the Creative Commons Attribution 4.0 License.
the Creative Commons Attribution 4.0 License.
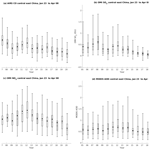
Changes in satellite retrievals of atmospheric composition over eastern China during the 2020 COVID-19 lockdowns
Robert D. Field
Jonathan E. Hickman
Igor V. Geogdzhayev
Kostas Tsigaridis
Susanne E. Bauer
We examined daily level-3 satellite retrievals of Atmospheric Infrared Sounder (AIRS) CO, Ozone Monitoring Instrument (OMI) SO2 and NO2, and Moderate Resolution Imaging Spectroradiometer (MODIS) aerosol optical depth (AOD) over eastern China to understand how COVID-19 lockdowns affected atmospheric composition. Changes in 2020 were strongly dependent on the choice of background period since 2005 and whether trends in atmospheric composition were accounted for. Over central east China during the 23 January–8 April lockdown window, CO in 2020 was between 3 % and 12 % lower than average depending on the background period. The 2020 CO was not consistently less than expected from trends beginning between 2005 and 2016 and ending in 2019 but was 3 %–4 % lower than the background mean during the 2017–2019 period when CO changes had flattened. Similarly for AOD, 2020 was between 14 % and 30 % lower than averages beginning in 2005 and 14 %–17 % lower compared to different background means beginning in 2016. NO2 in 2020 was between 30 % and 43 % lower than the mean over different background periods and between 17 % and 33 % lower than what would be expected for trends beginning later than 2011. Relative to the 2016–2019 period when NO2 had flattened, 2020 was 30 %–33 % lower. Over southern China, 2020 NO2 was between 23 % and 27 % lower than different background means beginning in 2013, the beginning of a period of persistently lower NO2. CO over southern China was significantly higher in 2020 than what would be expected, which we suggest was partly because of an active fire season in neighboring countries. Over central east and southern China, 2020 SO2 was higher than expected, but this depended strongly on how daily regional values were calculated from individual retrievals and reflects background values approaching the retrieval detection limit. Future work over China, or other regions, needs to take into account the sensitivity of differences in 2020 to different background periods and trends in order to separate the effects of COVID-19 on air quality from previously occurring changes or from variability in other sources.
- Article
(1396 KB) -
Supplement
(1314 KB) - BibTeX
- EndNote
In an effort to control the spread of COVID-19, the Chinese government implemented a range of restrictions on movement. These led to reductions in industrial and other work-related and personal activities starting 23 January 2020 in Wuhan, Hubei province, and then extending to other cities and regions in the days that followed. On 8 April 2020, Wuhan was the last city to reopen after a complete lockdown that prevented most people from leaving their homes. These measures have been linked to changes in air quality. A network of surface monitoring stations in northern China observed 35 % decreases in PM2.5 and 60 % decreases in NO2 concentrations during 29 January through 29 February, as compared to the preceding 3 weeks; CO and SO2 also declined (Shi and Brasseur, 2020). In and around Wuhan, decreases in NO2 and PM2.5 were similar to regional changes, but there was a slight increase in SO2 concentrations (Shi and Brasseur, 2020). Observations by the Tropospheric Monitoring Instrument (TROPOMI) showed large decreases in tropospheric NO2 column densities over Chinese cities, on the order of 40 % for 11 February to 24 March 2020 compared to the same period in 2019, ranging from roughly 25 % for cities not affected by lockdown to 60 % for Wuhan and Xi'an (Bauwens et al., 2020). Prospective simulations suggested that meteorology may limit the effect of reduced emissions on PM2.5 concentrations, with Chinese cities experiencing less than 20 % reductions (Wang et al., 2020).
The goal of our study was to consider these changes against pollution trends in China using NASA Earth Observing System data by combining several products to give a holistic view covering several emission sectors that are responsible for the observed changes. Over the last 2 to 3 decades, air pollution in China appears to have followed the pattern described by the environmental Kuznets curve (Selden and Song, 1994). This framework describes a relationship in which economic growth is initially accompanied by an increase in air pollution, when poverty remains widespread. But as growth continues, air pollution is expected to level off and decline as a consequence of changes in social awareness of environmental degradation and the economic, political, and technological capacity to limit it (Sarkodie and Strezov, 2019; Selden and Song, 1994).
Bottom-up and top-down assessments of air pollutant emissions and concentrations suggest that China has followed this pattern during the era of satellite monitoring of atmospheric composition, with concentrations of SO2, NO2, CO, and aerosol optical depth (AOD) mostly exhibiting marked and steady declines over the last decade. In the case of NO2, multi-instrument analyses, which extend the observational record beyond the lifetime of a single instrument, depict a consistent regional picture of NO2 trends in China since 1996 (Geddes et al., 2016; Georgoulias et al., 2019; Wang and Wang, 2020; Xu et al., 2020). Column totals show an increasing trend during the first part of the satellite record, but this trend is reversed sometime between 2010 and 2014 (Georgoulias et al., 2019; Krotkov et al., 2016; Lin et al., 2019; Xu et al., 2020; Si et al., 2019; Shah et al., 2020). The trend reversal has been attributed to a combination of emission control measures (Zheng et al., 2018a) and variations in economic growth (Krotkov et al., 2016).
Bottom-up estimates suggest that SO2 emissions peaked earlier, with declines starting around 2005, primarily as a result of power and industrial pollution control measures as well as the elimination of small industrial boilers (Sun et al., 2018; Zheng et al., 2018b). An earlier peak in SO2 emissions is consistent with observations by multiple satellite instruments, which revealed declines in SO2 column densities since 2005 (Fioletov et al., 2016; Krotkov et al., 2016; Wang and Wang, 2020; Zhang et al., 2017; Si et al., 2019).
AOD retrievals from the Along Track Scanning Radiometer instruments show a steady increase over southeastern China from 1995 to 2005 (Sogacheva et al., 2020) and a decline since 2005 in the MODIS AOD (He et al., 2019). The AOD peak has been argued to match the ∼ 2011 peak in NO2 (Zheng et al., 2018b; Xie et al., 2019), to match the ∼ 2005 peak of SO2, or to have occurred at some point in between (Ma et al., 2016), with more rapid decreases in AOD after 2011 (Lin et al., 2018). The recent decrease in AOD is also seen in Visible Infrared Imaging Radiometer Suite (VIIRS) retrievals (Sogacheva et al., 2020). Most mitigation of direct PM2.5 emissions since 2010 was by industry, with residential emissions also decreasing substantially (Zheng et al., 2018b). The decline in SO2 emissions also exerted an important influence, with the sulfate concentration of PM2.5 decreasing substantially between 2013 and 2017 (Shao et al., 2018), reflecting the negative trend in SO2 emissions.
The peak in concentrations of CO, which has an atmospheric lifetime ranging from weeks to months, is less easily identified. Some studies suggest that trends have been negative potentially throughout the 21st century (Han et al., 2018; Strode et al., 2016; Wang et al., 2018; Yumimoto et al., 2014; Zheng et al., 2018a), but others suggest that emissions and/or column densities were increasing or flat during at least the first decade of the century (Sun et al., 2018; Zhao et al., 2013, 2012). The negative trend has been attributed largely to reductions in emissions from industrial activity, as well as from residential and transportation sectors (Zheng et al., 2018a, b).
In addition to these long-term trends, a number of air pollutants also exhibit strong seasonal variation in China. Anthropogenic emissions of CO, SO2, and PM2.5 are highest in winter, reflecting large variation in emissions from the residential sector and, in the case of CO, increased emissions associated with cold-start processes in the transportation sector (Li et al., 2017). Outflow of CO and AOD has a spring maximum, resulting from transport of pollution, dust, and boreal biomass burning emissions (Han et al., 2018; Luan and Jaegle, 2013).
Changes in pollution over China have also come from short-term interventions. To improve air quality for the 2008 summer Olympics – a time when emissions in China were high and still increasing – the Chinese government imposed a series of strict emission control measures from July through 21 September 2008, which were qualitatively similar to the emission reductions expected to have accompanied the COVID-19 lockdown (UNEP, 2009). As a result, NO2 concentrations over Beijing were estimated to have declined by between 40 % and 60 % based on satellite observations, with substantial but smaller reductions in surrounding cities often on the order of 20 % to 30 % compared to previous years (Mijling et al., 2009; Witte et al., 2009). Regional reductions of SO2 and CO during the months of the games were estimated to be 13 % and 19 %, respectively (Witte et al., 2009). These results are broadly consistent with on-road observations (Wang et al., 2009) but larger than some surface observations comparing concentrations before and after the emission control measures were implemented (Wang et al., 2010).
