the Creative Commons Attribution 4.0 License.
the Creative Commons Attribution 4.0 License.
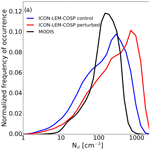
Detection and attribution of aerosol–cloud interactions in large-domain large-eddy simulations with the ICOsahedral Non-hydrostatic model
Montserrat Costa-Surós
Odran Sourdeval
Claudia Acquistapace
Holger Baars
Cintia Carbajal Henken
Christa Genz
Jonas Hesemann
Cristofer Jimenez
Marcel König
Jan Kretzschmar
Nils Madenach
Catrin I. Meyer
Roland Schrödner
Patric Seifert
Fabian Senf
Matthias Brueck
Guido Cioni
Jan Frederik Engels
Kerstin Fieg
Ksenia Gorges
Rieke Heinze
Pavan Kumar Siligam
Ulrike Burkhardt
Susanne Crewell
Corinna Hoose
Axel Seifert
Ina Tegen
Johannes Quaas
Clouds and aerosols contribute the largest uncertainty to current estimates and interpretations of the Earth’s changing energy budget. Here we use a new-generation large-domain large-eddy model, ICON-LEM (ICOsahedral Non-hydrostatic Large Eddy Model), to simulate the response of clouds to realistic anthropogenic perturbations in aerosols serving as cloud condensation nuclei (CCN). The novelty compared to previous studies is that (i) the LEM is run in weather prediction mode and with fully interactive land surface over a large domain and (ii) a large range of data from various sources are used for the detection and attribution. The aerosol perturbation was chosen as peak-aerosol conditions over Europe in 1985, with more than fivefold more sulfate than in 2013. Observational data from various satellite and ground-based remote sensing instruments are used, aiming at the detection and attribution of this response. The simulation was run for a selected day (2 May 2013) in which a large variety of cloud regimes was present over the selected domain of central Europe.
It is first demonstrated that the aerosol fields used in the model are consistent with corresponding satellite aerosol optical depth retrievals for both 1985 (perturbed) and 2013 (reference) conditions. In comparison to retrievals from ground-based lidar for 2013, CCN profiles for the reference conditions were consistent with the observations, while the ones for the 1985 conditions were not.
Similarly, the detection and attribution process was successful for droplet number concentrations: the ones simulated for the 2013 conditions were consistent with satellite as well as new ground-based lidar retrievals, while the ones for the 1985 conditions were outside the observational range.
For other cloud quantities, including cloud fraction, liquid water path, cloud base altitude and cloud lifetime, the aerosol response was small compared to their natural variability. Also, large uncertainties in satellite and ground-based observations make the detection and attribution difficult for these quantities. An exception to this is the fact that at a large liquid water path value (LWP > 200 g m−2), the control simulation matches the observations, while the perturbed one shows an LWP which is too large.
The model simulations allowed for quantifying the radiative forcing due to aerosol–cloud interactions, as well as the adjustments to this forcing. The latter were small compared to the variability and showed overall a small positive radiative effect. The overall effective radiative forcing (ERF) due to aerosol–cloud interactions (ERFaci) in the simulation was dominated thus by the Twomey effect and yielded for this day, region and aerosol perturbation −2.6 W m−2. Using general circulation models to scale this to a global-mean present-day vs. pre-industrial ERFaci yields a global ERFaci of −0.8 W m−2.
- Article
(5327 KB) - Full-text XML
- BibTeX
- EndNote
According to the Fifth Assessment Report of the Intergovernmental Panel on Climate Change (IPCC), clouds and aerosols are the largest contributors to uncertainty estimations and interpretations of the Earth’s changing energy budget (Boucher et al., 2013). In particular, aerosol–cloud interactions continue to be a challenge for climate models and consequently for climate change predictions (Stevens and Feingold, 2009; Feingold et al., 2016; Seinfeld et al., 2016). Changes in aerosol burden have an effect on cloud microphysical properties, with more aerosol leading to more numerous cloud droplets. The resulting change in cloud albedo (Twomey, 1974) implies a radiative forcing due to aerosol–cloud interactions.
Cloud adjustments to aerosol–cloud interactions are manifested as changes in horizontal (cloud fraction) and vertical extent (manifested as liquid water path; LWP) of cloudiness, with consequent impact on the Earth's radiation budget and, thus, climate. With increased aerosol loading and thus increased drop concentrations, at constant LWP, droplets are smaller. One hypothesis for a subsequent adjustment is that the precipitation rates are reduced, implying that the cloud lifetime increases (Albrecht, 1989), and consequently, LWP and cloud fraction increase. But, at the same time, other adjustment processes, such as responses of the cloud mixing and evaporation (Ackerman et al., 2004), occur that partly act in the opposite direction (Stevens and Feingold, 2009; Mülmenstädt and Feingold, 2018; Gryspeerdt et al., 2019). Recently, Toll et al. (2019) have found strong observational evidence that aerosols cause a weak average decrease in the amount of water in liquid-phase clouds compared with unpolluted clouds, since the aerosol-induced cloud water increases and decreases partially cancel each other out. The different adjustments take place at the same time and become more complex with ice- and mixed-phase processes. Because different effects can compensate each other (Stevens and Feingold, 2009; Mülmenstädt and Feingold, 2018), it is difficult to observe isolated cloud effects.
It is nevertheless key to observe how clouds behave as aerosols are perturbed (e.g. Quaas, 2015). Given the large variability of clouds, however, and the plethora of processes involved, it seems best to combine detailed observations with highly resolved modelling (e.g. Mülmenstädt and Feingold, 2018).
Cloud-resolving simulations are a very useful tool to investigate aerosol–cloud interactions, and much of the progress in process-level understanding is from conducting sensitivity studies with such models and analysing model output in detail (e.g. Ackerman et al., 2004; Khain et al., 2005; Xue and Feingold, 2006; Sandu et al., 2008; Small et al., 2009; Feingold et al., 2010; Fan et al., 2013; Seifert et al., 2015; Gordon et al., 2018). New capabilities now emerge with the possibility to perform cloud-resolving simulations over large domains, with realistic boundary conditions at the land surface and driven by the large-scale flow in the numerical weather prediction mode (e.g. Heinze et al., 2017; Miltenberger et al., 2018).
Following this idea, in the present study, mechanisms of aerosol–cloud interactions are analysed and evaluated, using observations and making use of a set ICOsahedral Non-hydrostatic Large Eddy Model (ICON-LEM) simulations over Germany. The key idea is to assess to what extent the model-simulated aerosol–cloud interaction effects might be detected and attributed in comparison to various observational datasets. This is done in terms of quantification of the impact on cloud macro- and microphysics, precipitation and radiation, as a response to the modification of cloud condensation nuclei (CCN) concentrations, the small aerosol particles necessary for water vapour to condensate and form cloud droplets. Two CCN input configurations were used in the highly resolved ICON-LEM simulations (156 m horizontal resolution), which were performed over Germany on a selected date (2 May 2013). For the control simulation, CCN concentrations as estimated for 2 May 2013 from a detailed aerosol model were used. For the perturbation, CCN concentrations valid for 1985 were selected. At this time, pollution in Europe was at its peak, about 4 times higher than at present (Smith et al., 2011; see later for more details). Note that in the present study only modifications to the CCN concentration have been taken into account and not to ice-nucleating particles (INPs). As well, the changes made to the CCN do not affect the scattering or the absorbing aerosol properties (aerosol–radiation interactions, in previous literature referred to as direct and semi-direct aerosol effects) but only affect the aerosol–cloud interactions (also called first aerosol indirect effect, cloud albedo effect or Twomey effect in previous literature and the cloud adjustments, such as the cloud lifetime effect or other rapid responses). The methodology section provides a description of the model (Sect. 2.1) and the CCN perturbation (Sect. 2.2) as well as the observations (Sect. 2.3) used in this study.
The results have been divided into several sections. In order to evaluate the CCN concentrations, in Sect. 3.1 aerosol optical depth (AOD) and CCN are compared with remote sensing observations from satellites and from the ground. After that, the mean vertical profiles of mass and number concentration from the model simulations are revised in Sect. 3.2, and the differences found between perturbed and control simulations are introduced. In the following sections, these differences are explored in comparison to several kinds of observations: liquid-cloud microphysics are compared to satellite and ground-based remote sensing observations in Sect. 3.3 and 3.4; and the aerosol effects on precipitation, as well as cloud boundaries and cloud cover, and their perturbation are analysed and discussed in Sect. 3.5 and 3.6, respectively. Details on the sensitivity to the CCN perturbation in different cloud regimes are discussed in Sect. 3.7. The simulated effects on the radiation budget are quantified in Sect. 3.8. The results are summarized and the conclusions are discussed in Sect. 4.
2.1 Model and simulation setup
The simulations are run with the ICON model, the atmospheric model jointly developed by the German Meteorological Service (Deutscher Wetterdienst; DWD) and the Max Planck Institute for Meteorology for numerical weather prediction (Zängl et al., 2015) and climate simulations (Giorgetta et al., 2018), respectively. Within the High Definition Clouds and Precipitation for Climate Prediction (HD(CP)2) project, another configuration of the model was developed to perform large-eddy simulations (Dipankar et al., 2015), whose physics package is used in the present investigation. A detailed model description is provided by Heinze et al. (2017); their study also provides a thorough model evaluation with various observations including those in the HD(CP)2 Observational Prototype Experiment (HOPE; Macke et al., 2017). The ICON-LEM model includes parameterizations for land surface processes, sub-grid turbulence (3D Smagorinsky turbulence), cloud microphysical processes and radiative transfer. A key feature is an advanced two-moment liquid- and ice-phase bulk microphysics scheme (Seifert and Beheng, 2006): CCN are prescribed in the study (see next section for more details) as temporally and spatially varying fields read in from offline calculations. The model version used here, however, has been updated to allow for the consumption scavenging of CCN; at droplet activation, a CCN is scavenged. For replenishment, CCN concentrations outside clouds are then relaxed back to the prescribed distribution with a relaxation timescale of 10 min. The simulation is performed in a limited-area setup of Germany. It is run in three one-way nested resolutions, and the triangular discretizations at each of the three domains in which ICON-LEM is run correspond to 625, 312 and 156 m horizontal resolution. This analysis focuses on the results at the highest resolution (156 m). In the vertical, 150 levels are used, with the grid stretching towards the model top at 21 km. The minimal layer thickness is 20 m near the surface, and the lowest 1000 m encompass 20 layers.
Due to the large computational cost (1 simulated hour consumes 13 real-time hours on 300 nodes, equivalent to about EUR 100 000 per simulated day of full economic cost of computing time), only a single day was chosen for the model study. Based on the evaluation results from Heinze et al. (2017), the date of 2 May 2013 has been selected. The key reason is that a wide range of cloud and precipitation regimes was present on this day (Fig. 1 illustrates the cloud conditions, based on satellite data). High pressure prevailed over Germany on that date, with low- to mid-level convective clouds that were locally produced. Specifically, at 10:00 UTC shallow convection started and finally lead to a peak of deeper precipitation-forming convection at 17:00 UTC. In the southern and eastern part of the domain, stronger convection occurred, accompanied by thick cloud layers in the afternoon along with a frontal passage. However, the convective clouds were shifted to higher latitudes in the model simulations. More details about the weather conditions are given in Heinze et al. (2017).
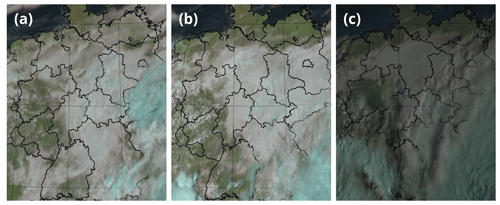
Figure 1Temporal sequence of satellite images at (a) 09:00 UTC, (b) 13:00 UTC and (c) 17:00 UTC on 2 May 2013 as a natural colour composite of the 0.6, 0.8 and 1.6 µm channels and the high-resolution visible channel from the Spinning Enhanced Visible and Infrared Imager (SEVIRI) instrument onboard the geostationary Meteosat satellite. Liquid clouds appear in shades of white, and an increase in ice content shifts the colour of the clouds towards cyan.
