org/10.3390/w3020445, 2011.
DIAS – Data Integration and Analysis System: APHRODITE monsoon Asia Precipitation data, DIAS Dataset Search and Discovery [data set], https://search.diasjp.net/en/dataset/APHRO_MA (last access: 23 January 2023), 2022.
Dominguez, F., Dall'erba, S., Huang, S., Avelino, A., Mehran, A., Hu, H., Schmidt, A., Schick, L., and Lettenmaier, D.: Tracking an atmospheric river in a warmer climate: from water vapor to economic impacts, Earth Syst. Dynam., 9, 249–266, https://doi.org/10.5194/esd-9-249-2018, 2018.
Endo, H., Kitoh, A., and Ueda, H.: A unique feature of the Asian summer monsoon response to global warming: The role of different land-sea thermal contrast change between the lower and upper troposphere, Sci. Online Lett. Atmos., 14, 57–63, https://doi.org/10.2151/SOLA.2018-010, 2018.
Espinoza, V., Waliser, D. E., Guan, B., Lavers, D. A., and Ralph, F. M.: Global analysis of climate change projection effects on atmospheric rivers, Geophys. Res. Lett., 45, 4299–4308, https://doi.org/10.1029/2017GL076968, 2018.
Eyring, V., Bony, S., Meehl, G. A., Senior, C. A., Stevens, B., Stouffer, R. J., and Taylor, K. E.: Overview of the Coupled Model Intercomparison Project Phase 6 (CMIP6) experimental design and organization, Geosci. Model Dev., 9, 1937–1958, https://doi.org/10.5194/gmd-9-1937-2016, 2016.
Fu, G., Liu, S., Li, X., Li, P., and Chen, L.: Characteristics of atmospheric rivers over the East Asia in middle summers from 2001 to 2016, J. Ocean Univ. China, 20, 235–243, https://doi.org/10.1007/s11802-021-4513-x, 2021.
Gertler, C. G., O'Gorman, P. A., Kravitz, B., Moore, J. C., Phipps, S. J., and Watanabe, S.: Weakening of the Extratropical Storm Tracks in Solar Geoengineering Scenarios, Geophys. Res. Lett., 47, e2020GL087348, https://doi.org/10.1029/2020GL087348, 2020.
Gimeno, L., Dominguez, F., Nieto, R., Trigo, R., Drumond, A., Reason, C. J. C., Taschetto, A. S., Ramos, A. M., Kumar, R., and Marengo, J.: Major mechanisms of atmospheric moisture transport and their role in extreme precipitation events, Annu. Rev. Environ. Resour., 41, 117–141, https://doi.org/10.1146/annurev-environ-110615-085558, 2016.
Guan, B., Waliser, D. E., Ralph, F. M., Fetzer, E. J., and Neiman, P. J.: Hydrometeorological characteristics of rain-on-snow events associated with atmospheric rivers, Geophys. Res. Lett., 43, 2964–2973, https://doi.org/10.1002/2016GL067978, 2016.
Hamada, A., Arakawa, O., and Yatagai, A.: An automated quality control method for daily rain-gauge data, Global Environ. Res., 15, 183–192, 2011.
Harvey, B. J., Cook, P., Shaffrey, L. C., and Schiemann, R.: The response of the northern hemisphere storm tracks and jet streams to climate change in the CMIP3, CMIP5, and CMIP6 Climate Models, J. Geophys. Res.-Atmos., 125, e2020JD032701, https://doi.org/10.1029/2020JD032701, 2020.
Heckendorn, P., Weisenstein, D., Fueglistaler, S., Luo, B. P., Rozanov, E., Schraner, M., Thomason, L. W., and Peter, T.: The impact of geoengineering aerosols on stratospheric temperature and ozone, Environ. Res. Lett., 4, 045108, https://doi.org/10.1088/1748-9326/4/4/045108, 2009.