The COVID-related lockdowns provide a similar natural experiment to the 2008 Beijing Olympics but on the other side of the Kuznets curve. The fact that the lockdowns occurred during years of decreasing air pollution needs to be taken into account in attributing changes in atmospheric composition to COVID-19 lockdowns, independent of the long-term trend. Following Chen et al.'s (2020) analysis of air quality improvements on mortality which controlled for changes in air quality since 2016, in this study we determine whether changes in 2020 in satellite retrievals of CO, SO2, NO2, and AOD departed significantly from the expected declines associated with the long-term decreases in concentrations resulting from pollution controls and technological change.
We used daily level-3 (L3) retrievals from four different instruments on three different NASA Earth Observing System satellites. The Atmospheric Infrared Sounder (AIRS) instrument aboard NASA's Aqua satellite is a 2300-channel infrared grating spectrometer in a sun-synchronous orbit with northward Equator crossing time of 13:30. AIRS carbon monoxide (CO) profiles are retrieved with horizontal resolution of 45 km at nadir, in a swath width of 30 fields of view or about 1600 km. The retrieval uses a cloud-clearing methodology providing CO with sensitivity that peaks around 500 hPa, with ∼ 0.8–1.2 degrees of freedom of signal for 50 %–70 % of scenes. More sampling and higher information content is obtained in clear scenes (Warner et al., 2013). We used the daily version 6 (AIRS3STD.006) product.
The Ozone Monitoring Instrument (OMI) aboard NASA's Aura satellite was launched in July 2004 and has a local Equator crossing time of roughly 13:45. OMI is a nadir-viewing spectrometer, which measures solar backscatter in the UV–visible range (Krotkov et al., 2017). We used NASA's L3 tropospheric NO2 column density standard product v3 (OMNO2d_003) and the OMI principal component analysis planetary boundary layer (PBL) SO2 product (OMSO2e_003), which grid retrievals to 0.25∘ resolution (Krotkov et al., 2017; Li et al., 2013). Both products are cloud-screened; only pixels that are at least 70 % cloud-free are included in the NO2 product, and those that are at least 80 % cloud-free are included in the SO2 product. The NO2 product relies on air mass factors (AMFs) calculated with the assistance of an atmospheric chemical transport model and are sensitive to model representations of emission, chemistry, and transport data. Instead of AMFs, the SO2 product uses spectrally dependent SO2 Jacobians but can be interpreted as having a fixed AMF that is representative of summertime conditions. We applied basic transient SO2 plume filtering, excluding retrievals with SO2 > 15 DU (Wang and Wang, 2020).
Because our trend analysis uses a seasonal mean as the response variable, we assume that random errors cancel out, leaving only systematic errors, which do not contribute to uncertainty in the trend analysis. Systematic errors in the OMI NO2 product have an uncertainty of 20 % (McLinden et al., 2014) and are associated with AMFs and tropospheric vertical column contents. The OMI NO2 products use an implicit aerosol correction to account for the optical effects of aerosols, but retrievals can be biased when aerosol loading is extreme (Castellanos et al., 2015). Under these conditions, the OMI NO2 retrieval is biased low by roughly 20 % to 40 % (Chimot et al., 2016). Note that any aerosol-related error would have the potential effect of underestimating the magnitude of decreases in NO2 column densities when comparing 2020 to previous years. Additional bias in the NO2 product may be introduced due to the reliance on nearly cloud-free pixels, in which greater sunlight may induce higher photochemical rates. For example, the current NO2 product is biased roughly 30 % low over the Canadian oil sands (McLinden et al., 2014). The level-2 OMI-NO2 product has been validated against in situ and surface-based observations showing good agreement (Lamsal et al., 2014). The use of fixed Jacobians in the SO2 product introduces systematic errors of 50 % to 100 % for cloud-free observations (Krotkov et al., 2016).
Starting in 2007, the quality of level 1B radiance data for some OMI viewing directions has been affected, known as the row anomaly. The L3 products used here exclude all pixels affected by the row anomaly from each observation, but the locations of the row anomaly pixels were dynamic between 2007 and 2011, which could affect any comparisons including those years. Since 2011, the pixels affected by the row anomaly problem are the same, so comparisons for data only since 2011 are not affected by changes in the row anomaly.
Moderate Resolution Imaging Spectroradiometer (MODIS) sensors observe the Earth from polar orbit, from the Terra satellite since 2000 and from Aqua since mid-2002. In this study we use MODIS-derived AOD at 550 nm obtained by merging Dark Target and Deep Blue retrievals (Sayer et al., 2014). Specifically, we use the Deep_Blue_Aerosol_Optical_Depth_550_Land_Mean field over land and the over ocean AOD_550_Dark_Target_Deep_Blue_Combined_Mean the from Collection 6.1 L3 Gridded products MYD08 and MOD08 (Hubanks et al., 2019), though very few retrievals over ocean are included in our analysis. L3 values are computed on 1∘ × 1∘ spatial grid from L2 AOD products with resolution of 10 km × 10 km. Over land 66 % of MODIS-retrieved Dark Target AOD values were shown to be ± 0.05 ± 0.15*AOD AErosol RObotic NETwork (AERONET)-observed values, with high correlation (R = 0.9) (Levy et al., 2010). Around 78 % of the Deep Blue retrievals are within the expected error range of ± 0.05± 0.20*AOD (Sayer et al., 2013). MODIS AOD data have been extensively used by the modeling and remote sensing scientific communities and inter-compared with a wide range of satellite AOD products (see Schutgens et al., 2020, and references therein).
We analyzed these retrievals over two large regions (Fig. 1). Central east China was comprised of Shaanxi, Hubei, Anhui, Jiangsu, Shanxi, Henan, Hebei, Shandong, Beijing, and Tianjin provinces. Southern China was comprised of Guizhou, Guangxi, Hunan, Jiangxi, Guangdong, Fujian, and Zhejiang provinces. Daily mean quantities were calculated across all valid retrievals falling within the provinces comprising the regions. For the OMI NO2 columns, individual retrievals were weighted by the L3 “weight” field, which is proportional to the fraction of the grid cell with higher-quality retrievals, identified as those have less than 30 % cloud fraction and not affected by the row anomaly problem. We also calculated the daily value from the median of all retrievals to understand whether individual high values (mainly SO2) had any effect on the significance of trends or differences between 2020 and different background periods. Monthly averages were calculated from the daily regional averages, with each day weighted in the monthly average by the number of valid retrievals so as to not overrepresent days with little satellite coverage or significant cloud cover. The monthly data were used to visually identify COVID-19-related changes against background seasonality and trends since 2005.
We examined the difference in the distribution of daily data during the 23 January to 8 April 2020 lockdown period to the same period during previous years since 2005. We compared 2020 to 2019 to different background periods and to the expected value for 2020 estimated from trends over different background periods. Given the uneven nature of changes in atmospheric composition over different parts of China identified in previous studies, background periods were defined for each possible starting year between 2005 and 2018, with each ending in 2019. Retrieved quantities in 2020 were compared to the background means over each period and to the value expected for 2020 estimated from the linear trend over each period. We tested the significance of these differences using bootstrap resampling (Efron and Gong, 1983) with a resampling size of 2000.
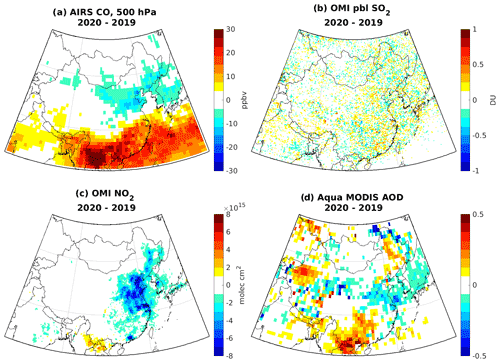
Figure 2The 2020–2019 differences during 23 January to 8 April over China in (a) AIRS carbon monoxide (CO) at 500 hPa, (b) OMI PBL sulfur dioxide (SO2), (c) OMI tropospheric nitrogen dioxide (NO2), and (d) Aqua MODIS aerosol optical depth (AOD).
We also considered how the analysis depended on how the lockdown period was defined. Emissions and pollution can decrease during the Chinese New Year holidays (Chen et al., 2020), which started as early as 23 January in 2012 and as late as 19 February in 2015, complicating COVID-19-related analyses of atmospheric composition over China (Bauwens et al., 2020; Chen et al., 2020). The timing and extent of lockdowns also varied between provinces and we assume that “slowdowns” could have happened before or after stricter, official lockdowns – for example, ground and air transportation remaining below lockdown levels nationally at least through 14 April 2020 (International Energy Agency, 2020). Excluding the holiday period from all years is a straightforward approach to excluding any New Year holiday effects but will exclude simultaneous lockdown effects during the initial, and presumably most strict, stages of the lockdown. Rather than specifying different combinations of New Year holiday period and provincial-level lockdown timing, we used 23 January–8 April as our baseline period (which will include all holiday periods since 2005) but examined the sensitivity of the statistics to the length of the lockdown period, namely a longer lockdown period beginning 1 week earlier and 1 week later, and a shorter lockdown period for February only. In interpreting the data, we put more confidence in 2020 differences that were insensitive to these choices.