Four simulations were conducted (Table 1). The control (C2R) and perturbed (P2R) simulations were initialized at 00:00 UTC on 2 May 2013 from simulations with the COSMO-DE (Consortium for Small-scale Modelling for Germany) model at 2.8 km resolution and were run in three nests from coarse (624 m horizontally) to intermediate (312 m) to fine (156 m) resolution as described in Heinze et al. (2017). For analysis, the daytime period between 08:00 and 20:00 UTC is chosen. In particular, the model output used in the study was, on the one hand, the so-called meteograms which were temporarily highly resolved (9 s) and available at 36 station locations and, on the other, 2D (at 1 min resolution) and 3D (available every 30 min and at 150 vertical levels) whole-domain data fields. The control simulation (C2R) used prescribed CCN distributions from 2013. The sensitivity simulation (P2R) that is analysed here in comparison to the control simulation (C2R) used prescribed CCN distributions from 1985 (see next section).
The extra pair of simulations (C1R and P1R, respectively) was conducted in a setup in which the ICON standard description of cloud optical properties in the radiation scheme was applied. In this approach, cloud optical thickness as input to the radiation is computed on the basis of solely the cloud liquid water mixing ratio, without taking into account variable cloud droplet concentrations. This second pair of simulations with CCN for 2013 and 1985 thus does not account for the radiative forcing due to aerosol–cloud interactions (in previous literature also called first aerosol indirect effect, cloud albedo effect or Twomey effect) but only for cloud adjustments (such as the cloud lifetime effect or other rapid responses). In turn, the first pair of simulations (C2R and P2R, respectively) allows for calculating the full effective radiative forcing due to aerosol–cloud interactions, including the Twomey effect. The difference between the two pairs of simulations thus allows for isolating the latter.
Table 1Summary of simulations for 2 May 2013. Control (“C”) runs are with (low) 2013 aerosol concentrations. Perturbed (“P”) runs are with (high) 1985 aerosol. The first set takes into account two moments of the cloud particles size distributions in radiation (“2R”), the specific mass and number, whereas the second one only accounts for one moment (“1R”), which is the specific mass.
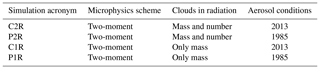
2.2 CCN for 2013 and 1985
The anthropogenic aerosol emissions in the Northern Hemisphere has exhibited a strong increase since industrialization starting in the early 18th century and accelerating from the 19th century onwards. Over Europe and North America, they reached a maximum in the 1980s and have declined since then (Smith et al., 2011; Cherian et al., 2014). Over Europe, the peak was at about 40 Tg sulfur per year. After that, they decreased to early 1900s levels because of the introduction of air quality policies and due to economic restructuring in Eastern Europe. Over the course of about 30 years from the mid-1980s (represented here by the year 1985) to the mid-2010s (represented here by the year 2013), a very substantial change in aerosol concentrations occurred (Smith et al., 2011).
A prerequisite to realistically simulate the aerosol–cloud interactions is a realistic representation of the aerosol concentrations and their capacity to serve as CCN. Compared to the earlier model version (Heinze et al., 2017; Hande et al., 2016), new time-varying 4D distributions of CCN concentrations were generated from the emissions valid for 2013 and for the peak-aerosol conditions around 1985. The simulation imposing these latter CCN concentrations is hereafter called “perturbed”, in comparison to the “control” run which employs CCN concentrations for 2013. The difference between peak aerosol in 1985 and 2013, rather than, e.g. a comparison of 2013 conditions to pre-industrial aerosol, has been chosen for two reasons: (i) the perturbation is much larger since the emissions in 2013 were much closer to pre-industrial than to 1985 levels and (ii) some observations for 1985 are available to assess the aerosol fields.
The CCN distributions were created with the regional coupled model system COSMO-MUSCAT, which consists of two online-coupled codes: the chemistry transport model Multi-Scale Chemistry Aerosol Transport (MUSCAT) (Wolke et al., 2004, 2012) and the Consortium for Small-scale Modelling (COSMO) model, which is the operational weather forecast model of the German Meteorological Service (Deutscher Wetterdienst; DWD) and other national meteorological services in Europe (Baldauf et al., 2011; Schättler et al., 2014). The COSMO model, which is a non-hydrostatic meteorological model and solves the governing equations on the basis of a terrain-following grid (Baldauf et al., 2011), provides MUSCAT with all required meteorological fields. Based on these fields, MUSCAT calculates the transport and transformation processes which include advection, turbulent diffusion and physicochemical conversion of particles and trace gases in the air, as well as sink processes (sedimentation and dry and wet deposition; Knoth and Wolke, 1998; Wolke et al., 2012). The emissions of anthropogenic primary particles and precursors of secondary aerosols are prescribed using emission fields from the European Monitoring and Evaluation Programme (http://www.emep.int/; European Monitoring and Evaluation Programme, 2019). The emission fluxes of natural primary aerosols (e.g. desert dust and primary marine particles) are computed online within the model depending on meteorological fields (surface wind speed and precipitation; Heinold et al., 2011b).
For the present study, two periods of the year 2013 were simulated with COSMO-MUSCAT, coinciding with the measurement campaigns of the HOPE experiment (Macke et al., 2017). It includes measurements around JOYCE (Jülich Observatory for Cloud Evolution; Löhnert et al., 2015) for the period from 26 March to 19 June and at Melpitz from 1 to 30 September. In order to estimate the aerosol concentrations in 1985, the concentrations of 2013 were scaled using scaling factors for black carbon (BC), sulfate (SU), ammonium sulfate (AS) and ammonium nitrate (AN; Genz et al., 2019). The scaling factors were derived based on the emission ratios between 2013 and 1985 for the different species. Genz et al. (2019) estimate that the concentration for BC, SU and AS was larger in 1985 by factors of 2.0, 5.3 and 3.9, respectively, compared to the 2013 concentrations.
Due to the high SO2 emissions in the 1980s, Genz et al. (2019) assumed that there was more than enough sulfate available in order to consume all ammonia to form ammonium sulfate. Therefore, ammonium nitrate is assumed to play a negligible role in the 1980s, and consequently its concentration for 1985 was set to zero. Natural aerosol species (sea salt, mineral dust and organic carbon) are assumed to be unchanged between the two simulations.
In Sect. 3.1 the AOD derived from the model simulations is compared to satellite AOD retrievals. Since the modelled AOD is calculated at 0.5 µm, it was scaled to the 0.63 µm wavelength (taking into account Ångström coefficients for the different species) at which the Advanced Very High Resolution Radiometer (AVHRR) retrieves AOD. The calculation of AOD from the modelled results was done following Meier et al. (2012). Moreover, for both HOPE campaigns, the modelled CCN number concentrations are compared to measurements with PollyXT (portable multiwavelength Raman and polarization lidar neXT generation) lidar systems (Engelmann et al., 2016). In the offline calculation based on COSMO-MUSCAT, the CCN number concentration of the multi-modal size distribution at a fixed supersaturation is calculated according to Abdul-Razzak and Ghan (2000). The calculation of the CCN number concentration (number of activated aerosol particles) follows Hande et al. (2016) and Genz et al. (2019), using the parameterization by Abdul-Razzak and Ghan (2000) for multi-modal size distributions. Details on the used hygroscopicity parameters as well as the derivation of number size distributions from the simulated speciated aerosol mass can be found in Genz et al. (2019). For the comparison to available observations, the CCN number concentration field at a fixed supersaturation is calculated. However, in order to provide CCN fields for ICON-LEM, time-varying 3D fields of the CCN number concentration at different constant updraught velocities were required. Abdul-Razzak and Ghan (2000) relate the aerosol composition and updraught velocity to the maximum supersaturation as air parcels ascend, which in the end determines the number of activated aerosol particles. The calculated CCN fields were then used in the ICON-LEM simulations replacing the fixed assumed CCN number concentration and distribution.
2.3 Observations
2.3.1 Satellite-based
AOD as retrieved in the PATMOS-x (Pathfinder Atmospheres – Extended) Advanced Very High Resolution Radiometer (AVHRR) retrievals uses the 0.63 µm channel. It is only retrieved over sea and in clear-sky cases. The product is a daily average of different NOAA satellites with AVHRR aboard. For 1985 the daily average contains NOAA 7, 8 and 9; and for 2013 data from NOAA 15, 18 and 19 and METOP-B (Meteorological Operational Satellite) satellites are used.
To compare distributions of liquid-cloud properties from the ICON-LEM simulations to satellite observations, the off-line diagnostic tool Cloud Feedback Model Intercomparison Project Observation Simulator Package (COSP; Bodas-Salcedo et al., 2011; Swales et al., 2018) was applied to the ICON simulation output. COSP allows for consistency between cloud properties simulated by ICON and retrieved by satellite observations such as the Moderate Resolution Imaging Spectroradiometer (MODIS). For 2 May 2013, collection 6 cloud products MOD06/MYD06 (Platnick et al., 2015, 2017) from four MODIS satellite overpasses within the domain (MODIS Aqua at 11:45 and 13:20 UTC; MODIS Terra at 09:55 and 11:35 UTC) were analysed. The ICON-COSP simulations were temporally and spatially matched to the satellite observations as well as regridded to the MODIS data resolution of 1 km. No sub-column variability is used in COSP, consistent with the lack of sub-pixel variability in MODIS retrievals. Only cloudy satellite pixels with assigned liquid-cloud phase as well as good quality and solar zenith angles below 50∘ were considered in order to exclude uncertain or problematic cloudy retrievals.
For liquid clouds, number concentration (Nd) is derived from cloud effective radius (re) and cloud optical thickness (τc) as in Quaas et al. (2006), where α = m−0.5 for
an approach that assumes an adiabatic growth of clouds (Grosvenor et al., 2018).
2.3.2 Ground-based
A comprehensive set of active and passive remote sensing instruments is part of the Leipzig Aerosol and Cloud Remote Observations System (LACROS; Bühl et al., 2013). Specifically, its multiwavelength-Raman-polarization lidar provides backscatter and extinction profiles almost continuously (Baars et al., 2016). In this study, it serves to retrieve aerosol profiling variables and CCN number concentration, re, liquid water content (ql), and Nd, which are compared with the model output. The CCN concentration profiles of the lidar measurements were calculated using the method described in Mamouri and Ansmann (2016) for measurements during the HOPE campaign (Sect. 3.1). Profiles of liquid-cloud microphysical properties were derived from ground-based remote sensing using the recently established dual-field-of-view (DFOV) lidar techniques (Grosvenor et al., 2018). Such observations are available at Leipzig, Germany (51.3∘ N, 12.4∘ E), beginning in 2013 and provide information about aerosol–cloud interaction processes (Schmidt et al., 2014) and allow for a climatological assessment (Sect. 3.4). Originally, the observations were based on the DFOV Raman lidar technique (Schmidt et al., 2013), which can only be applied to nighttime lidar observations in order to reduce effects of the solar background on the measurements of Raman-scattering lidar returns from nitrogen molecules. Progress that was made recently in the accuracy of polarization measurements with lidar (Jimenez et al., 2019) allows for applying an alternative technique for the profiling of liquid-cloud microphysical properties even during daytime (Jimenez et al., 2017, 2020). In this novel DFOV-polarization approach, the liquid water content, ql, profile is assumed to increase adiabatically with height from the cloud bottom, while the Nd remains constant. An inversion scheme exploits a non-ambiguous relation between re and the extinction coefficient, both dependent on Nd and ql, with the single-FOV depolarization ratio and the relative depolarization, quantities that a DFOV-polarization lidar can measure (Jimenez et al., 2017, 2020).