Hersbach, H., Bell, B., Berrisford, P., Hirahara, S., Horányi, A., Muñoz-Sabater, J., Nicolas, J., Peubey, C., Radu, R., Schepers, D., Simmons, A., Soci, C., Abdalla, S., Abellan, X., Balsamo, G., Bechtold, P., Biavati, G., Bidlot, J., Bonavita, M., De Chiara, G., Dahlgren, P., Dee, D., Diamantakis, M., Dragani, R., Flemming, J., Forbes, R., Fuentes, M., Geer, A., Haimberger, L., Healy, S., Hogan, R. J., Hólm, E., Janisková, M., Keeley, S., Laloyaux, P., Lopez, P., Lupu, C., Radnoti, G., de Rosnay, P., Rozum, I., Vamborg, F., Villaume, S., and Thépaut, J. N.: The ERA5 global reanalysis, Q. J. Roy. Meteorol. Soc., 146, 1999–2049, https://doi.org/10.1002/qj.3803, 2020.
IPCC – Intergovernmental Panel on Climate Change: Global warming of 1.5 ∘C, in: An IPCC Special Report on the impacts of global warming of 1.5 ∘C above pre-industrial levels and related global greenhouse gas emission pathways, in the context of strengthening the global response to the threat of climate change, sustainable development, and efforts to eradicate poverty, edited by: Masson-Delmotte, V., Zhai, P., Pörtner, H. O., Roberts, D., Skea, J., Shukla, P. R., Pirani, A., Moufouma-Okia, W., Péan, C., Pidcock, R., Connors, S., Matthews, J. B. R., Chen, Y., Zhou, X., Gomis, M. I., Lonnoy, E., Maycock, T., Tignor, M., and Waterfield, T., https://www.ipcc.ch/sr15/ (last access: 22 September 2022), 2018.
Jones, A., Haywood, J. M., Alterskjær, K., Boucher, O., Cole, J. N. S., Curry, C. L., Irvine, P. J., Ji, D., Kravitz, B., Egill Kristjánsson, J., Moore, J. C., Niemeier, U., Robock, A., Schmidt, H., Singh, B., Tilmes, S., Watanabe, S., and Yoon, J. H.: The impact of abrupt suspension of solar radiation management (termination effect) in experiment G2 of the Geoengineering Model Intercomparison Project (GeoMIP), J. Geophys. Res.-Atmos., 118, 9743–9752, https://doi.org/10.1002/jgrd.50762, 2013.
Jones, A., Haywood, J. M., Jones, A. C., Tilmes, S., Kravitz, B., and Robock, A.: North Atlantic Oscillation response in GeoMIP experiments G6solar and G6sulfur: Why detailed modelling is needed for understanding regional implications of solar radiation management, Atmos. Chem. Phys., 21, 1287–1304, https://doi.org/10.5194/acp-21-1287-2021, 2021.
Jones, A., Haywood, J. M., Scaife, A. A., Boucher, O., Henry, M., Kravitz, B., Lurton, T., Nabat, P., Niemeier, U., Séférian, R., Tilmes, S., and Visioni, D.: The impact of stratospheric aerosol intervention on the North Atlantic and Quasi-Biennial Oscillations in the Geoengineering Model Intercomparison Project (GeoMIP) G6sulfur experiment, Atmos. Chem. Phys., 22, 2999–3016, https://doi.org/10.5194/acp-22-2999-2022, 2022.
Jones, A. C., Haywood, J. M., and Jones, A.: Climatic impacts of stratospheric geoengineering with sulfate, black carbon and titania injection, Atmos. Chem. Phys., 16, 2843–2862, https://doi.org/10.5194/acp-16-2843-2016, 2016.
Jones, A. C., Haywood, J. M., Dunstone, N., Emanuel, K., Hawcroft, M. K., Hodges, K. I., and Jones, A.: Impacts of hemispheric solar geoengineering on tropical cyclone frequency, Nat. Commun., 81, 1–10, https://doi.org/10.1038/s41467-017-01606-0, 2017.