3.1 Regional patterns and seasonality
Figure 2 shows the 2020–2019 differences over China during the 23 January–8 April lockdown period for the four satellite-retrieved quantities. There were decreases of 5–10 ppbv in AIRS CO over central east China (Fig. 2a) and increases of 20–25 ppbv over southern China in 2020 compared to 2019. The increase in southern China is adjacent to a stronger positive CO anomaly over the upper Mekong regions of Myanmar, Thailand, and Laos. There were no coherent regional changes in OMI SO2 (Fig. 2b) but rather smaller localized differences of either sign. There were decreases in NO2 (Fig. 2c) across central east China exceeding 8 × 1015 molec cm−2 coincident with the weaker decrease in CO. Over southern China, there were comparable differences over Guangdong province, with smaller differences elsewhere. There was a decrease in MODIS AOD (Fig. 2d) in central east China coincident with the decreases in CO and NO2 but smaller in magnitude. There was a region of higher AOD in and northeast of the upper Mekong region coincident with the CO increase, both presumably because of biomass burning.
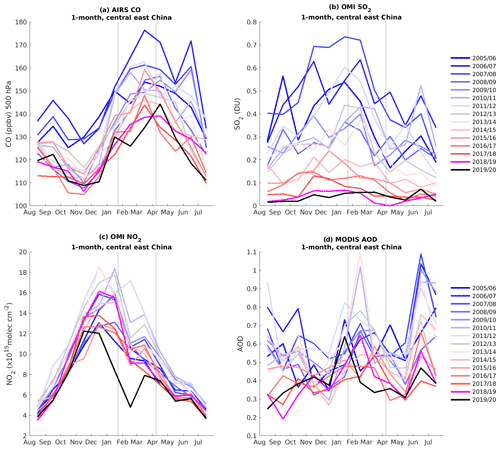
Figure 3Monthly mean (a) AIRS CO, (b) OMI PBL SO2, (c) OMI tropospheric NO2, and (d) MODIS AOD over central east China since 2005. As in Bauwens et al. (2020), each year starts in August to show any departure from the seasonal cycle during the 23 January to 8 April lockdown period, shown by the thin gray vertical lines.
To put the 2020/2019 difference maps in a longer-term and seasonal context, Fig. 3 shows monthly averages of the four retrieved quantities over central east China since 2005. There are seasonal CO peaks in March–April, June, and September, with the minima usually in November and December (Fig. 3a). There has been a decrease since 2005 in CO. The seasonal decrease from January to February in 2020 is similar to that which has occurred occasionally before, but the CO during February and March 2020 was the lowest for that time of the year since 2005. By April, CO had returned to levels typical of 2015–2019. The main characteristics of the monthly SO2 over the region are that it has decreased since 2005 (Fig. 3b) and that early 2020 SO2 was within the range of recent levels. There is a strong seasonal NO2 cycle (Fig. 3c), with a July–August minimum and December–January peak, which has been attributed to increased heating needs (Yu et al., 2017; Si et al., 2019) and longer chemical lifetime owing to lower OH and RO2 (Shah et al., 2020). NO2 has also decreased since 2011, and during most years, there is a departure from a smooth seasonal cycle in January and February associated with the Chinese New Year holiday period. January and February 2020 NO2 was considerably lower than previous years, increased during March, and had recovered to typical, recent levels by April. AOD has consistent seasonal peaks in summer, which have been attributed to hygroscopic growth and agricultural residue burning (Filonchyk et al., 2019), but had less regular seasonality otherwise and has decreased since 2011. AODs during February and particularly March of 2020 were lower than recent years, but during which time there was considerable variability in the monthly data.
Figure 4 shows the four retrieved quantities over southern China. There is a springtime maximum in CO (Fig. 4a), a less regular maximum during September–January, and an annual minimum in July. The range of CO is similar to central east China. CO over the last 5 years is lower than earlier in the record, and early 2020 CO was higher than recent years. SO2 (Fig. 4b) is lower than central east China, and any seasonal cycle is also hard to identify. The high June 2011 values are due to the Nabro eruption in Ethiopia (Fromm et al., 2014) which is still apparent in the time series despite excluding individual SO2 retrievals that are greater than 15 DU and are due to a combination of higher overall background values and individual retrievals with very high (>10 DU) SO2. NO2 (Fig. 4c) is lower than over central east China, but both regions share a similar seasonality. NO2 during January–April 2020 was slightly lower than in 2019. AOD (Fig. 4d) has weak seasonal peaks in October, March, and June; has decreased since 2011; and fell within the range of 2015–2019 in 2020.
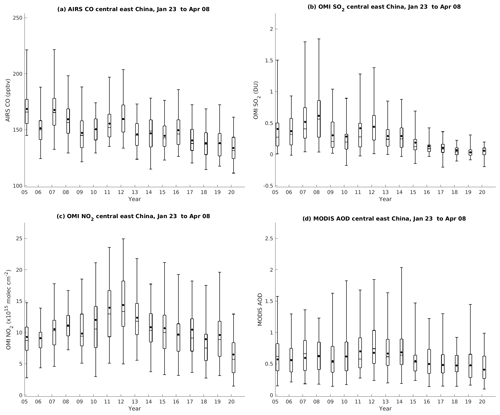
Figure 5The 23 January–8 April box plots over central east China for (a) AIRS CO, (b) OMI PBL SO2, (c) OMI tropospheric NO2, and (d) Aqua and Terra MODIS AOD from 2005 to 2020. The black box plots show the median, interquartile range, and 2.5th and 97.5th percentiles over all daily data, with the mean shown by the black dot.
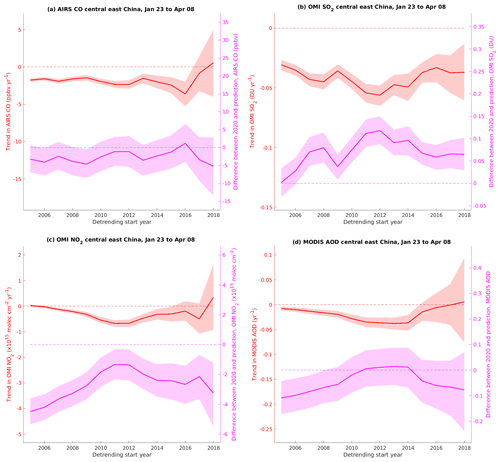
Figure 6Dependence of trends (red) and difference between 2020 observations and predicted value (magenta) on detrending start year over central east China for (a) AIRS CO, (b) OMI PBL SO2, (c) OMI tropospheric NO2, and (d) MODIS AOD. The solid line shows the mean of the estimate for each year and the shading shows the 95 % confidence interval.
3.2 Central east China
Figure 5 shows the CO, SO2, NO2, and AOD for 23 January–8 April of each year over central east China as box-and-whisker plots with the median, interquartile range, and 2.5th and 97.5th percentiles over all daily mean data as horizontal lines and the mean shown by the black dot. The associated statistics comparing 2020 and 2019 are provided in Table 1, and comparing 2020 to longer background periods with and without trends accounted for is shown in Tables S1–S4 in the Supplement. The AIRS CO is shown in Fig. 5a. The variation during 23 January–8 April of each year is due to weather-related factors and observational error. The mean CO of 133.5 ppbv in 2020 was 3.2 % less than the 2019 mean of 137.9 ppbv, which was only marginally significant, having a 95 % confidence interval (−6.3 %–0.1 %) spanning 0. During years prior, there were increases and decreases in CO from year to year, but an overall decreasing trend since 2005. To quantify if the 2020 departure was significant against this background, we compared the distribution of observed 2020 CO to the background average and to that which might be expected given any trends over the background period. Because there was no obvious starting year for the background period, we considered different periods starting in each year between 2005 and 2018 and ending in 2019 (Fig. 6a, Table S1). The difference between 2020 and the background depended strongly on the starting year of the background period, ranging from −11.5 % lower than the 2005–2019 mean to −3.1 % lower than over 2018–2019, but all were statistically significant. Significant trends over years beginning between 2005 and 2016 (shown in Fig. 6a by the red line and shading) ranged between −1.5 ppbv yr−1 when starting in 2013 to −3.6 ppbv yr−1 if starting in 2016. The uncertainty in the trends increased for trends over shorter periods and were, unsurprisingly, insignificant by 2017, with the 95 % confidence intervals of the trends spanning 0. The differences between the observed 2020 mean and the value predicted from the trend (magenta line) varied inversely with the trend and were always negative but, except for 2009, had 95 % confidence intervals (magenta shading) spanning 0 and therefore were not considered significant. Therefore, for CO, 2020 was significantly lower than the background period mean but not consistently lower than predicted given the decreasing trend during the background period, no matter how this period was defined. Results were similar for CO analyzed closer to the surface at 850 hPa (not shown), but where the retrieval has less sensitivity.