The cloud radar (8.6 mm wavelength), based in the Lindenberg Meteorological Observatory – Richard Assmann Observatory (MOL-RAO), is well suited for the study of thin, low-reflectivity clouds such as non-drizzling and drizzling stratocumulus clouds, due to its high sensitivity. The cloud radar transmits linear polarized radiation at 35.5 GHz and simultaneously receives the co- and cross-polarized backscattered signal. Observations in the zenith mode are used, with an integration time of 1 s and a 256 point Fourier transform for generating the Doppler spectrum. Forward simulations of radar Doppler spectra and their corresponding moments have been performed from the ICON-LEM simulation output using the radar forward simulator included in the Passive and Active Microwave radiative TRAnsfer (PAMTRA) framework (Maahn, 2015). The moments of the synthetic Doppler spectra are derived in the same way as for the observations (Acquistapace et al., 2017), and then the drizzle detection criterion described in Acquistapace et al. (2019) is applied to them. Only liquid clouds are selected following Cloudnet criteria (Illingworth et al., 2007). In Sect. 3.5 the ICON-LEM forward-simulated reflectivities have been compared to the radar observations for four different categories of drizzle, cloud droplets and rain drops.
The ceilometer network of DWD provides backscatter measurements and cloud base height (CBH) retrievals from Jenoptik ceilometers (model CHM15k) at very high temporal resolution (15 s) up to 15 km above ground at 51 stations across Germany (Wiegner and Geiß, 2012; Wiegner et al., 2014; Martucci et al., 2010, http://www.dwd.de/ceilomap; last access: 16 April 2020) within the ICON-LEM domain. The CBH is an output from ICON-LEM diagnostics, which is determined as the lowest cloudy grid cell of each column. The threshold for determining a cloudy grid cell in ICON-LEM is a sum of cloud water and cloud ice (qc and qi) larger than 10−8 kg kg−1. CBH, cloud occurrence as an estimator of cloud cover (CC; see methodology in Costa-Surós et al., 2013) and cloud persistence (CP) derived from the ceilometer network are compared with those simulated at the same locations with the ICON-LEM. The methodology to derive the CP is the following: the time resolution from 51 CBH time series from ceilometer observations (15 s resolution) was changed to 1 min, which matches the output frequency from ICON-LEM diagnostics. All three 12 h (8–20 h) time series (ceilometer observations and control and perturbed simulations) were split into 30 min intervals. For each of these 30 min intervals, the CBH between 0 and 3000 m was checked, and if there was a CBH, it was flagged as cloudy, and the neighbour cloudy pixels were assigned to one cloud, so a cloud persistence for each single cloud of the interval was assigned. Then each 30 min interval was classified according to its cloud cover as “clear sky” (0 %–5 %), “few clouds” (5 %–25 %), “scattered clouds” (25 %–50 %), “broken clouds” (50 %–87 %) or “overcast sky” (87 %–100 %). After that, a normalized histogram was plotted using the cloud persistence from the time intervals classified as “few”, “scattered” and “broken” clouds, i.e. cloud cover between 5 % and 87 % (see Sect. 3.6).
3.1 Evaluation of the perturbed and unperturbed CCN and aerosol distributions
A comparison of the AOD over the North and Baltic seas as retrieved from AVHRR for 1985 and 2013 and AOD simulated by COSMO-MUSCAT is shown in Fig. 2 and summarized in Table 2. Note that since AVHRR retrieves AOD only in cloudless cases over sea, AOD is only available over the North Sea and Baltic Sea region for a small fraction of the time (between 10 % and 12 % in 1985 and 35 % and 38 % in 2013, Table 2). Approximately double AOD levels on average are observed in March–June 1985 in comparison to March–June 2013. The average AOD and also its change between 1985 and 2013 are well captured by the model simulation, considering the observation and modelling uncertainty and natural variability. The geographical distribution over sea (Fig. 2) also shows consistency between the simulation and the satellite retrievals with strong differences between the polluted spring 1985 and the much cleaner spring 2013. Since not very many data points go into the average from the satellite, the distribution is more noisy compared to the simulation output that is available anytime. Despite this, it is evident that both observations and model consistently show the expected spatial gradient with larger AOD near the coast and less aerosol over open sea.
Table 2Median aerosol optical depth from AVHRR and COSMO-MUSCAT from 26 March to 19 June in 1985 and 2013. The 25th and 75th percentile ranges of regional variability of the temporal mean AOD are provided in brackets. The mean number of days (out of the possible 86 d) per grid box with valid AVHRR retrieval is shown next to the location.

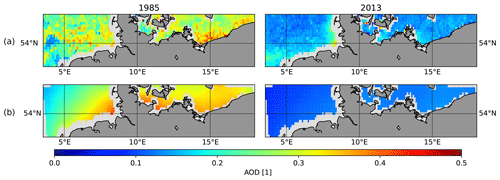
Figure 2Mean AOD retrieved from (a) AVHRR and simulated by (b) COSMO-MUSCAT for 1985 and 2013. The comparison period covers 26 March to 19 June for each of the years.
Profiles of CCN at 0.2 % supersaturation from both COSMO-MUSCAT and PollyXT lidar retrievals are shown in Fig. 3. The location and time period assessed is the HOPE campaign (Krauthausen, Germany) from 26 March to 19 June 2013. Model and retrieval agree to within the uncertainty in the boundary layer. The mean profiles for 2013 are overestimated by the model by 10 %–30 % in the boundary layer (from the surface to 1800 m in spring 2013 and 1200 m in autumn 2013). Above the boundary layer, the overestimation increases to more than a factor of 2, since the model tends to overestimate the vertical mixing between the boundary layer and free troposphere (Heinold et al., 2011a). The estimated CCN concentrations for 1985 are inconsistent with the observations; they exceed the concentration in 2013 by a factor of 2–5 in the boundary layer and up to an order of magnitude in the free troposphere. Similar results are found for the HOPE-Melpitz campaign during September 2013 (not shown).
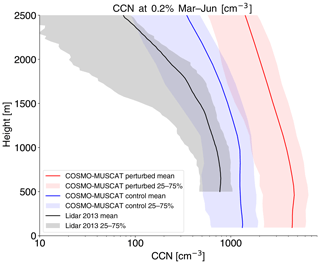
Figure 3Comparison of mean vertical profile of CCN number concentration at 0.2 % supersaturation simulated by COSMO-MUSCAT (aerosol conditions for 2013 in blue and 1985 in red) and retrieved from the PollyXT lidar measurements using the algorithm of Mamouri and Ansmann (2016) (black), from 26 March to 19 June 2013 during HOPE campaign in Krauthausen, Germany. The shaded area depicts the 25th to 75th percentiles of the set of single profiles included in the average. A factor of 2 of uncertainty is considered for the lidar retrievals, as outlined in Mamouri and Ansmann (2016) and Ansmann et al. (2019).
In conclusion, the imposed aerosol concentrations for 1985 (perturbed simulations) and 2013 (control simulations) match the distribution and mean values from the satellite retrievals over clear-sky ocean well, and it is evident that only the 2013 aerosol yields CCN profiles that are consistent with lidar retrievals from 2013.
3.2 Mean vertical hydrometeor profiles of number and mass concentrations: control vs. perturbed simulations
Domain-averaged hydrometeor profiles of number and mass concentration from the model output on 2 May 2013 from 08:00 to 20:00 UTC are shown in Fig. 4. The vertical profile of cloud droplet concentrations closely corresponds to the introduced CCN disturbance (vertical integrated relative increase of 147 %; Table 3). In the following sections (3.3 and 3.4) it is explored to which extent this perturbation is also seen by satellites and from ground-based remote sensing in terms of Nd retrievals. The total-water mass difference at about 2 km altitude (vertically integrated relative increase by 8.8 %) is mainly due to decreased rain in the perturbed simulation (−12.3 %). In the following sections, the changes in the LWP and liquid water content (ql) are investigated.
A slightly higher homogeneous cloud droplet freezing for the perturbed simulation is triggered by upward transport of cloud droplets. In turn, graupel number and mass concentrations are slightly higher in the cleaner environment in low to mid altitudes (3–4 km). On the contrary, at higher altitudes (6–10 km) the graupel mass is higher in the perturbed simulation.
Table 3All-sky domain-mean vertically integrated changes between the perturbed and control simulations (P2R–C2R) for number and mass concentrations for water species and for the total water as temporal average from 08:00 to 20:00 UTC. The absolute changes are given along with the temporal standard deviation of the domain-mean changes. The numbers in brackets are the relative changes.
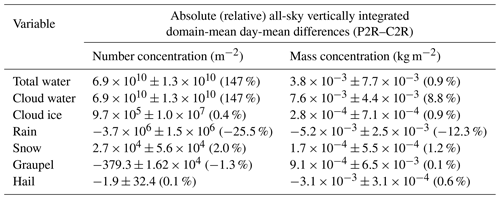
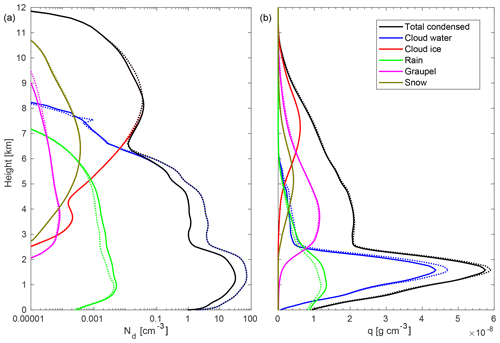
Figure 4Vertical profile of (all-sky) domain-mean number concentration (a) and mass concentration (b) of total water and individual particle species on 2 May 2013 from 08:00 to 20:00 UTC. The control (C2R) simulation is plotted as solid lines, and the perturbed (P2R) simulation is plotted as dotted lines. Note the logarithmic x axis for the number concentration panel. Hail not shown due to very low values. Also note that in the left panel the black solid line is over the blue solid one, and the blue and black dashed lines are also one over the other.
3.3 Liquid-cloud microphysics in comparison to satellite data
Normalized frequency of occurrence distributions of liquid water path (LWP) and cloud droplet number concentration (Nd) from the reference (C2R) and perturbed (P2R) ICON-LEM simulations as well as the corresponding satellite retrievals (MODIS) are shown in Fig. 5. The maximum peak of occurrence and 50th percentile values for Nd compare well between the ICON control simulation (C2R) and MODIS, although the distribution of Nd simulated by ICON-LEM is much broader, resulting in lower and higher 25th and 75th percentile values, respectively, compared to MODIS (Table 4). This can be partly explained by a range simulated by the model that is too large, which in turn can be partly related to a difference in the observed and simulated spatial distribution of clouds at the MODIS observation times. However, a part of the difference in the range of the distributions can be very likely attributed to the MODIS instrument characteristics, since optically very thin clouds are not observed or give problematic retrievals (Grosvenor et al., 2018), and for optically very thick clouds, the measurements can go into saturation (note that Nd is computed from the cloud optical thickness and cloud effective radius; see Eq. 1). The simulated Nd distribution for the perturbed simulation (P2R) is shifted to significantly higher values. A factor of about 2 between ICON control and ICON perturbed simulations is reflected in the percentile values.
For LWP values larger than about 10 g m−2, MODIS and ICON compare well, both showing occurrence peak values between 100 and 200 g m−2. However, ICON (control and perturbed) has a higher frequency of low LWP values, resulting in lower 25th, 50th and 75th percentile values. The model also does not show a bi-modal distribution as MODIS does. This bi-modal distribution is due to having two different cloudy scenes in the different overpasses, which happen at different times of the day, i.e. a cloudy scene with optically thinner clouds and thus lower LWP values and then scenes with optically thicker clouds and higher LWP values. However, even if the ICON-LEM output is sampled along the MODIS swath, it does not show this distinct behaviour but rather a smooth distribution. The difference between LWP from the ICON reference simulation (C2R) and ICON perturbed simulation (P2R) is small compared to the LWP variability and small in comparison to the model bias with respect to the MODIS retrievals. It is nevertheless a systematic increase in LWP that is simulated, even if it is small, as also expected from recent investigations of satellite data (Malavelle et al., 2017; Toll et al., 2017, 2019; Gryspeerdt et al., 2019). An exception is at large LWP values (larger than 200 g m−2; see Fig. 5), where the control simulation is much smaller than the perturbed one and closer to the satellite retrievals. This is firstly consistent with the expectation that an increase in Nd leads to an invigoration of convective clouds and, hence, deeper clouds with higher LWP values in the tail of the LWP distribution, where the convective cloud cores can be found. It, secondly, reflects the fact that the thick, precipitating clouds most strongly respond to precipitation delay in response to the CCN perturbation.