Jones, A. C., Hawcroft, M. K., Haywood, J. M., Jones, A., Guo, X., and Moore, J. C.: Regional Climate Impacts of Stabilizing Global Warming at 1.5 K Using Solar Geoengineering, Earth's Future, 6, 230–251, https://doi.org/10.1002/2017EF000720, 2018.
Kamae, Y., Watanabe, M., Kimoto, M., and Shiogama, H.: Summertime land–sea thermal contrast and atmospheric circulation over East Asia in a warming climate – Part II: Importance of CO2-induced continental warming, Clim. Dynam., 43, 2569–2583, https://doi.org/10.1007/s00382-014-2146-0, 2014.
Kamae, Y., Mei, W., and Xie, S. P.: Climatological relationship between warm season atmospheric rivers and heavy rainfall over east asia, J. Meteorol. Soc. Jpn., 95, 411–431, https://doi.org/10.2151/jmsj.2017-027, 2017a.
Kamae, Y., Mei, W., Xie, S. P., Naoi, M., and Ueda, H.: Atmospheric rivers over the Northwestern Pacific: Climatology and interannual variability, J. Climate, 30, 5605–5619, https://doi.org/10.1175/JCLI-D-16-0875.1, 2017b.
Kamae, Y., Imada, Y., Kawase, H., and Mei, W.: Atmospheric rivers bring more frequent and intense extreme rainfall events over East Asia under global warming, Geophys. Res. Lett., 48, e2021GL096030, https://doi.org/10.1029/2021GL096030, 2021.
Kim, J., Moon, H., Guan, B., Waliser, D. E., Choi, J., Gu, T. Y., and Byun, Y. H.: Precipitation characteristics related to atmospheric rivers in East Asia, Int. J. Climatol., 41, E2244–E2257, https://doi.org/10.1002/joc.6843, 2021.
Kim, J. S., Li, R. C. Y., and Zhou, W.: Effects of the Pacific-Japan teleconnection pattern on tropical cyclone activity and extreme precipitation events over the Korean peninsula, J. Geophys. Res.-Atmos., 117, 18109, https://doi.org/10.1029/2012JD017677, 2012.
Kravitz, B., Robock, A., Boucher, O., Schmidt, H., Taylor, K. E., Stenchikov, G., and Schulz, M.: The Geoengineering Model Intercomparison Project (GeoMIP), Atmos. Sci. Lett., 12, 162–167, https://doi.org/10.1002/asl.316, 2011.
Kravitz, B., Robock, A., Tilmes, S., Boucher, O., English, J. M., Irvine, P. J., Jones, A., Lawrence, M. G., MacCracken, M., Muri, H., Moore, J. C., Niemeier, U., Phipps, S. J., Sillmann, J., Storelvmo, T., Wang, H., and Watanabe, S.: The Geoengineering Model Intercomparison Project Phase 6 (GeoMIP6): Simulation design and preliminary results, Geosci. Model Dev., 8, 3379–3392, https://doi.org/10.5194/gmd-8-3379-2015, 2015.
Lawrence, M. G., Schäfer, S., Muri, H., Scott, V., Oschlies, A., Vaughan, N. E., Boucher, O., Schmidt, H., Haywood, J., and Scheffran, J.: Evaluating climate geoengineering proposals in the context of the Paris Agreement temperature goals, Nat. Commun., 91, 1–19, https://doi.org/10.1038/s41467-018-05938-3, 2018.
Liang, J. and Sushama, L.: Freezing rain events related to atmospheric rivers and associated mechanisms for western North America, Geophys. Res. Lett., 46, 10541–10550, https://doi.org/10.1029/2019GL084647, 2019.
Liang, J. and Yong, Y.: Climatology of atmospheric rivers in the Asian monsoon region, Int. J. Climatol., 41, E801–E818, https://doi.org/10.1002/joc.6729, 2021.