OMI SO2 (Fig. 5b) fluctuated over 2005 to 2011 and declined steadily afterward, during which variation also declined, becoming narrower to a degree not seen in the CO. The 2020 mean of 0.057 was 95 % higher than the 2019 mean of 0.031 but with a wide 95 % confidence interval (15 %–250 %). For different background periods (Table S2), 2020 SO2 ranged from 83 % less than the 2005–2019 mean to 30 % less than the 2016–2019 mean, with insignificant differences compared to more recent periods. Trends varied significantly from to −0.03 yr−1 over 2005–2019 to −0.06 DU yr−1 over 2012–2019 (Fig. 6b), during which the trend could explain a maximum of 32 % of the variation in the data. For periods starting in 2007 and after, the observed 2020 mean was significantly higher than predicted. Relative to the value predicted from the 2012–2019 trend of −0.06, the observed 2020 SO2 was 200 % higher; the large percent difference reflects a predicted value close to zero, and we note that the retrieved SO2 can be negative for individual values and averages (Li et al., 2013; Wang and Wang 2020). The observed 2020 SO2 was much higher than expected from trends calculated over 2016–2019 when SO2 was flat and with less variability, but the low SO2 approaching the detection limit over this period makes these estimates not particularly meaningful. Furthermore, the change in 2020 SO2 was strongly dependent on whether daily values were calculated from the mean or median of individual values over the region. For most background periods (Fig. S1b in the Supplement), the trends in the median values were still negative until 2015, but 2020 was only 8.4 % higher than predicted from the 2012–2019 trend and not significantly different from expected for trends beginning later. This likely reflects the greater influence of high individual retrieval values on the daily mean value compared to the median, even after the basic filtering of transient SO2 plumes.
OMI NO2 (Fig. 5c) increased from 2005 to 2011 and decreased thereafter with an apparent flattening since 2016. The 2020 mean NO2 of 6.5 × 1015 molec cm−2 was 32 % less than the 2019 mean of 9.6 × 1015 molec cm−2; the pronounced regional difference between 2020 and 2019 (Figs. 2c and 5c) in part reflects a 2019 uptick from 2018. For different background periods (Table S3), 2020 NO2 ranged from 43.3 % less than the 2010–2019 mean to 30 % less than the 2018–2019 mean, with all differences significant. Trends were negative and significant for starting years between 2007 and 2015 (Fig. 6c), with the strongest trend of −0.7 5 × 1015 for the period beginning in 2011. The 2020 NO2 was significantly less than the predicted value for all background periods but varied from 16.8 % less than predicted from the 2011–2019 trend to 27.1 % less than predicted from the 2015–2019 trend, the last period when there was a significant, although weak, decrease.
MODIS AOD (Fig. 5d) was flat or slightly increasing from 2005 to 2011, decreasing thereafter and with a flattening since 2016 similar to SO2 and NO2. The 2020 mean AOD of 0.41 was 14 % less than the 2019 mean of 0.48, but this was not significant. For different background periods (Table S4), 2020 AOD ranged from 30.2 % less than the 2007–2019 mean to 14.2 % less than the 2018–2019 mean, with confidence intervals for the differences becoming closer to spanning 0 for more recent periods. Trends were negative and significant for starting years between 2005 and 2014 (Fig. 6d), with the strongest decrease of 0.04 yr−1 over the 2012–2019 period. There was no significant difference between the observed and predicted 2020 mean for periods beginning in 2008 and later, when the trends were strongest, and which approached 0 after 2014.
3.3 Southern China
Figure 7 shows the distribution of daily CO, SO2, NO2, and AOD for 23 January–8 April of each year over southern China. The associated statistics comparing 2020 and 2019 are provided in Table 2. AIRS CO (Fig. 7a) in 2020 was 144.7 ppbv, 13 % higher than the 2019 mean of 128.5 ppbv, which can be seen in an upward shift in the distribution of the box plot. The 2020 CO was between 4.4 % and 8.8 % greater than the background mean for periods starting after 2014 (Table S5 in the Supplement) but not significantly different otherwise. CO decreased significantly for periods starting between 2005 and 2016 (Fig. 8a). When these trends are taken into account, 2020 CO was between 11.2 % and 18.7 % greater than predicted, and in all cases these differences were significant.
OMI SO2 (Fig. 7b) fluctuated from 2005 until 2013 and flattened afterwards, driven by fewer high individual SO2 values in later years, as in central east China. The 2020 mean of 0.003 DU was 116 % higher than the 2019 mean of −0.02 DU but also with a wide 95 % confidence interval (24 %–223 %). Year 2020 was less than the background mean periods starting between 2005 and 2011 (Table S6 in the Supplement) but not significantly different otherwise. SO2 trends were consistently negative for all periods (Fig. 8b), although not as strong as over central east China. Whether 2020 SO2 was greater than predicted from trends depended more on the background period than over central east China. Differences in 2020 were also not significantly different from predicted when daily values were calculated from the median SO2 of individual retrievals for any background period (Fig. S2b in the Supplement).
OMI NO2 (Fig. 7c) increased toward 2011 and 2012, declining after to 2005–2010 levels. The 2020 mean of 3.3 × 1015 molec cm−2 was 22 % less than the 2019 mean of 4.3 × 1015 molec cm−2. For longer background periods, 2020 was between 22.9 % and 30.6 % less than the mean (Table S7 in the Supplement), all of which were significant. NO2 trends were significantly negative when the start of the trend was calculated using years between 2007 and 2012 but not otherwise (Fig. 8c). The 2020 NO2 mean was significantly lower than predicted, except for when the trend was estimated beginning in 2011 or 2018. A 2-year trend cannot be interpreted meaningfully, especially without considering meteorological differences. Visually, however, it is hard to tell if the 2020 NO2 distribution represents a COVID-related departure or a decrease comparable to changes during recent previous years, unlike over central east China.
MODIS AOD (Fig. 7d) was comparable to NO2 in its increase toward 2012, decrease thereafter, and flattening during more recent years. The 2020 mean AOD of 0.38 was 12 % higher than the 2019 mean of 0.34 but with a 95 % confidence interval (−7 %–34 %) spanning 0. Similarly, 2020 was between 14 % and 22 % lower than during background periods beginning from 2005 to 2012 but not for more recent periods (Table S8 in the Supplement). The AOD trends were significantly negative for all start years until 2015. The 2020 mean was between 32 % and 47 % higher than predicted from trends for periods starting between 2010 and 2015 but was not different from predicted for trends starting in other years.
For both regions and all quantities, the differences between observed and predicted values for 2020 were insensitive to a longer lockdown period or to whether the bootstrap resampling was weighted by the number of valid retrievals each day. For a February-only lockdown period (Figs. S3 and S4 in the Supplement), the CO trends were more significant when starting in later years, but the differences between the observed and expected values remained insignificant over central east China. The SO2 trends for different periods were similar. The 2020 SO2 differences from what would be expected approached 0 for later periods but were also not consistently different when the median values of individual retrievals were used. Results for NO2 were unaffected. The AOD 2020 difference from what would be expected was stronger and technically significant but still with a very wide confidence interval and therefore difficult to interpret. We emphasize that while a February-only lockdown period is useful for comparison, it is problematic in not including the New Year's holiday periods from all previous years.