Table 4Median, 25th and 75th percentiles of liquid water path (LWP) and cloud droplet number concentration (Nd) from MODIS and ICON-LEM distributions.
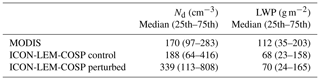
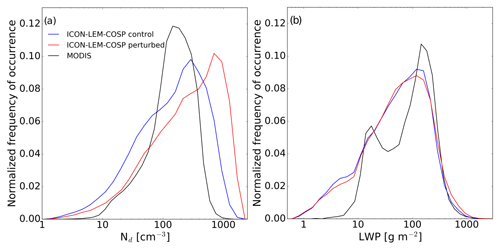
Figure 5Normalized frequency of occurrence distributions computed from ICON-LEM-COSP data from the 2 May 2013 control (C2R, blue) and perturbed (P2R, red) simulations, matched with satellite observations from MODIS (black) obtained at the four satellite overpass times on the same day for (a) Nd and (b) LWP.
In conclusion, the influence of the perturbed aerosol on Nd clearly can be detected and attributed in comparison to satellite retrievals, but the systematic increase in LWP is too small in comparison to natural variability and model bias to be detected and attributed, except for large LWP values (>200 g m−2). The systematic change in LWP, even if small compared to weather variability, implies a substantial contribution to the aerosol effective radiative forcing.
3.4 Liquid-cloud microphysics in comparison to ground-based remote sensing
Profiles of several cloud microphysical variables (Nd, re and ql) were retrieved from ground-based remote sensing as explained in Sect. 2.3.2. In order to derive comparable profiles from ICON-LEM that are best suited for evaluation against the lidar observations, the temporarily highly resolved (9 s) meteogram output was used. All profiles from 21 of the 36 stations for which meteogram output of ICON-LEM was available (Table A1) were in a first step screened for the occurrence of the presence of hydrometeors. The remaining 15 stations were not considered in the analysis because they were too close (approx. within 20 km) to an already considered station, which would lead to an unwanted weighting of the statistics towards a certain region of the ICON-LEM domain. This was specifically the case for the region of the HOPE campaign (Macke et al., 2017), for which output of 13 stations is available. Vertically continuous sequences of hydrometeors were classified as cloud layers to which cloud base height and cloud top height were assigned. Each identified cloud layer was in a subsequent processing step filtered in such a way that (i) precipitation was absent, (ii) ice was absent, (iii) LWP >150 g m−2, (iv) cloud depth <500 m and (v) 1000 m < cloud base height <4000 m. Such constraints are similar to the properties of cloud layers which were observed with the DFOV-polarization lidar at Leipzig. The Cloudnet processing suite (Illingworth et al., 2007), which operated based on cloud radar, lidar and microwave radiometer observations at Leipzig, was used to identify these conditions for the periods when valid DFOV-polarization observations were made.
For the evaluation of the ICON-LEM simulations, approx. 40 h of DFOV-polarization lidar observations with 3 min temporal resolution (i.e. 800 profiles) was averaged to yield mean vertical profiles of Nd, ql and re. The cloud periods considered were distributed over 27 d in the spring, summer and autumn seasons of 2017 and over heights between 1 and 4 km a.g.l. (above ground level). The resulting profile from the observations and the outputs from the ICON-LEM model for 1985 and 2013 aerosol conditions of 2 May 2013 are presented in Fig. 6. The results corroborate the satellite-based conclusions: for Nd, the simulation with the 1985 CCN is inconsistent with the lidar observations, and the one with the 2013 CCN matches the retrievals to within the uncertainty. A similar conclusion is drawn for the effective radius. For ql, in turn – as found before during the comparison to satellite-retrieved LWP – the model shows only little aerosol impact, and both realizations compare almost equally to the observations.
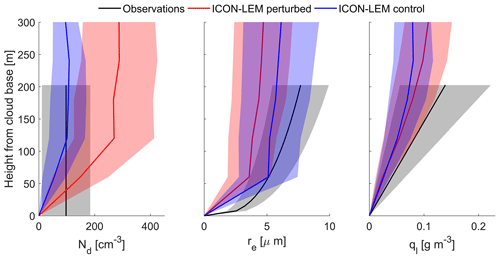
Figure 6Averaged cloud droplet number concentration (Nd), cloud droplet effective radius (re) and liquid water content (ql) profiles retrieved from lidar (in black and the temporal variability as shading in grey) and in ICON-LEM control (in blue) and perturbed (in red) simulations, as a function of height above cloud base. Note that measurements can only provide profiles up to 200 m into the cloud due to the strong extinction of the lidar signal.
3.5 Effects on precipitation
As discussed earlier, the model simulates large reductions in rain mass and number concentrations and increases in cloud liquid mass and number concentrations in the perturbed vs. control simulation (Fig. 4). To enquire into the role of the autoconversion process and find out to which extent these changes might be observable, forward simulations of control and perturbed meteogram profiles at the site of the MOL-RAO have been performed using the PAMTRA tool (Maahn et al., 2015). After that, the drizzle detection criterion described in Acquistapace et al. (2019) has been applied to the forward-simulated Doppler radar moments that were generated with PAMTRA. This approach is similar to the one presented in Rémillard et al. (2017). An ice cloud mask has been applied to the model output in order to filter out the ice pixels, therefore applying the drizzle detection criterion only to the pixels corresponding to liquid in the cloud mask in both observations and model output.
The distributions for the different classes of drizzle development are shown in Fig. 7 and summarized in Table 5. The postprocessed model results shown in Fig. 7 are consistent with the raw results summarized in Fig. 4: the perturbed simulation shows reflectivities that are shifted towards smaller values for all drizzle and precipitation classes, compared to the control simulation. Vice versa, the control simulation produces larger reflectivities, hence larger drops at every stage of drizzle formation, and produces larger raindrops. The mean simulated values of the reflectivities, from both control and perturbed, fall into the range of the observations of the MOL-RAO radar, except for the precipitation where both runs overestimate the reflectivities. This overestimation is also visible from the mean Doppler velocities (proxy of fall speed of hydrometeors) and spectrum width distributions (not shown). Mean Doppler velocities are too high (due to drops that are too large compared to reality), and this produces also broader spectra, giving larger spectrum widths compared to what is observed. A closer look into the radar signal suggests that the small reflectivity values for precipitation observations are due to insects detected by the radar despite that a clutter removal filter was applied to the radar spectra from 0 to 1600 m a.g.l. as preprocessing.
Table 5Median as well as 25th and 75th percentiles of the cloud radar reflectivity (Ze) distributions on 2 May 2013 for the four classes described in Fig. 7.

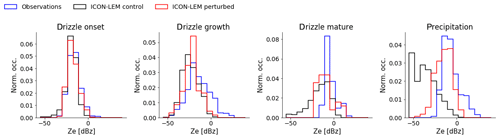
Figure 7Normalized distributions for the different classes of drizzle development in the Lindenberg Meteorological Observatory – Richard Assmann Observatory (MOL-RAO) on 2 May 2013. (a) Drizzle onset, corresponding to the small non-precipitating drizzle drops, larger than cloud droplets but not big enough to fall yet. This is only a signature in the skewness of the Doppler spectrum, not in reflectivity. (b) Drizzle growth, which contains the drops big enough to modify the spectra shape. (c) Drizzle mature, which is the drizzle precipitating inside the cloud. (d) Precipitation, which is the class of precipitation below cloud base. The radar observations are processed as in Acquistapace et al. (2019), and ICON-LEM meteogram output at the MOL-RAO site is processed using the PAMTRA forward operator and processed analogously. Black: observations; blue: control simulation (C2R); red: perturbed simulation (P2R).
In conclusion, despite the effort to make model and data comparable using the forward operator and despite the rather strong signal in the model, no detection and attribution of an aerosol signal could be achieved. In the future more comparisons are needed as the difference at one grid point only could arise from a different sampling of cloud life cycle.
3.6 Cloud macrophysics: cloud boundaries, cloud cover and cloud persistence
ICON-LEM cloud base height (CBH), as well as the calculated cloud cover (CC) and cloud persistence (CP) based on the CBH measurements, is compared with high-resolution ceilometer measurements (15 s temporal resolution) from the DWD ceilometer network (please see Sect. 2.3.2 for details). In Table 6, mean CBH and CC have been calculated over the 51 stations for 2 May 2013. On average, the model produces fewer clouds than observed, and the cloud base heights are too low in comparison to the ones measured by the ceilometer network. The problem is not due to issues with the initial- or boundary conditions, as the discrepancy to the reference observations is larger in the outer nests (312 and 624 m resolution, respectively; result not shown here). However, the cloud variability is very large, and the model output still is consistent with the data to within the uncertainty range. For both variables, the differences between control (C2R) and perturbed (P2R) simulations are so small, in comparison to the observations, that no significant deviations can be detected given the simulation and observation uncertainties. The absolute (relative) differences between perturbed and control simulations are for the mean CBH −4 m (−0.37 %) and for the CC 0.4 % (0.70 %). The same tendency is found in the all-domain simulation means (Table 7), where the perturbed ICON-LEM shows on average higher cloud tops and bases in comparison to the control simulations. The difference between mean cloud top pressures is −263 Pa (0.35 %), and the difference between mean cloud base pressures is −140 Pa (0.17 %). The perturbed simulation also shows higher total cloud cover by 0.16% (relative difference: 0.20 %) in the domain average (Table 7). The cloud fraction can also be assessed from MODIS satellite data and compared to the COSP-processed ICON-LEM output (as in Sect. 3.3 for LWP and Nd). The domain-averaged cloud fraction for the four MODIS overpasses is 0.84, compared to 0.49 and 0.50 for the control and perturbed ICON-LEM simulations, respectively. Despite the very different observational approaches and spatiotemporal sampling, the general conclusion is confirmed: ICON simulates fewer clouds than observed (a result that also has been noted by Heinze et al., 2017) and shows a positive response of cloud fraction to more aerosol.
Figure 8 shows normalized CBH distributions for the ceilometer network and for both the ICON-LEM control (C2R) and perturbed (P2R) simulations, which overestimate very low CBH (< 500 m) and underestimate higher CBH values (751–1751 m). Possibly the ceilometer network is not able to detect the latter reliably. Further analysis of 30 min periods exhibit that both simulations overestimate “clear sky” cases (0 %–5 %) and underestimate “overcast” skies (87 %–100 %). “Few” (5 %–25 %) and “scattered” (25 %–50 %) skies are also overestimated, and “broken clouds” (50 %–87 %) are slightly underestimated (not shown). Cloud persistence analysis for the group of few, scattered and broken cloud conditions (i.e. cloud cover between 5 % and 87 %) and with bases below 3000 m also shows small differences between control and perturbed distributions (Fig. 8). None of the simulations are able to capture the observed distributions for the short-lived clouds (less than 5 min), while the longer-lived clouds are overestimated.
Table 6Cloud base height (CBH), cloud persistence (CP) and cloud cover (CC) as measured at 51 ceilometer stations in Germany and from ICON-LEM output (2 May 2013 from 08:00 to 20:00 UTC), as well as CC retrieved from MODIS satellite and ICON-LEM output postprocessed with the COSP simulator. Uncertainty ranges are provided as 25th and 75th percentile ranges.
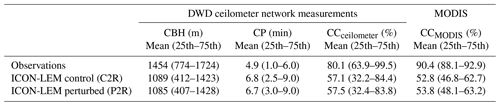
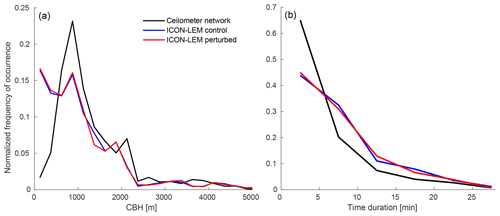
Figure 8(a) Normalized cloud base height distribution (bin size: 250 m) of 51 ceilometer stations over Germany on 2 May 2013 (08:00–20:00 UTC). (b) Normalized cloud lifetime (bin size: 5 min) for low-level clouds (< 3 km) of few, scattered and broken cloud conditions (i.e. cloud cover between 5 % and 87 %). Data from the DWD ceilometer network in Germany (black) and ICON-LEM C2R (blue) and P2R (red) simulations.