Liang, J. and Yong, Y.: Sensitivity of the simulated atmospheric rivers over East Asia to horizontal resolution in the HadGEM3-GC3.1 general circulation model, Atmos. Res., 275, 106244, https://doi.org/10.1016/j.atmosres.2022.106244, 2022.
Liang, J., Yong, Y., and Hawcroft, M. K.: Long-term trends in atmospheric rivers over East Asia, Clim. Dynam., https://doi.org/10.1007/S00382-022-06339-5, in press, 2022.
Liang, P., Dong, G., Zhang, H., Zhao, M., Ma, Y., Liang, P., Dong, G., Zhang, H., Zhao, M., and Ma, Y.: Atmospheric rivers associated with summer heavy rainfall over the Yangtze Plain, J. South. Hemisph. Earth Syst. Sci., 70, 54–69, https://doi.org/10.1071/ES19028, 2020.
Liu, Z., Lang, X., and Jiang, D.: Impact of stratospheric aerosol injection geoengineering on the summer climate over East Asia, J. Geophys. Res.-Atmos., 126, e2021JD035049, https://doi.org/10.1029/2021JD035049, 2021.
Lu, R., Ye, H., and Jhun, J. G.: Weakening of interannual variability in the summer East Asian upper-tropospheric westerly jet since the mid-1990s, Adv. Atmos. Sci., 28, 1246–1258, https://doi.org/10.1007/s00376-011-0222-5, 2011.
MacMartin, D. G., Ricke, K. L., and Keith, D. W.: Solar geoengineering as part of an overall strategy for meeting the 1.5 ∘C Paris target, Philos. T. Roy. Soc. A, 376, 1–19, https://doi.org/10.1098/RSTA.2016.0454, 2018.
Mann, G. W., Carslaw, K. S., Spracklen, D. V., Ridley, D. A., Manktelow, P. T., Chipperfield, M. P., Pickering, S. J., and Johnson, C. E.: Description and evaluation of GLOMAP-mode: A modal global aerosol microphysics model for the UKCA composition-climate model, Geosci. Model Dev., 3, 519–551, https://doi.org/10.5194/gmd-3-519-2010, 2010.
Millar, R. J., Fuglestvedt, J. S., Friedlingstein, P., Rogelj, J., Grubb, M. J., Matthews, H. D., Skeie, R. B., Forster, P. M., Frame, D. J., and Allen, M. R.: Emission budgets and pathways consistent with limiting warming to 1.5 ∘C, Nat. Geosci., 10, 741–747, https://doi.org/10.1038/NGEO3031, 2017.
Miller, D. K., Hotz, D., Winton, J., and Stewart, L.: Investigation of atmospheric rivers impacting the Pigeon River basin of the southern Appalachian Mountains, Weather Forecast., 33, 283–299, https://doi.org/10.1175/WAF-D-17-0060.1, 2018.
Morgenstern, O., Braesicke, P., O'Connor, F. M., Bushell, A. C., Johnson, C. E., Osprey, S. M., and Pyle, J. A.: Evaluation of the new UKCA climate-composition model – Part 1: The stratosphere, Geosci. Model Dev., 2, 43–57, https://doi.org/10.5194/gmd-2-43-2009, 2009.
Mulcahy, J. P., Jones, C., Sellar, A., Johnson, B., Boutle, I. A., Jones, A., Andrews, T., Rumbold, S. T., Mollard, J., Bellouin, N., Johnson, C. E., Williams, K. D., Grosvenor, D. P., and McCoy, D. T.: Improved aerosol processes and effective radiative forcing in HadGEM3 and UKESM1, J. Adv. Model. Earth Syst., 10, 2786–2805, https://doi.org/10.1029/2018MS001464, 2018.
Mullen, S. L.: Model Experiments on the Impact of Pacific Sea Surface Temperature Anomalies on Blocking Frequency, J. Climate, 2, 997–1013, https://doi.org/10.1175/1520-0442(1989)002<0997:meotio>2.0.co;2, 1989.