The degree to which the COVID-19 lockdowns in China resulted in changes in atmospheric composition depended strongly on the background period and whether existing trends were taken into account. For AIRS CO over central east China, the 2020 mean was 3 %–12 % lower compared to different background periods. Relative to mean CO concentrations during periods beginning between 2005 and 2016, there were significant decreases in CO but CO in 2020 was not consistently different from what would be expected from trends calculated over this period. These longer-term declines in CO concentrations do appear to flatten out in recent years; assuming that the flat CO during 2017–2019 would have persisted, we estimate a 3 %–4 % reduction in CO in 2020 relative to that period. For MODIS AOD, the 2020 mean was between 14 % and 30 % less than different background averages but not significantly different from what would be expected for trends beginning between 2008 and 2014. As with CO trends, the negative AOD trends in the region also appear to flatten in recent years. Relative to the flat AOD over 2016–2019, 2020 AOD was 14 %–17 % lower than the background mean; as with CO, this range would be the more meaningful estimate of changes in 2020 if we assume that this flattening were to persist. The 2020 SO2 was significantly lower than background averages calculated over most periods, ranging from 83 % less than over 2005–2019 to 30 % less than over 2016–2019. Compared to the 2012–2019 period when there were no significant SO2 increases, 2020 SO2 was 200 % greater than what would be expected based on a trend starting in 2021, only 8 % greater when the median of daily retrievals was used, and not significantly different from expected relative to the trends beginning later than 2012. SO2 concentrations were relatively flat from 2016–2019; when using 2016 as the first year of the trend, SO2 was significantly higher than the expected value when calculated from the mean of the daily SO2 retrievals but not significantly different when calculated from the median. We note also that analyses of SO2 and NO2 that include years prior to 2012 may be affected by changes in observation sample size due to changes in the OMI row anomaly.
OMI NO2 in 2020 over central east China was consistently lower than the background average and expected value from the trends. There was a 17 % decrease in 2020 relative to the value expected from a trend calculated over 2011–2019 but a 30 %–33 % decrease relative to the different background means since 2016 when the NO2 was relatively flat. Again assuming that this flattening were to persist, this latter range may be the more meaningful baseline for the 2020 decrease. For reference, Bauwens et al. (2020) reported a ∼ 40 % drop in OMI NO2 from 2019 to 2020 over cities affected by the lockdown using the QA4ECV retrieval (Boersma et al., 2018) and a ∼ 51 % drop in NO2 over the eight cities (Beijing, Jinan, Nanjing, Qingdao, Tianjin, Wuhan, Xi'an, and Zhengzhou) falling within our central east China region. Our analysis cannot be compared directly because we include non-urban areas and define the lockdown period differently, but we do note that NO2 during the same period in 2019 appeared to be anomalously high relative to the previous few years, which would make the decreases in 2020 appear more significant.
The modest decreases in CO and AOD over central east China were unexpected; given its high population density and level of industrial activity, lockdowns may have been anticipated to lead to larger decreases. In the case of MODIS AOD, these modest decreases were possibly due to contributions from other sources unaffected by COVID-19-related lockdowns – limitations in the MODIS AOD retrieval under cloudy conditions, climatological variability from other sources such as mineral dust, and meteorology favorable to secondary aerosol formation which could have offset lower emissions (Wang et al., 2020). The 2020 increase in SO2 is more difficult to interpret because of the discrepancies between daily values calculated from the mean or median of individual retrievals but is broadly consistent with surface observations that find no significant change in in situ surface SO2 over Wuhan in the daily mean and a slight increase in daytime SO2 possibly associated with increased residential heating and cooking (Shi and Brasseur, 2020).
Over southern China, retrieved 2020 SO2 was significantly lower than the background average only for periods beginning between 2005 and 2011. Significant departures from expected trends were uneven when using the mean value of daily retrievals and absent when using the median value. As with central east China, we conclude that no significant changes could be robustly detected in 2020 SO2. NO2 in 2020 was between 23 % and 32 % less than the background average for different periods. Here, the flattening in NO2 beginning in 2013 is easier to identify than over central east China because of the much higher NO2 during the 3 years prior; 2020 NO2 was 23 %–27 % less than different background means between 2013 and 2019. The more significant reductions in NO2 in central east China compared to the south is presumably due the former's greater population and industrialization and consequently higher pollution levels. This is consistent with Chen et al.'s (2020) detection of a larger 2020 decrease in surface NO2 in Wuhan compared to Shanghai. Retrieved CO in 2020 was between 4 % and 8 % greater than background averages beginning in 2014 but between 11 % and 19 % higher than what would be expected given the decreasing trends over any period. AOD in 2020 was lower than background averages calculated starting with years earlier than 2012 but higher or not significantly different from expected for trends calculated starting in years after 2012.
The focus of this analysis is on whether satellite retrievals of atmospheric composition over 2020 departed significantly from different background periods and expected values for 2020 when daily variability and trends are accounted for, but it is useful at a preliminary stage to speculate as to how different emission changes could have contributed to (1) why NO2 was robustly lower in 2020 over central east China compared to CO and AOD and (2) why CO and perhaps AOD were higher over southern China compared to what would be expected from recent trends.
Table 3The 2014 anthropogenic emission estimates by sector (in %) over China, excluding biomass burning, from the Community Emissions Data System (CEDS) for a representative set of constituents: black carbon (BC), carbon monoxide (CO), ammonia (NH3), nitrogen oxides (NOx), organic carbon (OC), and sulfur dioxide (SO2). Residential, commercial, and other sectors are combined as RCO.
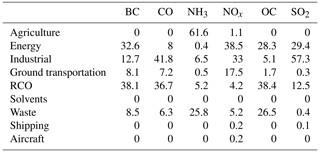
To understand why NO2 differences over central east China were more significant than other quantities, Table 3 shows the emissions by sector for a representative set of constituents from the Community Emissions Data System (CEDS) (Hoesly et al., 2018) over China for 2014, the most recent year available. Other bottom-up emission inventories will vary in absolute emission amounts and their sector contributions, particularly for more recent periods, but CEDS is the standard available emission dataset available globally as a baseline for the next Intergovernmental Panel on Climate Change (IPCC) assessment, in anticipation of assessing 2020 COVID-19-related changes to atmospheric composition in other regions, and for modeling studies involving a transboundary transport component. Across all species, energy production, industrial activity, transportation, residential/commercial/other (RCO), and waste disposal constitute the bulk of the emissions. Based on activity data for the first quarter of 2020, energy demand across China declined by 7 % compared to 2019, and transportation sector activity declined by 50 % to 75 % in regions with lockdowns in place (International Energy Agency, 2020). These sectors are direct or indirect sources of numerous pollutants, including SO2 (the precursor of sulfate aerosol), NOx, CO, and primary anthropogenic aerosols classified broadly as organic carbon (OC) and black carbon (BC). If we apply the 7 % reduction in energy production and midpoint 62.5 % reduction to transportation from the International Energy Agency (IEA) and assume a 20 % reduction in industrial emissions, 5 % reduction in waste emissions, and no change in RCO (with commercial decreases offset by residential increases), this yields a 10 % reduction in BC, 5 % reduction in OC, 14 % reduction in SO2, 14 % reduction in CO, and 21 % reduction in NO2. The larger reduction in NO2 relative to other emissions could partly explain why OMI NO2 column density changes over central east China were stronger than in the other retrievals.
Table 4Bottom-up biomass Global Fire Assimilation System (Kaiser et al., 2012) burning CO emission estimates from the upper Mekong region (17 to 24∘ N, 95 to 105∘ E) and AIRS CO over southern China from 23 January to 8 April, for 2005–2020.
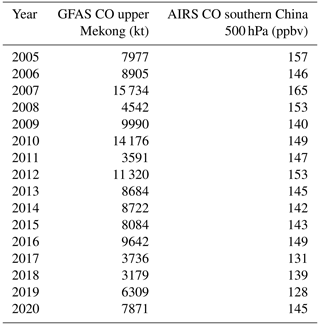
Following Si et al.'s (2019) consideration of biomass burning as a pollution source in China alongside anthropogenic sources, we considered transboundary smoke transport as a possible reason for the higher 2020 CO over southern China, guided by higher CO over the upper Mekong region in 2020 compared to 2019 (Fig. 2a) and the predominant westerly flow during this time of year (Reid et al., 2013). Table 4 compares 23 January–8 April AIRS CO over southern China to CO emission estimates from biomass burning from the Global Fire Assimilation System (GFAS) (Kaiser et al., 2012) over the upper Mekong region (17 to 25∘ N, 95 to 105∘ E) including parts of eastern Myanmar, northern Thailand, and northern Laos. From 2005 to 2020, variation in GFAS CO over this region explained a moderate (32 %) amount of variability in AIRS CO over southern China, suggesting it is a non-negligible contributor to variation in CO concentration and a contributor to higher CO in 2020. This illustrates that, at a minimum, sources such as biomass burning smoke and dust that are less affected by COVID-19-related measures will complicate attribution studies. To that end, modeling studies following Wang et al. (2020) will be required to isolate emissions, meteorological and chemical drivers of changes in atmospheric composition, and their effects at a process level. With proper instrument-equivalent comparisons, modeling studies will also help to identify the extent to which the lack of significant changes are due to retrieval limitations, namely low sensitivity near the surface where differences would presumably be more pronounced, particularly given remote emission sources such as dust, biomass burning smoke, and volcanic SO2, which will arrive at higher altitudes.