The comparison of CBH, CC and CP between model and ceilometer measurements shows systematic differences between either model simulation and the observations. Detection and attribution of differences between control (C2R) and perturbed simulations (P2R) is not feasible in this case. This is mostly because the effect of the aerosol perturbation on these quantities is small compared to the model bias.
3.7 Sensitivity to cloud regimes
The International Satellite Cloud Climatology Project (ISCCP) regime classification (Rossow and Schiffer, 1991) utilizes cloud top pressure and τc to define different cloud regimes. A consistent diagnostics is applied to the ICON output. From the top of the atmosphere down in each column, the first model grid point which has a condensed mass of cloud water plus cloud ice above a threshold of 0.01 g kg−1 is utilized to obtain the cloud top pressure (threshold from van den Heever et al., 2010).
An offline computation is used for the total column optical thickness. Cloud top pressure and total column optical depth categorize the clouds into the nine categories, namely: cumulus, stratocumulus, stratus (low-level clouds), altocumulus, altostratus, nimbostratus (mid-level clouds), cirrus, cirrostratus (high clouds) and deep convective clouds. We applied the classification to the perturbed (P2R) and reference (C2R) model simulations. The classified cloud regime distributions are very similar for the perturbed and control simulations (Fig. 9 shows, as an example, the distribution at 11:45 UTC). Applying the ISCCP classification to MODIS satellite data yields larger low- and mid-level cloud coverage, compared to the model, and less convective cloud coverage. The low- and mid-level clouds observed by MODIS are classified as ISCCP cirrus and cirrostratus clouds. A convective region (clouds with high column optical thickness and high cloud tops) in the south-east is misclassified by the model, compared to MODIS, while the convective region in the north-east is overrepresented in the model. A part of the difference in simulated and observed cloud types can be attributed to a difference in the spatial distribution of the cloud types at the MODIS overpass time. Both cirrus and convective clouds are most abundant in the late afternoon and evening when no MODIS satellite observations were available.
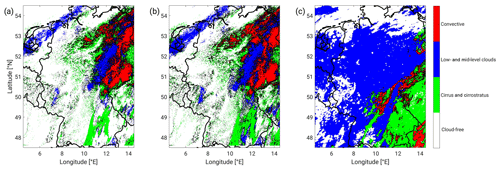
Figure 9Cloudy regimes after application of the ISCCP classifications to ICON-LEM (a) control, (b) perturbed model output and (c) MODIS at 11:45 UTC (MODIS overpass time).
Figure 10 displays a normalized frequency of occurrence distributions of Nd, cloud water path and cloud optical thickness for the entire domain for low- and mid-level, convective and high (cirrus and cirrostratus) clouds from the ICON-COSP simulator output, and MODIS retrievals at the four times of MODIS overpasses (Sect. 2.3). On the one hand, liquid-cloud droplet number concentration does show sensitivity for low- and mid-level clouds, since control simulations fits well to MODIS liquid number concentration and perturbed simulation has higher values (already seen in Sect. 3.3). On the other hand, cloud water path is barely sensitive to the CCN perturbation for all cloud regimes considered in the analysis (consistent with the result in Sect. 3.3). However, the control mean values are slightly closer to the observations in all cases. Besides, cloud optical thickness shows a notable increase in the perturbed simulation for low- and mid-level clouds, compared to the control simulation. On average, the control simulation is closer to MODIS retrievals than the perturbed simulation. For high and convective clouds, there is not much difference between control and perturbed simulations, and they partly fit to the MODIS distribution.
Looking at the different cloud regimes, we can conclude that (1) Nd is a suitable variable for the detection and attribution of changes of liquid clouds (low- and mid-level clouds), (2) there is a potential use of cloud water path (CWP) and cloud optical thickness (COT) for detection and attribution specifically for low- and mid-level clouds, and (3) the ice concentrations are too similar in the control and perturbed ICON-LEM simulations and so do not allow for an attribution of an aerosol signal of convective and high clouds (cirrus and cirrostratus), at least regarding CWP and COT variables.
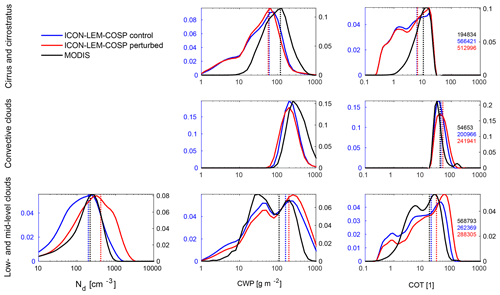
Figure 10Normalized frequency of occurrence distributions of droplet number concentration (left column, only for low, liquid clouds), cloud water path (centre column, liquid plus ice water) and cloud optical thickness (right column) of cirrus and cirrostratus clouds (top row), convective clouds (second row), and low- and mid-level clouds (bottom row) from the ICON-COSP simulator and MODIS (in total four overpasses on 2 May 2013). Left y axes are for ICON-LEM-COSP, and right y axes are for MODIS observations. Straight-dotted lines display means. The numbers on the right side show the number of pixels used for the normalized frequency of occurrence distribution calculation.
3.8 Radiative implications
The effective radiative forcing (ERF) due to aerosol–cloud interactions (ERFaci) has been estimated by subtracting the control simulation (C2R) domain averages from the perturbed (P2R) ones (Table 7). For the simulated case, the ERFaci is W m−2 in the top-of-the-atmosphere (TOA) net solar radiation () and 0.21±0.40 W m−2 for the TOA net thermal radiation (). Consistent with the expectation, the negative forcing in the solar spectrum is slightly reduced by a positive forcing in the terrestrial spectrum (Heyn et al., 2017).
Thanks to the extra simulations that did not use the number concentration in the radiation transfer computations (C1R and P1R), which also have two different 4D CCN concentration distributions (for 1985 and 2013), the adjustments to the RFaci (radiative forcing due to aerosol–cloud interactions), as far as they operate via cloud and precipitation microphysical and dynamical changes, were quantified. In these simulations, only the adjustments associated to aerosol forcing are responsible for the radiation changes. The results are noisy signals: the average changes in cloud fraction and LWP are not different from zero to within the temporal variability (not shown). On average, cloud fraction is simulated to decrease slightly ( %). This result is surprising and different from what is seen in satellite statistics (Gryspeerdt et al., 2019). Further analysis is ongoing. The consequence of the decreasing cloud cover, which is more important radiatively than the increase in LWP, is a positive radiative effect of W m−2. As the difference between the ERFaci and the adjustments, RFaci, or the cloud albedo effect (Twomey, 1974), is obtained as −2.85 W m−2.
In order to put this number into context, we assess the ERF computed by general circulation models. Within the 6th Coupled Model Intercomparison Project (CMIP6; Eyring et al., 2016), the Radiative Forcing Model Intercomparison Project (RFMIP; Pincus et al., 2016) defined a simulation dedicated to the assessment of the transient historical effective radiative forcing, the simulation “RFMIP-ERF-HistAerO3”. There are four models in the CMIP6 archive that submitted output for these simulations, namely the CanESM5 (Canadian Earth System Model, Swart et al., 2019), the GFDL-CM4 (Geophysical Fluid Dynamics Laboratory Coupled Model; Held et al., 2019), the MIROC6 (Model for Interdisciplinary Research on Climate; Tatebe et al., 2019) and the NorESM2-LM (Norwegian Earth System Model low-atmosphere–medium-ocean resolution; Bentsen et al., 2013; Kirkevåg et al., 2018), which supplied three, one, three and two ensemble members, respectively. The ERFaci is approximated by using the change in cloud radiative effect (CRE; the difference between all-sky and clear-sky top-of-atmosphere net radiation flux density here taken in the solar spectrum only) between two time periods (Quaas et al., 2009). When evaluating the difference in solar CRE of the individual years 2013 and 1850, one obtains as the difference in global annual mean, a multi-model mean of −0.81 W m−2, with an inter-model standard deviation of 0.34 W m−2 (using all ensemble members). For a 5-year average difference (2010 to 2014 and 1850 to 1854; the periods are not centered around the specific years because the simulations run from 1850 to 2014; Pincus et al., 2016), the values for the global annual mean are W m−2. For the domain of the ICON-LEM simulation, and only using May (only monthly output is available), the signal, defined as the difference 1985 minus 2013, is much more noisy, since it is averaged as much less. The mean and standard deviation are W m−2. To assess the uncertainty, we also computed the change in solar CRE for the months April and May (since the actual day is early May), averaged over the 5-year periods from 1983 to 1987 minus 2010 to 2014 and a larger domain (10∘ W to 30∘ E, 40 to 60∘ N). This yields a smaller value and much smaller standard deviation of W m−2. The scaling factor for the 5-year and bigger European domain is 3.6; the one for the single years and ICON-LEM domain is 5.8. The uncertainty in these scaling factors obtained from the general circulation models (GCMs) is very large. Nevertheless it may be instructive to know that the forcing for May, considering the large difference in aerosol levels over Europe between 1985 and 2013, is a factor of 4 to 6 larger than considering the global ERFaci between 2013 and 1850. The −2.6 W m−2 obtained in this simulation (Table 7) thus would imply a global, annual mean ERFaci for 2013 vs. 1850 of between −0.4 and −0.7 W m−2.
This study used a new type of large-eddy simulations which was carried out over a very large domain and driven as a numerical weather prediction with realistic initial and boundary conditions, including an interactive land surface. A large set of observational data from various sources is used aiming for detection and attribution. Four simulations with the ICON-LEM model over Germany were carried out with different time-varying prescribed CCN concentration distributions. The 4D CCN concentration inputs generated with COSMO-MUSCAT for 2 May 2013 and 1985 were demonstrated to be consistent with the satellite retrievals of AOD by several AVHRR instruments on different NOAA satellites. Furthermore, the control simulation (C2R) results are consistent with the CCN profile as retrieved from ground-based lidar at two sites in 2013, while the ones for the perturbed simulation (P2R), with 1985 conditions, are not.
In terms of cloud quantities, it was demonstrated that detection and attribution of the aerosol-induced changes of the droplet number concentration is also possible. The simulated cloud droplet number concentration for the 2013 aerosol simulation (C2R) is consistent with MODIS satellite retrievals, while the perturbed simulation (P2R) results are on average 2 times higher. An assessment for Nd also was possible from ground-based active remote sensing thanks to a new lidar retrieval technique. The result confirmed the conclusions on the basis of the satellite data, namely that Nd using the 2013 aerosol is consistent with the 2013 observations, while the perturbed-run output is not.
The other cloud quantities examined included cloud liquid water path, cloud fraction, cloud base height and cloud lifetime. Satellite data and network ground-based remote sensing were used as observational reference data. For all of these quantities, the ICON model simulated systematic changes between the perturbed (P2R) and control (C2R) aerosol runs. However, in each case, the difference between either model simulation and the observations was larger than the difference between the simulations in response to the different aerosol conditions. In addition, the natural cloud variability was large compared to the signal. A possible exception is that at large LWP values (>200 g m−2), the control simulation was consistent with the satellite retrievals, while the perturbed simulation showed LWP values which are too large. Small changes between perturbed and control simulations are found in the surface rain rate domain-averaged mean (−2.6 %). However, a detection and attribution even with detailed radar observations was impossible. The sensitivity analysis of different variables in different cloud regimes may well depend on the synoptic situation. It cannot be excluded that in other synoptic situations the sensitivity in mixed-phase clouds and maybe even high clouds may be significant.
The cloud changes lead to an increase in the cloud albedo, with changes in the solar radiation (ERFaci) at the TOA of −2.62 W m−2. Thanks to a model sensitivity study, the RFaci could be quantified as −2.85 W m−2. Using information from global models, this can be scaled up to the global scale and the present-day vs. pre-industrial timeframe, implying a global ERFaci of −0.8 W m−2.
Although the simulations in this study are limited to 1 d over the domain of Germany, this work shows the great potential of combining these new high-resolution simulations with a large set of observations for the detection and attribution of aerosol–cloud interactions. In the future this work should be complemented by extended analyses for longer time periods and more regions to further improve our understanding of aerosol–cloud interactions.