Myhre, G., Alterskjær, K., Stjern, C. W., Hodnebrog, Marelle, L., Samset, B. H., Sillmann, J., Schaller, N., Fischer, E., Schulz, M., and Stohl, A.: Frequency of extreme precipitation increases extensively with event rareness under global warming, Sci. Rep., 9, 1–10, https://doi.org/10.1038/s41598-019-52277-4, 2019.
Neff, W.: Atmospheric rivers melt Greenland, Nat. Clim. Change, 8, 857–858, https://doi.org/10.1038/s41558-018-0297-4, 2018.
O'Brien, T. A., Wehner, M. F., Payne, A. E., Shields, C. A., Rutz, J. J., Leung, L. R., Ralph, F. M., Collow, A., Gorodetskaya, I., Guan, B., Lora, J. M., McClenny, E., Nardi, K. M., Ramos, A. M., Tomé, R., Sarangi, C., Shearer, E. J., Ullrich, P. A., Zarzycki, C., Loring, B., Huang, H., Inda-Díaz, H. A., Rhoades, A. M., and Zhou, Y.: Increases in future AR count and size: Overview of the ARTMIP Tier 2 CMIP5/6 experiment, J. Geophys. Res.-Atmos., 127, e2021JD036013, https://doi.org/10.1029/2021JD036013, 2022.
O'Neill, B. C., Tebaldi, C., Van Vuuren, D. P., Eyring, V., Friedlingstein, P., Hurtt, G., Knutti, R., Kriegler, E., Lamarque, J. F., Lowe, J., Meehl, G. A., Moss, R., Riahi, K., and Sanderson, B. M.: The Scenario Model Intercomparison Project (ScenarioMIP) for CMIP6, Geosci. Model Dev., 9, 3461–3482, https://doi.org/10.5194/gmd-9-3461-2016, 2016.
Pan, M. and Lu, M.: A novel atmospheric river identification algorithm, Water Resour. Res., 55, 6069–6087, https://doi.org/10.1029/2018wr024407, 2019.
Pan, M. and Lu, M.: East Asia atmospheric river catalog: Annual cycle, transition mechanism, and precipitation, Geophys. Res. Lett., 47, 1–10, https://doi.org/10.1029/2020GL089477, 2020.
Park, C., Son, S. W., and Kim, H.: Distinct features of atmospheric rivers in the early versus late East Asian summer monsoon and their impacts on monsoon rainfall, J. Geophys. Res.-Atmos., 126, e2020JD033537, https://doi.org/10.1029/2020JD033537, 2021.
Payne, A. E. and Magnusdottir, G.: An evaluation of atmospheric rivers over the North Pacific in CMIP5 and their response to warming under RCP 8.5, J. Geophys. Res., 120, 11173–11190, https://doi.org/10.1002/2015JD023586, 2015.
Payne, A. E., Demory, M. E., Leung, L. R., Ramos, A. M., Shields, C. A., Rutz, J. J., Siler, N., Villarini, G., Hall, A., and Ralph, F. M.: Responses and impacts of atmospheric rivers to climate change, Nat. Rev. Earth Environ., 13, 143–157, https://doi.org/10.1038/s43017-020-0030-5, 2020.
Pohl, B., Favier, V., Wille, J., Udy, D. G., Vance, T. R., Pergaud, J., Dutrievoz, N., Blanchet, J., Kittel, C., Amory, C., Krinner, G., and Codron, F.: Relationship between weather regimes and atmospheric rivers in east antarctica, J. Geophys. Res.-Atmos., 126, e2021JD035294, https://doi.org/10.1029/2021JD035294, 2021.