The key implication of our study is that interpreting differences in 2020 retrievals of atmospheric composition depends strongly on how the background period is defined and whether trends over these periods are accounted for. Not taking these into account could lead to misattribution of changes in air quality to COVID-19 lockdowns. At a minimum, whether differences in 2020 are significant depends on the choice of background period, which is somewhat subjective. Leading up to 2020, there was an apparent flattening of decreasing trends beginning earlier in the decade across the retrievals; the considerable variability in the data made identifying this flattening easier in some cases than in others. We are more confident, for example, in our estimate of a 23 %–27 % decrease in 2020 NO2 over southern China relative to a flat background period than the 30 %–33 % decrease over central east China, where the recent variability was greater and the flattening less apparent. Revisiting this type of analysis in the years when regional economies have fully recovered post COVID-19 will help to distinguish between further decreases and flat trends and will lend themselves to using non-linear models in estimating the trends. We have approached the issue by comparing data for 2020 to what would have been expected given recent trends and by applying a single lockdown period to two large regions, with additional analyses to gauge the sensitivity of the 2020 differences to these choices. Other studies over China or elsewhere will inevitably use other approaches that more explicitly account for seasonality and meteorology, and which relate changes in pollution over smaller areas (e.g., single provinces or states) to region-specific lockdown measures and timing at a process level. Regardless of the approach, however, it is important to consider recent trends and variability. In places where pollution has decreased, not accounting for recent context could result in over-attribution of changes in pollution to COVID-19. In places where pollution has increased, such as parts of South Asia, this could result in under-attribution.
The MATLAB code used to process the satellite data is provided in the Supplement. The OMI OMSO2e_003 SO2 (Li et al., 2013) and OMNO2d_003 NO2 (Krotkov et al., 2017) data were retrieved from the Goddard Earth Sciences Data and Information Services Center (GES DISC) at https://acdisc.gesdisc.eosdis.nasa.gov/data/Aura_OMI_Level3/ (last access: 29 January 2021), as was the AIRS AIRS3STD.006 CO data (Warner et al., 2013) from https://acdisc.gesdisc.eosdis.nasa.gov/data/Aqua_AIRS_Level3/ (last access: 29 January 2021).
The MODIS MOD08_D3 and MYD08_D3 AOD data (Sayer et al., 2014) were retrieved from the Level-1 and Atmosphere Archive and Distribution System (LAADS) Distributed Active Archive Center (DAAC) at https://ladsweb.modaps.eosdis.nasa.gov/archive/allData/61/ (last access: 29 January 2021).
The supplement related to this article is available online at: https://doi.org/10.5194/acp-21-18333-2021-supplement.
All authors conceived of the study. RDF, IVG, and KT conducted the data analysis. RDF and JEH prepared the manuscript with contributions from all co-authors.
The contact author has declared that neither they nor their co-authors have any competing interests.
Publisher's note: Copernicus Publications remains neutral with regard to jurisdictional claims in published maps and institutional affiliations.
The authors thank two anonymous reviewers and the editor for their constructive feedback, which improved the interpretation of the data and conclusions drawn in the paper.
This research has been supported by the NASA (grant no. 80NSSC18M0133).
This paper was edited by Michel Van Roozendael and reviewed by two anonymous referees.
Bauwens, M., Compernolle, S., Stavrakou, T., Müller, J., van Gent, J., Eskes, H., Levelt, P. F., van der A., R., Veefkind, J. P., Vlietinck., J., Yu, H., and Zehner, C.: Impact of coronavirus outbreak on NO2 pollution assessed using TROPOMI and OMI observations, Geophys. Res. Lett., 2, 0–3, https://doi.org/10.1029/2020GL087978, 2020.
Boersma, K. F., Eskes, H. J., Richter, A., De Smedt, I., Lorente, A., Beirle, S., van Geffen, J. H. G. M., Zara, M., Peters, E., Van Roozendael, M., Wagner, T., Maasakkers, J. D., van der A, R. J., Nightingale, J., De Rudder, A., Irie, H., Pinardi, G., Lambert, J.-C., and Compernolle, S. C.: Improving algorithms and uncertainty estimates for satellite NO2 retrievals: results from the quality assurance for the essential climate variables (QA4ECV) project, Atmos. Meas. Tech., 11, 6651–6678, https://doi.org/10.5194/amt-11-6651-2018, 2018.
Castellanos, P., Boersma, K. F., Torres, O., and de Haan, J. F.: OMI tropospheric NO2 air mass factors over South America: effects of biomass burning aerosols, Atmos. Meas. Tech., 8, 3831–3849, https://doi.org/10.5194/amt-8-3831-2015, 2015.
Chen, K., Wang, M., Huang, C., Kinney, P. L., and Paul, A. T.: Air Pollution Reduction and Mortality Benefit during the COVID-19 Outbreak in China, Lancet Planetary Health, 4, E210–E212, https://doi.org/10.1016/S2542-5196(20)30107-8, 2020.
Chimot, J., Vlemmix, T., Veefkind, J. P., de Haan, J. F., and Levelt, P. F.: Impact of aerosols on the OMI tropospheric NO2 retrievals over industrialized regions: how accurate is the aerosol correction of cloud-free scenes via a simple cloud model?, Atmos. Meas. Tech., 9, 359–382, https://doi.org/10.5194/amt-9-359-2016, 2016.
Efron, B. and Gong, G.: A Leisurely Look at the Bootstrap, the Jacknife, and Cross-Validation, Am. Stat., 37, 36–48, https://doi.org/10.2307/2685844, 1983.
Filonchyk, M., Yan, H. W., and Zhang, Z. R.: Analysis of spatial and temporal variability of aerosol optical depth over China using MODIS combined Dark Target and Deep Blue product, Theor. Appl. Climatol., 137, 2271–2288, https://doi.org/10.1007/s00704-018-2737-5, 2019.
Fioletov, V. E., McLinden, C. A., Krotkov, N., Li, C., Joiner, J., Theys, N., Carn, S., and Moran, M. D.: A global catalogue of large SO2 sources and emissions derived from the Ozone Monitoring Instrument, Atmos. Chem. Phys., 16, 11497–11519, https://doi.org/10.5194/acp-16-11497-2016, 2016.
Fromm, M., Kablick, G., Nedoluha, G., Carboni, E., Grainger, R., Campbell, J., and Lewis, J.: Correcting the record of volcanic stratospheric aerosol impact: Nabro and Sarychev Peak, J. Geophys. Res.-Atmos., 119, 10343–10364, https://doi.org/10.1002/2014jd021507, 2014.
Geddes, J. A., Martin, R. V., Boys, B. L., and van Donkelaar, A.: Long-Term Trends Worldwide in Ambient NO2 Concentrations Inferred from Satellite Observations, Environ. Health Persp., 124, 281–289, https://doi.org/10.1289/ehp.1409567, 2016.
Georgoulias, A. K., van der A, R. J., Stammes, P., Boersma, K. F., and Eskes, H. J.: Trends and trend reversal detection in 2 decades of tropospheric NO2 satellite observations, Atmos. Chem. Phys., 19, 6269–6294, https://doi.org/10.5194/acp-19-6269-2019, 2019.
Han, H., Liu, J., Yuan, H. L., Jiang, F., Zhu, Y., Wu, Y., Wang, T. J., and Zhuang, B. L.: Impacts of Synoptic Weather Patterns and their Persistency on Free Tropospheric Carbon Monoxide Concentrations and Outflow in Eastern China, J. Geophys. Res.-Atmos., 123, 7024–7046, https://doi.org/10.1029/2017jd028172, 2018.
He, Q. Q., Gu, Y. F., and Zhang, M.: Spatiotemporal patterns of aerosol optical depth throughout China from 2003 to 2016, Sci. Total Environ., 653, 23–35, https://doi.org/10.1016/j.scitotenv.2018.10.307, 2019.
Hoesly, R. M., Smith, S. J., Feng, L., Klimont, Z., Janssens-Maenhout, G., Pitkanen, T., Seibert, J. J., Vu, L., Andres, R. J., Bolt, R. M., Bond, T. C., Dawidowski, L., Kholod, N., Kurokawa, J.-I., Li, M., Liu, L., Lu, Z., Moura, M. C. P., O'Rourke, P. R., and Zhang, Q.: Historical (1750–2014) anthropogenic emissions of reactive gases and aerosols from the Community Emissions Data System (CEDS), Geosci. Model Dev., 11, 369–408, https://doi.org/10.5194/gmd-11-369-2018, 2018.