Table A1List of stations with ICON-LEM meteogram output used in the scope of this study. The following station names are compositions of a standardized four-letter code designating airports and aerodromes (International Civil Aviation Organization – ICAO – codes) and their location: ETGB_Bergen, EDZE_Essen, ETGI_Idar-Oberstein, ETGK_Kummersbruck, Frankfurt_EDDF, Duesseldorf_EDDL, Hamburg_EDDH and Berlin_Tegel_EDDT.
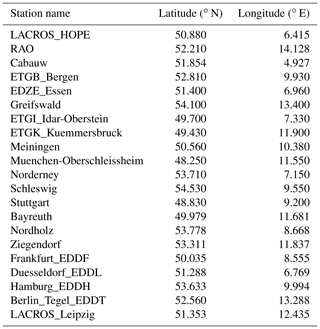
The model output data used for the development of the research in the frame of this scientific article is securely saved in tape archives at the Deutsches Klimarechenzentrum (DKRZ), which will be accessible for 10 years. Additionally, backup copies are stored in the University of Leipzig and University of Cologne backup services. The satellite-based observational data used in the present research are accessible throughout the Standardized Atmospheric Measurement Data (SAMD) web portal hosted by the Integrated Climate Data Center (ICDC) of the University of Hamburg. SAMD is registered at re3data (Registry of Research data Repositories) with the following DOI: https://doi.org/10.17616/R3D944 (re3data.org, 2017). The repository URL is the following: http://icdc.cen.uni-hamburg.de/projekte/samd.html (last access: 16 April 2020). The ground-based observational data are also securely stored either in the University of Cologne backup archives or in the Leibniz Institute for Tropospheric Research in Leipzig.
CCH, PS, UB, SC, CH, IT, AS and JQ conceived the study with input and revisions from all authors. MCS, OS, CA, HB, CCH, CG, JH, CJ, MK, NM, RS, PS and FS performed the analysis with contributions from the other authors. CG, JK, CIM, MB, GC, JFE, KF, KG, RH and PKS updated the ICON model with the necessary revisions for this study, created necessary input, and performed and postprocessed the simulations. MCS, OS and JQ wrote the paper with input from all authors.
The authors declare that they have no conflict of interest.
We thank Christine Knist and Ulrich Görsdorf from the Lindenberg Meteorological Observatory – Richard Assmann Observatory for providing us with data from the microwave radiometers and radar, respectively; Werner Thomas from the Deutscher Wetterdienst – Hohenpeißenberg Meteorological Observatory for providing us with high-resolution ceilometer data; and Björn Stevens from the Max Planck Institute for Meteorology for his advice regarding the “Radiative implications” section. This work is funded by the German Federal Ministry of Education and Research (BMBF) within the framework programme “Research for Sustainable Development (FONA)”, available at https://www.fona.de (last access: 16 April 2020), through the research programme “HD(CP) 2 – High Definition Clouds and Precipitation for Climate Prediction”. The authors gratefully acknowledge the computing time granted by the German Climate computing centre (Deutsches Klimarechenzentrum) and by the Forschungszentrum Jülich through JARA-HPC on the supercomputers JUQUEEN and JURECA. We thank two anonymous reviewers for their constructive comments.
This research has been supported by the German Federal Ministry of Education and Research (grant nos. FKZ 01LK1209C, FKZ 01LK1212C, FKZ 01LK1501E, FKZ 01LK1502I, FKZ 01LK1503A, FKZ 01LK1503E, FKZ 01LK1503F, FKZ 01LK1503G, FKZ 01LK1503H, FKZ 01LK1504A and FKZ 01LK1507A).
This paper was edited by Pedro Jimenez-Guerrero and reviewed by two anonymous referees.
Abdul-Razzak, H. and Ghan, J.: A parametrization of aerosol activation. 2. Multiple aerosol types, J. Geophys. Res., 105, 6837–6844, https://doi.org/10.1029/1999JD901161, 2000. a, b, c
Ackerman, A. S., Kirkpatrick, M. P., Stevens, D. E., and Toon, O. B.: The impact of humidity above stratiform clouds on indirect aerosol climate forcing, Nature, 432, 1014–1017, https://doi.org/10.1038/nature03137, 2004. a, b
Acquistapace, C., Kneifel, S., Löhnert, U., Kollias, P., Maahn, M., and Bauer-Pfundstein, M.: Optimizing observations of drizzle onset with millimeter-wavelength radars, Atmos. Meas. Tech., 10, 1783–1802, https://doi.org/10.5194/amt-10-1783-2017, 2017. a
Acquistapace, C., Löhnert, U., Maahn, M., and Kollias, P.: A New Criterion to Improve Operational Drizzle Detection with Ground-Based Remote Sensing, J. Atmos. Ocean. Tech., 36, 781–801, https://doi.org/10.1175/JTECH-D-18-0158.1, 2019. a, b, c
Albrecht, B.: Aerosols, Cloud Microphysics, and Fractional Cloudiness, Science, 245, 1227–1230, https://doi.org/10.1126/science.245.4923.1227, 1989. a
Ansmann, A., Mamouri, R.-E., Hofer, J., Baars, H., Althausen, D., and Abdullaev, S. F.: Dust mass, cloud condensation nuclei, and ice-nucleating particle profiling with polarization lidar: updated POLIPHON conversion factors from global AERONET analysis, Atmos. Meas. Tech., 12, 4849–4865, https://doi.org/10.5194/amt-12-4849-2019, 2019. a
Baars, H., Kanitz, T., Engelmann, R., Althausen, D., Heese, B., Komppula, M., Preißler, J., Tesche, M., Ansmann, A., Wandinger, U., Lim, J.-H., Ahn, J. Y., Stachlewska, I. S., Amiridis, V., Marinou, E., Seifert, P., Hofer, J., Skupin, A., Schneider, F., Bohlmann, S., Foth, A., Bley, S., Pfüller, A., Giannakaki, E., Lihavainen, H., Viisanen, Y., Hooda, R. K., Pereira, S. N., Bortoli, D., Wagner, F., Mattis, I., Janicka, L., Markowicz, K. M., Achtert, P., Artaxo, P., Pauliquevis, T., Souza, R. A. F., Sharma, V. P., van Zyl, P. G., Beukes, J. P., Sun, J., Rohwer, E. G., Deng, R., Mamouri, R.-E., and Zamorano, F.: An overview of the first decade of PollyNET: an emerging network of automated Raman-polarization lidars for continuous aerosol profiling, Atmos. Chem. Phys., 16, 5111–5137, https://doi.org/10.5194/acp-16-5111-2016, 2016. a
Baldauf, M., Seifert, A., Förstner, J., Majewski, D., Raschendorfer, M., and Reinhardt, T.: Operational Convective-Scale Numerical Weather Prediction with the COSMO Model: Description and Sensitivities, Mon. Weather Rev., 139, 3887–3905, https://doi.org/10.1175/MWR-D-10-05013.1, 2011. a, b
Bentsen, M., Bethke, I., Debernard, J. B., Iversen, T., Kirkevåg, A., Seland, Ø., Drange, H., Roelandt, C., Seierstad, I. A., Hoose, C., and Kristjánsson, J. E.: The Norwegian Earth System Model, NorESM1-M – Part 1: Description and basic evaluation of the physical climate, Geosci. Model Dev., 6, 687–720, https://doi.org/10.5194/gmd-6-687-2013, 2013. a
Bodas-Salcedo, A., Webb, M. J., Bony, S., Chepfer, H., Dufresne, J. L., Klein, S. A., Zhang, Y., Marchand, R., Haynes, J. M., Pincus, R., and John, V. O.: COSP: Satellite simulation software for model assessment, B. Am. Meteorol. Soc., 92, 1023–1043, https://doi.org/10.1175/2011BAMS2856.1, 2011. a
Boucher, O., Randall, D., Artaxo, P., Bretherton, C., Feingold, G., Forster, P., Kerminen, V.-M., Kondo, Y., Liao, H., Lohmann, U., Rasch, P., Satheesh, S. K., Sherwood, S., Stevens, B., and Zhang, X. Y.: Clouds and Aerosols, in: Climate Change 2013: The Physical Science Basis. Contribution of Working Group I to the Fifth Assessment Report of the Intergovernmental Panel on Climate Change, Cambridge University Press, 2013. a
Bühl, J., Seifert, P., Wandinger, U., Baars, H., Kanitz, T., Schmidt, J., Myagkov, A., Engelmann, R., Skupin, A., Heese, B., Klepel, A., Althausen, D., and Ansmann, A.: LACROS: the Leipzig Aerosol and Cloud Remote Observations System, Proc. SPIE 8890, Remote Sensing of Clouds and the Atmosphere XVIII; and Optics in Atmospheric Propagation and Adaptive Systems XVI, 889002, 6, https://doi.org/10.1117/12.2030911, 2013. a
Cherian, R., Quaas, J., Salzmann, M., and Wild, M.: Pollution trends over Europe constrain global aerosol forcing as simulated by climate models, Geophys. Res. Lett., 41, 2176–2181, https://doi.org/10.1002/2013GL058715, 2014. a
Costa-Surós, M., Calbó, J., González, J., and Martin-Vide, J.: Behavior of cloud base height from ceilometer measurements, Atmos. Res., 127, 64–76, https://doi.org/10.1016/j.atmosres.2013.02.005, 2013. a
Dipankar, A., Stevens, B., Heinze, R., Moseley, C., Zängl, G., Giorgetta, M., and Brdar, S.: Large eddy simulations using the general circulation model ICON, J. Adv. Model. Earth Sy., 7, 963–986, https://doi.org/10.1002/2015MS000431, 2015. a
Engelmann, R., Kanitz, T., Baars, H., Heese, B., Althausen, D., Skupin, A., Wandinger, U., Komppula, M., Stachlewska, I. S., Amiridis, V., Marinou, E., Mattis, I., Linné, H., and Ansmann, A.: The automated multiwavelength Raman polarization and water-vapor lidar PollyXT: the neXT generation, Atmos. Meas. Tech., 9, 1767–1784, https://doi.org/10.5194/amt-9-1767-2016, 2016. a
European Monitoring and Evaluation Programme: EMEP, available at: https://www.emep.int/ (last access: 16 April 2020), 2019. a
Eyring, V., Bony, S., Meehl, G. A., Senior, C. A., Stevens, B., Stouffer, R. J., and Taylor, K. E.: Overview of the Coupled Model Intercomparison Project Phase 6 (CMIP6) experimental design and organization, Geosci. Model Dev., 9, 1937–1958, https://doi.org/10.5194/gmd-9-1937-2016, 2016. a
Fan, J., Leung, L. R., Rosenfeld, D., Chen, Q., Li, Z., Zhang, J., and Yan, H.: Microphysical effects determine macrophysical response for aerosol impacts on deep convective clouds, P. Natl. Acad. Sci. USA, 110, E4581–E4590, https://doi.org/10.1073/pnas.1316830110, 2013. a
Feingold, G., Koren, I., Wang, H., Xue, H., and Brewer, W. A.: Precipitation-generated oscillations in open cellular, Nature, 466, 849–852, https://doi.org/10.1038/nature09314, 2010. a
Feingold, G., McComiskey, A., Yamaguchi, T., Johnson, J. S., Carslaw, K. S., and Schmidt, K. S.: New approaches to quantifying aerosol influence on the cloud radiative effect, P. Natl. Acad. Sci. USA, 113, 5812–5819, https://doi.org/10.1073/pnas.1514035112, 2016. a