Rutz, J. J., Shields, C. A., Lora, J. M., Payne, A. E., Guan, B., Ullrich, P., O'Brien, T., Leung, L. R., Ralph, F. M., Wehner, M., Brands, S., Collow, A., Goldenson, N., Gorodetskaya, I., Griffith, H., Kashinath, K., Kawzenuk, B., Krishnan, H., Kurlin, V., Lavers, D., Magnusdottir, G., Mahoney, K., McClenny, E., Muszynski, G., Nguyen, P. D., Prabhat, M., Qian, Y., Ramos, A. M., Sarangi, C., Sellars, S., Shulgina, T., Tome, R., Waliser, D., Walton, D., Wick, G., Wilson, A. M., and Viale, M.: The Atmospheric River Tracking Method Intercomparison Project (ARTMIP): Quantifying uncertainties in atmospheric river climatology, J. Geophys. Res.-Atmos., 124, 13777–13802, https://doi.org/10.1029/2019JD030936, 2019.
Ryu, Y., Moon, H., Kim, J., Kim, T. J., Boo, K. O., Guan, B., Kamae, Y., Mei, W., Park, C., Son, S. W., and Byun, Y. H.: A Multi-Inventory Ensemble Analysis of the Effects of Atmospheric Rivers on Precipitation and Streamflow in the Namgang-Dam Basin in Korea, Water Resour. Res., 57, e2021WR030058, https://doi.org/10.1029/2021WR030058, 2021.
Sellar, A. A., Jones, C. G., Mulcahy, J. P., Tang, Y., Yool, A., Wiltshire, A., O'Connor, F. M., Stringer, M., Hill, R., Palmieri, J., Woodward, S., de Mora, L., Kuhlbrodt, T., Rumbold, S. T., Kelley, D. I., Ellis, R., Johnson, C. E., Walton, J., Abraham, N. L., Andrews, M. B., Andrews, T., Archibald, A. T., Berthou, S., Burke, E., Blockley, E., Carslaw, K., Dalvi, M., Edwards, J., Folberth, G. A., Gedney, N., Griffiths, P. T., Harper, A. B., Hendry, M. A., Hewitt, A. J., Johnson, B., Jones, A., Jones, C. D., Keeble, J., Liddicoat, S., Morgenstern, O., Parker, R. J., Predoi, V., Robertson, E., Siahaan, A., Smith, R. S., Swaminathan, R., Woodhouse, M. T., Zeng, G., and Zerroukat, M.: UKESM1: Description and Evaluation of the U.K. Earth System Model, J. Adv. Model. Earth Syst., 11, 4513–4558, https://doi.org/10.1029/2019MS001739, 2019.
Shields, C. A., Rutz, J. J., Leung, L. Y., Martin Ralph, F., Wehner, M., Kawzenuk, B., Lora, J. M., McClenny, E., Osborne, T., Payne, A. E., Ullrich, P., Gershunov, A., Goldenson, N., Guan, B., Qian, Y., Ramos, A. M., Sarangi, C., Sellars, S., Gorodetskaya, I., Kashinath, K., Kurlin, V., Mahoney, K., Muszynski, G., Pierce, R., Subramanian, A. C., Tome, R., Waliser, D., Walton, D., Wick, G., Wilson, A., Lavers, D., Prabhat, Collow, A., Krishnan, H., Magnusdottir, G., and Nguyen, P.: Atmospheric River Tracking Method Intercomparison Project (ARTMIP): Project goals and experimental design, Geosci. Model Dev., 11, 2455–2474, https://doi.org/10.5194/gmd-11-2455-2018, 2018.
Shields, C. A., Rosenbloom, N., Bates, S., Hannay, C., Hu, A., Payne, A. E., Rutz, J. J., and Truesdale, J.: Meridional heat transport during atmospheric rivers in high-resolution CESM climate projections, Geophys. Res. Lett., 46, 14702–14712, https://doi.org/10.1029/2019GL085565, 2019.
Shields, C. A., Richter, J. H., Pendergrass, A., and Tilmes, S.: Atmospheric rivers impacting western North America in a world with climate intervention, npj Clim. Atmos. Sci., 51, 1–10, https://doi.org/10.1038/s41612-022-00260-8, 2022.