Hubanks, P., Platnick, S., King, M., and Ridgway, B.: MODIS Algorithm Theoretical Basis Document No. ATBD-MOD-30 for Level-3 Global Gridded Atmosphere Products (08_D3, 08_E3, 08_M3) and Users Guide (Collection 6.0 & 6.1, Version 4.4, 20 Feb 2019), NASA Goddard Space Flight Center, Greenbelt, MD, 2019.
International Energy Agency: Global Energy Review 2020: The impacts of the Covid-19 crisis on global energy demand and CO2 emissions, 2020, available at: https://www.iea.org/reports/global-energy-review-2020, last access: 4 May 2020.
Kaiser, J. W., Heil, A., Andreae, M. O., Benedetti, A., Chubarova, N., Jones, L., Morcrette, J.-J., Razinger, M., Schultz, M. G., Suttie, M., and van der Werf, G. R.: Biomass burning emissions estimated with a global fire assimilation system based on observed fire radiative power, Biogeosciences, 9, 527–554, https://doi.org/10.5194/bg-9-527-2012, 2012.
Krotkov, N. A., McLinden, C. A., Li, C., Lamsal, L. N., Celarier, E. A., Marchenko, S. V., Swartz, W. H., Bucsela, E. J., Joiner, J., Duncan, B. N., Boersma, K. F., Veefkind, J. P., Levelt, P. F., Fioletov, V. E., Dickerson, R. R., He, H., Lu, Z., and Streets, D. G.: Aura OMI observations of regional SO2 and NO2 pollution changes from 2005 to 2015, Atmos. Chem. Phys., 16, 4605–4629, https://doi.org/10.5194/acp-16-4605-2016, 2016.
Krotkov, N. A., Lamsal, L. N., Celarier, E. A., Swartz, W. H., Marchenko, S. V., Bucsela, E. J., Chan, K. L., Wenig, M., and Zara, M.: The version 3 OMI NO2 standard product, Atmos. Meas. Tech., 10, 3133–3149, https://doi.org/10.5194/amt-10-3133-2017, 2017.
Lamsal, L. N., Krotkov, N. A., Celarier, E. A., Swartz, W. H., Pickering, K. E., Bucsela, E. J., Gleason, J. F., Martin, R. V., Philip, S., Irie, H., Cede, A., Herman, J., Weinheimer, A., Szykman, J. J., and Knepp, T. N.: Evaluation of OMI operational standard NO2 column retrievals using in situ and surface-based NO2 observations, Atmos. Chem. Phys., 14, 11587–11609, https://doi.org/10.5194/acp-14-11587-2014, 2014.
Levy, R. C., Remer, L. A., Kleidman, R. G., Mattoo, S., Ichoku, C., Kahn, R., and Eck, T. F.: Global evaluation of the Collection 5 MODIS dark-target aerosol products over land, Atmos. Chem. Phys., 10, 10399–10420, https://doi.org/10.5194/acp-10-10399-2010, 2010.
Li, C., Joiner, J., Krotkov, N. A., and Bhartia, P. K.: A fast and sensitive new satellite SO2 retrieval algorithm based on principal component analysis: Application to the ozone monitoring instrument, Geophys. Res. Lett., 40, 6314–6318, https://doi.org/10.1002/2013gl058134, 2013.
Li, M., Zhang, Q., Kurokawa, J.-I., Woo, J.-H., He, K., Lu, Z., Ohara, T., Song, Y., Streets, D. G., Carmichael, G. R., Cheng, Y., Hong, C., Huo, H., Jiang, X., Kang, S., Liu, F., Su, H., and Zheng, B.: MIX: a mosaic Asian anthropogenic emission inventory under the international collaboration framework of the MICS-Asia and HTAP, Atmos. Chem. Phys., 17, 935–963, https://doi.org/10.5194/acp-17-935-2017, 2017.
Lin, C. Q., Liu, G., Lau, A. K. H., Li, Y., Li, C. C., Fung, J. C. H., and Lao, X. Q.: High-resolution satellite remote sensing of provincial PM2.5 trends in China from 2001 to 2015, Atmos. Environ., 180, 110–116, https://doi.org/10.1016/j.atmosenv.2018.02.045, 2018.
Lin, N., Wang, Y. X., Zhang, Y., and Yang, K.: A large decline of tropospheric NO2 in China observed from space by SNPP OMPS, Sci. Total Environ., 675, 337–342, https://doi.org/10.1016/j.scitotenv.2019.04.090, 2019.
Luan, Y. and Jaeglé, L.: Composite study of aerosol export events from East Asia and North America, Atmos. Chem. Phys., 13, 1221–1242, https://doi.org/10.5194/acp-13-1221-2013, 2013.
Ma, Z. W., Hu, X. F., Sayer, A. M., Levy, R., Zhang, Q., Xue, Y. G., Tong, S. L., Bi, J., Huang, L., and Liu, Y.: Satellite-Based Spatiotemporal Trends in PM2.5 Concentrations: China, 2004–2013, Environ. Health Persp., 124, 184–192, https://doi.org/10.1289/ehp.1409481, 2016.
McLinden, C. A., Fioletov, V., Boersma, K. F., Kharol, S. K., Krotkov, N., Lamsal, L., Makar, P. A., Martin, R. V., Veefkind, J. P., and Yang, K.: Improved satellite retrievals of NO2 and SO2 over the Canadian oil sands and comparisons with surface measurements, Atmos. Chem. Phys., 14, 3637–3656, https://doi.org/10.5194/acp-14-3637-2014, 2014.
Mijling, B., van der A, R. J., Boersma, K. F., Van Roozendael, M., De Smedt, I., and Kelder, H. M.: Reductions of NO2 detected from space during the 2008 Beijing Olympic Games, Geophys. Res. Lett., 36, L13801, https://doi.org/10.1029/2009gl038943, 2009.
Reid, J. S., Hyer, E. J., Johnson, R. S., Holben, B. N., Yokelson, R. J., Zhang, J. L., Campbell, J. R., Christopher, S. A., Di Girolamo, L., Giglio, L., Holz, R. E., Kearney, C., Miettinen, J., Reid, E. A., Turk, F. J., Wang, J., Xian, P., Zhao, G. Y., Balasubramanian, R., Chew, B. N., Janjai, S., Lagrosas, N., Lestari, P., Lin, N. H., Mahmud, M., Nguyen, A. X., Norris, B., Oanh, N. T. K., Oo, M., Salinas, S. V., Welton, E. J., and Liew, S. C.: Observing and understanding the Southeast Asian aerosol system by remote sensing: An initial review and analysis for the Seven Southeast Asian Studies (7SEAS) program, Atmos. Res., 122, 403–468, https://doi.org/10.1016/j.atmosres.2012.06.005, 2013.
Sarkodie, S. A., and Strezov, V.: A review on Environmental Kuznets Curve hypothesis using bibliometric and meta-analysis, Sci. Total Environ., 649, 128–145, https://doi.org/10.1016/j.scitotenv.2018.08.276, 2019.
Sayer, A. M., Hsu, N. C., Bettenhausen, C., and Jeong, M. J.: Validation and uncertainty estimates for MODIS Collection 6 “Deep Blue” aerosol data, J. Geophys. Res.-Atmos., 118, 7864–7872, https://doi.org/10.1002/jgrd.50600, 2013.
Sayer, A. M., Munchak, L. A., Hsu, N. C., Levy, R. C., Bettenhausen, C., and Jeong, M. J.: MODIS Collection 6 aerosol products: Comparison between Aqua's e-Deep Blue, Dark Target, and “merged” data sets, and usage recommendations, J. Geophys. Res.-Atmos., 119, 13965–13989, https://doi.org/10.1002/2014jd022453, 2014.
Schutgens, N., Sayer, A. M., Heckel, A., Hsu, C., Jethva, H., de Leeuw, G., Leonard, P. J. T., Levy, R. C., Lipponen, A., Lyapustin, A., North, P., Popp, T., Poulsen, C., Sawyer, V., Sogacheva, L., Thomas, G., Torres, O., Wang, Y., Kinne, S., Schulz, M., and Stier, P.: An AeroCom–AeroSat study: intercomparison of satellite AOD datasets for aerosol model evaluation, Atmos. Chem. Phys., 20, 12431–12457, https://doi.org/10.5194/acp-20-12431-2020, 2020.
Selden, T. M. and Song, D. Q.: Environmental Quality and Development – is there a Kuznets Curve for Air-Pollution Emissions?, J. Enviro. Econ. Manag., 27, 147–162, https://doi.org/10.1006/jeem.1994.1031, 1994.