Genz, C., Schrödner, R., Heinold, B., Henning, S., Baars, H., Spindler, G., and Tegen, I.: Estimation of Cloud Condensation Nuclei number concentrations and comparison to in-situ and lidar observations during the HOPE experiments, Atmos. Chem. Phys. Discuss., https://doi.org/10.5194/acp-2019-742, in review, 2019. a, b, c, d, e
Giorgetta, M. A., Brokopf, R., Crueger, T., Esch, M., Fiedler, S., Helmert, J., Hohenegger, C., Kornblueh, L., Köhler, M., Manzini, E., Mauritsen, T., Nam, C., Raddatz, T., Rast, S., Reinert, D., Sakradzija, M., Schmidt, H., Schneck, R., Schnur, R., Silvers, L., Wan, H., Zängl, G., and Stevens, B.: ICON-A, the Atmosphere Component of the ICON Earth System Model: I. Model Description, J. Adv. Model. Earth Sy., 10, 1638–1662, https://doi.org/10.1029/2017MS001242, 2018. a
Gordon, H., Field, P. R., Abel, S. J., Dalvi, M., Grosvenor, D. P., Hill, A. A., Johnson, B. T., Miltenberger, A. K., Yoshioka, M., and Carslaw, K. S.: Large simulated radiative effects of smoke in the south-east Atlantic, Atmos. Chem. Phys., 18, 15261–15289, https://doi.org/10.5194/acp-18-15261-2018, 2018. a
Grosvenor, D. P., Sourdeval, O., Zuidema, P., Ackerman, A., Alexandrov, M. D., Bennartz, R., Boers, R., Cairns, B., Chiu, C., Christensen, M., Deneke, H., Diamond, M., Feingold, G., Fridlind, A., Hünerbein, A., Knist, C., Kollias, P., Marshak, A., McCoy, D., Merk, D., Painemal, D., Rausch, J., Rosenfeld, D., Russchenberg, H., Seifert, P., Sinclair, K., Stier, P., B. van D., Wendisch, M., Werner, F., Wood, R., Zhang, Z., and Quaas, J.: Remote sensing of cloud droplet number concentration in warm clouds: A review of the current state of knowledge and perspectives, Rev. Geophys., 56, 409–453, https://doi.org/10.1029/2017RG000593, 2018. a, b, c
Gryspeerdt, E., Goren, T., Sourdeval, O., Quaas, J., Mülmenstädt, J., Dipu, S., Unglaub, C., Gettelman, A., and Christensen, M.: Constraining the aerosol influence on cloud liquid water path, Atmos. Chem. Phys., 19, 5331–5347, https://doi.org/10.5194/acp-19-5331-2019, 2019. a, b, c
Hande, L. B., Engler, C., Hoose, C., and Tegen, I.: Parameterizing cloud condensation nuclei concentrations during HOPE, Atmos. Chem. Phys., 16, 12059–12079, https://doi.org/10.5194/acp-16-12059-2016, 2016. a, b
Heinold, B., Tegen, I., Bauer, S., and Wendisch, M.: Regional modelling of Saharan dust and biomass-burning smoke. Part 2: Direct radiative forcing and atmospheric dynamic response, Tellus B, 63, 800–813, https://doi.org/10.1111/j.1600-0889.2011.00574.x, 2011a. a
Heinold, B., Tegen, I., Schepanski, K., Tesche, M., Esselborn, M., Freudenthaler, V., Gross, S., Kandler, K., Knippertz, P., Müller, D., Schladitz, A., Toledano, C., Weinzierl, B., Ansmann, A., Althausen, D., Müller, T., Petzold, A., and Wiedensohler, A.: Regional modelling of Saharan dust and biomass-burning smoke. Part 1: Model description and evaluation, Tellus B, 63, 781–799, https://doi.org/10.1111/j.1600-0889.2011.00570.x, 2011b. a
Heinze, R., Dipankar, A., Henken, C. C., Moseley, C., Sourdeval, O., Xie, X., Adamidis, P., Ament, F., Barthlott, C., Behrendt, A., Blahak, U., Bley, S., Brdar, S., Brueck, M., Crewell, S., Deneke, H., Girolamo, P. D., Evaristo, R., Frank, C., Gorges, K., Hanke, M., Hansen, A., Hoose, C., Jahns, T., Kalthoff, N., Klocke, D., Kneifel, S., Knippertz, P., Kuhn, A., Laar, T. V., Macke, A., Maurer, V., Meyer, C., Muppa, S., Neggers, R., Orlandi, E., Pantillon, F., Pospichal, B., Scheck, L., Seifert, A., Senf, F., Siligam, P., Simmer, C., Steinke, S., Stevens, B., Wapler, K., Weniger, M., Wulfmeyer, V., Zhang, D., and Quaas, J.: Large-eddy simulations over Germany using ICON: A comprehensive evaluation, Q. J. Roy. Meteor. Soc., 143, 69–100, https://doi.org/10.1002/qj.2947, 2017. a, b, c, d, e, f, g
Held, I. M., Guo, H., Adcroft, A., Dunne, J. P., Horowitz, L. W., Krasting, J., Shevliakova, E., Winton, M., Zhao, M., Bushuk, M., Wittenberg, A. T., Wyman, B., Xiang, B., Zhang, R., Anderson, W., Balaji, V., Donner, L., Dunne, K., Durachta, J., Gauthier, P. P. G., Ginoux, P., Golaz, J.-C., Griffies, S. M., Hallberg, R., Harris, L., Harrison, M., Hurlin, W., John, J., Lin, P., Lin, S.-J., Malyshev, S., Menzel, R., Milly, P. C. D., Ming, Y., Naik, V., Paynter, D., Paulot, F., Rammaswamy, V., Reichl, B., Robinson, T., Rosati, A., Seman, C., Silvers, L. G., Underwood, S., and Zadeh, N.: Structure and Performance of GFDL's CM4.0 Climate Model, J. Adv. Model. Earth Sy., 11, 3691–3727, https://doi.org/10.1029/2019MS001829, 2019. a
Heyn, I., Block, K., Mülmenstädt, J., Gryspeerdt, E., Kühne, P., Salzmann, M., and Quaas, J.: Assessment of simulated aerosol effective radiative forcings in the terrestrial spectrum, Geophys. Res. Lett., 44, 1001–1007, https://doi.org/10.1002/2016GL071975, 2017. a
Illingworth, A., Hogan, R., O'Connor, E., Bouniol, D., Brooks, M., Delanoé, J., Donovan, D., Eastment, J., Gaussiat, N., Goddard, J., Haeffelin, M., Baltink, H., Krasnov, O., Pelon, J., Piriou, J., Protat, A., Russchenberg, H., Seifert, A., Tompkins, A., G. van Z., Vinit, F., Willén, U., Wilson, D., and Wrench, C.: Cloudnet. Continuous Evaluation of Cloud Profiles in Seven Operational Models Using Ground-Based Observations, B. Am. Meteorol. Soc., 88, 883–898, https://doi.org/10.1175/BAMS-88-6-883, 2007. a, b
Jimenez, C., Ansmann, A., Donovan, D., Engelmann, R., Malinka, A., Schmidt, J., and Wandinger, U.: Retrieval of microphysical properties of liquid water clouds from atmospheric lidar measurements: Comparison of the Raman dual field of view and the depolarization techniques, Proc. SPIE, 10429, 1042907, https://doi.org/10.1117/12.2281806, 2017. a, b
Jimenez, C., Ansmann, A., Engelmann, R., Haarig, M., Schmidt, J., and Wandinger, U.: Polarization lidar: an extended three-signal calibration approach, Atmos. Meas. Tech., 12, 1077–1093, https://doi.org/10.5194/amt-12-1077-2019, 2019. a
Jimenez, C., Ansmann, A., Engelmann, R., Malinka, A., Schmidt, J., and Wandinger, U.: Retrieval of microphysical properties of liquid water clouds from atmospheric lidar measurements: Dual field of view depolarization technique, Atmos. Meas. Tech., in preparation, 2020. a, b
Khain, A., Rosenfeld, D., and Pokrovsky, A.: Aerosol impact on the dynamics and microphysics of deep convective clouds, Q. J. Roy. Meteor. Soc., 131, 2639–2663, https://doi.org/10.1256/qj.04.62, 2005. a
Kirkevåg, A., Grini, A., Olivié, D., Seland, Ø., Alterskjær, K., Hummel, M., Karset, I. H. H., Lewinschal, A., Liu, X., Makkonen, R., Bethke, I., Griesfeller, J., Schulz, M., and Iversen, T.: A production-tagged aerosol module for Earth system models, OsloAero5.3 – extensions and updates for CAM5.3-Oslo, Geosci. Model Dev., 11, 3945–3982, https://doi.org/10.5194/gmd-11-3945-2018, 2018. a
Knoth, O. and Wolke, R.: An explicit-implicit numerical approach for atmospheric chemistry-transport modeling, Atmos. Environ., 32, 1785–1797, https://doi.org/10.1016/S1352-2310(97)00476-7, 1998. a
Löhnert, U., Schween, J. H., Acquistapace, C., Ebell, K., Maahn, M., Barrera-Verdejo, M., Hirsikko, A., Bohn, B., Knaps, A., O'Connor, E., Simmer, C., Wahner, A., and Crewell, S.: JOYCE: Jülich Observatory for Cloud Evolution, B. Am. Meteorol. Soc., 96, 1157–1174, https://doi.org/10.1175/BAMS-D-14-00105.1, 2015. a
Maahn, M.: Exploiting vertically pointing Doppler radar for advancing snow and ice cloud observations, PhD thesis, University of Cologne, available at: http://kups.ub.uni-koeln.de/6002/ (last access: 16 April 2020), 2015. a
Maahn, M., Löhnert, U., Kollias, P., Jackson, R. C., and McFarquhar, G. M.: Developing and Evaluating Ice Cloud Parameterizations for Forward Modeling of Radar Moments Using in situ Aircraft Observations, J. Atmos. Ocean. Tech., 32, 880–903, https://doi.org/10.1175/JTECH-D-14-00112.1, 2015. a
Macke, A., Seifert, P., Baars, H., Barthlott, C., Beekmans, C., Behrendt, A., Bohn, B., Brueck, M., Bühl, J., Crewell, S., Damian, T., Deneke, H., Düsing, S., Foth, A., Di Girolamo, P., Hammann, E., Heinze, R., Hirsikko, A., Kalisch, J., Kalthoff, N., Kinne, S., Kohler, M., Löhnert, U., Madhavan, B. L., Maurer, V., Muppa, S. K., Schween, J., Serikov, I., Siebert, H., Simmer, C., Späth, F., Steinke, S., Träumner, K., Trömel, S., Wehner, B., Wieser, A., Wulfmeyer, V., and Xie, X.: The HD(CP)2 Observational Prototype Experiment (HOPE) – an overview, Atmos. Chem. Phys., 17, 4887–4914, https://doi.org/10.5194/acp-17-4887-2017, 2017. a, b, c
Malavelle, F. F., Haywood, J. M., Jones, A., Gettelman, A., Clarisse, L., Bauduin, S., Allan, R. P., Karset, I. H. H., Kristjánsson, J. E., Oreopoulos, L., Cho, N., Lee, D., Bellouin, N., Boucher, O., Grosvenor, D. P., Carslaw, K. S., Dhomse, S., Mann, G. W., Schmidt, A., Coe, H., Hartley, M. E., Dalvi, M., Hill, A. A., Johnson, B. T., Johnson, C. E., Knight, J. R., O'Connor, F. M., Partridge, D. G., Stier, P., Myhre, G., Platnick, S., Stephens, G. L., Takahashi, H., and Thordarson, T.: Strong constraints on aerosol-cloud interactions from volcanic eruptions, Nature, 546, 485–491, https://doi.org/10.1038/nature22974, 2017. a
Mamouri, R.-E. and Ansmann, A.: Potential of polarization lidar to provide profiles of CCN- and INP-relevant aerosol parameters, Atmos. Chem. Phys., 16, 5905–5931, https://doi.org/10.5194/acp-16-5905-2016, 2016. a, b, c
Martucci, G., Milroy, C., and O'Dowd, C. D.: Detection of Cloud-Base Height Using Jenoptik CHM15K and Vaisala CL31 Ceilometers, J. Atmos. Ocean. Tech., 27, 305–318, https://doi.org/10.1175/2009JTECHA1326.1, 2010. a