Storkey, D., Blaker, A. T., Mathiot, P., Megann, A., Aksenov, Y., Blockley, E. W., Calvert, D., Graham, T., Hewitt, H. T., Hyder, P., Kuhlbrodt, T., Rae, J. G. L., and Sinha, B.: UK Global Ocean GO6 and GO7: A traceable hierarchy of model resolutions, Geosci. Model Dev., 11, 3187–3213, https://doi.org/10.5194/gmd-11-3187-2018, 2018.
Sun, W., Wang, B., Chen, D., Gao, C., Lu, G., and Liu, J.: Global monsoon response to tropical and Arctic stratospheric aerosol injection, Clim. Dynam., 55, 2107–2121, https://doi.org/10.1007/s00382-020-05371-7, 2020.
Tilmes, S., Müller, R., and Salawitch, R.: The sensitivity of polar ozone depletion to proposed geoengineering schemes, Science, 320, 1201–1204, https://doi.org/10.1126/science.1153966, 2008.
Tilmes, S., Richter, J. H., Kravitz, B., Macmartin, D. G., Mills, M. J., Simpson, I. R., Glanville, A. S., Fasullo, J. T., Phillips, A. S., Lamarque, J. F., Tribbia, J., Edwards, J., Mickelson, S., and Ghosh, S.: CESM1(WACCM) Stratospheric Aerosol Geoengineering Large Ensemble Project, B. Am. Meteorol. Soc., 99, 2361–2371, https://doi.org/10.1175/BAMS-D-17-0267.1, 2018.
Tilmes, S., Richter, J. H., Kravitz, B., MacMartin, D. G., Glanville, A. S., Visioni, D., Kinnison, D. E., and Müller, R.: Sensitivity of total column ozone to stratospheric sulfur injection strategies, Geophys. Res. Lett., 48, e2021GL094058, https://doi.org/10.1029/2021GL094058, 2021.
Tilmes, S., Visioni, D., Jones, A., Haywood, J., Séférian, R., Nabat, P., Boucher, O., Bednarz, E. M., and Niemeier, U.: Stratospheric ozone response to sulfate aerosol and solar dimming climate interventions based on the G6 Geoengineering Model Intercomparison Project (GeoMIP) simulations, Atmos. Chem. Phys., 22, 4557–4579, https://doi.org/10.5194/acp-22-4557-2022, 2022.
Uccellini, L. W. and Johnson, D. R.: The coupling of upper and lower tropospheric jet streaks and implications for the development of severe convective storms, Mon. Weather Rev., 107, 682–703, https://doi.org/10.1175/1520-0493(1979)107<0682:TCOUAL>2.0.CO;2, 1979.
Visioni, D., Macmartin, D. G., Kravitz, B., Boucher, O., Jones, A., Lurton, T., Martine, M., Mills, M. J., Nabat, P., Niemeier, U., Séférian, R., and Tilmes, S.: Identifying the sources of uncertainty in climate model simulations of solar radiation modification with the G6sulfur and G6solar Geoengineering Model Intercomparison Project (GeoMIP) simulations, Atmos. Chem. Phys., 21, 10039–10063, https://doi.org/10.5194/acp-21-10039-2021, 2021.
Voigt, A. and Shaw, T. A.: Circulation response to warming shaped by radiative changes of clouds and water vapour, Nat. Geosci., 8, 102–106, https://doi.org/10.1038/ngeo2345, 2015.
Walters, D., Baran, A. J., Boutle, I., Brooks, M., Earnshaw, P., Edwards, J., Furtado, K., Hill, P., Lock, A., Manners, J., Morcrette, C., Mulcahy, J., Sanchez, C., Smith, C., Stratton, R., Tennant, W., Tomassini, L., Van Weverberg, K., Vosper, S., Willett, M., Browse, J., Bushell, A., Carslaw, K., Dalvi, M., Essery, R., Gedney, N., Hardiman, S., Johnson, B., Johnson, C., Jones, A., Jones, C., Mann, G., Milton, S., Rumbold, H., Sellar, A., Ujiie, M., Whitall, M., Williams, K., and Zerroukat, M.: The Met Office Unified Model Global Atmosphere 7.0/7.1 and JULES Global Land 7.0 configurations, Geosci. Model Dev., 12, 1909–1963, https://doi.org/10.5194/gmd-12-1909-2019, 2019.