Shah, V., Jacob, D. J., Li, K., Silvern, R. F., Zhai, S., Liu, M., Lin, J., and Zhang, Q.: Effect of changing NOx lifetime on the seasonality and long-term trends of satellite-observed tropospheric NO2 columns over China, Atmos. Chem. Phys., 20, 1483–1495, https://doi.org/10.5194/acp-20-1483-2020, 2020.
Shao, P. Y., Tian, H. Z., Sun, Y. J., Liu, H. J., Wu, B. B., Liu, S. H., Liu, X. Y., Wu, Y. M., Liang, W. Z., Wang, Y., Gao, J. J., Xue, Y. F., Bai, X. X., Liu, W., Lin, S. M., and Hu, G. Z.: Characterizing remarkable changes of severe haze events and chemical compositions in multi-size airborne particles (PM1, PM2.5 and PM10) from January 2013 to 2016-2017 winter in Beijing, China, Atmos. Environ., 189, 133–144, https://doi.org/10.1016/j.atmosenv.2018.06.038, 2018.
Shi, X. and Brasseur, G. P.: The Response in Air Quality to the Reduction of Chinese Economic Activities during the COVID-19 Outbreak, Geophys. Res. Lett., 47, e2020GL088070, https://doi.org/10.1029/2020GL088070, 2020.
Si, Y. D., Wang, H. M., Cai, K., Chen, L. F., Zhou, Z. C., and Li, S. S.: Long-term (2006-2015) variations and relations of multiple atmospheric pollutants based on multi-remote sensing data over the North China Plain, Environ. Pollut., 255, 113323, https://doi.org/10.1016/j.envpol.2019.113323, 2019.
Sogacheva, L., Popp, T., Sayer, A. M., Dubovik, O., Garay, M. J., Heckel, A., Hsu, N. C., Jethva, H., Kahn, R. A., Kolmonen, P., Kosmale, M., de Leeuw, G., Levy, R. C., Litvinov, P., Lyapustin, A., North, P., Torres, O., and Arola, A.: Merging regional and global aerosol optical depth records from major available satellite products, Atmos. Chem. Phys., 20, 2031–2056, https://doi.org/10.5194/acp-20-2031-2020, 2020.
Strode, S. A., Worden, H. M., Damon, M., Douglass, A. R., Duncan, B. N., Emmons, L. K., Lamarque, J.-F., Manyin, M., Oman, L. D., Rodriguez, J. M., Strahan, S. E., and Tilmes, S.: Interpreting space-based trends in carbon monoxide with multiple models, Atmos. Chem. Phys., 16, 7285–7294, https://doi.org/10.5194/acp-16-7285-2016, 2016.
Sun, W., Shao, M., Granier, C., Liu, Y., Ye, C. S., and Zheng, J. Y.: Long-Term Trends of Anthropogenic SO2, NOx, CO, and NMVOCs Emissions in China, Earths Future, 6, 1112–1133, https://doi.org/10.1029/2018ef000822, 2018.
United Nations Environment Program (UNEP): Independent Environmental Assessment: Beijing 2008 Olympic Games, Nairobi, Kenya, 2009.
Wang, M., Zhu, T., Zheng, J., Zhang, R. Y., Zhang, S. Q., Xie, X. X., Han, Y. Q., and Li, Y.: Use of a mobile laboratory to evaluate changes in on-road air pollutants during the Beijing 2008 Summer Olympics, Atmos. Chem. Phys., 9, 8247–8263, https://doi.org/10.5194/acp-9-8247-2009, 2009.
Wang, P., Chen, K., Zhu, S., Wang, P., and Zhang, H.: Severe air pollution events not avoided by reduced anthropogenic activities during COVID-19 outbreak, Resources, Conserv. Recycling, 158, 104814, https://doi.org/10.1016/j.resconrec.2020.104814, 2020.
Wang, P. C., Elansky, N. F., Timofeev, Y. M., Wang, G. C., Golitsyn, G. S., Makarova, M. V., Rakitin, V. S., Shtabkin, Y., Skorokhod, A. I., Grechko, E. I., Fokeeva, E. V., Safronov, A. N., Ran, L., and Wang, T.: Long-Term Trends of Carbon Monoxide Total Columnar Amount in Urban Areas and Background Regions: Ground- and Satellite-based Spectroscopic Measurements, Adv. Atmos. Sci., 35, 785–795, https://doi.org/10.1007/s00376-017-6327-8, 2018.
Wang, T., Nie, W., Gao, J., Xue, L. K., Gao, X. M., Wang, X. F., Qiu, J., Poon, C. N., Meinardi, S., Blake, D., Wang, S. L., Ding, A. J., Chai, F. H., Zhang, Q. Z., and Wang, W. X.: Air quality during the 2008 Beijing Olympics: secondary pollutants and regional impact, Atmos. Chem. Phys., 10, 7603–7615, https://doi.org/10.5194/acp-10-7603-2010, 2010.
Wang, Y., and Wang, J.: Tropospheric SO2 and NO2 in 2012–2018: Contrasting views of two sensors (OMI and OMPS) from space, Atmos. Environ., 223, 117214, https://doi.org/10.1016/j.atmosenv.2019.117214, 2020.
Warner, J., Carminati, F., Wei, Z., Lahoz, W., and Attié, J.-L.: Tropospheric carbon monoxide variability from AIRS under clear and cloudy conditions, Atmos. Chem. Phys., 13, 12469–12479, https://doi.org/10.5194/acp-13-12469-2013, 2013.
Witte, J. C., Schoeberl, M. R., Douglass, A. R., Gleason, J. F., Krotkov, N. A., Gille, J. C., Pickering, K. E., and Livesey, N.: Satellite observations of changes in air quality during the 2008 Beijing Olympics and Paralympics, Geophys. Res. Lett., 36, L17803, https://doi.org/10.1029/2009gl039236, 2009.
Xie, G. Q., Wang, M., Pan, J., and Zhu, Y.: Spatio-temporal variations and trends of MODIS C6.1 Dark Target and Deep Blue merged aerosol optical depth over China during 2000-2017, Atmos. Environ., 214, 116846, https://doi.org/10.1016/j.atmosenv.2019.116846, 2019.
Xu, J. H., Xie, H. M., Wang, K., Wang, J., and Xia, Z. S.: Analyzing the spatial and temporal variations in tropospheric NO2 column concentrations over China using multisource satellite remote sensing, J. Appl. Remote Sens., 14, 014519, https://doi.org/10.1117/1.jrs.14.014519, 2020.
Yu, S. M., Yuan, J. G., and Liang, X. Y.: Trends and Spatiotemporal Patterns of Tropospheric NO2 over China During 2005–2014, Water Air Soil Poll., 228, 447, https://doi.org/10.1007/s11270-017-3641-9, 2017.
Yumimoto, K., Uno, I., and Itahashi, S.: Long-term inverse modeling of Chinese CO emission from satellite observations, Environ. Pollut., 195, 308–318, https://doi.org/10.1016/j.envpol.2014.07.026, 2014.
Zhang, Y., Li, C., Krotkov, N. A., Joiner, J., Fioletov, V., and McLinden, C.: Continuation of long-term global SO2 pollution monitoring from OMI to OMPS, Atmos. Meas. Tech., 10, 1495–1509, https://doi.org/10.5194/amt-10-1495-2017, 2017.
Zhao, Y., Nielsen, C. P., McElroy, M. B., Zhang, L., and Zhang, J.: CO emissions in China: Uncertainties and implications of improved energy efficiency and emission control, Atmos. Environ., 49, 103–113, https://doi.org/10.1016/j.atmosenv.2011.12.015, 2012.
Zhao, Y., Zhang, J., and Nielsen, C. P.: The effects of recent control policies on trends in emissions of anthropogenic atmospheric pollutants and CO2 in China, Atmos. Chem. Phys., 13, 487–508, https://doi.org/10.5194/acp-13-487-2013, 2013.
Zheng, B., Chevallier, F., Ciais, P., Yin, Y., Deeter, M. N., Worden, H. M., Wang, Y. L., Zhang, Q., and He, K. B.: Rapid decline in carbon monoxide emissions and export from East Asia between years 2005 and 2016, Environ. Res. Lett., 13, 044007, https://doi.org/10.1088/1748-9326/aab2b3, 2018a.
Zheng, B., Tong, D., Li, M., Liu, F., Hong, C., Geng, G., Li, H., Li, X., Peng, L., Qi, J., Yan, L., Zhang, Y., Zhao, H., Zheng, Y., He, K., and Zhang, Q.: Trends in China's anthropogenic emissions since 2010 as the consequence of clean air actions, Atmos. Chem. Phys., 18, 14095–14111, https://doi.org/10.5194/acp-18-14095-2018, 2018b.