Meier, J., Tegen, I., Mattis, I., Wolke, R., Alados Arboledas, L., Apituley, A., Balis, D., Barnaba, F., Chaikovsky, A., Sicard, M., Pappalardo, G., Pietruczuk, A., Stoyanov, D., Ravetta, F., and Rizi, V.: A regional model of European aerosol transport: Evaluation with sun photometer, lidar and air quality data, Atmos. Environ., 47, 519–532, https://doi.org/10.1016/j.atmosenv.2011.09.029, 2012. a
Miltenberger, A. K., Field, P. R., Hill, A. A., Rosenberg, P., Shipway, B. J., Wilkinson, J. M., Scovell, R., and Blyth, A. M.: Aerosol–cloud interactions in mixed-phase convective clouds – Part 1: Aerosol perturbations, Atmos. Chem. Phys., 18, 3119–3145, https://doi.org/10.5194/acp-18-3119-2018, 2018. a
Mülmenstädt, J. and Feingold, G.: The Radiative Forcing of Aerosol–Cloud Interactions in Liquid Clouds: Wrestling and Embracing Uncertainty, Curr. Clim. Chang. Reports, 4, 23–40, https://doi.org/10.1007/s40641-018-0089-y, 2018. a, b, c
Pincus, R., Forster, P. M., and Stevens, B.: The Radiative Forcing Model Intercomparison Project (RFMIP): experimental protocol for CMIP6, Geosci. Model Dev., 9, 3447–3460, https://doi.org/10.5194/gmd-9-3447-2016, 2016. a, b
Platnick, S., King, M. D., Meyer, K. G., Wind, G., Amarasinghe, N., Marchant, B., Arnold, G. T., Zhang, Z., Hubanks, P. A., Ridgway, B., and Riédi, J.: MODIS Cloud Optical Properties: User Guide for the Collection 6 Level-2 MOD06/MYD06 Product and Associated Level-3 Datasets, Version 1.0, available at: https://modis-images.gsfc.nasa.gov/_docs/C6MOD06OPUserGuide.pdf (last access: 31 May 2016), 2015. a
Platnick, S., Meyer, K. G., King, M. D., Wind, G., Amarasinghe, N., Marchant, B., Arnold, G. T., Zhang, Z., Hubanks, P. A., Holz, R. E., Yang, P., Ridgway, W. L., and Riedi, J.: The MODIS Cloud Optical and Microphysical Products: Collection 6 Updates and Examples From Terra and Aqua, IEEE T. Geosci. Remote, 55, 502–525, https://doi.org/10.1109/TGRS.2016.2610522, 2017. a
Quaas, J.: Approaches to Observe Anthropogenic Aerosol-Cloud Interactions, Curr. Clim. Chang. Reports, 1, 297–304, https://doi.org/10.1007/s40641-015-0028-0, 2015. a
Quaas, J., Boucher, O., and Lohmann, U.: Constraining the total aerosol indirect effect in the LMDZ and ECHAM4 GCMs using MODIS satellite data, Atmos. Chem. Phys., 6, 947–955, https://doi.org/10.5194/acp-6-947-2006, 2006. a
Quaas, J., Ming, Y., Menon, S., Takemura, T., Wang, M., Penner, J. E., Gettelman, A., Lohmann, U., Bellouin, N., Boucher, O., Sayer, A. M., Thomas, G. E., McComiskey, A., Feingold, G., Hoose, C., Kristjánsson, J. E., Liu, X., Balkanski, Y., Donner, L. J., Ginoux, P. A., Stier, P., Grandey, B., Feichter, J., Sednev, I., Bauer, S. E., Koch, D., Grainger, R. G., Kirkevåg, A., Iversen, T., Seland, Ø., Easter, R., Ghan, S. J., Rasch, P. J., Morrison, H., Lamarque, J.-F., Iacono, M. J., Kinne, S., and Schulz, M.: Aerosol indirect effects – general circulation model intercomparison and evaluation with satellite data, Atmos. Chem. Phys., 9, 8697–8717, https://doi.org/10.5194/acp-9-8697-2009, 2009. a
re3data.org: SAMD; editing status 2017-10-17; re3data.org – Registry of Research Data Repositories, https://doi.org/10.17616/R3D944, 2017. a
Rémillard, J., Fridlind, A., Ackerman, A., Tselioudis, G., Kollias, P., Mechem, D., Chandler, H., Luke, E., Wood, R., Witte, M., and Ayers, J.: Use of cloud radar Doppler spectra to evaluate stratocumulus drizzle size distributions in large-eddy simulations with size-resolved microphysics, J. Appl. Meteorol. Clim., 56, 3263–3283, https://doi.org/10.1175/JAMC-D-17-0100.1, 2017. a
Rossow, W. and Schiffer, R.: ISCCP Cloud Data Products, B. Am. Meteorol. Soc., 72, 2–20, https://doi.org/10.1175/1520-0477(1991)072<0002:ICDP>2.0.CO;2, 1991. a
Sandu, I., Brenguier, J.-L., Geoffroy, O., Thouron, O., and Masson, V.: Aerosol Impacts on the Diurnal Cycle of Marine Stratocumulus, J. Atmos. Sci., 65, 2705–2718, https://doi.org/10.1175/2008jas2451.1, 2008. a
Schättler, U., Doms, G., and Schraff, C.: A Description of the Nonhydrostatic Regional COSMO-Model. Part VII: User's Guide, Deutscher Wetterdienst, Offenbach, 2014. a
Schmidt, J., Wandinger, U., and Malinka, A.: Dual-field-of-view Raman lidar measurements for the retrieval of cloud microphysical properties, Appl. Optics, 52, 2235–2247, https://doi.org/10.1364/AO.52.002235, 2013. a
Schmidt, J., Ansmann, A., Bühl, J., Baars, H., Wandinger, U., Müller, D., and Malinka, A. V.: Dual‐FOV Raman and Doppler lidar studies of aerosol‐cloud interactions: Simultaneous profiling of aerosols, warm‐cloud properties, and vertical wind, J. Geophys. Res.-Atmos., 119, 5512–5527, https://doi.org/10.1002/2013JD020424, 2014. a
Seifert, A. and Beheng, K. D.: A two-moment cloud microphysics parameterization for mixed-phase clouds. Part 1: Model description, Meteorol. Atmos. Phys., 92, 45–66, https://doi.org/10.1007/s00703-005-0112-4, 2006. a
Seifert, A., Heus, T., Pincus, R., and Stevens, B.: Large-eddy simulation of the transient and near-equilibrium behavior of precipitating shallow convection, J. Adv. Model. Earth Sy., 7, 1918–1937, https://doi.org/10.1002/2015MS000489, 2015. a
Seinfeld, J. H., Bretherton, C., Carslaw, K. S., Coe, H., DeMott, P. J., Dunlea, E. J., Feingold, G., Ghan, S., Guenther, A. B., Kahn, R., Kraucunas, I., Kreidenweis, S. M., Molina, M. J., Nenes, A., Penner, J. E., Prather, K. A., Ramanathan, V., Ramaswamy, V., Rasch, P. J., Ravishankara, A. R., Rosenfeld, D., Stephens, G., and Wood, R.: Improving our fundamental understanding of the role of aerosol-cloud interactions in the climate system, P. Natl. Acad. Sci. USA, 113, 5781–5790, https://doi.org/10.1073/pnas.1514043113, 2016. a
Small, J. D., Chuang, P. Y., Feingold, G., and Jiang, H.: Can aerosol decrease cloud lifetime?, Geophys. Res. Lett., 36, 1–5, https://doi.org/10.1029/2009GL038888, 2009. a
Smith, S. J., van Aardenne, J., Klimont, Z., Andres, R. J., Volke, A., and Delgado Arias, S.: Anthropogenic sulfur dioxide emissions: 1850–2005, Atmos. Chem. Phys., 11, 1101–1116, https://doi.org/10.5194/acp-11-1101-2011, 2011. a, b, c
Stevens, B. and Feingold, G.: Untangling aerosol effects on clouds and precipitation in a buffered system., Nature, 461, 607–613, https://doi.org/10.1038/nature08281, 2009. a, b, c
Swales, D. J., Pincus, R., and Bodas-Salcedo, A.: The Cloud Feedback Model Intercomparison Project Observational Simulator Package: Version 2, Geosci. Model Dev., 11, 77–81, https://doi.org/10.5194/gmd-11-77-2018, 2018. a
Swart, N. C., Cole, J. N. S., Kharin, V. V., Lazare, M., Scinocca, J. F., Gillett, N. P., Anstey, J., Arora, V., Christian, J. R., Hanna, S., Jiao, Y., Lee, W. G., Majaess, F., Saenko, O. A., Seiler, C., Seinen, C., Shao, A., Sigmond, M., Solheim, L., von Salzen, K., Yang, D., and Winter, B.: The Canadian Earth System Model version 5 (CanESM5.0.3), Geosci. Model Dev., 12, 4823–4873, https://doi.org/10.5194/gmd-12-4823-2019, 2019. a
Tatebe, H., Ogura, T., Nitta, T., Komuro, Y., Ogochi, K., Takemura, T., Sudo, K., Sekiguchi, M., Abe, M., Saito, F., Chikira, M., Watanabe, S., Mori, M., Hirota, N., Kawatani, Y., Mochizuki, T., Yoshimura, K., Takata, K., O'ishi, R., Yamazaki, D., Suzuki, T., Kurogi, M., Kataoka, T., Watanabe, M., and Kimoto, M.: Description and basic evaluation of simulated mean state, internal variability, and climate sensitivity in MIROC6, Geosci. Model Dev., 12, 2727–2765, https://doi.org/10.5194/gmd-12-2727-2019, 2019. a
Toll, V., Christensen, M., Gassó, S., and Bellouin, N.: Volcano and Ship Tracks Indicate Excessive Aerosol-Induced Cloud Water Increases in a Climate Model, Geophys. Res. Lett., 44, 12492–12500, https://doi.org/10.1002/2017GL075280, 2017. a
Toll, V., Christensen, M., Quaas, J., and Bellouin, N.: Weak average liquid-cloud-water response to anthropogenic aerosols, Nature, 572, 51–55, https://doi.org/10.1038/s41586-019-1423-9, 2019. a, b
Twomey, S.: Pollution and the Planetary Albedo, Atmos. Environ., 8, 1251–1256, https://doi.org/10.1016/0004-6981(74)90004-3, 1974. a, b
van den Heever, S. C., Stephens, G. L., and Wood, N. B.: Aerosol Indirect Effects on Tropical Convection Characteristics under Conditions of Radiative–Convective Equilibrium, J. Atmos. Sci., 68, 699–718, https://doi.org/10.1175/2010jas3603.1, 2010. a
Wiegner, M. and Geiß, A.: Aerosol profiling with the Jenoptik ceilometer CHM15kx, Atmos. Meas. Tech., 5, 1953–1964, https://doi.org/10.5194/amt-5-1953-2012, 2012. a
Wiegner, M., Madonna, F., Binietoglou, I., Forkel, R., Gasteiger, J., Geiß, A., Pappalardo, G., Schäfer, K., and Thomas, W.: What is the benefit of ceilometers for aerosol remote sensing? An answer from EARLINET, Atmos. Meas. Tech., 7, 1979–1997, https://doi.org/10.5194/amt-7-1979-2014, 2014. a
Wolke, R., Knoth, O., Hellmuth, O., Schröder, W., and Renner, E.: The parallel model system LM-MUSCAT for chemistry-transport simulations: Coupling scheme, parallelization and applications, Adv. Parallel Comput., 13, 363–369, https://doi.org/10.1016/S0927-5452(04)80048-0, 2004. a
Wolke, R., Schröder, W., Schrödner, R., and Renner, E.: Influence of grid resolution and meteorological forcing on simulated European air quality: A sensitivity study with the modeling system COSMO-MUSCAT, Atmos. Environ., 53, 110–130, https://doi.org/10.1016/j.atmosenv.2012.02.085, 2012. a, b
Xue, H. and Feingold, G.: Large-Eddy Simulations of Trade Wind Cumuli: Investigation of Aerosol Indirect Effects, J. Atmos. Sci., 63, 1605–1622, https://doi.org/10.1175/JAS3706.1, 2006. a
Zängl, G., Reinert, D., Rípodas, P., and Baldauf, M.: The ICON (ICOsahedral Non-hydrostatic) modelling framework of DWD and MPI-M: Description of the non-hydrostatic dynamical core, Q. J. Roy. Meteor. Soc., 141, 563–579, https://doi.org/10.1002/qj.2378, 2015. a