Wang, L., Yang, Z., Gu, X., and Li, J.: Linkages Between Tropical Cyclones and Extreme Precipitation over China and the Role of ENSO, Int. J. Disast. Risk Sci., 11, 538–553, https://doi.org/10.1007/s13753-020-00285-8, 2020.
Wang, Q., Moore, J. C., and Ji, D.: A statistical examination of the effects of stratospheric sulfate geoengineering on tropical storm genesis, Atmos. Chem. Phys., 18, 9173–9188, https://doi.org/10.5194/acp-18-9173-2018, 2018.
WCRP – World Climate Research Programme: CMIP6 project data, ESGF [data set], https://esgf-node.llnl.gov/projects/cmip6/, last access: 28 July 2022.
Wick, G. A., Neiman, P. J., and Ralph, F. M.: Description and validation of an automated objective technique for identification and characterization of the integrated water vapor signature of atmospheric rivers, IEEE T. Geosci. Remote, 51, 2166–2176, https://doi.org/10.1109/TGRS.2012.2211024, 2013.
Wille, J. D., Favier, V., Jourdain, N. C., Kittel, C., Turton, J. V., Agosta, C., Gorodetskaya, I. V., Picard, G., Codron, F., Dos Santos, C. L., Amory, C., Fettweis, X., Blanchet, J., Jomelli, V., and Berchet, A.: Intense atmospheric rivers can weaken ice shelf stability at the Antarctic Peninsula, Commun. Earth Environ., 3, 1–14, https://doi.org/10.1038/s43247-022-00422-9, 2022.
Williamson, P. and Turley, C.: Ocean acidification in a geoengineering context, Philos. T. Roy. Soc. A, 370, 4317–4342, https://doi.org/10.1098/RSTA.2012.0167, 2012.
Wood, N., Staniforth, A., White, A., Allen, T., Diamantakis, M., Gross, M., Melvin, T., Smith, C., Vosper, S., Zerroukat, M., and Thuburn, J.: An inherently mass-conserving semi-implicit semi-Lagrangian discretization of the deep-atmosphere global non-hydrostatic equations, Q. J. Roy. Meteorol. Soc., 140, 1505–1520, https://doi.org/10.1002/QJ.2235, 2014.
Xia, L., Nowack, J. P., Tilmes, S., and Robock, A.: Impacts of stratospheric sulfate geoengineering on tropospheric ozone, Atmos. Chem. Phys., 17, 11913–11928, https://doi.org/10.5194/acp-17-11913-2017, 2017.
Yatagai, A., Krishnamurti, T. N., Kumar, V., Mishra, A. K., and Simon, A.: Use of APHRODITE rain gauge-based precipitation and TRMM 3B43 products for improving asian monsoon seasonal precipitation forecasts by the superensemble method, J. Climate, 27, 1062–1069, https://doi.org/10.1175/JCLI-D-13-00332.1, 2014.
Yool, A., Popova, E. E., and Anderson, T. R.: MEDUSA-2.0: An intermediate complexity biogeochemical model of the marine carbon cycle for climate change and ocean acidification studies, Geosci. Model Dev., 6, 1767–1811, https://doi.org/10.5194/gmd-6-1767-2013, 2013.
Zhang, W. and Villarini, G.: Uncovering the role of the East Asian jet stream and heterogeneities in atmospheric rivers affecting the western United States, P. Natl. Acad. Sci. USA, 115, 891–896, https://doi.org/10.1073/pnas.1717883115, 2018.
Zhao, M.: Simulations of atmospheric rivers, their variability, and response to global warming using GFDL's new high-resolution general circulation model, J. Climate, 33, 10287–10303, https://doi.org/10.1175/JCLI-D-20-0241.1, 2020.